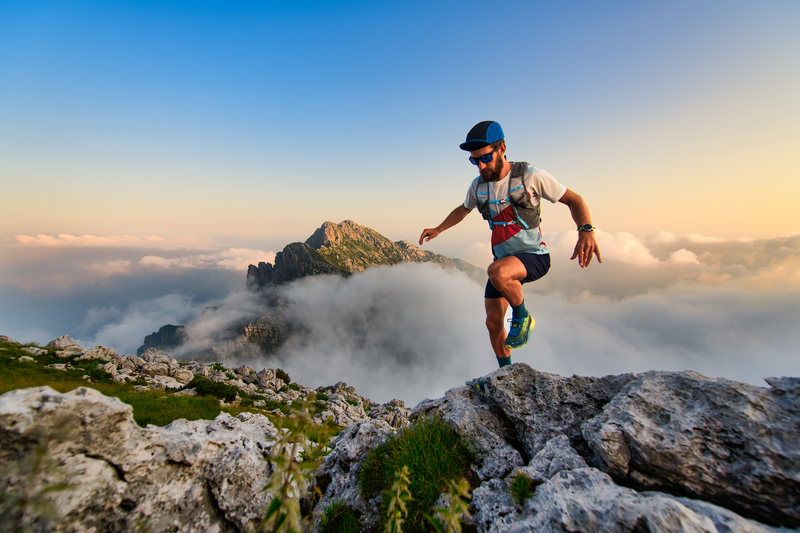
94% of researchers rate our articles as excellent or good
Learn more about the work of our research integrity team to safeguard the quality of each article we publish.
Find out more
ORIGINAL RESEARCH article
Front. Plant Sci. , 23 June 2023
Sec. Plant Abiotic Stress
Volume 14 - 2023 | https://doi.org/10.3389/fpls.2023.1212559
This article is part of the Research Topic Harnessing Crop Biodiversity and Genomics Assisted Pre-Breeding Approaches for Next Generation Climate-Smart Varieties, Volume II View all 7 articles
Introduction: Over four billion people around the world rely on bread wheat (Triticum aestivum L.) as a major constituent of their diet. The changing climate, however, threatens the food security of these people, with periods of intense drought stress already causing widespread wheat yield losses. Much of the research into the wheat drought response has centred on the response to drought events later in development, during anthesis or grain filling. But as the timing of periods of drought stress become increasingly unpredictable, a more complete understanding of the response to drought during early development is also needed.
Methods: Here, we utilized the YoGI landrace panel to identify 10,199 genes which were differentially expressed under early drought stress, before weighted gene co-expression network analysis (WGCNA) was used to construct a co-expression network and identify hub genes in modules particularly associated with the early drought response.
Results: Of these hub genes, two stood out as novel candidate master regulators of the early drought response – one as an activator (TaDHN4-D1; TraesCS5D02G379200) and the other as a repressor (uncharacterised gene; TraesCS3D02G361500).
Discussion: As well as appearing to coordinate the transcriptional early drought response, we propose that these hub genes may be able to regulate the physiological early drought response due to potential control over the expression of members of gene families well-known for their involvement in the drought response in many plant species, namely dehydrins and aquaporins, as well as other genes seemingly involved in key processes such as, stomatal opening, stomatal closing, stomatal morphogenesis and stress hormone signalling.
Triticum aestivum L. (bread wheat) is relied upon by billions of people as a primary source of both calories and protein (Pfeifer et al., 2014; Food and Agriculture Organization of the United Nations et al., 2018). As the global population continues to grow, the number of livelihoods that will be dependent on the success of wheat crop yields is staggering. To meet this demand, therefore, the yields of key crops like wheat need to increase by at least 50% in the coming decades (Godfray et al., 2010; Tilman et al., 2011; Ray et al., 2013). The changing climate poses a major threat to this necessary yield increase, however, with rising global temperatures leading to the depletion of water supplies and periods of intense drought stress (Hansen et al., 2006). Drier growth conditions paired with reduced water supply is of particular concern for the agricultural sector, as it accounts for between 80 and 90% of all freshwater usage, with cereal crop cultivation alone accounting for 27% (Hoekstra and Mekonnen, 2012; Ray et al., 2013; Dunn et al., 2019). In the coming decades, climate change will cause changes in precipitation patterns that may affect wheat-growing regions especially severely, with recent work finding that up to 60% of the current global wheat-growing area may face severe water scarcity by the end of the century, compared to only 15% currently (Trnka et al., 2019). As well as the threat that future drought events pose to wheat crops, drought stress has been causing significant damage around the world for the last few decades, with 161Mha of wheat harvested areas experiencing yield loss through drought between 1983 and 2009, equating to an economic loss of $47 billion (Iizumi et al., 2018; Kim et al., 2019). Therefore, the cultivation of drought tolerant wheat varieties is of paramount importance, if global wheat crops are to be protected against the effects of water shortage in a climate where water supplies are becoming increasingly scarce.
In the present work we examined the effect of drought stress exposure during early development on gene expression in spring habit wheat landrace accessions. With spring wheat often being sown during March in the Northern hemisphere, the present work mimics drought stress events that occur during April once plants have germinated and established in fields. Although much of the work concerning the effect of drought stress on wheat growth studies the perturbation’s effect on yield (Aprile et al., 2009; Zhang et al., 2018; Kim et al., 2019; Qaseem et al., 2019; Senapati et al., 2019; Abou-Elwafa and Shehzad, 2021; Lan et al., 2022; Wan et al., 2022), periods of water shortage are becoming increasingly common during the early stages of spring wheat growth, all around the world. April 2022, for example, was an incredibly dry month for many of the world’s largest wheat-producing countries, with almost 50% of the United States experiencing moderate to exceptional drought (NOAA National Centers for Environmental Information, 2022b), whilst large parts of Europe (including major spring wheat-producing nations such as the United Kingdom) experienced a drier month than normal (NOAA National Centers for Environmental Information, 2022a). The pressing nature of this threat to wheat crops is perhaps reflected in the increasing amount of research into the effect of drought stress on the early growth of wheat, over the last few years (Guo et al., 2017; Sallam et al., 2018; Ahmed et al., 2020; Ahmed et al., 2022; Mahpara et al., 2022; Nardino et al., 2022; Sharma et al., 2022). These works largely identify tolerant cultivars for use in breeding programs, but do not aim to understand the genetic control of the drought response at this stage of development – something that is relatively understudied, despite its importance (Ajigboye et al., 2017; Mao et al., 2020; Vuković et al., 2022). The need, therefore, to better understand the genetic control of the early drought response in order to aid the production of drought tolerant wheat varieties is already present, and likely to become more pressing as temperatures increase and precipitation patterns change over the coming decades.
Due to the sheer number of genes involved in complex processes, such as the drought response, identifying those which play the most pivotal roles can be difficult. The use of weighted gene co-expression network analysis (WGCNA), however, identifies groups of genes which are co-expressed across samples, from which we can identify candidate master-regulators of these groups of genes (Langfelder and Horvath, 2008; Langfelder and Horvath, 2012). Such master-regulators of drought-responsive genes, therefore, are likely to be those which play key roles in the drought response. The approach has been utilized successfully to identify “hub genes” in wheat encoding proteins such as transcription factors, heat shock proteins (HSPs) and regulators of stress hormone signaling (Lv et al., 2020; Du et al., 2022), which act to determine a plant’s degree of drought tolerance via their regulation of other drought-responsive genes. The present work employs a similar approach, but is distinct from these works due to its use of wheat landraces: genetically and phenotypically diverse cultivars selected by local farmers to grow successfully in a vast array of climates around the world (Zeven, 1998). We have previously exemplified the genetic diversity of the YoGI landrace panel, before utilizing it to identify candidate master-regulators, and genetic markers, of basal early thermotolerance (Barratt et al., 2023), but the present work represents a novel study into the use of gene expression data from wheat landraces under drought stress, to identify candidate master-regulators of the transcriptional early drought response.
14 spring habit accessions with a range of drought tolerance levels were used in the present work (Supplementary Data S1). Genomic tile plots visualising the A, B, and D genomes for each accession in the YoGI landrace panel (Barratt et al., 2023) were used to exclude accessions with significant genomic dominance or putative rearrangement, and to ensure all accessions used were hexaploid. Seeds were sown in Levington Advance Seed & Modular F2S compost mixed with Aggregate Industries Garside Sands 16/30 sand (80:20 ratio), treated with CaLypso insecticide (Bayer CropScience Ltd., 0.083ml mixed with 100ml water, applied to each liter of compost) and grown under long day (16/8h, 20°C/14°C) glasshouse conditions.
Four replicates of each accession per group were watered normally (twice-daily watering, average soil moisture content (SMC) = 36.6%), until plants in the drought group reached Zadoks’ growth scale 13 (GS13; Zadoks et al., 1974) whereby stress was applied by withholding water for a ten-day period. Normal watering then resumed for three-days to serve as a recovery period. Four replicates of each accession were grown at the same time, but not exposed to drought stress. All above-ground tissue from plants was harvested 13 days after GS13, before biomass was dried for two days at 70°C and weighed on a scale.
6cm of leaf tissue was collected from wheat seedlings upon reaching GS13 and at the end of the drought period. Tissue was collected individually for each sample, and immediately immersed in liquid nitrogen to prevent nucleic acid degradation. Tissue samples were stored at -80°C for later processing. At each sampling stage, as well as after drought recovery (13 days after GS13), SMC% was recorded using an ML3 Thetaprobe Soil Moisture Sensor with an HH2 Moisture Meter (Delta-T Devices, Cambridge, United Kingdom) to quantify the severity of the drought stress treatment. The probe was inserted into the soil to its full depth before moisture levels were recorded. Mean SMC% of conditions, at each time point, were compared via two-sample t-test.
Total RNA was extracted from ~100 mg of individual leaf tissue samples using the E.Z.N.A Plant RNA Kit (Omega Bio-Tek, GA, USA) including a DNase treatment, according to the manufacturer’s protocol. RNA concentration was quantified using a Qubit 4 Fluorometer (Life Technologies, CA, USA), while RNA quality was assessed via both NanoDrop ND-1000 Spectrophotometer (Thermo-Fisher Scientific, MA, USA) and an Agilent Technology 2100 Bioanalyzer (Agilent Technologies, CA, USA). Samples with RNA Integrity Number (RIN) values greater than seven were deemed acceptable for use in subsequent analysis. Replicates were pooled into one sample per accession, per treatment, at equimolar proportions. Samples were stored at -80°C and shipped on dry ice to Novogene (Cambridge, United Kingdom) for sequencing, using the Illumina Novaseq 6000 platform (Illumina, CA, USA) with a 150bp paired-end strategy. Our experimental design included both technical and biological replication. Prior to sequencing, we pooled RNA from 4 replicate plants per accession, per condition (pre- or post-drought) to help control the effect of the environment on the transcriptome, whilst the different accessions provided biological replication for each treatment.
After sequencing, quality control was carried out using FastQC (www.bioinformatics.babraham.ac.uk/projects/fastqc/). Raw reads were then filtered by trimming low quality sequences (average Phred score < 15), trimming short length reads (< 36bp), and clipping Illumina adapters using Trimmomatic v0.39 (Bolger et al., 2014).
Salmon (Patro et al., 2017) was used to map reads to the IWGSC Triticum aestivum v1.0 reference assembly (GCA Accession: GCA_900519105.1) and the updated IWGSC Triticum aestivum v1.1 gene model annotation. Reference genome and gene model annotation files used can be found on the International Wheat Genome Sequencing Consortium (IWGSC) website (https://www.wheatgenome.org). Salmon’s mapping-based mode was used to create an index from the reference genome, and then for quantification of the trimmed reads. Salmon output files were prepared for differential expression analysis using the R (version 4.1.2.; R R Core Team, 2021) package TxImport (version 1.24; Soneson et al., 2015), generating a table containing transcript abundance (TPM), counts, and length from the Salmon quantification files.
Transcriptome data were initially explored using Principal Components Analysis (PCA) function of DESeq2 (version; 1.36.0; Love et al., 2014). Differential expression analysis was performed on the raw count data using the R package DESeq2. Genes with < 10 reads were filtered out before running DESeq2. An additive model was used to identify differentially expressed genes (DEGs) between pre- and post-drought samples. Expression fold changes were shrunk using the R package “Ashr” (version; 2.2-54; Stephens, 2017) to account for variability in lowly expressed genes while preserving large fold changes.
Only genes with a log2FoldChange greater/less than 1.5/-1.5 and an FDR-adjusted (Benjamini and Hochberg, 1995) p-value < 0.05 were considered significantly differentially expressed and carried forward for GO enrichment analysis. Differential expression contrasts were visualised via volcano plots, made using the “ggplot2” package (version 3.4.0; Wickham, 2009) in R.
To identify gene ontology (GO) terms significantly enriched amongst upregulated and downregulated DEGs, identified via DESeq2, GO enrichment analysis was conducted. Because GO terms were only present for the IWGSC RefSeqv1.0 genome annotation, we adopted an approach used previously (Borrill et al., 2019; Andleeb et al., 2023), whereby GO terms are transferred from the v1.0 annotation to the v1.1 annotation. This approach transfers the GO terms only from genes which were >99% identical across >90% of the sequence. The list of these genes can be found in Andleeb et al. (2023). IWGSC v1.0 GO terms were retrieved from: https://opendata.earlham.ac.uk/wheat/under_license/toronto/Ramirez-Gonzalez_etal_2018-06025-Transcriptome-Landscape/data/TablesForExploration/FunctionalAnnotation.rds. This RDS file was read in to R using the readRDS() function (in base R), prior to analysis.
GO terms associated with upregulated and downregulated DEGs were collated into two groups and submitted to the agriGO Singular Enrichment Analysis tool (Du et al., 2010; Tian et al., 2017). A Fisher’s exact test was performed for each DEG group with the GO terms of all genes obtained after count filtering by DESeq2 serving as background; 0.05 as the p-value threshold; Hochberg (FDR) as the multi-test adjustment method (Benjamini and Hochberg, 1995), and 5 as the minimum number of mapping entries threshold. A GO term was considered enriched when its FDR-adjusted p-value was < 0.05. GO terms that were significantly enriched amongst upregulated and downregulated genes, compared to the background, were obtained for Biological Process (BP), Molecular Function (MF), and Cellular Component (CC) categories, elucidating gene function and localisation within these DEG groups.
TPM data obtained from leaf tissue samples taken before and after drought stress exposure, described here, were used to construct a single co-expression network in R (version 3.6.3), using the WGCNA package (version 1.72-1; Langfelder and Horvath, 2008; Langfelder and Horvath, 2012). 21,870 genes were removed due to too many zero values, leaving 84,888 genes, from 28 samples (14 accessions before and after drought stress) for network construction. Blockwise network construction and module detection was conducted using the blockwiseModules() function according to its default parameters, with several exceptions: network type = signed hybrid, maximum block size = 5000, soft threshold power = 16 (the first power to exceed a scale-free topology fit index of 0.9), minimum module size = 30, merge cut height = 0.25. The exportNetworkToCytoscape() function was used after module detection to create edge and node files for module visualization in Cytoscape. A threshold of 0.1 was used to filter out weak connections between genes.
The agriGO v2.0 Singular Enrichment Analysis tool (Du et al., 2010; Tian et al., 2017) was used to identify gene ontology (GO) terms significantly enriched in each module. To do this, GO terms of genes in each module were compared to GO terms of all genes in the co-expression network. The parameters used were the same as those described for the DEG GO term enrichment analysis above. GO terms used were also retrieved using the method described above.
10,199 of the 84,888 genes included in the network were deemed to be DEGs – equating to 12% of all genes. If DEGs were distributed across modules accordingly to module size, we would expect each module to contain this proportion of DEGs. To determine whether the observed proportion of DEGs in each module was significantly greater than this predicted proportion, we used a one-proportion Z test. Modules were deemed to be significantly enriched in DEGs if p < 0.05.
To identify hub genes, degree (connection) scores were calculated for each gene within a module, either using the Cytoscape (version 3.9.1.; Shannon et al., 2003) network analyser tool (Assenov et al., 2008), or by counting the number of connections to and from each gene in the WGCNA edge file, using the table() function in R. The script used to calculate degree scores in R is available on GitHub (https://github.com/andreaharper/HarperLabScripts/). Cytoscape was used to visualize modules, and for hub gene identification in the majority of cases, however particularly large modules are often difficult to load, view and analyze in Cytoscape. In these cases (modules containing ~2000 genes or more), R was used to calculate degree score in the same way as in Cytoscape (i.e. counting the number of connections to and from each gene in the WGCNA edge file). Those genes in a module with the highest degree scores (most connections) were identified as the central hubs. In some cases, however, multiple genes within a module shared the highest degree score, whilst in other modules, the highest scoring genes were not found to be differentially expressed under drought conditions. In these cases, the highest-scoring DEG was identified as the module’s hub gene, as these genes are both differentially expressed and well connected within the module, and so are more likely to regulate the transcriptional drought response, than a well-connected non-DEG. Those modules found to be significantly enriched in the “response to water” (GO:0009414) GO term (black and turquoise) were also amongst the largest in the co-expression network. These modules, therefore, likely contain genes involved in diverse processes – so, to focus on the response to water, subnetworks were created using genes annotated with the “response to water” (GO:0009414) GO term within the module as guide genes. It was thought that by only examining the connections to and from these genes, the subsequently identified hub gene would be a better candidate regulator of the drought response, than the hub gene of the entire, much larger, module. As with the other modules, the most well-connected DEG was identified as the hub gene in these subnetworks.
Drought stress was found to have a significant effect on plant growth, as both fresh and dry weight differed significantly (t-test: both p < 2.2e-16) between stressed and control plants (Figure 1A). Soil Moisture Content (SMC%) was measured over the course of the experiment (Figure 1B), with the drought stress treatment causing SMC% values of the control and drought groups to differ significantly (p < 2.2e-16) ten days after Zadoks’ growth scale 13 (GS13; Zadoks et al., 1974). No significant difference was identified between the two groups at GS13, before the start of the drought period (p = 0.179). A significant difference in SMC% was observed between the two groups at harvest (p = 0.0006), however. Although statistically significant, the difference in SMC% between the groups at harvest was slight, with average SMC% for both the control (45.9%) and drought (49.5%) groups being within the expected ranges for normal watering conditions. Data used to produce Figures 1A, 1B are available in Supplementary Data S2.
Figure 1 Drought treatment resulted in substantial differences across the panel in both phenotypic measurements and transcriptomic profiles. Ten days of drought stress was found to significantly reduce (t-test: both p < 2.2e-16) average fresh weight by 42.9% and average dry weight by 34.8% (A), while soil moisture content (SMC%) was significantly different at the end of the drought treatment (B). No significant difference was observed between control and drought groups at the start of the drought period. ‘Harvest’ refers to the end of the 3-day recovery period after the end of the drought period, where all above-ground biomass was harvested per individual. Asterisks (A, B) represent significance thresholds; ‘***’ represents p < 0.001. ‘N.S’ represents no significance. An initial exploration of samples and expression data suggested that samples before and after drought had distinct transcriptional profiles (C, D). Principal component analysis (PCA) of variance-stabilised transcript counts from all 28 samples (C) showed clear separation between the two groups on PC1, while differential expression analysis identified 10,199 DEGs with differing expression before and after drought, visualised via a volcano plot (D). Dashed lines indicate DEG thresholds: vertical lines represent the log2FC thresholds of ±1.5, horizontal lines represent the p-value threshold of 0.05. DEGs that meet the criteria are beyond these threshold lines, coloured in light blue. The x-axis represents the log2FC, while the y-axis represents the negative log10 of the p-value for each gene.
921.6 Gb of raw data was generated as a result of sequencing with the Illumina paired-end Novaseq 6000 platform. From 28 samples (pooled RNA samples from 4 replicate plants, for each of the 14 accessions, before and after drought stress), 1.465 x 109 reads were generated; an average of 97.3% and 92.6% of bases had a q-value of ≥ 20 and ≥ 30, respectively, with an error probability of 0.03. GC content of the reads ranged from 53.4% to 57.2%. Data quality was assessed using FastQC, with data for each sample being deemed acceptable, before pre-processing and then quantification with Salmon. Average mapping rate across all samples was 61%. Raw sequence read data were deposited in NCBI’s Gene Expression Omnibus (GSE225797).
Counts of all 28 samples were variance-stabilised using DESeq2 and analysed using principal component analysis (PCA, Figure 1C). The clustering of the samples indicated that the variance within each group was smaller than the variance between groups, however there was more variance on PC2 after drought than before. PC1 and PC2 accounted for 67.9% of the total variance; PC1 (which explained 62.7% of the variation) was able to provide separation between the samples taken before and after drought stress, while PC2 provided separation potentially relating to a spread of tolerance phenotypes across the accessions, albeit explaining far less of the overall variance than PC1.
To investigate the genes that responded to drought stress in wheat leaves during early growth stages, we carried out differential expression analysis between samples taken before and after drought stress. Genes were deemed to be differentially expressed (DEGs) when their FDR-adjusted p-value < 0.05, and their log2FoldChange greater/less than 1.5/-1.5.
We identified a total of 10,199 DEGs; 6051 and 4148 with significantly increased and decreased expression, respectively, in response to drought (Figure 1D). Wide dispersion of the genes in Figure 1D suggests a high level of difference in gene expression between the two groups. Normalised expression data from DESeq2 and differential expression analysis results can be found in Supplementary Data S3, S4, respectively.
To investigate gene function among DEGs, we conducted GO enrichment analysis on both the upregulated and downregulated genes. 231 GO terms were enriched amongst the upregulated genes, while 258 GO terms were enriched amongst from downregulated genes. Output from GO enrichment analyses can be found in Supplementary Data S5, S6.
GO terms related to the stress response were enriched amongst upregulated genes, such as; “response to water” (GO:0009415, FDR = 4.90e-27), “response to stress” (GO:0006950, FDR = 9.70e-11), “response to abiotic stimulus” (GO:0009628, FDR = 9.50e-16), and “response to oxidative stress” (GO:0006979, FDR = 0.0016). Other enriched terms were related to cell wall maintenance (“cell wall organization or biogenesis”, GO:1903338, FDR = 5.10e-07; “cell wall biogenesis”, GO:0042546, FDR = 2.10e-05), and regulation of gene expression and transcription (“regulation of RNA transcription, DNA-templated”, GO:0006355, FDR = 5.00e-19; “regulation of gene expression”, GO:0010468, FDR = 4.60e-18). The most significant enriched GO term was “response water”, followed by “response to acid chemical” (GO:0001101, FDR = 4.90e-27), and “oxidation-reduction process” (GO:0055114, FDR = 1.70e-23).
By contrast, GO terms enriched amongst downregulated genes were related to processes such as photosynthesis (“photosynthesis”, GO:0015979, FDR = 3.10e-76; “thylakoid”, GO:0009579, FDR = 1.20e-72; “chloroplast”, GO:0009507, FDR = 3.70e-07), homeostasis (“cellular homeostasis”, GO:0019725, FDR = 1.00e-11), and substance transport (“transport”, GO:0006810, FDR = 0.0019).
The co-expression network contained 84,888 genes, housed within 81 modules (Supplementary Data S7). Mean module size was 1048, whilst median module size was 165. Module size ranged from 30 to 19,380 genes.
To identify modules associated with the drought response, we conducted GO enrichment analysis on each module, using all the genes included in network construction as background. We expected that modules containing genes involved in regulating the drought response would be enriched in stress-associated GO terms such as “response to water” (GO:0009414), “response to stress” (GO:0006950), or “response to abiotic stimulus” (GO:0009628). 10 of the 81 modules were significantly enriched in such GO terms (Table 1), with the black and turquoise modules being enriched in the GO term “response to water” (FDR = 4.8e-08 and 0.029, respectively).
Table 1 10 modules were significantly enriched in GO terms related to the stress response, according to GO enrichment analysis by the AgriGO v2.0 Singular Enrichment Analysis tool (Du et al., 2010; Tian et al., 2017).
To gain further insight into which modules may contain genes particularly associated with the drought response, DEG enrichment analysis was conducted. 10,199 genes (12% of the genes included in the co-expression network) were deemed to be DEGs. If the number of DEGs was distributed across modules according to size, we would expect 12% of the genes in each module to be DEGs. We found that 17 modules contained a significantly higher proportion of DEGs than expected (Table 2), and so represent groups of co-expressed genes involved in the drought response – the hub genes of these modules, therefore, are promising candidates for master-regulators of the transcriptional drought response.
Combined, these analyses identified modules which were particularly stress-associated, either as a result of the enrichment of stress-associated GO terms, or the enrichment of DEGs. Only hub genes from those modules listed in Tables 1, 2, therefore, were examined further to determine whether they may be promising candidate master-regulators of the transcriptional early drought response.
The hub genes within those modules deemed to stress-associated (Tables 1, 2) may act as master-regulators of the transcriptional drought response, as they are significantly co-expressed with many stress-associated and/or drought-responsive genes. These hub genes (Table 3) seemingly play roles in diverse processes, such as stress hormone signalling (TraesCS6A02G340100 and TraesCS4D02G325200) or the biotic stress response (TraesCS5A02G052600 and TraesCSU02G171500). One hub gene, meanwhile, was found to be drought-responsive in the present work, but is likely a key actor in photosynthesis, and so is probably required to aid growth and development under normal conditions (TraesCS6D02G247400), whereas others were completely uncharacterized and do not share sequence identity with any well understood gene (TraesCS3D02G361500, TraesCS4D02G251500, TraesCS4A02G212000, and TraesCS4A02G190700), making their potential role as regulators of the drought response completely novel. Modules which were particularly large likely contained genes involved in diverse processes. Some of the largest modules were also significantly enriched in the “response to water” (GO:0009414) GO term, therefore to identify candidate master-regulators of processes of interest (namely, the drought response) subnetworks were created using genes annotated with this GO term as guide genes. This was done for the black and turquoise modules, with the subsequent subnetworks’ hub genes (TraesCS5D02G379200 and TraesCS6D02G234700, respectively) being identified as dehydrins.
Table 3 Hub genes identified in stress-associated modules may be strong candidates for master-regulators of the drought response, based on their high number of connections to other genes within stress-associated modules.
Hub genes in these stress-associated modules (Table 3) represent valuable targets for further inquiry into the regulation of the transcriptional drought response, and as targets for breeders in for the production of drought tolerant varieties. However, two of these hub genes, TraesCS5D02G379200 (TaDHN4-D1) and TraesCS3D02G361500 (uncharacterised gene), were deemed to be particularly promising candidates as master-regulators of both the transcriptional and physiological drought responses, due to the likely functions of the genes they were connected to in the co-expression network. TraesCS5D02G379200 may regulate the expression of a suite of fellow dehydrins, as well as stress-responsive transcription factors and genes which may affect stomatal dynamics – all of which show significant up-regulation of expression under drought stress. TraesCS3D02G361500 may also regulate the expression of genes likely involved in controlling stomatal dynamics, as well as other potentially guard cell-localized genes involved in stomatal morphogenesis, and several aquaporins – however, unlike TraesCS5D02G379200, the hub, and the genes it is connected to, are downregulated significantly under drought stress.
It is widely believed that landraces are an important genetic resource available to breeders for the production of more climate-resilient wheat varieties, thanks to their extensive phenotypic and genetic diversity (Zeven, 1998; Reynolds et al., 2007; Corrado and Rao, 2017; Schmidt et al., 2019; Cseh et al., 2021; Tehseen et al., 2022). This diversity has been extensively exploited in grass crops such as rice and barley, with many landrace accessions either being screened for drought tolerance (Van Oosterom et al., 1993; Tardy et al., 1998; Munasinghe et al., 2017; Dbira et al., 2018; Kumar et al., 2019; Mishra et al., 2019; Boudiar et al., 2020; Sabouri et al., 2022; Bakhshi and Shahmoradi, 2023), utilized to identify the genetic determinants of drought tolerance (Yu et al., 2012; Fan et al., 2015; Reinert et al., 2016; Hoang et al., 2019; Beena et al., 2021), or used to better understand the drought response (Cantalapiedra et al., 2017; Khodaeiaminjan et al., 2023). Wheat landraces, however, remain relatively underutilized in the study of drought tolerance and the drought response (Dodig et al., 2012; Lin et al., 2019; Naderi et al., 2020; Gómez-Espejo et al., 2022). After highlighting both its extensive genetic diversity, and its usefulness in the study of early thermotolerance (Barratt et al., 2023), here we show the YoGI landrace panel can also be used to effectively study the response to early drought stress, and aid the production of drought tolerant wheat varieties.
The effect of drought stress on yield is well studied (Zhang et al., 2018; Kim et al., 2019; Qaseem et al., 2019; Senapati et al., 2019; Abou-Elwafa and Shehzad, 2021; Lan et al., 2022; Wan et al., 2022), but as the climate continues to change, periods of water shortage coinciding with the early growth stages of spring wheat crop growth are likely to become more common around the world. There has already been evidence of this, with major spring wheat-producing countries such as the USA and the UK experiencing drier than average periods in the months after spring wheat sowing (NOAA National Centers for Environmental Information, 2022a; NOAA National Centers for Environmental Information, 2022b). The majority of the work examining the effect of drought stress on wheat seedling growth has not aimed to identify regulators of the drought response during this early stage of development, however (Guo et al., 2017; Sallam et al., 2018; Ahmed et al., 2020; Ahmed et al., 2022; Mahpara et al., 2022; Nardino et al., 2022; Sharma et al., 2022) – something that remains relatively understudied (Ajigboye et al., 2017; Mao et al., 2020; Vuković et al., 2022). The present work, therefore, takes a novel approach to elucidate how the early drought response is transcriptionally controlled in wheat landraces, and represents a promising step towards the production of more drought tolerant varieties.
Our analysis demonstrates that the expression profiles of spring wheat are vastly different before and after drought; over 10,000 genes were differentially expressed between the two groups. GO term enrichment analysis of DEGs indicated that growth and development was deprioritized; DEGs annotated with photosynthesis-, and chlorophyll-related GO terms were largely downregulated. Similarly, there was widespread downregulation of genes annotated with enriched Cellular Component GO terms such as “thylakoid”, suggesting a reduction in light-dependent reactions. Photosynthetic regulation is associated with both oxidative and drought stress responses; stress-related changes in photosynthetic activity under various environmental stress conditions have been identified in other cereals such as rice (Gan et al., 2019; Yu et al., 2020b), as plants seek to limit damage to critical components. Downregulation of genes involved in photosynthesis under drought is common among grasses, with studies in Miscanthus (De Vega et al., 2021), Brachypodium distachyon (Priest et al., 2014), and rice (Liang et al., 2021) demonstrating similar trends.
Reduced photosynthetic activity can result in an excess of absorbed light energy, inducing the generation of toxic reactive oxygen species (ROS; Pospíšil, 2016). GO enrichment analyses conducted on both up- and downregulated DEGs identified a number of enriched GO terms (such as “response to oxidative stress”) involved in both the production and mitigation of ROS and other oxidative agents. Both up- and down-regulation of genes involved in cellular oxidation and reduction has previously been observed in other grasses, like rice (Sirohi et al., 2020). ROS accumulation, while promoting immune responses and stomatal guard cell closure (Song et al., 2014), can also cause oxidative damage to DNA and photosynthetic machinery, potentially leading to cell death (Huang et al., 2019; Ye et al., 2021). DEGs annotated with such terms were primarily identified as peroxidases and oxidases; their presence among both up- and downregulated DEGs is likely due to their cellular localization, mediating ROS accumulation in some tissues over others (Csiszár et al., 2012).
Our GO enrichment of the upregulated genes identified a number of DEGs annotated with drought- and osmotic-stress enriched GO terms. These genes included a variety of dehydrins and other late embryogenesis abundant (LEA) genes, known key actors in various abiotic stress responses in wheat (Kosová et al., 2014; Hassan et al., 2015; Liu et al., 2019). Studies in species such as B. distachyon and O. sativa were similarly able to identify an upregulation of dehydrins (Smita et al., 2013; Sancho et al., 2022), suggesting that this is a common response among grasses.
Downregulation of genes under the term “transport”, which included genes involved in water transport processes, likely facilitated the conservation of water for critical organelles and guard cells, as well as mediating water loss by decreasing membrane permeability (Maurel et al., 2008; Patel and Mishra, 2021).
These trends in the expression of stress and growth-associated genes indicate a shift towards stress-mitigation, often seen with abiotic stresses such as harsh drought (Zhang et al., 2020).
The black module was significantly enriched in DEGs (Table 2), as well as the GO term “response to water” (FDR-adjusted p-value = 4.8E-08, Table 1), suggesting the module houses genes which play key roles in the drought response. Due to the size of the module (2184 genes), it is likely to contain genes involved in various processes besides the drought response. To focus on those genes most likely to play a role in the drought response, a subnetwork was created using the genes within the module which possessed the significantly enriched GO term “response to water” as guide genes. The subnetwork contained 1544 genes, and 6562 connections between genes (Figure 2A).
Figure 2 Drought-associated modules house candidate master-regulators of the early drought response. To focus on genes likely involved in the drought response within the large black module, a subnetwork was created (A), whereas the ivory module (C) was small enough to be analysed in its entirety. The hub genes within the black subnetwork (TraesCS5D02G379200) and ivory module (TraesCS3D02G361500) are highlighted in yellow and enlarged. Expression of TraesCS5D02G379200 (B) was found to be significantly upregulated (log2FC = 5.87) in response to drought stress, whereas TraesCS3D02G361500 (D) expression was significantly downregulated (log2FC = -3.75).
The central hub gene was TraesCS5D02G379200, which possessed the enriched GO term “response to water” and was connected to 1222 other genes in both the full module (where it had the sixth highest degree score) and the subnetwork. The gene shares 100% sequence identity with Aegilops tauschii subsp. strangulata dehydrin DHN2, but has been classed as TaDHN4-D1 in recent work (Hao et al., 2022). We found that expression of the gene was upregulated significantly (log2FC = 5.87) after drought stress (Figure 2B); consistent with the commonly observed expression responses of dehydrins in response to drought stress (Sun et al., 2021; Tiwari and Chakrabarty, 2021). TaDHN4 belongs to the YSK2 sub-family of dehydrins (Wang et al., 2014), a sub-family shown to increase stress tolerance when overexpressed in Arabidopsis (Brini et al., 2007) and whose expression, consistent with the present work, was most strongly upregulated in dehydrated leaves of wheat seedlings (Wang et al., 2014). Four of the five most well-connected genes in the subnetwork were homeologues, or duplicates, of TaDHN4; TraesCS5D02G379200 (hub, TaDHN4-D1), TraesCS5B02G372100 (TaDHN4-B1), TraesCS5B02G372200 (TaDHN4-B2) and TraesCS5A02G369900 (TaDHN4-A2) – suggesting both that all homeologues share similar expression responses, and that there is likely functional redundancy amongst the homeologues, meaning they may all play roles in regulating the drought response.
Further support for the hypothesis that the hub gene may act as a master-regulator of the drought response comes from the genes it is connected to in the subnetwork. We found that the hub was connected to 220 DEGs in the subnetwork, 62.3% of all DEGs within it. Amongst these DEGs were several other members of the dehydrin family, besides the hub’s homeologues and duplicates to which it was also connected: TraesCS5B02G426800 (log2FC = 9.89) encodes T. aestivum dehydrin Rab15-like, whilst TraesCS6A02G350600 (log2FC = 8.39) is T.aestivum dehydrin DHN3-like. The hub gene is also connected to other genes with different functions related to the drought response: TraesCS2D02G364500 (log2FC = 8.83), and its homeologue TraesCS2A02G367700, are T. aestivum chromosome D caleosin (Clo10) – a member of another drought-responsive gene family thought to be involved in the drought response, via action on stomatal aperture and transpiration (Aubert et al., 2010; Kim et al., 2011). The hub may also have far-reaching effects on global gene expression, due to its connection to drought-responsive transcription factors such as TaNAC29, TraesCS2A02G367700 (log2FC = 7.32), which has been shown to increase drought and salinity tolerance when expressed in Arabidopsis (Huang et al., 2015). The hub’s connection to drought-responsive genes with these kinds of functions further suggests that it may act as a master-regulator of the drought response.
Dehydrins act as molecular chaperones to maintain protein structure and functional folding under stressful cellular conditions, so the hub gene’s ability to regulate gene expression may not be immediately apparent. Recent evidence, however, suggests that there are multiple potential mechanisms by which dehydrins can control the expression of other genes. This can occur as a result of their chaperone activity, protecting transcription factors and other transcriptional regulators from damage by cellular stress, ensuring their function and subsequent effect on gene expression is maintained (Tiwari and Chakrabarty, 2021). There is also emerging evidence that dehydrins themselves may act as transcription factors, with rice lines overexpressing OsDhn-Rab16D showing increased expression of ABA signalling and stress-responsive genes (Tiwari et al., 2019). Dehydrins may also effect gene expression by binding directly to DNA and protecting it from damage by ROS during stress events; this is not a commonly-observed role played by dehydrins, however, only being reported in grape and citrus (Hara et al., 2009; Boddington and Graether, 2019). Each of these roles would rely on the hub gene protein being localized in the nucleus, but, according to recent work, the hub gene appears to be localized to the cytoplasm (Hao et al., 2022). In the present work, we have seen evidence that the hub gene dehydrin may act to control the expression of other drought-responsive dehydrins, as well as several other stress-responsive genes which seemingly play roles in the drought response, suggesting either the hub gene may in fact be localized to the nucleus under drought stress, or that the protection it provides transcriptional regulators in the cytoplasm is sufficient to allow them to act functionally once translocated to the nucleus.
The ivory module (Figure 2C) was identified as drought-associated, as it was significantly enriched in DEGs (Table 2). The most well-connected gene in the module was TraesCS3D02G361500, with its homeologues (TraesCS3A02G368600 and TraesCS3B02G400100) also amongst the top five most well-connected genes in the module. Expression of the hub gene, T. aestivum uncharacterized LOC123079795, was found to be downregulated under drought stress (log2FC = -3.75, Figure 2D), suggesting the gene may play a repressive role during the transcriptional and physiological drought responses.
35 of the 41 genes the hub was connected to were also DEGs, all of which were downregulated under drought stress, with several having functions related to the drought response. TraesCS1A02G070200 (log2FC = -4.79) is T. aestivum jasmonate-induced oxygenase 1-like, and also shared some sequence identity (69%) to a large region of its Arabidopsis namesake, and orthologue (identified using Ensembl Plants; Yates et al., 2022), AtJOX1. The gene is a negative regulator of jasmonic acid (JA) signaling, conducting hydroxylation of JA, inactivating it in the signaling pathway (Caarls et al., 2017). JA is known to accumulate in plant cells during drought stress and increase tolerance to drought stress in wheat (Wasternack, 2014; Ali and Baek, 2020; Wang et al., 2021). JA has also been shown to act in unison with ABA to control stomatal closure in Arabidopsis (Hossain et al., 2011), suggesting the hub gene may be able to determine stomatal aperture via control over TraesCS1A02G070200 expression, and subsequently, JA signaling.
The hub gene is also connected to several other DEGs potentially involved in regulating stomatal opening. AtAO1 plays a role in programmed cell death via its production of reactive oxygen species, as well as a role in protoxylem differentiation in root tissue (Møller and McPherson, 1998; Ghuge et al., 2015a; Ghuge et al., 2015b), and is the Arabidopsis orthologue (identified using Ensembl Plants; Yates et al., 2022) of TraesCS4B02G282700 (log2FC = -4.61) which encodes T. aestivum primary amine oxidase 1-like. As well as this, AtAO1 expression was found to be both induced by methyl-jasmonate, and localized in guard cells, and other tissues involved in regulating water homeostasis – leading the authors to suggest that the gene may play a key role in regulating stomatal closure (Ghuge et al., 2015b). Previous work suggests AtAO1 promotes stomatal closure, however here we see TraesCS4B02G282700 expression being downregulated under drought stress, suggesting it may act to repress stomatal closure in wheat. TraesCS4A02G398700 (log2FC = -4.2) was also connected to the hub gene, and similarly may play role in stomatal dynamics. The gene is T. aestivum GDSL esterase/lipase APG-like, whilst also sharing sequence identity (66%) with large regions of AtGGL19, a gene found to be expressed in Arabidopsis guard, pavement and mesophyll cells, whose expression was also downregulated under drought stress, suggesting the gene may play a role in stomatal closure (Xiao et al., 2021). These observations, paired with the downregulation of TraesCS4A02G398700 under drought stress, suggest the gene may act to repress stomatal closure. TraesCS1B02G176000 was another downregulated DEG (log2FC = -3.96) connected to the hub gene, and encodes T. aestivum cytokinin dehydrogenase 3-like. The gene appears to also be involved in stomatal biology, as a result of its inactivation of cytokinins. However, overexpression of TraesCS1B02G176000’s Arabidopsis namesake, AtCKX3, improved drought tolerance in tomato and Arabidopsis thanks to reduced transpiration, likely from reduced leaf area and stomatal density (Werner et al., 2010; Farber et al., 2016). The downregulation of TraesCS1B02G176000 in the present work, however, suggests it may act to increase water loss, unlike its Arabidopsis namesake. Despite the gene’s name, TraesCS1B02G176000 showed the highest level of sequence identity to AtCKX6 – a guard cell-localized gene with a potential role in stomatal morphogenesis (Werner et al., 2003). Because of this, and its downregulation under drought stress in the present work, we suggest that TraesCS1B02G176000 may play a positive role in stomatal morphogenesis, as reducing the production of stomata under drought stress is likely to limit the amount of water loss via transpiration (Bertolino et al., 2019).
Two genes involved in water transport were also connected to the hub. TraesCS4D02G024400, T. aestivum protein NRT1/PTR FAMILY 8.3-like, was downregulated under drought stress (log2FC = -3.34) and shares sequence identity (63%) with a large region of its namesake, AtNPF8.3. The gene appears to play a role in water uptake in germinating Arabidopsis seeds, as knockout mutant seeds showed a 17% lower water content compared to WT (Choi et al., 2020). TraesCS4B02G310900 (log2FC = -1.74) also appears to be involved in water transport, as it is T. aestivum aquaporin TIP1-1-like, but shares marginally more sequence identity with AtTIP2 (73%) than AtTIP1 (72%). The downregulation of these genes under drought stress in the present work, paired with their membership of a module containing so many potential guard cell-localized genes, suggests that these genes may act to control guard cell turgidity, via their control of water movement in and out of the cells. When guard cells are turgid, stomata are open, whilst flaccid guard cells cause stomata to close – suggesting that the downregulation of these water uptake genes in response to drought stress may be a mechanism to cause stomatal closure, and prevent excess moisture loss under water shortage. Recent work has shed light on the relationship between water uptake proteins, such as aquaporins, and stomatal dynamics (Grondin et al., 2015; Ding and Chaumont, 2020a; Ding and Chaumont, 2020b; Cui et al., 2021), suggesting the hub may act to reduce water loss via its downregulation of these water uptake genes under drought stress.
Here, we present the YoGI landrace panel as a valuable resource for the study of the transcriptional control of the drought response, and useful tool for breeders in the development of climate-resilient wheat varieties. We identified thousands of genes differentially expressed before and after exposure to drought stress during early development. The use of co-expression network analysis allowed us to identify several hub genes which may act as master-regulators of the transcriptional early drought response. Two very promising candidate hub genes, however, may act to coordinate both the transcriptional and physiological early drought responses, as they potentially control the drought-responsive expression of stress-associated genes such as dehydrins, aquaporins and genes involved in stomatal dynamics. Further work is required, however, to make the link between the potential action of these hub genes on drought-responsive gene expression, and the physiological drought response.
The datasets presented in this study can be found in online repositories. The original data is publicly available at NCBI, GSE225797.
LB, IR and AH conceived and planned the project. LB and IR performed plant growth experiments and RNA extraction. IR and SF performed transcriptome data mapping. LB and IR conducted transcriptomic analyses. LB, IR and AH wrote the manuscript, and all authors reviewed it. All authors contributed to the article and approved the submitted version.
This work was supported by UK Biotechnology and Biological Sciences Research Council White Rose Doctorial Training Partnerships (DTP) in Mechanistic Biology (BB/M011151/1, BB/T007222/1). The authors thank the Germplasm Resource Unit at John Innes Centre, UK (Grant number BBS/E/J/000PR8000) for providing germplasm and sharing the genotype and genetic map information, as well as CIMMYT, Mexico, and the Crop Research Institute, Czechia for providing additional germplasm.
We thank the horticultural staff at the University of York for their help in maintaining and growing plants, as well as Sally James and Lesley Gilbert from the Technology Facility at the University of York for their assistance in assessing RNA quality prior to sequencing.
The authors declare that the research was conducted in the absence of any commercial or financial relationships that could be construed as a potential conflict of interest.
All claims expressed in this article are solely those of the authors and do not necessarily represent those of their affiliated organizations, or those of the publisher, the editors and the reviewers. Any product that may be evaluated in this article, or claim that may be made by its manufacturer, is not guaranteed or endorsed by the publisher.
The Supplementary Material for this article can be found online at: https://www.frontiersin.org/articles/10.3389/fpls.2023.1212559/full#supplementary-material
Abou-Elwafa, S. F., Shehzad, T. (2021). Genetic diversity, GWAS and prediction for drought and terminal heat stress tolerance in bread wheat (Triticum aestivum l.). Genet. Resour. Crop Evol. 68, 711–728. doi: 10.1007/s10722-020-01018-y
Ahmed, H. G. M.-D., Zeng, Y., Shah, A. N., Yar, M. M., Ullah, A., Ali, M. (2022). Conferring of drought tolerance in wheat (Triticum aestivum l.) genotypes using seedling indices. Front. Plant Sci. 13. doi: 10.3389/fpls.2022.961049
Ahmed, H. G. M.-D., Zeng, Y., Yang, X., Anwaar, H. A., Mansha, M. Z., Hanif, C. M. S., et al. (2020). Conferring drought-tolerant wheat genotypes through morpho-physiological and chlorophyll indices at seedling stage. Saudi J. Biol. Sci. 27, 2116–2123. doi: 10.1016/j.sjbs.2020.06.019
Ajigboye, O. O., Lu, C., Murchie, E. H., Schlatter, C., Swart, G., Ray, R. V. (2017). Altered gene expression by sedaxane increases PSII efficiency, photosynthesis and growth and improves tolerance to drought in wheat seedlings. Pestic. Biochem. Physiol. 137, 49–61. doi: 10.1016/j.pestbp.2016.09.008
Ali, M. S., Baek, K.-H. (2020). Jasmonic acid signaling pathway in response to abiotic stresses in plants. Int. J. Mol. Sci. 21, 621. doi: 10.3390/ijms21020621
Andleeb, T., Knight, E., Borrill, P. (2023). Wheat NAM genes regulate the majority of early monocarpic senescence transcriptional changes including nitrogen remobilization genes. G3 13. doi: 10.1093/g3journal/jkac275
Aprile, A., Mastrangelo, A. M., De Leonardis, A. M., Galiba, G., Roncaglia, E., Ferrari, F., et al. (2009). Transcriptional profiling in response to terminal drought stress reveals differential responses along the wheat genome. BMC Genomics 10, 279. doi: 10.1186/1471-2164-10-279
Asard, H., Barbaro, R., Trost, P., Bérczi, A. (2013). Cytochromes b561: ascorbate-mediated trans-membrane electron transport. Antioxid. Redox Signal. 19, 1026–1035. doi: 10.1089/ars.2012.5065
Assenov, Y., Ramírez, F., Schelhorn, S.-E., Lengauer, T., Albrecht, M. (2008). Computing topological parameters of biological networks. Bioinformatics 24, 282–284. doi: 10.1093/bioinformatics/btm554
Aubert, Y., Vile, D., Pervent, M., Aldon, D., Ranty, B., Simonneau, T., et al. (2010). RD20, a stress-inducible caleosin, participates in stomatal control, transpiration and drought tolerance in arabidopsis thaliana. Plant Cell Physiol. 51, 1975–1987. doi: 10.1093/pcp/pcq155
Bakhshi, B., Shahmoradi, S. S. (2023). Simultaneous selection of high-yielding and drought-tolerant barley landraces using GT, GYT and GYSI methodologies. Cereal Res. Commun. 51, 237–248. doi: 10.1007/s42976-022-00290-1
Barratt, L. J., He, Z., Fellgett, A., Wang, L., McQueen Mason, S., Bancroft, I., et al. (2023). Co-Expression network analysis of diverse wheat landraces reveals marker of early thermotolerance and candidate master-regulator of thermotolerance genes. Plant J. doi: 10.1111/tpj.16248
Beena, R., Kirubakaran, S., Nithya, N., Manickavelu, A., Sah, R. P., Abida, P. S., et al. (2021). Association mapping of drought tolerance and agronomic traits in rice (Oryza sativa l.) landraces. BMC Plant Biol. 21, 484. doi: 10.1186/s12870-021-03272-3
Benjamini, Y., Hochberg, Y. (1995). Controlling the false discovery rate: a practical and powerful approach to multiple testing. J. R. Stat. Soc Ser. B Stat. Methodol. 57, 289–300. doi: 10.1111/j.2517-6161.1995.tb02031.x
Bertolino, L. T., Caine, R. S., Gray, J. E. (2019). Impact of stomatal density and morphology on water-use efficiency in a changing world. Front. Plant Sci. 10. doi: 10.3389/fpls.2019.00225
Boddington, K. F., Graether, S. P. (2019). Binding of a vitis riparia dehydrin to DNA. Plant Sci. 287, 110172. doi: 10.1016/j.plantsci.2019.110172
Bolger, A. M., Lohse, M., Usadel, B. (2014). Trimmomatic: a flexible trimmer for illumina sequence data. Bioinformatics 30, 2114–2120. doi: 10.1093/bioinformatics/btu170
Borrill, P., Harrington, S. A., Simmonds, J., Uauy, C. (2019). Identification of transcription factors regulating senescence in wheat through gene regulatory network modelling. Plant Physiol. 180, 1740–1755. doi: 10.1104/pp.19.00380
Boudiar, R., Casas, A. M., Gioia, T., Fiorani, F., Nagel, K. A., Igartua, E. (2020). Effects of low water availability on root placement and shoot development in landraces and modern barley cultivars. Agronomy 10, 134. doi: 10.3390/agronomy10010134
Brini, F., Hanin, M., Lumbreras, V., Amara, I., Khoudi, H., Hassairi, A., et al. (2007). Overexpression of wheat dehydrin DHN-5 enhances tolerance to salt and osmotic stress in arabidopsis thaliana. Plant Cell Rep. 26, 2017–2026. doi: 10.1007/s00299-007-0412-x
Caarls, L., Elberse, J., Awwanah, M., Ludwig, N. R., de Vries, M., Zeilmaker, T., et al. (2017). Arabidopsis JASMONATE-INDUCED OXYGENASES down-regulate plant immunity by hydroxylation and inactivation of the hormone jasmonic acid. Proc. Natl. Acad. Sci. U. S. A. 114, 6388–6393. doi: 10.1073/pnas.1701101114
Cantalapiedra, C. P., García-Pereira, M. J., Gracia, M. P., Igartua, E., Casas, A. M., Contreras-Moreira, B. (2017). Large Differences in gene expression responses to drought and heat stress between elite barley cultivar Scarlett and a Spanish landrace. Front. Plant Sci. 8. doi: 10.3389/fpls.2017.00647
Chen, H.-Y., Hsieh, E.-J., Cheng, M.-C., Chen, C.-Y., Hwang, S.-Y., Lin, T.-P. (2016). ORA47 (octadecanoid-responsive AP2/ERF-domain transcription factor 47) regulates jasmonic acid and abscisic acid biosynthesis and signaling through binding to a novel cis-element. New Phytol. 211, 599–613. doi: 10.1111/nph.13914
Choi, M.-G., Kim, E. J., Song, J.-Y., Choi, S.-B., Cho, S.-W., Park, C. S., et al. (2020). Peptide transporter2 (PTR2) enhances water uptake during early seed germination in arabidopsis thaliana. Plant Mol. Biol. 102, 615–624. doi: 10.1007/s11103-020-00967-3
Corrado, G., Rao, R. (2017). Towards the genomic basis of local adaptation in landraces. Diversity 9, 51. doi: 10.3390/d9040051
Cseh, A., Poczai, P., Kiss, T., Balla, K., Berki, Z., Horváth, Á., et al. (2021). Exploring the legacy of central European historical winter wheat landraces. Sci. Rep. 11, 23915. doi: 10.1038/s41598-021-03261-4
Csiszár, J., Gallé, A., Horváth, E., Dancsó, P., Gombos, M., Váry, Z., et al. (2012). Different peroxidase activities and expression of abiotic stress-related peroxidases in apical root segments of wheat genotypes with different drought stress tolerance under osmotic stress. Plant Physiol. Biochem. 52, 119–129. doi: 10.1016/j.plaphy.2011.12.006
Cui, Y., Zhao, Y., Lu, Y., Su, X., Chen, Y., Shen, Y., et al. (2021). In vivo single-particle tracking of the aquaporin AtPIP2;1 in stomata reveals cell type-specific dynamics. Plant Physiol. 185, 1666–1681. doi: 10.1093/plphys/kiab007
Danyluk, J., Houde, M., Rassart, E., Sarhan, F. (1994). Differential expression of a gene encoding an acidic dehydrin in chilling sensitive and freezing tolerant gramineae species. FEBS Lett. 344, 20–24. doi: 10.1016/0014-5793(94)00353-x
Danyluk, J., Perron, A., Houde, M., Limin, A., Fowler, B., Benhamou, N., et al. (1998). Accumulation of an acidic dehydrin in the vicinity of the plasma membrane during cold acclimation of wheat. Plant Cell 10, 623–638. doi: 10.1105/tpc.10.4.623
Dbira, S., Al Hassan, M., Gramazio, P., Ferchichi, A., Vicente, O., Prohens, J., et al. (2018). Variable levels of tolerance to water stress (Drought) and associated biochemical markers in Tunisian barley landraces. Molecules 23, 613. doi: 10.3390/molecules23030613
De Vega, J. J., Teshome, A., Klaas, M., Grant, J., Finnan, J., Barth, S. (2021). Physiological and transcriptional response to drought stress among bioenergy grass miscanthus species. Biotechnol. Biofuels 14, 60. doi: 10.1186/s13068-021-01915-z
Ding, L., Chaumont, F. (2020a). Aquaporin mediating stomatal closure is associated with water conservation under mild water deficit. bioRxiv 2020, 4.15.042234. doi: 10.1101/2020.04.15.042234
Ding, L., Chaumont, F. (2020b). Are aquaporins expressed in stomatal complexes promising targets to enhance stomatal dynamics? Front. Plant Sci. 11. doi: 10.3389/fpls.2020.00458
Dodig, D., Zorić, M., Kandić, V., Perović, D., Šurlan-Momirović, G. (2012). Comparison of responses to drought stress of 100 wheat accessions and landraces to identify opportunities for improving wheat drought resistance. Plant Breed. 131, 369–379. doi: 10.1111/j.1439-0523.2011.01941.x
Du, L., Huang, X., Ding, L., Wang, Z., Tang, D., Chen, B., et al. (2022). TaERF87 and TaAKS1 synergistically regulate TaP5CS1/TaP5CR1-mediated proline biosynthesis to enhance drought tolerance in wheat. New Phytol. 237 (1), 232–250. doi: 10.1111/nph.18549
Du, Z., Zhou, X., Ling, Y., Zhang, Z., Su, Z. (2010). agriGO: a GO analysis toolkit for the agricultural community. Nucleic Acids Res. 38, W64–W70. doi: 10.1093/nar/gkq310
Dunn, J., Hunt, L., Afsharinafar, M., Meselmani, M. A., Mitchell, A., Howells, R., et al. (2019). Reduced stomatal density in bread wheat leads to increased water-use efficiency. J. Exp. Bot. 70, 4737–4748. doi: 10.1093/jxb/erz248
Fan, Y., Shabala, S., Ma, Y., Xu, R., Zhou, M. (2015). Using QTL mapping to investigate the relationships between abiotic stress tolerance (drought and salinity) and agronomic and physiological traits. BMC Genomics 16, 43. doi: 10.1186/s12864-015-1243-8
Farber, M., Attia, Z., Weiss, D. (2016). Cytokinin activity increases stomatal density and transpiration rate in tomato. J. Exp. Bot. 67, 6351–6362. doi: 10.1093/jxb/erw398
Feng, Q., Cubría-Radío, M., Vavrdová, T., De Winter, F., Schilling, N., Huysmans, M., et al. (2023). Repressive ZINC FINGER OF ARABIDOPSIS THALIANA proteins promote programmed cell death in the arabidopsis columella root cap. Plant Physiol. 192 (2), 1151–1167. doi: 10.1093/plphys/kiad130
Food and Agriculture Organization of the United Nations, United Nations International Children’s Emergency Fund, World Health Organization, World Food Programme, International Fund for Agriculture Development (2018). The state of food security and nutrition in the world 2018: building climate resilience for food security and nutrition (Rome, Italy: Food & Agriculture Org). Available at: https://play.google.com/store/books/details?id=LC9uDwAAQBAJ.
Gan, P., Liu, F., Li, R., Wang, S., Luo, J. (2019). Chloroplasts- beyond energy capture and carbon fixation: tuning of photosynthesis in response to chilling stress. Int. J. Mol. Sci. 20, 5046. doi: 10.3390/ijms20205046
Ghuge, S. A., Carucci, A., Rodrigues-Pousada, R. A., Tisi, A., Franchi, S., Tavladoraki, P., et al. (2015a). The apoplastic copper AMINE OXIDASE1 mediates jasmonic acid-induced protoxylem differentiation in arabidopsis roots. Plant Physiol. 168, 690–707. doi: 10.1104/pp.15.00121
Ghuge, S. A., Carucci, A., Rodrigues-Pousada, R. A., Tisi, A., Franchi, S., Tavladoraki, P., et al. (2015b). The MeJA-inducible copper amine oxidase AtAO1 is expressed in xylem tissue and guard cells. Plant Signal. Behav. 10, e1073872. doi: 10.1080/15592324.2015.1073872
Godfray, H. C. J., Beddington, J. R., Crute, I. R., Haddad, L., Lawrence, D., Muir, J. F., et al. (2010). Food security: the challenge of feeding 9 billion people. Science 327, 812–818. doi: 10.1126/science.1185383
Gómez-Espejo, A. L., Sansaloni, C. P., Burgueño, J., Toledo, F. H., Benavides-Mendoza, A., Reyes-Valdés, M. H. (2022). Worldwide selection footprints for drought and heat in bread wheat (Triticum aestivum l.). Plants 11, 2289. doi: 10.3390/plants11172289
Greenshields, D. L., Liu, G., Selvaraj, G., Wei, Y. (2005). Differential regulation of wheat quinone reductases in response to powdery mildew infection. Planta 222, 867–875. doi: 10.1007/s00425-005-0029-7
Grondin, A., Rodrigues, O., Verdoucq, L., Merlot, S., Leonhardt, N., Maurel, C. (2015). Aquaporins contribute to ABA-triggered stomatal closure through OST1-mediated phosphorylation. Plant Cell 27, 1945–1954. doi: 10.1105/tpc.15.00421
Guo, Q., Wang, Y., Zhang, H., Qu, G., Wang, T., Sun, Q., et al. (2017). Alleviation of adverse effects of drought stress on wheat seed germination using atmospheric dielectric barrier discharge plasma treatment. Sci. Rep. 7, 16680. doi: 10.1038/s41598-017-16944-8
Hansen, J., Sato, M., Ruedy, R., Lo, K., Lea, D. W., Medina-Elizade, M. (2006). Global temperature change. Proc. Natl. Acad. Sci. U. S. A. 103, 14288–14293. doi: 10.1073/pnas.0606291103
Hao, Y., Hao, M., Cui, Y., Kong, L., Wang, H. (2022). Genome-wide survey of the dehydrin genes in bread wheat (Triticum aestivum l.) and its relatives: identification, evolution and expression profiling under various abiotic stresses. BMC Genomics 23, 73. doi: 10.1186/s12864-022-08317-x
Hara, M., Shinoda, Y., Tanaka, Y., Kuboi, T. (2009). DNA Binding of citrus dehydrin promoted by zinc ion. Plant Cell Environ. 32, 532–541. doi: 10.1111/j.1365-3040.2009.01947.x
Hassan, N. M., El-Bastawisy, Z. M., El-Sayed, A. K., Ebeed, H. T., Nemat Alla, M. M. (2015). Roles of dehydrin genes in wheat tolerance to drought stress. J. Advert. Res. 6, 179–188. doi: 10.1016/j.jare.2013.11.004
Hoang, G. T., Van Dinh, L., Nguyen, T. T., Ta, N. K., Gathignol, F., Mai, C. D., et al. (2019). Genome-wide association study of a panel of Vietnamese rice landraces reveals new QTLs for tolerance to water deficit during the vegetative phase. Rice 12, 4. doi: 10.1186/s12284-018-0258-6
Hoekstra, A. Y., Mekonnen, M. M. (2012). The water footprint of humanity. Proc. Natl. Acad. Sci. U. S. A. 109, 3232–3237. doi: 10.1073/pnas.1109936109
Hossain, M. A., Munemasa, S., Uraji, M., Nakamura, Y., Mori, I. C., Murata, Y. (2011). Involvement of endogenous abscisic acid in methyl jasmonate-induced stomatal closure in arabidopsis. Plant Physiol. 156, 430–438. doi: 10.1104/pp.111.172254
Huang, H., Ullah, F., Zhou, D.-X., Yi, M., Zhao, Y. (2019). Mechanisms of ROS regulation of plant development and stress responses. Front. Plant Sci. 10. doi: 10.3389/fpls.2019.00800
Huang, Q., Wang, Y., Li, B., Chang, J., Chen, M., Li, K., et al. (2015). TaNAC29, a NAC transcription factor from wheat, enhances salt and drought tolerance in transgenic arabidopsis. BMC Plant Biol. 15, 268. doi: 10.1186/s12870-015-0644-9
Iizumi, T., Kotoku, M., Kim, W., West, P. C., Gerber, J. S., Brown, M. E. (2018). Uncertainties of potentials and recent changes in global yields of major crops resulting from census- and satellite-based yield datasets at multiple resolutions. PloS One 13, e0203809. doi: 10.1371/journal.pone.0203809
Kang, K., Kim, Y.-S., Park, S., Back, K. (2009). Senescence-induced serotonin biosynthesis and its role in delaying senescence in rice leaves. Plant Physiol. 150, 1380–1393. doi: 10.1104/pp.109.138552
Khodaeiaminjan, M., Knoch, D., Ndella Thiaw, M. R., Marchetti, C. F., Kořínková, N., Techer, A., et al. (2023). Genome-wide association study in two-row spring barley landraces identifies QTL associated with plantlets root system architecture traits in well-watered and osmotic stress conditions. Front. Plant Sci. 14. doi: 10.3389/fpls.2023.1125672
Kim, W., Iizumi, T., Nishimori, M. (2019). Global patterns of crop production losses associated with droughts from 1983 to 2009. J. Appl. Meteorol. Climatol. 58, 1233–1244. doi: 10.1175/JAMC-D-18-0174.1
Kim, Y. Y., Jung, K. W., Yoo, K. S., Jeung, J. U., Shin, J. S. (2011). A stress-responsive caleosin-like protein, AtCLO4, acts as a negative regulator of ABA responses in arabidopsis. Plant Cell Physiol. 52, 874–884. doi: 10.1093/pcp/pcr039
Komiya, R., Ikegami, A., Tamaki, S., Yokoi, S., Shimamoto, K. (2008). Hd3a and RFT1 are essential for flowering in rice. Development 135, 767–774. doi: 10.1242/dev.008631
Komiya, R., Yokoi, S., Shimamoto, K. (2009). A gene network for long-day flowering activates RFT1 encoding a mobile flowering signal in rice. Development 136, 3443–3450. doi: 10.1242/dev.040170
Kosová, K., Vítámvás, P., Prášil, I. T. (2014). Wheat and barley dehydrins under cold, drought, and salinity - what can LEA-II proteins tell us about plant stress response? Front. Plant Sci. 5. doi: 10.3389/fpls.2014.00343
Kumar, K. P., Binodh, A. K., Saravanan, S., Senthil, A., Kumar, N. S. (2019). Rapid screening for drought tolerance in traditional landraces of rice (Oryza sativa l.) at seedling stage under hydroponics. Electron. J. Plant Breed. 10, 636. doi: 10.5958/0975-928x.2019.00080.2
Lan, Y., Chawade, A., Kuktaite, R., Johansson, E. (2022). Climate change impact on wheat performance–effects on vigour, plant traits and yield from early and late drought stress in diverse lines. Int. J. Mol. Sci. 23, 3333. doi: 10.3390/ijms23063333
Langfelder, P., Horvath, S. (2008). WGCNA: an r package for weighted correlation network analysis. BMC Bioinf. 9, 559. doi: 10.1186/1471-2105-9-559
Langfelder, P., Horvath, S. (2012). Fast r functions for robust correlations and hierarchical clustering. J. Stat. Software 46, 1–17. doi: 10.18637/jss.v046.i11
Lee, J. W., Lee, S. H., Han, J. W., Kim, G. H. (2020). Early light-inducible protein (ELIP) can enhance resistance to cold-induced photooxidative stress in chlamydomonas reinhardtii. Front. Physiol. 11, 1083. doi: 10.3389/fphys.2020.01083
Liang, Y., Tabien, R. E., Tarpley, L., Mohammed, A. R., Septiningsih, E. M. (2021). Transcriptome profiling of two rice genotypes under mild field drought stress during grain-filling stage. AoB Plants 13, lab043. doi: 10.1093/aobpla/plab043
Lin, Y., Yi, X., Tang, S., Chen, W., Wu, F., Yang, X., et al. (2019). Dissection of phenotypic and genetic variation of drought-related traits in diverse Chinese wheat landraces. Plant Genome 12, 1–14. doi: 10.3835/plantgenome2019.03.0025
Liu, H., Xing, M., Yang, W., Mu, X., Wang, X., Lu, F., et al. (2019). Genome-wide identification of and functional insights into the late embryogenesis abundant (LEA) gene family in bread wheat (Triticum aestivum). Sci. Rep. 9, 13375. doi: 10.1038/s41598-019-49759-w
Liu, J., Yang, H., Lu, Q., Wen, X., Chen, F., Peng, L., et al. (2012). PsbP-domain protein1, a nuclear-encoded thylakoid lumenal protein, is essential for photosystem I assembly in arabidopsis. Plant Cell 24, 4992–5006. doi: 10.1105/tpc.112.106542
Love, M. I., Huber, W., Anders, S. (2014). Moderated estimation of fold change and dispersion for RNA-seq data with DESeq2. Genome Biol. 15, 550. doi: 10.1186/s13059-014-0550-8
Lv, L., Dong, C., Liu, Y., Zhao, A., Zhang, Y., Li, H., et al. (2022). Transcription-associated metabolomic profiling reveals the critical role of frost tolerance in wheat. BMC Plant Biol. 22, 333. doi: 10.1186/s12870-022-03718-2
Lv, L., Zhang, W., Sun, L., Zhao, A., Zhang, Y., Wang, L., et al. (2020). Gene co-expression network analysis to identify critical modules and candidate genes of drought-resistance in wheat. PloS One 15, e0236186. doi: 10.1371/journal.pone.0236186
Mahpara, S., Zainab, A., Ullah, R., Kausar, S., Bilal, M., Latif, M. I., et al. (2022). The impact of PEG-induced drought stress on seed germination and seedling growth of different bread wheat (Triticum aestivum l.) genotypes. PloS One 17, e0262937. doi: 10.1371/journal.pone.0262937
Mao, H., Li, S., Wang, Z., Cheng, X., Li, F., Mei, F., et al. (2020). Regulatory changes in TaSNAC8-6A are associated with drought tolerance in wheat seedlings. Plant Biotechnol. J. 18, 1078–1092. doi: 10.1111/pbi.13277
Maurel, C., Verdoucq, L., Luu, D.-T., Santoni, V. (2008). Plant aquaporins: membrane channels with multiple integrated functions. Annu. Rev. Plant Biol. 59, 595–624. doi: 10.1146/annurev.arplant.59.032607.092734
Mishra, S. S., Behera, P. K., Panda, D. (2019). Genotypic variability for drought tolerance-related morpho-physiological traits among indigenous rice landraces of jeypore tract of odisha, India. J. Crop Improv. 33, 254–278. doi: 10.1080/15427528.2019.1579138
Møller, S. G., McPherson, M. J. (1998). Developmental expression and biochemical analysis of the arabidopsis atao1 gene encoding an H2O2-generating diamine oxidase. Plant J. 13, 781–791. doi: 10.1046/j.1365-313x.1998.00080.x
Munasinghe, S. P., Somaratne, S., Weerakoon, S. R. (2017). Screening of Sri Lankan rice (Oryza sativa l.) landraces for drought tolerance. Trop. Agric. Res. 28, 183–191. doi: 10.4038/tar.v28i2.8195
Münch-Garthoff, S., Neuhaus, J. M., Boller, T., Kemmerling, B., Kogel, K. H. (1997). Expression of beta-1,3-glucanase and chitinase in healthy, stem-rust-affected and elicitor-treated near-isogenic wheat lines showing Sr5-or Sr24-specified race-specific rust resistance. Planta 201, 235–244. doi: 10.1007/BF01007709
Naderi, S., Fakheri, B.-A., Maali-Amiri, R., Mahdinezhad, N. (2020). Tolerance responses in wheat landrace bolani are related to enhanced metabolic adjustments under drought stress. Plant Physiol. Biochem. 150, 244–253. doi: 10.1016/j.plaphy.2020.03.002
Nardino, M., Perin, E. C., Aranha, B. C., Carpes, S. T., Fontoura, B. H., de Sousa, D. J. P., et al. (2022). Understanding drought response mechanisms in wheat and multi-trait selection. PloS One 17, e0266368. doi: 10.1371/journal.pone.0266368
NOAA National Centers for Environmental Information (2022a) State of the climate: monthly global climate report for April 2022. Available at: https://www.ncei.noaa.gov/access/monitoring/monthly-report/global/202204 (Accessed October 25, 2022).
NOAA National Centers for Environmental Information (2022b) U.S. drought monitor update for April 12, 2022. Available at: https://www.ncei.noaa.gov/news/us-drought-monitor-update-april-12-2022#:~:text=According%20to%20the%20April%2012,decrease%20from%20last%20week's%2048.0%25 (Accessed October 25, 2022).
Nolan, T., Chen, J., Yin, Y. (2017). Cross-talk of brassinosteroid signaling in controlling growth and stress responses. Biochem. J. 474, 2641–2661. doi: 10.1042/BCJ20160633
Ogiso-Tanaka, E., Matsubara, K., Yamamoto, S.-I., Nonoue, Y., Wu, J., Fujisawa, H., et al. (2013). Natural variation of the RICE FLOWERING LOCUS T 1 contributes to flowering time divergence in rice. PloS One 8, e75959. doi: 10.1371/journal.pone.0075959
Patel, J., Mishra, A. (2021). Plant aquaporins alleviate drought tolerance in plants by modulating cellular biochemistry, root-architecture, and photosynthesis. Physiol. Plant 172, 1030–1044. doi: 10.1111/ppl.13324
Patro, R., Duggal, G., Love, M. I., Irizarry, R. A., Kingsford, C. (2017). Salmon provides fast and bias-aware quantification of transcript expression. Nat. Methods 14, 417–419. doi: 10.1038/nmeth.4197
Pfeifer, M., Kugler, K. G., Sandve, S. R., Zhan, B., Rudi, H., Hvidsten, T. R., et al. (2014). Genome interplay in the grain transcriptome of hexaploid bread wheat. Science 345, 1250091. doi: 10.1126/science.1250091
Pospíšil, P. (2016). Production of reactive oxygen species by photosystem II as a response to light and temperature stress. Front. Plant Sci. 7. doi: 10.3389/fpls.2016.01950
Priest, H. D., Fox, S. E., Rowley, E. R., Murray, J. R., Michael, T. P., Mockler, T. C. (2014). Analysis of global gene expression in brachypodium distachyon reveals extensive network plasticity in response to abiotic stress. PloS One 9, e87499. doi: 10.1371/journal.pone.0087499
Qaseem, M. F., Qureshi, R., Shaheen, H. (2019). Effects of pre-anthesis drought, heat and their combination on the growth, yield and physiology of diverse wheat (Triticum aestivum l.) genotypes varying in sensitivity to heat and drought stress. Sci. Rep. 9, 6955. doi: 10.1038/s41598-019-43477-z
Ray, D. K., Mueller, N. D., West, P. C., Foley, J. A. (2013). Yield trends are insufficient to double global crop production by 2050. PloS One 8, e66428. doi: 10.1371/journal.pone.0066428
R Core Team (2021). R: a language and environment for statistical computing (Vienna, Austria: R Foundation for Statistical Computing). Available at: https://www.R-project.org/.
Reinert, S., Kortz, A., Léon, J., Naz, A. A. (2016). Genome-wide association mapping in the global diversity set reveals new QTL controlling root system and related shoot variation in barley. Front. Plant Sci. 7. doi: 10.3389/fpls.2016.01061
Reynolds, M., Dreccer, F., Trethowan, R. (2007). Drought-adaptive traits derived from wheat wild relatives and landraces. J. Exp. Bot. 58, 177–186. doi: 10.1093/jxb/erl250
Runquist, M., Kruger, N. J. (1999). Control of gluconeogenesis by isocitrate lyase in endosperm of germinating castor bean seedlings. Plant J. 19, 423–431. doi: 10.1046/j.1365-313x.1999.00533.x
Sabouri, A., Dadras, A. R., Azari, M., Saberi Kouchesfahani, A., Taslimi, M., Jalalifar, R. (2022). Screening of rice drought-tolerant lines by introducing a new composite selection index and competitive with multivariate methods. Sci. Rep. 12, 2163. doi: 10.1038/s41598-022-06123-9
Sallam, A., Mourad, A. M. I., Hussain, W., Stephen Baenziger, P. (2018). Genetic variation in drought tolerance at seedling stage and grain yield in low rainfall environments in wheat (Triticum aestivum l.). Euphytica 214, 169. doi: 10.1007/s10681-018-2245-9
Sancho, R., Catalán, P., Contreras-Moreira, B., Juenger, T. E., Des Marais, D. L. (2022). Patterns of pan-genome occupancy and gene coexpression under water-deficit in brachypodium distachyon. Mol. Ecol. 31, 5285–5306. doi: 10.1111/mec.16661
Schmidt, J., Tricker, P. J., Eckermann, P., Kalambettu, P., Garcia, M., Fleury, D. (2019). Novel alleles for combined drought and heat stress tolerance in wheat. Front. Plant Sci. 10. doi: 10.3389/fpls.2019.01800
Senapati, N., Stratonovitch, P., Paul, M. J., Semenov, M. A. (2019). Drought tolerance during reproductive development is important for increasing wheat yield potential under climate change in Europe. J. Exp. Bot. 70, 2549–2560. doi: 10.1093/jxb/ery226
Shannon, P., Markiel, A., Ozier, O., Baliga, N. S., Wang, J. T., Ramage, D., et al. (2003). Cytoscape: a software environment for integrated models of biomolecular interaction networks. Genome Res. 13, 2498–2504. doi: 10.1101/gr.1239303
Sharma, V., Kumar, A., Chaudhary, A., Mishra, A., Rawat, S., B., B. Y., et al. (2022). Response of wheat genotypes to drought stress stimulated by PEG. Stresses 2, 26–51. doi: 10.3390/stresses2010003
Shin, N.-H., Trang, D. T., Hong, W.-J., Kang, K., Chuluuntsetseg, J., Moon, J.-K., et al. (2019). Rice senescence-induced receptor-like kinase (OsSRLK) is involved in phytohormone-mediated chlorophyll degradation. Int. J. Mol. Sci. 21, 260. doi: 10.3390/ijms21010260
Singh, A., Desgagné-Penix, I. (2017). Transcriptome and metabolome profiling of narcissus pseudonarcissus “King alfred” reveal components of amaryllidaceae alkaloid metabolism. Sci. Rep. 7, 17356. doi: 10.1038/s41598-017-17724-0
Sirohi, P., Yadav, B. S., Afzal, S., Mani, A., Singh, N. K. (2020). Identification of drought stress-responsive genes in rice (Oryza sativa) by meta-analysis of microarray data. J. Genet. 99, 35. doi: 10.1007/s12041-020-01195-w
Smita, S., Katiyar, A., Pandey, D. M., Chinnusamy, V., Archak, S., Bansal, K. C. (2013). Identification of conserved drought stress responsive gene-network across tissues and developmental stages in rice. Bioinformation 9, 72–78. doi: 10.6026/97320630009072
Soneson, C., Love, M. I., Robinson, M. D. (2015). Differential analyses for RNA-seq: transcript-level estimates improve gene-level inferences. F1000Res. 4, 1521. doi: 10.12688/f1000research.7563.1
Song, Y., Miao, Y., Song, C.-P. (2014). Behind the scenes: the roles of reactive oxygen species in guard cells. New Phytol. 201, 1121–1140. doi: 10.1111/nph.12565
Stephens, M. (2017). False discovery rates: a new deal. Biostatistics 18, 275–294. doi: 10.1093/biostatistics/kxw041
Sun, Z., Li, S., Chen, W., Zhang, J., Zhang, L., Sun, W., et al. (2021). Plant dehydrins: expression, regulatory networks, and protective roles in plants challenged by abiotic stress. Int. J. Mol. Sci. 22, 12619. doi: 10.3390/ijms222312619
Tardy, F., Créach, A., Havaux, M. (1998). Photosynthetic pigment concentration, organization and interconversions in a pale green Syrian landrace of barley (Hordeum vulgareL., tadmor) adapted to harsh climatic conditions. Plant Cell Environ. 21, 479–489. doi: 10.1046/j.1365-3040.1998.00293.x
Tehseen, M. M., Tonk, F. A., Tosun, M., Istipliler, D., Amri, A., Sansaloni, C. P., et al. (2022). Exploring the genetic diversity and population structure of wheat landrace population conserved at ICARDA genebank. Front. Genet. 13. doi: 10.3389/fgene.2022.900572
Tian, T., Liu, Y., Yan, H., You, Q., Yi, X., Du, Z., et al. (2017). agriGO v2.0: a GO analysis toolkit for the agricultural community 2017 Update. Nucleic Acids Res. 45, W122–W129. doi: 10.1093/nar/gkx382
Tilman, D., Balzer, C., Hill, J., Befort, B. L. (2011). Global food demand and the sustainable intensification of agriculture. Proc. Natl. Acad. Sci. U. S. A. 108, 20260–20264. doi: 10.1073/pnas.1116437108
Tiwari, P., Chakrabarty, D. (2021). Dehydrin in the past four decades: from chaperones to transcription co-regulators in regulating abiotic stress response. Curr. Res. Biotechnol. 3, 249–259. doi: 10.1016/j.crbiot.2021.07.005
Tiwari, P., Indoliya, Y., Singh, P. K., Singh, P. C., Chauhan, P. S., Pande, V., et al. (2019). Role of dehydrin-FK506-binding protein complex in enhancing drought tolerance through the ABA-mediated signaling pathway. Environ. Exp. Bot. 158, 136–149. doi: 10.1016/j.envexpbot.2018.10.031
Trnka, M., Feng, S., Semenov, M. A., Olesen, J. E., Kersebaum, K. C., Rötter, R. P., et al. (2019). Mitigation efforts will not fully alleviate the increase in water scarcity occurrence probability in wheat-producing areas. Sci. Adv. 5, eaau2406. doi: 10.1126/sciadv.aau2406
Van Oosterom, E. J., Ceccarelli, S., Peacock, J. M. (1993). Yield response of barley to rainfall and temperature in Mediterranean environments. J. Agric. Sci. 121, 307–313. doi: 10.1017/S0021859600085488
Vuković, R., Čamagajevac, I.Š., Vuković, A., Šunić, K., Begović, L., Mlinarić, S., et al. (2022). Physiological, biochemical and molecular response of different winter wheat varieties under drought stress at germination and seedling growth stage. Antioxidants (Basel) 11, 693. doi: 10.3390/antiox11040693
Wan, C., Dang, P., Gao, L., Wang, J., Tao, J., Qin, X., et al. (2022). How does the environment affect wheat yield and protein content response to drought? a meta-analysis. Front. Plant Sci. 13. doi: 10.3389/fpls.2022.896985
Wang, X., Li, Q., Xie, J., Huang, M., Cai, J., Zhou, Q., et al. (2021). Abscisic acid and jasmonic acid are involved in drought priming-induced tolerance to drought in wheat. Crop J. 9, 120–132. doi: 10.1016/j.cj.2020.06.002
Wang, Y., Xu, H., Zhu, H., Tao, Y., Zhang, G., Zhang, L., et al. (2014). Classification and expression diversification of wheat dehydrin genes. Plant Sci. 214, 113–120. doi: 10.1016/j.plantsci.2013.10.005
Wäspi, U., Misteli, B., Hasslacher, M., Jandrositz, A., Kohlwein, S. D., Schwab, H., et al. (1998). The defense-related rice gene Pir7b encodes an alpha/beta hydrolase fold protein exhibiting esterase activity towards naphthol AS-esters. Eur. J. Biochem. 254, 32–37. doi: 10.1046/j.1432-1327.1998.2540032.x
Wasternack, C. (2014). Action of jasmonates in plant stress responses and development–applied aspects. Biotechnol. Adv. 32, 31–39. doi: 10.1016/j.biotechadv.2013.09.009
Werner, T., Motyka, V., Laucou, V., Smets, R., Van Onckelen, H., Schmülling, T. (2003). Cytokinin-deficient transgenic arabidopsis plants show multiple developmental alterations indicating opposite functions of cytokinins in the regulation of shoot and root meristem activity. Plant Cell 15, 2532–2550. doi: 10.1105/tpc.014928
Werner, T., Nehnevajova, E., Köllmer, I., Novák, O., Strnad, M., Krämer, U., et al. (2010). Root-specific reduction of cytokinin causes enhanced root growth, drought tolerance, and leaf mineral enrichment in arabidopsis and tobacco. Plant Cell 22, 3905–3920. doi: 10.1105/tpc.109.072694
Wickham, H. (2009). ggplot2: elegant graphics for data analysis (New York, USA: Springer Science & Business Media). Available at: https://play.google.com/store/books/details?id=bes-AAAAQBAJ.
Xiao, C., Guo, H., Tang, J., Li, J., Yao, X., Hu, H. (2021). Expression pattern and functional analyses of arabidopsis guard cell-enriched GDSL lipases. Front. Plant Sci. 12. doi: 10.3389/fpls.2021.748543
Xu, W., Lv, H., Zhao, M., Li, Y., Qi, Y., Peng, Z., et al. (2016). Proteomic comparison reveals the contribution of chloroplast to salt tolerance of a wheat introgression line. Sci. Rep. 6, 32384. doi: 10.1038/srep32384
Yates, A. D., Allen, J., Amode, R. M., Azov, A. G., Barba, M., Becerra, A., et al. (2022). Ensembl genomes 2022: an expanding genome resource for non-vertebrates. Nucleic Acids Res. 50, D996–D1003. doi: 10.1093/nar/gkab1007
Ye, C., Zheng, S., Jiang, D., Lu, J., Huang, Z., Liu, Z., et al. (2021). Initiation and execution of programmed cell death and regulation of reactive oxygen species in plants. Int. J. Mol. Sci. 22, 12942. doi: 10.3390/ijms222312942
Yu, S., Liao, F., Wang, F., Wen, W., Li, J., Mei, H., et al. (2012). Identification of rice transcription factors associated with drought tolerance using the ecotilling method. PloS One 7, e30765. doi: 10.1371/journal.pone.0030765
Yu, B., Liu, J., Wu, D., Liu, Y., Cen, W., Wang, S., et al. (2020b). Weighted gene coexpression network analysis-based identification of key modules and hub genes associated with drought sensitivity in rice. BMC Plant Biol. 20, 478. doi: 10.1186/s12870-020-02705-9
Yu, A., Xie, Y., Pan, X., Zhang, H., Cao, P., Su, X., et al. (2020a). Photosynthetic phosphoribulokinase structures: enzymatic mechanisms and the redox regulation of the Calvin-Benson-Bassham cycle. Plant Cell 32, 1556–1573. doi: 10.1105/tpc.19.00642
Yuenyong, W., Sirikantaramas, S., Qu, L.-J., Buaboocha, T. (2019). Isocitrate lyase plays important roles in plant salt tolerance. BMC Plant Biol. 19, 472. doi: 10.1186/s12870-019-2086-2
Zadoks, J. C., Chang, T. T., Konzak, C. F. (1974). A decimal code for the growth stages of cereals. Weed Res. 14, 415–421. doi: 10.1111/j.1365-3180.1974.tb01084.x
Zeven, A. C. (1998). Landraces: a review of definitions and classifications. Euphytica 104, 127–139. doi: 10.1023/A:1018683119237
Zhang, J., Zhang, S., Cheng, M., Jiang, H., Zhang, X., Peng, C., et al. (2018). Effect of drought on agronomic traits of rice and wheat: a meta-analysis. Int. J. Environ. Res. Public Health 15, 839. doi: 10.3390/ijerph15050839
Keywords: drought, transcriptomics, network analysis, triticum aestivum (bread wheat), landrace, hub gene
Citation: Barratt LJ, Reynolds IJ, Franco Ortega S and Harper AL (2023) Transcriptomic and co-expression network analyses on diverse wheat landraces identifies candidate master regulators of the response to early drought. Front. Plant Sci. 14:1212559. doi: 10.3389/fpls.2023.1212559
Received: 26 April 2023; Accepted: 06 June 2023;
Published: 23 June 2023.
Edited by:
Baris Uzilday, Ege University, TürkiyeReviewed by:
Bruno Contreras-Moreira, Spanish National Research Council (CSIC), SpainCopyright © 2023 Barratt, Reynolds, Franco Ortega and Harper. This is an open-access article distributed under the terms of the Creative Commons Attribution License (CC BY). The use, distribution or reproduction in other forums is permitted, provided the original author(s) and the copyright owner(s) are credited and that the original publication in this journal is cited, in accordance with accepted academic practice. No use, distribution or reproduction is permitted which does not comply with these terms.
*Correspondence: Andrea L. Harper, YW5kcmVhLmhhcnBlckB5b3JrLmFjLnVr
†These authors share first authorship
Disclaimer: All claims expressed in this article are solely those of the authors and do not necessarily represent those of their affiliated organizations, or those of the publisher, the editors and the reviewers. Any product that may be evaluated in this article or claim that may be made by its manufacturer is not guaranteed or endorsed by the publisher.
Research integrity at Frontiers
Learn more about the work of our research integrity team to safeguard the quality of each article we publish.