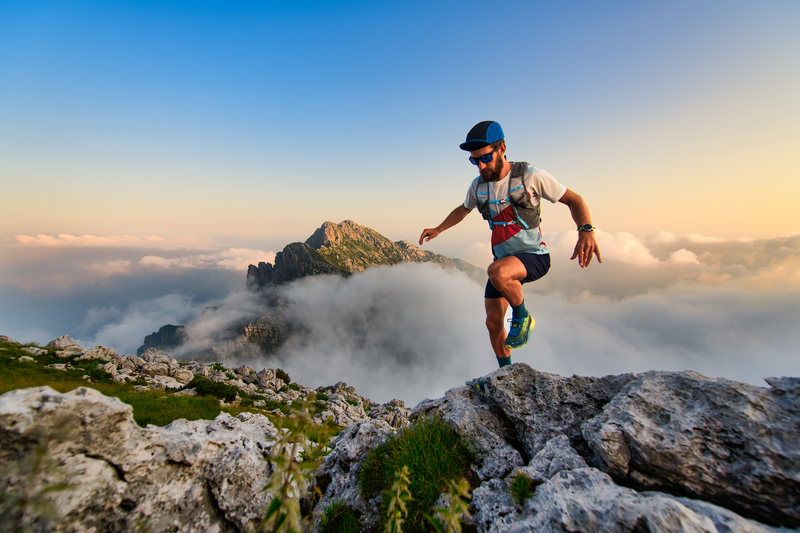
94% of researchers rate our articles as excellent or good
Learn more about the work of our research integrity team to safeguard the quality of each article we publish.
Find out more
REVIEW article
Front. Plant Sci. , 10 May 2023
Sec. Plant Breeding
Volume 14 - 2023 | https://doi.org/10.3389/fpls.2023.1163785
This article is part of the Research Topic Plant Genetic and Genomic Resources for Sustained Crop Improvement View all 9 articles
Parts of this article's content have been modified or rectified in:
Erratum: Genetic resources and breeding of maize for Striga resistance: a review
The potential yield of maize (Zea mays L.) and other major crops is curtailed by several biotic, abiotic, and socio-economic constraints. Parasitic weeds, Striga spp., are major constraints to cereal and legume crop production in sub-Saharan Africa (SSA). Yield losses reaching 100% are reported in maize under severe Striga infestation. Breeding for Striga resistance has been shown to be the most economical, feasible, and sustainable approach for resource-poor farmers and for being environmentally friendly. Knowledge of the genetic and genomic resources and components of Striga resistance is vital to guide genetic analysis and precision breeding of maize varieties with desirable product profiles under Striga infestation. This review aims to present the genetic and genomic resources, research progress, and opportunities in the genetic analysis of Striga resistance and yield components in maize for breeding. The paper outlines the vital genetic resources of maize for Striga resistance, including landraces, wild relatives, mutants, and synthetic varieties, followed by breeding technologies and genomic resources. Integrating conventional breeding, mutation breeding, and genomic-assisted breeding [i.e., marker-assisted selection, quantitative trait loci (QTL) analysis, next-generation sequencing, and genome editing] will enhance genetic gains in Striga resistance breeding programs. This review may guide new variety designs for Striga-resistance and desirable product profiles in maize.
Maize (Zea mays L., 2n = 2x = 20) is a staple cereal crop after rice and wheat worldwide, accounting for 30–70% of the total caloric consumption (Kamara et al., 2005; Shiferaw et al., 2011; Yacoubou et al., 2021b). The annual global production of maize was estimated at 1,210,235,135.14 tons in 2021 (FAOSTAT, 2022). The main maize producers are the United States of America (592,356,330.09 tons), China (272,762,124 tons), Brazil (88,461,943 tons), Argentina (60,525,80 tons), Ukraine (42,109,850 tons), India (31,650,000 tons), and Mexico (27,503,477.82 tons), accounting for about 66% of the global production per annum (FAOSTAT, 2022). In Africa, annual maize production was estimated at 96,637,314.23 million tons in 2021, representing 7.98% of the world’s production (FAOSTAT, 2022). South Africa is the largest maize producer in Africa, with an estimated annual production of 16,870,705 million tons, followed by Nigeria (12,745,000 tons), Ethiopia (10,722,000 tons), Egypt (7,500,000 tons), and Kenya (3,303,000 tons) (FAO, 2022).
In sub-Saharan Africa (SSA), 14 countries have the highest per capita consumption of maize (Ranum et al., 2014). For instance, in Benin, the mean per capita consumption of maize per annum is 85 kg (Hongbete et al., 2017). Maize is produced in all agroecological zones in Africa on smallholder landholding varying between 0.5 and 2 hectares (ha) (Achigan-Dako et al., 2014). SSA has the lowest maize yields globally, estimated at 3 tons/ha (FAO, 2022), compared with global mean yields of 5 to 10 tons ha-1 (FAO, 2022). The low productivity of maize and major crops in SSA is attributable to an array of production constraints, including biotic factors (e.g., parasitic and competitive weeds, field and storage insect pests, and pre- and post-harvest diseases) (Cairns et al., 2013; Rachidatou et al., 2018; Yacoubou et al., 2021a), and abiotic factors including heat and drought stresses (Cairns et al., 2013; Ali et al., 2015). Heat and drought stress, coupled with low soil fertility, are some of the common abiotic challenges affecting maize productivity throughout the region (Badu-Apraku and Fakorede, 2017; Lobulu et al., 2019), predisposing major cereal and legume crops to parasitic weeds of the genus Striga.
Forty Striga species have been reported worldwide (Gethi and Smith, 2004; Reda and Verkleij, 2004). Among these, 33 species have been reported in Africa and 11 affect major grain crops (Gethi and Smith, 2004; Ejeta et al., 2007). Striga hermonthica (Del.) Benth and Striga asiatica (L.) Kuntze are the most economically important in cereal production systems. Striga hermonthica is present in most SSA regions, affecting Western, Central, and Eastern Africa (Figure 1A, C, and D), while S. asiatica (Figure 1B) is predominant in Southern Africa (Ejeta, 2007; Parker, 2012; Shayanowako et al., 2018a).
Figure 1 Striga hermonthica infested-maize in Benin (A), sorghum in Ethiopia (B), pearl millet in Burkina-Faso (C), and S. asiatica infested maize in Tanzania (D). (Photo A supplied by Dr. Fen Beed, B by H Shimelis, C by S Al-Babili, and D by E Mrema).
Unlike sorghum, which has co-evolved with Striga weed in Africa, maize is exceptionally susceptible to the parasite, particularly in marginal and low input production environments (Adewale et al., 2020; Badu-Apraku et al., 2020a; Shayanowako et al., 2020). Maize yield losses exceeding 60% are common under Striga infestation (Jamil et al., 2012; Lobulu et al., 2019). Global Striga-infested area in SSA is estimated to be 2.4 million ha leading to a yield loss of 1.6 tons per year valued at US$ 383 million (Woomer et al., 2008). Methods to control Striga include cultural practices, chemical herbicides, and biological agents. However, these are often unavailable or inadequate (Mrema et al., 2017), and farmers often resort to hand hoeing, which is ineffective because most of the damage to the maize plant occurs before the parasitic plants emerge (Ejeta, 2007; Stanley et al., 2021). There is little use of selective herbicides in the region because of the high cost and the complex cropping systems (e.g., intercropping cereals and legumes) (Hearne, 2009). The use of host resistance against Striga parasitism is widely favored because of its cost effectiveness, safety, and practicality, making it readily deployable in low-input maize production environments (Badu-Apraku et al., 2016; Adu et al., 2019). Breeders strive to develop maize genotypes that are either (i) resistant, inhibiting or allowing few Striga seeds to germinate and attach onto the host roots through low production of strigolactones (SL), root barriers for incompatibility, and hypersensitive and antibiosis reactions toxic to the parasite, or (ii) tolerant by being insensitive to high levels of parasitic attachments with minimum yield loss.
Presently, three component traits are used to rate the performance of a genotype under Striga infestation: (1) Striga emergence count, (2) Striga damage rating, and (3) crop grain yield (GY) performance. Striga emergence count determines the extent of suppression of Striga attachments, which reflects active host resistance. Striga damage ratings and GY response of the host are measures of tolerance used to rate the degree to which a maize plant can withstand the phytotoxic effects of Striga among genotypes that support many parasitic attachments. Ideally, Striga-resistant maize would be attractive in that it would also cause a reduction in the Striga seed bank compared with tolerance (Rodenburg and Bastiaans, 2011). However, Striga resistance has quantitative inheritance and is controlled by multiple minor genes with additive gene action. Durable resistance is yet to be reported in maize. Highly tolerant genotypes allow parasitic attachments of Striga plants resulting in the seed set and dispersal of the weed (Yacoubou et al., 2021b). Furthermore, resistance genes may be effective only in a specific region or agroecologies due to genotype by environment interaction effect (Oswald and Ransom, 2002).
Hindrances to developing maize cultivars with durable resistance include several aspects, such as a focus on a narrow spectrum of resistance traits during breeding. For example, most of the previous breeding programs involving field and control environment screening did not consider the SL biosynthesis effect, which is the basis of Striga-resistant traits. It has been reported that SL analogs induce suicidal germination of Striga spp. seed in soil (Kountche et al., 2019). Jamil et al. (2022) evaluated the efficacy of three potent SL analogs under laboratory, greenhouse, and farmers’ field conditions. The authors confirmed that the selected SL analogs appear to make promising candidates under field conditions that led to 43 and 60% reduction of Striga emergence in pearl millet and sorghum, respectively. Li et al. (2023) identified SL, zealactol, and zealactonoic acid compounds. The reported components stimulate less Striga germination than the earlier reported SL and zealactone from maize. The authors demonstrated that maize genotypes primarily producing zealactol suffered less Striga damage than genotypes with higher zealactone content. Genes controlling Striga resistance might range from major genes, that is, those responsible for the production of SL (Lopez-Raez et al., 2009) to quantitative traits loci (QTLs) influencing the expression of resistance in the field (Amusan, 2010; Badu-Apraku et al., 2020a). Field resistance to Striga determines the ultimate purpose of varietal improvement.
The application of modern genomic tools has been effective in dissecting complex traits conditioning biotic and abiotic tolerance (Gedil and Menkir, 2019). Hence, developing and applying genomic tools for Striga resistance will be critical in accelerating breeding for Striga resistance. Genomic-assisted breeding complements the traditional approaches in integrating resistance to Striga and other quantitatively inherited traits of importance to food security (Michel et al., 2017). Striga tolerance is highly effective in maize, while it may not provide complete protection due to partial resistance (Shayanowako et al., 2018b). Additionally, environmental factors such as poor soil fertility, erratic rainfall, and high temperatures favor the high fecundity of Striga (Chidiebere et al., 2015). Hence, there is a need to integrate host tolerance and other Striga management methods to reduce the damage caused by the parasite.
The development of Striga-resistant and market-preferred maize cultivars is among the primary goals of public and private maize breeders. The design of maize ideotypes for Striga resistance/tolerance and desirable product profiles depends on integrating multiple winning and essential traits based on host-parasite interaction. Hence, exploiting the genetic variation and defense mechanisms present in the host against the parasites may accelerate the adoption of novel breeding approaches in maize. In light of the above background, this review aims to present the genetic and genomic resources, research progress, and opportunities in the genetic analysis of Striga resistance and yield components in maize. Information presented in this paper may guide new variety designs in maize for Striga-resistance and desirable product profiles.
A successful genetic improvement for Striga resistance depends on the host’s natural or induced genetic variation. Several genetic sources of Striga resistance have been reported in maize (Gurney et al., 2002; Rich and Ejeta, 2008) and other cereal crops, including sorghum (Gurney et al., 2002; Rich et al., 2004), rice, and pearl millet (Wilson et al., 2000). Table 1 presents some of their genetic sources with their unique resistant traits. The summary presents variability of Striga resistance sources, including landraces, cultivars, wild relatives, breeding lines, and single cross hybrids for each of the crops. It also portrays Striga resistance traits such as a low haustorium initiation, Striga germination, Striga attachment, a hypersensitive reaction by the host, and parasitic mortality.
Table 2 presents gene banks and databases of maize genotypes with Striga resistance. Striga-resistant varieties are curated at International Institute of Tropical Agriculture (IITA/)Nigeria, CIMMYT/Zimbabwe, KARI/Kenya, NARLWEYO Seed company/Uganda, and Meru Agro seed company/Tanzania. These gene banks and databases serve to acquire new plant materials for Striga resistance breeding and genetic conservation for medium and long-term use (Smale and Jamora, 2020).
Several Striga resistance mechanisms are reported. The mechanisms act either before (pre-attachment) or after physical contact with the host (post-attachment). In the case of pre-attachment resistance, the host produces low levels of SL, below which the Striga receptors perceive the germination stimulants insensitive to SL, inducing less parasitic germination (Ejeta and Gressel, 2007).
Host resistance has been identified in an opened-pollinated maize variety (OPV) KSTP 94 in western Kenya, where S. hermonthica is dominant (Yoneyama et al., 2015). Post-attachment resistance occurs after Striga attachment to the host. Maize inbred line ZD05 and an open-pollinated variety KSTP 94, are some of the sources of resistance identified. ZD05 exhibits low levels of Striga attachment and high mortality of attached parasites compared with the susceptible inbred line 5057 (Amusan et al., 2008). KSTP 94 develops low numbers of Striga attachments and biomass compared with the susceptible inbred line CML 144 (Mutinda et al., 2018). The two maize varieties have different resistance mechanisms, which facilitate the introgression of Striga resistance genes into farmer-preferred and susceptible germplasm.
The genetic variation between germplasm resources within plant species is useful for the development of improved varieties with desirable traits, including Striga resistance (Holme et al., 2019; Latpate, 2019). This enables variety design with market-preferred traits, resistance/tolerance against biotic and abiotic factors, and adaptation to diverse agro-ecological zones.
Table 3 presents some modern maize genotypes reported to be Striga resistant/tolerant and possessing good agronomic traits. Lower Striga damage rating and lower Striga emergence count are the main components of Striga resistance in host crops. Genetic variation existing in gene pools may occur naturally in landraces, elite varieties, cultivated and obsolete cultivars, or artificially created through mutation breeding or genetic engineering (Hawkes, 1991). Major sources of Striga resistance gene in maize have been derived from wild relatives, landraces, synthetics, composites, and elite breeding lines (Taba, 1995; Sachs, 2009) as described in the following section. The novel genetic sources are ideal for use in pre-breeding and breeding programs for improving Striga resistance and agronomic traits.
Landraces are dynamic populations of cultivated crop species with a historical origin and distinct identity, often genetically diverse, locally adapted, and derived through a set of farmers’ practices and knowledge of seed selection and field management (Azeez et al., 2018). Landraces or farmers’ varieties serve as genetic resources that can provide essential characteristics such as resistance to diseases and pests, grain quality, and contribute to extending the genetic base of modern cultivars. The International Plant Genetic Resources Institute (IPGRI) maize landraces represent a genetic reservoir for yield improvement in maize (wumasi et al., 2017). The 196 maize landraces reported by Nelimor et al. (2020) represent gene pools from Burkina-Faso, Ghana, and Togo, for specific agronomic traits including GY, stay green trait, number of ear per plant, ear length, and number of rows per ear. Other maize landraces were previously reported by Meseka et al. (2013) from the northern Guinea savanna and Sudan savanna in West and Central Africa and have shown some drought-adaptive traits.
Some maize landraces reported with Striga resistance traits are presented in Table 1. Despite the paucity of information on Striga resistance genes in landraces, genotypes with marked tolerance have been identified (Kim et al., 1999). In a study by Karaya et al. (2012), 420 landraces triggered a lower level of Striga germination than commercial checks. The genetic composition of these maize landraces is yet to be confirmed. Genes causing lower levels of S. hermonthica emergence in both pot and field trials have been reported in Kenya (Midega et al., 2016). Germplasms with various levels of Striga resistance were reported in Western and Eastern Africa (Amusan et al., 2008; Rich and Ejeta, 2008; Badu-Apraku and Yallou, 2009; Mutinda et al., 2018).
Significant progress has been achieved in identifying maize genotypes with Striga resistance. Synthetic varieties have greatly improved breeding for Striga resistance in maize. Synthetic varieties are random mating populations produced by crossing a group of inbreds with superior general combining ability (Osei et al., 2014). Their prime advantage is that farmers can save their seeds for the next crop cycle. Reportedly, the synthetic population developed by the IITA from 1994 to 1998 with various Striga resistance levels is a major source of Striga resistance in maize (Kim et al., 1998). The IITA-West and Central Africa programs developed other sources of resistant maize germplasm through the Collaborative Maize Research Network (WECAMAN) (Badu-Apraku et al., 2007b). The germplasm has been used as a source of resistance genes in developing maize genotypes such as 2008 TZEE-W STR, 2009 TZEE-OR2 STR, FERKE TZEE-W STR, TZEE-W DT C0 STR C5, 2012 TZEE-W DT STR C5, and 2008 SYN EE-W DT STR, which produces a high GY under Striga infestation (Badu-Apraku et al., 2010b; Badu-Apraku et al., 2016; Oluwaranti et al., 2020). The established resistant genotypes can serve as checks in breeding programs for Striga resistance assessment (Badu-Apraku et al., 2008; Menkir et al., 2010). Genetically diverse S. hermonthica-resistant inbred lines have been developed by the IITA through trait introgression from resistant germplasm, including some of the synthetics (Gasura et al., 2019).
Other important sources of Striga resistance are composites, which are varieties developed by mixing the seeds of phenotypically promising lines. Composites are maintained through open pollination. Open-pollinated varieties (OPVs) are developed through random pollination and seeds from the best-looking individuals are harvested from a heterogeneous population. Kim (1996) reported that composites developed by IITA in 1994 provided a broad genetic pool for resistance breeding against S. hermonthica. These composites varieties has been successfully introduced, leading to the development of resistant inbred lines, hybrids, and synthetics (Badu-Apraku et al., 2006; Badu-Apraku et al., 2009; Gasura et al., 2021). Furthermore, OPVs, synthetics, single-cross hybrids, and composites from KARI (now KALRO)/Kenya, and the National Crops Resources Research Institute/Uganda (Table 3) are important sources of Striga resistance.
Induced mutagenesis causes a target organism’s deoxyribonucleic acid (DNA) to change, resulting in one or more gene mutations. This will enable the creation of new valuable traits that can be introgressed in well-adapted cultivars (Kozjak and Meglič, 2012). Mutational events can be permanent and heritable genetic changes resulting in phenotypic variation (Ahloowalia and Maluszynski, 2001). However, spontaneous mutation rates in higher plants are low at 10−6 to 10−7 per locus (Kovalchuk et al., 2000; Jiang and Ramachandran, 2010). Thus induced mutagenesis is an important strategy to increase mutation frequencies (Maluszynski et al., 2000). Mutagenesis has already been used to improve many useful traits in plants (Maluszynski et al., 2000; Wanga et al., 2020).
Selection of mutants for Striga resistance has been reported in sorghum. The inheritance of low Striga germination stimulant activity was conditioned through a mutant allele expressed in homozygous recessive individuals (Gobena et al., 2017). However, seed mutagenesis of maize using acetolactate synthase inhibiting herbicide (imazapyr), dressed as a drench or as a coating led to the development of herbicide-resistance (Kanampiu et al., 2001; Dan et al., 2017). This enabled an effective, inexpensive, and productive measure to control Striga, with immediate benefit to farmers (Paul and Canon, 2008; Babatima et al., 2011). Kiruki et al. (2006) reported that azide-based mutagenesis rendered new mutants that suppressed S. hermonthica seed germination and parasitism in maize varieties leading to the conversion of Striga-susceptible to Striga-resistant maize mutants. These findings confirm that, although natural mutations are known to induce resistance to Striga, the process can also be accelerated in the laboratory using azide-based mutagenesis (Kiruki et al., 2006).
A major limiting factor in breeding maize for yield and yield components and resistance to parasitic weeds, insect pests, and diseases is the narrow base of the genetic diversity of domesticated maize varieties. Wild relatives of maize and their progenitors are reportedly major sources of Striga resistance. Teosinte (Zea diploperennis), and eastern gamagrass (Tripsacum dactyloides L.) have been used in breeding programs as sources of Striga resistance (Abdoul-Raouf et al., 2017). The inbred line ZD05 selected for its field resistance to S. hermonthica acquired genes from Z. diploperennis (Amusan et al., 2008). This breeding line has been useful in developing new ideotypes with economic traits and Striga resistance by the IITA/Nigeria in collaboration with the National Agricultural Research System (Diskin et al., 2008). Gurney et al. (2003) evaluated the susceptibility of T. dactyloides and a hybrid from T. dactyloides and Z. mays. The authors found that T. dactyloides produces a signal that inhibits S. hermonthica haustorial development. This trait could be introgressed into elite maize genotypes for their resistance to Striga.
Figure 2 presents maize’s wild relatives and the gene pool classified into three groups: primary gene pool, secondary gene pool, and tertiary gene pool, each with different wild genotypes. The primary gene pool includes Zea huehuetenangensis, Z. mexicana, and Z.parviglumis, while the secondary gene pool are Z. diploperennis, Z. luxurians, Z. nicaraguensis, and Z. perennis. The tertiary gene pool consists of all the species of the genus Tripsacum. These show that there is still more useful genetic diversity available in wild species that could enhance Striga resistance in modern maize (Wani et al., 2022). These gene pools could be utilized in modern maize breeding for Striga resistance through detecting and transferring useful alleles using hybridization and genetic engineering approaches.
Figure 2 Gene pool classification of maize’s wild relatives. Adapted from Wani et al., 2022.
Table 4 presents the rates of yield gains realized in Striga resistance breeding programs in maize. Information on the genetic progress in crop traits and yield improvement is helpful for direct production or trait integration. Also, genetic modifications and the use of breeding methodologies and strategies are dependent on yield gains (Badu-Apraku et al., 2013). The IITA has recorded a significant genetic gain in maize through released varieties possessing Striga resistance (Kamara et al., 2005; Badu-Apraku et al., 2014; Badu-Apraku et al., 2016). GY of the Striga-resistant and -tolerant maize varieties under Striga infestation enabled measuring the breeding progress (Badu-Apraku et al., 2013; Ifie et al., 2015). The increase in the genetic gain confirms the substantial progress in breeding for high-yielding and Striga resistance.
Table 4 Rates of yield gains realized in Striga resistance in maize breeding program under Striga-infested environments.
Plant breeding aims to develop genetically improved crop cultivars with economic benefits for small-scale and commercial farmers (Shimelis and Laing, 2012). This involves creating or expanding new genetic variations and selecting and fixing desirable genotypes in the progeny (Kumar and Shukla, 2014). The desired variation may be found in existing germplasms and artificially created through controlled crosses, induced mutations, and polyploidization. Appropriate methods and genetic analysis tools are required to detect and select the progeny that contains the target genotype to accomplish set breeding goals. Conventional, demand-led, and modern plant breeding methods are recent technologies used for trait introgression (Joshi, 2017). The development of new varieties through conventional breeding can take more than 10 years. However, integrating modern plant breeding methods [e.g., marker-assisted selection (MAS), quantitative trait loci (QTL) analysis, next-generation sequencing (NGS), and genome editing] can enhance variety development in a short time and at the lowest cost with a focus on performance and quality that meet the market requirement through the demand-led breeding approach (Matova et al., 2022).
The main conventional breeding methods in cross-pollinated crops, such as maize, include mass selection, family selection, hybrid breeding, synthetic varieties, backcrossing, and recurrent selection. Hybrid breeding, backcrossing, and recurrent selection are the common breeding methods widely used in developing Striga tolerance/resistance maize varieties (Badu-Apraku et al., 2014).
Hybrid breeding involves crossing genetically complementary inbred lines to combine genes in desirable hybrids. It enables the development of new progenies and inbred lines with several traits (Anushma et al., 2021). The more divergent their parents are, the higher the heterosis in their hybrid or offspring (Reif et al., 2005). The main challenges in hybrid breeding are creating improved populations with desirable agronomic traits and local adaptation while keeping the populations genetically distant to explore hybrid vigor. Also, there is a need to develop efficient seed production of experimental hybrids (Flavio, 2013). Maize hybrids have been widely developed for Striga resistance and high yields. For instance, Menkir and Meseka (2019) reported maize hybrid series H01 to H16 (e.g., single crosses, three-way crosses, and double crosses) developed over three breeding periods that yielded 64% higher and supported 30% or less parasite emergence and damage than their checks (Gethi and Smith, 2004). Other Striga resistance in three-way-cross and single-cross hybrids were reported by Menkir et al. (2012) and were consistent against various S. hermonthica ecotypes in different regions. Some of the three-way hybrids were developed by crossing a common hybrid as a female parent with white grain inbred lines derived from ACRSYN-W and ZEADIPLO BC4 (Menkir et al., 2012). These hybrids are critical genetic resources for Striga resistance gene deployment programs through the breeding of hybrids and OPVs in the target production environments.
Recurrent selection uses repeated cycles of selection and breeding aimed at accumulating useful genes for genetic improvement, especially traits conditioned by polygenes (White, 2004; Darrah et al., 2019). It is an ideal breeding approach to steadily improve quantitative traits in a breeding population. This technique has been widely used to improve maize for Striga resistance and economic traits (Badu-Apraku et al., 2007a; Menkir and Kling, 2007; Badu-Apraku et al., 2008). Menkir and Kling (2007) reported a Striga-resistant variety TZL COMP1-W developed using selfed progeny (S1 and S2 lines) or full-sib family selection schemes. The advanced cycle (C6) of that variety significantly outyielded the base population (C0) by 1628 kg ha−1 and sustained a yield loss of 36%, which was nearly half of the yield loss (70%) recorded in C0 (Menkir and Kling, 2007). The synthetic variety 2000 Syn EE-W derived from TZEE-W Pop STR C0 after a cycle of S1 family recurrent selection and the improved populations, TZEE-W Pop STR C3 and TZEE-Y Pop STR C3, are reported to be the highest yielding entries under Striga-infested and Striga free environments (Menkir and Kling, 2007). These results have shown that reccurent selection is an effective method for increasing the frequency of desirable genes in a population.
Backcrossing is a breeding method that is used to transfer one or a few desirable genes from a donor parent to an agronomically superior and elite recipient parent. It is a form of recurrent hybridization and selection by which an excellent characteristic is added to an otherwise desirable genetic background. The goal is to develop an ideotype containing the novel allele and all other essential traits in the recurrent parent. It works well when the variety to be improved is an inbred line and the trait to be introgressed is monogenic or oligogenic (Thomas et al., 2016). Badu-Apraku et al. (2007a) reported the introgression of Striga resistance into maize by backcrossing, generation of S1 progenies, selection of Striga-resistant S1 lines, and two cycles of recombination of the selected S1 lines under artificial Striga infestation. Akaogu et al. (2019) also reported an increase in Striga-resistant levels in an inbred line TZdEI 352 through backcrossing. The two maize populations, TZE-W Pop DT STR C4 and TZE-Y Pop DT STR C4, were developed using backcrossing, inbreeding, hybridization, and continuous selection. These are valuable Striga-resistant populations with outstanding yield under Striga infestation. The germplasms derived from backcrossing have been useful in developing S. hermonthica-resistant OPVs of maize (Badu-Apraku et al., 2007a).
The development of homozygous and contrasting inbred lines are essential for maize breeding programs for hybrid or synthetic variety breeding with Striga resistance (Chaikam et al., 2019). The traditional inbred line development techniques requires six to eight generations of selfing and selection to achieve homozygosity. Conversely, the doubled haploid (DH) method enables the production of homozygote inbred lines from haploid individuals instantaneously (Trindade, 2022). The DH plants are derivatives of haploid plants with a doubled set of the chromosome. The DH method significantly speeds up the selection process by fixing genes in the homozygous state (Zargar et al., 2022). The DH method has several benefits, including creating lines with 100% homozygosity. Derived lines exhibit maximum genetic variability for selection and test crosses reducing breeding costs and increasing selection efficiency among experimental hybrids. Also, the DH technology exposes recessive and minor genes in homozygous backgrounds
DH lines are developed in vivo or in vitro. The in vitro method involves the use of haploid pollen or anther or female egg cells to induce haploid embryos. This is followed by chromosome doubling of the haploid individuals using chemical agents. The method has shown little success in reliably producing the large number of DH lines required for maize breeding programs (Chaikam et al., 2019; Mitiku, 2022). Hence, the method has yet to be standardized for maize breeding. The in vivo haploid induction has been successfully used in DH production in maize breeding programs (Mitiku, 2022). The in vivo procedures include haploidization following wide crosses and intra-specific crosses. Inter-generic or inter-specific crosses with cultivated maize genotype deliver haploid individuals, which are subjected to chromosome doubling to extract desirable DHs with agronomic traits and Striga resistance. During wide crosses, the chromosomes of the distantly related species are eliminated during early embryogenesis, leaving a haploid embryo (Ishii et al., 2016). Also, during intra-specific crosses, a selected maize parent can be crossed using pollen grains from a distant parent following pollen treatment with chemical or physical mutagenic agents followed by embryo rescue (Trentin, 2019). Striga resistance breeding has been attempted through maize-sorghum hybridization. However, the hybridization between sorghum and maize was only feasible when the crossing was supplemented with a 2, 4-D auxin hormone (Mueni, 2018). The most effective and quickest in vivo method to create DH lines is using a haploid inducer line. Crosses involving a standard maize genotype with a haploid inducer line generate haploid seeds enabling the development of DHs after treating tissue culture established seedling plants with chemical agents such as colchicine (Segui-Simarro et al., 2021). Figure 3 summarizes a scheme showing a rapid method to develop haploids and DHs in maize using a haploid inducer line. Briefly, the technique involves pollination from the inducer line onto silks of the standard genotype, e.g. Striga resistance line. After pollination, the haploid embryos are rescued on a suitable culture media, followed by chromosome doubling using colchicine to develop DH lines. In the absence of colchicine treatment, the callus formed gives rise to haploid individuals that can be selfed to create DH plants.
Figure 3 Scheme depicting the rapid development of DH lines in maize using inducer line. Note the fluorescent marker (haploid embryo) and the purple marker (diploid embryo). The embryos that lack purple color or with fluorescent signals are haploids and will be subjected to chromosome doubling in the culture media (N6) using colchicine.
Wilde et al. (2010) reported the effectiveness of the DH method enabling the exploitation of minor gene effects for low nitrogen adaptation in some European maize landraces. Maize breeders in the Water Efficient Maize for Africa (WEMA) project used the DH method to develop drought-tolerant inbred lines (Beyene et al., 2013). The authors reported that subsequent DH-derived hybrids outyielded commercial hybrid checks that were developed conventionally. Furthermore, the genetic variance of DH lines for GY, plant height, ear height, and leaf length was twice as high than the parental source of the S1 families.
The DH method can be successfully used in the selection of Striga-resistant maize germplasm for breeding. To produce DH resistant to Striga, the breeder should cross a female line resistant to Striga (recipient parent) with a male haploid inducer (donor parent). The seed of the F1 plants is sampled to differentiate haploid and diploid embryos. Only haploid embryos will be selected and transferred into a culture media. The haploid embryo can be cultured with a chromosome-doubling chemical reagent such as colchicine leading to callus formation and subsequently the DH plants, which are homozygous for the genes of interest, that is, Striga resistance. Alternatively, the haploid embryos can be cultured without any chemical reagent to developing haploid plants that could be selfed to select DH plants with Striga resistance. The DH approach will enhance the breeding efficiency for Striga-resistant hybrids or open-pollinated varieties. Furthermore, the use of the DH lines with effective molecular markers enables the identification of targeted QTLs linked to agronomic traits of importance at a lower cost (Mayor and Bernardo, 2009). Molecular markers associated with complex polygenic economic traits can be effectively discerned in DH populations. This will limit the number of genetic materials handled and assayed during phenotyping while increasing the frequency of favorable alleles (Prasanna, 2014). DH lines in tropical maize have been successfully developed using temperate haploid inducers (Prigge et al., 2011). In another study, Battistelli et al. (2013) reported the successful development of DH lines in tropical maize using Krasnodar Embryo Marker Synthetic (KEMS). Furthermore, Beyene et al. (2013) evaluated DH-derived testcross performance under drought stress and non-stress conditions. The authors reported significant differences in trait performance between the drought stress and well-watered conditions for all measured traits except for anthesis date. This suggests that DH derivatives perform better than genotypes developed through the conventional inbreeding platform owing to their homozygosity and genetic stability. Therefore, through DH technology, successful Striga-resistance breeding can be attained in maize
These studies suggest the possibility of successful Striga-resistant DH development in maize.
Until recently, conventional breeding was based on the phenotypic selection of target traits, which could be qualitative or quantitative (Hee-Jong et al., 2015). Phenotypic traits can be quickly and readily selected when the trait of interest is conditioned by major genes. This will yield easily distinguishable genotypes. In contrast, quantitative traits, including Striga resistance and yield-influencing traits, are challenging to select phenotypically due to the involvement of polygenes with minor genetic effect and their low heritability (Nazeer et al., 2010; Schuster, 2011; Araus and Cairns, 2014). The other limit of phenotypic selection in segregating populations is that only the traits conditioned by dominant genes are selected for the next round of crossing, because recessive genes are hidden and may be lost in advanced populations. Furthermore, the duration of selection cycles takes several years, which delays the development and release of new varieties. Therefore, many breeding programs have stagnated because of timelapse and the paucity of analytical tools for phenotyping and genetic analyses (Hee-Jong et al., 2015; Rincent et al., 2018). Recent advances in high-throughput phenotyping and genotyping have provided fast genetic information for accelerated breeding.
The damage inflicted by Striga is through the extraction of the host’s metabolites and the secretion of phytotoxic compounds that leave the host with a diseased-like appearance. The stay green (SG) genotype selection may be an essential component of Striga resistance in maize. Genotypes with SG character have the capacity to maintain their leaves photosynthetically active. This will subsequently improve grain development and filling, including under stress conditions (Vadez et al., 2013).
Kamal et al. (2019) reported functional and non-functional SG genotypes. The functional SG genotypes maintain their photosynthetic capacity compared with the non-SG genotypes. Functional SG genotypes delay the onset of senescence, or the senescence syndrome is initiated and grows slowly (Thomas and Ougham, 2014). Conversely, in the non-functional SG genotypes, senescence is commenced on an average time scale; however, leaf greenness is extended because of the failure of the chlorophyll degradation pathway, with a decline in photosynthetic capacity (Kamal et al., 2019). Both forms of SG may be associated with Striga resistance and GY improvement in maize.
Also, SG has been identified as an important component of the genetic improvement of several crops. For instance, Luche et al. (2013) reported the association between SG and GY improvement in wheat, while Silva et al. (2004) reported the association between SG and higher grain quality in wheat. The association between SG with tolerance to abiotic and biotic stresses has also been reported by Kassahun et al. (2009) and Joshi et al. (2006) in sorghum and wheat, respectively. SG has been extensively used to improve tolerance to drought and heat in sorghum (Sanchez et al., 2002; Vadez et al., 2013) and maize (Wang et al., 2012; Cerrudo et al., 2017). There is ongoing research at the International Crops Research Institute for the Semi-Arid Tropics (ICRISAT) to transfer QTLs conditioning SG into genetically diverse, tropically adapted elite sorghum varieties for drought tolerance through marker-assisted backcrossing (MABC) (Isaac et al., 2019). Yang et al. (2017) studied SG QTLs in maize using a heterogeneous inbred family approach and found that the SG-associated parameters were significantly correlated with crop yield. These QTLs could be targeted for marker-assisted backcross transfer into maize varieties to improve maize yield under Striga infestation.
High-throughput phenotyping platforms (HTPP) have revolutionized phenomics in plant breeding programs in the last 10 years (Jangra et al., 2021). HTPP relies on high data recording capacities, data collection and analysis speed, and non-destructive, non-invasive, and remote-sensing phenotyping techniques (Prasanna et al., 2013). Its utilization allows for the screening of many plants at various phenological stages so that desired traits can be rapidly screened at the initial stages, eliminating the need to wait for plant maturation in the field (Cabrera-Bosquet et al., 2012). HTPP can be used in the laboratory and field. The following platforms are some examples of HTPP used in cereal breeding programs: the ScanAlyzer HTPP platform developed by LemnaTec (Virlet et al., 2016), PHENOPSIS developed by Optimalog, on contract by the Laboratory of Plant Ecophysiological responses to Environmental stresses, in Montpellier, France (Granier et al., 2006), and DuPont-Pioneer’s FAST Corn (Jin et al., 2021).
Near-infrared spectroscopy (NIRS) is a chemometric technique that combines spectroscopy and mathematics to rapidly produce indirect, quantitative estimates of the concentration of organic compounds. This technique has been widely implemented in laboratory analysis of grain traits in several crops, such as maize, sorghum, and wheat, due to its low cost, rapidity, high precision, and repeatability for assessing several plant traits (Jangra et al., 2021). Imaging Technologies for Plant Phenotyping, Visible Light Imaging, and Fluorescence are also high-throughput phenomic platforms that have emerged as promising methods for the high-throughput phenotyping of crops (Das et al., 2015; Knecht et al., 2016; Jangra et al., 2021). Quality and yield, spikelet number, main orthogonal grain dimensions, maize cob, ear, and kernel attributes have been studied using the technology of structural imaging (Igathinathane et al., 2009; Miller et al., 2017; Makanza et al., 2018). All these platforms are opportunities to be explored to speed up phenotyping for Striga resistance in maize. Using high-throughput genotyping (HTG) in combination with high-throughput digital phenotyping, it is possible to increase marker density and improve the precision and resolution of QTL detection (Bhat et al., 2020).
Genetic markers can be grouped into two categories: classical markers, including biochemical markers, and DNA/molecular markers. Biochemical markers or isozymes are multi-molecular forms of enzymes that are coded by various genes but have the same functions (Bailey, 1983). Biochemical markers have been successfully applied in the detection of genetic diversity, population structure, gene flow, and population subdivision (Nadeem et al., 2017). Abdellatif and Khidr (2010) examined the genetic diversity of new maize hybrids based on simple sequence repeat (SSR) markers and biochemical markers and concluded that both molecular (e.g., SSR, ISSR, and RAPD) and biochemical markers (e.g., seed storage protein content) are efficient tools. Rahim (2019) reported significant variation among maize accessions using morpho-biochemical markers. Biochemical markers are easy to use and cost effective. However, they are fewer in number and detect less polymorphism (Mondini et al., 2009; Nadeem et al., 2017).
Striga seed germination requires the detection of SL, a group of chemical compounds released from host plants’ roots (Mishra et al., 2016). After the Striga seed germination, the radicle of the germinating seed penetrates the host root, subsequently forming a haustorium to establish a xylem–xylem connection with the host, through which to withdraw water and nutrients (Jamil et al., 2011). Maize plants that release high levels of SL promote higher levels of Striga infestation (Jamil et al., 2011; Jamil et al., 2012). Hence, maize varieties with low levels of SL secretion should have reduced levels of Striga infestation, thereby reducing maize yield losses in the field under Striga infestation. Quantification of the release of SL could serve as biochemical markers to select for Striga resistance maize genotypes.
MAS is the process of using morphological, biochemical, or DNA markers to select plants carrying genomic regions involved in expressing phenotypic traits of interest (Choudhary et al., 2008). Molecular markers, genetic maps, and associated low-cost genotyping technologies powered by advances in genomic sequencing and the advent of single-nucleotide polymorphism (SNP) markers have facilitated MAS for qualitative and quantitative traits (Boopathi, 2020; Rajesh et al., 2021). The selection can occur at different stages in a maize breeding program. The first possible stage is when selecting elite lines to create inbred lines (Ragot et al., 2007). At that stage, markers are used to distinguish heterotic groups from which inbred lines are developed by crossing elite lines within heterotic groups and hybrids with high heterosis are developed by crossing lines from different heterotic groups (Semagn et al., 2012). Several studies have been conducted to determine Striga-resistant heterotic groups. For example, Menkir et al. (2005) identified heterotic groups of Striga-resistant inbred lines among source populations using amplified fragment length polymorphism (AFLP) and SSR markers. Mengesha et al. (2017) reported four heterotic groups of Striga-resistant inbred lines using SNPs. These inbred lines are available for Striga-resistant breeding programs.
The most direct use of MAS in maize is MABC where targeted genes are introgressed into elite inbred lines (Crosbie et al., 2006). MABC has been implemented to improve traits in maize and other cereal crops. For example, Ribaut and Ragot (2007) used MABC to improve drought adaptation in maize varieties. Yohannes et al. (2015); Ali et al. (2016), and Ngugi et al. (2015) reported MABC for Striga resistance in sorghum. However, no study has been yet reported on MABC in Striga resistance in maize. Marker-assisted recurrent selection (MARS) is another commonly used method to increase the frequency of favorable alleles in crops by enhancing the efficiency of recurrent selection and accelerate the progress of the procedure (Kebede et al., 2021). Beyene et al. (2016) reported the use of MARS to improve maize GY under drought stress by crossing two elite Striga-resistant inbred lines (Acr.SynW-S2-173-B∗ 4) and (TZLComp.1C4-S1-37-5-B∗ 3), which are also tolerant to drought. The authors reported GY increased from the original to the advanced selection occurred only under drought stress (Abdulmalik et al., 2017). SNP markers linked to Striga resistance in maize have been widely discovered. SNPs have become the markers of choice due to their low cost per data point, high genomic abundance, locus specificity, co-dominance, the potential for high-throughput analysis, and lower genotyping error rates (Lipka et al., 2012; Adu et al., 2019). The SNPs reported by Stanley et al. (2021); Mengesha et al. (2017), (Pfunye et al., 2021) suggest the possibility of successful MAS in the future.
Integrating MAS with conventional breeding techniques will accelerate breeding and genetic gain. A persistent problem with the use of discrete genomic markers is that their presence in a genome does not guarantee their phenotypic expression, which is particularly important with polygenic, quantitative traits, where multiple SNPs are involved in the expression of a single trait, such as Striga resistance.
Genomic tools such as molecular markers help identify, locate, and mapping genes responsible for economic traits such as Striga resistance and agronomic traits in maize. The technology involved linkage mapping and association or linkage disequilibrium (LD) mapping. Genetic recombination is the basis for linkage and association mapping (Wang and Qin, 2017). Linkage mapping is traditionally used based on genetic recombination events during the development of populations (Sonah et al., 2015). Mapping populations developed through initial biparental crosses lead to the development of F2, backcrosses [BCs], recombinant inbred lines [RILs], DHs, and near-isogenic lines [NILs], with a small number of accumulated recombination events (Balasubramanian et al., 2009). The QTL intervals found are extended over several centimorgans (cMs), and a genetic distance translates into large genomic regions with many candidate genes. This renders linkage mapping with a relatively low-mapping resolution and allele richness (Xu et al., 2017). Conversely, association mapping is a complementary tool to linkage mapping that resolves complex trait variation by exploiting historical and evolutionary recombination events at the population level (Xu and Crouch, 2008; Myles et al., 2009).
With the advent of rapid genome-wide, high-density marker data using high-throughput and NGS technologies, genome-wide association studies (GWAS) have become a standard tool for identifying resistance genes and loci (Adewale et al., 2020). GWAS, also known as whole-genome association study (WGAS), identifies genomic regions controlling quantitative economic traits (Adewale et al., 2020). It includes collecting a sample population, phenotyping, genotyping, and testing the association between phenotypes and genotypes using statistical approaches. GWAS is used to evaluate the association between each genotyped marker and a phenotype of interest that has been scored across a large number of individuals to guide MAS (Korte and Farlow, 2013).
However, GWAS has some limitations in identifying unknown genes (Lipka et al., 2012). The first limitation is the diversity panels of crop species that often represent a strong population structure, leading to false positives because of spurious associations between the phenotypes and unlinked markers (Benavente and Giménez, 2021; Phuke et al., 2022). The second limitation is the large extent of LD, which often ranges over several hundred kilobases. This may result in the inclusion of many candidate genes in a single LD block exhibiting a significant signal, thus entailing the need for additional experiments to conclusively identify the causal gene(s) (Yano et al., 2016). A nested association mapping population (NAM), a multiparent advanced generation intercrossing population (MAGIC), and random-open-parent association mapping (ROAM) are suitable for GWAS, because they are enriched in variations and have inconspicuous population structures that are more powerful for QTL mapping (Cavanagh et al., 2008; Tian et al., 2011; Huang et al., 2021). The NAM approach provides high-genetic variation while avoiding the complications of genetic structure, whereas MAGIC can examine the effect of loci, unbiased due to the balanced contributions from all founders (Wang and Qin, 2017). ROAM improves genetic resolution and statistical power to identify variants with minor effects and low frequency (Fang and Luo, 2019).
With a well-defined population and an appropriate statistical model, GWAS is among the most prominent and reliable approaches for detecting the whole genetic architecture of a trait. Table 5 summarizes to date candidate genes/QTLs conferring resistance to Striga. The first QTLs associated with Striga resistance in maize were reported by Amusan (2010). Two QTLs were mapped on Chromosome 6 in the populations derived from an F2-mapping population involving a cross between the susceptible maize inbred lines 5057 and the resistant ZD05. These two QTLs accounted for 55% of observed phenotype variation for incompatible responses to S. hermonthica infestation on host roots. The second report was in a study conducted by Adewale et al. (2020) using GWAS. The locus S9_154,978,426 on Chromosome 9 was found at 2.61 Mb close to the ZmCCD1 gene, which is known to be associated with reducing SL production in the maize roots (Adewale et al., 2020). Badu-Apraku et al. (2020a) identified 14 QTL associated with S. hermonthica resistance indicator traits, comprising three QTLs for GY, four QTLs for ear per plant, and seven QTLs for S. hermonthica damage, at 10 weeks after planting across environments, and found 154 candidate genes associated with Striga resistance/tolerance traits. Another 12 QTLs associated with S. hermonthica resistance traits were found in an F2:3 population involving a cross between yellow inbred lines, TZEEI 79 (Striga resistant/tolerant) and TZdEEI 11 (Striga susceptible) by Badu-Apraku et al. (2020c) using QTL mapping. Pfunye et al. (2021) identified three SNPs on Chromosomes 5, 6, and 7 linked to the total Striga plant emergence. Gowda et al. (2021) reported 57 SNPs significantly associated with Striga resistance indicator traits and GY under artificial Striga infestation with low to moderate effect. They found 32 candidate genes physically near the significant SNPs with roles in plant defense against biotic stresses.
Few studies have reported on QTLs and genes linked to Striga resistance in maize. Using GWAS, Okunlola et al. (2023) identified 22 SNP markers significantly associated with GY and Striga damage rating at 10 weeks after planting, the number of emerged Striga plants at 8 and 10 weeks after planting, and ear aspect. There are several SNPs and QTLs involved in Striga resistance. Hence, the breeder needs to filter useful markers for MAS.
The development and application of molecular markers in crop genetics have gained remarkable success in the past three decades. The use of molecular markers in crop improvement programs started from low-throughput morphological markers (Soltabayeva et al., 2021), restriction fragment length polymorphism (RFLP) (Powell et al., 1996; Ahmar et al., 2020) to SNP markers based on NGS (Jenkins and Gibson, 2002; De Donato et al., 2013). Maize populations have been genotyped using several methods, such as Random Amplified Polymorphic DNA (RAPD) (Carvalho et al., 2004), RFLP (Garcia et al., 2004), and AFLP (Schrag et al., 2006). The SNPs are the most widely used markers for assessing the genetic diversity and association mapping in maize (Dos Santos et al., 2016; Kasoma et al., 2020; Tomkowiak et al., 2021). The SNPs can be observed through various experimental protocols.
Genotyping by sequencing (GBS) (De Donato et al., 2013), restriction-associated DNA (RAD), complexity reduction of polymorphic sequences (CRoPS) (van Orsouw et al., 2007), and diversity arrays technology (DArT) are among available approaches to do SNPs discovering and genotyping. However, GBS is the most popular approach to the identification of SNPs in plants (Negro et al., 2019; Heinrich et al., 2020; Lee et al., 2021). The GBS is a novel application of NGS protocols for discovering and genotyping SNPs in crop genomes and populations. The development of NGS technologies enhanced the genomics landscape in crop breeding. Several NGS technologies such as Roche 454 FLX Titanium, Illumina, MiSeq, and HiSeq 2500 have been developed. These technologies provide various genotyping platforms with great improvements in coverage, time, and costs (Bevan et al., 2017).
The DArT is a sequence-independent, high-throughput, reproducible, cost-effective, and whole (Jaccoud et al., 2001) genome genotyping technology. The DArT method allows simultaneous detection of several thousand DNA polymorphisms (depending on the species) arising from single-base changes and small insertions and deletions (InDels). The process involves scoring the presence or absence of DNA fragments in genomic representations generated from genomic DNA samples through a process of complexity reduction (Jaccoud et al., 2001). This is achieved using a combination of restriction enzymes that separate low-copy sequences (most informative for marker discovery and typing) from the repetitive fraction of the genome. The combination of the complexity reduction of the DArT method with NGS led to DArTseq technology for identifying DArT markers through the DArTseq protocol (Kilian et al., 2012; Raman et al., 2014). DArTseq is the most preferred method of GBS (Sanchez-Sevilla et al., 2015). The initial DArT implementation on the microarray platform involved fluorescent labeling of representations and hybridization to dedicated DArT arrays. The DArTseq method deploys sequencing of the representations on the NGS platforms (https://www.diversityarrays.com/technology-and-resources/dartseq/). Most recent studies on genotyping maize for Striga resistance used the DArTseq method (Adewale et al., 2020; Badu-Apraku et al., 2020a; Badu-Apraku et al., 2020c; Yacoubou et al., 2021c; Zebire et al., 2021). However, more reliable markers linked to Striga resistance in maize should be explored to facilitate breeding for Striga-resistant maize through MAS.
Abiotic and biotic stress, including Striga damage, cause an extensive loss to agricultural production and productivity worldwide (Gull et al., 2019). A combination of breeding approaches and new technologies could significantly improve crop stress resistance/tolerance in the field. Genetic engineering, also called genetic modification (GM), uses laboratory-based technologies to produce a desired trait by adding a gene from one species to an organism from a similar or different species (Mackelprang and Lemaux, 2020; Sahu, 2020). GM of crop plants has been achieved for GY and other economic traits improvement. For example, herbicide-resistant maize and soybean and Bt-derived maize, soybean, and cotton are some of the genetically engineered crops developed to date (Mittler and Blumwald, 2010; Basnet et al., 2022). Several studies, including meta-analyses, have reported the economic potential of genetic engineering crops to harness crop productivity (Areal et al., 2012; Klumper and Qaim, 2014; Pellegrino et al., 2018). There are increasing cases that GM products are safe for human consumption and the environment (Fernbach et al., 2019). However, the risks and benefits of GMOs have led to fears that are still being debated (Blagoevska et al., 2021).
Genome editing (GE) is a suite of advanced biotechnological techniques that allows for the precise and efficient modification of an organism’s genome. The technology includes mega-nucleases or homing endonucleases (HEs), zinc finger nucleases (ZFNs), transcription activator-like effector nucleases (TALENs), and Type II clustered regularly interspaced short palindromic repeat (CRISPR)/CRISPR-associated protein (Cas) (Hua et al., 2019; Ku and Ha, 2020; Trindade, 2022). These GE systems generate targeted DNA double-strand breaks (DSBs) in the genome. The potential of CRISPR in maize improvement was reported by various authors. Feng et al. (2016) and Zhu et al. (2016) reported efficient target genome modification in maize using the CRISPR/Cas9 system. More recently, aromatic maize has been created using CRISPR/Cas9 (Wang et al., 2021). However, no study has reported on the use of genetic editing for Striga resistance improvement in maize.
The above techniques are promising and offer new opportunities for developing new Striga-resistant maize varieties in the shortest time possible. The availability of Striga resistance genes in maize will facilitate the use of genetic engineering and gene editing for Striga-resistant maize development. Therefore, there is a need to identify more Striga-resistant genes or QTLs that confer resistance to maize. The development of high-throughput DNA-sequencing technology and the establishment of a large number of “omics” databases will facilitate the identification of useful Striga resistance traits for genome editing in maize.
The parasitic weed Striga has a devastating impact on cereals, especially maize production in SSA. Many organizations have been involved in developing strategies to combat the parasite; thus, several control methods have been developed and tested. This has led to the development of a range of genetic resources of maize existing for Striga resistance. Several maize varieties with partial resistance have been developed and released in Africa. However, no best practice is available to control the Striga parasite in maize, needing integrated management spearheaded by Striga-resistance breeding. Primary genetic resources of maize for Striga resistance, including landraces, wild relatives, mutants, and synthetic varieties, reported in this paper may guide new variety designs in maize for Striga-resistance and desirable product profiles. Integrating conventional breeding, mutation breeding, and genomic-assisted breeding (i.e., MAS, QTL analysis, NGS, and genome editing) will enhance genetic gains in Striga resistance breeding programs.
ED: Conceptualization; Investigation; Methodology; Resources; Writing original draft; Writing – Review & Editing. HS: Conceptualization; Funding acquisition; Methodology; Project administration; Resources; Supervision; Validation; Visualization; Writing – Review & Editing. EM: Validation; Visualization; Writing – Review & Editing. AS: Validation; Visualization; Writing – Review & Editing. ML: Funding acquisition; Resources; Supervision; Validation; Visualization; Writing – Review & Editing.
The University of KwaZulu-Natal (UKZN) and the Organisation for Women in Science for the Developing World (OWSD) are acknowledged for the financial support of this study.
The authors declare that the research was conducted in the absence of any commercial or financial relationships that could be construed as a potential conflict of interest.
All claims expressed in this article are solely those of the authors and do not necessarily represent those of their affiliated organizations, or those of the publisher, the editors and the reviewers. Any product that may be evaluated in this article, or claim that may be made by its manufacturer, is not guaranteed or endorsed by the publisher.
Abdellatif, K. F., Khidr, Y. A. (2010). Genetic diversity of new maize hybrids based on SSR markers as compared with other molecular and biochemical markers. J. Crop Sci. Biotechnol. 13, 139–145. doi: 10.1007/s12892-010-0068-x
Abdoul-Raouf, S. M., Ju, Q., Jianyu, M., Zhizhai, L. (2017). Utilization of wild relatives for maize (Zea mays l.) improvement. Afr. J. Plant Sci. 11, 105–113. doi: 10.5897/AJPS2017.1521
Abdulmalik, R. O., Menkir, A., Meseka, S. K., Unachukwu, N., Ado, S. G., Olarewaju, J. D., et al. (2017). Genetic gains in grain yield of a maize population improved through marker-assisted recurrent selection under stress and non-stress conditions in West Africa. Front. Plant Sci. 8, 11. doi: 10.3389/fpls.2017.00841
Achigan-Dako, E. G., Houdegbe, A. C., Glèlè, M., Nono-Womdim, R. (2014). Analyse du système de production et de distribution des semences de maïs (Zea mays l.) au sud-bénin. Biotechnology Agronomy Société Environnement 18 (1), 49–60.
Adewale, S. A., Badu-Apraku, B., Akinwale, R. O., Paterne, A. A., Gedil, M., Garcia-Oliveira, A. L. (2020). Genome-wide association study of Striga resistance in early maturing white tropical maize inbred lines. BMC Plant Biol. 20, 203. doi: 10.1186/s12870-020-02360-0
Adu, B. G., Badu-Apraku, B., Akromah, R., Garcia-Oliveira, A. L., Awuku, F. J., Gedil, M. (2019). Genetic diversity and population structure of early-maturing tropical maize inbred lines using SNP markers. PloS One 14, e0214810. doi: 10.1080/15427528.2019.1661055
Ahloowalia, B. S., Maluszynski, M. (2001). Induced mutations–a new paradigm in plant breeding. Euphytica 118.2, 167–173. doi: 10.1023/A:1004162323428
Ahmar, S., Gill, R. A., Jung, K. H., Faheem, A., Qasim, M. U., Mubeen, M., et al. (2020). Conventional and molecular techniques from simple breeding to speed breeding in crop plants: recent advances and future outlook. Int. J. Mol. Sci. 21, 2107–2590. doi: 10.3390/ijms21072590
Akaogu, I. C., Badu-Apraku, B., Tongoona, P., Ceballos, H., Gracen, V., Offei, S. K., et al. (2019). Inheritance of Striga hermonthica adaptive traits in an early-maturing white maize inbred line containing resistance genes from Zea diploperennis. Plant Breed. 138, 546–552. doi: 10.1111/pbr.12707
Akinwale, R. O., Badu-Apraku, B., Fakorede, M. A. B. (2013). Evaluation of Striga-resistant early maize hybrids and test locations under Striga-infested and Striga-free environments. Afr. Crop Sci. J. 21.1, 1–19.
Ali, R., Hash, C. T., Damris, O., Elhussein, A., Mohamed, A. H. (2016). Introgression of striga resistance into popular Sudanese sorghum varieties using marker assisted selection. World J. Biotechnol. 1, 48–55. doi: 10.33865/wjb.001.01.0006
Ali, F., Kanwal., N., Ahsan, M., Ali, Q., Bibi, I., Niazi, N. K. (2015). Multivariate analysis of grain yield and its attributing traits in different maize hybrids grown under heat and drought stress. Scientifica (Cairo) 2015, 563869. doi: 10.1155/2015/563869
Aliyu, L., Lagoke, S., Carsky, R., Kling, J., Omotayo, O., Shebayan, J. (2004). Technical and economic evaluation of some Striga control packages in maize in the Nigerian Guinea savanna. Crop Prot. 23, 65–69. doi: 10.1016/S0261-2194(03)00141-8
Amusan, I. O. (2010). Mechanisms and quantitative trait loci for striga hermonthica resistance in maize (Zea mays l.) inbred line (Lafayette, Indiana: Purdue University), 1–188.
Amusan, I. O., Rich, P. J., Menkir, A., Housley, T., Ejeta, G. (2008). Resistance to Striga hermonthica in a maize inbred line derived from Zea diploperennis. New Phytol. 178, 157–166. doi: 10.1111/j.1469-8137.2007.02355.x
Anushma, P., Dhanyasree, K., Rafeekher, M. (2021). Wide hybridization for fruit crop improvement: a review. Int. J. Chem. Stud. 9, 769–773. doi: 10.22271/chemi.2021.v9.i1k.11317
Araus, J. L., Cairns, J. E. (2014). Field high-throughput phenotyping: the new crop breeding frontier. Trends Plant Sci. 19, 52–61. doi: 10.1016/j.tplants.2013.09.008
Areal, F. J., Riesgo, L., RodrÍGuez-Cerezo, E. (2012). Economic and agronomic impact of commercialized GM crops: a meta-analysis. J. Agric. Sci. 151, 7–33. doi: 10.1017/S0021859612000111
Azeez, M. A., Adubi, A. O., Durodola, F. A. (2018). “Landraces and crop genetic improvement,” in Rediscovery of landraces as a resource for the future (Ladoke Akintola University of Technology: IntechOpen).
Babatima, D., Khamaldin, M., Khamaldin, M., Ephraim, M., Ephraim, S., Victor, S., et al. (2011). Imazapyr-resistant maize technology adoption for witch weed control in western Kenya. Afr. Crop Sci. J. 19 (3), 173–182.
Badu-Apraku, B., Adewale, S., Paterne, A., Gedil, M., Asiedu, R. (2020a). Identification of QTLs controlling Resistance/Tolerance to Striga hermonthica in an extra-early maturing yellow maize population. Agronomy-Basel 10, 18. doi: 10.3390/agronomy10081168
Badu-Apraku, B., Adewale, S., Paterne, A. A., Gedil, M., Toyinbo, J., Asiedu, R. (2020c). Identification of QTLs for grain yield and other traits in tropical maize under Striga infestation. PloS One 15, 20. doi: 10.1371/journal.pone.0239205
Badu-Apraku, B., Adu, G. B., Yacoubou, A. M., Toyinbo, J., Adewale, S. (2020b). Gains in genetic enhancement of early maturing maize hybrids developed during three breeding periods under Striga-infested and Striga-free environments. Agronomy-Basel 10, 19. doi: 10.3390/agronomy10081188
Badu-Apraku, B., Ewool, M., Yallou, C. G. (2010). Registration of Striga-resistant tropical extra-early maize population. J. Plant Registrations 4, 60–66. doi: 10.3198/jpr2009.05.0276crg
Badu-Apraku, B., Fakorede, M. A. B. (2017). “Maize in sub-Saharan Africa: importance and production constraints,” in Advances in genetic enhancement of early and extra-early maize for Sub-Saharan Africa (Switzerland: Springer, Cham), 3–10.
Badu-Apraku, B., Fakorede, M. A. B., Fontem Lum, A. (2007a). Evaluation of experimental varieties from recurrent selection for Striga resistance in two extra-early maize populations in the savannas of West and central Africa. Exp. Agric. 43, 183–200. doi: 10.1017/S0014479706004601
Badu-Apraku, B., Fakorede, M. A. B., Lum, A. F. (2008). S-1 family selection in early-maturing maize populations in Striga-infested and Striga-free environments. Crop Sci. 48, 1984–1994. doi: 10.2135/cropsci2007.11.0639
Badu-Apraku, B., Fakorede, M. A. B., Menkir, A. (2009). Recurrent selection for Striga resistance in early and extra-early maize populations in West Africa. Afr. Crop Sci. Conf. Proc. 9, 415–424.
Badu-Apraku, B., Fakorede, M. A., Oyekunle, M. (2014). Agronomic traits associated with genetic gains in maize yield during three breeding eras in West Africa. Maydica 59, 9.
Badu-Apraku, B., Menkir, A., Kling, J. G., Fakorede, M. A. B. (2006). Registration of 16 Striga-resistant early maturing tropical maize inbred lines. Crop Sci. 46, 1410–1411. doi: 10.2135/cropsci2005.11-0408
Badu-Apraku, B., Menkir, A., Lum, A., Fakorede, M., Ibikunle, O. (2007b). “Recent advances in breeding for Striga-resistant extra-early maize for the savannas of West and Central Africa. Demand-driven technologies for sustainable maize production in West and Central Africa. IITA Ibadan, Nigeria. 515.
Badu-Apraku, B., Talabi, A. O., Fakorede, M. A. B., Fasanmade, Y., Gedil, M., Magorokosho, C., et al. (2019). Yield gains and associated changes in an early yellow bi-parental maize population following genomic selection for Striga resistance and drought tolerance. BMC Plant Biol. 19, 17. doi: 10.1186/s12870-019-1740-z
Badu-Apraku, B., Yallou, C. G. (2009). Registration of Striga-resistant and drought-tolerant tropical early maize populations TZE-W pop DT STR C4 and TZE-y pop DT STR C4. J. Plant Registrations 3, 86–90. doi: 10.3198/jpr2008.06.0356crg
Badu-Apraku, B., Yallou, C. G., Alidu, H., Talabi, A. O., Akaogu, I. C., Annor, B., et al. (2016). Genetic improvement of extra-early maize cultivars for grain yield and Striga resistance during three breeding eras. Crop Sci. 56, 2564–2578. doi: 10.2135/cropsci2016.02.0089
Badu-Apraku, B., Yallou, C. G., Obeng-Antwi, K., Alidu, H., Talabi, A. O., Annor, B., et al. (2017). Yield gains in extra-early maize cultivars of three breeding eras under multiple environments. Agron. J. 109, 418–431. doi: 10.2134/agronj2016.10.0566
Badu-Apraku, B., Yallou, C. G., Oyekunle, M. (2013). Genetic gains from selection for high grain yield and Striga resistance in early maturing maize cultivars of three breeding periods under Striga-infested and Striga-free environments. Field Crops Res. 147, 54–67. doi: 10.1016/j.fcr.2013.03.022
Bailey, D. C. (1983). “Isozymic variation and plant breeders’ rights,” in Developments in plant genetics and breeding, vol. 1. (Amsterdam: Elsevier), 425–440.
Balasubramanian, S., Schwartz, C., Singh, A., Warthmann, N., Kim, M. C., Maloof, J. N., et al. (2009). QTL mapping in new arabidopsis thaliana advanced intercross-recombinant inbred lines. PloS One 4, e4318. doi: 10.1371/journal.pone.0004318
Basnet, P., Dhital, R., Rakshit, A. (2022). Biopesticides: a genetics, genomics, and molecular biology perspective. Biopesticides 2, 107–116. doi: 10.1016/B978-0-12-823355-9.00019-5
Battistelli, G. M., Von Pinho, R. G., Justus, A., Couto, E. G., Balestre, M. (2013). Production and identification of doubled haploids in tropical maize. Genet. Mol. Res. 12, 4230–4242. doi: 10.4238/2013.October.7.9
Benavente, E., Giménez, E. (2021). Modern approaches for the genetic improvement of rice, wheat, and maize for abiotic constraints-related traits: a comparative overview. Agronomy 11, 376. doi: 10.3390/agronomy11020376
Bevan, M. W., Uauy, C., Wulff, B. B., Zhou, J., Krasileva, K., Clark, M. D. (2017). Genomic innovation for crop improvement. Nature 543, 346–354. doi: 10.1038/nature22011
Beyene, Y., Mugo, S., Semagn, K., Asea, G., Trevisan, W., Tarekegne, A., et al. (2013). Genetic distance among doubled haploid maize lines and their testcross performance under drought stress and non-stress conditions. Euphytica 192, 379–392. doi: 10.1007/s10681-013-0867-5
Beyene, Y., Semagn, K., Crossa, J., Mugo, S., Atlin, G. N., Tarekegne, A., et al. (2016). Improving maize grain yield under drought stress and non-stress environments in sub-Saharan Africa using marker-assisted recurrent selection. Crop Sci. 56, 344–353. doi: 10.2135/cropsci2015.02.0135
Bhat, J. A., Deshmukh, R., Zhao, T., Patil, G., Deokar, A., Shinde, S., et al. (2020). ). harnessing high-throughput phenotyping and genotyping for enhanced drought tolerance in crop plants. J. Biotechnol. 324, 248–260. doi: 10.1016/j.jbiotec.2020.11.010
Blagoevska, K., Ilievska, G., Jankuloski, D., Dimzoska, B. S., Crceva, R., Angeleska, A. (2021). “The controversies of genetically modified food,” in IOP conference series: earth and environmental science (United Kingdom: IOP publishing), vol. 854. , 012009.
Boopathi, N. M. (2020). “Marker-assisted selection (MAS),” in Genetic mapping and marker-assisted selection (Singapore: Springer), 343–388.
Cabrera-Bosquet, L., Crossa, J., von Zitzewitz, J., Serret, M. D., Araus, J. L. (2012). High-throughput phenotyping and genomic selection: the frontiers of crop breeding converge. J. Integrated Plant Biol. 54, 312–320. doi: 10.1111/j.1744-7909.2012.01116.x
Cairns, J. E., Hellin, J., Sonder, K., Araus, J. L., MacRobert, J. F., Thierfelder, C., et al. (2013). Adapting maize production to climate change in sub-Saharan Africa. Food Secur. 5, 345–360. doi: 10.1007/s12571-013-0256-x
Carvalho, V. P., Ruas, C. F., Ferreira, J. M., Moreira, R. M., Ruas, P. M. (2004). Genetic diversity among maize (Zea mays l.) landraces assessed by RAPD markers. Genet. Mol. Biol. 27, 228–236. doi: 10.1590/S1415-47572004000200017
Cavanagh, C., Morell, M., Mackay, I., Powell, W. (2008). From mutations to MAGIC: resources for gene discovery, validation and delivery in crop plants. Curr. Opin. Plant Biol. 11, 215–221. doi: 10.1016/j.pbi.2008.01.002
Cerrudo, D., González Pérez, L., Mendoza Lugo, J., Trachsel, S. (2017). Stay-green and associated vegetative indices to breed maize adapted to heat and combined heat-drought stresses. Remote Sens. 9 (13), 235. doi: 10.3390/rs9030235
Chaikam, V., Molenaar, W., Melchinger, A. E., Boddupalli, P. M. (2019). Doubled haploid technology for line development in maize: technical advances and prospects. Theor. Appl. Genet. 132, 3227–3243. doi: 10.1007/s00122-019-03433-x
Chidiebere, N. A., Amuche, N. J., Eyiuche, N. J. (2015) Witchweed (Striga asiatica): a destructive crop plant parasitic weeds. Available at: https://nwezejustus.wordpress.com/2015/07/28/witchweed-striga-asiatica-a-destructive-crop-plant-parasitic-weeds/.
Choudhary, K., Choudhary, O. P., Shekhawat, N. S. (2008). Marker-assisted selection: a novel approach for crop improvement. American-Eurasian J. Agron. 1 (2), 26–30.
Crosbie, T. M., Eathington, S. R., Johnson, G. R., Sr., Edwards, M., Reiter, R., Stark, S., et al. (2006). “Plant breeding: past, present, and future,” in Plant breeding: the arnel r. hallauer international symposium (Monsanto: Wiley Online Library.), 3–50.
Dan, M., Craufurd, P. Q., Kanampiu, F. (2017). Herbicide Resistant Maize Seed Production, And Handling. (CIMMYT Mexico: Internattional Maize and Wheat Improvement CIMMYT), 17.
Darrah, L. L., McMullen, M. D., Zuber, M. S. (2019). “Breeding, genetics, and seed corn production,” in Corn (St Paul, USA: American Association of Cereal Chemists), 19–41.
Das, A., Schneider, H., Burridge, J., Ascanio, A. K. M., Wojciechowski, T., Topp, C. N., et al. (2015). Digital imaging of root traits (DIRT): a high-throughput computing and collaboration platform for field-based root phenomics. Plant Methods 11 (1), 1–12. doi: 10.1186/s13007-015-0093-3
De Donato, M., Peters, S. O., Mitchell, S. E., Hussain, T., Imumorin, I. G. (2013). Genotyping-by-sequencing (GBS): a novel, efficient and cost-effective genotyping method for cattle using next-generation sequencing. PloS One 8, e62137. doi: 10.1371/journal.pone.0062137
Dhliwayo, V., Gasura, E., Nyakurwa, C. S., Mabasa, S., Mashingaidze, A. B., Setimela, P. (2021). Germplasm bred for resistance to Striga hermonthica exhibited high resistance levels to Striga asiatica compared to commercial checks. Adv. Agric. 2021, 11. doi: 10.1155/2021/9915370
Diskin, S. J., Li, M., Hou, C., Yang, S., Glessner, J., Hakonarson, H., et al. (2008). Adjustment of genomic waves in signal intensities from whole-genome SNP genotyping platforms. Nucleic Acids Res. 36, e126. doi: 10.1093/nar/gkn556
Dos Santos, J. P., Pires, L. P., de Castro Vasconcellos, R. C., Pereira, G. S., Von Pinho, R. G., Balestre, M. (2016). Genomic selection to resistance to stenocarpella maydis in maize lines using DArTseq markers. BMC Genet. 17, 86. doi: 10.1186/s12863-016-0392-3
Ejeta, G. (2007). Breeding for Striga resistance in sorghum: exploitation of an intricate host-parasite biology. Crop Sci. 47, S216–S227. doi: 10.2135/cropsci2007.04.0011IPBS
Ejeta, G., Gressel, J. (2007). Integrating new technologies for striga control: towards ending the witch-hunt (Singapore: World Scientific Publishing Company), 3–16.
Ejeta, G., Rich, P. J., Mohamed, A. (2007). Dissecting a complex trait to simpler components for effective breeding of sorghum with a high level of Striga resistance. integrating new technologies for Striga control: towards ending the witch-hunt. World Sci., 87–98. doi: 10.1142/9789812771506_0007
Fang, C., Luo, J. (2019). Metabolic GWAS-based dissection of genetic bases underlying the diversity of plant metabolism. Plant J. 97, 91–100. doi: 10.1111/tpj.14097
Feng, C., Yuan, J., Wang, R., Liu, Y., Birchler, J. A., Han, F. (2016). Efficient targeted genome modification in maize using CRISPR/Cas9 system. J. Genet. Genomics 43, 37–43. doi: 10.1016/j.jgg.2015.10.002
Fernbach, P. M., Light, N., Scott, S. E., Inbar, Y., Rozin, P. (2019). Extreme opponents of genetically modified foods know the least but think they know the most. Nat. Hum. Behav. 3, 251–256. doi: 10.1038/s41562-018-0520-3
Flavio, B. (2013). Traditional and modern plant breeding methods with examples in rice (Oryza sativa l.). J. Agric. Food Chem. 61, 8277–8286. doi: 10.1021/jf305531j
Food and Agriculture Organization of the United Nations. FAOSTAT Statistical Database (2022). https://www.fao.org/faostat/en/#data
Garcia, A. A., Benchimol, L. L., Barbosa, A. M., Geraldi, I. O., Souza, C. L., Jr., Souza, A. (2004). Comparison of RAPD, RFLP, AFLP, and SSR markers for diversity studies in tropical maize inbred lines. Genet. Mol. Biol. 27, 579–588. doi: 10.1590/S1415-47572004000400019
Gasura, E., Nyandoro, B., Mabasa, S., Setimela, P. S., Kyalo, M., Yao, N. (2021). Breeding strategy for resistance to Striga asiatica (L.) kuntze based on genetic diversity and population structure of tropical maize (Zea mays l.) lines. Genet. Resour. Crop Evol. 69, 987–996. doi: 10.1007/s10722-021-01274-6
Gasura, E., Setimela, P., Mabasa, S., Rwafa, R., Kageler, S., Nyakurwa, C. (2019). Response of IITA maize inbred lines bred for Striga hermonthica resistance to Striga asiatica and associated resistance mechanisms in southern Africa. Euphytica 215, 15. doi: 10.1007/s10681-019-2467-5
Gedil, M., Menkir, A. (2019). An integrated molecular and conventional breeding scheme for enhancing genetic gain in maize in Africa. Front. Plant Sci. 10, 1430. doi: 10.3389/fpls.2019.01430
Gethi, J., Smith, M. E. (2004). Genetic responses of single crosses of maize to Striga hermonthica (Del.) benth. and Striga asiatica (L.) kuntze. Crop Sci. 44.6, 2068–2077. doi: 10.2135/cropsci2004.2068
Gobena, D., Shimels, M., Rich, P. J., Ruyter-Spira, C., Bouwmeester, H., Kanuganti, S., et al. (2017). Mutation in sorghum low germination stimulant alters strigolactones and causes Striga resistance. Proc. Natl. Acad. Sci. United States America 114, 4471–4476. doi: 10.1073/pnas.1618965114
Gowda, M., Makumbi, D., Das, B., Nyaga, C., Kosgei, T., Crossa, J., et al. (2021). Genetic dissection of Striga hermonthica (Del.) benth. resistance via genome-wide association and genomic prediction in tropical maize germplasm. Theor. Appl. Genet. 134, 941–958. doi: 10.1007/s00122-020-03744-4
Granier, C., Aguirrezabal, L., Chenu, K., Cookson, S. J., Dauzat, M., Hamard, P., et al. (2006). PHENOPSIS, an automated platform for reproducible phenotyping of plant responses to soil water deficit in Arabidopsis thaliana permitted the identification of an accession with low sensitivity to soil water deficit. New Phytology 169, 623–635. doi: 10.1111/j.1469-8137.2005.01609.x
Gull, A., Lone, A. A., Wani, N. U. I. (2019). Biotic and abiotic stresses in plants. Abiotic biotic Stress Plants, 1–9. doi: 10.5772/intechopen.85832
Gurney, A. L., Grimanelli, D., Kanampiu, F., Hoisington, D., Scholes, J. D., Press, M. C. (2003). Novel sources of resistance to Striga hermonthica in Tripsacum dactyloides, a wild relative of maize. New Phytol. 160, 557–568. doi: 10.1046/j.1469-8137.2003.00904.x
Gurney, A. L., Press, M. C., Scholes, J. D. (2002). Can wild relatives of sorghum provide new sources of resistance or tolerance against Striga species? Weed Res. 42 (4), 317–324. doi: 10.1093/jxb/46.12.1817
Gurney, A. L., Slate, J., Press, M. C., Scholes, J. D. (2006). A novel form of resistance in rice to the angiosperm parasite Striga hermonthica. New Phytol. 169, 199–208. doi: 10.1111/j.1469-8137.2005.01560.x
Haussmann, B. I., Hess, D. E., Welz, H.-G., Geiger, H. H. (2000). Improved methodologies for breeding Striga-resistant sorghums. Field Crops Res. 66, 195–211. doi: 10.1016/S0378-4290(00)00076-9
Hawkes, J. G. (1991). The importance of genetic resources in plant breeding. Biol. J. Linn. Soc. 43 (1), 3–10. doi: 10.1111/j.1095-8312.1991.tb00578.x
Hearne, S. J. (2009). Control - the Striga conundrum. Pest Manage. Sci. 65, 603–614. doi: 10.1002/ps.1735
Hee-Jong, K., Suk-Yoon, K., Thomson, M. (2015). Current technologies in plant molecular breeding. a guide book of plant molecular breeding for researchers Vol. 3 (Springer).
Heinrich, F., Wutke, M., Das, P. P., Kamp, M., Gültas, M., Link, W., et al. (2020). Identification of regulatory SNPs associated with vicine and convicine content of vicia faba based on genotyping by sequencing data using deep learning. Genes 11, 614. doi: 10.3390/genes11060614
Holme, I. B., Gregersen, P. L., Brinch-Pedersen, H. (2019). Induced genetic variation in crop plants by random or targeted mutagenesis: convergence and differences. Front. inPlant Sci. 10, 1468. doi: 10.3389/fpls.2019.01468
Hongbete, F., Kindossi, J. M., Hounhouigan, J. D., Nago, M. C. (2017). Production et qualité nutritionnelle des épis de maïs frais bouillis consommés au bénin. Int. J. Biol. Chem. Sci. 11 (5), 2378–2392. doi: 10.4314/ijbcs.v11i5.34
Hua, K., Zhang, J., Botella, J. R., Ma, C., Kong, F., Liu, B., et al. (2019). Perspectives on the application of genome-editing technologies in crop breeding. Mol. Plant 12, 1047–1059. doi: 10.1016/j.molp.2019.06.009
Huang, C., Shen, C., Wen, T., Gao, B., Zhu, D., Li, D., et al. (2021). Genome-wide association mapping for agronomic traits in an 8-way upland cotton MAGIC population by SLAF-seq. Theor. Appl. Genet. 134, 2459–2468. doi: 10.1007/s00122-021-03835-w
Ifie, B. E., Badu-Apraku, B., Gracen, V., Danquah, E. Y. (2015). Genetic analysis of grain yield of IITA and CIMMYT early-maturing maize inbreds under Striga-infested and low-soil-nitrogen environments. Crop Sci. 55, 610–623. doi: 10.2135/cropsci2014.07.0470
Igathinathane, C., Pordesimo, L. O., Batchelor, W. D. (2009). Major orthogonal dimensions measurement of food grains by machine vision using image J. Food Res. Int. 42, 76–84. doi: 10.1016/j.foodres.2008.08.013
Isaac, K. G., Allan, P. G., Charles, T. H., Fran, R. B., Catherine, J. H. (2019). A comparative assessment of the performance of a stay-green sorghum (Sorghum bicolor (L) moench) introgression line developed by marker-assisted selection and its parental lines. Afr. J. Biotechnol. 18, 548–563. doi: 10.5897/AJB2019.16826
Ishii, T., Karimi-Ashtiyani, R., Houben, A. (2016). Haploidization via chromosome elimination: means and mechanisms. Annu. Rev. Plant Biol. 67, 421–438. doi: 10.1146/annurev-arplant-043014-114714
Jaccoud, D., Peng, K., Feinstein, D., Kilian, A. (2001). Diversity arrays: a solid-state technology for sequence information independent genotyping. Nucleic Acids Res. 29 (9), e45–e45. doi: 10.1093/nar/29.4.e25
Jamil, M., Kanampiu, F. K., Karaya, H., Charnikhova, T., Bouwmeester, H. J. (2012). Striga hermonthica parasitism in maize in response to n and p fertilizers. Field Crops Res. 134, 1–10. doi: 10.1016/j.fcr.2012.03.015
Jamil, M., Rodenburg, J., Charnikhova, T., Bouwmeester, H. J. (2011). Pre-attachment Striga hermonthica resistance of new rice for Africa (NERICA) cultivars based on low strigolactone production. New Phytol. 192, 964–975. doi: 10.1111/j.1469-8137.2011.03850.x
Jamil, M., Wang, J. Y., Yonli, D., Ota, T., Berqdar, L., Traore, H., et al. (2022). Striga hermonthica suicidal germination activity of potent strigolactone analogs: evaluation from laboratory bioassays to field trials. Plants (Basel) 11 (8), 1045. doi: 10.3390/plants11081045
Jangra, S., Chaudhary, V., Yadav, R. C., Yadav, N. R. (2021). High-throughput phenotyping: a platform to accelerate crop improvement. Phenomics 1, 31–53. doi: 10.1007/s43657-020-00007-6
Jenkins, S., Gibson, N. (2002). High-throughput SNP genotyping. Comp. Funct. Genomics 3, 57–66. doi: 10.1002/cfg.130
Jiang, S. Y., Ramachandran, S. (2010). Assigning biological functions to rice genes by genome annotation, expression analysis, and mutagenesis. Biotechnol. Lett. 32, 1753–1763. doi: 10.1007/s10529-010-0377-7
Jin, X., Zarco-Tejada, P. J., Schmidhalter, U., Reynolds, M. P., Hawkesford, M. J., Varshney, R. K., et al. (2021). High-throughput estimation of crop traits: a review of ground and aerial phenotyping platforms. IEEE Geosci. Remote Sens. Magazine 9, 200–231. doi: 10.1109/MGRS.2020.2998816
Joshi, B. K. (2017). Plant breeding in Nepal past present and future. J. Agric. Forestry Univ. 1, 1–33.
Joshi, A. K., Kumari, M., Singh, V. P., Reddy, C. M., Kumar, S., Rane, J., et al. (2006). Stay green trait: variation, inheritance and its association with spot blotch resistance in spring wheat (Triticum aestivum l.). Euphytica 153, 59–71. doi: 10.1007/s10681-006-9235-z
Kamal, N. M., Alnor Gorafi, Y. S., Abdelrahman, M., Abdellatef, E., Tsujimoto, H. (2019). Stay-green trait: a prospective approach for yield potential, and drought and heat stress adaptation in globally important cereals. Internatioanl J. Mol. Sci. 20, 1–26. doi: 10.3390/ijms20235837
Kamara, A. Y., Menkir, A., Fakorede, M. A. B., Ajala, S. O., Badu-Apraku, B., Kureh, I. (2005). Agronomic performance of maize cultivars representing three decades of breeding in the Guinea savannas of West and central Africa. J. Agric. Sci. 142, 567–575. doi: 10.1017/S0021859604004575
Kanampiu, F., Kanampiua, Ransoma, J. K., Gressel, J. (2001). Imazapyr seed dressings for Striga control on acetolactate synthase target-site resistant maize. Crop Prot. 20, 885–895. doi: 10.1016/S0261-2194(01)00038-2
Kanampiu, F., Omanya, G., Muchiri, N., Nang’ayo, F., Werehire, P., Tyrell, D., et al. (2005). Launch of STRIGAWAY (IR-maize) technology for Striga control in Africa. (Nairobi Kenya: CIMMYT) 5–7.
Karaya, H., Njoroge, K., Mugo, S. N., Ariga, E. S., Kanampiu, F., Nderitu, J. H. (2012). Determination of levels of Striga germination stimulants for maize gene bank accessions and elite inbred lines. Int. J. Plant Production 6, 209–224.
Kasoma, C., Shimelis, H., Laing, M. D., Shayanowako, A. I. T., Mathew, I. (2020). Revealing the genetic diversity of maize (Zea mays l.) populations by phenotypic traits and DArTseq markers for variable resistance to fall armyworm. Genet. Resour. Crop Evol. 68, 243–259. doi: 10.1007/s10722-020-00982-9
Kassahun, B., Bidinger, F. R., Hash, C. T., Kuruvinashetti, M. S. (2009). Stay-green expression in early generation sorghum [Sorghum bicolor (L.) moench] QTL introgression lines. Euphytica 172, 351–362. doi: 10.1007/s10681-009-0108-0
Kebede, D., Mengesha, W., Menkir, A., Abe, A., Garcia-Oliveira, A. L., Gedil, M. (2021). Marker-based enrichment of provitamin a content in two tropical maize synthetics. Sci. Rep. 11, 14998. doi: 10.1038/s41598-021-94586-7
Kilian, A., Wenzl, P., Huttner, E., Carling, J., Xia, L., Blois, H., et al. (2012). Diversity arrays technology: a generic genome profiling technology on open platforms. Methods Mol. Biol. 888, 67–89. doi: 10.1007/978-1-61779-870-2_5
Kim, S. K. (1994). Genetics of maize tolerance of Striga-hermonthica. Crop Sci. 34, 900–907. doi: 10.2135/cropsci1994.0011183X003400040012x
Kim, S. K. (1996). Horizontal resistance: core to a research breakthrough to combat Striga in Africa. Integrated Pest Manage. Rev. 1 (4), 229–249. doi: 10.1007/BF00139766
Kim, S., Akintunde, A., Walker, P. (1999). Responses of maize inbreds during development of Striga hermontica infestation. Maydica. 44 (4), 333–9.
Kim, S. K., Fajemisin, J. M., Thé, C., Adepoju, A., Kling, J., Badu-Apraku, B., et al. (1998). Development of synthetic maize populations for resistance to Striga hermonthica. Plant Breed. 117 (3), 203–209. doi: 10.1111/j.1439-0523.1998.tb01927.x
Kiruki, S., Onek, L. A., Limo, M. (2006). Azide-based mutagenesis suppresses Striga hermonthica seed germination and parasitism on maize varieties. Afr. J. Biotechnol. 5, 866–870.
Klumper, W., Qaim, M. (2014). A meta-analysis of the impacts of genetically modified crops. PloS One 9, e111629. doi: 10.1371/journal.pone.0111629
Knecht, A. C., Campbell, M. T., Caprez, A., Swanson, D. R., Walia, H. (2016). Image harvest: an open-source platform for high-throughput plant image processing and analysis. J. Exp. Botanic 67, 3587–3599. doi: 10.1093/jxb/erw176
Konate, L., Baffour, B. A., Traore, D. (2017). Combining ability and heterotic grouping of early maturing provitamin a maize inbreds across Striga-infested and optimal growing environments. J. Agric. Environ. Int. Dev. 111, 157–173. doi: 10.12895/jaeid.20171.572
Korte, A., Farlow, A. (2013). The advantages and limitations of trait analysis with GWAS: a review. Plant Methods 9, 1–9. doi: 10.1186/1746-4811-9-29
Kountche, B. A., Hash, C. T., Dodo, H., Laoualy, O., Sanogo, M. D., Timbeli, A., et al. (2013). Development of a pearl millet Striga-resistant gene pool: response to five cycles of recurrent selection under Striga-infested field conditions in West Africa. Field Crops Res. 154, 82–90. doi: 10.1016/j.fcr.2013.07.008
Kountche, B. A., Jamil, M., Yonli, D., Nikiema, M. P., Blanco-Ania, D., Asami, T., et al. (2019). Suicidal germination as a control strategy for Striga hermonthica (Benth.) in smallholder farms of sub-Saharan Africa. Plants People Planet 1, 107–118. doi: 10.1002/ppp3.32
Kovalchuk, I., Kovalchuk, O., Hohn, B. (2000). Genome-wide variation of the somatic mutation frequency in transgenic plants. EMBO J. 19, 4431–4438. doi: 10.1093/emboj/19.17.4431
Kozjak, P., Meglič, V. (2012). Mutagenesis in plant breeding for disease and pest resistance. Mutagenesis, 195–220.
Ku, H. K., Ha, S. H. (2020). Improving nutritional and functional quality by genome editing of crops: status and perspectives. Front. Plant Sci. 11, 577313. doi: 10.3389/fpls.2020.577313
Kumar, V., Shukla, Y. M. (2014). Pre-breeding: its applications in crop improvement. Double Helix Res. 16, 199–202.
Lee, Y.-R., Kim, C. W., Han, J., Choi, H. J., Han, K., Lee, E. S., et al. (2021). Genotyping-by-Sequencing derived genetic linkage map and quantitative trait loci for sugar content in onion (Allium cepa l.). Plants 10, 2267. doi: 10.3390/plants10112267
Li, C., Dong, L., Durairaj, J., Guan, J. C., Yoshimura, M., Quinodoz, P., et al. (2023). Maize resistance to witchweed through changes in strigolactone biosynthesis. Science 379, 94–99. doi: 10.1126/science.abq4775
Lipka, A. E., Tian, F., Wang, Q., Peiffer, J., Li, M., Bradbury, P. J., et al. (2012). GAPIT: genome association and prediction integrated tool. Bioinformatics 28, 2397–2399. doi: 10.1093/bioinformatics/bts444
Lobulu, J., Shimelis, H., Laing, M., Mushongi, A. A. (2019). Maize production constraints, traits preference and current Striga control options in western Tanzania: farmers’ consultation and implications for breeding. Acta Agriculturae Scandinavica Section B-Soil Plant Sci. 69, 734–746. doi: 10.1080/09064710.2019.1652680
Lopez-Raez, J. A., Matusova, R., Cardoso, C., Jamil, M., Charnikhova, T., Kohlen, W., et al. (2009). Strigolactones: ecological significance and use as a target for parasitic plant control. Pest Manage. Sci. 65, 471–477. doi: 10.1002/ps.1692
Luche, H., Silva, J.A.G.d., Nörnberg, R., Silveira, S.F.S.d., Baretta, D., et al. (2013). Desempenho per se e parâmetros genéticos de linhagens de trigo com expressão do caráter “stay−green”. Pesquisa Agropecuária Bras. 48, 167–173. doi: 10.1590/S0100-204X2013000200006
Mackelprang, R., Lemaux, P. G. (2020). Genetic engineering and editing of plants: an analysis of new and persisting questions. Annu. Rev. Plant Biol. 71, 659–687. doi: 10.1146/annurev-arplant-081519-035916
Makanza, R., Zaman-Allah, M., Cairns, M., Eyre, J. E., Burgueño, J., Pacheco, J., et al. (2018). High-throughput method for ear phenotyping and kernel weight estimation in maize using ear digital imaging. Plant Methods 14 (1), 1–13. doi: 10.1186/s13007-018-0317-4
Maluszynski, M., Nichterlein, K., Zanten, L. V., Ahloowalia, B. S. (2000). Officially released mutant varieties-the FAO/IAEA database. Mutat. Breed. Rev. 12, 2–84.
Matova, P. M., Kamutando, C. N., Warburton, M. L., Williams, W. P., Magorokosho, C., Shimelis, H., et al. (2022). New techniques for breeding maize (Zea mays) varieties with fall armyworm resistance and market-preferred traits for sub-Saharan Africa. Plant Breed. 142 (1), 1–11. doi: 10.1111/pbr.13063
Mayor, P. J., Bernardo, R. (2009). Genome-wide selection and marker-assisted recurrent selection in doubled haploid versus F2 populations. Crop Sci. 49, 1719–1725. doi: 10.2135/cropsci2008.10.0587
Mengesha, W. A., Menkir, A., Unakchukwu, N., Meseka, S., Farinola, A., Girma, G., et al. (2017). Genetic diversity of tropical maize inbred lines combining resistance to Striga hermonthica with drought tolerance using SNP markers. Plant Breed. 136, 338–343. doi: 10.1111/pbr.12479
Menkir, A., Adetimirin, V. O., Yallou, C. G., Gedil, M. (2010). Relationship of genetic diversity of inbred lines with different reactions to Striga hermonthica (Del.) benth and the performance of their crosses. Crop Sci. 50, 602–611. doi: 10.2135/cropsci2009.05.0247
Menkir, A., Kling, J. G. (2007). Response to recurrent selection for resistance to Striga hermonthica (Del.) benth in a tropical maize population. Crop Sci. 47, 674–684. doi: 10.2135/cropsci2006.07.0494
Menkir, A., Kling, J. G., Badu-Apraku, B., Ibikunle, O. (2006). Registration of 26 tropical maize germplasm lines with resistance to Striga hermonthica. Crop Sci. 46 (2), 1007. doi: 10.2135/cropsci2005.0143
Menkir, A., Kling, J. G., Badu-Apraku, B., Ingelbrecht, I. (2005). Molecular marker-based genetic diversity assessment of Striga-resistant maize inbred lines. Theor. Appl. Genet. 110, 1145–1153. doi: 10.1007/s00122-005-1946-3
Menkir, A., Makumbi, D., Franco, J. (2012). Assessment of reaction patterns of hybrids to Striga hermonthica (Del.) benth. under artificial infestation in Kenya and Nigeria. Crop Sci. 52, 2528–2537. doi: 10.2135/cropsci2012.05.0307
Menkir, A., Meseka, S. (2019). Genetic improvement in resistance to Striga in tropical maize hybrids. Crop Sci. 59, 2484–2497. doi: 10.2135/cropsci2018.12.0749
Meseka, S., Fakorede, M., Ajala, S., Badu-Apraku, B., Menkir, A. (2013). Introgression of alleles from maize landraces to improve drought tolerance in an adapted germplasm. J. Crop Improvement 27, 96–112. doi: 10.1080/15427528.2012.729259
Michel, S., Ametz, C., Gungor, H., Akgöl, B., Epure, D., Grausgruber, H., et al. (2017). Genomic assisted selection for enhancing line breeding: merging genomic and phenotypic selection in winter wheat breeding programs with preliminary yield trials. Theor. Appl. Genet. 130, 363–376. doi: 10.1007/s00122-016-2818-8
Midega, C. A. O., Pickett, J., Hooper, A., Pittchar, J., Khan, Z. R. (2016). Maize landraces are less affected by Striga hermonthica relative to hybrids in Western Kenya. Weed Technol. 30, 21–28. doi: 10.1614/WT-D-15-00055.1
Miller, N. D., Haase, N. J., Lee, J., Kaeppler, S. M., de Leon, N., Spalding, E. P. (2017). A robust, high-throughput method for computing maize ear, cob, and kernel attributes automatically from images. Plant J. 89, 169–178. doi: 10.1111/tpj.13320
Mishra, S., Upadhyay, S., Shukla, R. K. (2016). The role of strigolactones and their potential cross-talk under hostile ecological conditions in plants. Front. Physiol. 7, 691. doi: 10.3389/fphys.2016.00691
Mitiku, T. (2022). Review on haploid and double haploid maize (Zea mays) breeding technology. Int. J. Agric. Sci. Food Technol. 8 (1), 052–058. doi: 10.17352/ijasft
Mittler, R., Blumwald, E. (2010). Genetic engineering for modern agriculture: challenges and perspectives. Annu. Rev. Plant Biol. 61, 443–462. doi: 10.1146/annurev-arplant-042809-112116
Mondini, L., Noorani, A., Pagnotta, M. (2009). Assessing plant genetic diversity by molecular tools. Diversity 1, 19–35. doi: 10.3390/d1010019
Mrema, E., Shimelis, H., Laing, M., Bucheyeki, T. (2017). Farmers’ perceptions of sorghum production constraints and Striga control practices in semi-arid areas of Tanzania. Int. J. Pest Manage. 63, 146–156. doi: 10.1080/09670874.2016.1238115
Mueni, M. I. (2018). Developing striga resistance in maize through maize-sorghum hybridization (kenyatta university), 96.
Mutinda, S. M., Masanga, J., Mutuku, J. M., Runo, S., Alakonya, A. (2018). KSTP 94, an open-pollinated maize variety has postattachment resistance to purple witchweed (Striga hermonthica). Weed Sci. 66, 525–529. doi: 10.1017/wsc.2018.24
Myles, S., Peiffer, J., Brown, P. J., Ersoz, E. S., Zhang, Z., Costich, D. E., et al. (2009). Association mapping: critical considerations shift from genotyping to experimental design. Plant Cell 21, 2194–2202. doi: 10.1105/tpc.109.068437
Nadeem, M. A., Nawaz, M. A., Shahid, M. Q., Doğan, Y., Comertpay, G., Yıldız, M., et al. (2017). DNA Molecular markers in plant breeding: current status and recent advancements in genomic selection and genome editing. Biotechnol. Biotechnol. Equip. 32, 261–285. doi: 10.1080/13102818.2017.1400401
Nazeer, W., Ali, Z., Ali, A., Hussain, T. (2010). Genetic behaviour for some polygenic yield contributing traits in wheat (Triticum aestivum l.). J. Agric. Res. 03681157), 48.
Negro, S. S., Millet, E. J., Madur, D., Bauland, C., Combes, V., Welcker, C., et al. (2019). Genotyping-by-sequencing and SNP-arrays are complementary for detecting quantitative trait loci by tagging different haplotypes in association studies. BMC Plant Biol. 19, 318. doi: 10.1186/s12870-019-1926-4
Nelimor, C., Badu-Apraku, B., Nguetta, S. P., Tetteh, A. Y., Garcia-Oliveira, A. L. (2020). Phenotypic characterization of maize landraces from sahel and coastal West Africa reveals marked diversity and potential for genetic improvement. J. Crop Improvement 34, 122–138. doi: 10.1080/15427528.2019.1674760
Ngugi, K., Ngugi, A. J., Osama, S., Mugoya, C. (2015). Combating Striga weed in sorghum by transferring resistance quantitative trait loci through molecular marker-assisted introgression. J. Plant Breed. Genet. 3, 67–76. doi: 10.1016/j.ijfoodmicro.2008.12.030
Okora, J., Ngesa, H., Ayako, P. (2006). Development of striga-resistant maize for western Kenya (KARI).
Okunlola, G., Badu-Apraku, B., Ariyo, O., Agre, P., Offernedo, Q., Ayo-Vaughan, M. (2023). Genome-wide association studies of Striga resistance in extra-early maturing quality protein maize inbred lines. G3 (Bethesda) 13 (2), jkac237. doi: 10.1093/g3journal/jkac237
Oluwaranti, A., Salami, A., Bankole, F., Akintayo, V., Eyiowuawi, O. (2020). Genotypic response of maize to micro and macro nutrients as influenced by Arbuscular mycorrhiza fungi (glomus facultative) in a rainforest location. Ife J. Agric. 32 (3), 45–59.
Osei, M. K., Asante, M. D., Agyeman, A., Adebayo, M. A., Adu-Dapaah, H. (2014). “Plant breeding: a tool for achieving food sufficiency,” in Sustainable horticultural systems (Switzerland: Springer, Cham), 253–274.
Oswald, A., Ransom, J. K. (2002). Response of maize varieties to transplanting in Striga-infested fields. Weed Sci. 50, 392–396. doi: 10.1614/0043-1745(2002)050[0392:ROMVTT]2.0.CO;2
Parker, C. (2012). Parasitic weeds: a world challenge. Weed Sci. 60, 269–276. doi: 10.1614/WS-D-11-00068.1
Paul, W. L., Canon, N. S. (2008). Striga management through herbicide resistance. A Public-Private Partnership in Action 307, 489–496.
Pellegrino, E., Bedini, S., Nuti, M., Ercoli, L. (2018). Impact of genetically engineered maize on agronomic, environmental and toxicological traits: a meta-analysis of 21 years of field data. Sci. Rep. 8, 3113. doi: 10.1038/s41598-018-21284-2
Pfunye, A., Rwafa, R., Mabasa, S., Gasura, E. (2021). Genome-wide association studies for Striga asiatica resistance in tropical maize. Int. J. Genomics 2021, 8. doi: 10.1155/2021/9979146
Phuke, R. M., Bhoyar, P. I., Ambati, D., Udupa, S., Gaddameedi, A., Gupta, V., et al. (2022). “Genome-wide association studies and genomic predictions for climate change resilience in wheat,” in Next-generation plant breeding approaches for stress resilience in cereal crops (Singapore: Springer.), 95–120.
Powell, W., Morgante, M., Andre, C., Hanafey, M., Vogel, J., Tingey, S., et al. (1996). The comparison of RFLP, RAPD, AFLP and SSR (microsatellite) markers for germplasm analysis. Mol. Breed. 2, 225–238. doi: 10.1007/BF00564200
Prasanna, B. M. (2014). Maize research-for-development scenario: challenges and opportunities for Asia 12th Asian maize conference and expert consultation on maize for food, feed, and nutritional security. Book of Extended Summaries 30, 2–11.
Prasanna, B. M., Araus, J. L., Crossa, J., Cairns, J. E., Palacios, N., Das, B., et al. (2013). “High-throughput and precision phenotyping for cereal breeding programs,” in Cereal genomics II (Dordrecht: Springer), 341–374.
Prigge, V., Sánchez, C., Dhillon, B. S., Schipprack, W., Araus, J. L., Bänziger, M., et al. (2011). Doubled haploids in tropical maize: effects of inducers and source germplasm on in vivo haploid induction rates. Crop Sci. 51, 1498–1506. doi: 10.2135/cropsci2010.10.0568
Prigge, V., Schipprack, W., Mahuku, G., Atlin, G. N., Melchinger, A. E. (2012). Development of in vivo haploid inducers for tropical maize breeding programs. Euphytica 185, 481–490. doi: 10.1007/s10681-012-0657-5
Rachidatou, S., Serge, N., Jean, A., Jean, B. (2018). Fiche technique de reconnaissance des mauvaises herbes en culture du maïs au bénin et méthodes de lutte. Plant Prot. 28, 16.
Ragot, M., Lee, M., Guimaraes, E. (2007). “Marker-assisted selection in maize: current status, potential, limitations, and perspectives from the private and public sectors,” in Marker-assisted selection: current status and future perspectives in crops, livestock, forestry, and fish (Rome: Food and Agriculture Organization of the United Nations Rome), 117–150.
Rahim, F. (2019). Characterization of Zea mays l. through morphological, biochemical and molecular markers. Appl. Ecol. Environ. Res. 17 (3), 6445–6456. doi: 10.15666/aeer/1703_64456456
Rajesh, M., Ramesh, S., Perera, L., Manickavelu, A. (2021). “Quantitative trait loci (QTL) and association mapping for major agronomic traits,” in The coconut genome (Switzerland: Springer), 91–101.
Ramaiah, K. (1986). Summary report of striga research carried out in Burkina Faso from 1979 to 1985 (Burkina-Faso: ICRISAT/Burkina Faso Cooperative Program), 4881. ICRISAT, BP.
Raman, H., Raman, R., Kilian, A., Detering, F., Carling, J., Coombes, N., et al. (2014). Genome-wide delineation of natural variation for pod shatter resistance in Brassica napus. PloS One 9, 101–673. doi: 10.1371/journal.pone.0101673
Ranum, P., Pena-Rosas, J. P., Garcia-Casal, M. N. (2014). Global maize production, utilization, and consumption. Ann. New York Acad. Sci. 1312, 105–112. doi: 10.1111/nyas.12396
Reda, F., Verkleij, J. (2004). The biology and control of Striga: a review. pest management. J. Ethiopia (Ethiopia) 8, 1–13.
Reif, J., Hallauer, A., Melchinger, A. (2005). Heterosis and heterotic patterns in maize. Maydica 50, 215–223.
Ribaut, J. M., Ragot, M. (2007). Marker-assisted selection to improve drought adaptation in maize: the backcross approach, perspectives, limitations, and alternatives. J. Exp. Botanic 58, 351–360. doi: 10.1093/jxb/erl214
Rich, P. J., Ejeta, G. (2008). Towards effective resistance to Striga in African maize. Plant Signaling Behav. 3, 618–621. doi: 10.4161/psb.3.9.5750
Rich, P. J., Grenier, U., Ejeta, G. (2004). Striga resistance in the wild relatives of sorghum. Crop Sci. 44, 2221–2229. doi: 10.2135/cropsci2004.2221
Rincent, R., Charpentier, J. P., Faivre-Rampant, P., Paux, E., Le Gouis, J., Bastien, C., et al. (2018). Phenomic selection is a low-cost and high-throughput method based on indirect predictions: proof of concept on wheat and poplar. G3 (Bethesda) 8, 3961–3972. doi: 10.1534/g3.118.200760
Rodenburg, J., Bastiaans, L. (2011). Host-plant defense against Striga spp.: reconsidering the role of tolerance. Weed Res. 51, 438–441. doi: 10.1111/j.1365-3180.2011.00871.x
Sachs, M. M. (2009). Maize genetic resources. Biotechnol. Agric. Forestry 63, 197–209. doi: 10.1007/978-3-540-68922-5_14
Sahu, V. K. (2020). Perspectives of genetic engineering in agriculture. Advance Biotechnol. bioscience 6, 121. doi: 10.22271/ed.book.908
Sanchez, A. C., Subudhi, P. K., Rosenow, D. T., Nguyen, H. T. (2002). Mapping QTLs associated with drought resistance in sorghum (Sorghum bicolor l. Moench). Plant Mol. Biol. 48 (5), 713–726. doi: 10.1023/A:1014894130270
Sanchez-Sevilla, J. F., Horvath, A., Botella, M. A., Gaston, A., Folta, K., Kilian, A., et al. (2015). Diversity arrays technology (DArT) marker platforms for diversity analysis and linkage mapping in a complex crop, the octoploid cultivated strawberry (Fragaria x ananassa). PloS One 10, 144–960. doi: 10.1371/journal.pone.0144960
Schrag, T. A., Melchinger, A. E., Sorensen, A. P., Frisch, M. (2006). Prediction of single-cross hybrid performance for grain yield and grain dry matter content in maize using AFLP markers associated with QTL. Theor. Appl. Genet. 113, 1037–1047. doi: 10.1007/s00122-006-0363-6
Schuster, I. (2011). Marker-assisted selection for quantitative traits. Crop Breed. Appl. Biotechnol. S1, 50–55. doi: 10.1590/S1984-70332011000500008
Segui-Simarro, J. M., Jacquier, N. M. A., Widiez, T. (2021). Overview of In vitro and In vivo doubled haploid technologies. Methods Mol. Biol. 2287, 3–22. doi: 10.1007/978-1-0716-1315-3_1
Semagn, K., Magorokosho, C., Vivek, B. S., Makumbi, D., Beyene, Y., Mugo, S., et al. (2012). Molecular characterization of diverse CIMMYT maize inbred lines from eastern and southern Africa using single nucleotide polymorphic markers. BMC Genomics 13 (1), 1–11. doi: 10.1186/1471-2164-13-113
Shaibu, A. S., Badu-Apraku, B., Ayo-Vaughan, M. A. (2021). Enhancing drought tolerance and Striga hermonthica resistance in maize using newly derived inbred lines from the wild maize relative, Zea diploperennis. Agronomy-Basel 11, 21. doi: 10.3390/agronomy11010177
Shayanowako, A. I.T., Shimelis, H., Laing, M. D., Mwadzingeni, L. (2020). Striga resistance and compatibility of maize genotypes to a biocontrol agent, Fusarium oxysporum f.sp.strigea. Journal of Crop Improvement 34, 437–454.
Shayanowako, A. T., Laing, M., Shimelis, H., Mwadzingeni, L. (2018b). Resistance breeding and biocontrol of Striga asiatica (L.) kuntze in maize: a review. Acta Agriculturae Scandinavica Section B-Soil Plant Sci. 68, 110–120. doi: 10.1080/09064710.2017.1370493
Shayanowako, A. I. T., Shimelis, H., Laing, M. D., Mwadzingeni, L. (2018a). Genetic diversity of maize genotypes with variable resistance to Striga asiatica based on SSR markers. Cereal Res. Commun. 46, 668–678. doi: 10.1556/0806.46.2018.044
Shiferaw, B., Prasanna, B. M., Hellin, J., Bänziger, M. (2011). Crops that feed the world 6. past successes and future challenges to the role played by maize in global food security. Food Secur. 3, 307–327. doi: 10.1007/s12571-011-0140-5
Shimelis, H., Laing, M. (2012). Timelines in conventional crop improvement: pre-breeding and breeding procedures. Aust. J. Crop Sci. 6 (11), 1542–1549.
Silva, S. A., Carvalho, F. I. F. D., Nedel, J. L., Vasconcellos, N. J. S. D., Cruz, P. J., Simioni, D., et al. (2004). Composição de subunidades de gluteninas de alto peso molecular (HMW) em trigos portadores do caráter” stay-green”. Ciec. Rural 34, 679–683. doi: 10.1590/S0103-84782004000300005
Simon, Z., Kasozi, L. C., Patrick, R., Abubaker, M. (2018). Gene action for grain yield and agronomic traits in selected maize inbred lines with resistance to Striga hermonthica in Uganda. J. Food Secur. 6 (4), 155–162. doi: 10.12691/jfs-6-4-3
Smale, M., Jamora, N. (2020). Valuing genebanks. Food Secur. 12, 905–918. doi: 10.1007/s12571-020-01034-x
Soltabayeva, A., Ongaltay, A., Omondi, J. O., Srivastava, S. (2021). Morphological, physiological, and molecular markers for salt-stressed plants. Plants (Basel) 10, 1–18. doi: 10.3390/plants10020243
Sonah, H., O’Donoughue, L., Cober, E., Rajcan, I., Belzile, F. (2015). Identification of loci governing eight agronomic traits using a GBS-GWAS approach and validation by QTL mapping in soya bean. Plant Biotechnol. J. 13, 211–221. doi: 10.1111/pbi.12249
Stanley, A. E., Menkir, A., Ifie, B., Paterne, A. A., Unachukwu, N. N., Meseka, S., et al. (2021). Association analysis for resistance to Striga hermonthica in diverse tropical maize inbred lines. Scientific Reports 11, 1–14. doi: 10.1038/s41598-021-03566-4
Thomas, H., Ougham, H. (2014). The stay-green trait. J. Exp. Botanic 65, 3889–3900. doi: 10.1093/jxb/eru037
Thomas, L., William, B., Suza, W. (2016). Marker assisted-backcrossing (Africa: Plant Breeding E-Learning), 48.
Tian, F., Bradbury, P. J., Brown, P. J., Hung, H., Sun, Q., Flint-Garcia, S., et al. (2011). Genome-wide association study of leaf architecture in the maize nested association mapping population. Nat. Genet. 43, 159–162. doi: 10.1038/ng.746
Tomkowiak, A., Bocianowski, J., Spychała, J., Grynia, J., Sobiech, A., Kowalczewski, P.Ł. (2021). DArTseq-based high-throughput silicoDArT and SNP markers applied for association mapping of genes related to maize morphology. Int. J. Mol. Sci. 22, 5840. doi: 10.3390/ijms22115840
Trentin, H. U. (2019). Study and improvement of maize maternal haploid inducers (Ames, Lowa: Iowa State University), 138.
Trindade, R. D. S. (2022). The use of doubled haploid technology for lines development in maize breeding. Rev. Bras. Milho e Sorgo 21, 18. doi: 10.18512/rbms2022v21e1289
Tugendhat, E. (2017). Transforming the Uganda maize system (Uganda: Palladium Make it Possible), 18.
Vadez, V., Santosh, D., Kholova, J., Ramu, P., Hash, C. T. (2013). Molecular breeding for stay-green: progress and challenges in sorghum. Trans. Genomics Crop breeding: abiotic stress yield Qual. 2, 125–141. doi: 10.1002/9781118728482.ch8
van Orsouw, N. J., Hogers, R. C., Janssen, A., Yalcin, F., Snoeijers, S., Verstege, E., et al. (2007). Complexity reduction of polymorphic sequences (CRoPS): a novel approach for large-scale polymorphism discovery in complex genomes. PloS One 2, e1172. doi: 10.1371/journal.pone.0001172
Virlet, N., Sabermanesh, K., Sadeghi-Tehran, P., Hawkesford, M. J. (2016). Field scanalyzer: an automated robotic field phenotyping platform for detailed crop monitoring. Funct. Plant Biol. 44, 143–153. doi: 10.1071/FP16163
Wang, A.-y., Li, Y., Zhang, C.-q. (2012). QTL mapping for stay-green in maize (Zea mays). Can. J. Plant Sci. 92, 249–256. doi: 10.4141/cjps2011-108
Wang, Y., Liu, X., Zheng, X., Wang, W., Yin, X., Liu, H., et al. (2021). Creation of aromatic maize by CRISPR/Cas. J. Integr. Plant Biol. 63, 1664–1670. doi: 10.1111/jipb.13105
Wang, H., Qin, F. (2017). Genome-wide association study reveals natural variations contributing to drought resistance in crops. Front. Plant Sci. 8, 1110. doi: 10.3389/fpls.2017.01110
Wanga, M. A., Shimelis, H., Horn, L. N., Sarsu, F. (2020). The effect of single and combined use of gamma radiation and ethylmethane sulfonate on early growth parameters in sorghum. Plants (Basel) 9, 15. doi: 10.3390/plants9070827
Wani, S. H., Samantara, K., Razzaq, A., Kakani, G., Kumar, P. (2022). Back to the wild: mining maize (Zea mays l.) disease resistance using advanced breeding tools. Mol. Biol. Rep. 49 (6), 5787–5803. doi: 10.1007/s11033-021-06815-x
White, T. L. (2004). “Tree breeding principles: breeding theory and genetic testing,” in Encyclopedia of forest sciences (Elsevier), 1551–1561.
Wilde, K., Burger, H., Prigge, V., Presterl, T., Schmidt, W., Ouzunova, M., et al. (2010). Testcross performance of doubled-haploid lines developed from European flint maize landraces. Plant Breed. 129, 181–185. doi: 10.1111/j.1439-0523.2009.01677.x
Wilson, J. P., Hess, D. E., Hanna, W. W. (2000). Resistance to Striga hermonthica in wild accessions of the primary gene pool of Pennisetum glaucum. Phytopathology 90 (10), 1169–1172. doi: 10.1094/PHYTO.2000.90.10.1169
Woomer, P. L., Bokanga, M., Odhiambo, G. D. (2008). Striga management and the African farmer. Outlook Agric. 37 (4), 277–282. doi: 10.5367/000000008787167790
Wumasi, P. T., Tetteh, A. Y., Adade, K. B., Asare, S., Akromah, R. (2017). Morphological diversity and relationships among the IPGRI maize (Zea mays l.) landraces held in IITA. Maydica 62, 1–9.
Xu, Y., Crouch, J. H. (2008). Marker-assisted selection in plant breeding: from publications to practice. Crop Sci. 48, 391–407. doi: 10.2135/cropsci2007.04.0191
Xu, Y., Li, P., Yang, Z., Xu, C. (2017). Genetic mapping of quantitative trait loci in crops. Crop J. 5, 175–184. doi: 10.1016/j.cj.2016.06.003
Yacoubou, A. M., Wallis, N. Z., Menkir, A., Zinsou, V. A., Onzo, A., Garcia-Oliveira, A. L., et al. (2021b). Breeding maize (Zea mays) for Striga resistance: past, current and prospects in sub-Saharan africa. Plant Breed. 140, 195–210. doi: 10.1111/pbr.12896
Yacoubou, A.-M., Wallis, N. Z., Salami, H. A., Yaoitcha, A. S., Menkir, A., Tayo, O., et al. (2021a). Agronomic performance of S1 maize lines derived from a bi-parental cross under infested and striga free environments. Eur. Sci. J. ESJ 17 (25), 306. doi: 10.19044/esj.2021.v17n25p306
Yacoubou, A. M., Wallis, N. Z., Unachukwu, N., Salami, H. A., Hounfodji, N. S., Paterne, A. (2021c). Genetic characterization of early generation lines using SNPS makers and agronomic traits for resistance to Striga improvement in maize. Int. Journals Sci. High Technol. 27 (2), 294–304. doi: 10.19044/esj.2021.v17n25p306
Yang, Z., Li, X., Zhang, N., Wang, X., Zhang, Y., Ding, Y., et al. (2017). Mapping and validation of the quantitative trait loci for leaf stay-green-associated parameters in maize. Plant Breed. 136, 188–196. doi: 10.1111/pbr.12451
Yano, K., Yamamoto, E., Aya, K., Takeuchi, H., Lo, P. C., Hu, L., et al. (2016). Genome-wide association study using whole-genome sequencing rapidly identifies new genes influencing agronomic traits in rice. Nat. Genet. 48, 927–934. doi: 10.1038/ng.3596
Yohannes, T., Abraha, T., Kiambi, D., Folkertsma, R., Hash, C. T., Ngugi, K., et al. (2015). Marker-assisted introgression improves Striga resistance in an eritrean farmer-preferred sorghum variety. Field Crops Res. 173, 22–29. doi: 10.1016/j.fcr.2014.12.008
Yoneyama, K., Arakawa, R., Ishimoto, K., Kim, H. I., Kisugi, T., Xie, X. N., et al. (2015). Difference in Striga-susceptibility is reflected in strigolactone secretion profile, but not in compatibility and host preference in arbuscular mycorrhizal symbiosis in two maize cultivars. New Phytol. 206, 983–989. doi: 10.1111/nph.13375
Zargar, M., Zavarykina, T., Voronov, S., Pronina, I., Bayat, M. (2022). The recent development in technologies for attaining doubled haploid plants in vivo. Agriculture 12 (10), 1595. doi: 10.3390/agriculture12101595
Zebire, D., Menkir, A., Adetimirin, V., Mengesha, W., Meseka, S., Gedil, M. (2021). Identifying suitable tester for evaluating Striga-resistant lines using DArTseq markers and agronomic traits. PloS One 16, 18. doi: 10.1371/journal.pone.0253481
Keywords: doubled haploid, genetic resources, gene editing, genomic resources, maize breeding, quantitative traits loci, Striga species
Citation: Dossa EN, Shimelis H, Mrema E, Shayanowako AIT and Laing M (2023) Genetic resources and breeding of maize for Striga resistance: a review. Front. Plant Sci. 14:1163785. doi: 10.3389/fpls.2023.1163785
Received: 11 February 2023; Accepted: 07 April 2023;
Published: 10 May 2023.
Edited by:
Sambasivam Periyannan, University of Southern Queensland, AustraliaReviewed by:
Andres Garcia Lor, Valencian Institute for Agricultural Research (IVIA), SpainCopyright © 2023 Dossa, Shimelis, Mrema, Shayanowako and Laing. This is an open-access article distributed under the terms of the Creative Commons Attribution License (CC BY). The use, distribution or reproduction in other forums is permitted, provided the original author(s) and the copyright owner(s) are credited and that the original publication in this journal is cited, in accordance with accepted academic practice. No use, distribution or reproduction is permitted which does not comply with these terms.
*Correspondence: Emeline Nanou Dossa, ZG9zc2FlbWVsaW5lQGdtYWlsLmNvbQ==
Disclaimer: All claims expressed in this article are solely those of the authors and do not necessarily represent those of their affiliated organizations, or those of the publisher, the editors and the reviewers. Any product that may be evaluated in this article or claim that may be made by its manufacturer is not guaranteed or endorsed by the publisher.
Research integrity at Frontiers
Learn more about the work of our research integrity team to safeguard the quality of each article we publish.