Erratum: Meta-QTL and haplo-pheno analysis reveal superior haplotype combinations associated with low grain chalkiness under high temperature in rice
- 1Department of Botany, University of Delhi, Delhi, India
- 2Department of Computer Science, Kansas State University, Manhattan, KS, United States
- 3Department of Plant Molecular Biology, University of Delhi, New Delhi, India
- 4Department of Plant Molecular Biology and Biotechnology, Indira Gandhi Krishi Vishwavidyalaya, Chattisgarh, India
- 5Division of Plant Physiology, Indian Council of Agricultural Research (ICAR), New Delhi, India
- 6National Institute for Plant Biotechnology, Indian Council of Agricultural Research (ICAR), New Delhi, India
- 7Centre for Genetic Manipulation of Crop Plants, New Delhi, India
- 8Department of Plant and Soil Science, Texas Tech University, Lubbock, TX, United States
Chalk, an undesirable grain quality trait in rice, is primarily formed due to high temperatures during the grain-filling process. Owing to the disordered starch granule structure, air spaces and low amylose content, chalky grains are easily breakable during milling thereby lowering head rice recovery and its market price. Availability of multiple QTLs associated with grain chalkiness and associated attributes, provided us an opportunity to perform a meta-analysis and identify candidate genes and their alleles contributing to enhanced grain quality. From the 403 previously reported QTLs, 64 Meta-QTLs encompassing 5262 non-redundant genes were identified. MQTL analysis reduced the genetic and physical intervals and nearly 73% meta-QTLs were narrower than 5cM and 2Mb, revealing the hotspot genomic regions. By investigating expression profiles of 5262 genes in previously published datasets, 49 candidate genes were shortlisted on the basis of their differential regulation in at least two of the datasets. We identified non-synonymous allelic variations and haplotypes in 39 candidate genes across the 3K rice genome panel. Further, we phenotyped a subset panel of 60 rice accessions by exposing them to high temperature stress under natural field conditions over two Rabi cropping seasons. Haplo-pheno analysis uncovered haplotype combinations of two starch synthesis genes, GBSSI and SSIIa, significantly contributing towards the formation of grain chalk in rice. We, therefore, report not only markers and pre-breeding material, but also propose superior haplotype combinations which can be introduced using either marker-assisted breeding or CRISPR-Cas based prime editing to generate elite rice varieties with low grain chalkiness and high HRY traits.
1 Highlights
A combination of natural variations in starch metabolism genes SSIIa and GBSSI is associated with low grain chalkiness and high head yield under high temperature in rice.
2 Introduction
Rice (Oryza sativa L.), a primary staple food, is life for about half of the world’s population. Besides providing food security, rice farming generates secure livelihood for millions across the world (Le, 2016). Asia is a major producer as well as consumer of rice accounting for over 90% of world’s production and consumption (www.fao.org; Hossain et al., 2006; Muthayya et al., 2014). Asia (~4.67 billion) followed by Africa (~1.37 billion) are the two most populous continents and even though the population growth rate in Africa is nearly 3 times (2.45%) more than Asia (0.83%), the absolute population increase in Asia far exceeds that of Africa. Notwithstanding that surplus rice is being produced and consumption has been declining in Asia, it is predicted that the exponential increase in population will soon outstrip the incremental gains in rice production. The predicted gap in demand and supply is likely to be further widened by the climate change-accompanied abiotic factors that adversely affect the yield and quality of rice grains. Predictive modeling by the Intergovernmental Panel on Climate Change [IPCC, Sixth Assessment Report, 2021 (www.ipcc.ch)] estimates that even if all man-made activities are ceased, global temperature will continue to rise by 1.5°C to 2°C in the next two decades, which would be catastrophic to agriculture globally, including rice cultivation. Although rice is primarily cultivated in humid tropical and subtropical climates, minor increase in growth temperatures, especially high night-time temperatures, results in reduced spikelet fertility and sub-optimum grain characteristics. This includes, increased chalky grain ratio which causes significant reduction in grain yield and inferior quality attributes (Lyman et al., 2013; Wu et al., 2016).
Rice grains, in their journey from fields to the consumer, are scrutinized on several interrelated grain quality characteristics such as appearance, cooking, palatability and nutritional value. While the intermediaries, such as millers, are primarily interested in higher recovery of intact grain (also known as head rice yield or HRY), the consumer acceptance depends on the flavor, appearance and cooking quality (He et al., 1999). Grain chalk, an opaque and disordered area in the endosperm due to increased air spaces between the malformed starch granules (Lisle et al., 2000) is a highly unacceptable trait that accounts for many inferior grain characteristics. Based on the position of chalk deposition in rice grain, chalkiness is categorized as white-back, white-base, white-belly, white-core and milky-white (Tashiro and Wardlaw, 1991). The degree of chalkiness influences the recovery of head rice and since rice is preferably consumed as an intact whole kernel head rice fetches 40-50% higher price than the broken grains (Cooper et al., 2008; Chen et al., 2011; Zhao and Fitzgerald, 2013; Sreenivasulu et al., 2015). Consequently, besides boosting rice production, improvement of rice grain milling quality, with respect to high HRY and low grain chalkiness, are the key challenges in rice improvement programs worldwide (Nelson et al., 2011; Zhao and Fitzgerald, 2013).
Rice endosperm starch is composed of two main components: amylose and branched chain amylopectin. While granule-bound starch synthase I (GBSSI/Waxy) elongates amylose, soluble starch synthases (SSs), starch branching enzymes (BEs) and starch debranching enzymes (DEBs) are involved in synthesis and branching of amylopectin (Smith et al., 1997). Perturbations in the starch metabolism pathway leads to an aberrant amylose-amylopectin composition and altered starch structure, culminating in formation of chalk (Butardo et al., 2011). Both the expression and activity of starch metabolism genes and proteins, respectively, are modulated by high temperatures for e.g., starch synthesis genes are downregulated, while genes for starch degrading enzymes are upregulated by heat stress (Yamakawa et al., 2007; Liu et al., 2010b; Yamakawa and Hakata, 2010; Lin et al., 2017). Functional analysis studies have identified several genes like pyruvate orthophosphate dikinase (PPDK), starch synthase IIIa (SSIIIa), UDP-glucose pyrophosphorylase (UGPase 1) and cell-wall invertase, associated with chalk formation in rice (Kang et al., 2005; Fujita et al., 2007; Wang et al., 2008; Woo et al., 2008). However, in view of the spatial and temporal complexity of starch synthesis and deposition in developing rice grains, chalkiness appears to be a complex quantitative trait with polygenic inheritance.
Several research groups have mapped quantitative trait loci (QTL) for grain chalkiness and its associated traits (Table 1). Few chalk-associated QTLs have been fine mapped such as Chalk5 that codes for a vacuolar H+-translocating pyrophosphatase and whose enhanced expression contributes to increased chalkiness (Li et al., 2014). Similarly, non-synonymous variations in OsAGPS1 gene within the qACE9 QTL affected chalk accumulation (Gao et al., 2016a). Takehara et al. (2018) dissected the Apq1 QTL to three genes and based on their temporal expression identified OsSUS3 as the candidate gene. However, the majority of QTLs from independent studies have not yet been fine mapped and have overlapping genomic regions owing to variable experimental designs and environment, genetic background of parents, population size, type and density of molecular markers and the statistical approach utilized for downstream analysis. Traditionally, the fine mapping of QTL to mine the causal gene/(s) involves mapping in the advanced generation (e.g., RILs) with an increased number of markers to obtain a condition where chances of recombination between the markers and traits are subtle. An alternative approach that can help in fine mapping, is meta-analysis of QTLs (MQTL) that are identified from multiple studies. MQTL analysis is fast emerging as an effective approach for reducing the confidence intervals (CI) of overlapping QTLs so that promising markers and genes associated with the concern trait could be rapidly mined.
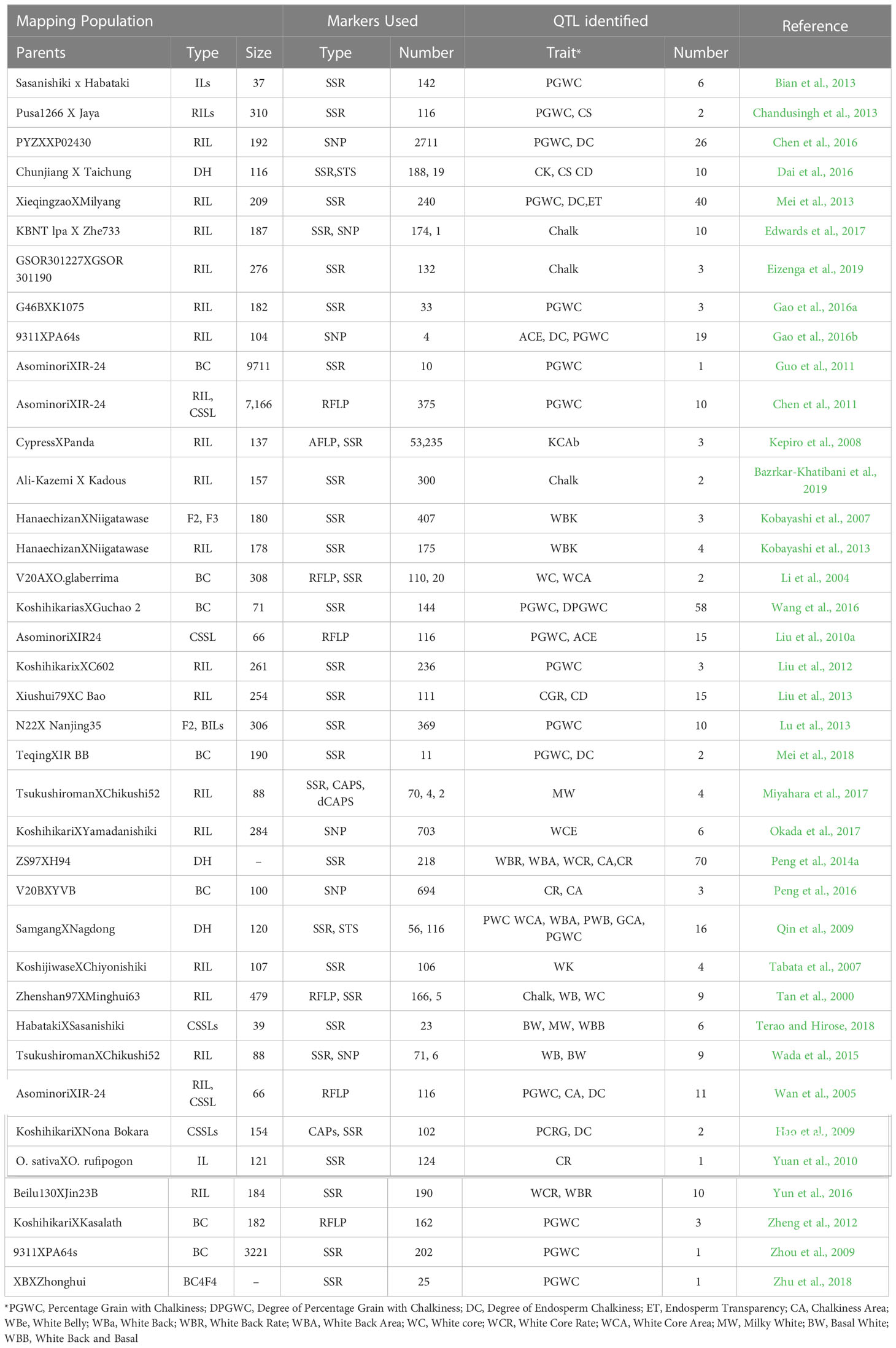
Table 1 Summary of the grain chalkiness QTL mapping studies in rice used to perform the meta-analysis.
We performed a Meta-QTL analysis of the QTLs reported for grain chalkiness in rice to discover the meta-regions associated with it. The identified MQTLs encompassed 5262 genes, which were further shortlisted to 49 based on their differential expression patterns in chalk-associated expression datasets. Further, we performed a haplo-pheno analysis for two of the most important starch synthesis genes and identified haplotype combinations that contribute to low grain chalk under high temperature stress. Our study highlights the relationship between high temperatures and rice grain chalk formation, by establishing the (1) relevance of a QTL meta-analysis in the identification of consensus and precise QTLs associated with grain chalkiness, (2) role and importance of differentially regulated genes from the identified meta-QTL genes, (3) identification of common cis-regulating elements in the candidate genes, (4) discovery of haplotypic variations in the candidate genes across the 3K rice genome panel, (5) haplo-pheno analysis of promising candidate genes in a subset of the 3K panel, and (6) association of haplo-pheno data with the starch granules morphology in the rice endosperm. Our work identifies superior allelic combinations which can be readily employed for generation of climate-resilient rice varieties.
3 Material and methods
3.1 Literature survey, QTL data collection, and input file preparation
Survey of literature published till 2021 on QTLs contributing towards rice grain chalkiness was performed resulting in the identification of 403 QTLs encompassing several chalk related traits. A total of 38 independent mapping studies (compiled as Table 1) involving 30 biparental rice populations were included in the MQTL analysis. QTL data constituting name, trait, chromosome, log of odds (LOD) score, phenotypic variance (R2) value, position and confidence intervals (CI) were compiled in the text format and used as QTL input file. For studies having missing data on LOD score and R2 we assumed them to be 2.5 and 10%, respectively, as previously suggested by Khahani et al. (2019). A genetic map file containing information about the genetic distances of markers on each linkage group was organized for each study. To incorporate QTLs derived from the studies based on SNP markers, the physical position of the flanking markers was ascertained on the rice genome and closest markers on the reference map were used.
3.2 QTL projection on consensus map and meta-analysis of QTLs
Prior to the meta-analysis, a consensus map was built by integrating the genetic map information of all 38 studies listed in Table 1 with a rice reference map (Temnykh et al., 2001) using BioMercator v4.2.3 (Arcade et al., 2004; Sosnowski et al., 2012). QTLs having variable CI were then projected onto the consensus map and the meta-analysis of only the projected QTLs was performed by merging all traits as rice grain chalk. We applied the two-step algorithm developed by Veyrieras (Veyrieras 2007), wherein first, the best model, having the lowest Akaike Information Criterion (AIC) value, corresponding to the estimated number of MQTLs was computed. In the second step, appropriate parameters for the meta-analysis were set, including the number of MQTLs to be mapped, following which the MQTLs were generated.
3.3 Identification of candidate genes within the meta-QTL regions
For determining the genes underlying the identified MQTLs for rice grain chalkiness, the physical locations of confidence interval (CI) flanking markers were retrieved from the Gramene Marker Database (http://archive.gramene.org/qtl/) (Tello-Ruiz et al., 2022). In case the physical position of a particular marker was not available, the position of the adjacent genetic marker was utilized for identifying the genomic coordinates. The locus IDs of all genes spanning the marker intervals of respective MQTL regions were batch downloaded from the Rice Annotation Project Database (RAP) (Sakai et al., 2013). The obtained genes were then compared with the rice grain chalkiness expression datasets from five microarray-based studies (Yamakawa et al., 2007; Yamakawa and Hakata, 2010; Liu et al., 2010b; Ishimaru et al., 2019; Bakku et al., 2020) and one RNA-seq study (Lin et al., 2017). Genes common between at least two expression studies and the current analysis were considered as candidate genes regulating rice grain chalk. Gene ontology information of these chalk associated 49 candidate genes was collected from ShinyGO 0.76 database (Ge et al., 2020).
3.4 In silico promoter analysis and expression profile
The promoter sequence comprising 1.5 kb upstream from the translational start site (ATG) was downloaded from the Rice Genome Annotation Project Database (http://rice.uga.edu/) for the identified fundamental set of chalk associated candidate genes. All sequences were analyzed for motifs and regulatory elements on the PlantCare database (Lescot et al., 2002). To unravel the expression pattern of the candidate genes in rice endosperms we retrieved their expression data using Genevestigator (Hruz et al., 2008).
3.5 Haplotype analysis
An inbuilt tool of SNP seek database (Mansueto et al., 2016) was used to conduct haplotype analysis of the candidate genes in the 3k rice genome panel using Nipponbare as the reference genome. The number of haplotypes were discovered using the default parameters with calinski criterion (Calinski and Harabasz 1974) for determining the ‘k’ group. Only non-synonymous SNPs were considered while carrying out the analysis.
3.6 Plant materials and growth conditions
A subset of sixty rice genotypes from the 3K rice genome panel were chosen for grain chalk phenotyping based on the availability of seeds and uniformity in their phenology. Two independent experiments were conducted during Rabi 2020 and 2021 at IGKV, Raipur (21.2514°N, 81.6296°E). Weather data was recorded on a daily basis and plants were exposed to temperatures between 38-41°C during their seed filling stages. In each year four replicates of 15 plants each were grown in two independent fields and seeds were pooled before assessing grain chalk scores. Plants in both years were grown in open fields under fully flooded conditions to study the effect of high temperature stress coinciding with panicle initiation till physiological maturity.
3.7 Rice grain chalk phenotyping
Rice grains harvested at physiological maturity were dehusked using the automatic rice husker TR-250 (Kett, USA) and polished before the chalk imaging analysis. The polished grains were arranged on a transparent sheet kept on the scanner surface in three replicates each containing a non-redundant set of 50 randomly selected seeds. Seeds were scanned at a resolution of 800 dots per inch (dpi) using an Epson Perfection V850 Pro photo scanner. Images were saved in TIFF (.tif) file format for further image analysis. Further image processing and chalk score estimation was performed with Gradient-weighted Class Activation Mapping (Grad-CAM) tool and according to the methodology described by Wang et al. (2022).
3.8 Haplotypic and phenotypic correlation analysis
We phenotyped a subset of sixty rice genotypes from the 3K rice panel and categorized them as high chalk, intermediate chalk and low chalk to find out a correlation between the chalk score phenotype and the allelic variation in genotypes with respect to the identified candidate genes. Significance test of the means of haplotypic groups and Tukey’s post hoc analyses was computed in R.
3.9 Scanning electron microscopic observation of starch granules
Scanning electron microscopy (SEM) of rice grains was performed to validate the chalk score values as determined by the image analysis. Mature and dry seeds were transversely fractured by putting pressure in the center of the seed by a surgical blade and were coated with 0.1 nm Chromium using Quorum Q150V plus Sputter coater (Peng et al., 2014b). The coated seeds were visualized under 5 keV electron beam energy using TESCAN Clara Scanning Electron Microscope (Tescan Orsay Holding, Czech Republic).
4 Results
4.1 Grain chalk QTLs in rice
Studies on discovery of QTLs associated with grain chalk in rice have used varied attributes for quantifying phenotypic variations. However, the maximum number of studies employed “percentage of grains with chalkiness” (PGWC) as a measurement of trait. Grain chalk has also been accredited to other quantifiable attributes such as degree of percentage grains with chalkiness (DPGWC), degree of endosperm chalkiness (DC), endosperm transparency (ET), chalkiness area (CA), white belly (WBe), white back (WBa), white back rate (WBR), white back area (WBA), white core (WC), white core rate (WCR), white core area (WCA), milky white (MW), basal white (BW) and white back and basal (WBB) (Figure 1A). We, therefore, collated information on 403 QTLs for all these attributes from 38 different mapping studies (see Table 1 for references) to perform a MQTL analysis for the grain chalkiness trait in rice. These QTLs were discovered from 30 biparental crosses with a population size ranging from 37 to 3221 lines. Diverse molecular markers used in these studies included 4035 Simple Sequence Repeats (SSRs), 3416 Single Nucleotide Polymorphisms (SNPs), 1045 Restriction Fragment Length Polymorphisms (RFLPs), 532 Amplified Fragment Length Polymorphisms (AFLPs), 4 Cleaved Amplified Polymorphisms (CAPs), 2 Derived Cleaved Amplified Polymorphisms (dCAPs) and 135 Sequence Tagged Sites (STSs) (Table 1). The number of QTLs varied between 1 to 70 per population, with chromosome 6 having the highest number of QTLs (52) and chromosome 11 having the lowest number of QTLs (12). Confidence intervals (CI) of QTLs varied from 0.52 to 95.8 cM and the phenotypic variance ranged from 0.09% (Li et al., 2004) to 87.2% (Tan et al., 2000).
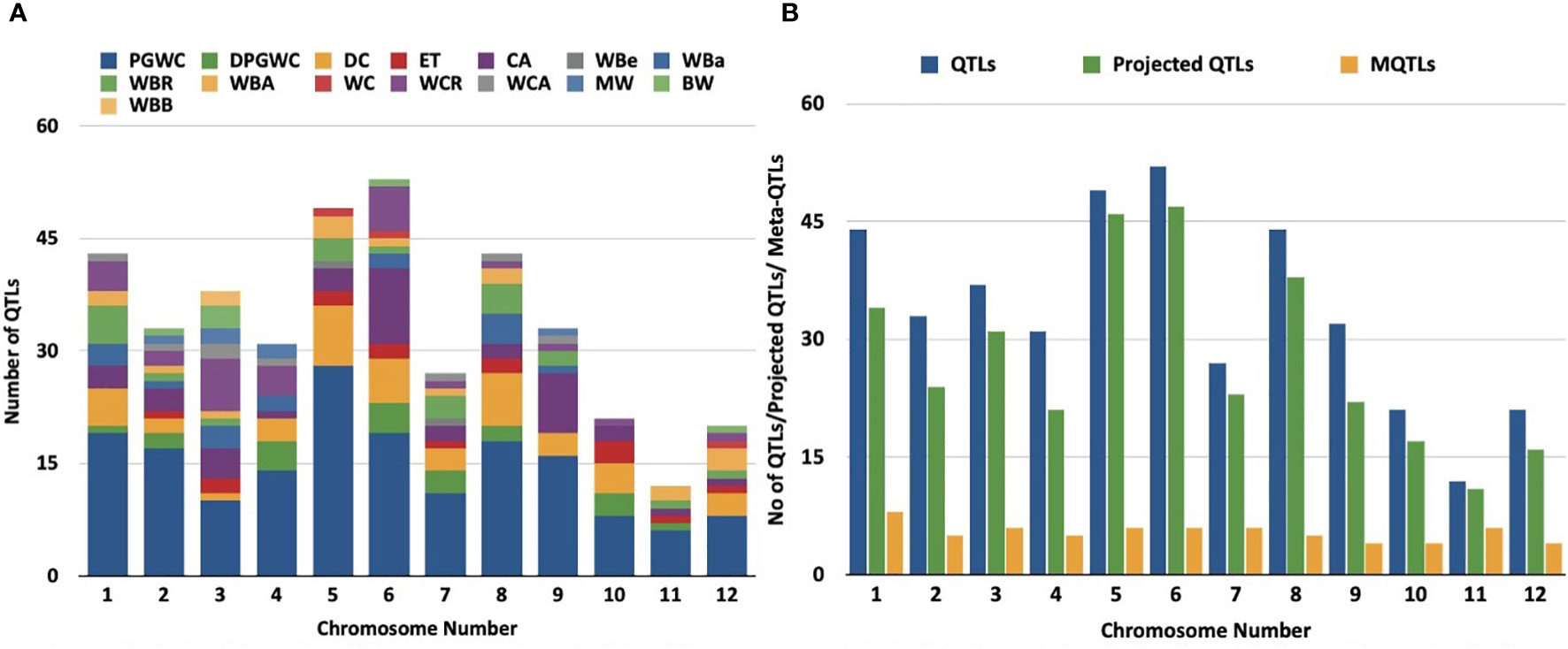
Figure 1 Distribution of QTLs and meta-QTLs associated with rice grain chalk on different chromosomes of rice. (A) Trait-wise distribution of initial QTLs used for the meta-QTL analysis. (PGWC, Percentage Grain with Chalkiness; DPGWC, Degree of Percentage Grain with Chalkiness; DC, Degree of Endosperm Chalkiness; ET, Endosperm Transparency; CA, Chalkiness Area; WBe, White Belly; WBa, White Back; WBR, ; WBA, White Back Area; WC, White core; WCR, White core rate; WCA, White core area; MW, Milky white; BW, Basal White; WBB, White Back and Basal) (B) The distribution of QTLs, projected QTLs and meta-QTLs on twelve rice chromosomes.
4.2 Distribution of grain chalk meta-QTLs on the rice genome
We next generated a consensus map from the 38 QTL maps and the rice reference map (Temnykh et al., 2001). The consensus map consisted of 11,141 markers with a genetic length of 2052.37cM. From the 403 initially retrieved QTLs, 330 (82%) were successfully projected on the consensus maps (Figure 1B). Meta-analysis of the 330 projected QTLs identified 64 MQTLs (Table 2, Figure 2; Supplementary Figure 1) on the basis of Veyrieras algorithm of BioMercator v4.2.3. The best MQTL model for each chromosome was selected on the basis of values of AIC, AIC correction (AICc), AIC 3 candidate (AIC3), Bayesian information criterion (BIC), and Average Weight of Evidence (AWE) for each chromosome. The 64 MQTLs were randomly scattered across all the twelve rice chromosomes and their number varied from 2 on Chromosome 8 to 8 on each Chromosomes 1 and 3 (Figure 2). Going strictly by definition, only MQTLs identified from at least 2 overlapping QTLs clustered on a consensus map are considered for the downstream analysis, while MQTLs derived from a single QTL are omitted (Yang et al., 2019; Kumar and Nadarajah, 2020). Therefore, MQTLs 1.6, 1.8, 4.2, 4.5, 11.1, 11.2, and 11.3 which were derived from a single QTL were not considered for further analysis (Table 2). Clustering of multiple QTLs identified from independent mapping populations that were phenotyped in different environments on the consensus map signifies the importance of the MQTLs identified. We, therefore, used a cut off of 10 QTLs clustering together to predict the most significant MQTLs. MQTL 6.1 derived from 14 overlapping QTLs (mean R2 value of 23.7%), and MQTL 6.2 derived from 11 QTLs (mean R2 value of 19.6%) indicated that short arm of Chromosome 6 plays an important role in formation of grain chalk. Similarly, MQTL 5.2 was derived due to clustering of 12 QTLs (mean R2 value of 18%) suggesting that this genomic region of Chromosome 5 is also important for grain chalkiness in rice. The CI (95% confidence) of the MQTLs ranged from 0.03 to 15.49 cM with a median value of 2.81 cM. Similarly, the physical positions were also reduced and varied from 214 bp to 4.07 Mb with a median value of 0.65 Mb. Notably, the CI of 48 MQTLs (73%) was less than 5 cM, and 52 MQTLs (94%) exhibited a physical distance narrower than 2 Mb. Overall, the meta-analysis resulted in significant reductions in the CIs and physical distance of initial QTLs thereby minimizing the number of candidate genes for further analysis Figure 3A.
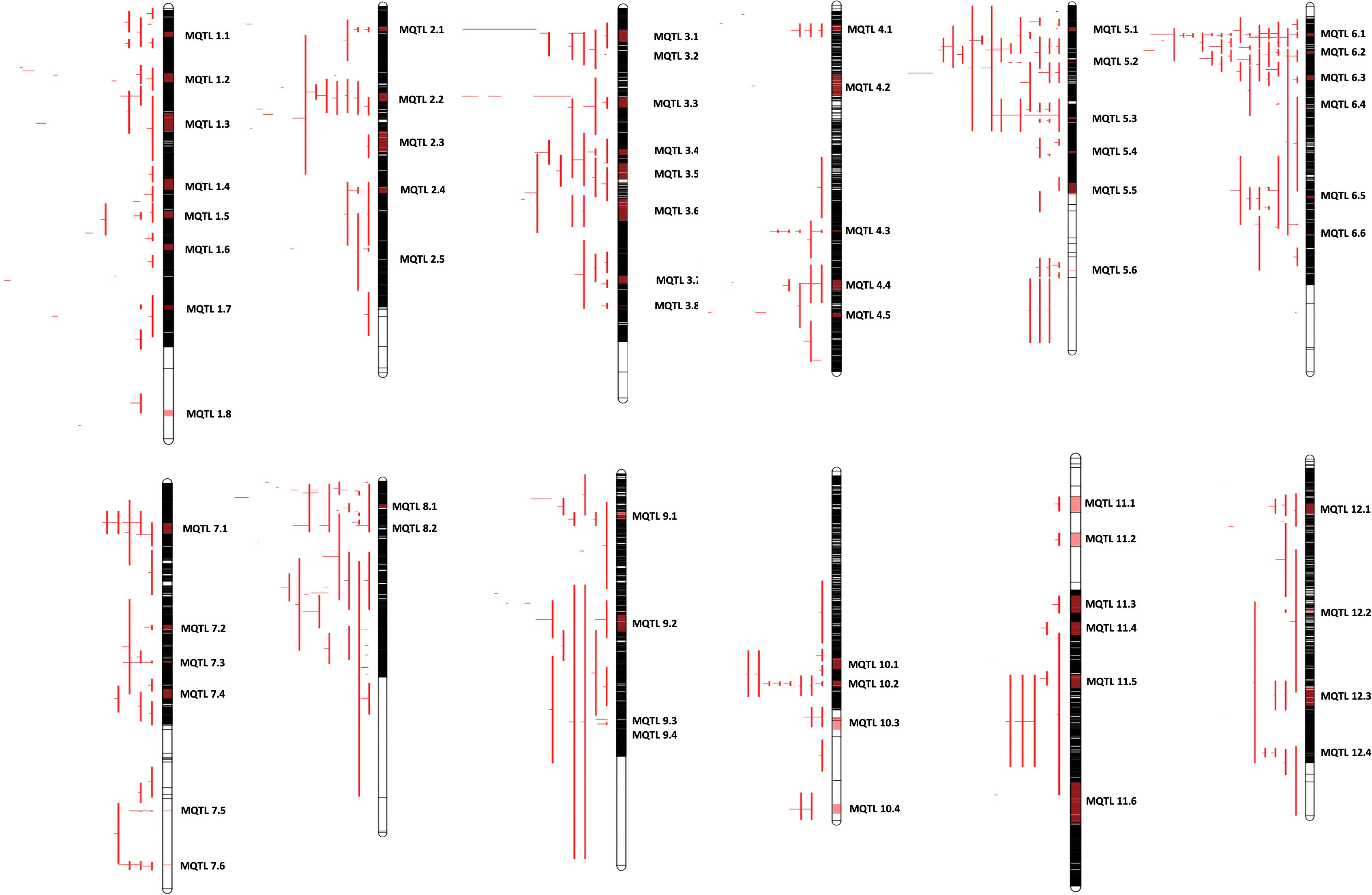
Figure 2 Distribution of meta-QTLs on rice chromosomes. Rice chromosomes are represented by the vertical bars. The names of consensus markers along with their position (cM) are present on the right of the chromosomes. QTLs mapped in various studies are highlighted in different colours on the left of the chromosomes. Coloured blocks on the chromosomes represent the identified meta-QTLs. Vertical bars on the left side of the chromosome represent the confidence interval (CI) of QTL and horizontal bars represent the phenotypic variance.
4.3 Candidate gene mining within meta-QTL regions
Since the majority of QTL regions from which MQTLs were derived have not yet been fine mapped, we considered all the genes within MQTLs as putative candidate genes. IDs of the genes for each MQTL were batch retrieved from the Rice Annotation Project Database (RAP), and a total of 5262 non-redundant genes were present within the 64 MQTL regions (Supplementary Table 1). Maximum number of genes were in the MQTL 11.6 (383) followed by MQTL 11.3 (256) and MQTL 5.5 (245), while the lowest number of genes were found within MQTLs 6.4 (1) and 12.4 (6) (Table 2). A common approach for further shortlisting the putative candidates is to ascertain the differentially expressed (DEGs) MQTL genes for the studied trait (Kong et al., 2020; Mirdar Mansuri et al., 2020; Raza et al., 2020; Khahani et al., 2021). Therefore, we overlapped all MQTL candidate genes with the 6 previously published expression datasets for grain chalkiness in rice (Yamakawa et al., 2007; Yamakawa and Hakata, 2010; Liu et al., 2010b; Lin et al., 2017; Ishimaru et al., 2019; Bakku et al., 2020). The investigation revealed that 314 MQTL genes (Figure 3B, Supplementary Table 2) were reported to be differentially expressed by Yamakawa and Hakata (2010). Similarly, 60, 56, 50, 48 and 26 genes were categorized as DEGs by Lin et al. (2017); Bakku et al. (2020), Ishimaru et al. (2019), Liu et al. (2010b) and Yamakawa et al. (2007), respectively (Figure 3B, Supplementary Tables 3-7). With an aim to discover a core set of chalk-associated genes we further shortlisted the DEGs on the basis of their presence in at least 2 expression datasets leading to the identification of 49 candidate genes (Supplementary Table 8; Figure 3C). Gene ontology (GO) analysis revealed that out of 49 candidates, 7 genes were involved in the carbohydrate metabolism. Besides these 9 genes belonged to the stress response pathway, 5 genes each belonged to the peptide metabolism pathway and seed storage proteins, 2 genes each were members of alpha amylase inhibitors, serine carboxypeptidases and carboxylic acid metabolic process. One member each was annotated as amino acid transporter and monosaccharide transporter, while 15 genes possessed non-significant annotations (Figure 3D).
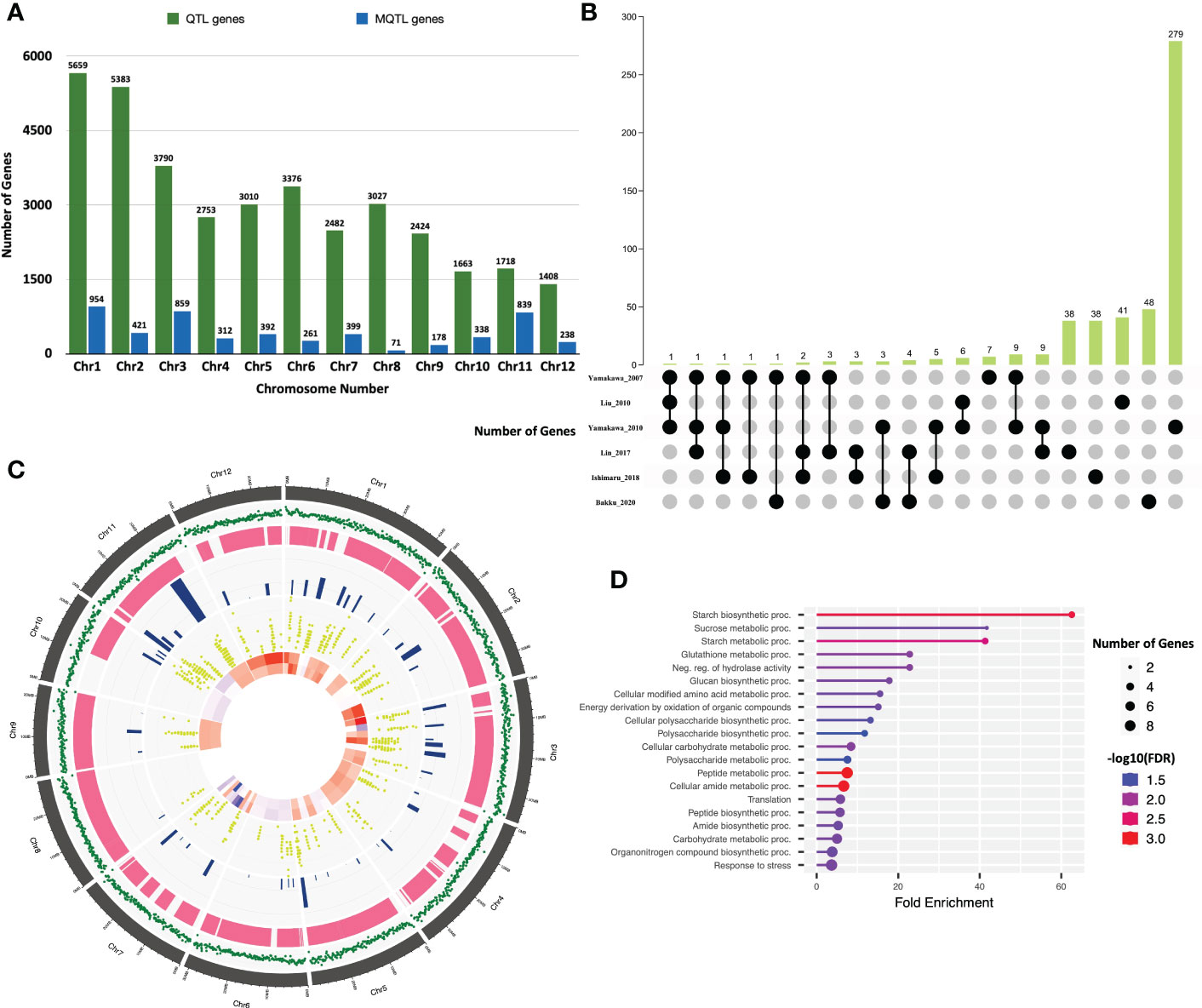
Figure 3 Candidate genes underlying the meta-QTL regions. (A) Chromosome-wise distribution of genes underlying QTLs and the identified meta-QTLs in rice. (B) Upset plot highlighting the number of differentially regulated genes identified in six rice grain chalk-related transcriptomic studies. (C) Distribution pattern of the gene density, QTLs, meta-QTLs, meta-QTL spanning genes and candidate genes identified on the rice genome. The outermost circle represents the chromosomes position on the rice genome in Mb. The second circle with green color outlines the gene density on the rice genome. The third and fourth inner circles display the number of initial chalk QTLs and identified meta-QTLs, respectively. The fifth circle represents the MQTL underlying genes., while the innermost circle represents heatmap of the identified candidate genes (red represents upregulation, while blue signifies downregulation). (D) Gene Ontology enrichment analysis of 49 candidate genes representing the biological process. Created using ShinyCircos Yu et al., 2018.
4.4 Cis-regulatory elements in the promoter of candidate genes
Although synthesis of starch is initiated in leaves where it serves for growth and development, seed endosperm being the sink is at the center of starch metabolism in rice (MacNeill et al., 2017). Consequently, many genes are co-expressed in the endosperm for regulating the optimal quality and quantity of starch granules. We, therefore, investigated whether the 49 candidate genes had common cis-elements in their promoters (Figure 4A) that might regulate their spatial and temporal expression. The 1.5 kb putative promoter region of all these genes were enriched with important cis-regulatory elements (CREs) elements, such as stress-responsive element (STRE; AGGGG), activation sequence-1 like promoter element (AS-1; TGACG), purine (A or G) and pyrimidine (C or T) element (RY; TGAGTCA) and GCN4 (CATGCATG). The AGGGG motif termed as stress-responsive element (STRE), initially identified in yeast, is responsive to several stresses (Martinez-Pastor et al., 1996). The AS-1 cis-element is present in genes regulated by auxin, MeJa and salicylic acid (Miao and Lam, 1995; Abdullah-Zawawi et al., 2021; Kabir et al., 2021). This element has also been reported in the promoters of several Glutathione-S-transferase genes (Hu et al., 2011) and abiotic stress response genes (Garretón et al., 2002). All the 49 investigated genes had the AS-1 and STRE motifs, suggesting that they might play a direct or indirect role in starch metabolism during unfavorable conditions. The GCN4 motif is crucial for seed-specific expression (Wu et al., 1998), while the RY element coordinates with other CREs and also plays a key role in seed-specific gene regulation (Ezcurra et al., 1999). A total of 17 genes either had GCN4 or RY motifs in their promoters indicating that these genes are possibly co-expressed in rice seeds. Further, with the help of Genevestigator expression datasets we observed that 41 out of 49 genes were differentially expressed in the rice endosperms (Figure 4B), indicating their role in the formation of rice grain chalk.
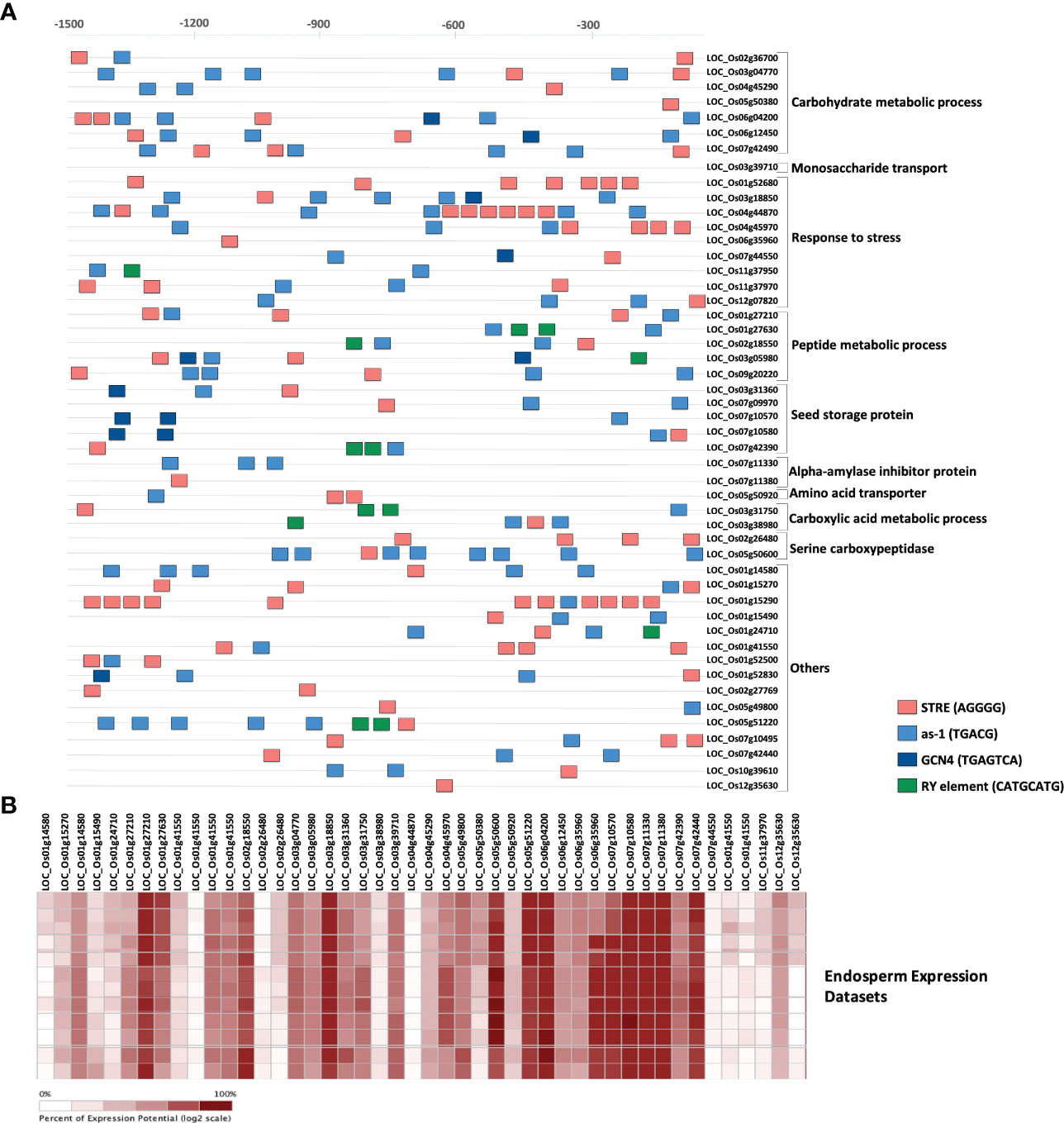
Figure 4 Spatio-temporal expression of candidate genes. (A) In silico identification of regulatory elements in the putative promoter region of the identified fundamental set and known chalk-responsive genes. Coloured boxes indicate as-1 element (blue), RY element (green), STRE (salmon) and GCN4 motif (dark blue) in 1500 bp upstream promoter regions. Scale is presented above the figure. (B) Heatmap highlighting the percent expression pattern of candidate genes in the endosperm, figure created using Genevestigator.
4.5 Haplotype analysis of 49 candidate genes reveals genetic diversity across the 3K rice genome panel
The 3K rice genome (RG) project is a giga dataset of publicly available genome sequences which can be used to mine genotypic variations in a population and link them to the phenotypic variability for exploring genetic diversity of different agronomic traits in rice (Li et al., 2014). We, therefore, performed a haplotype analysis of the 49 candidate genes to discover the non-synonymous variations in their coding sequences (CDS) across the 3K RG panel (Supplementary Table 9). Interestingly, 39 of the 49 analyzed candidate genes possessed non-synonymous SNPs ranging from 2 to 8, while the remaining 10 genes did not show any non-synonymous variations. Five of the seven carbohydrate metabolism-related genes contained non-synonymous SNPs in their coding sequences. We discovered 6 haplotypes and 1 allelic variation in sucrose transporter 5 (SUT5) and vacuolar invertase (VIN2), respectively, which were not reported previously and never before associated with the grain chalkiness and its related attributes. However, haplotypes in GBSSI and SSIIa have previously been implicated in starch metabolism and therefore we decided to explore the allelic variability in their CDS across the 3K panel and the subpanel of 60 accessions (Figure 5).
Waxy (Wx) gene, which encodes the enzyme GBSSI, converts ADP-glucose to amylose in the endosperm of cereals (Sano, 1984; Webb, 1991). Allelic variations of Wx gene in rice; Wxlv, Wxa, Wxin, Wxb, Wxla/mw, Wxmq, Wxop/hp and wx (Supplementary Table 10), linked to varied amylose content (AC values), are distinguished by six polymorphic sites namely Int1-1, Ex2-112, Ex4-53, Ex4-77, Ex6-62, and Ex10-115 (Figure 5B) (Cai et al., 1998; Isshiki et al., 1998; Mikami et al., 1999; Sato et al., 2002; Wanchana et al., 2003; Mikami et al., 2008; Mikami et al., 2008; Liu et al., 2009; Yang et al., 2013; Zhang et al., 2019). GBSSI has 1 splice site SNP and 5 non-synonymous SNPs that give rise to 8 haplotype combinations. We were able to detect 6 of the 8 haplotype combinations in the entire 3K panel. Two hundred and one accessions (7%) had missing entries in the 3K panel either due to lower sequencing depth or sequencing errors and were therefore excluded from the analysis. Out of the remaining 2826 accessions, haplotype GGAAT (H2) had the highest frequency and was present in 738 accessions, whereas other haplotypes GGAAC (H1), TGAAC (H4), GGACC (H5), GGGAC (H3) and TGACC (H6) were observed in 23.7%, 22.4%, 19.8%, 1.8% and 1% of accessions, respectively (Figure 6B). A ‘G’ to ‘A’ change at Ex4-53 (Exon 4 th, 53 rd nucleotide) results in low amylose content and give rise to milky endosperms known as “milky queen” phenotype in seeds (Sato et al., 2002). Interestingly, the entire 3K panel had ‘G’ at Ex4-53 (Figure 5C) indicating that the “milky queen” phenotype was selected during varietal development. Owing to the presence of G in all the accessions, we were unable to find Wxmq haplotype in the panel. Further, four haplotypes (GGAAC, GGAAT, GGGAC and TGAAC) were represented in the subset panel consisting of 60 accessions.
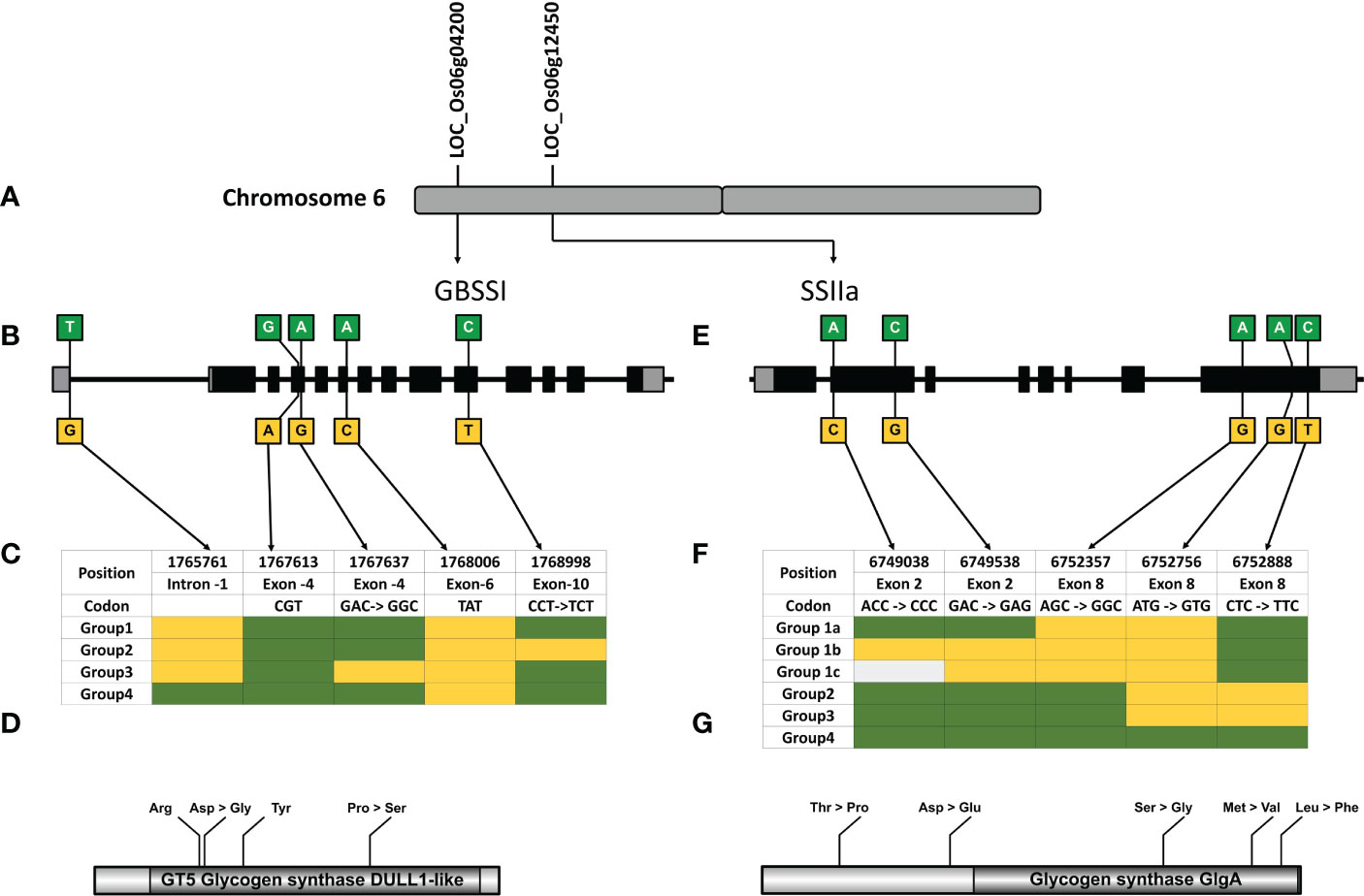
Figure 5 Haplotype analysis of Granule Bound Starch Synthase I (GBSSI) and Starch Synthase IIa(SSIIa). (A) The genes coding for GBSSI and SSIIa are located on the short arm of chromosome 6. (B) Gene diagram showing the non-synonymous variations of GBSSI, comprising 13 exons (grey boxes-UTR, Black boxes- CDS). (C) Haplotype table showing the SNP positions within GBSSI, four haplotypes are constructed based on the sequence variations in 25 rice genotypes (green indicates the reference allele Nipponbare, yellow indicates the SNPs and light grey shows missing data). (D) Depiction of GBSSI protein and amino acid changes in the GT-5 Glycogen Synthase DULL like domain predicted using NCBI CDD (E) Gene diagram showing the non-synonymous variations of SSIIagene comprised of 8 exons. (F) Haplotype table showing the SNP positions within SSIIa, four haplotypes, color codes same as (B). (G) Depiction of SSIIa protein and amino acid changes in the Glycogen Synthase Glga domain predicted using NCBI CDD.
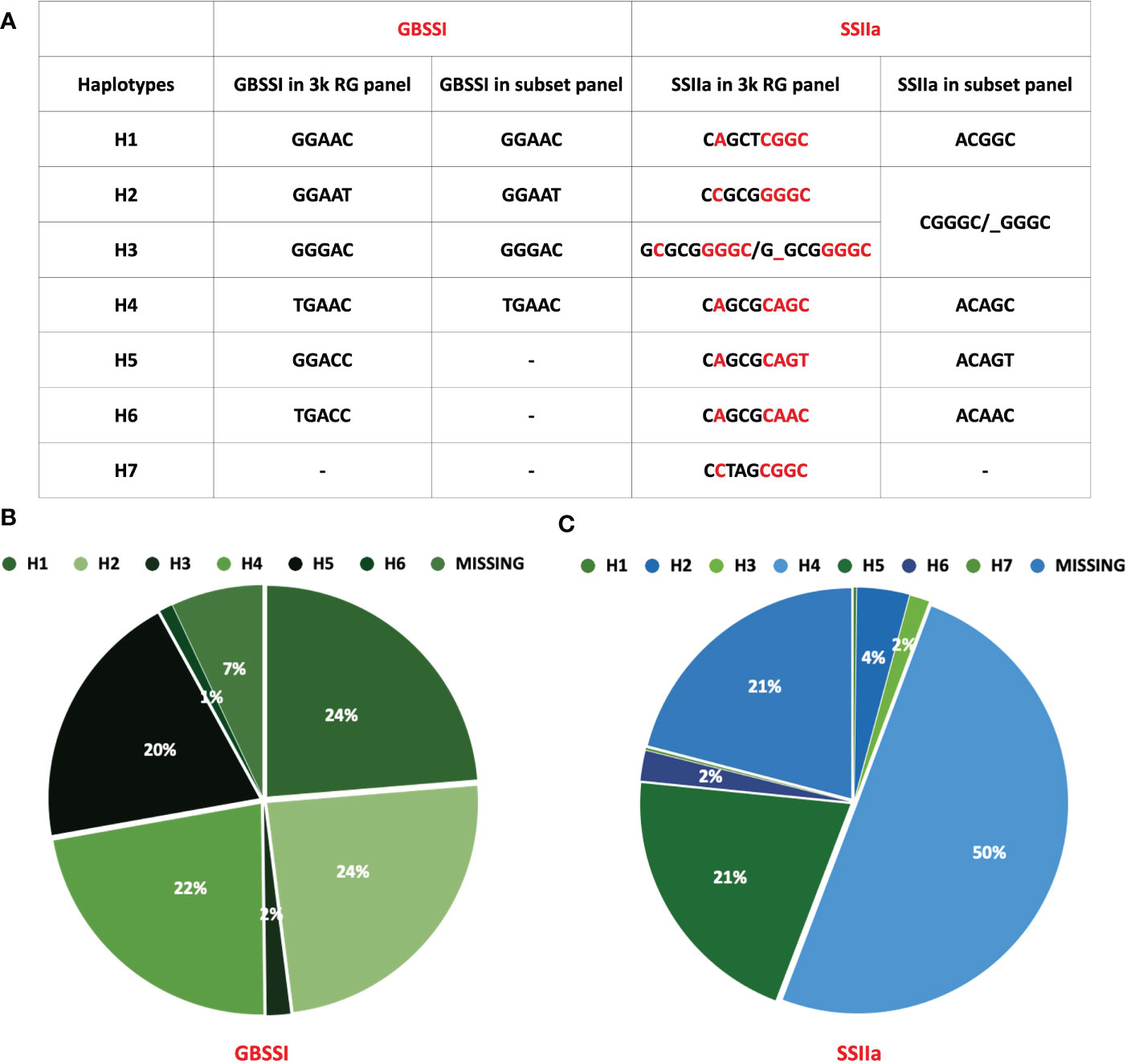
Figure 6 Distribution of haplotypes of GBSSI and SSIIa in rice 3k and its subset panel. (A) Table depicting the number of haplotypes in the 3k panel Vs. the subset panel for both genes, (B) Pie chart showing the % distribution of GBSSI haplotype in the 3k RG panel, (C) Pie chart highlighting the % distribution of haplotypes in SSIIa across 3k RG panel.
Soluble starch synthase IIa gene (SSIIa) elongates glucan chains from ADP-glucose units to form amylopectin in conjunction with branching and debranching enzymes (Denyer et al., 1995; Denyer et al., 1996; Tetlow and Emes, 2014). We discovered that SSIIa had 7 haplotypes arising from a combination of 9 non-synonymous SNPs (H1-CAGCTCGGC, H2-CCGCGGGGC, H3-GCGCGGGGC, H4-CAGCGCAGC, H5-CAGCGCAGT, H6-CAGCGCAAC, H7-CCTAGCGGC), in the entire 3K RG panel (Figure 6A). While 625 accessions had missing data, in the remaining 2402 accessions H4 was most predominant (50% of accessions). Other haplotypes in the descending order of their frequency (Figure 6C) were H7 (0.16%), H1 (0.2%), H3 (1.5%), H6 (2.3%), H2 (4%) and H5 (20.8%). However, we could only detect 5 SNPs giving rise to 6 haplotypes in the subset panel of 60 accessions (Figurse 6 e, f). The missing 4 SNPs C, G/T, C/A and G/T resulted in 3 combinations “CGCT” (H1), “CGCG” (H2-H6) and “CTAG” (H7). While the combination of missing SNP “CGCT” was present in 21% of accessions, “CTAG” was present in only 2% of accessions. Out of the 6 haplotype combinations in the gene encoding for SSII-a enzyme, 4 haplotype combinations arising from 3 SNPs (H1-GGC, H2-AGC, H3-AGT and H4-AAC), are associated with chain length of amylopectin, activity of starch synthase, gelatinization temperature and alkali spread score (Umemoto et al., 2004; Umemoto and Aoki, 2005; Waters et al., 2006). All the 3 SNPs resulting in 4 haplotypes are present in the catalytic domain of the enzyme (Figure 5G) and therefore are important for enzyme activity, which was more for the haplotypes H1 and H2 and less for H3 and H4 (Umemoto et al., 2004; Umemoto and Aoki, 2005).
4.6 Identification of superior haplotypes for granule bound starch synthase I and starch synthase IIa by haplo-pheno analysis
Since haplotypes in GBSSI and SSIIa are important for their activity and likely to affect starch quality, we investigated their haplotype behavior with respect to the grain chalkiness in the subset panel of 60 accessions. These accessions were subjected to high temperature stress under natural field conditions during the grain filling stages over two Rabi seasons in Raipur, India. The amount of chalk (expressed as “chalk score”) was determined by scanning the polished rice grains and processing the images through a Gradient-weighted Class Activation Mapping (Grad-CAM) tool (Wang et al., 2022). The chalk score ranged from 0.046 to 0.075 and the accessions were distributed in low chalk (CS ≤ 0.05), intermediate chalk (0.05 > CS ≤ 0.065) and high chalk (CS > 0.065) categories (Supplementary Table 11, Figure 7A). Seven accessions were in the low chalk category, thirty-three in the intermediate and twenty were in the high chalk category. Inferential statistics ascertained that the means of all three chalk categories were significantly different (Figure 7B). Analysis of variance (ANOVA) determined that the variability of chalk score with respect to all the 4 haplotypes of SSIIa and GBSSI were significantly different and had p-values of ≤ 0.05 and ≤ 0.1, respectively. However, after Tukey’s post hoc analyses, differences in the mean CS values for only GBSSI haplotypes GGAAC and GGGAC were significant (Figure 7C). Out of the 4 possible haplotypes in the catalytic domain of SSIIa, significant differences in the mean CS values were seen between GGC and AGT, GGC and AGC and GGC and AAC (Figure 7D). To find whether and which combination of haplotypes for GBSSI and SSIIa associate with low grain chalkiness under high temperature stress, we performed a correlation analysis of different haplotype combinations with the chalkiness phenotypes. Interestingly, all the seven accessions with lowest mean CS, had the haplotypic combination of GGAAC (for GBSSI) and GGC (for SSIIa). Fifteen accessions having an intermediate CS, also had a similar haplotype combination. The other 18 accessions with intermediate CS had the following haplotype combinations for GBSSI/SSIIa; GGAAC/AGC, GGAAT/GGC, GGAAT/AGC, GGAAT/AGT, GGGAC/AGT, GGAAC/GGC, TGAAC/GGC and TGAAC/AGT. Five high CS accessions had the haplotype combinations (GBSSI/SSIIa) of GGGAC/AGT, while four had GGAAC/AGC, three each had GGAAC/AGT and GGAAC/GGC, two had TGAAC/AAC and 1 each had GGAAT/AGC, GGAAT/AGT and GGAAT/GGC.
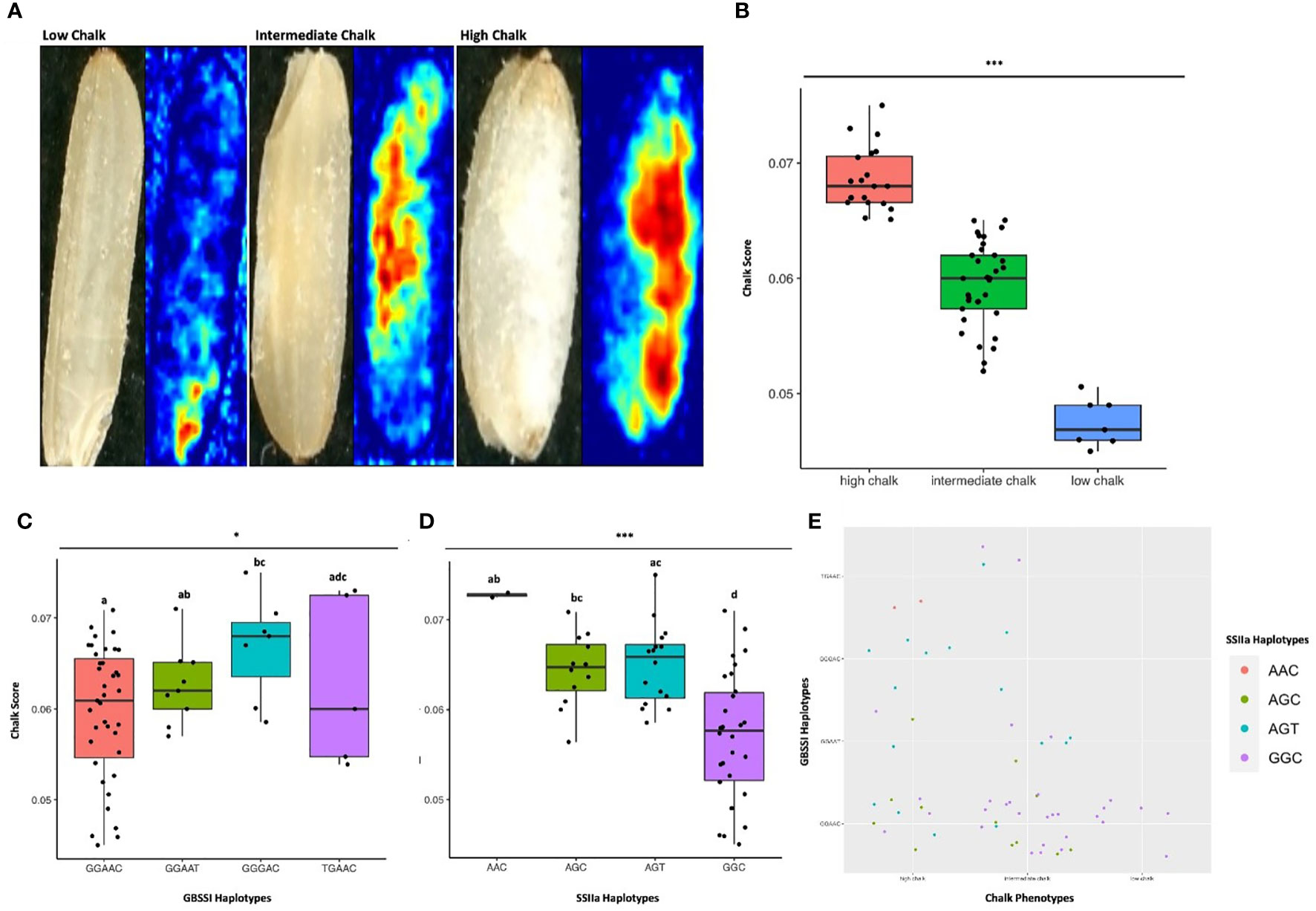
Figure 7 Haplo-pheno analysis of Granule Bound Starch Synthase I and Starch Synthase II a. (A) Rice seed scans and their corresponding heat maps highlighting the chalky area (red) in less chalk, intermediate chalk and high chalk seed types. (B) Box plot depicting the chalky score of 60 rice genotypes (grown in two crop seasons) categorized as high, intermediate and low chalk. (C) Box plot of the chalk score data categorized according to the GBSS I haplotypes. (D) Box plot of the chalk score data categorized according to the SSIIa haplotypes. (E) Comparison of the haplotypic combination of GBSS I and SSIIa. One-way ANOVA was conducted for determining the statistical significance of haplotype means. Chalk score data designated with the same alphabet are not significantly different at p ≤ 0.1(.) or p ≤ 0.05 (*) or p ≤ 0.001 (***) as per Tukey Post hoc test for Honest Significance Difference (HSD) analysis.
4.7 Scanning electron microscopy validated the correlation between chalk score and haplotypic combinations
Six accessions, two from each category of low, intermediate and high chalk score were selected in order to find correlation between haplotypes and packaging of starch grains in chalky and non-chalky seeds. The seeds of the accessions CX160 and IRIS 313-11462 having low chalk score with GGAAC/GGC haplotypic combination showed dense packaging of starch granules under scanning electron microscope. The amyloplast and the starch grains in them were very tightly packed and no space was visible between them. This probably contributed to the translucent appearance of the grain (Figures 8A1, B1). Whereas, the accessions IRIS 313-10417 and IRIS 313-11245, with GGAAT/AGC, GGAAT/AGT haplotypic combination grouped under the intermediate chalky category, exhibited slightly bulged starch granules with visible spaces between individual amyloplasts (shown with red arrow, Figures 8C1, D1). In contrast, the high chalk genotypes Nipponbare and IRIS 313-11198 with TGAAC/AAC and TGAAC/AAC haplotypic combinations showed visibly round starch granules (indicative of loose packaging) instead of polyhedral and with noticeable air spaces between them. Presence of air spaces and loose packaging scatters light leading to opaque or chalky appearance as shown in Figures 8E1, F1. A magnified view of the starch granules is shown in the lower panel (Figures 8A2-F2). The SEM data validates a clear association between haplotypic combinations and phenotypic data relating to degree of seed chalkiness.
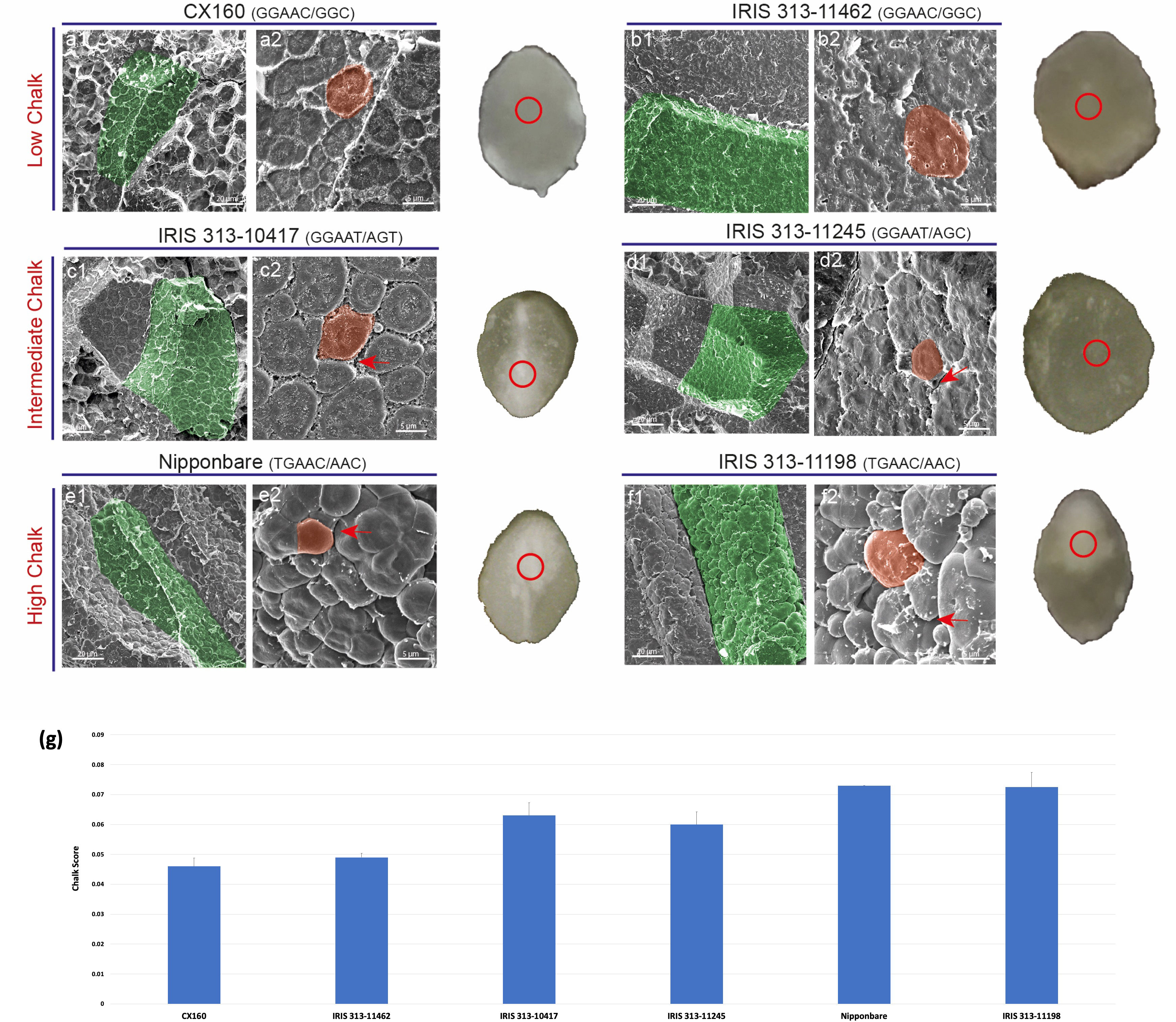
Figure 8 Scanning electron micrographs of transversely fractured mature rice seeds. (A1-B2) Endosperm cell (green) of low chalk genotypes is tightly packed with starch grains (orange). (C1-D1) Micrographs of intermediate chalk genotypes with slightly bulged starch granules with visible spaces between individual amyloplasts. (E1-F2) Images of transversely cut chalky endosperm region filled with spherical starch grains instead of polygonal as in a1 and b1. Air spaces (shown by red arrows) can be seen between the grains in e1 and f1. The red circle on a transverse cut seed shows the region selected for analysis.
5 Discussion
Mapping of quantitative trait linked genomic regions in segregating populations from diverse genetic backgrounds has led to the identification of many grain chalk associated QTLs. Majority of these studies employed markers providing low genetic resolution and therefore the identified QTLs span a large CI. Furthermore, many QTLs from independent studies map to similar genomic regions. It is, therefore, important that common and consistent regions of overlapping QTLs are identified for their effective utilization in trait improvement programs. A meta-QTL analysis aptly serves to achieve this goal (Goffinet and Gerber, 2000) and has been successfully applied to several crop plants, including rice. In this study, we performed a meta-analysis of rice grain chalk QTLs to precisely identify the most significant QTL regions and their underlying candidate genes. We were able to restrict the most important genetic information of 403 QTLs within 64 meta-QTLs. To comprehensively identify chalk-associated candidate genes, expression of the genes underlying these meta-QTLs was assessed in the published grain chalk-associated expression datasets, leading to the identification of a core set of 49 genes. We then performed a promoter analysis to identify the common cis-regulating elements, followed by a haplotype analysis of the 49 candidate genes across the 3K RG panel which revealed natural variations in these genes. Owing to the direct connection of genes involved in sugar transport and metabolism, we correlated haplotypes in 6 sugar metabolism genes with the grain chalkiness in a subset of 60 accessions. Our analysis revealed haplotype combinations in GBSSI and SSIIa that are associated with presence of low chalk.
5.1 Meta-analysis complemented with gene expression underpins key genes involved in grain starch metabolism
Conversion to starch from UDP/ADP-Glucose is a multistep process that begins with the transport of sucrose, its conversion to glucose followed by synthesis of amylose and amylopectin (Figure 9A). Genes and proteins involved in each of these steps are themselves regulated at transcriptional, post-transcriptional and post-translational levels. Majority of the QTL mapping studies for grain chalk have identified genes directly involved in starch synthesis, while other studies have led to the discovery of genes which code for regulators. In the MQTL analysis we identified 7 genes that code for proteins belonging to the starch metabolism pathway, owing to their discovery in overlapping QTLs derived from different mapping studies. This further proves that regulation of sugar metabolism governs the core process of chalk formation.
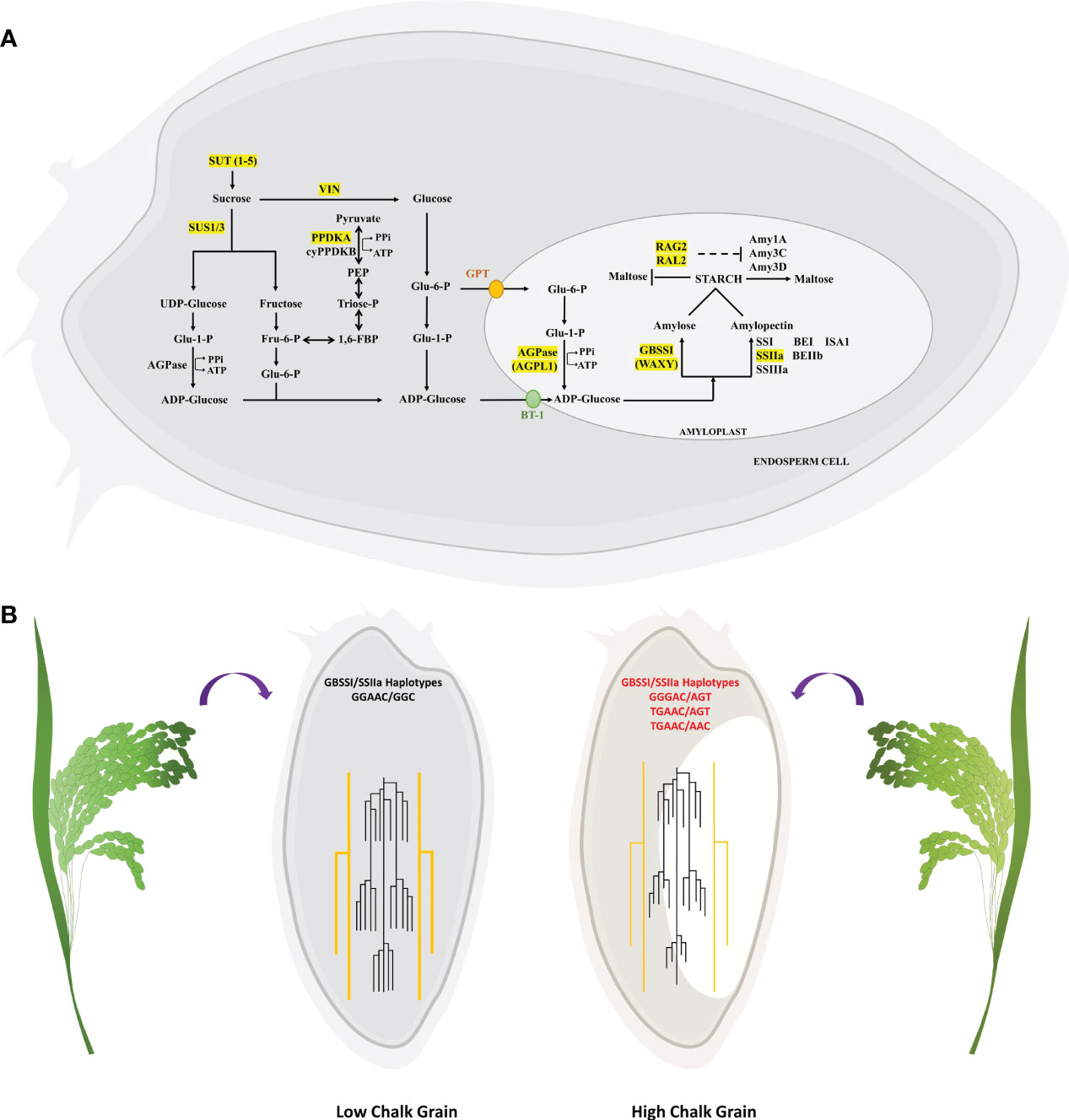
Figure 9 Depiction of identified candidate genes in the starch metabolism pathway and their haplotypic combinations affecting grain chalkiness. (A) Starch metabolism pathway in seeds begins with the sucrose transporters (SUTs) that translocate sucrose into the seeds, followed by its breakdown into glucose and fructose by the enzymatic action of invertases (VIN) and sucrose synthases (SUS). Finally glucose-1-phosphate (Glu-6-P) is converted to ADP-glucose by ADP-glucose pyrophosphorylase(AGPase), which is converted to starch by the combined action of Granule Bound Starch Synthase I (GBSSI) which forms amylose and Starch Synthase IIa (SSIIa) which forms linear glucan chains leading to formation of amylopectin. During HT amylases (Amy) breakdown starch to malto-oligosaccharides. RAG2/RAL2 from alpha-amylase inhibitor family are thought to suppress the amylase activity. (B) Grains with low chalk have high amylose and longer chain length while high chalk grains have low amylose and short chain amylopectin. Low grain chalk seeds have superior haplotypic combination of GBSSI and SSIIa when compared with high chalk seeds.
Sucrose transporters (SUTs) mediate the proton symport coupled transport of sucrose across the plasma membrane through the phloem to developing grains (Aoki et al., 2003). The OsSUT gene family comprises five members OsSUT 1, 2, 3, 4 and 5 that demonstrate overlapping expression patterns during grain filling and therefore might exhibit partial functional redundancy (Aoki et al., 2003). OsSUT5, one of the candidate sugar metabolism gene identified in the current study lies in MQTL 2.4, which is contributed by QTLs qPGWC2 (Chen et al., 2011), qAEC2 and qDEC2 (Wan et al., 2005). Structure-based modeling experiments and electrophysiology assays in Xenopus oocytes revealed that five of the six charged amino acids (D177, R188, D331, R335 and E336) are important for sucrose transport by OsSUT1 (Sun et al., 2012). All the five functionally important amino acids were conserved in OsSUT5 (unpublished data) across the 3K panel. This signifies the importance of functional redundancy in sucrose transporters for mobilization of sugars to sink to ensure normal grain filling. Further, haplotype analysis revealed 6 non-synonymous haplotypes of SUT5 gene in the entire 3K panel (Supplementary Table 9). However, none of the haplotypes were in the wobble base of the codons for the five functionally important amino acids. None of the haplotypes grouped with the chalk score data in the 60 accessions which implies that their relevance will need further experimentation. Nonetheless, the formation of grain chalk is regulated by a aleurone-specific TF, OsNF-YB1, which binds to the promoters of OsSUT genes and transcriptionally activates their expression (Bai et al., 2016). In this respect, it would therefore be important to assess the polymorphism at “CCAAT” boxes in the promoters of OsSUT genes in future. Sucrose synthase (SUS) enzyme is encoded by 7 conserved SUS genes with compensatory functions (Hirose et al., 2008; Cho et al., 2011) and catalyzes the conversion of sucrose and uridine diphosphate (UDP) into fructose and UDP-glucose (Huang et al., 2016). OsSUS3, a candidate gene in this study present in MQTL 7.4 [contributing QTLs qWBA, qWCA, qWBR and qET(Peng et al., 2014a; Yun et al., 2016; Mei et al., 2013)] was predominantly expressed in the endosperm and the aleurone layers at the onset of starch synthesis (Huang et al., 1996). Our data revealed that the SUS3 gene had 1 allelic variation in the coding region of the 3K panel (Supplementary Table 9), which is in contrast to previous reports where presence of 2 (6 SNPs) and 11 haplotypes in the coding sequence have been reported (Lestari et al., 2011; Takehara et al., 2018). Additionally, haplotypes were also identified in the non-coding region of the SUS3 gene which included the promoter (Lestari et al., 2011; Takehara et al., 2018). It was, however, surprising that none of the previously identified haplotypes in the SUS3 CDS were seen in the 3K panel. Only 3 of the varieties (IR64, Milyang 23 and Lemont) harboring the reported haplotypes were part of the 3K sequenced panel. We believe that these haplotypes were not detected in the variant analysis of the 3K panel because the SNP dataset was a filtered dataset [cutoff values of minor allele frequency (MAF) > 1% and missing < 20%; Mansueto et al., 2016]. Nonetheless the SUS3 alleles in the promoter and coding region from Habataki, an indica variety were important for low grain chalk under high temperature stress as introgression of Habataki SUS3 alleles in Koshihikari (Apq1-NIL), a japonica variety, led to increased percentage of perfect grains (Takehara et al., 2018).
Sucrose flux in the plant metabolic pathways is regulated by isoforms of invertases (Sturm, 1999) which catalyze the irreversible cleavage of sucrose into glucose and fructose. Rice has eight neutral invertases (NINs, cytosolic), nine cell wall invertases (CWINs) and two vacuolar invertases (VINs). Our study identified OsINV2 as one of the candidate genes in MQTL 4.4 [contributed by QTLs qDPGWC, qPGWC, qWCR4.1, qWCR4.2, qch (Peng et al., 2014a; Wang et al., 2016; Yun et al., 2016; Edwards et al., 2017)]. OsVIN2 and its homologue OsVIN3 regulate the sink size by controlling hexose to sucrose ratio and drive cell expansion by turgor generated due to influx of osmotic solutes (Koch, 2004; Sergeeva et al., 2006; Morey et al., 2019). Consequently, vin2 and vin3 mutations lead to decreased seed size and shorter and lighter panicles (Morey et al., 2018; Lee et al., 2019; Morey et al., 2019; Xu et al., 2019; Deng et al., 2020). Other than being present as a candidate gene in the above-mentioned grain chalk-associated QTLs, OsVIN2 has not been directly implicated in the grain chalkiness. However, the OsCWIN member OsCIN2 mapped to the grain incomplete filling 1 (OsGIF1) locus was associated with grain filling and chalkiness in rice (Wang et al., 2008; Wang et al., 2018). Expression of GIF1 is unaffected, whereas both OsVIN2 and VIN3 are down regulated by high temperature and therefore it is plausible that the reduced expression of VINs contribute to reduced starch synthesis leading to increased grain chalkiness.
Glucose and fructose are also generated from phosphoenolpyruvate (PEP) which is synthesized by the action of pyruvate orthophosphate dikinase (PPDK) enzyme. Rice, a C3 plant has 3 PPDK genes, OsPPDKA encoding a cytosolic isoform (OsPPDKA); OsPPDKB, expressing a cytosolic PPDKB (cyOsPPDKB); and the chloroplastic PPDK (chOsPPDKB) (Imaizumi et al., 1997; Moons et al., 1998). Our study identified OsPPDKA as one of the candidate genes from MQTL 3.5 [contributing QTLs qPGWC3.1, qPGWC3.2, qWB, qWBA, qWBK, qET and qCA (Kobayashi et al., 2007; Mei et al., 2013; Kobayashi et al., 2013; Peng et al., 2014a; Wang et al., 2016)]. It had only 2 non-synonymous haplotypes in the 3K panel, which could not be correlated with the chalk phenotype. Mutation in cyPPDKB gene resulted in chalky grains and a floury endosperm (Kang et al., 2005). As compared to PPDKA, PPDKB has a higher expression during the milky stage in rice kernels (Kang et al., 2005). On the other hand, PPDKB is down-regulated, while PPDKA is induced in response to heat stress during grain filling in Nipponbare (Yamakawa et al., 2007). We believe that PPDKB and PPDKA have compensatory roles and their stoichiometric levels determine quantity and quality of starch under high temperature. However, this hypothesis needs further validation by comparing the relative expression of PPDKA and PPDKB in rice accessions having contrasting grain chalk phenotypes.
One of the key regulatory steps in starch biosynthesis is conversion of glucose-1-phosphate to ADP-glucose catalyzed by the heterotetrameric enzyme complex (Okita, 1992; Tetlow et al., 2004; Lee et al., 2007). The heterotetramer has 2 small (OsAGPS1 and AGPS2a/b) and 2 large subunits (AGPL1-4). AGPL1, was identified as a candidate gene in MQTL 5.5 [contributed by QTLs qPGWC5.1 and qPGWC5.2 (Chen et al., 2016; Wang et al., 2016)]. Interestingly, we did not find any non-synonymous variations in AGPL1 throughout the 3K rice genome panel. This is in agreement with Lu and Park (2012) who also could not find any variation in the AGPL1 gene in 104 rice accessions. ADP-glucose pyrophosphorylase genes, AGPL2 and AGPS2b, exhibit polymorphisms that do not alter their protein (Kharabian-Masouleh et al., 2011; Wei et al., 2017) and conservation of protein sequence is likely a general feature of all the ADP-glucose pyrophosphorylase enzyme subunits. AGPL1 is localized in amyloplasts and is expressed in the early stages of grain filling, whereas AGPL2 is localized to cytosol and is expressed in the middle to late stages of grain filling (Lee et al., 2007) and it is likely that their spatio-temporal expression determines the quantity and quality of starch.
Plants exposed to high temperatures require energy to maintain cellular homoeostasis (Sadok and Jagadish, 2020) for which it breaks down reserve starch by increasing the expression and activity of α-amylases in the ripening grains. The timing of HT stress is crucial because if it happens during the grain filling stages, increased expression and activity of α-amylases affects the formation of starch granules and at the same time increases the hydrolysis of the already deposited starch, ultimately producing chalky grains (Zakaria et al., 2002; Iwasawa et al., 2009). RNAi-mediated suppression of α-amylases reduces grain chalk formation under high temperature, conversely their overexpression enhances chalk even under ambient temperatures (Asatsuma et al., 2006; Hakata et al., 2012; Nakata et al., 2017). RAG2, a candidate gene from MQTL7.1 [contributing QTLs qDPGWC7.1, qDPGWC7.2, qPGWC7.1, qPGWC7.2, qPGWC7.3 (Wang et al., 2016)] is a 16-kDa alpha-amylase/trypsin inhibitor in rice. It is expressed in the developing seeds and is involved in both the regulation of grain yield and quality (Zhou et al., 2017). Rice grains of RAG2-RNAi lines display high chalk with loosely packed starch as compared to the wild type (Zhou et al., 2017). RAL2, also a candidate gene from MQTL 7.1, displays high homology with RAG2 and may have a similar function. We hypothesize that both RAG2 and RAL2 proteins might inhibit α-amylase activity under high temperature but further experimental validation is needed. Our study revealed that RAG2 and RAL2 genes have 4 and 2 haplotypes respectively, in the 3K RG panel. We found 2 haplotypes each for RAG2 and RAL2 in the subset panel of 60 accessions, which did not correlate with the degree of chalkiness.
We also discovered β-amylase, another starch hydrolyzing enzyme of amylase family, as a candidate gene from MQTL 3.1 [contributing QTLs qWCR, qBW, qWB, qPGWC3.1 and qPGWC 3.2 (Chen et al., 2011; Wada et al., 2015; Wang et al., 2016; Yun et al., 2016)]. Haplotype analysis in the 3K RG panel revealed that beta-amylase is conserved and had no haplotypic variations. The expression of beta-amylase increases under high temperature (Yamakawa et al., 2007) and its activity in chalky grains is higher than in non-chalky grains (Nakamura et al., 2021). An endosperm-specific β-amylase gene from barley, ESDBamy is expressed in early endosperm and a shrunken endosperm mutant shows reduced starch deposition due to high levels of its expression (Jung et al., 2001). Absence of non-synonymous haplotypes indicate that differential steady state mRNA levels of β-amylase gene might be a contributing factor for variable amounts of starch in different accessions by inequitably affecting the inhibition of starch deposition. Both RAG2 and RAL2 are induced by high temperature and it is plausible that α- and β-amylases are targets of RAG2 and RAL2, respectively. Further experiments involving expression analysis of these genes in multiple accessions with varying degrees of chalk, protein-protein interactions and enzymatic assays will be required to validate these possibilities and possibly provide additional strategies to regulate formation of grain chalk in rice.
Another major group of candidate genes were either seed storage proteins or those involved in nitrogen metabolism. We identified 5 genes each for seed storage proteins and nitrogen metabolism pathway in our meta-QTL analysis. Since both starch and proteins in grains are synthesized using sugar as the carbon source (Bao et al., 2015; Xin et al., 2019), an optimal coordination between the C and N metabolism during grain filling is crucial, but less worked upon. Reports suggest that the nitrogen metabolism pathway and synthesis of storage proteins in particular may lead to grain chalk formation in rice (Han et al., 2012; Xi et al., 2021). A significant negative correlation between prolamin content and chalkiness has also been discovered (Wang et al., 2021). It would be interesting to systematically investigate these promising candidate genes to define their roles in grain chalkiness.
5.2 Natural variations of GBSSI and SSIIa influence the quality of rice grains
Amylose and amylopectin synthesis in the rice endosperm are regulated GBSSI and SSIIa enzymes, respectively, genes for which are located on chromosome 6 (Wang et al., 1990, Figure 5A). GBSSI, a candidate gene identified in the current study from MQTL 6.1, was found in 14 mapped QTLs [qWCR, qCR, qWBA, qPCG1, qPCG2, qDC1, qDC2, qDC3, qACE, qPGWC1, qPGWC2, qCA, qWC and qWB (Peng et al., 2014a; Mei et al., 2013; Kobayashi et al., 2007; Zheng et al., 2012; Lu et al., 2013; Gao et al., 2016a)]. Similarly, MQTL 6.3 from which SSIIa gene was identified as a candidate, was derived from 7 overlapping QTLs [qDPGWC, qWCR, qCA, qET, qCA, qch, qWBR (Wang et al., 2016; Mei et al., 2013; Tan et al., 2000; Peng et al., 2014a; Yun et al., 2016)]. Such a high number of QTLs from many different studies signifies that these genomic regions and the underlying candidate genes immensely contribute to the grain chalk trait.
It is known that the diversity of grain amylose content is largely due to allelic variations at the Wx locus (Tian et al., 2009; Biselli et al., 2014; Zhang et al., 2019). To date, at least eight Wx alleles, Wxlv, Wxa, Wxin, Wxb, Wxop/hp, Wxmq, Wxmp, and wx, have been shown to be associated with the five possible amylose types observed in rice cultivars (Cai et al., 1998; Sato et al., 2002; Larkin and Park, 2003; Wanchana et al., 2003; Mikami et al., 2008; Liu et al., 2009; Yang et al., 2013; Zhang et al., 2019). In the present study we identified Wx alleles, Wxlv, Wxa, Wxb and Wxop/hp leading to the detection of 4 GBSSI haplotypes. Previously Wxlv has been associated with high amylose content (Supplementary Table 10, Zhang et al., 2019), which could result in a low chalk accumulation phenotype. Wxlv corresponded with 4 low, 4 intermediate and 3 high chalk phenotypes in our study, indicating that allelic combinations of other alleles with the Wxlv allele possibly determines the degree of chalkiness. While Wxa allele was primarily associated with 5 intermediate and 1 high chalk type rice, accessions having high chalk almost always had Wxb and Wxop/hp alleles.
Most of the indica rice cultivars possess active-type SSIIa (wild-type), whereas typical japonica rice cultivars possess mutant SSIIa harboring three amino acid substitutions in the active site, thus exhibiting only 10% of SSIIa activity relative to indica rice (Nakamura et al., 2005). Low SSIIa activity in japonica rice leads to a reduction in amylopectin branches with degree of polymerization (DP) of 13-24, and an increase in short amylopectin chains with DP ≤ 12 (Umemoto et al., 1999; Umemoto et al., 2002; Nakamura et al., 2005). The four haplotypic combinations in our study were derived from the three substitutions. The GGC haplotype was associated with low and intermediate chalk accessions, intermediate chalk accessions had AGC, while AGT and AAC were linked with high chalk accessions, indicating that a combination of substitutions in the active site of SSIIa enzyme is a critical determinant of chalk formation.
Since, elevated temperatures affect the accumulation of starch (Umemoto et al., 1995) by disturbing the proportion of amylose to amylopectin (Inouchi et al., 2000), therefore, understanding how various allelic combinations of closely located starch biosynthetic genes, GBSSI and SSIIa, affect starch properties and grain chalkiness is of utmost importance. Haplotypes GGGAC, TGAAC in GBSSI (Cai et al., 1998; Mikami et al., 1999; Larkin and Park, 2003) and AGT and AAC in SSIIa (Umemoto et al., 1999; Umemoto et al., 2002; Nakamura et al., 2005) have low amylose and low amylopectin. Our chalky score and scanning electron microscopy data revealed that the combinations GGGAC/AGT, TGAAC/AGT and TGAAC/AAC in accessions invariably led to high chalk accumulation (Figure 9B). Starch quality in rice grains is a trait that has evolved in response to strong selection of starch synthesis pathway genes during domestication (Whitt et al., 2002). Rice varieties with high amylose levels (20–30%) tend to form discrete, non-cohesive grains when cooked and are found in South and Southeast Asian rices classified as indica and tropical japonica variety groups, whereas varieties with low amylose, a characteristic of temperate japonica variety, are common in northeast Asia and form cohesive cooked grains (Morishima and Sano, 1992; Juliano and Villareal, 1993). Because GBSSI and SSIIa are closely located on chromosome 6, it is possible that these two genes may have undergone co-selection during domestication. Further, rice germplasm harboring different haplotypic combinations of these starch synthesizing genes have likely been selected on the basis of regional palatability. Nevertheless, the haplotype combinations associated with low grain chalk will be helpful in developing elite varieties with high head rice yield.
6 Conclusions and future directions
Meta-QTL analysis is a powerful tool for detecting the most precise and concurrent QTLs for any agronomic trait in crops. Identification of differentially expressed MQTL genes utilizing trait-specific transcriptome datasets, provides additional means for refining the process of shortlisting the significant candidate genes. Harnessing information of haplotypic diversity from sequenced accessions and associating it with the phenotype can provide information on novel allelic/haplotype combinations contributing to traits of economic importance. Our work combined all these approaches and unveiled haplotype combinations in two main starch synthesis genes, which can be genetically linked with varying degrees of grain chalkiness in rice. The favorable allelic combinations that promote biosynthesis of optimum quality starch and thereby contribute to reduced grain chalk, can be backcrossed with high-yielding elite varieties or CRISPR-Cas based prime editing to generate superior rice plants with low grain chalkiness and high HRY traits.
Data availability statement
The original contributions presented in the study are included in the article/Supplementary Material. Further inquiries can be directed to the corresponding author.
Author contributions
MA and AK planned and designed the research. AK, DS, and KK performed the meta-analysis. AK and DS mined the candidate genes and performed the in-silico promoter analysis. AK and S analyzed expression data. AK, DS, CW, DC, AP, GC, and MS did the phenotyping. AK, MI, and PK performed the haplotype analysis. AK and PS performed the haplo-pheno analysis. SK and VV prepared the SEM images. AK, MA, SK-A, AG, and SJ wrote the manuscript. All authors contributed to the article and approved the submitted version.
Funding
Support to the laboratory of MA was provided by National Agricultural Science Fund (NASF) grant (NASF/ABP-7O14/2018-19) and Faculty Research Program (FRP) grant ((IoE/FRP/LS/2020/27) from Institute of Eminence (IoE), Delhi University.
Acknowledgments
SK-A acknowledges the Faculty Research Program (FRP) grant (IoE/2021/12/FRP) from Institute of Eminence (IoE), Delhi University. AK is grateful for the research fellowship from the Council of Scientific and Industrial Research (CSIR), India.
Conflict of interest
The authors declare that the research was conducted in the absence of any commercial or financial relationships that could be construed as a potential conflict of interest.
Publisher’s note
All claims expressed in this article are solely those of the authors and do not necessarily represent those of their affiliated organizations, or those of the publisher, the editors and the reviewers. Any product that may be evaluated in this article, or claim that may be made by its manufacturer, is not guaranteed or endorsed by the publisher.
Supplementary material
The Supplementary Material for this article can be found online at: https://www.frontiersin.org/articles/10.3389/fpls.2023.1133115/full#supplementary-material
References
Abdullah-Zawawi, M. R., Ahmad-Nizammuddin, N. F., Govender, N., Harun, S., Mohd-Assaad, N., Mohamed-Hussein, Z. A. (2021). Comparative genome-wide analysis of WRKY, MADS-box and MYB transcription factor families in arabidopsis and rice. Sci. Rep. 11 (1), 1–18. doi: 10.1038/s41598-021-99206-y
Aoki, N., Hirose, T., Scofield, G. N., Whitfeld, P. R., Furbank, R. T. (2003). The sucrose transporter gene family in rice. Plant Cell Physiol. 44 (3), 223–232. doi: 10.1093/pcp/pcg030
Arcade, A., Labourdette, A., Falque, M., Mangin, B., Chardon, F., Charcosset, A., et al. (2004). BioMercator: integrating genetic maps and QTL towards discovery of candidate genes. Bioinformatics 20 (14), 2324–2326. doi: 10.1093/bioinformatics/bth230
Asatsuma, S., Sawada, C., Kitajima, A., Asakura, T., Mitsui, T. (2006). α-amylase affects starch accumulation in the rice grain. J. Appl. Glycosci. 53, 187–192. doi: 10.5458/jag.53.187
Bai, A. N., Lu, X. D., Li, D. Q., Liu, J. X., Liu, C. M. (2016). NF-YB1-regulated expression of sucrose transporters in aleurone facilitates sugar loading to rice endosperm. Cell Res. 26 (3), 384–388. doi: 10.1038/cr.2015.116
Bakku, R. K., Rakwal, R., Shibato, J., Cho, K., Kikuchi, S., Yonekura, M., et al. (2020). Transcriptomics of mature rice (Oryza sativa l. koshihikari) seed under hot conditions by DNA microarray analyses. Atmosphere 11 (5), 528.
Bao, A., Zhao, Z., Ding, G., Shi, L., Xu, F., Cai, H. (2015). The stable level of glutamine synthetase 2 plays an important role in rice growth and in carbon-nitrogen metabolic balance. Int. J. Mol. Sci. 16 (6), 12713–12736. doi: 10.3390/ijms160612713
Bazrkar-Khatibani, L., Fakheri, B. A., Hosseini-Chaleshtori, M., Mahender, A., Mahdinejad, N., Ali, J. (2019). Genetic mapping and validation of quantitative trait loci (QTL) for the grain appearance and quality traits in rice (Oryza sativa l.) by using recombinant inbred line (RIL) population. Int. J. Genomics 2019.
Bian, J. M., Shi, H., Li, C. J., Zhu, C. L., Yu, Q. Y., Peng, X. S., et al. (2013). QTL mapping and correlation analysis for 1000-grain weight and percentage of grains with chalkiness in rice. J. Genet. 92 (2), 281–287. doi: 10.1007/s12041-013-0267-6
Biselli, C., Cavalluzzo, D., Perrini, R., Gianinetti, A., Bagnaresi, P., Urso, S., et al. (2014). Improvement of marker-based predictability of apparent amylose content in japonica rice through GBSSI allele mining. Rice 7 (1), 1–18. doi: 10.1186/1939-8433-7-1
Butardo, V. M., Fitzgerald, M. A., Bird, A. R., Gidley, M. J., Flanagan, B. M., Larroque, O., et al. (2011). Impact of down-regulation of starch branching enzyme IIb in rice by artificial microRNA-and hairpin RNA-mediated RNA silencing. J. Exp. Bot. 62 (14), 4927–4941. doi: 10.1093/jxb/err188
Cai, X. L., Wang, Z. Y., Xing, Y. Y., Zhang, J. L., Hong, M. M. (1998). Aberrant splicing of intron 1 leads to the heterogeneous 5′ UTR and decreased expression of waxy gene in rice cultivars of intermediate amylose content. Plant J. 14 (4), 459–465. doi: 10.1046/j.1365-313X.1998.00126.x
Caliński, T., Harabasz, J. (1974). A dendrite method for cluster analysis. Communications in Statistics-theory and Methods 3(1), 1–27.
Chandusingh, P. R., Singh, N. K., Prabhu, K. V., Vinod, K. K., Singh, A. K. (2013). Molecular mapping of quantitative trait loci for grain chalkiness in rice (Oryza sativa l.). Indian J. Genet. Plant Breed. 73 (3), 244–251. doi: 10.5958/j.0975-6906.73.3.036
Chen, L., Gao, W., Chen, S., Wang, L., Zou, J., Liu, Y., et al. (2016). High-resolution QTL mapping for grain appearance traits and co-localization of chalkiness-associated differentially expressed candidate genes in rice. Rice 9 (1), 1–17. doi: 10.1186/s12284-016-0121-6
Chen, H. M., Zhao, Z. G., Jiang, L., Wan, X. Y., Liu, L. L., Wu, X. J., et al. (2011). Molecular genetic analysis on percentage of grains with chalkiness in rice (Oryza sativa l.). Afr. J. Biotechnol. 10 (36), 6891–6903.
Cho, J. I., Kim, H. B., Kim, C. Y., Hahn, T. R., Jeon, J. S. (2011). Identification and characterization of the duplicate rice sucrose synthase genes OsSUS5 and OsSUS7 which are associated with the plasma membrane. Molecules Cells 31 (6), 553–561. doi: 10.1007/s10059-011-1038-y
Cooper, N. T. W., Siebenmorgen, T. J., Counce, P. A. (2008). Effects of nighttime temperature during kernel development on rice physicochemical properties. Cereal Chem. 85 (3), 276–282. doi: 10.1094/CCHEM-85-3-0276
Dai, L., Wang, L., Leng, Y., Yang, Y., Huang, L., Chen, L., et al. (2016). Quantitative trait loci mapping for appearance quality in short-grain rice. Crop Sci. 56 (4), 1484–1492. doi: 10.2135/cropsci2015.07.0404
Deng, X., Han, X., Yu, S., Liu, Z., Guo, D., He, Y., et al. (2020). OsINV3 and its homolog, OsINV2, control grain size in rice. Int. J. Mol. Sci. 21 (6), 2199. doi: 10.3390/ijms21062199
Denyer, K., Clarke, B., Hylton, C., Tatge, H., Smith, A. M. (1996). The elongation of amylose and amylopectin chains in isolated starch granules. Plant J. 10 (6), 1135–1143. doi: 10.1046/j.1365-313X.1996.10061135.x
Denyer, K., Hylton, C. M., Jenner, C. F., Smith, A. M. (1995). Identification of multiple isoforms of soluble and granule-bound starch synthase in developing wheat endosperm. Planta 196 (2), 256–265. doi: 10.1007/BF00201382
Edwards, J. D., Jackson, A. K., McClung, A. M. (2017). Genetic architecture of grain chalk in rice and interactions with a low phytic acid locus. Field Crops Res. 205, 116–123. doi: 10.1016/j.fcr.2017.01.015
Eizenga, G. C., Chen, M. H., Jia, M. H., Jackson, A. K., Edwards, J. D. (2019). Registration of the estrela× NSFTV199 rice recombinant inbred line mapping population. J. Plant Registrations 13 (3), 469–478. doi: 10.3198/jpr2019.04.0019crmp
Ezcurra, I., Ellerström, M., Wycliffe, P., Stålberg, K., Rask, L. (1999). Interaction between composite elements in the napA promoter: both the b-box ABA-responsive complex and the RY/G complex are necessary for seed-specific expression. Plant Mol. Biol. 40 (4), 699–709. doi: 10.1023/A:1006206124512
FAO (2019) Food and agricultural organization of the united nations. FAOSTAT. Available at: http://www.fao.org/faostat/en/#data/QC.
Fujita, N., Yoshida, M., Kondo, T., Saito, K., Utsumi, Y., Tokunaga, T., et al. (2007). Characterization of SSIIIa-deficient mutants of rice: the function of SSIIIa and pleiotropic effects by SSIIIa deficiency in the rice endosperm. Plant Physiol. 144 (4), 2009–2023. doi: 10.1104/pp.107.102533
Gao, Y., Liu, C., Li, Y., Zhang, A., Dong, G., Xie, L., et al. (2016b). QTL analysis for chalkiness of rice and fine mapping of a candidate gene for qACE9. Rice 9 (1), 1–10. doi: 10.1186/s12284-016-0114-5
Gao, F. Y., Zeng, L. H., Ling, Q. I. U., Lu, X. J., Ren, J. S., Wu, X. T., et al. (2016a). QTL mapping of grain appearance quality traits and grain weight using a recombinant inbred population in rice (Oryza sativa l.). J. Integr. Agric. 15 (8), 1693–1702. doi: 10.1016/S2095-3119(15)61259-X
Garretón, V., Carpinelli, J., Jordana, X., Holuigue, L. (2002). The as-1 promoter element is an oxidative stress-responsive element and salicylic acid activates it via oxidative species. Plant Physiol. 130 (3), 1516–1526. doi: 10.1104/pp.009886
Ge, S. X., Jung, D., Yao, R. (2020). ShinyGO: a graphical gene-set enrichment tool for animals and plants. Bioinformatics 36 (8), 2628–2629. doi: 10.1093/bioinformatics/btz931
Goffinet, B., Gerber, S. (2000). Quantitative trait loci: a meta-analysis. Genetics 155 (1), 463–473. doi: 10.1093/genetics/155.1.463
Guo, T., Liu, X., Wan, X., Weng, J., Liu, S., Liu, X., et al. (2011). Identification of a stable quantitative trait locus for percentage grains with white chalkiness in rice (Oryza sativa). J. Integr. Plant Biol. 53 (8), 598–607. doi: 10.1111/j.1744-7909.2011.01041.x
Hakata, M., Kuroda, M., Miyashita, T., Yamaguchi, T., Kojima, M., Sakakibara, H., et al. (2012). Suppression of α-amylase genes improves quality of rice grain ripened under high temperature. Plant Biotechnol. J. 10 (9), 1110–1117. doi: 10.1111/j.1467-7652.2012.00741.x
Han, X., Wang, Y., Liu, X., Jiang, L., Ren, Y., Liu, F., et al. (2012). The failure to express a protein disulphide isomerase-like protein results in a floury endosperm and an endoplasmic reticulum stress response in rice. J. Exp. Bot. 63 (1), 121–130. doi: 10.1093/jxb/err262
Hao, W., Zhu, M. Z., Gao, J. P., Sun, S. Y., Lin, H. X. (2009). Identification of quantitative trait loci for rice quality in a population of chromosome segment substitution lines. J. Integr. Plant Biol. 51 (5), 500–512. doi: 10.1111/j.1744-7909.2009.00822.x
He, P., Li, S. G., Qian, Q., Ma, Y. Q., Li, J. Z., Wang, W. M., et al. (1999). Genetic analysis of rice grain quality. Theor. Appl. Genet. 98 (3), 502–508. doi: 10.1007/s001220051098
Hirose, T., Scofield, G. N., Terao, T. (2008). An expression analysis profile for the entire sucrose synthase gene family in rice. Plant Sci. 174 (5), 534–543. doi: 10.1016/j.plantsci.2008.02.009
Hossain, M., Bose, M. L., Mustafi, B. A. (2006). Adoption and productivity impact of modern rice varieties in Bangladesh. developing economies 44 (2), 149–166. doi: 10.1111/j.1746-1049.2006.00011.x
Hruz, T., Laule, O., Szabo, G., Wessendorp, F., Bleuler, S., Oertle, L., et al. (2008). Genevestigator v3: a reference expression database for the meta-analysis of transcriptomes. Adv. Bioinf. 2008. doi: 10.1155/2008/420747
Hu, T., He, S., Yang, G., Zeng, H., Wang, G., Chen, Z., et al. (2011). Isolation and characterization of a rice glutathione s-transferase gene promoter regulated by herbicides and hormones. Plant Cell Rep. 30 (4), 539–549. doi: 10.1007/s00299-010-0964-z
Huang, J. W., Chen, J. T., Yu, W. P., Shyur, L. F., Wang, A. Y., Sung, H. Y., et al. (1996). Complete structures of three rice sucrose synthase isogenes and differential regulation of their expressions. Biosci. biotechnol. Biochem. 60 (2), 233–239. doi: 10.1271/bbb.60.233
Huang, Y. C., Hsiang, E. C., Yang, C. C., Wang, A. Y. (2016). New insight into the catalytic properties of rice sucrose synthase. Plant Mol. Biol. 90 (1), 127–135. doi: 10.1007/s11103-015-0401-3
Imaizumi, N., Ku, M. S., Ishihara, K., Samejima, M., Kaneko, S., Matsuoka, M. (1997). Characterization of the gene for pyruvate, orthophosphate dikinase from rice, a C3 plant, and a comparison of structure and expression between C3 and C4 genes for this protein. Plant Mol. Biol. 34 (5), 701–716. doi: 10.1023/A:1005884515840
Inouchi, N., Ando, H., Asaoka, M., Okuno, K., Fuwa, H. (2000). The effect of environmental temperature on distribution of unit chains of rice amylopectin. Starch-Stärke 52 (1), 8–12. doi: 10.1002/(SICI)1521-379X(200001)52:1<8::AID-STAR8>3.0.CO;2-Q
Ishimaru, T., Parween, S., Saito, Y., Shigemitsu, T., Yamakawa, H., Nakazono, M., et al. (2019). Laser microdissection-based tissue-specific transcriptome analysis reveals a novel regulatory network of genes involved in heat-induced grain chalk in rice endosperm. Plant Cell Physiol. 60 (3), 626–642. doi: 10.1093/pcp/pcy233
Isshiki, M., Morino, K., Nakajima, M., Okagaki, R. J., Wessler, S. R., Izawa, T., et al. (1998). A naturally occurring functional allele of the rice waxy locus has a GT to TT mutation at the 5′ splice site of the first intron. Plant J. 15 (1), 133–138. doi: 10.1046/j.1365-313X.1998.00189.x
Iwasawa, N., Umemoto, T., Hiratsuka, M., Nitta, Y., Matsuda, T., Kondo, M. (2009). Structural characters of milky-white rice grains caused by high temperature and shading during grain-filling. Jpn J. Crop Sci. 78, 322–323.
Juliano, B. O., Villareal, C. P. (1993). Grain quality evaluation of world rices. Int. Rice Res. Inst.
Jung, W., Skadsen, R. W., Peterson, D. M. (2001). Characterization of a novel barley β-amylase gene expressed only during early grain development. Seed Sci. Res. 11 (4), 325–333.
Kabir, S. M. T., Hossain, M. S., Bashar, K. K., Honi, U., Ahmed, B., Emdad, E. M., et al. (2021). Genome-wide identification and expression profiling of AP2/ERF superfamily genes under stress conditions in dark jute (Corchorus olitorius l.). Ind. Crops Products 166, 113469. doi: 10.1016/j.indcrop.2021.113469
Kang, H. G., Park, S., Matsuoka, M., An, G. (2005). White-core endosperm floury endosperm-4 in rice is generated by knockout mutations in the C4-type pyruvate orthophosphate dikinase gene (OsPPDKB). Plant J. 42 (6), 901–911. doi: 10.1111/j.1365-313X.2005.02423.x
Kepiro, J. L., McClung, A. M., Chen, M. H., Yeater, K. M., Fjellstrom, R. G. (2008). Mapping QTLs for milling yield and grain characteristics in a tropical japonica long grain cross. J. Cereal Sci. 48 (2), 477–485. doi: 10.1016/j.jcs.2007.12.001
Khahani, B., Tavakol, E., Shariati J, V. (2019). Genome-wide meta-analysis on yield and yield-related QTLs in barley (Hordeum vulgare l.). Mol. Breed. 39 (4), 1–16. doi: 10.1007/s11032-019-0962-y
Khahani, B., Tavakol, E., Shariati, V., Rossini, L. (2021). Meta-QTL and ortho-MQTL analyses identified genomic regions controlling rice yield, yield-related traits and root architecture under water deficit conditions. Scientific reports 11(1), 6942.
Kharabian-Masouleh, A., Waters, D. L., Reinke, R. F., Henry, R. J. (2011). Discovery of polymorphisms in starch-related genes in rice germplasm by amplification of pooled DNA and deeply parallel sequencing. Plant Biotechnol. J. 9 (9), 1074–1085. doi: 10.1111/j.1467-7652.2011.00629.x
Kobayashi, A., Genliang, B., Shenghai, Y., Tomita, K. (2007). Detection of quantitative trait loci for white-back and basal-white kernels under high temperature stress in japonica rice varieties. Breed. Sci. 57 (2), 107–116. doi: 10.1270/jsbbs.57.107
Kobayashi, A., Sonoda, J., Sugimoto, K., Kondo, M., Iwasawa, N., Hayashi, T., et al. (2013). Detection and verification of QTLs associated with heat-induced quality decline of rice (Oryza sativa l.) using recombinant inbred lines and near-isogenic lines. Breed. Sci. 63 (3), 339–346.
Koch, K. (2004). Sucrose metabolism: regulatory mechanisms and pivotal roles in sugar sensing and plant development. Curr. Opin. Plant Biol. 7 (3), 235–246. doi: 10.1016/j.pbi.2004.03.014
Kong, W., Zhang, C., Qiang, Y., Zhong, H., Zhao, G., Li, Y. (2020). Integrated RNA-seq analysis and meta-QTLs mapping provide insights into cold stress response in rice seedling roots. Int. J. Mol. Sci. 21 (13), 4615. doi: 10.3390/ijms21134615
Kumar, I. S., Nadarajah, K. (2020). A meta-analysis of quantitative trait loci associated with multiple disease resistance in rice (Oryza sativa l.). Plants 9 (11), 1491. doi: 10.3390/plants9111491
Larkin, P. D., Park, W. D. (2003). Association of waxy gene single nucleotide polymorphisms with starch characteristics in rice (Oryza sativa l.). Mol. Breed. 12 (4), 335–339. doi: 10.1023/B:MOLB.0000006797.51786.92
Le, T. T. (2016). Effects of climate change on rice yield and rice market in Vietnam. J. Agric. Appl. Economics 48 (4), 366–382. doi: 10.1017/aae.2016.21
Lee, S. K., Hwang, S. K., Han, M., Eom, J. S., Kang, H. G., Han, Y., et al. (2007). Identification of the ADP-glucose pyrophosphorylase isoforms essential for starch synthesis in the leaf and seed endosperm of rice (Oryza sativa l.). Plant Mol. Biol. 65 (4), 531–546. doi: 10.1007/s11103-007-9153-z
Lee, D. W., Lee, S. K., Rahman, M. M., Kim, Y. J., Zhang, D., Jeon, J. S. (2019). The role of rice vacuolar invertase2 in seed size control. Molecules Cells 42 (10), 711.
Lescot, M., Déhais, P., Thijs, G., Marchal, K., Moreau, Y., Van de Peer, Y., et al. (2002). PlantCARE, a database of plant cis-acting regulatory elements and a portal to tools for in silico analysis of promoter sequences. Nucleic Acids Res. 30 (1), 325–327. doi: 10.1093/nar/30.1.325
Lestari, P., Lee, G., Ham, T. H., Woo, M. O., Piao, R., Jiang, W., et al. (2011). Single nucleotide polymorphisms and haplotype diversity in rice sucrose synthase 3. J. Heredity 102 (6), 735–746. doi: 10.1093/jhered/esr094
Li, Y., Fan, C., Xing, Y., Yun, P., Luo, L., Yan, B., et al. (2014). Chalk5 encodes a vacuolar h+-translocating pyrophosphatase influencing grain chalkiness in rice. Nat. Genet. 46 (4), 398–404. doi: 10.1038/ng.2923
Li, J. Y., Wang, J., Zeigler, R. S. (2014). The 3,000 rice genomes project: new opportunities and challenges for future rice research. Gigascience 3 (1), 2047–217X. doi: 10.1186/2047-217X-3-8
Li, J., Xiao, J., Grandillo, S., Jiang, L., Wan, Y., Deng, Q., et al. (2004). QTL detection for rice grain quality traits using an interspecific backcross population derived from cultivated Asian (O. sativa l.) and African (O. glaberrima s.) rice. Genome 47 (4), 697–704.
Lin, Z., Wang, Z., Zhang, X., Liu, Z., Li, G., Wang, S., et al. (2017). Complementary proteome and transcriptome profiling in developing grains of a notched-belly rice mutant reveals key pathways involved in chalkiness formation. Plant Cell Physiol. 58 (3), 560–573. doi: 10.1093/pcp/pcx001
Lisle, A. J., Martin, M., Fitzgerald, M. A. (2000). Chalky and translucent rice grains differ in starch composition and structure and cooking properties. Cereal Chem. 77 (5), 627–632. doi: 10.1094/CCHEM.2000.77.5.627
Liu, X., Guo, T., Wan, X., Wang, H., Zhu, M., Li, A., et al. (2010b). Transcriptome analysis of grain-filling caryopses reveals involvement of multiple regulatory pathways in chalky grain formation in rice. BMC Genomics 11 (1), 1–15. doi: 10.1186/1471-2164-11-730
Liu, L., Ma, X., Liu, S., Zhu, C., Jiang, L., Wang, Y., et al. (2009). Identification and characterization of a novel waxy allele from a yunnan rice landrace. Plant Mol. Biol. 71 (6), 609–626. doi: 10.1007/s11103-009-9544-4
Liu, X., Wang, Y., Wang, S. W. (2012). QTL analysis of percentage of grains with chalkiness in japonica rice (Oryza sativa). Genet. Mol. Res. 11 (1), 717–724. doi: 10.4238/2012.March.22.1
Liu, F., Xu, W., Song, Q., Tan, L., Liu, J., Zhu, Z., et al. (2013). Microarray-assisted fine-mapping of quantitative trait loci for cold tolerance in rice. Mol. Plant 6 (3), 757–767. doi: 10.1093/mp/sss161
Liu, G., Zhu, H., Liu, S., Zeng, R., Zhang, Z., Li, W., et al. (2010a). Unconditional and conditional QTL mapping for the developmental behavior of tiller number in rice (Oryza sativa l.). Genetica 138 (8), 885–893. doi: 10.1007/s10709-010-9471-y
Lu, B., Yang, C., Xie, K., Zhang, L., Wu, T., Li, L., et al. (2013). Quantitative trait loci for grain-quality traits across a rice F2 population and backcross inbred lines. Euphytica 192 (1), 25–35. doi: 10.1007/s10681-012-0822-x
Lu, F. H., Park, Y. J. (2012). Sequence variations in OsAGPase significantly associated with amylose content and viscosity properties in rice (Oryza sativa L.). Genetics Research 94(4), 179–189.
Lyman, N. B., Jagadish, K. S., Nalley, L. L., Dixon, B. L., Siebenmorgen, T. (2013). Neglecting rice milling yield and quality underestimates economic losses from high-temperature stress. PloS One 8 (8), e72157. doi: 10.1371/journal.pone.0072157
MacNeill, G. J., Mehrpouyan, S., Minow, M. A., Patterson, J. A., Tetlow, I. J., Emes, M. J., et al. (2017). Starch as a source, starch as a sink: the bifunctional role of starch in carbon allocation. J. Exp. Bot. 68 (16), 4433–4453. doi: 10.1093/jxb/erx291
Mansueto, L., Fuentes, R. R., Chebotarov, D., Borja, F. N., Detras, J., Abriol-Santos, J. M., et al. (2016). SNP-seek II: A resource for allele mining and analysis of big genomic data in oryza sativa. Curr. Plant Biol. 7, 16–25. doi: 10.1016/j.cpb.2016.12.003
Martinez-Pastor, M. T., Marchler, G., Schüller, C., Marchler-Bauer, A., Ruis, H., Estruch, F. (1996). The saccharomyces cerevisiae zinc finger proteins Msn2p and Msn4p are required for transcriptional induction through the stress response element (STRE). EMBO J. 15 (9), 2227–2235. doi: 10.1002/j.1460-2075.1996.tb00576.x
Mei, D., Yu, Z., Gong, C., Chen, X., Zhu, Q., Wang, S. (2018). Validation and fine mapping a multifunction region qPCG10/qDC10 conferring chalkiness and grain shape of rice. Crop Sci. 58 (2), 639–649. doi: 10.2135/cropsci2017.07.0405
Mei, D. Y., Zhu, Y. J., Yu, Y. H., Fan, Y. Y., Huang, D. R., Zhuang, J. Y. (2013). Quantitative trait loci for grain chalkiness and endosperm transparency detected in three recombinant inbred line populations of indica rice. J. Integr. Agric. 12 (1), 1–11. doi: 10.1016/S2095-3119(13)60199-9
Miao, Z. H., Lam, E. (1995). Targeted disruption of the TGA3 locus in arabidopsis thaliana. Plant J. 7 (2), 359–365. doi: 10.1046/j.1365-313X.1995.7020359.x
Mikami, I., Aikawa, M., Hirano, H. Y., Sano, Y. (1999). Altered tissue-specific expression at the wx gene of the opaque mutants in rice. Euphytica 105 (2), 91–97. doi: 10.1023/A:1003457209225
Mikami, I., Uwatoko, N., Ikeda, Y., Yamaguchi, J., Hirano, H. Y., Suzuki, Y., et al. (2008). Allelic diversification at the wx locus in landraces of Asian rice. Theor. Appl. Genet. 116 (7), 979–989. doi: 10.1007/s00122-008-0729-z
Mirdar Mansuri, R., Shobbar, Z. S., Babaeian Jelodar, N., Ghaffari, M., Mohammadi, S. M., Daryani, P. (2020). Salt tolerance involved candidate genes in rice: an integrative meta-analysis approach. BMC Plant Biol. 20 (1), 1–14.
Miyahara, K., Wada, T., Sonoda, J. Y., Tsukaguchi, T., Miyazaki, M., Tsubone, M., et al. (2017). Detection and validation of QTLs for milky-white grains caused by high temperature during the ripening period in japonica rice. Breed. Sci. 67(4), 333–339. doi: 10.1270/jsbbs.16203
Moons, A., Valcke, R., Van, M. (1998). Low-oxygen stress and water deficit induce cytosolic pyruvate orthophosphate dikinase (PPDK) expression in roots of rice, a C3 plant. Plant J. 15 (1), 89–98. doi: 10.1046/j.1365-313X.1998.00185.x
Morey, S. R., Hirose, T., Hashida, Y., Miyao, A., Hirochika, H., Ohsugi, R., et al. (2018). Genetic evidence for the role of a rice vacuolar invertase as a molecular sink strength determinant. Rice 11 (1), 1–13. doi: 10.1186/s12284-018-0201-x
Morey, S. R., Hirose, T., Hashida, Y., Miyao, A., Hirochika, H., Ohsugi, R., et al. (2019). Characterisation of a rice vacuolar invertase isoform, OsINV2, for growth and yield-related traits. Funct. Plant Biol. 46 (8), 777–785. doi: 10.1071/FP18291
Morishima, H. I. R. O. K. O., Sano, Y. O. S. H. I. O. (1992). Evolutionary studies in cultivated rice. Oxf. Surv. Evol. Biol. 8, 135.
Muthayya, S., Sugimoto, J. D., Montgomery, S., Maberly, G. F. (2014). An overview of global rice production, supply, trade, and consumption. Ann. New york Acad. Sci. 1324 (1), 7–14. doi: 10.1111/nyas.12540
Nakamura, Y., Francisco, P. B., Hosaka, Y., Sato, A., Sawada, T., Kubo, A., et al. (2005). Essential amino acids of starch synthase IIa differentiate amylopectin structure and starch quality between japonica and indica rice varieties. Plant Mol. Biol. 58 (2), 213–227. doi: 10.1007/s11103-005-6507-2
Nakamura, S., Satoh, A., Aizawa, M., Ohtsubo, K. I. (2021). Characteristics of physicochemical properties of chalky grains of japonica rice generated by high temperature during ripening. Foods 11 (1), 97. doi: 10.3390/foods11010097
Nakata, M., Fukamatsu, Y., Miyashita, T., Hakata, M., Kimura, R., Nakata, Y., et al. (2017). High temperature-induced expression of rice α-amylases in developing endosperm produces chalky grains. Front. Plant Sci. 8, 2089. doi: 10.3389/fpls.2017.02089
Nelson, J. C., McClung, A. M., Fjellstrom, R. G., Moldenhauer, K. A. K., Boza, E., Jodari, F., et al. (2011). Mapping QTL main and interaction influences on milling quality in elite US rice germplasm. Theor. Appl. Genet. 122 (2), 291–309. doi: 10.1007/s00122-010-1445-z
Okada, S., Suehiro, M., Ebana, K., Hori, K., Onogi, A., Iwata, H., et al. (2017). Genetic dissection of grain traits in yamadanishiki, an excellent sake-brewing rice cultivar. Theor. Appl. Genet. 130 (12), 2567–2585. doi: 10.1007/s00122-017-2977-2
Okita, T. W. (1992). Is there an alternative pathway for starch synthesis? Plant Physiol. 100 (2), 560–564.
Peng, Y., Hu, Y., Mao, B., Xiang, H., Shao, Y., Pan, Y., et al. (2016). Genetic analysis for rice grain quality traits in the YVB stable variant line using RAD-seq. Mol. Genet. Genomics 291 (1), 297–307. doi: 10.1007/s00438-015-1104-9
Peng, B., Wang, L., Fan, C., Jiang, G., Luo, L., Li, Y., et al. (2014a). Comparative mapping of chalkiness components in rice using five populations across two environments. BMC Genet. 15 (1), 1–14. doi: 10.1186/1471-2156-15-49
Peng, C., Wang, Y., Liu, F., Ren, Y., Zhou, K., Lv, J., et al. (2014b). FLOURY ENDOSPERM 6 encodes a CBM 48 domain-containing protein involved in compound granule formation and starch synthesis in rice endosperm. Plant J. 77 (6), 917–930. doi: 10.1111/tpj.12444
Qin, Y., Kim, S. M., Sohn, J. K. (2009). Genetic analysis and QTL mapping for grain chalkiness characteristics of brown rice (Oryza sativa l.). Genes Genomics 31 (2), 155–164. doi: 10.1007/BF03191148
Raza, Q., Riaz, A., Bashir, K., Sabar, M. (2020). Reproductive tissues-specific meta-QTLs and candidate genes for development of heat-tolerant rice cultivars. Plant Mol. Biol. 104 (1), 97–112. doi: 10.1007/s11103-020-01027-6
Sadok, W., Jagadish, S. K. (2020). The hidden costs of nighttime warming on yields. Trends Plant Sci. 25 (7), 644–651. doi: 10.1016/j.tplants.2020.02.003
Sakai, H., Lee, S. S., Tanaka, T., Numa, H., Kim, J., Kawahara, Y., et al. (2013). Rice annotation project database (RAP-DB): an integrative and interactive database for rice genomics. Plant Cell Physiol. 54 (2), e6–e6. doi: 10.1093/pcp/pcs183
Sano, Y. (1984). Differential regulation of waxy gene expression in rice endosperm. Theor. Appl. Genet. 68 (5), 467–473. doi: 10.1007/BF00254822
Sato, H., Suzuki, Y., Sakai, M., Imbe, T. (2002). Molecular characterization of wx-mq, a novel mutant gene for low-amylose content in endosperm of rice (Oryza sativa l.). Breed. Sci. 52 (2), 131–135. doi: 10.1270/jsbbs.52.131
Sergeeva, L. I., Keurentjes, J. J., Bentsink, L., Vonk, J., van der Plas, L. H., Koornneef, M., et al. (2006). Vacuolar invertase regulates elongation of arabidopsis thaliana roots as revealed by QTL and mutant analysis. Proc. Natl. Acad. Sci. 103 (8), 2994–2999. doi: 10.1073/pnas.0511015103
Smith, A. M., Denyer, K., Martin, C. (1997). The synthesis of the starch granule. Annu. Rev. Plant Biol. 48 (1), 67–87. doi: 10.1146/annurev.arplant.48.1.67
Sosnowski, O., Charcosset, A., Joets, J. (2012). BioMercator V3: An upgrade of genetic map compilation and quantitative trait loci meta analysis algorithms. Bioinformatics 28 (15), 2082–2083. doi: 10.1093/bioinformatics/bts313
Sreenivasulu, N., Butardo, V. M., Jr., Misra, G., Cuevas, R. P., Anacleto, R., Kavi Kishor, P. B. (2015). Designing climate-resilient rice with ideal grain quality suited for high-temperature stress. J. Exp. Bot. 66 (7), 1737–1748. doi: 10.1093/jxb/eru544
Sturm, A. (1999). Invertases. primary structures, functions, and roles in plant development and sucrose partitioning. Plant Physiol. 121 (1), 1–8.
Sun, Y., Lin, Z., Reinders, A., Ward, J. M. (2012). Functionally important amino acids in rice sucrose transporter OsSUT1. Biochemistry 51 (15), 3284–3291. doi: 10.1021/bi201934h
Tabata, M., Hirabayashi, H., Takeuchi, Y., Ando, I., Iida, Y., Ohsawa, R. (2007). Mapping of quantitative trait loci for the occurrence of white-back kernels associated with high temperatures during the ripening period of rice (Oryza sativa l.). Breed. Sci. 57 (1), 47–52. doi: 10.1270/jsbbs.57.47
Takehara, K., Murata, K., Yamaguchi, T., Yamaguchi, K., Chaya, G., Kido, S., et al. (2018). Thermo-responsive allele of sucrose synthase 3 (Sus3) provides high-temperature tolerance during the ripening stage in rice (Oryza sativa l.). Breed. Sci. 68(3), 336–342. doi: 10.1270/jsbbs.18007
Tan, Y. F., Xing, Y. Z., Li, J. X., Yu, S. B., Xu, C. G., Zhang, Q. (2000). Genetic bases of appearance quality of rice grains in shanyou 63, an elite rice hybrid. Theor. Appl. Genet. 101 (5), 823–829. doi: 10.1007/s001220051549
Tashiro, T. W. I. F., Wardlaw, I. F. (1991). The effect of high temperature on kernel dimensions and the type and occurrence of kernel damage in rice. Aust. J. Agric. Res. 42 (3), 485–496. doi: 10.1071/AR9910485
Tello-Ruiz, M. K., Jaiswal, P., Ware, D. (2022). Gramene: A resource for comparative analysis of plants genomes and pathways. Methods Mol. Biol. 2443, 101–131. doi: 10.1007/978-1-0716-2067-0_5
Temnykh, S., DeClerck, G., Lukashova, A., Lipovich, L., Cartinhour, S., McCouch, S. (2001). Computational and experimental analysis of microsatellites in rice (Oryza sativa l.): frequency, length variation, transposon associations, and genetic marker potential. Genome Res. 11 (8), 1441–1452.
Terao, T., Hirose, T. (2018). Temperature-dependent QTLs in indica alleles for improving grain quality in rice: increased prominence of QTLs responsible for reduced chalkiness under high-temperature conditions. Mol. Breed. 38 (5), 1–20. doi: 10.1007/s11032-018-0807-0
Tetlow, I. J., Emes, M. J. (2014). A review of starch-branching enzymes and their role in amylopectin biosynthesis. IUBMB Life 66 (8), 546–558. doi: 10.1002/iub.1297
Tetlow, I. J., Morell, M. K., Emes, M. J. (2004). Recent developments in understanding the regulation of starch metabolism in higher plants. J. Exp. Bot. 55 (406), 2131–2145. doi: 10.1093/jxb/erh248
Tian, Z., Qian, Q., Liu, Q., Yan, M., Liu, X., Yan, C., et al. (2009). Allelic diversities in rice starch biosynthesis lead to a diverse array of rice eating and cooking qualities. Proc. Natl. Acad. Sci. 106 (51), 21760–21765. doi: 10.1073/pnas.0912396106
Umemoto, T., Aoki, N. (2005). Single-nucleotide polymorphisms in rice starch synthase IIa that alter starch gelatinisation and starch association of the enzyme. Funct. Plant Biol. 32 (9), 763–768. doi: 10.1071/FP04214
Umemoto, T., Aoki, N., Lin, H., Nakamura, Y., Inouchi, N., Sato, Y., et al. (2004). Natural variation in rice starch synthase IIa affects enzyme and starch properties. Funct. Plant Biol. 31 (7), 671–684. doi: 10.1071/FP04009
Umemoto, T., Nakamura, Y., Ishikura, N. (1995). Activity of starch synthase and the amylose content in rice endosperm. Phytochemistry 40 (6), 1613–1616. doi: 10.1016/0031-9422(95)00380-P
Umemoto, T., Terashima, K., Nakamura, Y., Satoh, H. (1999). Differences in amylopectin structure between two rice varieties in relation to the effects of temperature during grain-filling. Starch-Stärke 51 (2-3), 58–62. doi: 10.1002/(SICI)1521-379X(199903)51:2<58::AID-STAR58>3.0.CO;2-J
Umemoto, T., Yano, M., Satoh, H., Shomura, A., Nakamura, Y. (2002). Mapping of a gene responsible for the difference in amylopectin structure between japonica-type and indica-type rice varieties. Theor. Appl. Genet. 104 (1), 1–8. doi: 10.1007/s001220200000
Wada, T., Miyahara, K., Sonoda, J. Y., Tsukaguchi, T., Miyazaki, M., Tsubone, M., et al. (2015). Detection of QTLs for white-back and basal-white grains caused by high temperature during ripening period in japonica rice. Breed. Sci. 65 (3), 216–225. doi: 10.1270/jsbbs.65.216
Wan, X. Y., Wan, J. M., Weng, J. F., Jiang, L., Bi, J. C., Wang, C. M., et al. (2005). Stability of QTLs for rice grain dimension and endosperm chalkiness characteristics across eight environments. Theor. Appl. Genet. 110 (7), 1334–1346. doi: 10.1007/s00122-005-1976-x
Wanchana, S., Toojinda, T., Tragoonrung, S., Vanavichit, A. (2003). Duplicated coding sequence in the waxy allele of tropical glutinous rice (Oryza sativa l.). Plant Sci. 165 (6), 1193–1199. doi: 10.1016/S0168-9452(03)00326-1
Wang, C., Caragea, D., Kodadinne Narayana, N., Hein, N. T., Bheemanahalli, R., Somayanda, I. M., et al. (2022). Deep learning based high-throughput phenotyping of chalkiness in rice exposed to high night temperature. Plant Methods 18 (1), 1–23. doi: 10.1186/s13007-022-00839-5
Wang, L., Chen, L., Wang, P., Wang, Z., Zheng, H., Ma, H., et al. (2016). Detecting the QTL of rice chalkiness traits using advanced backcrossing population. J. Nanjing Agric. Univ. 39 (2), 183–190.
Wang, X., Wang, K., Yin, T., Zhao, Y., Liu, W., Shen, Y., et al. (2021). Nitrogen fertilizer regulated grain storage protein synthesis and reduced chalkiness of rice under actual field warming. Front. Plant Sci. 12. doi: 10.3389/fpls.2021.715436
Wang, E., Wang, J., Zhu, X., Hao, W., Wang, L., Li, Q., et al. (2008). Control of rice grain-filling and yield by a gene with a potential signature of domestication. Nat. Genet. 40 (11), 1370–1374. doi: 10.1038/ng.220
Wang, Z. Y., Wu, Z. L., Xing, Y. Y., Zheng, F. G., Guo, X. L., Zhang, W. G., et al. (1990). Nucleotide sequence of rice waxy gene. Nucleic Acids Res. 18 (19), 5898. doi: 10.1093/nar/18.19.5898
Wang, L., Yuan, J., Ma, Y., Jiao, W., Ye, W., Yang, D. L., et al. (2018). Rice interploidy crosses disrupt epigenetic regulation, gene expression, and seed development. Mol. Plant 11 (2), 300–314. doi: 10.1016/j.molp.2017.12.006
Waters, D. L., Henry, R. J., Reinke, R. F., Fitzgerald, M. A. (2006). Gelatinization temperature of rice explained by polymorphisms in starch synthase. Plant Biotechnol. J. 4 (1), 115–122. doi: 10.1111/j.1467-7652.2005.00162.x
Wei, X., Jiao, G., Lin, H., Sheng, Z., Shao, G., Xie, L., et al. (2017). GRAIN INCOMPLETE FILLING 2 regulates grain filling and starch synthesis during rice caryopsis development. J. Integr. Plant Biol. 59 (2), 134–153. doi: 10.1111/jipb.12510
Whitt, S. R., Wilson, L. M., Tenaillon, M. I., Gaut, B. S., Buckler, E. S., IV (2002). Genetic diversity and selection in the maize starch pathway. Proc. Natl. Acad. Sci. 99 (20), 12959–12962. doi: 10.1073/pnas.202476999
Woo, M. O., Ham, T. H., Ji, H. S., Choi, M. S., Jiang, W., Chu, S. H., et al. (2008). Inactivation of the UGPase1 gene causes genic male sterility and endosperm chalkiness in rice (Oryza sativa l.). Plant J. 54 (2), 190–204. doi: 10.1111/j.1365-313X.2008.03405.x
Wu, Y. C., Chang, S. J., Lur, H. S. (2016). Effects of field high temperature on grain yield and quality of a subtropical type japonica rice–Pon-Lai rice. Plant Production Sci. 19 (1), 145–153. doi: 10.1080/1343943X.2015.1128091
Wu, C. Y., Suzuki, A., Washida, H., Takaiwa, F. (1998). The GCN4 motif in a rice glutelin gene is essential for endosperm-specific gene expression and is activated by opaque-2 in transgenic rice plants. Plant J. 14 (6), 673–683. doi: 10.1046/j.1365-313x.1998.00167.x
Xi, M., Wu, W., Xu, Y., Zhou, Y., Chen, G., Ji, Y., et al. (2021). Grain chalkiness traits is affected by the synthesis and dynamic accumulation of the storage protein in rice. J. Sci. Food Agric. 101 (14), 6125–6133. doi: 10.1002/jsfa.11269
Xin, W., Zhang, L., Zhang, W., Gao, J., Yi, J., Zhen, X., et al. (2019). An integrated analysis of the rice transcriptome and metabolome reveals differential regulation of carbon and nitrogen metabolism in response to nitrogen availability. Int. J. Mol. Sci. 20 (9), 2349. doi: 10.3390/ijms20092349
Xu, X., Ren, Y., Wang, C., Zhang, H., Wang, F., Chen, J., et al. (2019). OsVIN2 encodes a vacuolar acid invertase that affects grain size by altering sugar metabolism in rice. Plant Cell Rep. 38 (10), 1273–1290. doi: 10.1007/s00299-019-02443-9
Yamakawa, H., Hakata, M. (2010). Atlas of rice grain filling-related metabolism under high temperature: joint analysis of metabolome and transcriptome demonstrated inhibition of starch accumulation and induction of amino acid accumulation. Plant Cell Physiol. 51 (5), 795–809. doi: 10.1093/pcp/pcq034
Yamakawa, H., Hirose, T., Kuroda, M., Yamaguchi, T. (2007). Comprehensive expression profiling of rice grain filling-related genes under high temperature using DNA microarray. Plant Physiol. 144 (1), 258–277. doi: 10.1104/pp.107.098665
Yang, L., Christensen, D. A., McKinnon, J. J., Beattie, A. D., Yu, P. (2013). Effect of altered carbohydrate traits in hulless barley (Hordeum vulgare l.) on nutrient profiles and availability and nitrogen to energy synchronization. J. Cereal Sci. 58 (1), 182–190.
Yang, L. M., Liu, H. L., Zhao, H. W., Wang, J. G., Sun, J., Zheng, H. L., et al. (2019). Mapping quantitative trait loci and meta-analysis for cold tolerance in rice at booting stage. Euphytica 215 (5), 1–15. doi: 10.1007/s10681-019-2410-9
Yu, Y., Ouyang, Y., Yao, W. (2018). shinyCircos: an R/Shiny application for interactive creation of circos plot. Bioinformatics 34 (7), 1229–1231. doi: 10.1093/bioinformatics/btx763
Yuan, P. R., Kim, H. J., Chen, Q. H., Ju, H. G., Ji, S. D., Ahn, S. N. (2010). Mapping QTLs for grain quality using an introgression line population from a cross between oryza sativa and o. rufipogon. J. Crop Sci. Biotechnol. 13 (4), 205–212. doi: 10.1007/s12892-010-0094-8
Yun, Y. T., Chung, C. T., Lee, Y. J., Na, H. J., Lee, J. C., Lee, S. G., et al. (2016). QTL mapping of grain quality traits using introgression lines carrying oryza rufipogon chromosome segments in japonica rice. Rice 9 (1), 1–14. doi: 10.1186/s12284-016-0135-0
Zakaria, S., Matsuda, T., Tajima, S., Nitta, Y. (2002). Effect of high temperature at ripening stage on the reserve accumulation in seed in some rice cultivars. Plant production Sci. 5 (2), 160–168. doi: 10.1626/pps.5.160
Zhang, Changquan, Zhu, J., Chen, S., Fan, X., Li, Q., et al. (2019). "Wxlv, the ancestral allele of rice waxy gene.". Mol. Plant 12, 1157–1166. doi: 10.1016/j.molp.2019.05.011
Zhao, X., Fitzgerald, M. (2013). Climate change: implications for the yield of edible rice. PloS One 8 (6), e66218. doi: 10.1371/journal.pone.0066218
Zheng, L., Zhang, W., Liu, S., Chen, L., Liu, X., Chen, X., et al. (2012). Genetic relationship between grain chalkiness, protein content, and paste viscosity properties in a backcross inbred population of rice. J. Cereal Sci. 56 (2), 153–160. doi: 10.1016/j.jcs.2012.05.003
Zhou, L., Chen, L., Jiang, L., Zhang, W., Liu, L., Liu, X., et al. (2009). Fine mapping of the grain chalkiness QTL qPGWC-7 in rice (Oryza sativa l.). Theor. Appl. Genet. 118 (3), 581–590. doi: 10.1007/s00122-008-0922-0
Zhou, W., Wang, X., Zhou, D., Ouyang, Y., Yao, J. (2017). Overexpression of the 16-kD a α-amylase/trypsin inhibitor RAG 2 improves grain yield and quality of rice. Plant Biotechnol. J. 15 (5), 568–580. doi: 10.1111/pbi.12654
Keywords: starch metabolism, grain chalkiness, meta-QTL analysis, haplotype, haplo-pheno analysis, granule bound starch synthase I, starch synthase IIa
Citation: Kumari A, Sharma D, Sharma P, Sahil, Wang C, Verma V, Patil A, Imran M, Singh MP, Kumar K, Paritosh K, Caragea D, Kapoor S, Chandel G, Grover A, Jagadish SVK, Katiyar-Agarwal S and Agarwal M (2023) Meta-QTL and haplo-pheno analysis reveal superior haplotype combinations associated with low grain chalkiness under high temperature in rice. Front. Plant Sci. 14:1133115. doi: 10.3389/fpls.2023.1133115
Received: 28 December 2022; Accepted: 17 February 2023;
Published: 08 March 2023.
Edited by:
Shouvik Das, Regional Centre for Biotechnology (RCB), IndiaReviewed by:
Jitendra Kumar Thakur, International Centre for Genetic Engineering and Biotechnology, IndiaOmar Borsani, Universidad de la República, Uruguay
Venkata Ravi Prakash Reddy, Acharya N. G. Ranga Agricultural University, India
Copyright © 2023 Kumari, Sharma, Sharma, Sahil, Wang, Verma, Patil, Imran, Singh, Kumar, Paritosh, Caragea, Kapoor, Chandel, Grover, Jagadish, Katiyar-Agarwal and Agarwal. This is an open-access article distributed under the terms of the Creative Commons Attribution License (CC BY). The use, distribution or reproduction in other forums is permitted, provided the original author(s) and the copyright owner(s) are credited and that the original publication in this journal is cited, in accordance with accepted academic practice. No use, distribution or reproduction is permitted which does not comply with these terms.
*Correspondence: Manu Agarwal, YWdhcndhbG03MUBnbWFpbC5jb20=