- 1Department of Plant Pathology, North Dakota State University, Fargo, ND, United States
- 2Department of Agronomy, Horticulture and Plant Science, South Dakota State University, Brookings, SD, United States
- 3Cereal Disease Laboratory, United States Department of Agriculture-Agricultural Research Service (USDA-ARS), St. Paul, MN, United States
- 4Cereal Crops Research Unit, Edward T. Schafer Agricultural Research Center, United States Department of Agriculture-Agricultural Research Service (USDA-ARS), Fargo, ND, United States
Leaf rust, caused by Puccinia triticina (Pt) is among the most devastating diseases posing a significant threat to global wheat production. The continuously evolving virulent Pt races in North America calls for exploring new sources of leaf rust resistance. A diversity panel of 365 bread wheat accessions selected from a worldwide population of landraces and cultivars was evaluated at the seedling stage against four Pt races (TDBJQ, TBBGS, MNPSD and, TNBJS). A wide distribution of seedling responses against the four Pt races was observed. Majority of the genotypes displayed a susceptible response with only 28 (9.8%), 59 (13.5%), 45 (12.5%), and 29 (8.1%) wheat accessions exhibiting a highly resistant response to TDBJQ, TBBGS, MNPSD and, TNBJS, respectively. Further, we conducted a high-resolution multi-locus genome-wide association study (GWAS) using a set of 302,524 high-quality single nucleotide polymorphisms (SNPs). The GWAS analysis identified 27 marker-trait associations (MTAs) for leaf rust resistance on different wheat chromosomes of which 20 MTAs were found in the vicinity of known Lr genes, MTAs, or quantitative traits loci (QTLs) identified in previous studies. The remaining seven significant MTAs identified represent genomic regions that harbor potentially novel genes for leaf rust resistance. Furthermore, the candidate gene analysis for the significant MTAs identified various genes of interest that may be involved in disease resistance. The identified resistant lines and SNPs linked to the QTLs in this study will serve as valuable resources in wheat rust resistance breeding programs.
Introduction
Global wheat production is continuously constrained by the emergence of new and more virulent races of pathogens causing several economically important diseases. Among these, fungal pathogens are known to cause several important foliar diseases in wheat including three cereal rusts: leaf rust caused by Puccinia triticina (Pt), stem rust caused by Puccinia graminis f. sp. tritici (Pgt), and stripe rust caused by Puccinia striiformis f. sp. tritici (Pst). Wheat rusts threaten wheat production in the United States (US) by accounting for yield losses in the value of millions of dollars annually. Of the three wheat rusts, leaf rust (LR) is regarded as the most common, extensively distributed, and devastating disease causing 3.25% yield losses annually to global wheat production (Kolmer, 2005; Savary et al., 2019). The recurrent and across-the-board occurrence of leaf rust can lead to epidemic conditions with yield losses ranging from 15% to more than 50% when infections occur during early plant growth stages on susceptible cultivars (Singh et al., 2002; Huerta-Espino et al., 2011). Serious yield losses are incurred in terms of reduced kernels per head and decreased kernel weight (Bolton et al., 2008). In the US alone, ~40-60 races of Pt are reported annually (Kolmer et al., 2007) and yield losses valued at $350 million were reported between 2000 and 2004 (Huerta-Espino et al., 2011).
Host resistance is the most efficient and cost-effective strategy to manage leaf rust and wheat breeding programs throughout the world are deploying rust resistance genes in commercial cultivars to fight against this disease (Gill et al., 2019). Around 80 leaf rust resistance (Lr) genes have been identified and cataloged in wheat till date (Prasad et al., 2020). Of the identified genes, eleven Lr genes have been cloned, viz. Lr1 (Cloutier et al., 2007), Lr9 (Wang et al., 2022), Lr10 (Feuillet et al., 2003), Lr13 (Hewitt et al., 2021; Yan et al., 2021), Lr14a (Kolodziej et al., 2021), Lr21 (Huang et al., 2003), Lr22a (Thind et al., 2017), Lr34 (Krattinger et al., 2009), Lr42 (Lin et al., 2022), Lr58 (Wang et al., 2022), and Lr67 (Moore et al., 2015). Genetic resistance can be classified into two categories, namely seedling/all-stage resistance (ASR) and adult plant resistance (APR). The seedling resistance is largely qualitative resistance usually controlled by a single major gene, effective at all the developmental stages of the plant life cycle. ASR is associated with a hypersensitive response, a programmed cell death that restricts the pathogen growth and spread. The majority of the studied and characterized leaf rust resistance genes are seedling resistance genes, with Lr76 (Bansal et al., 2017), Lr79 (Qureshi et al., 2018) and Lr80 (Kumar et al., 2021b) being the recent additions to this group. On the other hand, non-race specific, adult-plant resistance (APR) is often partial resistance at the adult plant stage controlled by multiple minor-effect genes with an additive effect and only a sizable proportion of the identified Lr genes belong to this category. Among the characterized Lr genes, Lr34 (Dyck, 1987), Lr46 (Singh et al., 1998), and Lr67 (Hiebert et al., 2010) are broad-spectrum APR genes, providing partial resistance against all three wheat rusts and powdery mildew (Blumeria graminis f. sp. tritici). In addition to these genes, Lr68 (Herrera-Foessel et al., 2012), Lr74 (Chhetri et al., 2016), Lr77 (Kolmer et al., 2018b), and Lr78 (Kolmer et al., 2018a) have also been characterized in hexaploid wheat as APR genes against leaf rust. The race-specific Lr genes provide resistance against specific races but the continuously evolving virulent pathogen races render these genes ineffective. Thus, it is important to find novel sources of leaf rust resistance that offer resistance to many different leaf rust races in order to improve the overall durability of resistance in released wheat cultivars.
The recent advancements in sequencing approaches and harmonized community efforts have made genome-wide association studies (GWAS) as an important method for studying the marker-trait associations (Zhu et al., 2008; Tibbs Cortes et al., 2021). In contrast to bi-parental linkage mapping, GWAS extensively utilizes the ancient recombination events that occurred in natural populations for the identification of genomic regions associated with traits of interest. In wheat, GWAS has been used successfully for dissecting the genetic basis of agronomic traits (Sukumaran et al., 2015; Gao et al., 2015; Ward et al., 2019; Sidhu et al., 2020), disease resistance (Gyawali et al., 2018; Phan et al., 2018; Zhu et al., 2020; AlTameemi et al., 2021), quality traits (Kristensen et al., 2018; Chen et al., 2019; Yang et al., 2020) and insect resistance (Joukhadar et al., 2013; Mondal et al., 2016).
In the Great Plains region of the US, leaf rust is the most prevalent rust disease. The hard red spring wheat cultivars grown in the northern plains have resistance genes that include Lr2a, Lr10, Lr16, Lr21, Lr23, and Lr34 (Kolmer, 2019). However, the effectiveness of some of these genes has been reduced due to the continuous emergence of new virulent pathogen races. For example, Lr21 had been deployed for leaf rust resistance since the mid-2000s and is found in some hard red spring cultivars like Glenn, Faller, and RB07. However, virulent races against this gene have been identified in North Dakota and Minnesota (https://www.ars.usda.gov/midwest-area/stpaul/cereal-disease-lab/docs/lr21-virulence-detected/ ). Thus, exploring and identifying new resistance sources is highly needed. In the current study, a highly diverse panel of bread wheat accessions from different regions of the world was evaluated for ASR against leaf rust races prevalent in the Northern Great Plains of the US. A high-resolution multi-locus GWAS was also performed to identify genomic regions associated with LR resistance to facilitate the development of resistant wheat cultivars for the future.
Materials and methods
Plant material and P. triticina races
We used a diverse panel of 365 hexaploid wheat accessions, including landraces and cultivars from different regions of the world (Supplementary Table S1). The 365 accessions were selected from a larger collection of 890 diverse accessions of hexaploid and tetraploid wheat that was previously resequenced using the sequence capture assay (He et al., 2019). The accessions were obtained from the USDA National Small Grains Collection gene bank and grown for one round of purification and seed increase. The metadata for the 365 accessions can be found in the online repository (http://wheatgenomics.plantpath.ksu.edu/1000EC ). Four prevalent Pt races (TDBJQ, TBBGS, MNPSD, TNBJS) in the Northern Great Plains of the US were selected for screening the wheat lines at the seedling stage. TBBGS (virulent on genes Lr1, Lr2a, Lr2c, Lr3, Lr10, Lr21, Lr28, and Lr39) was the most predominant race in Minnesota, North Dakota, and South Dakota in 2020. Furthermore, MNPSD (virulent on genes Lr1, Lr3, Lr9, Lr24, Lr3ka, Lr17, Lr30, LrB, Lr10, Lr14a, and Lr39), TNBJS (virulent on genes Lr1, Lr2a, Lr2c, Lr3, Lr9, Lr24, Lr10, Lr14a, Lr21, Lr28, and Lr39) and TDBJQ (virulent on genes Lr1, Lr2a, Lr2c, Lr3, Lr24, Lr10, Lr14a, Lr21, and Lr28) are the other important races in the region.
Phenotyping assays for leaf rust seedling screening
The 365 wheat accessions were evaluated in two independent experiments for seedling response to LR. For the leaf rust screening, wheat seedlings at the two-leaf stage were evaluated for their reactions to described races in the biosafety level 2 (BSL 2) facility at Dalrymple Research Greenhouse Complex, North Dakota Agricultural Experiment Station (AES), Fargo. Briefly, five seedlings per each accession along with susceptible checks were used for phenotypic screening for each LR race. Plants were grown in a 50-cell tray containing PRO-MIX LP-15 (www.pthorticulture.com ) sterilized soil mix and maintained in a rust-free greenhouse growth room set to 22°C/18°C (day/night) with 16 h/8 h day/night photoperiod. At two-leaf stage, the seedlings were inoculated with fresh urediniospores suspended in SOLTROL-170 mineral oil (Philips Petroleum) at a final concentration of 105 spores mL-1 using an inoculator pressurized by an air pump. The inoculated seedlings were placed in a dark dew chamber at 20°C overnight and then transferred back to the growth room. The infection types (IT) were scored about 12 to 14 days after inoculation, using 0-4 scale, where ‘0’ = no visible uredinia, ‘;’ = hypersensitive flecks, ‘1’ = small uredinia with necrosis, ‘2’ = small to medium-sized uredinia with green islands and surrounded by necrosis or chlorosis, ‘3’ = medium-sized uredinia with or without chlorosis, ‘4’ = large uredinia without chlorosis (Stakman et al., 1962). For each IT, ‘+’ or ‘-’ was used to represent variations from the predominant type. A ‘/’ was used for separating the heterogeneous IT scores between leaves with the most prevalent IT listed first. For plants with different ITs within leaves, a range of IT was recorded with the most predominant IT was listed first. The IT scores were converted to a 0-9 linearized scale referred as infection response (IR) (Zhang et al., 2014). Genotypes with linearized IR scores of 0-4 were considered as highly resistant, 5-6 as moderately resistant, and 7-9 as susceptible.
Phenotypic data analysis
Phenotypic data were analyzed as described previously in (Gill et al., 2021). In brief, a mixed model analysis was used to obtain the best linear unbiased estimates (BLUEs) for phenotypic responses from each of the four isolates using following equation:
where yij is the trait of interest, μ is the overall mean, Ri is the effect of the ith independent replicate/experiment, Gj is the effect of the jth genotype, and eij is the residual error effect associated with the ith replication, and jth genotype. The broad-sense heritability (H2) for IR was estimated for independent nurseries as follows:
where σg2 and σe2 are the genotype and error variance components, respectively. The linear mixed model analysis was performed in META-R (Alvarado et al., 2020) based on the ‘LME4’ R-package (Bates et al., 2015) for the heritability estimation. The Pearson’s correlations among the phenotype responses from four races were estimated based on the BLUEs for each trait using ‘psych’ package in R environment (R Core Team, 2018). The visualization of descriptive statistics was performed using R package ‘ggplot2’ (Wickham, 2016).
Genotyping, population structure, and linkage disequilibrium
The 365 accessions used in the current study were previously sequenced using the exome sequence capture assay, resulting in the identification of around 7.3 million single nucleotide polymorphisms (SNPs) (He et al., 2019). A VCF file containing a filtered set of about 3 million SNPs (http://wheatgenomics.plantpath.ksu.edu/1000EC ) was used to extract the genotyping information. The extracted genotyping data was subjected to quality control by removing the sites with > 75% missing data, > 5% heterozygotes, and< 5% minimum allele frequency (MAF), leaving 302,524 high-quality SNPs for downstream analysis. The missing genotypes from selected SNPs were imputed by Beagle v4.1 (beagle.27Jan18.7e1.jar; https://faculty.washington.edu/browning/beagle/b4_1.html ) (Browning and Browning, 2007) using parameters defined earlier (He et al., 2019) and the imputed set of 302,524 SNPs was used to perform GWAS.
Further, population structure and linkage disequilibrium (LD) analyses were performed using a pruned set of 14,185 SNPs. The LD-based pruning (r2 > 0.2) was performed in PLINK v1.90 using ‘indep-pairwise’ function (Purcell et al., 2007). The population stratification was assessed using parallel iterations of a Bayesian model-based clustering algorithms STRUCTURE v2.3.4 (Pritchard et al., 2000; Chhatre and Emerson, 2017) assuming ten fixed populations (K =1-10) with ten independent runs for each K using a burn-in period of 10,000 iterations followed by 10,000 Monte-Carlo iterations. The optimal value of K was identified using STRUCTURE HARVESTER v0.6.9, which is based on an ad-hoc statistic-based approach (Evanno et al., 2005; Earl and vonHoldt, 2012). Further, the principal component analysis (PCA) was performed using 14,185 SNPs with R package ‘SNPRelate’ (Zheng et al., 2012). The first two principal components were plotted as a scatterplot to observe any stratification based on various factors using R package ‘ggplot2’ (Wickham, 2016). The linkage disequilibrium (LD) between SNPs was assessed as the squared correlation coefficient (r2) of alleles. The LD decay distance was estimated and visualized for the whole genome and individual sub-genomes using ‘PopLDdecay’ program (Zhang et al., 2019a).
Marker-trait associations
Genome-wide association analyses were performed using a panel of 365 accessions with 3,02,524 high-quality SNPs to identify marker-trait associations for reaction to four Pt isolates. Initially, we used two different GWAS algorithms, including the mixed linear model (MLM) (Yu et al., 2006) and a Fixed and random model Circulating Probability Unification (FarmCPU) (Liu et al., 2016). The quantile-quantile (QQ) plots were used to compare the two algorithms that revealed that FarmCPU showed better control of false positives and false negatives. Hence, FarmCPU was used to report GWAS results for all four isolates. In brief, FarmCPU is an improved multiple-locus model that controls false positives by fitting the associated markers detected from the iterations as cofactors to perform marker tests within a fixed-effect model. The FarmCPU was implemented through Genomic Association and Prediction Integrated Tool (GAPIT) version 3.0 in the R environment (Wang and Zhang, 2021), and the first two principal components were included to account for the population structure based upon visual examination of the scree plot and DeltaK statistic from STRUCTURE analysis. The Bonferroni correction-based threshold to declare an association as significant generally proves too stringent as it accounts for all the SNPs in the dataset rather than independent tests. Thus, most studies rely on an exploratory threshold or a corrected Bonferroni threshold based on independent tests (Halder et al., 2019; Pang et al., 2020; Kumar et al., 2021a). In our case, we used an exploratory threshold of -log10(P) = 5.00 which is strict compared to the commonly used threshold of -log10(P) = 3.00 and suitable for a multi-locus model, which generally does not require multiple corrections (Zhang et al, 2019b). Furthermore, we evaluated the effect of the accumulation of resistant alleles for significant marker-trait associations (MTAs) on the phenotypic performance of the genotypes. The panel of 365 accessions was grouped based on the number of resistant alleles for significant MTAs carried by each accession. These groups were compared using a pairwise t-test to assess the additive effect of the resistant alleles on the disease reaction of respective isolates.
Candidate gene analysis
The candidate gene analysis was performed for selected stable MTAs to identify the putative candidate genes. As the SNPs were physically mapped to Chinese Spring RefSeq v1.0, we used IWGSC Functional Annotation v1.0 to retrieve high-confidence genes within +/- 1 Mb of the significant MTAs. The wheat gene expression browser (http://www.wheat-expression.com/ ) (Borrill et al., 2016) and a thorough review of the literature was used to exclude unlikely genes from the candidate regions.
Results
Phenotypic response of hexaploid wheat accessions to leaf rust
To identify new sources of leaf rust resistance, a panel of 365 hexaploid wheat accessions was phenotypically characterized at the seedling stage with the four Pt races. The panel displayed large variations for the disease score ranging from immune response (IT = 0, IR = 0) to highly susceptible response (IT = 4, IR = 9) (Figure 1; Tables 1 and 2; Supplementary Tables S2, S3). The mean linearized infection response scores of the wheat genotypes were 6.4, 6.3, 5.4, and 6.3 for Pt races TDBJQ, TBBGS, MNPSD, and, TNBJS, respectively (Table 1; Supplementary Table S4). The distributions of infection responses for all races except MNPSD were skewed towards susceptible scores (IR >7). About 45-50% of the lines displayed susceptible reactions against TDBJQ, TBBGS and, TNBJS, whereas only 12.7% of lines were susceptible to MNPSD with majority of the lines exhibiting a moderately resistance response (Figure 1, Table 2). A total of 28 (9.8%), 59 (13.5%), 45 (12.5%) and 29 (8.1%) wheat lines were highly resistant to TDBJQ, TBBGS, MNPSD and, TNBJS, respectively (Table 2). Majority of the resistant accessions against these Pt races were from the Americas followed by Europe, Asia and Africa (Supplementary Table S5). Individually, TDBJQ, TBBGS, MNPSD, and TNBJS had 30.5%, 29%, 28.6%, and 36.7% accessions from the Americas (Supplementary Table S5). Further, there were 71 (19.5%) lines that displayed a resistance response (IR<=6) against all four races (Supplementary Table S6). Out of these 71 resistant accessions, 18.3% were of North American origin (Supplementary Table S6). The proportion of lines showing resistance ranged from 21.4% to 27.9% for the combination of three races and 29.9% to 46.6% for the combination of two races (Table 3). Positive but weak correlations were observed among the seedling plant infection responses to the four Pt races and Pearson’s r value ranged from 0.16 to 0.39 (Supplementary Figure S1). The broad-sense heritability of infection response for the Pt races was high (0.8), showing a large portion of phenotypic variation being explained by the genotypic component (Table 1).
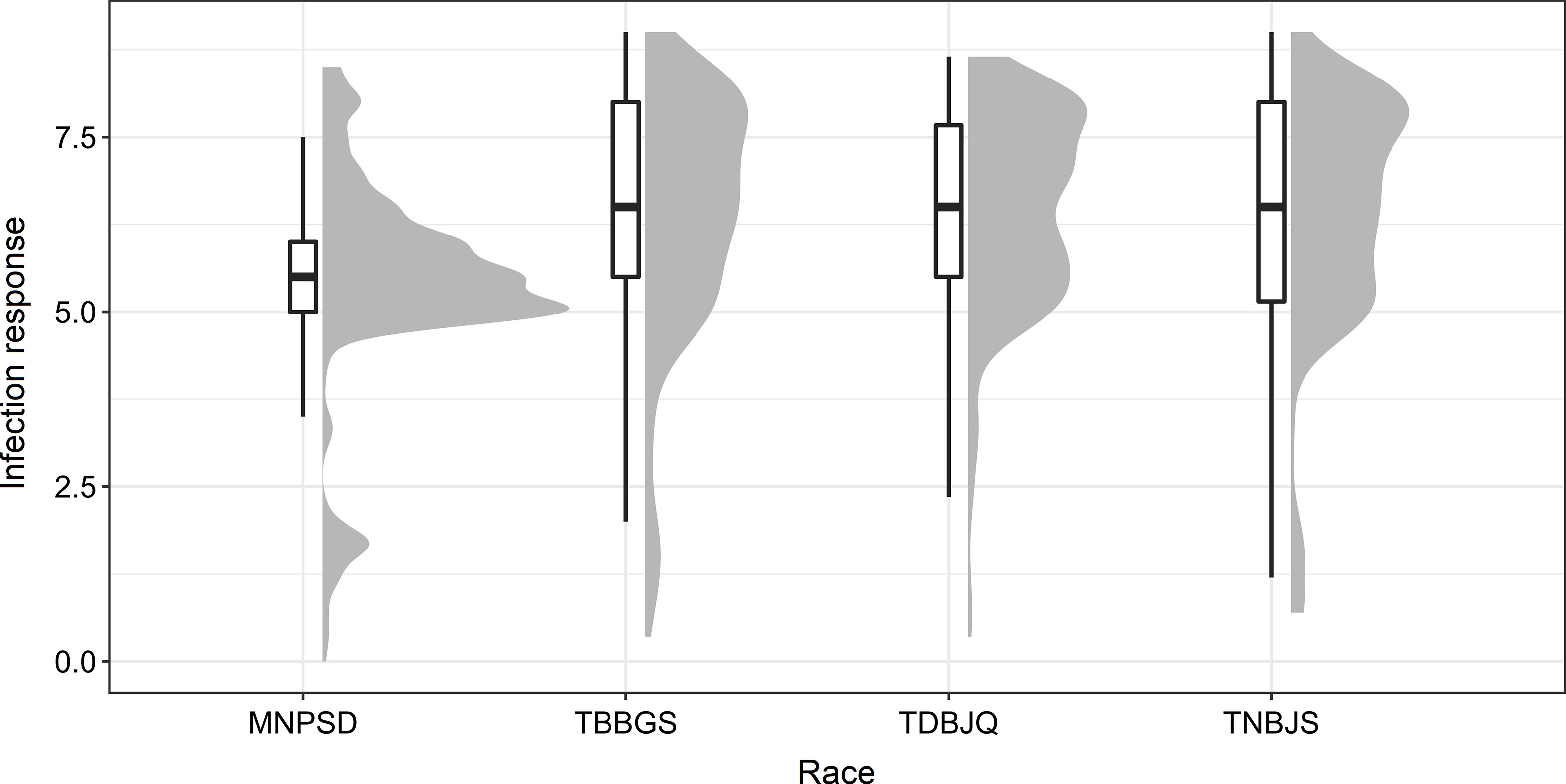
Figure 1 Distribution of infection response (IR) against various races of Pt observed during the seedling evaluation of 365 accessions using boxplots and histograms. The X-axis represents the four different Pt races and the Y-axis represents the IR in 0-9 scale.
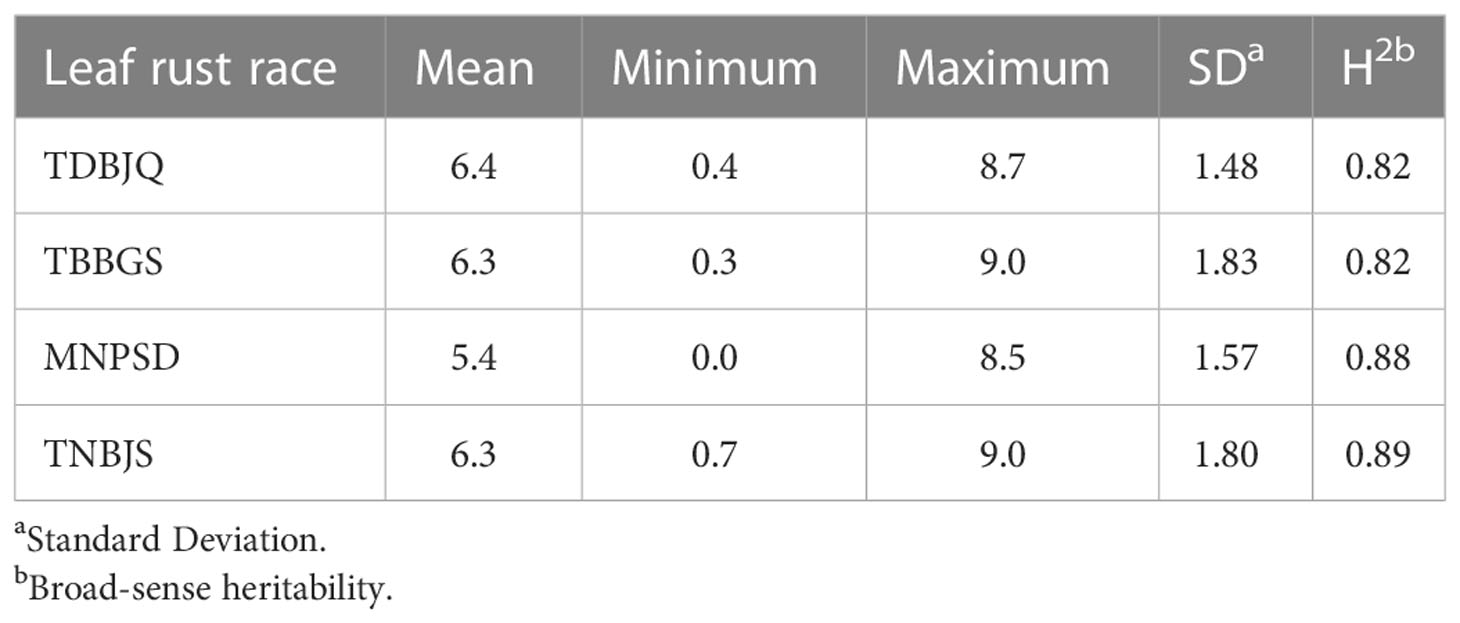
Table 1 Descriptive statistical analysis of the infection response (IR) calculated from the infection types of the 365 wheat genotypes to P. triticina races TDBJQ, TBBGS, MNPSD, and TNBJS.
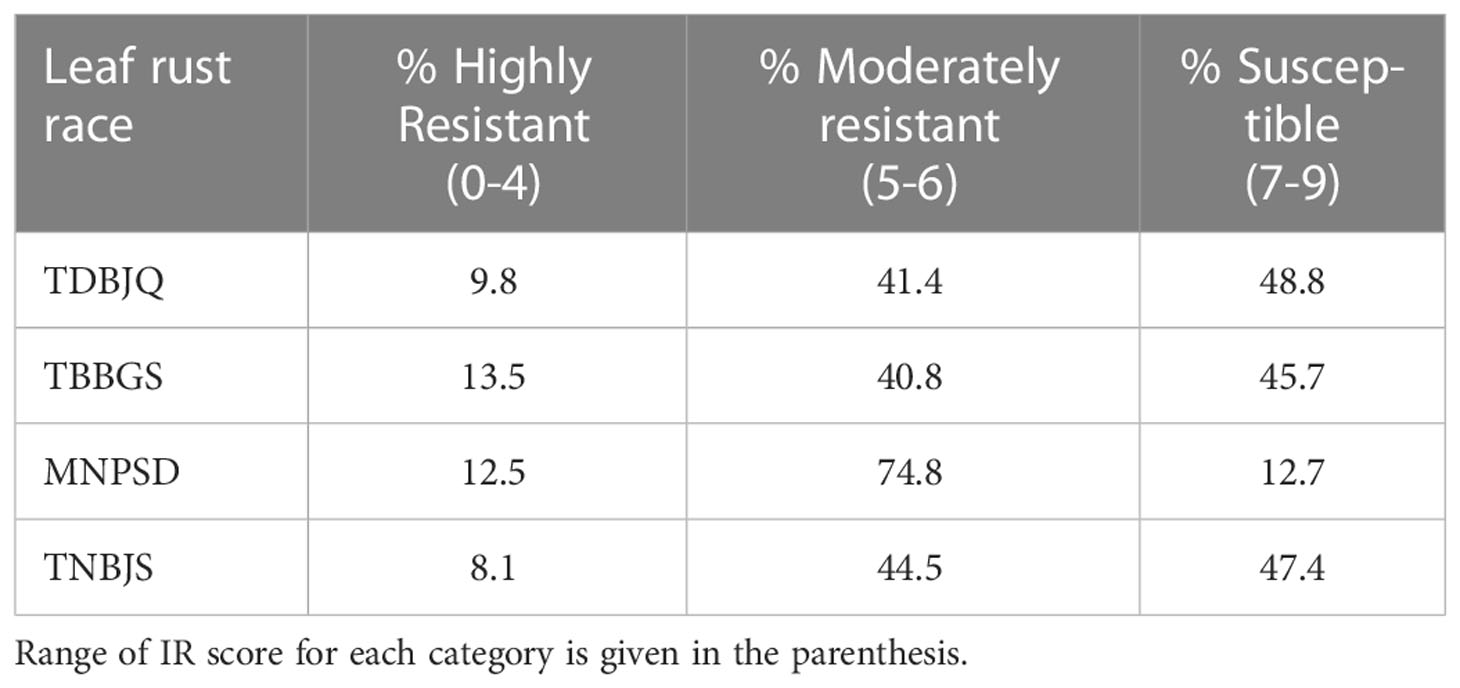
Table 2 Percent distribution of the diversity panel accessions based on their linearized seedling infection responses (IRs) against P. triticina races TDBJQ, TBBGS, MNPSD, and TNBJS.
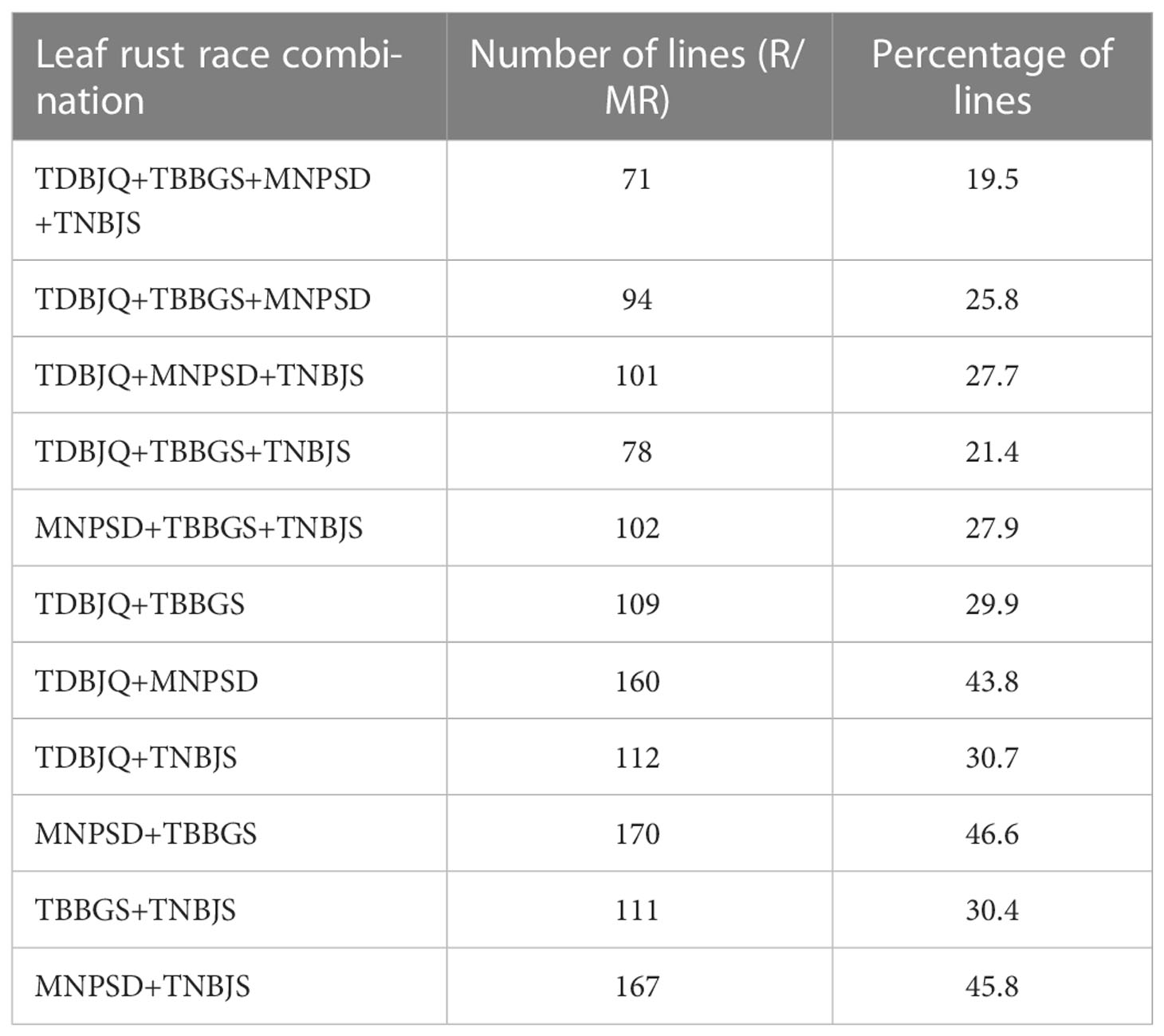
Table 3 Number and percentage of lines resistant to different combinations of the four P. triticina races.
Population structure and linkage disequilibrium analysis
The STRUCTURE analysis used to infer the population structure revealed two major subpopulations (P1 and P2 hereafter) in the panel of 365 accessions based on the peak of DeltaK statistic (Figure 2A). The subpopulation P1 comprised 82 accessions while P2 was comparatively larger comprising the remaining 283 accessions. Further, we assessed a relationship between the two subpopulations and various characteristics including geographic origin, type, and growth habit of the studied accessions. The subpopulation P1 mostly represented the spring wheat accessions as 78 of the 82 accessions had spring growth habit. In contrast, the subpopulation P2 comprised accessions with both spring (216), winter (53), and facultative (14) growth habits. Additionally, P1 mainly represented landraces (51) with comparatively few cultivars (15); whereas, P2 includes cultivated accessions with 144 cultivars and 69 landraces. Based on geographical origin, P1 includes accessions from Asia (45) and Africa (23) with a few accessions from Europe (3) and the Americas (7). In contrast, P2 includes majority of accessions from Europe (89) and the Americas (95) and a good number of accessions from Asia (40) and Africa (37).
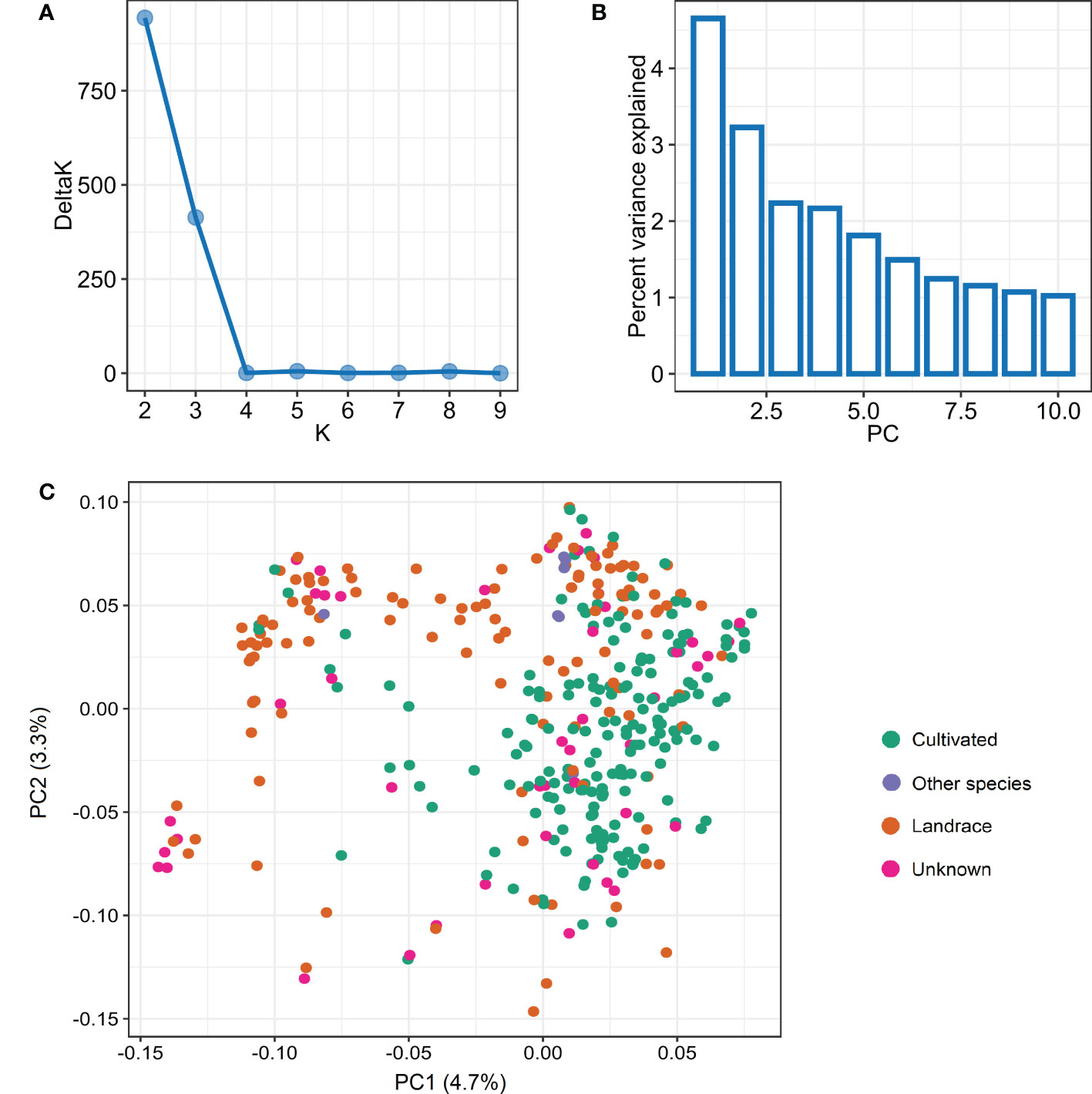
Figure 2 Population structure analysis in panel of 365 wheat accessions based on the 3,02,524 SNPs. (A) Evanno plot of Delta-K statistic from the STRUCTURE analysis. (B) Scree plot for first 10 components obtained from principal component analysis (PCA). (C) Scatterplot based on the first two components (PC1 and PC2) from PCA.
The principal component analysis also revealed two subpopulations within the studied germplasm, with the first two principal components explaining a genetic variation of 4.7% and 3.3%, respectively (Figures 2B, C). The first two principal components were plotted to visually differentiate the accessions by origin and growth habit. The PCA results showed a differentiation among the accessions belonging to landrace or cultivar categories (Figure 2C). However, we did not observe a clear differentiation based on growth habit and geographic origin (Supplementary Figure S2). The linkage disequilibrium (LD) decay was estimated based on LD value (r2) for the whole genome as well as individual sub-genomes. For the whole genome, the LD decay was found to be about 1.5 Mb (Supplementary Figure S3). The LD decay for the three sub-genomes A, B, and D revealed different patterns, with A and B showing smaller LD decay distances as compared to the D sub-genome (Supplementary Figure S3).
Genome-wide association analyses for leaf rust resistance
The phenotypic data of Pt screening was subjected to GWAS to identify genomic regions and SNP markers associated with leaf rust resistance. The GWAS was performed using BLUEs calculated from disease scores data for individual Pt races. We identified a total of 27 significant MTAs on twelve chromosomes: 1A, 1B, 2A, 2B, 2D, 3B, 4A, 4B, 4D, 5A, 5B, and 6B for response against the four Pt races. Individually, we detected nine, nine, one, and eight significant MTAs for responses against races MNPSD, TBBGS, TDBJQ, and TNBJS, respectively (Figure 3, Table 4). The nine MTAs for race MNPSD were identified on eight different chromosomes including 1A, 2B, 2D, 3B, 4A, 4B, 5A, and 5B (Figure 3, Table 4). The most significant MTA for MNPSD (scaffold9496_550027; -log10P = 11.5) was observed on chromosome 3B physically mapped to 456 Mb and had a SNP effect of 0.91 (Table 4). For response against TBBGS, a total of nine MTAs were identified on seven different chromosomes: 1B, 2A, 3B, 4B, 5A, 5B, and 6B (Figure 3, Table 4). Of note, the MTAs scaffold145719_3415472, scaffold20863_2950181, and scaffold81142-6_3121151 identified on chromosome 1B, 2A, and 5B showed a significant effect value of -0.81, 0.66, and -0.80, respectively. Further, eight significant MTAs were detected for TNBJS mapped on six different chromosomes including 1A, 1B, 2B, 2D, 3B, and 4A (Figure 3, Table 4). Among these eight MTAs, the most significant MTA (scaffold63719_1362898) was identified on chromosome 4A at 625 Mb and had a SNP effect value of 0.81. In contrast to the other races, we identified only one MTA (scaffold38811_1402219) for isolate TDBJQ mapped at 503 Mb on chromosome 4D (Figure 3, Table 4).
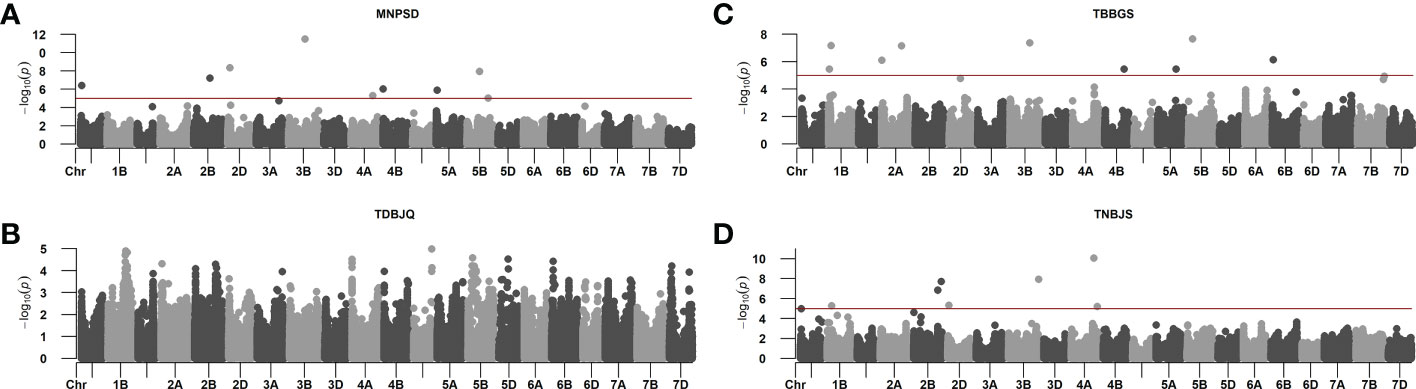
Figure 3 Manhattan plots from genome-wide association studies showing the distinct peaks for identified MTAs in response to (A) MNPSD, (B) TDBJQ, (C) TBBGS, and (D) TNBJS races of P. triticina. The red horizontal line represents the threshold used to report MTAs for each race.
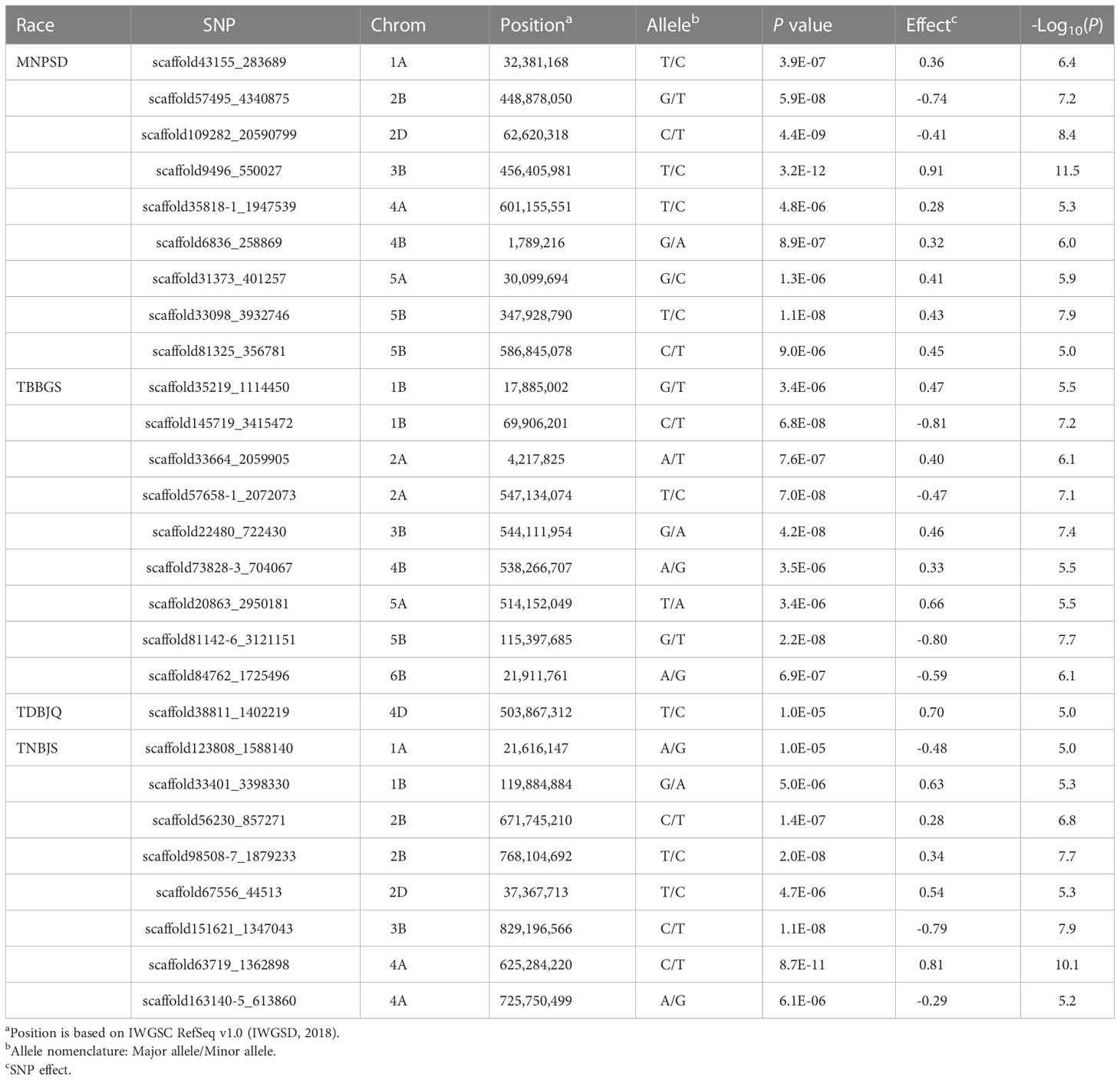
Table 4 Summary of the marker-trait associations (MTAs) identified for resistance to P. triticina races MNPSD, TBBGS, TDBJQ, and TNBJS.
Furthermore, we evaluated the additive effect of resistant alleles of significant MTAs on mean infection response from individual races. As we identified only one MTA for TDBJQ, data from only three races were used for this analysis. Overall, we observed a significant negative association between the number of resistant alleles and infection response for all three races suggesting that the accumulation of resistant alleles in genotypes reduces infection response (Figure 4). The accessions with two or more resistant alleles exhibited a lower infection response against all individual races.
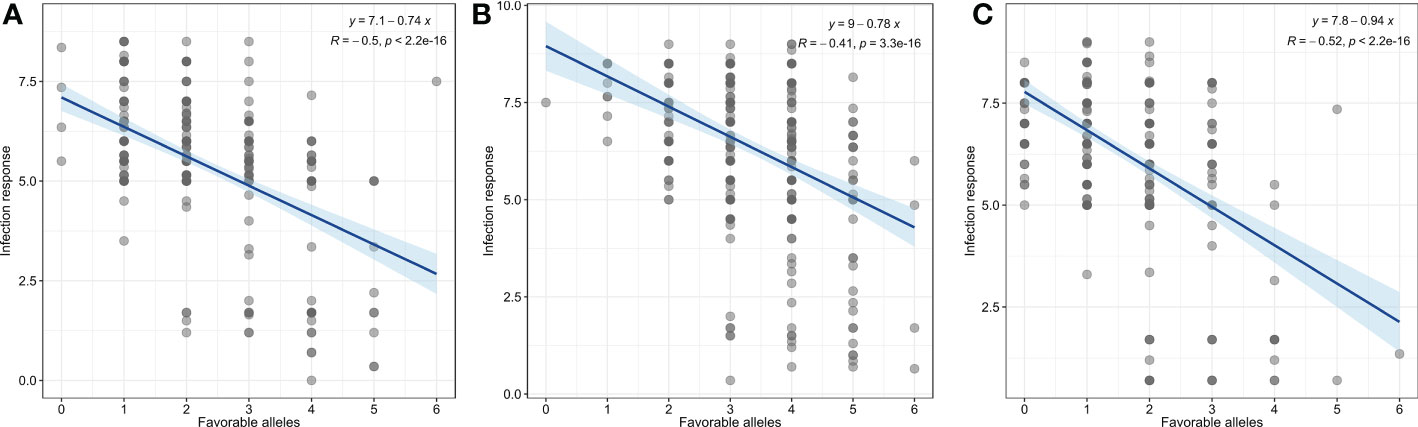
Figure 4 Linear regression plots of seedling response toward P. triticina races (A) MNPSD, (B) TBBGS and, (C) TNBJS to the number of favorable alleles of identified MTAs for respective races.
Candidate gene analysis for significant marker-trait associations
The significant MTAs were further analyzed to identify candidate genes underneath or in close vicinity of the MTAs in the wheat genome. Among the 27 MTAs, 20 were mapped in the proximity of previously reported MTAs (Supplementary Table S7), QTLs or genes for LR resistance, validating the importance of these regions. Thus, we selected these regions for identifying putative genes of interest that may play a role in LR resistance. Further, we investigated the local LD decay in these 20 regions by constructing haplotype blocks. Out of the 20 regions, LD decay rate was found to be less than 1 Mb in 17 regions (data not shown). Henceforth, a 1 Mb window around the associated SNP was used to retrieve high-confidence genes using CS RefSeq v1.1. In total, we identified 252 high confidence (HC) genes in +/- 1 Mb of the significant MTAs (Supplementary Table S8). Further, a thorough comparison with literature identified genes of interest that encode known plant disease resistance proteins such as intracellular nucleotide-binding and leucine-rich repeat (NLR) receptors, proteins with kinase domains, ATP-binding cassette (ABC) transporters, F-box-like domain-containing proteins, defensins, receptor kinase proteins, and others (Supplementary Table S9).
Discussion
Wheat germplasm collections serve as major resources for enriching the wheat genetic diversity against various biotic and abiotic stresses including pathogens such as rusts. Utilizing the untapped value of these genetic repositories can help identify potential novel sources of genes and QTLs and thereby advance the course of broadening the resistance diversity against leaf rust. Association studies have been successfully deployed in both bread wheat and durum wheat for detecting genomic regions associated with leaf rust resistance at seedling and adult plant stages (Aoun et al., 2016; Gao et al., 2016; Li et al., 2016; Sapkota et al., 2019; Leonova et al., 2020; Aoun et al., 2021). Foliar diseases of wheat like leaf rust are among the most important and destructive diseases in the Northern Great Plains of the US. Identifying novel sources of resistance against leaf rust is a continuing challenge due to the constant evolution of Pt populations causing the resistance breakdown of the existing Lr genes. In 2020, 15 virulence phenotypes were identified from 140 isolates in North Dakota, Minnesota, and South Dakota with TBBGS (42.1%), MNPSD (17.9%), and TNBJS (5.7%) being the most predominant phenotypes (https://www.ars.usda.gov/midwest-area/stpaul/cereal-disease-lab/docs/cereal-rusts/race-surveys/ ). Therefore, we evaluated a global wheat diversity panel of 365 hexaploid wheat accessions for identifying genetic loci harboring novel resistance genes for leaf rust against four important and prevalent races namely, TDBJQ, TBBGS, MNPSD, and TNBJS, at the seedling stage.
Our phenotypic evaluations identified a substantial number of resistant accessions from both North and South America (Supplementary Table S5) against the Pt races. Further, we identified 59 spring, 10 winter, and 2 facultative growth habit accessions out of the 71 resistant lines carrying race-specific resistance to all four Pt races that are prevalent in the north-central region of the United States (Supplementary Table S6). The majority of the accessions are cultivated lines from the Americas (38%) followed by Europe (25.3%), Asia (22.5%), and Africa (9.9%) (Supplementary Table S6). Leaf rust resistance in a sizeable proportion (18.3%) of North American lines could be due to their selection against Pt races predominant in North America. Similar results were reported by (Gao et al., 2016), where high resistance was observed in a sub-population consisting mostly North American lines when screened against multiple Pt races common in that region. The resistance response to Pt races, TNBJS and TBBGS showed a slightly higher correlation (0.39) (Supplementary Figure S1) as compared to the other races which could be due to the similarities in the virulence profile as both races are virulent on Lr1, Lr2a, Lr2c, Lr3, Lr10, Lr21, Lr28, and Lr39. Out of the 71 resistant lines, 10 wheat accessions displayed a highly resistant response (IR<= 4) against a combination of three different races, and only 3 lines were highly resistant (IR<= 4) against all four races (Supplementary Table S6). These lines may contain one or more existing or novel genes and can serve as a potential source for transferring this resistance into wheat cultivars by the breeding programs.
For conducting GWAS, a high quality set of SNPs was used to identify marker trait associations for reaction to four Pt isolates (Supplementary Table S10). GWAS with FarmCPU algorithm identified 27 MTAs for leaf rust resistance on the eleven wheat chromosomes (Table 4). The BLUEs were not normally distributed, so we tried BoxCox transformation to normalize the data. However, the transformation did not improve the distribution of BLUEs (Supplementary Figure S4), hence we used non-transformed data for performing GWAS. Out of 27 MTAs, 20 were identified in the vicinity of previously reported genes, MTAs, or QTLs associated with leaf rust resistance. An important MTA (scaffold33664_2059905) was identified for LR resistance against TBBGS which mapped around 4 Mb on the short arm of chromosome 2A. Interestingly, this region (0.5 – 7.8 Mb) has been reported to harbor three known Lr genes including Lr17 (Dyck and Kerber, 1977), Lr37 (Bariana and McIntosh, 1993; Blaszczyk et al, 2004), and Lr65 (Wang et al., 2010; Mohler et al., 2012; Zhang et al., 2021a), and a recently identified QTL for LR resistance (Fatima et al., 2020) (Supplementary Table S7). A long chromosomal fragment of 25-38 cM containing Lr37, Yr17, and Sr38 was introduced from a wild wheat relative Aegilops ventricosa and located on a 2NS/2AS translocation in a winter wheat cultivar ‘VPM1’ (Bariana and McIntosh, 1993). Lr37 is an adult plant resistance gene but expresses a seedling resistance response of 2+ at temperatures below 20°C (Park and McIntosh, 1994; Kolmer, 1996). Recently this segment was also found to provide resistance against wheat blast. In addition to Lr37, this MTA (scaffold33664_2059905) colocalized with WMS636 (4.9 Mb), a distal flanking marker associated with genes Lr17a (Bremenkamp-Barrett et al, 2008) and the two makers, AltID-11(0.55Mb) and Alt-92 (0.61Mb), flanking Lr65 (Zhang et al., 2021b). Since TBBGS is avirulent against Lr65 and the identified MTA colocalized with markers tightly linked to Lr65, it is highly likely that the locus identified in this study is identical to the Lr65 locus. Zhang et al. (2021b) identified TraesCS2A02G001500 encoding NB-ARC and LRR domains as the most prominent candidate gene for Lr65. On chromosome 4B, we identified an MTA (scaffold73828-3_704067) associated with response to TBBGS in the vicinity of two known Lr genes, Lr12 (Dyck et al., 1966) and Lr31 (Singh and McIntosh, 1984). Lr12 is a race specific adult plant resistance gene and is completely linked or identical to Lr31 which is a seedling resistance gene that requires another complementary gene Lr27/Sr2/Yr30 to function (Singh and McIntosh, 1984; Singh et al., 1999; Mago et al., 2011; Singh and Bowden, 2011). However, we did not identify any association in the 3BS region harboring Lr27 in this study.
Three MTAs (scaffold35219_1114450, scaffold145719_3415472 and scaffold33401_3398330) were identified on chromosome 1B against TBBGS and TNBJS. Two known Lr genes, Lr33 (Dyck, 1987) and Lr75 (Singla et al., 2017) were previously mapped on chromosome 1B. Lr75 is an adult plant resistance gene and was first identified in the Swiss cultivar ‘Forno’ (Schnurbusch et al., 2004; Singla et al., 2017). The physical position of scaffold145719_3415472 approximately co-localized with the physical location of GWM604, a distal flanking marker linked to Lr75 on CS RefSeq v1.0 (Singla et al., 2017). Given that the scaffold145719_3415472 was identified for seedling resistance to LR, it is unlikely that this MTA represents Lr75. Another MTA on chromosome 1B (scaffold33401_3398330) was mapped 9.2 Mb apart from a diagnostic marker (BOBWHITE_C39153_131) linked to Lr33 (Che et al., 2019). Interestingly, scaffold35219_1114450, scaffold145719_3415472 and scaffold33401_3398330 were mapped within 1 Mb of several previously reported MTAs (IAAV8117, BS00083533_51, and BS00084722_51) for leaf and stripe rust resistance (Zhang et al., 2021a) validating their role in response to Pt (Supplementary Table S7).
We identified two MTAs (scaffold56230_857271 and scaffold98508-7_1879233) for race TNBJS on chromosome 2BL close to WMS382, a marker associated with Lr50 (Brown-Guedira et al., 2003). Lr50 was transferred from wild wheat, T. timopheevi armeniacum and was previously mapped on chromosome 2BL (Brown-Guedira et al., 2003). Furthermore, scaffold56230_857271, and scaffold98508-7_1879233 on 2BL mapped in close vicinity of previously reported MTAs (AX-95006189 and AX-94481202) for LR resistance (Kumar et al., 2020). Another MTA, scaffold151621_1347043 was mapped in the vicinity of a recently reported MTA (AX-94671785) for LR resistance (Vikas et al., 2022) (Supplementary Table S7).
In response to MNPSD, we detected an MTA (scaffold109282_20590799) which mapped on chromosome 2D near aWPT-0330, a marker linked to a seedling resistance gene, Lr2 (Dyck and Samborski, 1974; Tsilo et al., 2014). Similarly, scaffold81325_356781 on chromosome 5B mapped ~ 8 Mbp apart from marker BOBWHITE_REP_C50349_139 (Carpenter et al., 2017), which is linked to Lr18 (Dyck and Samborski, 1968). Further studies are needed to determine the relationship between the identified MTAs and the postulated Lr genes in close proximity to MTAs. Joukhadar et al. (2020) conducted a GWAS study using 2,300 hexaploid wheat genotypes including worldwide landraces, cultivars and synthetic backcross derivatives for adult plant resistance to leaf rust, stripe and stem rust across multiple Australian environments. Of the 365 wheat accessions used in our study, 257 genotypes overlapped with the adult plant screening panel (Joukhadar et al., 2020); interestingly no common resistant lines and significant marker-trait associations for leaf rust were found among the two studies.
In addition, our analyses also identified two novel MTAs (scaffold9496_550027 and scaffold57658-1_2072073) that were located in genomic regions where no previously reported Lr genes or MTAs have been reported in wheat (Supplementary Table S7). Further, we also identified five putatively novel MTAs (scaffold33098_3932746, scaffold57495_4340875, scaffold163140-5_613860, scaffold151621_1347043, and scaffold56230_857271) mapped at >10 Mb apart from the previously reported MTAs in various GWAS studies (Supplementary Table S7). A total of three, one, and three significant novel MTAs were identified for resistance against MNPSD, TBBGS, and TNBJS, respectively. One of these seven MTAs, scaffold57495_4340875, detected on chromosome 2B was found near Lr35, (Kerber and Dyck, 1990) an APR gene expressing at two-leaf stage. BCD260 (Seyfarth et al., 1999), a marker linked to the Lr35, was about 30 Mb apart scaffold57495_4340875. Given Lr35 confers APR and the associated marker is far away from the identified MTA, it is unlikely the same locus. Another MTA, scaffold163140-5_613860 against race TNBJS was mapped on the region harboring known gene Lr28 (McIntosh et al., 1982) on chromosome 4A. However, TNBJS is virulent on Lr28 which eliminates the possibility of association of Lr28 with scaffold163140-5_613860. Further, scaffold163140-5_613860 was also mapped in the vicinity of a recently reported MTA (AX-95106749) for adult plant LR resistance (Vikas et al., 2022). Since, scaffold163140-5_613860 was identified for seedling resistance to LR, it is unlikely that this MTA represents AX-95106749. Similarly, scaffold56230_857271, scaffold151621_1347043, and scaffold33098_3932746, identified on chromosomes 2B, 3B, and 5B represent a novel locus associated with TNBJS and MNPSD respectively, as no previously reported Lr gene has been detected in this region.
Next, we selected 17 significant MTAs based on LD decay rate of less than 1Mbp to identify candidate genes with putative role in disease resistance (Supplementary Tables S8, S9). The putative candidate genes belonged to different disease resistance encoding gene families such as leucine-rich repeats receptor-like kinases (LRR-RLKs), nucleotide-binding site leucine-rich repeats (NBS-LRRs), serine/threonine-protein phosphatase domain containing proteins, ABC transporters, zinc finger proteins, and others (Supplementary Table S9). Zinc finger domains have been identified in various disease resistance genes from several crops, indicating their significant role in conferring host-plant resistance (Epple et al., 2003; Ciftci-Yilmaz and Mittler, 2008; Emerson and Thomas, 2009). Further, serine/threonine-protein phosphatase domain containing proteins are known to be involved in regulation of plant defense and stress responses (País et al., 2009; Máthé et al., 2019). LRR-RLKs and ABC transporters have known to be involved in a wide variety of developmental and defense-related processes (Torii, 2004; Krattinger et al., 2009; Kang et al., 2011). Additionally, a few more genes encoding putative proteins of interest including plant defensins, germin-like proteins, E3 ubiquitin-protein ligase, cytochrome P450 family protein, F-box proteins (FBPs), and others were identified (Supplementary Tables S8, S9). These identified genes could serve as valuable information in future gene cloning efforts of these genomic regions.
In conclusion, we identified valuable sources of LR resistance against multiple Pt races. The SNP markers reported as associated with resistance can facilitate the deployment of these QTLs through marker-assisted selection in breeding programmes.
Data availability statement
The original contributions presented in the study are included in the article/Supplementary Material. Further inquiries can be directed to the corresponding authors.
Ethics statement
The authors declare that the experiments comply with the current laws of the country.
Author contributions
UG and SS conceptualized the experiment and designed the methodology; SK, and UG performed the investigation; SK and HG performed the data curation, data analysis, and visualization; SK and HG performed the software implementation; SK, HG, SS, and UG wrote the original manuscript; SK, HG, JK, RG, SS, and UG contributed to the interpretation of results. All authors contributed to the article and approved the submitted version.
Funding
This project was collectively supported by the USDA National Institute of Food and Agriculture, Hatch project number ND02243, North Dakota Wheat Commission, and the USDA Agriculture and Food Research Initiative Competitive Grants 2022-68013-36439 (Wheat-CAP) from the USDA National Institute of Food and Agriculture. The funders had no role in the study design, data collection, analysis, decision to publish, or manuscript preparation.
Acknowledgments
The authors would like to thank Julie Hochhalter for providing the greenhouse resources at Dalrymple Research Greenhouse complex at Fargo, ND.
Conflict of interest
The authors declare that the research was conducted in the absence of any commercial or financial relationships that could be construed as a potential conflict of interest.
Publisher’s note
All claims expressed in this article are solely those of the authors and do not necessarily represent those of their affiliated organizations, or those of the publisher, the editors and the reviewers. Any product that may be evaluated in this article, or claim that may be made by its manufacturer, is not guaranteed or endorsed by the publisher.
Supplementary material
The Supplementary Material for this article can be found online at: https://www.frontiersin.org/articles/10.3389/fpls.2023.1090163/full#supplementary-material
Supplementary Figure S1 | Correlation analysis among the phenotypic data of 365 wheat accessions evaluated for their reaction to P. triticina races TDBJQ, TBBGS, MNPSD, and TNBJS.
Supplementary Figure S2 | Scatterplot based on the first two components (PC1 and PC2) from PCA for (A) growth habit and (B) geographic origin.
Supplementary Figure S3 | Intra-chromosomal linkage disequilibrium in the diversity panel for (A) whole genome and, individually in the (B) A, (C) B, and (D) D sub-genomes.
Supplementary Figure S4 | Box-cox transformations performed for Pt races TDBJQ, TBBGS, MNPSD, and TNBJS to normalize the data.
Supplementary Table S1 | List of accessions included in the study and their respective exome capture ID, origin, growth habit and type.
Supplementary Table S2 | Infection type (IT) ratings on a 0-4 scale for response against four P. triticina races.
Supplementary Table S3 | Infection type (IT) ratings converted to a 1-9 linear scale (Infection response, IR) for response against four P. triticina races.
Supplementary Table S4 | Best linear unbiased estimates (BLUEs) for phenotypic responses from each of the four Pt races used for conducting GWAS.
Supplementary Table S5 | Distribution of resistant accessions (IR 0-6) against four Pt races based on their geographical distribution.
Supplementary Table S6 | List of 71 accessions highly resistant (IR < = 4) and moderately resistant (IR 5-6) to different combinations of the four Pt races. The lines highlighted in yellow represent the highly resistant lines against a combination of three or all the four races.
Supplementary Table S7 | Comparison of the identified MTAs in this study with significant MTAs from previous GWAS studies.
Supplementary Table S8 | List of all high confidence genes identified for significant MTAs against Pt races, MNPSD, TBBGS, and TNBJS, with their functional annotations.
Supplementary Table S9 | Candidate genes with putative functions of interest identified for significant MTAs against Pt races, MNPSD, TBBGS, and TNBJS.
Supplementary Table S10 | The distribution of 302,524 SNPs across 21 wheat chromosomes.
References
AlTameemi, R., Gill, H. S., Ali, S., Ayana, G., Halder, J., Sidhu, J. S., et al. (2021). Genome-wide association analysis permits characterization of stagonospora nodorum blotch (SNB) resistance in hard winter wheat. Sci. Rep. 11, 12570. doi: 10.1038/s41598-021-91515-6
Alvarado, G., Rodríguez, F. M., Pacheco, A., Burgueño, J., Crossa, J., Vargas, M., et al. (2020). META-r: A software to analyze data from multi-environment plant breeding trials. Crop J. 8, 745–756. doi: 10.1016/j.cj.2020.03.010
Aoun, M., Breiland, M., Kathryn Turner, M., Loladze, A., Chao, S., Xu, S. S., et al. (2016). Genome-wide association mapping of leaf rust response in a durum wheat worldwide germplasm collection. Plant Genome 9, 3. doi: 10.3835/plantgenome2016.01.0008
Aoun, M., Rouse, M. N., Kolmer, J. A., Kumar, A., Elias, E. M. (2021). Genome-wide association studies reveal all-stage rust resistance loci in elite durum wheat genotypes. Front. Plant Sci. 12. doi: 10.3389/fpls.2021.640739
Bansal, M., Kaur, S., Dhaliwal, H. S., Bains, N. S., Bariana, H. S., Chhuneja, P., et al. (2017). Mapping of Aegilops umbellulata -derived leaf rust and stripe rust resistance loci in wheat. Plant Pathol. 66, 38–44. doi: 10.1111/ppa.12549
Bariana, H. S., McIntosh, R. A. (1993). Cytogenetic studies in wheat. XV. location of rust resistance genes in VPM1 and their genetic linkage with other disease resistance genes in chromosome 2A. Genome 36, 476–482. doi: 10.1139/g93-065
Bates, D., Mächler, M., Bolker, B. M., Walker, S. C. (2015). Fitting linear mixed-effects models using lme4. J. Stat. Software 67, 1–48. doi: 10.18637/jss.v067.i01
Błaszczyk, L., Goyeau, H., Huang, X. Q., Röder, M., Stpień, Ł., Chełkowski, J. (2004). Identifying leaf rust resistance genes and mapping gene Lr37 on the microsatellite map of wheat. Cell. Mol. Biol. Lett. 9, 869–878.
Bolton, M. D., Kolmer, J. A., Garvin, D. F. (2008). Wheat leaf rust caused by Puccinia triticina. Mol. Plant Pathol. 9, 563–575. doi: 10.1111/j.1364-3703.2008.00487.x
Borrill, P., Ramirez-Gonzalez, R., Uauy, C. (2016). expVIP: a customizable RNA-seq data analysis and visualization platform. Plant Physiol. 170, 2172–2186. doi: 10.1104/pp.15.01667
Bremenkamp-Barrett, B., Faris, J. D., Fellers, J. P. (2008). Molecular mapping of the leaf rust resistance gene Lr17a in wheat. Crop Sci. 48, 1124–1128. doi: 10.2135/cropsci2007.07.0379
Brown-Guedira, G. L., Singh, S., Fritz, A. K. (2003). Performance and mapping of leaf rust resistance transferred to wheat from Triticum timopheevii subsp. armeniacum. Phytopathology 93, 784–789. doi: 10.1094/PHYTO.2003.93.7.784
Browning, S. R., Browning, B. L. (2007). Rapid and accurate haplotype phasing and missing-data inference for whole-genome association studies by use of localized haplotype clustering. Am. J. Hum. Genet. 81, 1084–1097. doi: 10.1086/521987
Carpenter, N. R., Griffey, C. A., Baudoin, A. B., Holliday, J. A., Saghai-Maroof, M. A. (2017). Identification and mapping of resistance to puccinia striiformis and puccinia triticina in soft red winter wheat [Doctoral dissertation] (Blacksburg (VA: Virginia Tech).
Che, M., Hiebert, C. W., McCartney, C. A., Zhang, Z., McCallum, B. D. (2019). Mapping and DNA marker development for Lr33 from the leaf rust resistant line KU168-2. Euphytica 215, 29. doi: 10.1007/s10681-019-2343-3
Chen, J., Zhang, F., Zhao, C., Lv, G., Sun, C., Pan, Y., et al. (2019). Genome-wide association study of six quality traits reveals the association of the TaRPP13L1 gene with flour colour in Chinese bread wheat. Plant Biotechnol. J. 17, 2106–2122. doi: 10.1111/pbi.13126
Chhatre, V. E., Emerson, K. J. (2017). StrAuto: Automation and parallelization of STRUCTURE analysis. BMC Bioinfo. 18, 192. doi: 10.1186/s12859-017-1593-0
Chhetri, M., Bansal, U., Toor, A., Lagudah, E., Bariana, H. (2016). Genomic regions conferring resistance to rust diseases of wheat in a W195/BTSS mapping population. Euphytica 209, 637–649. doi: 10.1007/s10681-016-1640-3
Ciftci-Yilmaz, S., Mittler, R. (2008). The zinc finger network of plants. Cell. Mol. Life Sci. 65, 1150–1160. doi: 10.1007/s00018-007-7473-4
Cloutier, S., McCallum, B. D., Loutre, C., Banks, T. W., Wicker, T., Feuillet, C., et al. (2007). Leaf rust resistance gene Lr1, isolated from bread wheat (Triticum aestivum l.) is a member of the large psr567 gene family. Plant Mol. Biol. 65, 93–106. doi: 10.1007/s11103-007-9201-8
Dyck, P. L. (1987). The association of a gene for leaf rust resistance with the chromosome 7D suppressor of stem rust resistance in common wheat. Genome 29, 467–469. doi: 10.1139/g87-081
Dyck, P. L., Kerber, E. R. (1977). Inheritance of leaf rust resistance in wheat cultivars rafaela and EAP 26127 and chromosome location.of gene Lr17. Can. J. Genet. Cytol. 19, 355–358. doi: 10.1139/g77-038
Dyck, P. L., Samborski, D. J. (1968). Genetics of resistance to leaf rust in the common wheat varieties Webster, loros, brevit, Carina, malakof and centenario. Can. J. Genet. Cytol. 10, 7–17. doi: 10.1139/g68-002
Dyck, P. L., Samborski, D. J. (1974). Inheritance of virulence in Puccinia recondita on alleles at the Lr2 locus for resistance in wheat. Can. J. Genet. Cytol. 16, 323–332. doi: 10.1139/g74-036
Dyck, P. L., Samborski, D. J., Anderson, R. G. (1966). Inheritance of adult-plant leaf rust resistance derived from the common wheat varieties exchange and frontana. Can. J. Genet. Cytol. 8, 665–671. doi: 10.1139/g66-082
Earl, D. A., vonHoldt, B. M. (2012). STRUCTURE HARVESTER: a website and program for visualizing STRUCTURE output and implementing the evanno method. Conserv. Genet. Resour. 4, 359–361. doi: 10.1007/s12686-011-9548-7
Emerson, R. O., Thomas, J. H. (2009). Adaptive evolution in z inc finger transcription factors. PloS Genet. 5, e1000325. doi: 10.1371/journal.pgen.1000325
Epple, P., Mack, A. A., Morris, V. R. F., Dangl, J. L. (2003). Antagonistic control of oxidative stress-induced cell death in Arabidopsis by two related, plant-specific zinc finger proteins. Proc. Natl. Acad. Sci. 100, 6831–6836. doi: 10.1073/pnas.1130421100
Evanno, G., Regnaut, S., Goudet, J. (2005). Detecting the number of clusters of individuals using the software STRUCTURE: A simulation study. Mol. Ecol. 14, 2611–2620. doi: 10.1111/j.1365-294X.2005.02553.x
Fatima, F., McCallum, B. D., Pozniak, C. J., Hiebert, C. W., McCartney, C. A., Fedak, G., et al. (2020). Identification of new leaf rust resistance loci in wheat and wild relatives by array-based SNP genotyping and association genetics. Front. Plant Sci. 11. doi: 10.3389/fpls.2020.583738
Feuillet, C., Travella, S., Stein, N., Albar, L., Nublat, A., Keller, B. (2003). Map-based isolation of the leaf rust disease resistance gene Lr10 from the hexaploid wheat ( Triticum aestivum l.) genome. Proc. Natl. Acad. Sci. 100, 15253–15258. doi: 10.1073/pnas.2435133100
Gao, L., Turner, M. K., Chao, S., Kolmer, J., Anderson, J. A. (2016). Genome wide association study of seedling and adult plant leaf rust resistance in elite spring wheat breeding lines. Euphytica 11, e0148671. doi: 10.1371/journal.pone.0148671
Gao, F., Wen, W., Liu, J., Rasheed, A., Yin, G., Xia, X., et al. (2015). Genome-wide linkage mapping of QTL for yield components, plant height and yield-related physiological traits in the Chinese wheat cross zhou 8425B/Chinese spring. Front. Plant Sci. 6. doi: 10.3389/fpls.2015.01099
Gill, H. S., Halder, J., Zhang, J., Brar, N. K., Rai, T. S., Hall, C., et al. (2021). Multi-trait multi-environment genomic prediction of agronomic traits in advanced breeding lines of winter wheat. Front. Plant Sci. 12. doi: 10.3389/fpls.2021.709545
Gill, H. S., Li, C., Sidhu, J. S., Liu, W., Wilson, D., Bai, G., et al. (2019). Fine mapping of the wheat leaf rust resistance gene Lr42. Int. J. Mol. Sci. 20, 2445. doi: 10.3390/ijms20102445
Gyawali, S., Chao, S., Vaish, S. S., Singh, S. P., Rehman, S., Vishwakarma, S. R., et al. (2018). Genome wide association studies (GWAS) of spot blotch resistance at the seedling and the adult plant stages in a collection of spring barley. Mol. Breed. 38, 62. doi: 10.1007/s11032-018-0815-0
Halder, J., Zhang, J., Ali, S., Sidhu, J. S., Gill, H. S., Talukder, S. K., et al. (2019). Mining and genomic characterization of resistance to tan spot, stagonospora nodorum blotch (SNB), and fusarium head blight in Watkins core collection of wheat landraces. BMC Plant Biol. 19, 1–15. doi: 10.1186/s12870-019-2093-3
He, F., Pasam, R., Shi, F., Kant, S., Keeble-Gagnere, G., Kay, P., et al. (2019). Exome sequencing highlights the role of wild-relative introgression in shaping the adaptive landscape of the wheat genome. Nat. Genet. 51, 896–904. doi: 10.1038/s41588-019-0382-2
Herrera-Foessel, S. A., Singh, R. P., Huerta-Espino, J., Rosewarne, G. M., Periyannan, S. K., Viccars, L., et al. (2012). Lr68: A new gene conferring slow rusting resistance to leaf rust in wheat. Theor. Appl. Genet. 124, 1475–1486. doi: 10.1007/S00122-012-1802-1
Hewitt, T., Zhang, J., Huang, L., Upadhyaya, N., Li, J., Park, R., et al. (2021). Wheat leaf rust resistance gene Lr13 is a specific Ne2 allele for hybrid necrosis. Mol. Plant 14, 1025–1028. doi: 10.1016/j.molp.2021.05.010
Hiebert, C. W., Thomas, J. B., McCallum, B. D., Humphreys, D. G., DePauw, R. M., Hayden, M. J., et al. (2010). An introgression on wheat chromosome 4DL in RL6077 (Thatcher*6/PI 250413) confers adult plant resistance to stripe rust and leaf rust (Lr67). Theor. Appl. Genet. 121, 1083–1091. doi: 10.1007/s00122-010-1373-y
Huang, L., Brooks, S. A., Li, W., Fellers, J. P., Trick, H. N., Gill, B. S. (2003). Map-based cloning of leaf rust resistance gene Lr21 from the large and polyploid genome of bread wheat. Genetics 164, 655–664. doi: 10.1093/genetics/164.2.655
Huerta-Espino, J., Singh, R. P., Germán, S., McCallum, B. D., Park, R. F., Chen, W. Q., et al. (2011). Global status of wheat leaf rust caused by Puccinia triticina. Euphytica 179, 143–160. doi: 10.1007/s10681-011-0361-x
Joukhadar, R., El-Bouhssini, M., Jighly, A., Ogbonnaya, F. C. (2013). Genome-wide association mapping for five major pest resistances in wheat. Mol. Breed. 32, 943–960. doi: 10.1007/s11032-013-9924-y
Joukhadar, R., Hollaway, G., Shi, F., Kant, S., Forrest, K., Wong, D., et al. (2020). Genome-wide association reveals a complex architecture for rust resistance in 2300 worldwide bread wheat accessions screened under various Australian conditions. Theor. Appl. Genet. 133, 2695–2712. doi: 10.1007/s00122-020-03626-9
Kang, J., Park, J., Choi, H., Burla, B., Kretzschmar, T., Lee, Y., et al. (2011). Plant ABC transporters. Arabidopsis book. 9, e0153. doi: 10.1199/tab.0153
Kerber, E. R., Dyck, P. L. (1990). Transfer to hexaploid wheat of linked genes for adult-plant leaf rust and seedling stem rust resistance from an amphiploid of Aegilops speltoides × Triticum monococcum. Genome 33, 530–537. doi: 10.1139/g90-079
Kolmer, J. A. (1996). Genetics of resistance to wheat leaf rust. Annu. Rev. Phytopathol. 34, 435–455. doi: 10.1146/annurev.phyto.34.1.435
Kolmer, J. A. (2005). Tracking wheat rust on a continental scale. Curr. Opin. Plant Biol. 8, 441–449. doi: 10.1016/j.pbi.2005.05.001
Kolmer, J. A. (2019). Virulence of Puccinia triticina, the wheat leaf rust fungus, in the united states in 2017. Plant Dis. 103, 2113–2120. doi: 10.1094/PDIS-09-18-1638-SR
Kolmer, J. A., Bernardo, A., Bai, G., Hayden, M. J., Chao, S. (2018a). Adult plant leaf rust resistance derived from toropi wheat is conditioned by Lr78 and three minor QTL. Phytopathology 108, 246–253. doi: 10.1094/PHYTO-07-17-0254-R
Kolmer, J. A., Jin, Y., Long, D. L. (2007). Wheat leaf and stem rust in the united states. Aust. J. Agric. Res. 58, 631–638. doi: 10.1071/AR07057
Kolmer, J. A., Su, Z., Bernardo, A., Bai, G., Chao, S. (2018b). Mapping and characterization of the new adult plant leaf rust resistance gene Lr77 derived from Santa fe winter wheat. Theor. Appl. Genet. 131, 1553–1560. doi: 10.1007/s00122-018-3097-3
Kolodziej, M. C., Singla, J., Saínchez-Martın, J., Zbinden, H., S íimkovaí, H., Karafiaítovaí, M., et al. (2021). A membrane-bound ankyrin repeat protein confers race-specific leaf rust disease resistance in wheat. Nat. Commun. 12, 956. doi: 10.1038/s41467-020-20777-x
Krattinger, S. G., Lagudah, E. S., Spielmeyer, W., Singh, R. P., Huerta-Espino, J., McFadden, H., et al. (2009). A putative ABC transporter confers durable resistance to multiple fungal pathogens in wheat. Science 323, 1360–1363. doi: 10.1126/science.1166453
Kristensen, P. S., Jahoor, A., Andersen, J. R., Cericola, F., Orabi, J., Janss, L. L., et al. (2018). Genome-wide association studies and comparison of models and cross-validation strategies for genomic prediction of quality traits in advanced winter wheat breeding lines. Front. Plant Sci. 9. doi: 10.3389/fpls.2018.00069
Kumar, S., Bhardwaj, S. C., Gangwar, O. P., Sharma, A., Qureshi, N., Kumaran, V. V., et al. (2021b). Lr80: A new and widely effective source of leaf rust resistance of wheat for enhancing diversity of resistance among modern cultivars. Theor. Appl. Genet. 134, 849–858. doi: 10.1007/s00122-020-03735-5
Kumar, D., Kumar, A., Chhokar, V., Gangwar, O. P., Bhardwaj, S. C., Sivasamy, M., et al. (2020). Genome-wide association studies in diverse spring wheat panel for stripe, stem, and leaf rust resistance. Front. Plant Sci. 11. doi: 10.3389/fpls.2020.00748
Kumar, D., Sharma, S., Sharma, R., Pundir, S., Singh, V. K., Chaturvedi, D., et al. (2021a). Genome-wide association study in hexaploid wheat identifies novel genomic regions associated with resistance to root lesion nematode (Pratylenchus thornei). Sci. Rep. 11, 3572. doi: 10.1038/s41598-021-80996-0
Leonova, I. N., Skolotneva, E. S., Salina, E. A. (2020). Genome-wide association study of leaf rust resistance in Russian spring wheat varieties. BMC Plant Biol. 20, 135. doi: 10.1186/s12870-020-02333-3
Lin, G., Chen, H., Tian, B., Sehgal, S., Singh, L., Xie, J., et al. (2022). Cloning of the broadly effective wheat leaf rust resistance gene Lr42 transferred from Aegilops tauschii. Nat. Commun. 13, 3044. doi: 10.1038/s41467-022-30784-9
Liu, X., Huang, M., Fan, B., Buckler, E. S., Zhang, Z. (2016). Iterative usage of fixed and random effect models for powerful and efficient genome-wide association studies. PloS Genet. 12, e1005767. doi: 10.1371/journal.pgen.1005767
Li, G., Xu, X., Bai, G., Carver, B. F., Hunger, R., Bonman, J. M., et al. (2016). Genome-wide association mapping reveals novel QTL for seedling leaf rust resistance in a worldwide collection of winter wheat. Plant Genome 9, 1–12. doi: 10.3835/plantgenome2016.06.0051
Mago, R., Tabe, L., McIntosh, R. A., Pretorius, Z., Kota, R., Paux, E., et al. (2011). A multiple resistance locus on chromosome arm 3BS in wheat confers resistance to stem rust (Sr2), leaf rust (Lr27) and powdery mildew. Theor. Appl. Genet. 123, 615–623. doi: 10.1007/s00122-011-1611-y
Máthé, C., Garda, T., Freytag, C., M-Hamvas, M. (2019). The role of serine-threonine protein phosphatase PP2A in plant oxidative stress signaling–facts and hypotheses. Int. J. Mol. Sci. 20, 3028. doi: 10.3390/ijms20123028
McIntosh, R. A., Miller, T. E., Chapman, V. (1982). Cytogenetical studies in wheat XII. Lr28 for resistance to Puccinia recondita and Sr34 for resistance to P. graminis tritici. z. Pflanzenzucht 89, 295–306.
Mohler, V., Singh, D., Singrün, C., Park, R. F. (2012). Characterization and mapping of Lr65 in spelt wheat ‘Altgold rotkorn.’. Plant Breed. 131, 252–257. doi: 10.1111/j.1439-0523.2011.01934.x
Mondal, S., Rutkoski, J. E., Velu, G., Singh, P. K., Crespo-Herrera, L. A., Guzmán, C., et al. (2016). Harnessing diversity in wheat to enhance grain yield, climate resilience, disease and insect pest resistance and nutrition through conventional and modern breeding approaches. Front. Plant Sci. 7. doi: 10.3389/fpls.2016.00991
Moore, J. W., Herrera-Foessel, S., Lan, C., Schnippenkoetter, W., Ayliffe, M., Huerta-Espino, J., et al. (2015). A recently evolved hexose transporter variant confers resistance to multiple pathogens in wheat. Nat. Genet. 47, 1494–1498. doi: 10.1038/ng.3439
País, S. M., Teresa Téllez-Iñón, M., Capiati, D. A. (2009). Serine/Threonine protein phosphatases type 2A and their roles in stress signaling. Plant Signal. Behav. 4, 1013–1015. doi: 10.4161/psb.4.11.9783
Pang, Y., Liu, C., Wang, D., St. Amand, P., Bernardo, A., Li, W., et al. (2020). High-resolution genome-wide association study identifies genomic regions and candidate genes for important agronomic traits in wheat. Mol. Plant 13, 1311–1327. doi: 10.1016/j.molp.2020.07.008
Park, R. F., McIntosh, R. A. (1994). Adult plant resistances to Puccinia recondita f. sp. tritici in wheat. New Z. J. Crop Hortic. Sci. 22, 151–158. doi: 10.1080/01140671.1994.9513819
Phan, H. T. T., Rybak, K., Bertazzoni, S., Furuki, E., Dinglasan, E., Hickey, L. T., et al. (2018). Novel sources of resistance to septoria nodorum blotch in the vavilov wheat collection identified by genome-wide association studies. Theor. Appl. Genet. 131, 1223–1238. doi: 10.1007/s00122-018-3073-y
Prasad, P., Savadi, S., Bhardwaj, S. C., Gupta, P. K. (2020). The progress of leaf rust research in wheat. Fungal Biol. 124, 537–550. doi: 10.1016/j.funbio.2020.02.013
Pritchard, J. K., Stephens, M., Donnelly, P. (2000). Inference of population structure using multilocus genotype data. Genetics 155, 945–959. doi: 10.1093/genetics/155.2.945
Purcell, S., Neale, B., Todd-Brown, K., Thomas, L., Ferreira, M. A. R., Bender, D., et al. (2007). PLINK: A tool set for whole-genome association and population-based linkage analyses. Am. J. Hum. Genet. 81, 559–575. doi: 10.1086/519795
Qureshi, N., Bariana, H., Kumran, V. V., Muruga, S., Forrest, K. L., Hayden, M. J., et al. (2018). A new leaf rust resistance gene Lr79 mapped in chromosome 3BL from the durum wheat landrace Aus26582. Theor. Appl. Genet. 131, 1091–1098. doi: 10.1007/s00122-018-3060-3
R Core Team (2018). R: A language and environment for statistical computing, Vol. 2015. R Foundation for Statistical Computing, Vienna, Austria. Available at: https://www.R-project.org/
Sapkota, S., Hao, Y., Johnson, J., Buck, J., Aoun, M., Mergoum, M. (2019). Genome-wide association study of a worldwide collection of wheat genotypes reveals novel quantitative trait loci for leaf rust resistance. Plant Genome 12, 190033. doi: 10.3835/plantgenome2019.05.0033
Savary, S., Willocquet, L., Pethybridge, S. J., Esker, P., McRoberts, N., Nelson, A. (2019). The global burden of pathogens and pests on major food crops. Nat. Ecol. Evol. 3, 430–439. doi: 10.1038/s41559-018-0793-y
Schnurbusch, T., Paillard, S., Schori, A., Messmer, M., Schachermayr, G., Winzeler, M., et al. (2004). Dissection of quantitative and durable leaf rust resistance in Swiss winter wheat reveals a major resistance QTL in the Lr34 chromosomal region. Theor. Appl. Genet. 108, 477–484. doi: 10.1007/s00122-003-1444-4
Seyfarth, R., Feuillet, C., Schachermayr, G., Winzeler, M., Keller, B. (1999). Development of a molecular marker for the adult plant leaf rust resistance gene Lr35 in wheat. Theor. Appl. Genet. 99, 554–560. doi: 10.1007/s001220051268
Sidhu, J. S., Singh, D., Gill, H. S., Brar, N. K., Qiu, Y., Halder, J., et al. (2020). Genome-wide association study uncovers novel genomic regions associated with coleoptile length in hard winter wheat. Front. Genet. 10. doi: 10.3389/fgene.2019.01345
Singh, S., Bowden, R. L. (2011). Molecular mapping of adult-plant race-specific leaf rust resistance gene Lr12 in bread wheat. Mol. Breed. 28, 137–142. doi: 10.1007/s11032-010-9467-4
Singh, R. P., Huerta-Espino, J., Roelfs, A. P., Curtis, B. C. (2002). “The wheat rusts,” in Bread wheat-improvement and production. Eds. Curtis, B. C., Rajaram, S., Macherson, H. G. (Rome: FAO Plant Production and Protection Series), 227–249.
Singh, R. P., McIntosh, R. A. (1984). Complementary genes for reaction to Puccinia recondita tritici in Triticum aestivum . i. genetic and linkage studies. Can. J. Genet. Cytol. 26, 723–735. doi: 10.1139/g84-115
Singh, R. P., Mujeeb-Kazi, A., Huerta-Espino, J. (1998). Lr46 : A gene conferring slow-rusting resistance to leaf rust in wheat. Phytopathology 88, 890–894. doi: 10.1094/PHYTO.1998.88.9.890
Singh, D., Park, R. F., McIntosh, R. A. (1999). Genetic relationship between the adult plant resistance gene Lr12 and the complementary gene Lr31 for seedling resistance to leaf rust in common wheat. Plant Pathol. 48, 567–573. doi: 10.1046/j.1365-3059.1999.00391.x
Singla, J., Lüthi, L., Wicker, T., Bansal, U., Krattinger, S. G., Keller, B. (2017). Characterization of Lr75: a partial, broad-spectrum leaf rust resistance gene in wheat. Theor. Appl. Genet. 130, 1–12. doi: 10.1007/s00122-016-2784-1
Stakman, E. C., Stewart, D. M., Loegering, W. Q. (1962). Identification of physiologic races of puccinia graminis var. tritici (Washington, DC: USDA Agricultural Research Service), E617.
Sukumaran, S., Dreisigacker, S., Lopes, M., Chavez, P., Reynolds, M. P. (2015). Genome-wide association study for grain yield and related traits in an elite spring wheat population grown in temperate irrigated environments. Theor. Appl. Genet. 128, 353–363. doi: 10.1007/s00122-014-2435-3
Thind, A. K., Wicker, T., Šimková, H., Fossati, D., Moullet, O., Brabant, C., et al. (2017). Rapid cloning of genes in hexaploid wheat using cultivar-specific long-range chromosome assembly. Nat. Biotechnol. 35, 793–796. doi: 10.1038/nbt.3877
Tibbs Cortes, L., Zhang, Z., Yu, J. (2021). Status and prospects of genome-wide association studies in plants. Plant Genome 14, e20077. doi: 10.1002/tpg2.20077
Torii, K. U. (2004). Leucine-rich repeat receptor kinases in plants: structure, function, and signal transduction pathways. Int. Rev. Cytol. 234, 1–46. doi: 10.1016/s0074-7696(04)34001-5
Tsilo, T. J., Kolmer, J. A., Anderson, J. A. (2014). Molecular mapping and improvement of leaf rust resistance in wheat breeding lines. Phytopathology 104, 865–870. doi: 10.1094/PHYTO-10-13-0276-R
Vikas, V. K., Pradhan, A. K., Budhlakoti, N., Mishra, D. C., Chandra, T., Bhardwaj, S. C., et al. (2022). Multi-locus genome-wide association studies (ML-GWAS) reveal novel genomic regions associated with seedling and adult plant stage leaf rust resistance in bread wheat (Triticum aestivum l.). Heredity (Edinb). 128, 434–449. doi: 10.1038/s41437-022-00525-1
Wang, Y., Abrouk, M., Gourdoupis, S., Koo, D., Karafiaítovaí, M., Molnaír, I., et al. (2022). An unusual tandem kinase fusion protein confers leaf rust resistance in wheat. Res. Square 185, 2961–2974.e19. doi: 10.21203/rs.3.rs-1807889/v1
Wang, Y., Peng, H., Liu, G., Xie, C., Ni, Z., Yang, T., et al. (2010). Identification and molecular mapping of a leaf rust resistance gene in spelt wheat landrace altgold. Euphytica 174, 371–375. doi: 10.1007/s10681-010-0134-y
Wang, J., Zhang, Z. (2021). GAPIT version 3: Boosting power and accuracy for genomic association and prediction. Genomics Proteomics Bioinf. 19 (4), 629–640. doi: 10.1016/j.gpb.2021.08.005
Ward, B. P., Brown-Guedira, G., Kolb, F. L., Van Sanford, D. A., Tyagi, P., Sneller, C. H., et al. (2019). Genome-wide association studies for yield-related traits in soft red winter wheat grown in Virginia. PloS One 14, e0208217. doi: 10.1371/journal.pone.0208217
Wickham, H. (2016). ggplot2: Elegant graphics for data analysis (New York: Springer-Verlag). Available at: https://cran.r-project.org/web/packages/ggplot2/citation.html.
Yang, Y., Chai, Y., Zhang, X., Lu, S., Zhao, Z., Wei, D., et al. (2020). Multi-locus GWAS of quality traits in bread wheat: mining more candidate genes and possible regulatory network. Front. Plant Sci. 11. doi: 10.3389/fpls.2020.01091
Yan, X., Li, M., Zhang, P., Yin, G., Zhang, H., Gebrewahid, T. W., et al. (2021). High-temperature wheat leaf rust resistance gene Lr13 exhibits pleiotropic effects on hybrid necrosis. Mol. Plant 14, 1029–1032. doi: 10.1016/j.molp.2021.05.009
Yu, J., Pressoir, G., Briggs, W. H., Bi, I. V., Yamasaki, M., Doebley, J. F., et al. (2006). A unified mixed-model method for association mapping that accounts for multiple levels of relatedness. Nat. Genet. 38, 203–208. doi: 10.1038/ng1702
Zhang, D., Bowden, R. L., Yu, J., Carver, B. F., Bai, G. (2014). Association analysis of stem rust resistance in U.S. winter wheat. PloS One 9, e103747. doi: 10.1371/journal.pone.0103747
Zhang, C., Dong, S.-S., Xu, J.-Y., He, W.-M., Yang, T.-L. (2019a). PopLDdecay: a fast and effective tool for linkage disequilibrium decay analysis based on variant call format files. Bioinformatics 35, 1786–1788. doi: 10.1093/bioinformatics/bty875
Zhang, Y.-M., Jia, Z., Dunwell, J. M. (2019b). The application of new multi-locus GWAS methodologies in the genetic dissection of complex traits. Front. Plant Sci. 10. doi: 10.3389/fpls.2019.00100
Zhang, Q., Wei, W., Zuansun, X., Zhang, S., Wang, C., Liu, N., et al. (2021b). Fine mapping of the leaf rust resistance gene Lr65 in spelt wheat “Altgold”. Front. Plant Sci. 12. doi: 10.3389/fpls.2021.666921
Zhang, P., Yan, X., Gebrewahid, T. W., Zhou, Y., Yang, E., Xia, X., et al. (2021a). Genome-wide association mapping of leaf rust and stripe rust resistance in wheat accessions using the 90K SNP array. Theor. Appl. Genet. 134, 1233–1251. doi: 10.1007/s00122-021-03769-3
Zheng, X., Levine, D., Shen, J., Gogarten, S. M., Laurie, C., Weir, B. S. (2012). A high-performance computing toolset for relatedness and principal component analysis of SNP data. Bioinformatics 28, 3326–3328. doi: 10.1093/bioinformatics/bts606
Zhu, Z., Chen, L., Zhang, W., Yang, L., Zhu, W., Li, J., et al. (2020). Genome-wide association analysis of fusarium head blight resistance in Chinese elite wheat lines. Front. Plant Sci. 11. doi: 10.3389/fpls.2020.00206
Keywords: leaf rust, Puccinia triticana, GWAS - genome-wide association study, seedling resistance, wheat, QTL, SNP, marker assisted selection (MAS)
Citation: Kaur S, Gill HS, Breiland M, Kolmer JA, Gupta R, Sehgal SK and Gill U (2023) Identification of leaf rust resistance loci in a geographically diverse panel of wheat using genome-wide association analysis. Front. Plant Sci. 14:1090163. doi: 10.3389/fpls.2023.1090163
Received: 05 November 2022; Accepted: 16 January 2023;
Published: 01 February 2023.
Edited by:
Ahmed Amri, International Center for Agriculture Research in the Dry Areas (ICARDA), EthiopiahReviewed by:
Sukhwinder Singh, The International Maize and Wheat Improvement Center (CIMMYT), IndiaSivakumar Sukumaran, The University of Queensland, Australia
Copyright © 2023 Kaur, Gill, Breiland, Kolmer, Gupta, Sehgal and Gill. This is an open-access article distributed under the terms of the Creative Commons Attribution License (CC BY). The use, distribution or reproduction in other forums is permitted, provided the original author(s) and the copyright owner(s) are credited and that the original publication in this journal is cited, in accordance with accepted academic practice. No use, distribution or reproduction is permitted which does not comply with these terms.
*Correspondence: Upinder Gill, dXBpbmRlci5naWxsQG5kc3UuZWR1; Sunish K. Sehgal, c3VuaXNoLnNlaGdhbEBzZHN0YXRlLmVkdQ==
†These authors have contributed equally to this work