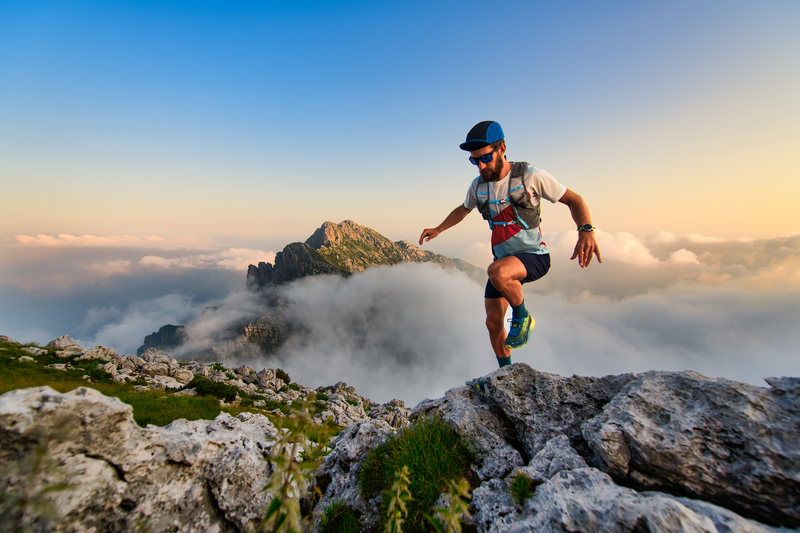
95% of researchers rate our articles as excellent or good
Learn more about the work of our research integrity team to safeguard the quality of each article we publish.
Find out more
ORIGINAL RESEARCH article
Front. Plant Sci. , 17 October 2022
Sec. Crop and Product Physiology
Volume 13 - 2022 | https://doi.org/10.3389/fpls.2022.996672
This article is part of the Research Topic Forage Crop Improvement for Improved Livestock Production and Nutrition View all 16 articles
Biomass yield and Feed Quality are the most important traits in alfalfa (Medicago sativa L.), which directly affect its economic value. Drought stress is one of the main limiting factors affecting alfalfa production worldwide. However, the genetic and especially the molecular mechanisms for drought tolerance in alfalfa are poorly understood. In this study, linkage mapping was performed in an F1 population by combining 12 phenotypic data (biomass yield, plant height, and 10 Feed Quality-related traits). A total of 48 significant QTLs were identified on the high-density genetic linkage maps that were constructed in our previous study. Among them, nine main QTLs, which explained more than 10% phenotypic variance, were detected for biomass yield (one), plant height (one), CP (two), ASH (one), P (two), K(one), and Mg (one). A total of 31 candidate genes were identified in the nine main QTL intervals based on the RNA-seq analysis under the drought condition. Blast-P was further performed to screen candidate genes controlling drought tolerance, and 22 functional protein candidates were finally identified. The results of the present study will be useful for improving drought tolerance of alfalfa varieties by marker-assisted selection (MAS), and provide promising candidates for further gene cloning and mechanism study.
Abiotic stresses, such as drought, cold, and salinity, are misfortunes for agriculture, which seriously limit crop productivity. In particular, drought is an increasing worldwide threat (Gupta et al., 2020). Economic losses in crop production were almost $30 billion in the past decade due to drought stress (Gupta et al., 2020). By 2050, water demand for agriculture could be double and economic losses in crop production due to water scarcity could be higher owing to climate change (Gleick, 2000; Tang, 2019; Gupta et al., 2020).
Alfalfa, the “Queen of Forages”, has a capacity to produce high yields and high-quality forage. The dry matter yield of alfalfa ranges from 12 to 19 t ha-1 (Nešić et al., 2005). On the other hand, alfalfa is a rich in protein, with over 20% crude protein content in alfalfa hay (Long et al., 2022). Furthermore, alfalfa is well recognized for its high concentration of macroelements (such as N, P, and Ca), microelements (such as Fe, Zn, Cu, and Mn), and vitamins (such as A, E, and K), all of which are beneficial to animal health (Radović et al., 2009). All those characteristics confirm that alfalfa has a superior value in feeding animals. However, alfalfa production and yield stability are severely affected by drought (Ashrafi et al., 2018; Ines et al., 2022). It has been reported that severe drought causes severe economic losses (Nadeem et al., 2019). Slama et al. (2011) studied eight cultivars of Medicago sativa and found that the biomass production was reduced by 55–75% for plants subjected to a water deficit condition (Slama et al., 2011). As a result, developing alfalfa cultivars with improved water use efficiency (WUE) and drought tolerance is critical for sustainable alfalfa production in water-limited areas.
Traditional breeding programs to develop new alfalfa varieties are time-consuming and costly. Incorporating marker-assisted selection (MAS) into breeding programs holds one of the promises to meet the demand for alfalfa production. Although, MAS has been widely used in several important crops, such as maize (Samayoa et al., 2019), wheat (Kumar et al., 2018a), and soybean (Kim et al., 2020). It is rarely employed for the commercial development of improved alfalfa varieties. Identifying and developing genetic loci robustly associated with alfalfa drought tolerance is the first step to developing drought resistant varieties by MAS. Quantitative trait loci (QTL) mapping and genome-wide association studies (GWAS) have been used to identify QTLs that influence complex quantitative traits, such as drought resistance. To date, some QTLs/SNPs for drought resistance have been detected by linkage or association mapping. For instance, Ray et al. (2015) identified 10 and 15 QTL associated with increased or reduced forage yield during drought stress in two backcross (BC1) mapping populations. Santantonio et al. (2019) performed linkage mapping of forage yield, WUE, carbon and nitrogen metabolism in the same populations under drought conditions (Santantonio et al., 2019). A diversity panel of 198 alfalfa accessions was used to identify SNPs associated with drought tolerance using association mapping (Zhang et al., 2015; Yu, 2017; Lin et al., 2020). In a greenhouse, Zhang et al. (2015) identified 19 and 15 loci associated with drought resistance index and relative leaf water content, respectively. In the field, SNPs associated with biomass yield and 26 Feed Quality-related traits under water deficit have been identified in the same panel (Yu, 2017; Lin et al., 2020).
Functional genes that contribute to drought tolerance were identified using homology-based cloning, including MsMYB2L (Song et al., 2019), MsZIP (Li et al., 2013), MsHSP17.7 (Li et al., 2016), MsZEP (Zhang et al., 2016), MsHSP70 (Li et al., 2017b), MsCML46 (Du et al., 2021), MsVDAC (Yang et al., 2021b), MsWRKY11 (Wen et al., 2021). However, little is known about genetic factors that contribute to drought tolerance in alfalfa. In the present study, we evaluated biomass yield, plant height, and 10 Feed Quality traits in an F1 mapping population under water deficit. Linkage mapping and RNA-sequencing (RNA-seq) analyses were performed to identify the QTLs associated with drought tolerance, and candidate genes by screening the differentially expressed genes (DEGs) within the QTL intervals. The detected QTLs are valuable resources for alfalfa genetic improvement by MAS, and further investigation of candidate genes can provide insights into genetic factors of alfalfa resistance to drought and other abiotic stresses.
The F1 population consisting of 150 progenies was used for evaluating and identifying loci associated with drought tolerance. Mapping population development, genotyping, and construction of genetic linkage maps were provided in our previous study (Jiang et al., 2022). Briefly, the mapping population was developed by crossing two tetraploid alfalfa plants, Cangzhou (CF000735, paternal parent, P1) and Zhongmu NO.1 (CF0032020, maternal parent, P2). The two parents vary in drought tolerance capacity with P1 better than P2. In greenhouse, both parents were imposed to water stress at 40% field capacity for 4 weeks, while control pots maintained 100% field capacity throughout the period of regrowth cycle. The biomass yield of plants under drought stress decreased significantly by 27.4% and 47.3%, as compared to control plants for P1 and P2, respectively. According to the method of Li et al. (2014), alleles segregating with a ratio of less than 2:1 were considered as single-dose allele (SDA) markers. A total of 7,252 and 7,404 high-quality SDA markers were obtained for P1 and P2 parents, respectively; which were then used to construct high-density linkage maps by JoinMap 4.0 software (Van Ooijen, 2006).
In 2016, cloned plants of the F1 population and two parents were transplanted to the field of the Chinese Academy of Agricultural Sciences Research Station at Langfang, Hebei Province (39.59°N, 116.59°E). The field experiment used a randomized complete block design with three replications, where one cloned plant of 150 progenies and two parents were planted in each replication. At the field site, the rainfall during the three alfalfa first growth cycles of 2018, 2019, and 2020 was approximately 24.7, 35.6, and 77.8mm, which was far less than the water requirement for normal growth of alfalfa (Cole et al., 1970; Dobrenz et al., 1971). Drought treatment was applied to the plants by withholding water before the first cut. Thus, three forage regrowth cycles (the first cut of 2018, 2019 and 2020) experienced significant water stress. In the early flowering stage, we measured biomass yield (BY), plant height (PH), and ten quality-related traits, including: the content of crude protein (CP), neutral detergent fiber (NDF), acid detergent fiber (ADF), lignin, dry matter (DM), ASH, K, Ca, Mg, and P. Plant height was the length of the longest stem, and biomass was the plant’s fresh weight when the stubble height is 4 ~ 5 cm. The BY and PH of individual plants were measured when the first flower appeared. The Feed Quality was measured using near-infrared reflectance spectroscopy (Foss NIRS 1650), and the details were described by Yang et al. (2021a). Statistical analysis of 12 traits was estimated by the R package psych. The best linear unbiased estimation (BLUE) and broad-sense heritability (H2) of the 12 traits were estimated by the ANOV function in the IciMapping software (Meng et al., 2015).
Combining BLUE values of each trait and the genetic linkage maps, QTL in response to water deficit were identified in the mapping population using the Inclusive Composite Interval Mapping with an additive effect (ICIM-ADD) in QTL IciMapping software (Meng et al., 2015). The QTL with a LOD value ≥ 3.0 was selected as significant QTL. QTLs were named as: q + phenotype + linkage group no., or qFT + linkage group no. + an ordered number to designate QTL in a single linkage group. For example, qBY6.4-1 indicates the first QTL associated with biomass yield under a water deficit condition in the Chr6.4 linkage group.
In brief, RNA-seq sequences were filtered using fastp (Chen et al., 2018), and mapped to the XingJiangDaYe reference genome (Chen et al., 2020b) using hisat2 (Kim et al., 2019). Samtools was used to generate and sort BAM files (Li et al., 2009). FeatureCounts v2.0.1 was used to generate read counts for each sample (Liao et al., 2014). The FPKM (Fragments Per Kilobase Million) was utilized to normalize and estimate gene expression values. The |log2 (FoldChange)| ≥ 2 and P ≤ 0.01 were used as thresholds to assess the significance of gene expression difference. TBtools was used to do a Gene Ontology (GO) enrichment analysis of the differentially expressed genes. (Chen et al., 2020a). Three RNA-sequencing datasets were used for the discovery of differentially expressed candidate genes associated with drought tolerance within the QTL region. The datasets were submitted to NCBI and signed for project numbers as follow: The first dataset, PRJNA525327 (https://www.ncbi.nlm.nih.gov/bioproject/PRJNA525327/), was an RNA-seq transcriptome profiling of two alfalfa genotypes (drought-sensitive and drought-resistant) root tissue under PEG-induced drought stress. The second one, PRJNA765383 (https://www.ncbi.nlm.nih.gov/bioproject/PRJNA765383/), was an RNA-seq of two genotypes (drought-sensitive and drought-resistant) of alfalfa leaf under drought conditions. The third RNA-seq project (PRJNA450305, https://www.ncbi.nlm.nih.gov/bioproject/PRJNA450305/) was alfalfa seedlings (Zhongmu No.1) treated with 400 mM mannitol under different treatment time points (0, 12, and 24 h).
The flanking markers of main QTLs, which explained more than 10% of the phenotypic variance, were used to obtain the physical location of QTLs on the XingJiangDaYe reference genome. The genes located on the flanking markers and within the physical intervals were extracted for further analysis. The first two RAN-seq datasets resulted in four comparative groups (G1~G4). The extracted genes, which were differentially expressed in two or more comparative groups, were used to further identify if they were differentially expressed in different treatment time points in the third RNA-seq project. If a candidate is identified by combining the information on linkage mapping and RNA-seq analysis, then, it will be annotated based on BLSAT search in Ensembl (https://ensembl.gramene.org/).
Quantitative real-time PCR (qRT-PCR) was performed to confirm whether the expression of candidate genes was induced by drought stress. Alfalfa seeds (Zhongmu No.1) were germinated in the MS medium. After seven days germination, the seedlings with similar growth were transferred to a hydroponic pot filled with 1/2 MS nutrient solution (pH = 5.8). Ten days after transfer, PEG-6000 (20%) was used to simulate drought treatment. Total RNA for each sample was isolated from three palnts under a time course of drought treatments (0, 1, 3, 6, 12, and 24 h). Using Ms-actin gene as the reference gene, qRT-PCR was implemented in triplicate for each treatment with the 7500 Real-Time PCR System (Applied Biosystem, CA, USA). The relative gene expression level was calculated by the 2-△△Ct method.
The BLUE values of the 12 traits including yield, plant height and 10 quality-related traits were used for statistical analysis. Two parents appeared to have substantial variations in these measured traits except for ADF and DM (Table S1). For example, the BY of the P2 parent was significantly higher than that of the P1 parent. A frequency distribution histogram based on the F1 population revealed a nearly normal distribution for 12 traits, indicating that the traits measured were quantitative traits (Figure S1). As shown in Figure S2, a positive correlation was observed between BY and PH in the F1 population with a correlation coefficient of 0.69 (Figure S2), indicating that the genotypes with higher PH tended to have higher yields. For quality related traits, CP was positively correlated with lignin (0.34, P < 0.001), Ca (0.48, P < 0.001), P (0.74, P < 0.001), and K (0.65, P < 0.001), while negatively correlated with Mg (-0.27, P < 0.01), ADF (−0.44, P < 0.001), and NDF (-0.37, P < 0.001). For lignin, it was positively correlated with ADF (0.55, P < 0.001) and NDF (0.57, P < 0.001) (Figure S2). The correlations we observed were consistent with those in the previous reports (Yang et al., 2021a).
The H2 of the 12 traits in the F1 population have a high variation ranging from 0.28 to 0.61 (Table S1). BY and PH had higher heritability of 0.50 and 0.61, respectively, suggesting that high proportion of the variability of these traits were contributed by genetic factor. Among the 10 Feed Quality-related traits, DM and ADF had the lowest heritability, at 0.28 and 0.32, respectively, indicating that a high proportion of the variability in these traits came from environmental factors, with a smaller contribution from genetic differences.
Under water deficit, BY, PH, and ten quality-related traits of the alfalfa population were evaluated during three consecutive years from 2018 to 2020. Using a threshold of LOD value higher than 3, a total of 48 QTLs were identified with phenotypic variance explanations ranging from 2.93% to 16.03% (Table 1). For two yield related traits, BY and PH, we identified 11 QTLs in two parents with six for BY and five for PH. We detected 37 QTLs related to ten quality traits with 8, 1, 3, 7, 1, 9, 3, and 5 for content of CP, lignin, NDF, ASH, Ca, P, K, and Mg, respectively, while, no QTL was detected for ADF and DM (Table 1).
Among these significant QTLs, nine showed more than 10% of the phenotypic variation explained (PVE) individually, with four in P1 and five in P2 (Table 1; Figure 1). PVE by four main QTLs, qPH7.3, qCP3.3, qASH5.4, and qP4.2, ranged from 10.70 to 16.03% in the P1 parent, with the highest PVE on qPH7.3 and the lowest PVE on qASH5.4 (Table 1; Figure 1A). In the P2 parent, five main QTLs of qBY6.4-2, qCP3.4, qP7.1-2, qK8.2, and qMg4.3 explained 10.13, 10.77, 10.72, 14.00, and 13.43% of the phenotypic variation, respectively (Table 1; Figure 1B). For BY, one main QTL, qBY6.4-2, was located on Chr6.4, while one PH-related main QTL, qPH7.3, was located on Chr7.3 with a LOD score of 7.03. For ten Feed Quality traits, seven main QTL were identified, including: two for CP, one for ash, two for P, one for K, and one for Mg. In contrast, no main QTL was detected for lignin, ADF, NDF, DM, and Ca (Table 1).
Figure 1 Main QTLs (PVE > 10%) detected in this study. (A, B) Main QTLs in the P1 and P2 parent, respectively.
Among the nine main QTLs, three QTLs from P1 and three from P2 were also independently identified in single environment (Table S2). They were considered as consistent QTLs. Specifically, qCP3.3, qCP3.4, and qK8.2 were also identified in 2019, 2020, and 2020, respectively. qBY6.4-2 and qPH7.3 were detected in two years. The last one, qP4.2, was identified in three grown environments (2018, 2019, and 2020) at a position of 81.5 ~ 82.5 cM.
In order to elucidate the drought-induced transcripts in alfalfa, we performed transcriptome analysis in leaf and root. Four groups (G1 ~ G4) were constructed by comparing the same genotype of root and leaf tissues under different conditions (control and drought) (Figure 2A; Tables S3 , S4). In root, the number of DEGs (drought vs. control) was approximately equal in the two comparative groups. There were 2,600 genes that were up-regulated in G1, while 2,671 genes were up-regulated in G2. Meanwhile, 5,524 and 4,897 down-regulated genes were identified in G1 and G2, respectively (Figure 2A). In contrast, compared to the 1,606 up- and 2,438 down-regulated DEGs identified in the G4, 5,357 up- and 4,109 down-regulated DEGs were identified in G3, indicating that the drought responsive transcriptomic program in leaf had bigger difference than those in root in different alfalfa genotypes (Figure 2A). In drought-resistant genotypes, drought condition upregulated 1,606 and 2,671 genes, and downregulated 2,438 and 4,897 genes in the leaf (G4) and root (G2) respectively. Not surprisingly, drought conditions resulted in more common DEGs in root, with 4,986 in root and 1,973 in leaf (Figure 2B).
Figure 2 Differentially expressed genes (DEGs) in root and leaf. (A), Number of upregulated genes (red) and downregulated genes (blue) in four comparative groups (G1 ~ G4). You can see the specific information of these four comparative groups in the Table S3. (B), Venn diagram of DEGs among root and leaf. (C, D), Bubble chart of GO enrichment analysis of the DEGs in root (C) and leaf (D). The ordinate represents different GO terms (biological progress) and the abscissa represents enrichment Score. Circle size represents the gene number while circle color represents the value of −log10 (P). The P value was corrected by Benjamini & Hochberg (BH) method.
Through GO enrichment analysis, the common DEGs in alfalfa root and leaf were then classified into the biological process (BP), cellular component (CC), and molecular function (MF) (Figures 2C, D; Tables S5 , S6). The common DEGs in root were enriched in the BP terms “response to acid chemical”, “monocarboxylic acid biosynthetic process”, “monocarboxylic acid biosynthetic process”, “oxoacid metabolic process”, “organic acid metabolic process”, “organic hydroxy compound biosynthetic process” and so on (Figure 2C; Table S5). These GO terms were also enriched in leaf (Table S6), indicating that these common genes were possibly involved in responding to drought stress. Subsequent GO enrichment analysis of 274 common DEGs in all four comparative groups resulted that these DEGs had a role in “response to water deprivation”, “response to osmotic stress” and “response to abscisic acid” and other responses (Figure 3, Table S7). Based on their functions, these genes likely play crucial roles in drought tolerance.
Linkage mapping revealed nine major QTL (PVE > 10%), which were selected as genetic regions to identify candidate genes responsive to drought tolerance. We first located their physical positions on the XingJiangDaYe reference genome. For instance, two physical intervals of qPH7.3 and qCP3.4 were less than 1Mb. We therefore screened candidate genes within 1cM upstream and downstream of these two QTL flanking markers. In this way, a total of 1,455 genes were extracted from the nine main QTL intervals (Table 2). Among them, three genes (MS.gene013861, MS.gene065379, and MS.gene034021) were located on flanking markers. chr4.3:85323781, the left flanking markers of qMg4.3, was lined with MS.gene034021, which was differentially expressed in G1 and G2 (Table S8).
To detect the candidate genes within the nine intervals, we combined the linkage mapping and RNA-seq analysis results. For G1, a total of 82 DEGs located in the nine main QTL regions, and the number of DEGs within each QTL ranged from 2 (qBY6.4-2/qPH7.3) to 24 (qCP3.3) (Table 2; Figure 4A). Also, 83, 80, and 37 DEGs located in the nine main QTL regions for G2, G3, and G4, respectively (Table 2; Figures 4A–D). Additionally, there were 52 common DEGs in roots (G1 and G2) and 22 common DEGs located in the nine QTL regions in leaves (G3 and G4) (Table 2; Figures 4E, F). Interestingly, the abovementioned DEGs in leaves had different expression patterns. For example, MS.gene08151 was down-regulated in drought condition in a drought-resistant genotype, while it was up-regulated in a drought-sensitive genotype (Figure 4F). However, the same phenomenon was not observed in root.
Figure 4 Combined transcriptome analysis and linkage mapping revealing the potential causal genes for drought response in alfalfa. (A–D) Distribution of DEGs within nine main QTL regions in four comparative groups, respectively. Green, blue, and red colors represent common DEGs among root, leaf, and four comparative groups, respectively. (E), Heatmap of 52 common DEGs among root. (F) Heatmap of 22 common DEGs among leaf.
To obtain more evidence to determine the reliable candidate genes, we further analysis the expression pattern of these genes in drought conditions at different time points (0h, 12h, and 24h). Among them, 31 candidates within QTL regions were differentially expressed at different time points (Figure S3). Notably, five candidate genes were differentially expressed across four comparative groups and at different treatment time, of which two (MS.gene068413 and MS.gene068423) were differentially expressed in qP4.2, two (MS.gene037560 and MS.gene037580) in qMg4.3, and one (MS.gene027542) in qASH5.4 (Table 2; Figures 4A–D).
Furthermore, functional annotation of the 31 identified genes revealed that 22 of them may be involved in drought stress regulation (Table 3). We investigated the expression level of all 22 candidate genes by qRT-PCR analysis, and found that all candidate genes were significantly induced under drought stress compared with untreated control (Figure 5). The results revealed that 13 candidate genes were consistent with the transcriptome results under drought stress with eight up-regulated genes (MS.gene012838, MS.gene38684, MS.gene012839, MS.gene068423, MS.gene068413, MS.gene037560, MS.gene037580, MS.gene067823) and five down-regulated genes (MS.gene73180, MS.gene33495, MS.gene33688, MS.gene33704, MS.gene97835) (Table S8; Figure 5). The expression patterns of the rest nine genes were different between the RNA-seq and qPT-PCR. For example, the expression level of MS.gene99452 was down-regulated under drought stress in RNA-seq (Table S8). However, it was up-regulated in qRT-PCR with the highest expression level at 6 h after drought treatment (Figure 5). Differences in expression patterns may be due to differences in drought treatment conditions and the inherent genetic variation between the materials used for different experiments. These candidate genes identified in our study should be considered as putative candidates, further investigation should be performed.
Analyzing the correlation coefficient in alfalfa forage yield and quality during drought stress can provide useful insight into phenotypic relationships between these traits in water-limited environments. To this end, we collected phenotypic data of two yield-related and 10 quality-related traits in the F1 mapping population for three years through drought-stressed growth cycle. P content was positively correlated with BY (0.34) and CP content (0.74), suggesting that applying P fertilizer may be beneficial to yield and CP content under drought conditions. For CP, it was negatively correlated (-0.37) with NDF, while the two traits had no significant correlation in a previous study (Yang et al., 2021a). Our results provided useful information to explore the relationship between alfalfa yield and quality during drought stress.
Achieving a high yield and high nutritional value is a main goal in alfalfa breeding. Forage yield and quality are easily influenced by the environment, such as drought stress. Previous studies suggested that biomass yield and Feed Quality under drought conditions may involve different mechanisms compared to non-stress conditions (Yu, 2017; Lin et al., 2020). It is therefore crucial to identify QTL associated with forage yield and quality under water deficit for genetic gain of alfalfa. In this study, we collected 12 traits with the heritability ranging from 0.28 to 0.61 and then performed linkage mapping. However, 48 QTL were detected for only ten traits. There were no QTL mapped for ADF and DM, probably due to the low heritability and no significant differences were observed between the two traits in the two parents. MAS has been one of the most efficient breeding methods due to the advantages of reducing the time and labor required in field tests (Collard and Mackill, 2008; Kumar et al., 2018b). Moreover, quantitative genetics theory predicted that the efficiency of MAS was inversely related to the heritability of the traits (Knapp, 1998; Oladosu et al., 2019). Water scarcity tolerance was a complex trait controlled by polygenes with low to medium heritability (Elena, 2022). Hence, utilizing the QTLs identified in this study, particularly those quality related QTLs of low to moderate heritability, can significantly reduce the time and resources required for breeding efforts.
Transcriptome analysis is an effective method for the identification of differentially expressed stress-responsive genes (Sathik et al., 2018; Liu et al., 2020). Ascertaining the DEGs that change upon water stress in alfalfa is of crucial in understanding the genetic base of drought tolerance. In alfalfa, although drought-responsive DEGs have been reported in previous studies (Ma et al., 2021; Singer et al., 2021), there were some limitations due to the lack of a reference genome. The release of a high-quality alfalfa reference genome has given new power to transcriptional analysis (Chen et al., 2020b). In this study, we aligned the sequencing data to the XingJiangDaYe reference genome and finally obtained a total of 274 DEGs through root and leaf. Through GO enrichment analysis, the most significant enrichment terms were “arabinan catabolic process” and “arabinan metabolic process”, which played a role in maintaining the flexibility of the plant cell wall during water deficit (Moore et al., 2008a; Moore et al., 2008b). In addition, terms related to cell wall biosynthesis such as “xylan catabolic process”, “hemicellulose metabolic process”, and “xylan metabolic process” were also enriched in these DEGs, indicating that changes in the expression of cell wall-related genes were a vital and integral component of alfalfa’s response to water stress. Understanding how the wall adapts to loss of water should provide new insight into crop improvement (Lenk et al., 2019; Ganie and Ahammed, 2021).
Alfalfa is a self-incompatible plant, and the traditional QTL fine mapping and map-based cloning are limited by constructing near-isogenic lines. It has been reported that integration of conventional linkage mapping and RNA-seq can rapidly identify candidates associated with complex traits of interest to replace the fine-mapping process (Park et al., 2019; Derakhshani et al., 2020). In this study, we finally identified 22 drought responsive genes by integrating QTL and DEGs. Plants have evolved complex networks of drought stress response. Abscisic acid (ABA) regulates a number of physiological responses in drought-stressed plants, ensuring a balance of optimal development and stress tolerance (Soma et al., 2021). MS.gene38710 was annotated as an ABA-responsive element binding factor that can be phosphorylated by upstream genes to regulate the expression of drought-responsive genes (Feng et al., 2019; Zhang et al., 2020). In plants, the phosphatidylethanolamine-binding protein (PEBP) family has been identified to have a crucial role in the regulation of plant growth and developmental processes. Meanwhile, omics data suggested that the PEBP family was also involved in the drought stress response (Manoharan et al., 2016; Li et al., 2017a; Schneider et al., 2019). OsMFT1, a member of the PEBP family, has been shown to improve rice drought tolerance by interacting with two key drought-related transcription factors, OsbZIP66 and OsMYB26 (Chen et al., 2021). In our study, three PEBP family members (MS.gene068423, MS.gene068413, and MS.gene037560) were identified in two main QTLs, suggesting that they may play an important role in alfalfa drought response. In addition, some differential genes located in the main QTL interval should also be focused on in future research. For example, MS.gene034021 was annotated as Zein-binding protein, which has not been reported to be related to plant drought resistance. However, it was differentially expressed in G1 and G2 with the high log2FoldChange value of -7.40 and -8.36, respectively. Further molecular investigation is needed to illustrate the function of these genes in drought response.
In the present study, nine main QTLs associated with biomass yield, plant height, and the content of CP, ASH, K, Mg, and P under water deficit condition were identified in the F1 population. The integration of linkage mapping with RNA-seq analysis under water stress, 22 DEGs, excavated from QTL-regions, were identified as potential candidates. The closely linked markers and candidate genes identified in the present study will provide a tool for the MAS breeding program, and new insight for further revealing the molecular mechanism of drought tolerance in alfalfa.
RNA-seq raw data used in this study was downloaded from the SRA database in NCBI with the Bioproject accession numbers of PRJNA525327, PRJNA765383, and PRJNA450305.
QY, JK and ZW conceived and designed the experiments and developed the mapping population. XJ, TY, CW, and Ting Gao collected phenotypic data. XJ, FZ, and AY performed data analysis. XJ and AY wrote the manuscript. L-XY, ZW, and JK revised and finalized the manuscript. All authors contributed to the article and approved the submitted version.
This work was supported by the National Natural Science Foundation of China (32071868) and the earmarked fund for China Agriculture Research System (CARS-35-04) and the key research project of Ningxia province for alfalfa breeding program (2019NYY203) and Agricultural Science and Technology Innovation Program (ASTIP-IAS14).
The authors declare that the research was conducted in the absence of any commercial or financial relationships that could be construed as a potential conflict of interest.
All claims expressed in this article are solely those of the authors and do not necessarily represent those of their affiliated organizations, or those of the publisher, the editors and the reviewers. Any product that may be evaluated in this article, or claim that may be made by its manufacturer, is not guaranteed or endorsed by the publisher.
The Supplementary Material for this article can be found online at: https://www.frontiersin.org/articles/10.3389/fpls.2022.996672/full#supplementary-material
Supplementary Figure 1 | Frequency distributions of best linear unbiased estimation (BLUE) values of 12 phenotypic data in the F1 population.
Supplementary Figure 2 | Phenotypic correlations of 12 traits in F1 population. Numbers indicate the magnitude of the correlation coefficient. Negatively correlated variables are red, whereas positively correlated variables are blue, with the intensity depending on the magnitude of the correlation. Asterisks indicate the significance level, *P < 0.05, **P < 0.01, ***P < 0.001.
Supplementary Figure 3 | Hierarchical clustering analysis of DEGs in 0h, 6h, and 24h after drought stress. Red, blue and white elements in the matrix indicate up-regulated, down-regulated, and no change genes, respectively.
Supplementary Table 1 | Statistical analysis of 12 traits (BLUE values) in two parents and F1 population.
Supplementary Table 2 | Consistent QTLs detected in this study. The main QTLs, which were independently identified in a single environment, were considered as consistent QTLs.
Supplementary Table 3 | Information of four comparative groups (G1-G4).
Supplementary Table 4 | The list of differentially expressed genes (DEGs) in four comparative groups. The DEGs threshold was set at P ≤ 0.01 and |log2 fold change (FC)| > 2.
Supplementary Table 5 | GO enrichment analysis of the 4,986 common DEGs in root. The 4,986 DEGs were identified in both G1 and G2.
Supplementary Table 6 | GO enrichment analysis of the 1,973 common DEGs in leaf. The 1,973 DEGs were identified in both G3 and G4.
Supplementary Table 7 | GO enrichment analysis of the 274 common DEGs among root and leaf. The 274 DEGs were identified in all four comparative groups.
Supplementary Table 8 | List of the position information, the log2FoldChange value, and the annotation information of 1,455 genes within nine main QTL regions.
Ashrafi, M., Azimi-Moqadam, M.-R., Moradi, P., MohseniFard, E., Shekari, F., Kompany-Zareh, M. (2018). Effect of drought stress on metabolite adjustments in drought tolerant and sensitive thyme. Plant Physiol. Biochem. 132, 391–399. doi: 10.1016/j.plaphy.2018.09.009
Chen, C., Chen, H., Zhang, Y., Thomas, H. R., Frank, M. H., He, Y., et al. (2020a). TBtools: An integrative toolkit developed for interactive analyses of big biological data. Mol. Plant 13, 1194–1202. doi: 10.1016/j.molp.2020.06.009
Chen, Y., Shen, J., Zhang, L., Qi, H., Yang, L., Wang, H., et al. (2021). Nuclear translocation of OsMFT1 that is impeded by OsFTIP1 promotes drought tolerance in rice. Mol. Plant 14, 1297–1311. doi: 10.1016/j.molp.2021.05.001
Chen, H., Zeng, Y., Yang, Y., Huang, L., Tang, B., Zhang, H., et al. (2020b). Allele-aware chromosome-level genome assembly and efficient transgene-free genome editing for the autotetraploid cultivated alfalfa. Nat. Commun. 11, 2494. doi: 10.1038/s41467-020-16338-x
Chen, S., Zhou, Y., Chen, Y., Gu, J. (2018). Fastp: an ultra-fast all-in-one FASTQ preprocessor. Bioinformatics 34, i884–i890. doi: 10.1093/bioinformatics/bty560
Cole, D., Dobrenz, A., Massengale, M., Wright, L. N. (1970). Water requirement and its association with growth components and protein content of alfalfa (Medicago sativa l.) 1. Crop Sci. 10, 237–240. doi: 10.2135/cropsci1970.0011183X001000030008x
Collard, B. C., Mackill, D. J. (2008). Marker-assisted selection: an approach for precision plant breeding in the twenty-first century. Philos. Trans. R. Soc. B: Biol. Sci. 363, 557–572. doi: 10.1098/rstb.2007.2170
Derakhshani, B., Jafary, H., Maleki Zanjani, B., Hasanpur, K., Mishina, K., Tanaka, T., et al. (2020). Combined QTL mapping and RNA-seq profiling reveals candidate genes associated with cadmium tolerance in barley. PloS One 15, e0230820. doi: 10.1371/journal.pone.0230820
Dobrenz, A., Cole, D., Massengale, M. (1971). Yield components and leaf characteristics associated with the water requirement of alfalfa 1. Crop Sci. 11, 124–125. doi: 10.2135/cropsci1971.0011183X001100010045x
Du, B., Chen, N., Song, L., Wang, D., Cai, H., Yao, L., et al. (2021). Alfalfa (Medicago sativa l.) MsCML46 gene encoding calmodulin-like protein confers tolerance to abiotic stress in tobacco. Plant Cell Rep. 40, 1907–1922. doi: 10.1007/s00299-021-02757-7
Elena, B. (2022). Improving drought tolerance: Can comparative transcriptomics support strategic rice breeding? Plant Stress 3, 100058. doi: 10.1016/j.stress.2022.100058
Feng, R. J., Ren, M. Y., Lu, L. F., Peng, M., Guan, X., Zhou, D. B., et al. (2019). Involvement of abscisic acid-responsive element-binding factors in cassava (Manihot esculenta) dehydration stress response. Sci. Rep. 9, 12661. doi: 10.1038/s41598-019-49083-3
Ganie, S. A., Ahammed, G. J. (2021). Dynamics of cell wall structure and related genomic resources for drought tolerance in rice. Plant Cell Rep. 40, 437–459. doi: 10.1007/s00299-020-02649-2
Gleick, P. H. (2000). The world's water 2000-2001: the biennial report on freshwater resources (Washington, DC: Island Press).
Gupta, A., Rico-Medina, A., Caño-Delgado, A. I. (2020). The physiology of plant responses to drought. Science 368, 266–269. doi: 10.1126/science.aaz7614
Ines, S., Talbi, O., Nasreddine, Y., Rouached, A., Gharred, J., Jdey, A., et al. (2022). Drought tolerance traits in medicago species: A review. Arid Land Res. Manage. 36, 67–83. doi: 10.1080/15324982.2021.1936289
Jiang, X., Yang, T., Zhang, F., Yang, X., Yang, C., He, F., et al. (2022). RAD-Seq-Based high-density linkage maps construction and quantitative trait loci mapping of flowering time trait in alfalfa (Medicago sativa l.). Front. Plant Sci. 13, 899681. doi: 10.3389/fpls.2022.899681
Kim, J.-M., Kim, K.-H., Jung, J., Kang, B. K., Lee, J., Ha, B.-K., et al. (2020). Validation of marker-assisted selection in soybean breeding program for pod shattering resistance. Euphytica 216, 1–9. doi: 10.1007/s10681-020-02703-w
Kim, D., Paggi, J. M., Park, C., Bennett, C., Salzberg, S. L. (2019). Graph-based genome alignment and genotyping with HISAT2 and HISAT-genotype. Nat. Biotechnol. 37, 907–915. doi: 10.1038/s41587-019-0201-4
Knapp, S. J. (1998). Marker-assisted selection as a strategy for increasing the probability of selecting superior genotypes. Crop Sci. 38, 1164–1174. doi: 10.2135/cropsci1998.0011183X003800050009x
Kumar, A., Jain, S., Elias, E. M., Ibrahim, M., Sharma, L. K. (2018a). “An overview of QTL identification and marker-assisted selection for grain protein content in wheat,” in Eco-friendly agro-biological techniques for enhancing crop productivity, 245–274. (Singapore: Springer). doi: 10.1007/978-981-10-6934-5_11
Kumar, A., Sandhu, N., Dixit, S., Yadav, S., Swamy, B., Shamsudin, N. A. A. (2018b). Marker-assisted selection strategy to pyramid two or more QTLs for quantitative trait-grain yield under drought. Rice 11, 1–16. doi: 10.1186/s12284-018-0227-0
Lenk, I., Fisher, L. H., Vickers, M., Akinyemi, A., Didion, T., Swain, M., et al. (2019). Transcriptional and metabolomic analyses indicate that cell wall properties are associated with drought tolerance in brachypodium distachyon. Int. J. Mol. Sci. 20, 1758. doi: 10.3390/ijms20071758
Liao, Y., Smyth, G. K., Shi, W. (2014). featureCounts: an efficient general purpose program for assigning sequence reads to genomic features. Bioinformatics 30, 923–930. doi: 10.1093/bioinformatics/btt656
Li, H., Handsaker, B., Wysoker, A., Fennell, T., Ruan, J., Homer, N., et al. (2009). The sequence alignment/map format and SAMtools. Bioinformatics 25, 2078–2079. doi: 10.1093/bioinformatics/btp352
Li, J. X., Hou, X. J., Zhu, J., Zhou, J. J., Huang, H. B., Yue, J. Q., et al. (2017a). Identification of genes associated with lemon floral transition and flower development during floral inductive water deficits: A hypothetical model. Front. Plant Sci. 8, 1013. doi: 10.3389/fpls.2017.01013
Li, Z., Long, R., Zhang, T., Wang, Z., Zhang, F., Yang, Q., et al. (2017b). Molecular cloning and functional analysis of the drought tolerance gene MsHSP70 from alfalfa (Medicago sativa l.). J. Plant Res. 130, 387–396. doi: 10.1007/s10265-017-0905-9
Li, Z.-y., Long, R.-c., Zhang, T.-j., Yang, Q.-c., Kang, J.-m. (2016). Molecular cloning and characterization of the MsHSP17. 7 gene from Medicago sativa l. Mol. Biol. Rep. 43, 815–826. doi: 10.1007/s11033-016-4008-9
Li, X., Wei, Y., Acharya, A., Jiang, Q., Kang, J., Brummer, E. C. (2014). A saturated genetic linkage map of autotetraploid alfalfa (Medicago sativa l.) developed using genotyping-by-Sequencing is highly syntenous with the medicago truncatula genome. G3 Genesgenetics 4, 1971–1979. doi: 10.1534/g3.114.012245
Lin, S., Medina, C. A., Boge, B., Hu, J., Fransen, S., Norberg, S., et al. (2020). Identification of genetic loci associated with forage quality in response to water deficit in autotetraploid alfalfa (Medicago sativa l.). BMC Plant Biol. 20, 303. doi: 10.1186/s12870-020-02520-2
Li, Y., Sun, Y., Yang, Q., Fang, F., Kang, J., Zhang, T. (2013). Isolation and characterization of a gene from Medicago sativa l., encoding a bZIP transcription factor. Mol. Biol. Rep. 40, 1227–1239. doi: 10.1007/s11033-012-2165-z
Liu, J., Deng, J. L., Tian, Y. (2020). Transcriptome sequencing of the apricot (Prunus armeniaca l.) and identification of differentially expressed genes involved in drought stress. Phytochemistry 171, 112226. doi: 10.1016/j.phytochem.2019.112226
Long, R., Zhang, F., Zhang, Z., Li, M., Chen, L., Wang, X., et al. (2022). Genome assembly of alfalfa cultivar zhongmu-4 and identification of SNPs associated with agronomic traits. Genomics Proteomics Bioinf 20, 14–28. doi: 10.1016/j.gpb.2022.01.002
Manoharan, R. K., Han, J. S., Vijayakumar, H., Subramani, B., Thamilarasan, S. K., Park, J. I., et al. (2016). Molecular and functional characterization of FLOWERING LOCUS T homologs in allium cepa. Molecules 21, 217. doi: 10.3390/molecules21020217
Ma, Q., Xu, X., Wang, W., Zhao, L., Ma, D., Xie, Y. J. P. P. (2021). Comparative analysis of alfalfa (Medicago sativa l.) seedling transcriptomes reveals genotype-specific drought tolerance mechanisms. Plant Physiol. Biochem. 166, 203–214. doi: 10.1016/j.plaphy.2021.05.008
Meng, L., Li, H., Zhang, L., Wang, J. (2015). QTL IciMapping: Integrated software for genetic linkage map construction and quantitative trait locus mapping in biparental populations. Crop J. 3, 269–283. doi: 10.1016/j.cj.2015.01.001
Moore, J. P., Farrant, J. M., Driouich, A. (2008a). A role for pectin-associated arabinans in maintaining the flexibility of the plant cell wall during water deficit stress. Plant Signaling Behav. 3, 102–104. doi: 10.4161/psb.3.2.4959
Moore, J. P., Vicré-Gibouin, M., Farrant, J. M., Driouich, A. (2008b). Adaptations of higher plant cell walls to water loss: drought vs desiccation. Physiologia Plantarum 134, 237–245. doi: 10.1111/j.1399-3054.2008.01134.x
Nadeem, M., Li, J., Yahya, M., Sher, A., Ma, C., Wang, X., et al. (2019). Research progress and perspective on drought stress in legumes: a review. Int. J. Mol. Sci. 20, 2541. doi: 10.3390/ijms20102541
Nešić, Z., Tomić, Z., Žujović, M., Krnjaja, V. (2005). Production characteristics of domestic alfalfa (Medicago sativa l.) cultivars in agroecological conditions of srem district. Biotechnol. Anim. Husbandry 21, 169–174. doi: 10.2298/BAH0502169N
Oladosu, Y., Rafii, M. Y., Samuel, C., Fatai, A., Magaji, U., Kareem, I., et al. (2019). Drought resistance in rice from conventional to molecular breeding: A review. Int. J. Mol. Sci. 20, 3519. doi: 10.3390/ijms20143519
Park, M., Lee, J.-H., Han, K., Jang, S., Han, J., Lim, J.-H., et al. (2019). A major QTL and candidate genes for capsaicinoid biosynthesis in the pericarp of capsicum chinense revealed using QTL-seq and RNA-seq. Theor. Appl. Genet. 132, 515–529. doi: 10.1007/s00122-018-3238-8
Radović, J., Sokolović, D., Marković, J. (2009). ALFALFA-MOST IMPORTANT PERENNIAL FORAGE LEGUME IN ANIMAL HUSBANDRY. Biotechnol. Anim. Husbandry 25, 465–475. doi: 10.2298/BAH0906465R
Ray, I. M., Han Y, E. L., Meenach, C. D., Santantonio, N., Sledge, M. K., Pierce, C. A., et al. (2015). Identification of quantitative trait loci for alfalfa forage biomass productivity during drought stress. Crop Sci. 55, 2012–2033. doi: 10.2135/cropsci2014.12.0840
Samayoa, L. F., Butrón, A., Malvar, R. A. (2019). Usefulness of marker-assisted selection to improve maize for increased resistance to sesamia nonagrioides attack with no detrimental effect on yield. Ann. Appl. Biol. 174, 219–222. doi: 10.1111/aab.12480
Santantonio, N., Pierce, C. A., Steiner, R. L., Ray, I. M. (2019). Genetic mapping of water use efficiency and carbon and nitrogen metabolism in drought-stressed alfalfa. Crop Sci. 59, 92–106. doi: 10.2135/cropsci2018.05.0307
Sathik, M., Luke, L. P., Rajamani, A., Kuruvilla, L., Sumesh, K., Thomas, M. (2018). De novo transcriptome analysis of abiotic stress-responsive transcripts of hevea brasiliensis. Mol. Breed. 38, 1–17. doi: 10.1007/s11032-018-0782-5
Schneider, S., Turetschek, R., Wedeking, R., Wimmer, M. A., Wienkoop, S. (2019). A protein-linger strategy keeps the plant on-hold after rehydration of drought-stressed beta vulgaris. Front. Plant Sci. 10, 381. doi: 10.3389/fpls.2019.00381
Singer, S. D., Subedi, U., Lehmann, M., Burton Hughes, K., Feyissa, B. A., Hannoufa, A., et al. (2021). Identification of differential drought response mechanisms in medicago sativa subsp. sativa and falcata through comparative assessments at the physiological, biochemical, and transcriptional levels. Plants 10, 2107. doi: 10.3390/plants10102107
Slama, I., Tayachi, S., Jdey, A., Rouached, A., Abdelly, C. (2011). Differential response to water deficit stress in alfalfa (Medicago sativa) cultivars: Growth, water relations, osmolyte accumulation and lipid peroxidation. Afr. J. Biotechnol. 10, 16250–16259. doi: 10.5897/AJB11.1202
Soma, F., Takahashi, F., Yamaguchi-Shinozaki, K., Shinozaki, K. (2021). Cellular phosphorylation signaling and gene expression in drought stress responses: ABA-dependent and ABA-independent regulatory systems. Plants (Basel Switzerland) 10, 756. doi: 10.3390/plants10040756
Song, Y., Lv, J., Qiu, N., Bai, Y., Yang, N., Dong, W. (2019). The constitutive expression of alfalfa MsMYB2L enhances salinity and drought tolerance of arabidopsis thaliana. Plant Physiol. Biochem. 141, 300–305. doi: 10.1016/j.plaphy.2019.06.007
Tang, QJSCES (2019). Global change hydrology: Terrestrial water cycle and global change. Science China. Earth Sciences 63, 459–462. doi: 10.1007/s11430-019-9559-9
Van Ooijen, J. (2006). JoinMap® 4, software for the calculation of genetic linkage maps in experimental populations Vol. 33 (Wageningen: Kyazma BV).
Wen, W., Wang, R., Su, L., Lv, A., Zhou, P., An, Y. (2021). MsWRKY11, activated by MsWRKY22, functions in drought tolerance and modulates lignin biosynthesis in alfalfa (Medicago sativa l.). Environ. Exp. Bot. 184, 104373. doi: 10.1016/j.envexpbot.2021.104373
Yang, C., Zhang, F., Jiang, X., Yang, X., He, F., Wang, Z., et al. (2021a). Identification of genetic loci associated with crude protein content and fiber composition in alfalfa (Medicago sativa l.) using QTL mapping. Front. Plant Sci. 12. doi: 10.3389/fpls.2021.608940
Yang, M., Duan, X., Wang, Z., Yin, H., Zang, J., Zhu, K., et al. (2021b). Overexpression of a voltage-dependent anion-selective channel (VDAC) protein-encoding gene, MsVDAC, from Medicago sativa confers cold and drought tolerance to transgenic tobacco. Genes 12, 1706. doi: 10.3390/genes12111706
Yu, L. X. (2017). Identification of single-nucleotide polymorphic loci associated with biomass yield under water deficit in alfalfa (Medicago sativa l.) using genome-wide sequencing and association mapping. Front. Plant Sci. 8, 1152. doi: 10.3389/fpls.2017.01152
Zhang, H., Liu, D., Yang, B., Liu, W. Z., Mu, B., Song, H., et al. (2020). Arabidopsis CPK6 positively regulates ABA signaling and drought tolerance through phosphorylating ABA-responsive element-binding factors. J. Exp. Bot. 71, 188–203. doi: 10.1093/jxb/erz432
Zhang, Z., Wang, Y., Chang, L., Zhang, T., An, J., Liu, Y., et al. (2016). MsZEP, a novel zeaxanthin epoxidase gene from alfalfa (Medicago sativa), confers drought and salt tolerance in transgenic tobacco. Plant Cell Rep. 35, 439–453. doi: 10.1007/s00299-015-1895-5
Zhang, T., Yu, L. X., Zheng, P., Li, Y., Rivera, M., Main, D., et al. (2015). Identification of loci associated with drought resistance traits in heterozygous autotetraploid alfalfa (Medicago sativa l.) using genome-wide association studies with genotyping by sequencing. PloS One 10, e0138931. doi: 10.1371/journal.pone.0138931
Keywords: alfalfa, biomass yield, Feed Quality, drought, linkage mapping, RNA-Seq
Citation: Jiang X, Yu A, Zhang F, Yang T, Wang C, Gao T, Yang Q, Yu L-X, Wang Z and Kang J (2022) Identification of QTL and candidate genes associated with biomass yield and Feed Quality in response to water deficit in alfalfa (Medicago sativa L.) using linkage mapping and RNA-Seq. Front. Plant Sci. 13:996672. doi: 10.3389/fpls.2022.996672
Received: 18 July 2022; Accepted: 26 September 2022;
Published: 17 October 2022.
Edited by:
Jorge Fernando Pereira, Embrapa Gado de Leite, BrazilReviewed by:
Meki Muktar, International Livestock Research Institute, EthiopiaCopyright © 2022 Jiang, Yu, Zhang, Yang, Wang, Gao, Yang, Yu, Wang and Kang. This is an open-access article distributed under the terms of the Creative Commons Attribution License (CC BY). The use, distribution or reproduction in other forums is permitted, provided the original author(s) and the copyright owner(s) are credited and that the original publication in this journal is cited, in accordance with accepted academic practice. No use, distribution or reproduction is permitted which does not comply with these terms.
*Correspondence: Junmei Kang, a2FuZ2p1bm1laUBjYWFzLmNu; Zhen Wang, d2FuZ3poZW5AY2Fhcy5jbg==
†These authors have contributed equally to this work
Disclaimer: All claims expressed in this article are solely those of the authors and do not necessarily represent those of their affiliated organizations, or those of the publisher, the editors and the reviewers. Any product that may be evaluated in this article or claim that may be made by its manufacturer is not guaranteed or endorsed by the publisher.
Research integrity at Frontiers
Learn more about the work of our research integrity team to safeguard the quality of each article we publish.