Corrigendum: Integrated analysis of small RNAs, transcriptome and degradome sequencing reveal the drought stress network in Agropyron mongolicum Keng
- 1Agricultural College, Inner Mongolia Agricultural University, Hohhot, China
- 2Inner Mongolia Academy of Agricultural & Animal Husbandry Sciences, Hohhot, China
- 3College of Grassland, Resources and Environment, Inner Mongolia Agricultural University, Hohhot, China
Agropyron mongolicum (A. mongolicum) is an excellent gramineous forage with extreme drought tolerance, which lives in arid and semiarid desert areas. However, the mechanism that underlies the response of microRNAs (miRNAs) and their targets in A. mongolicum to drought stress is not well understood. In this study, we analyzed the transcriptome, small RNAome (specifically the miRNAome) and degradome to generate a comprehensive resource that focused on identifying key regulatory miRNA-target circuits under drought stress. The most extended transcript in each collection is known as the UniGene, and a total of 41,792 UniGenes and 1,104 miRNAs were identified, and 99 differentially expressed miRNAs negatively regulated 1,474 differentially expressed target genes. Among them, eight miRNAs were unique to A. mongolicum, and there were 36 target genes. A weighted gene co-expression network analysis identified five hub genes. The miRNAs of five hub genes were screened with an integration analysis of the degradome and sRNAs, such as osa-miR444a-3p.2-MADS47, bdi-miR408-5p_1ss19TA-CCX1, tae-miR9774_L-2R-1_1ss11GT-carC, ata-miR169a-3p-PAO2, and bdi-miR528-p3_2ss15TG20CA-HOX24. The functional annotations revealed that they were involved in mediating the brassinosteroid signal pathway, transporting and exchanging sodium and potassium ions and regulating the oxidation–reduction process, hydrolase activity, plant response to water deprivation, abscisic acid (ABA) and the ABA-activated signaling pathway to regulate drought stress. Five hub genes were discovered, which could play central roles in the regulation of drought-responsive genes. These results show that the combined analysis of miRNA, the transcriptome and degradation group provides a useful platform to investigate the molecular mechanism of drought resistance in A. mongolicum and could provide new insights into the genetic engineering of Poaceae crops in the future.
Introduction
Global warming increases the environmental stress on crops, making it difficult to fully utilize their genetic potential (Boyer, 1982). The total yield of principal crops decreases by approximately 70% each year owing to ecological pressures (Shubha and Tyagi, 2010; Zurbriggen et al., 2010). Among all the ecological pressures, the impact and restriction of drought on agriculture are particularly prominent (Esfahanian et al., 2017). However, plants that grow in some barren and arid environments have evolved highly effective self-regulatory systems to manage drought. Plants of the Agropyron genus are significant pasture resources that have adapted to drought, low temperature and salinity and play essential roles in ecological restoration (Che and Li, 2007; Han et al., 2017). Agropyron mongolicum (Poaceae, 2n = 2x = 14), a representative member of Agropyron, is an excellent perennial grazing grass in the arid steppe, which has soft stems and leaves, a highly developed root system, early greening, strong tillering ability, good palatability, adaptability, and is known for its strong drought resistance (Zhao et al., 2010b; Zhang et al., 2019). It can be used for pasture and ecological restoration in cold and arid regions and provides superior gene resources to breed drought resistance and improve forage and wheat (Triticum aestivum) crops (Du et al., 2017a). However, the molecular drought resistance mechanism of A. mongolicum is still in its infancy and merits more attention and urgent research work.
Drought can cause physiological and morphological changes in plants, which negatively affect their growth and productivity (Chandra et al., 2021). MicroRNAs (miRNAs) play crucial roles in plant-environment interactions (Niu et al., 2016; Song et al., 2019). miRNAs range from 18 to 25 nucleotides (nt) and are a class of endogenous small non-coding RNAs (Carrington and Ambros, 2003; Song et al., 2019). miRBase (miRbase V22.1), a professional research database of miRNA, contains 38,589 mature miRNAs of 271 species, which include 9,168 mature miRNAs of 74 dicotyledonous and monocotyledonous plants. On the basis of sequence complementarity, miRNAs directly target mRNAs to cleave or translationally repress them, thus, completing the regulation of plant function (Frank et al., 2000; Flynt and Lai, 2008). Currently, miRNAs involved in abiotic stress (drought, salinity and temperature) responses have been reported in Arabidopsis thaliana, tobacco (Nicotiana tabacum), rice (Oryza sativa) and other plants. They include miR156, miR159, miR169, miR395 (Ali et al., 2017), miR444 (Jiao et al., 2020; Kannan et al., 2022), miR408 (Hajyzadeh et al., 2015; Taier et al., 2021), and miR528 (Chen et al., 2021; Wang et al., 2021a). miRNAs regulate transcription factors, plant hormones, antioxidant systems and other functional target genes, such as MYB33, OsNAC2, ARF22, DREB, NtCAT1, ARF, TIR1, and OsMADS27 (Reyes and Nam-Hai, 2007; David and Franck, 2011; Hajyzadeh et al., 2015; Yin et al., 2015; Jiang et al., 2018; Qiu et al., 2018; Shi et al., 2018; Kannan et al., 2022) to respond to abiotic stress.
miRNA-led stress regulatory networks are considered novel tools for the development of abiotic stress tolerance in crops (Ali et al., 2017). The integrated miRNAs-target genes interaction network can intuitively reflect the relationship between genes at the global level. The hub genes are the core regulatory genes in the network and play a crucial role in the stability of network. The integrated analysis of miRNA and target mRNAs has been widely used in human medicine, animal growth and development, gene expression regulatory mechanisms and other fields, but research reports on its use in plants are limited. The general workflow for constructing microRNA-mediated gene regulatory network was introduced (Meng et al., 2011). The regulatory network of auxin, miR390, TAS3 and ARFs on the root growth of Arabidopsis thaliana (A. thaliana) was analyzed (Elena et al., 2010). Floral transcriptomes in woodland strawberries (Fragaria vesca) uncovered developing receptacles and anther gene networks and identified the hub genes FveLOM and FveWUS1 (Hollender et al., 2014). A cadmium phytoremediation miRNA-target mRNA network was constructed in hyperaccumulating Sedum alfredia, and the hub genes AAP3 and ARF4 may play a key regulatory role (Han et al., 2016). Two cold-resistant modules of peanut (Arachis hypogeae) were found by a weighted gene co-expression network analysis (WGCNA). They obtained the hub genes involved in soluble sugar, polyamine and the G-lignin biosynthetic pathway at low temperatures (Wang et al., 2021c). A total of 13 hub genes were identified in cotton (Gossypium hirsutum) in which the expression of Gh_A06G1257 was significantly the highest in different tissues, and it was identified as regulating drought stress by a gene silencing technique (Gereziher et al., 2021). The hub gene and regulatory network of cotton were discovered under salt and drought stress (Bano et al., 2022). The research model of gene interaction networks can explore hub genes and the complex regulatory network of drought and salt stress. In this study, the drought-related modules were studied based on the transcriptome data to obtain a comprehensive drought gene regulatory network, which is conducive to the in-depth understanding and analysis of the function of hub genes in complex networks.
The level of tolerance of plants to drought conditions is coordinated by the action of different drought-responsive genes about other stress components, such as high temperature and salt stress, which stimulate signal transduction pathways and are complex and mutagenic (Oladosu et al., 2019; Gereziher et al., 2021). Exploring the molecular mechanism of drought resistance in A. mongolicum requires a comprehensive analytical method, and the current high-throughput sequencing technology provides an effective platform (Mutz et al., 2013; Han et al., 2016). The integrated analysis of transcriptome, sRNAs and degradome offers the feasibility to select and identify the drought stress gene regulatory network and hub genes of A. mongolicum.
The regulatory mechanism of drought resistance and hub genes in A. mongolicum is still in its early stages. The regulation of drought resistance is complicated, which restricts the development and utilization of gene resources in response to drought stress. By integrating the transcriptome, sRNAs, and degradome analysis, this study aimed to identify the hub genes of drought resistance and establish a co-expression regulatory network of hub genes in A. mongolicum. The findings are meaningful to identify drought resistance hub genes and understand the molecular mechanism of drought resistance in A. mongolicum, which should promote genetic engineering research on drought resistance in Poaceae crops.
Materials and methods
Plant materials and drought stress treatment
Mature seeds of A. mongolicum were collected from the Inner Mongolia Agricultural University’s (Hohhot, China) forage test station in the Inner Mongolia Autonomous Region. The lemma was removed from the intact seeds, which were disinfected with a (1,1.3) sodium hypochlorite solution (v/v) for 15 min and then rinsed five times with sterilized distilled water. The seeds were planted in a germination box and cultured in a controlled climate growth chamber at 24°C with 16 h of daylight with an illumination intensity of 30,000 Lx (BIC-300; Boxun, Shanghai, China). Sterilized distilled water was sprayed quantitatively during the seed germination. When the seedlings grew to be 5–8 cm high, they were transplanted into a germination box with 20% Hoagland’s nutrient solution and cultured until the three-leaf one heart phase. The seedlings were then exposed to a drought treatment with 25% polyethylene glycol (PEG)-6,000. Their leaves were sampled at 0 h (CK), 12 h (D_12 h), 24 h (D_24 h), 48 h (D_48 h), 3 days (D_3 days), 5 days (D_5 days), and 7 days (D_7 days), and rewatered at 24 h (R_24 h). The sample weight of each biological replicate was 1 g of fresh leaves. The samples were frozen in liquid nitrogen and stored at −80°C.
Total RNA extraction
The total RNA was extracted from the samples using the TRIzol reagent (Invitrogen, Carlsbad, CA, United States). The quantity and purity of RNA were evaluated using a NanoDrop ND-1000 (Thermo Fisher Scientific, Waltham, MA, United States), and the integrity and concentration of RNA were assessed using an Agilent Bioanalyzer 2,100 (Agilent Technology, Santa Clara, CA, United States; RIN number > 7.0, OD260/280 > 1.8). The total RNA collected from each treatment and control group was utilized to create the library and transcriptome, sRNA and degradome sequencing. The samples were sequenced de novo by Hangzhou LC Biology Co., Ltd. (Hangzhou, China).
Transcriptome sequencing and de novo assembly analysis
Certified total RNA was purified twice using poly-T oligo linked magnetic beads to obtain poly (A) RNA. Following purification, the mRNA was fragmented into minute fragments under increased temperature using divalent cations. The cleaved RNA fragments were then reverse-transcribed to form the final cDNA library, which was consistent with the manufacturer’s instructions for the RNA-Seq sample preparation kit (Illumina, San Diego, CA, United States), with an average insert size of 300 bp (±50 bp) for the paired-end libraries. The paired-end samples were sequenced on an Illumina HiSeq 6,000 at LC Sciences (Houston, TX, United States) according to the manufacturer’s instructions.
Cutadapt (Martin, 2011) and in-house Perl scripts were used to delete the reads with adapter contamination, low-quality bases, and uncertain bases. The sequence quality was then confirmed using FastQC,1 which included the Q20, Q30, N50 and GC content of the clean data. All the downstream analyses relied on high quality clean data. Trinity 2.4.02 was used to accomplish de novo transcriptome assembly (Grabherr et al., 2011). Trinity groups transcripts into clusters, and the most extended transcript in each collection is known as the UniGene.
All the assembled UniGenes were aligned against the non-redundant (Nr) protein database,3 Gene Ontology (GO),4 SwissProt,5 Kyoto Encyclopedia of Genes and Genomes (KEGG)6 and eggNOG7 databases using DIAMOND (Buchfink et al., 2014) with a threshold of E-value < 0.00001.
Differentially expressed unigene analysis
Salmon (Patro et al., 2017) was used to identify the levels of expression for UniGenes by calculating the transcript per million (TPM; Mortazavi et al., 2008). The differentially expressed unigenes were selected with log2 (fold change) ≥1 or log2 (fold change) ≤ −1 and with the statistical significance of p ≤ 0.05 by the R package edgeR (Smyth, 2010). GO and KEGG enrichment analyses were then performed on the differentially expressed genes (DEGs) using in-house Perl scripts.
sRNA sequencing and miRNA basic data analysis
A TruSeq Small RNA Sample Prep Kit (Illumina) was used to create the miRNA library. The experiment was conducted according to the manufacturer’s instructions. An Illumina HiSeq 2,500 platform was used to sequence the cDNA library, and single-end (SE50) sequencing was utilized (50 bp). To obtain clean reads, raw reads were submitted to an in-house tool designated ACGT101-miR (LC Sciences), which removed adaptor dimers, repetitions, junk sequences and typical RNA families (rRNA, tRNA, snRNA, and snoRNA). The subsequent use of a BLAST search mapped unique sequences that were 18–25 nt long to miRBase 22.0 to identify the miRNAs. The miRNAs matched to the database are known, while those that do not map to it are unique miRNAs in A. mongolicum.
To better understand the overall evolutionary relationship and conservation of miRNA, the family classification of miRNA, the frequency of miRNA in other species, the number of miRNA precursors in different species and the base preference of miRNA were statistically analyzed.
Analysis of the differentially expressed miRNAs
Based on the experimental design, the differential expression of miRNAs was evaluated using a t-test. The significance threshold was set at 0.01 and 0.05 in each test. Differentially expressed miRNAs were assigned a value of p ≤ 0.05 and a|log2 (fold change)| ≥ 1, and a cluster diagram of the miRNA expression (norm value) was constructed. The up- and downregulated miRNAs were simultaneously enumerated from differentially expressed miRNAs.
Degradome sequencing, target identification and analysis
The total RNA (20 μg) was purified using poly-T oligo-attached magnetic beads (Thermo Fisher Scientific), and the mRNA was collected using poly (A). The captured mRNA was coupled using 5′ adaptor primers (Genewiz, Plainfield, NJ, United States). The attached product was transferred into a new centrifuge tube and supplemented with KAPA Pure Beads (kk8000; Roche Diagnostics Application, Indianapolis, IN, United States). The reverse transcription was amplified with reverse transcriptase (m0368; New England Biolabs, Ipswich, MA, United States). The degradation group sequencing library was built with an NEBNext Ultra II RNA Library Prep Kit (e7770; New England Biolabs). Single-ended sequencing was performed using an Illumina HiSeq 2,500 (Hangzhou LC Biology Co., Ltd.) as previously described (Ma et al., 2010).
The target gene was predicted using the Cleaveland 4.0 algorithm (Addo-Quaye et al., 2009), and oligomap was used to accurately match the mRNAs from different species to the A. mongolicum degradation group sequence (Berninger et al., 2008). The redundant sequences were removed from the effective data using norm reads per million (NRPM). The Needle program in EMBoss was used to collect all the sequences that matched the target genes in the miRNA library, and the target genes were scored according to the plant miRNA/target pairing standard (Allen et al., 2005). In addition, the degradome reads were mapped to the A. mongolicum transcriptome data as previously described (Xu et al., 2013; Yang et al., 2013). The number of unique genes and the mapping ratio between ideal mRNA and degradation group fragments were counted to assess the degradation group sequencing.
Integrated analysis of transcriptome, miRNA and degradome sequencing
All the miRNAs and their target genes were obtained based on an integrated analysis of the transcriptome, miRNA, and degradome group data. The connection pairs between differential miRNAs and their differential target genes were created using a threshold of p ≤ 0.05. The miRNAs and their target gene pairings with negative regulatory relationships were chosen. The stats package in the R package (version 3.6.1) was used to calculate the cluster data, and the heatmap software (version 1.0.12) was used to create a cluster heat map for the DEGs in negative regulatory mode. The transcription function was annotated.
Construction of the gene co-expression network and screening of the hub gene
A co-expression network analysis was performed based on the transcriptome data using the WGCNA R package (version 1.69). The unigenes of missing values were removed, and the expression data of mRNA was regenerated after filtering. The sample clustering tree was then constructed; the outlier samples were removed, and the appropriate scale-free key parameter β was selected. To ensure a scale-free network, power = 4 was chosen to build a systematic clustering tree between the genes. All the other settings were left at the default levels, including the module size of gene modules, the number of modules, computing the feature vector value between modules, and performing module clustering analysis. The webpage of the Horvath lab8 contains a thorough description of WGCNA that explains the analytical stages (Langfelder and Horvath, 2008).
The module constructed by WGCNA and drought-related physiological indicators were used for correlation analysis to select specific modules related to drought resistance. The connectivity top 20 in the module were defaulted to be hub genes. The candidate hub genes related to drought resistance were screened from the high connective and specificity genes by GO enrichment analysis. The targeted relationships between the hub genes and miRNAs were confirmed by degradome sequencing data. The drought resistance candidate hub genes of the A. mongolicum regulatory network were mapped using Cytoscape (version 3.9.1).
RT-qPCR analysis of miRNA and potential drought resistant candidate hub genes
Quantitative reverse transcription PCR (RT-qPCR) was performed on selected miRNA and candidate hub genes related to drought resistance. Primer Premier 6.0 software was used to design the primers, and the primers were synthesized by Sangon Biotech Co., Ltd. (Shanghai, China; Table 1). An miRcute Plus miRNA qPCR Detection Kit (FP411) and an miRcute Plus miRNA First-Strand cDNA Kit (KR211) from TianGen (Beijing, China) were used for reverse transcription and RT-qPCR of the miRNA. A FastQuant RT Kit (KR106; TianGen) and MonAmp SYBR Green qPCR Mix (MQ10201S; Monad Biotech Co., Ltd.) were used for reverse transcription and RT-qPCR of the candidate hub genes. The relative levels of expression were calculated by the 2^ –ΔΔct method using U6 as internal standards (Livak and Schmittgen, 2001).
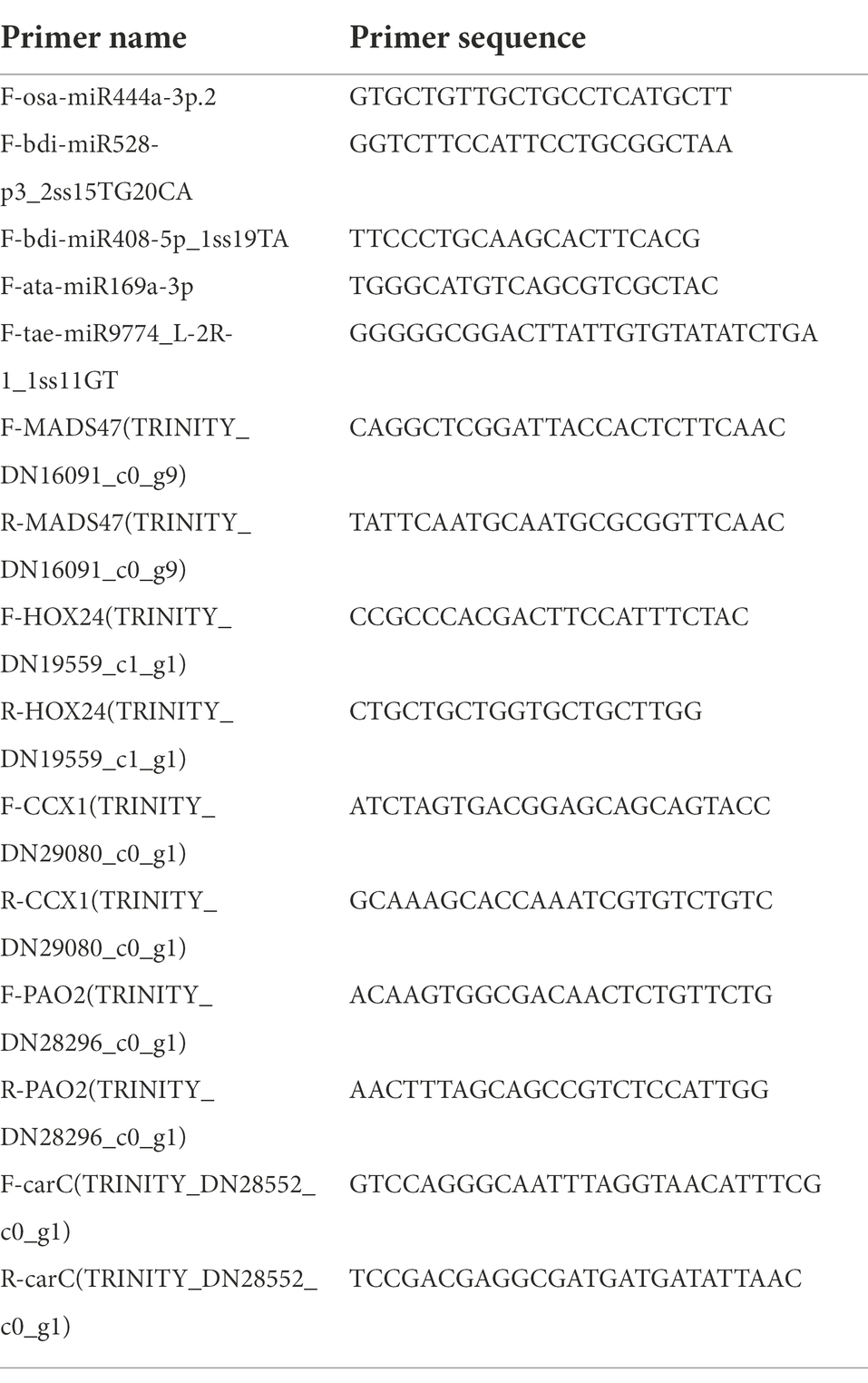
Table 1. A list of primers used for validation in RT-qPCR. RT-qPCR, quantitative reverse transcription PCR.
Results
Transcriptome sequencing in Agropyron mongolicum under drought treatments
To profile the expression of genes in A. mongolicum in response to drought stress, eight libraries were constructed from leaf samples (CK: control, D_12 h, D_24 h, D_48 h, D_3 d, D_5 d, D_7 d: plants under drought stress, R_24 h: plants rewatering after drought stress). More than 145.27 Gb raw reads were generated, and 139.46 Gb clean sRNA reads were obtained. The average Q20 of the sample was 97.445%. Each raw read and clean read of each sample were tallied (Supplementary Table 1A). After quality control, the transcripts were assembled into 41,792 unigenes, and the unigene lengths ranged from 201 bp to 16,861 bp (Table 2; Supplementary Figure 1).
Functional annotation and enrichment analysis of the unigenes
GO functional annotation and enrichment were performed on the transcriptome sequenced UniGenes. A total of 18,259 UniGenes were found to be enriched based on the GO analysis, which accounts for 43.69% of all the UniGenes (Supplementary Table 1B). These terms were primarily involved in biological processes, cell component and molecular function. The GO terms included response to salt stress, response to water deprivation, and response to wounding (Figure 1A) according to the study of GO enrichment in A. mongolicum. The enrichment of transcripts was analyzed using KEGG. The transcripts were primarily enriched in five significant pathways, including organic system, metabolism, genetic information processing, environmental information processing and cellular processes. The main pathways involved carbohydrate metabolism, amino acid metabolism and lipid metabolism. In addition, they also participated in translation, folding, sorting and degradation, transcription, replication and repair, signal transduction, membrane transport, transport and catabolism (Figure 1B).
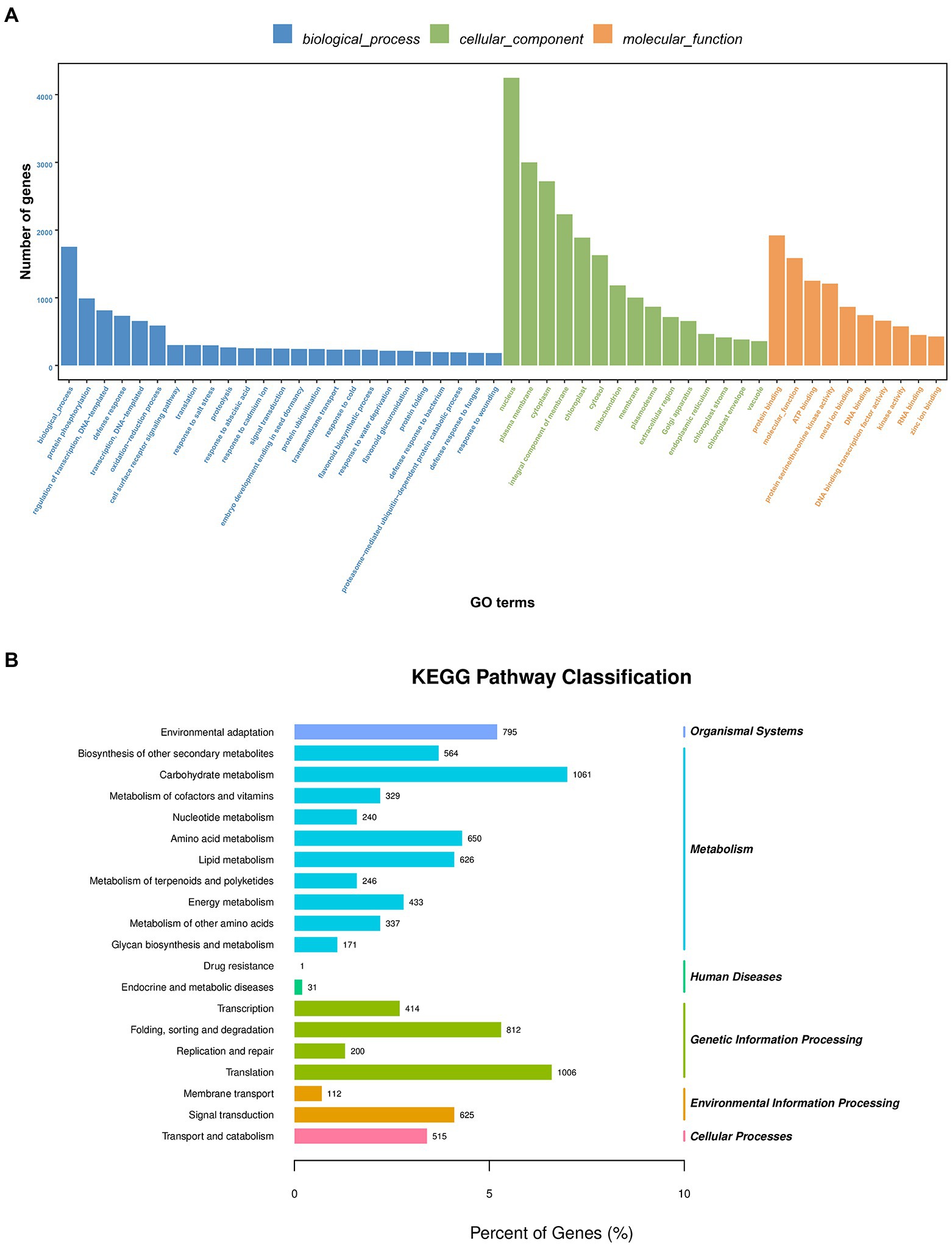
Figure 1. GO classification (A) and KEGG pathway enrichment (B) of UniGenes. GO, Gene Ontology; KEGG, Kyoto Encyclopedia of Genes and Genomes.
Analysis of the differentially expressed genes
In contrast to the control group, the number of DEGs was screened in each comparison group (Figures 2A,B). A total of 1,166 DEGs were obtained in the seven comparison groups (D_12 h vs. CK, D_24 h vs. CK, D_48 h vs. CK, D_3 d vs. CK, D_5 d vs. CK, D_7 d vs. CK, and R_24 h vs. CK). The number of DEGs generally increased as the drought lasted longer, while the number of DEGs reduced in a comparison group (48 h vs. CK). After 12 h of drought treatment, there were 1,789 upregulated genes and 1,183 downregulated genes compared with the control. There were 3,399 upregulated genes and 2,153 downregulated genes compared with the CK after 24 h of drought treatment. The number of upregulated DEGs was 3,565, 4,270, 5,105, 6,511, and 7,521 compared with the CK at 48 h, 3 d, 5 d, 7 d, and R_24 h under drought treatment, respectively, whereas the number of downregulated DEGs was 1,733, 2,096, 3,052, 5,519, and 7,739, respectively. The information on all the DEGs in seven comparisons is shown in Supplementary Table 2.
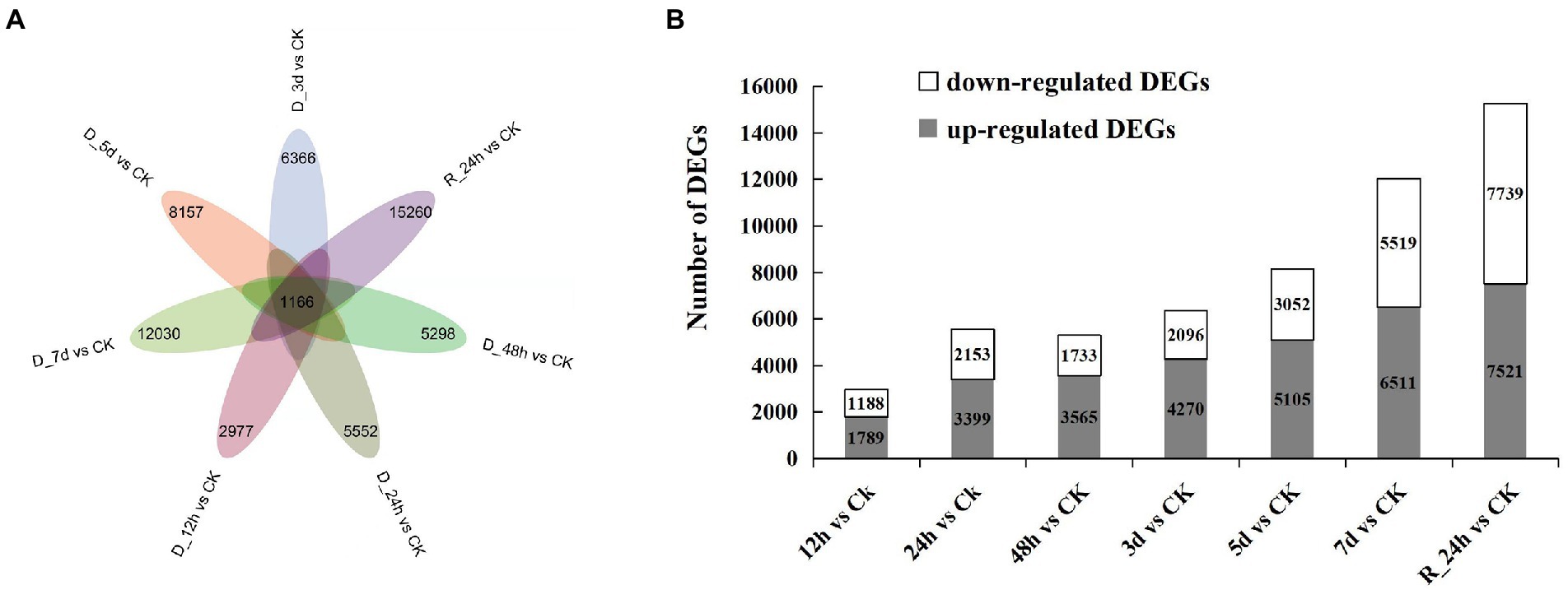
Figure 2. Drought responsive genes in Agropyron mongolicum. (A) Venn diagrams of differentially expressed genes in the treatment and control groups. (B) The number of differentially expressed genes under drought stress compared with the control.
Sequencing and identification of the miRNAs
The number of known mature miRNA families of A. mongolicum was 71 (Supplementary Figure 2A). In contrast, the pre-miRNA sequences of A. mongolicum were highly similar to the known pre-miRNAs in 46 plant species (Supplementary Figure 2B). All the miRNAs were categorized into four groups (gp1, gp2, gp3, and gp4) based on their abundance in the miRNA database and sequencing reads (Supplementary Table 3). miRNA sequences that matched the pre-miRNA sequences in the miRNA database were classified as known miRNAs, whereas those that did not were classified as novel miRNA candidates of A. mongolicum. A total of 895 mature miRNAs had been obtained, and the length of known miRNA sequences ranged from 18 to 25 nt, with those that were 21 nt accounting for 49.50%. There were 209 novel miRNAs, and the sizes ranged from 19 to 24 nt, accounting for 77.51% at 21 nt (Supplementary Tables 4, 5A; Supplementary Figures 2C,D). The minimal folding free energy index (MFEI) offers a standard for comparing the MFE of pre-miRNA of different lengths of A. mongolicum. The minimal folding energy (MFE) is related to the sequence length of pre-miRNA (Zhang et al., 2006). The MFEI of the novel miRNA in A. mongolicum ranged from 0.90 to 2.30 kcal/mol (Supplementary Table 6). It was indicative of the production of a stable hairpin structure.
Differentially expressed miRNAs under drought stress
A total of 101 differentially expressed miRNAs (p ≤ 0.05) were found among the 24 libraries. The distribution of differential expression miRNAs between the control and treatments was examined (Figure 3A). Compared with the control, the number of upregulated genes (29 miRNAs) was the largest at 7 days under drought stress, and the number of downregulated genes (23 miRNAs) was the largest at 24 h under drought stress. To analyze 101 differentially expressed miRNAs of A. mongolicum under drought stress, including 12 novel and 89 known miRNAs (Supplementary Table 7), a clustering diagram was generated based on the normal values of miRNA expression (Figure 3B). The expression of miRNAs in the same family was similar under drought stress treatment. The miR156 family, such as osa-miR156_L+R+1, ata-miR156a-5p, and bdi-miR156b-5p, were significantly upregulated under drought treatment. The expression of miR1137 family was upregulated at 5 days, 7 days, and R_24 h. The expression of miR166 family, including osa-miR166b-5p and gma-miR166a-5p_1ss19AG, was downregulated. Among the 12 differentially expressed novel miRNAs, PC-5p-5881_2,234 was downregulated under drought treatment and upregulated at R_24 h. In general, the level of expression of the four novel miRNAs, which included PC-5p-10048_1508, PC-5p-52255_298, PC-5p-882_11429, and PC-3p-85874_131, were downregulated to different degrees at 12 h to 7 days under drought treatment, and their levels of expression were downregulated at 24 h of rehydration. The levels of expression of four novel miRNAs (PC-3p-93182_112, PC-3p-94625_109, PC-5p-57663_259, and PC-3p-95140_107) were upregulated at 5 and 7 days under drought stress and rehydration at 24 h. Three novel miRNAs (PC-5p-55424_274, PC-5p-41033_410, and PC-5p-77163_159) were downregulated at 24 h under drought stress and upregulated from 3 days under drought stress to 24 h of rehydration (Figure 3B). These data suggest that the expression of miRNAs changes with the prolongation of drought stress and do not always remain up- or downregulated.
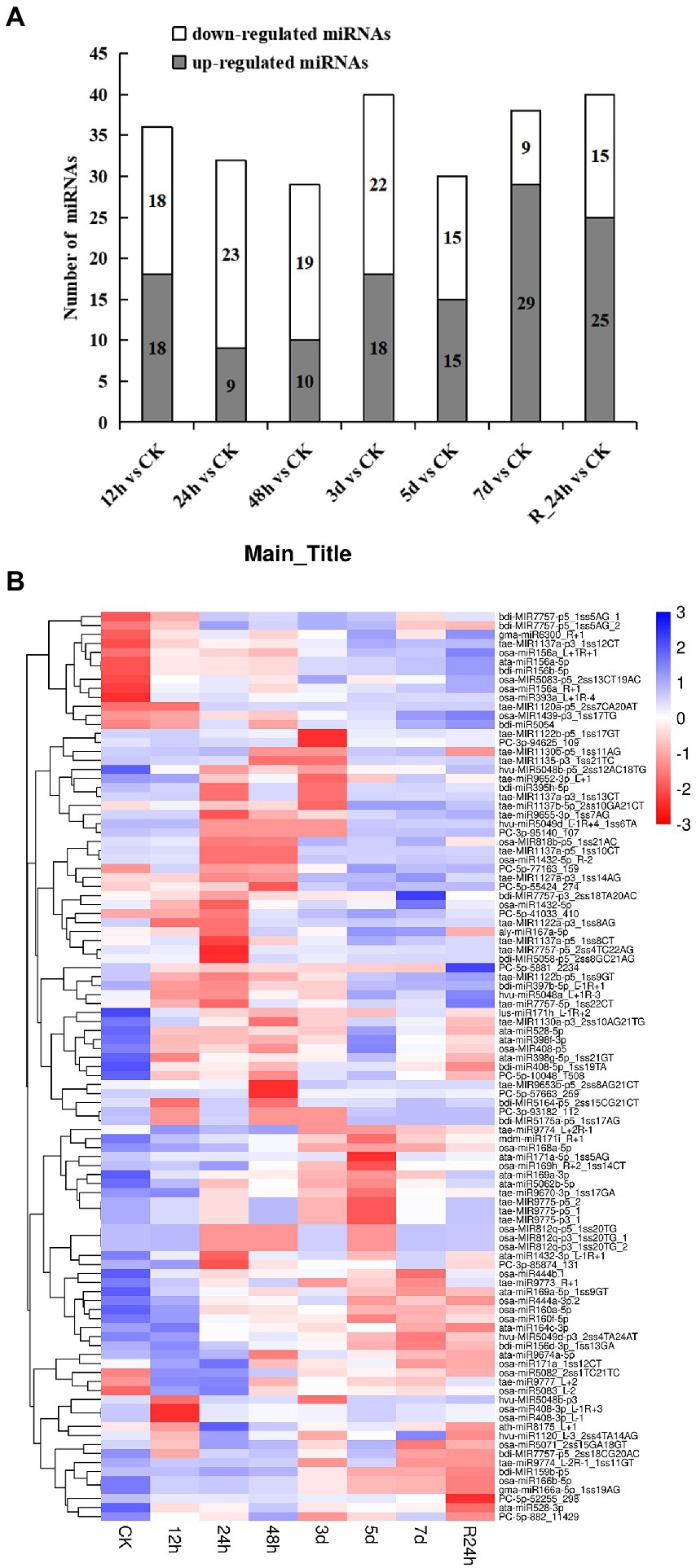
Figure 3. Differentially expressed miRNAs under drought stress. (A) The number of differentially expressed miRNAs compared with the control. (B) Hierarchical clustering of differentially expressed miRNAs in eight different durations of drought treatment [CK (0 h), 12 h, 24 h, 12 h, 48 h, 3 days, 5 days, 7 days, and R24 h]. Red indicates a lower level of expression of the miRNAs, and blue indicates higher levels of expression of miRNAs. The names of the samples are shown at the bottom, and the name of the differentially expressed gene is shown on the right. The norm expression of miRNA was standardized using a Z-score. The absolute signal intensity ranges from −3 to +3, and the corresponding color changes from red to blue.
Degradome sequencing analysis
After removing the unqualified sequences, 28,885, 26,523, 17,691, 31,196, 28,063, 40,389, 35,357, and 38,874 unique reads were obtained from the CK and each drought treatment group (Supplementary Table 5B), respectively. The ratios of acquired unique mapped reads were 46.83, 53.01, 52.98, 53.63, 53.30, 56.17, 57.03, and 54.35%, respectively (Supplementary Table 5B). The ratios of covered transcripts in the five treatment groups were 80.80, 80.01, 82.18, 81.81, and 81.40%, respectively. The ratios of covered transcripts in the control and drought treatments were 76.52, 77.87, and 71.96% at CK, 12 h, and 24 h of drought stress, respectively. The target genes were separated into five categories (Category 0, 1, 2, 3, and 4; Xu et al., 2013; Yang et al., 2013). A total of 90, 71, 2,591, 2,758, and 3,972 target genes of miRNAs in A. mongolicum were in each category (Supplementary Table 5C). A total of 676 miRNAs that regulate 46,456 target genes were identified by degradome sequencing (Supplementary Table 8), and 137 novel miRNAs regulated 1,394 target genes (Supplementary Table 9).
Integration analysis of miRNAs and their target genes under drought stress
To investigate the trends of miRNA and mRNA changes in A. mongolicum under different stages of drought stress, the differentially expressed miRNAs and DEGs from miRNA were integrated and analyzed based on transcriptome and degradome sequencing data. The miRNAs and their target genes with negative regulatory mode were screened in the control group compared with the seven drought-treated groups based on the general model (cleavage) of the miRNA regulation of target genes in plants (Llave et al., 2002). A total of 91 known miRNAs that belonged to 59 miRNA families were found to negatively regulate 1,438 target genes (Figures 4A,B; Supplementary Table 10), indicating that the miRNAs and target genes are not all regulated one-to-one but are regulated in a network-like radial pattern. One of the target genes (norm value of the highest) was chosen to participate in the heatmap when one miRNA regulated many target genes (Figures 4A,B). Eight differentially expressed novel miRNAs of A. mongolicum regulated 36 target genes. Seven upregulated the expression of miRNAs, such as PC-3p-105150_88, PC-3p-68960_194, PC-3p-77094_159, PC-5p-41033_410, PC-5p-77163_159, PC-5p-78312_155, and PC-5p-87000_128, and PC-3p-85874_131 was a downregulated miRNA (Figure 4A; Supplementary Table 11). A total of 36 target genes under drought stress were annotated as uncharacterized oxidoreductase, protein MAK16 homolog, magnesium-chelatase subunit ChlH chloroplastic, NADP-thioredoxin reductase C precursor, chloroplast ferredoxin-dependent glutamate synthase, and heat shock protein (HSP)-interacting protein. All of them were related to drought or adversity stress, and the function of three target genes was annotated as unnamed protein products. The functions of the two target genes were unknown (Table 3).
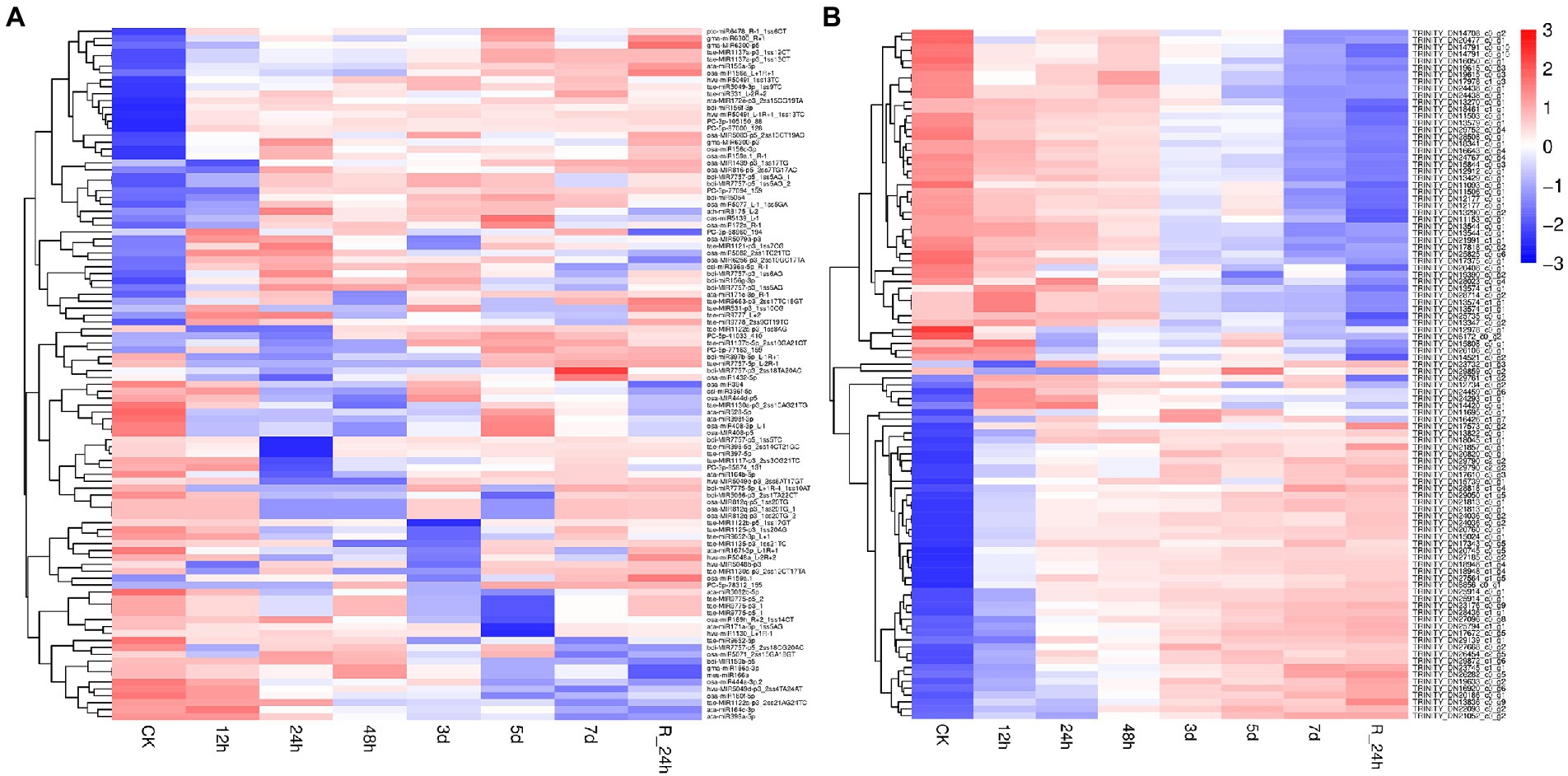
Figure 4. A combined view of the levels of expression between differentially expressed miRNAs (A) and their target genes (B) in Agropyron mongolicum in eight different drought treatment durations. The original expression values of miRNAs and their target genes were normalized by Z-score normalization.
Gene co-expression network module construction
A WGCNA analysis indicated that genes with similar functions cluster together in the same module (Langfelder and Horvath, 2008). To identify hub genes and their interacting genes of A. mongolicum involved in drought resistance, 41,793 genes were used to construct gene co-expression networks and modules, which yielded 39 modules (Figure 5A).The turquoise module was the largest and contained 10,766 genes, while the smallest module was the plum1 module, which included 36 genes (Supplementary Table 12). The module heat map of gene clustering was constructed based on the correlation of expression of feature genes. The gene clusters with highly correlated expression corresponded to a branch of the clustering tree. The clustering heat map drawn by the neighbor relationship was consistent with the clustering results drawn by dynamic shea (Figure 5B). A total of 39 modules of all genes from 24 samples were used to create cluster heatmaps for A. mongolicum (Figure 5C). The violet and darkolivegreen modules were highly correlated with drought-treated samples at D_24 h2 and D_24 h3. The red and salmon modules positively correlated with drought-treated samples at D_24 h3; and the turquoise module positively correlated with the drought-treated samples at 5 d, 7 d, and R_24 h. The steelblue module was positively associated with drought-treated samples at 12 h, 5 days, 7 days, and R_24 h. The modules involved in drought stress were initially screened by a module-trait interrelationship heatmap.
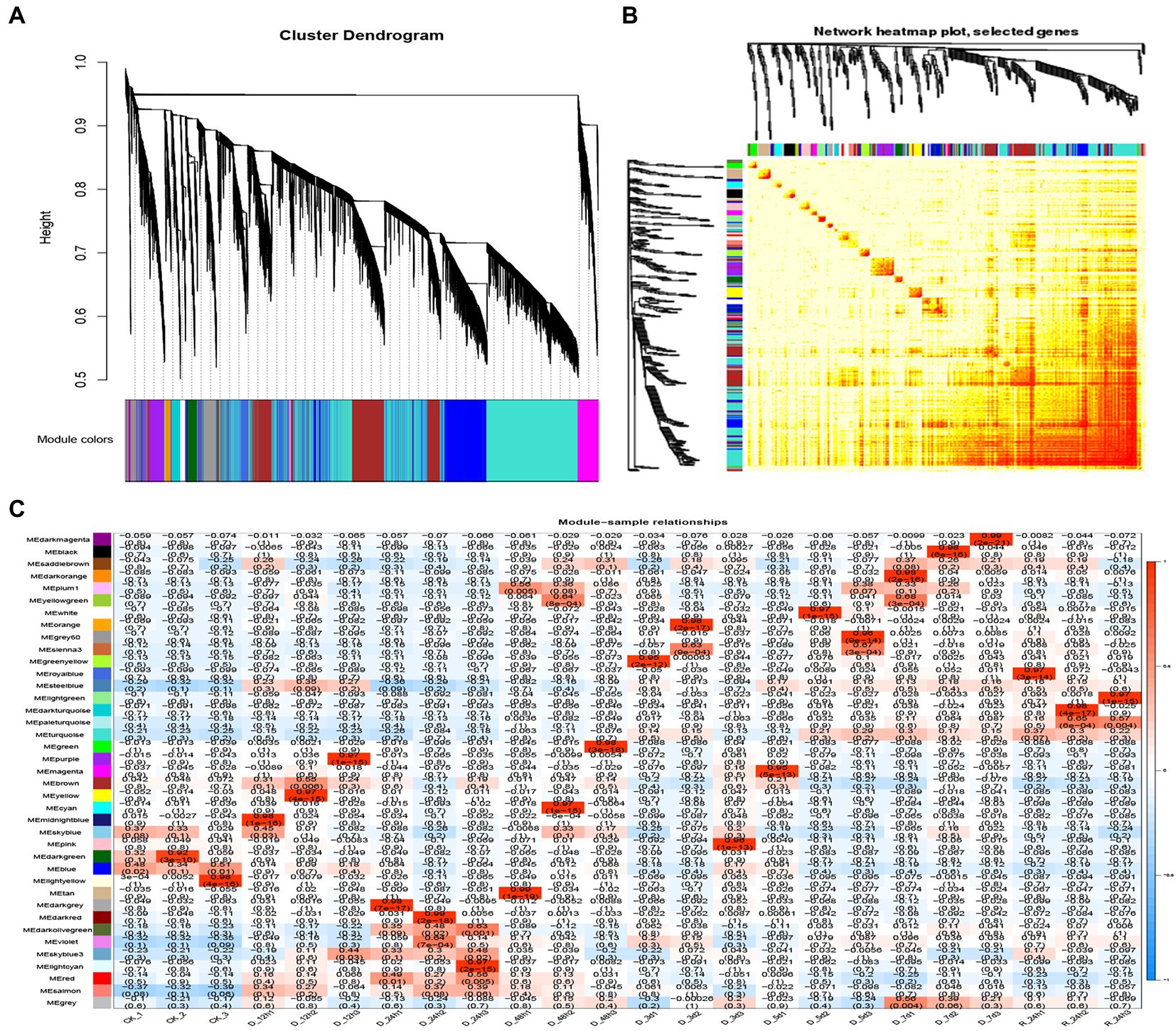
Figure 5. Correlation between sample clustering of the modules and genes. (A) Clustering dendrograms of the genes. Based on the dynamic hybrid branch cutting method, color strips were used for simple visualization of the module assignment (branch cutting). (B) 41,793 gene and module correlation clustering diagrams. (C) Heat map of the correlations between the modules and genes of different samples. The colors that range from blue through white to red indicate low through intermediate to high correlations, respectively.
Analysis of the drought-related modules
Relative conductivity, proline (Pro) and malondialdehyde (MDA) contents are closely related to the drought tolerance of plants. The use of module-trait correlation analysis facilitates the study of complex drought effects. The relative conductivity, Pro and MDA contents of A. mongolicum were measured under 25% PEG-6000 treatment (Supplementary Figure 3). In A. mongolicum, a heat map of correlations between the module and trait was created using 39 modules and physiological indices of drought resistance, such as relative conductivity and the contents of MDA and Pro (Figure 6). A correlation analysis showed that the turquoise module positively correlated with relative conductivity and Pro, with correlation coefficients of 0.73 (p = 5e−05) and 0.69 (p = 2e−04), respectively, and the correlation coefficient of MDA was 0.29 (p = 0.2). The steelblue module significantly and positively correlated with relative conductivity, MDA, and Pro, with correlation coefficients of 0.49 (p = 0.02), 0.44 (p = 0.03), and 0.5 (p = 0.01), respectively. The violet module positively correlated with relative conductivity and Pro with correlation coefficients of 0.43 (p = 0.04) and 0.44 (p = 0.03), respectively, and the correlation coefficient of MDA was 0.36 (p = 0.08). The blue module significantly and negatively correlated with relative conductivity, MDA, and Pro with correlation coefficients of −0.65 (p = 7e−04), −0.53 (p = 0.007), and −0.68 (p = 3e−04), respectively.
GO and pathway analysis of genes in specific modules
Under drought treatment, the GO and KEGG analyses showed the functions and pathways of genes in specific modules of A. mongolicum. Biological processes, molecular functions, and cellular components were enriched in the GO analysis of turquoise, steelblue, violet, and blue modules (Figures 7A–D). In biological processes, it was primarily enriched for the regulation of transcription, DNA-templated (GO:0006355), protein phosphorylation (GO:0006468), signal transduction (GO:0009737), brassinosteroid-mediated signaling pathway (GO:0009742), abscisic acid (ABA)−activated signaling pathway (GO:0009737, GO:0009788, GO:0009737, GO:0009738), cell surface receptor signaling pathway (GO:0007166), positive regulation of protein ubiquitination (GO:0031398), and response to water deprivation (GO:0009414) processes. The biological processes played an essential role in the mechanism of A. mongolicum related to drought resistance. Cellular Component analysis revealed that the four modules were primarily enriched in the nucleus (GO:0005634), plasma membrane (GO:0006351, GO:0005886, and GO:0005829), cytoplasm (GO:0005737), cytosol and chloroplast (GO:0016021 and GO:0009941) components. The results showed that cellular components, such as the nucleus, cytoplasm, plasma membrane and chloroplast, responded to drought stress. Molecular function was primarily enriched to protein binding (GO:0005515), metal ion binding (GO:0046872, GO:0008270), kinase activity (GO:0016301) and other functions, which could play an important role in drought regulation in A. mongolicum. The KEGG pathway from four modules of A. mongolicum was primarily enriched for transport and catabolism under drought stress, signal transduction, amino acid metabolism, carbohydrate metabolism, and environmental adaptation, indicating that these pathways responded to stimulation in response to drought (Figures 7E–H).
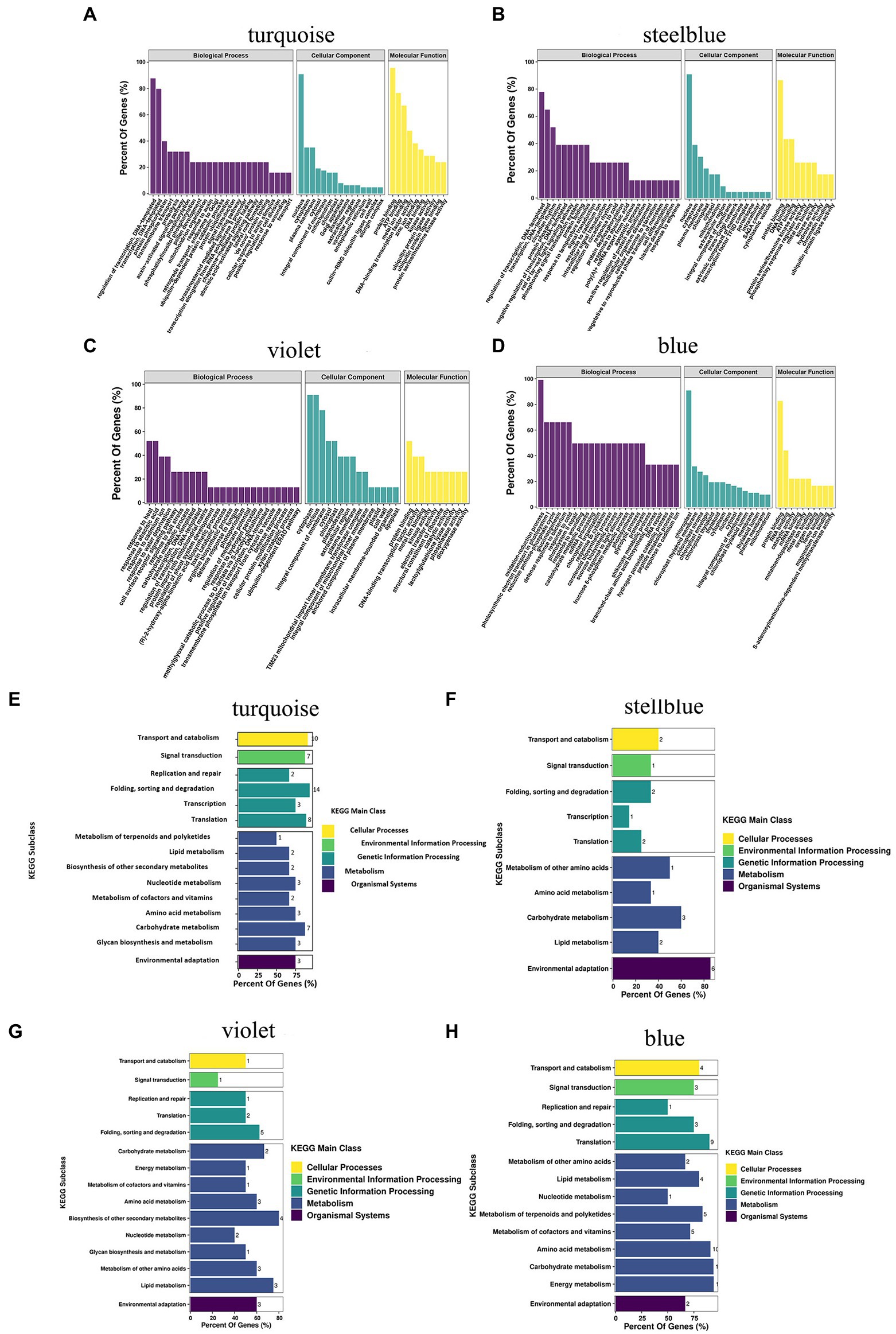
Figure 7. GO classification and putative KEGG pathways of specific modules related to drought resistance in Agropyron mongolicum. The GO classification and KEGG pathway of turquoise, steelblue, violet, and blue modules are represented by (A), (B), (C), (D), (E), (F), (G), and (H), respectively. GO, Gene Ontology; KEGG, Kyoto Encyclopedia of Genes and Genomes.
Analysis of drought-resistant hub genes
The top 20 genes in the four modules related to the drought tolerance of A. mongolicum with connectivity were used as hub genes, and the genes that interacted with the hub genes were selected (Supplementary Table 12). The differentially expressed miRNAs with a targeted relationship to the hub genes were screened based on the degradome and miRNA sequencing data. The five hub genes that targeted/regulated differentially expressed miRNAs were analyzed, including MADS47 (TRINITY_DN16091_c0_g9, GO Function annotation: brassinosteroid mediated signaling pathway) targeted/regulated osa-miR444a-3p.2 in the violet module (p < 0.01), CCX1 (TRINITY_DN29080_c0_g1, GO Function annotation: sodium and potassium ion transport) targeted/regulated bdi-miR408-5p_1ss19TA in the turquoise module (p < 0.05), steelblue module PAO2 (TRINITY_DN28296_c0_g1, GO Function annotation: oxidation–reduction process, peroxisome) targeted/regulated ata-miR169a-3p (p < 0.01) and carC (TRINITY_DN28552_c0_g1, GO Function annotation: hydrolase activity) targeted/regulated tae-miR9774_L-2R-1_1ss11GT in the blue module (p < 0.01). The HOX24 (TRINITY_DN19559_c1_g1) targeted/regulated bdi-miR528-p3_2ss15TG20CA was found in the violet module, and the GO term of the HOX24 gene was annotated as a signaling pathway in the response to water deprivation and in response to ABA and the ABA−activated signaling pathway, indicating that HOX24 may play an important role in drought stress resistance. The regulatory network and function of hub genes and interacting genes were deeply explored to better understand the mechanism of drought resistance in A. mongolicum (Figure 8). When A. mongolicum was subjected to drought stress, PAO2 controlled the functional genes of water deficit and regulatory proteins (TRINITY_DN18888_c0_g1, TRINITY_DN22628_c0_g2, and TRINITY_DN19796_c0_g3), suggesting that PAO2 may indirectly controls the enzyme activity-related proteins, ubiquitin-protein transferase activity, MAPK signaling pathway, and other functional genes. This indicates that PAO2 may play a key role in the gene coexpression regulatory network. carC would activate many of the genes that regulate chloroplast functions in response to drought stress and then regulate redox, carbohydrate metabolism, and phosphatase activity. MADS47 and HOX24 are the hub genes in the violet module with the connectivity of top1 and top15. These two genes may have direct interactions and co-regulate the downstream functional genes of kinase activity, oxidation–reduction and chloroplast. Secondly, the genes that govern enzyme activity, chloroplast, cytoplasm, protein ubiquitination, and cell components function are activated to achieve drought regulation. MADS47 is presumed to be the master gene in this module based on gene connectivity. There were 7,993 genes with interactions with CCX1, and 905 of the genes were chosen for functions related to water deficiency response, MAPK signaling pathway, and protein ubiquitination, including 204 genes for ion binding, transport, and exchange; 138 genes for protein ubiquitination; 122 genes for membrane function; and 102 genes for phytohormones, followed by redox regulation (90), chloroplast (82), water deficiency response (49), sugar (42). CCX1 of A. mongolicum would activate the interacting genes under drought stress, which mobilizes drought defense mechanisms to manage drought. The regulatory networks provide important references for subsequent studies of drought resistance mechanisms in A. mongolicum.
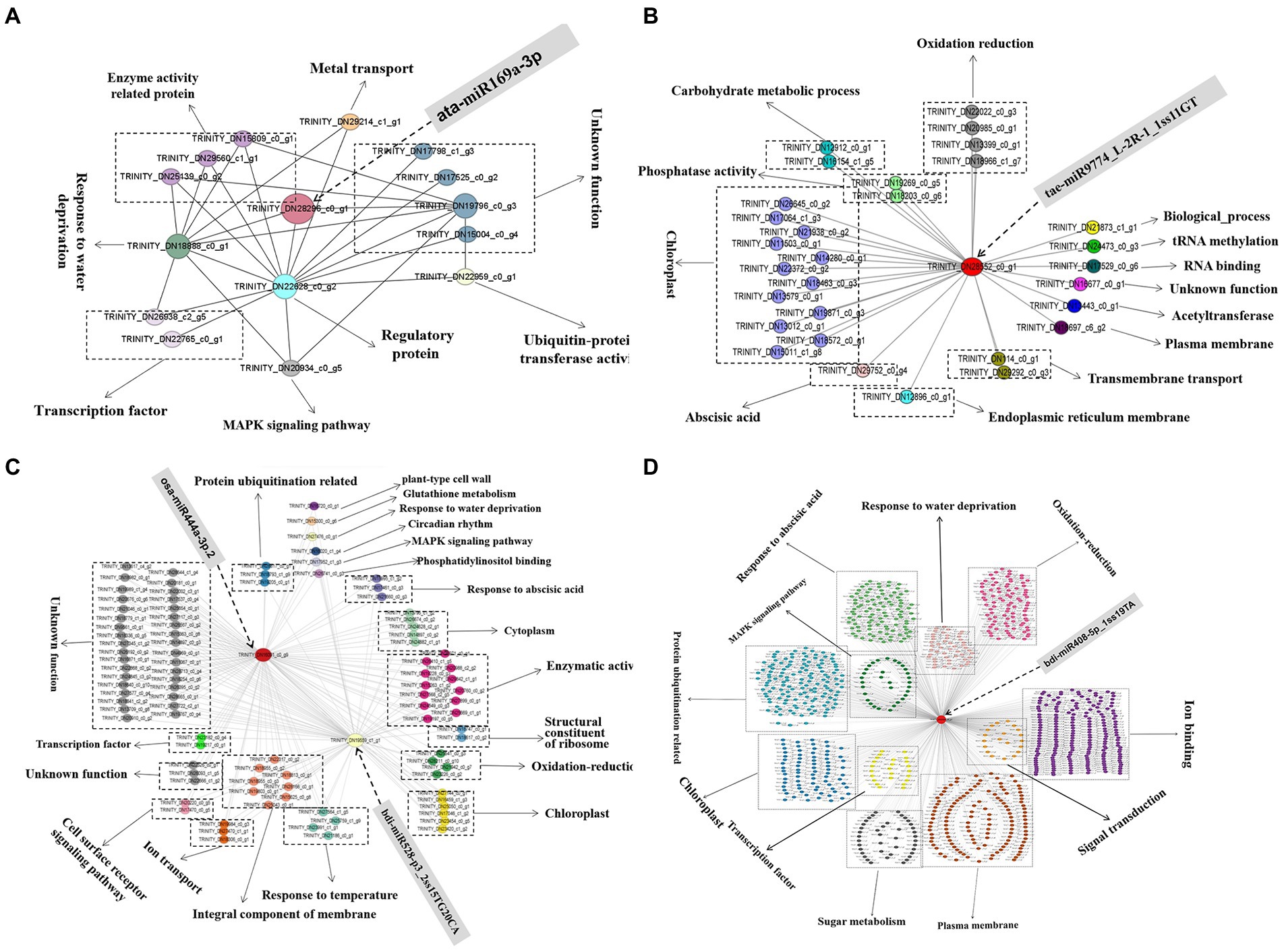
Figure 8. The coexpression subnetwork of five hub genes. Red-colored nodes suggest their central role in the network, and other nodes of the same color indicate the same function. (A), (B), (C), and (D) represent the coexpression subnetwork of TRINITY_DN28296_c0_g1 (PAO2), TRINITY_ DN28552_c0_g1 (carC), TRINITY_DN16091_c0_g9 (MADS47), TRINITY_DN19559_c1 _g1 (HOX24), and TRINITY_DN29080_c0_g1 (CCX1), respectively. A part of the gene ID corresponds to the gene name: Os06g0107700 (TRINITY_DN22022_c0_g3), XB3 (TRINITY_DN22959_c0_g1), CLPC1 (TRINITY_DN21467_c0_g3), and BPM2 (TRINITY_DN19691_c0_g7).
Correlation analysis of miRNAs and their candidate hub genes for drought resistance
Five miRNAs and their target genes were chosen for RT-qPCR to better understand the connection between the expression of miRNA and its drought-resistant potential hub genes (Figure 9). The patterns of expression of four miRNA-target gene pairings (bid-miR408-5p_1ss19TA-CCX1, ata-miR169a-3p-PAO2, osa-miR444a-3p.2-MADS47, and bdi-miR528-p3_2ss15TG20CA-HOX24) were all negatively regulated. The levels of expression of four miRNAs decreased as the drought treatment period increased, but the relative expression of their target genes increased. The levels of expression of target the genes increased after 24 h of rehydration. The relative expression of tae-miR9774_ L-2R-1_1ss11GT and target gene carC decreased overall.
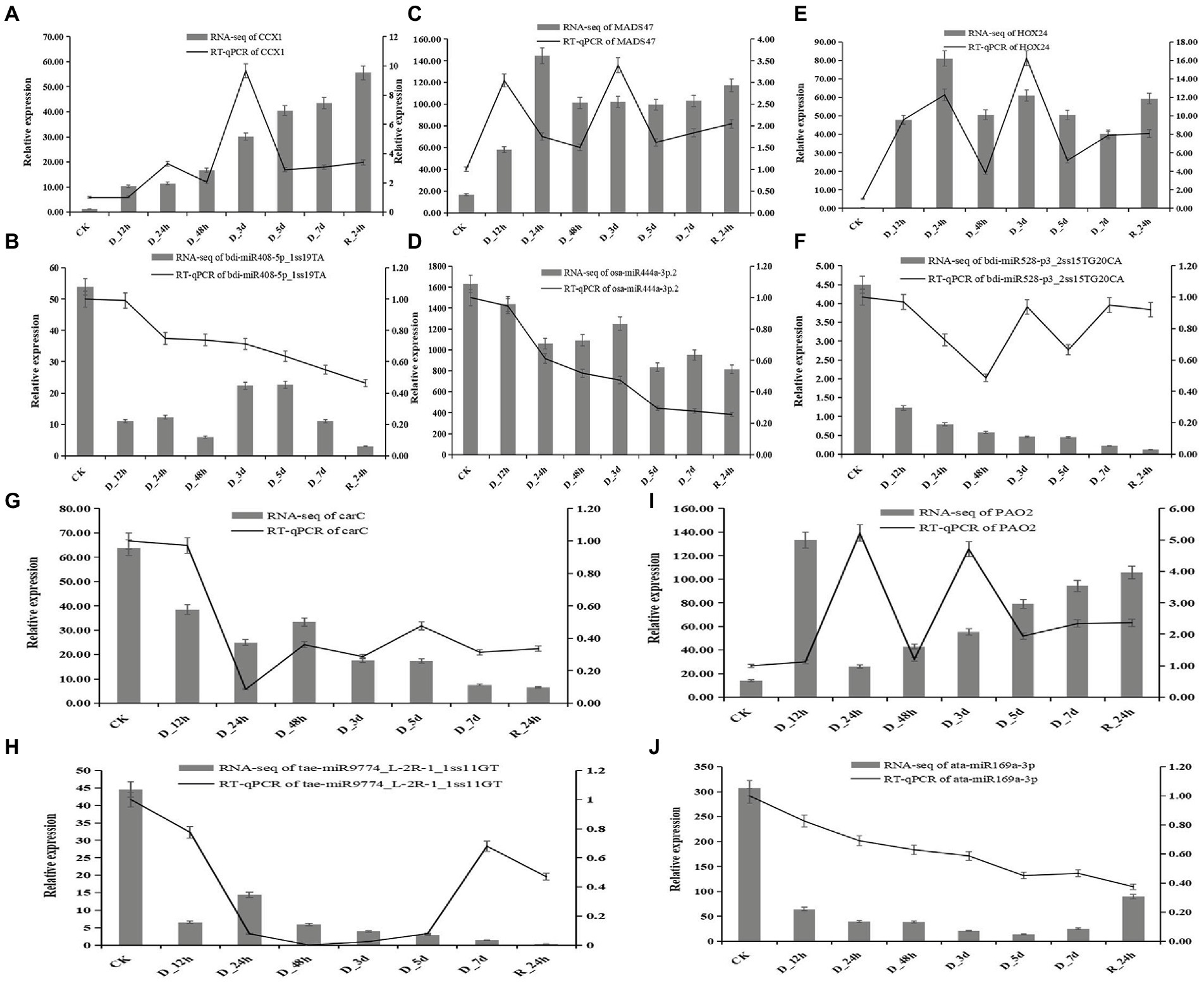
Figure 9. Correlation of the expression between miRNAs and the hub genes at eight durations of different drought treatments (25% PEG solution PEG6000). bdi-miR408-5p_1ss19TA target CCX1 (A,B); osa-miR444a-3p.2 target MADS47 (C,D); bdi-miR528-p3_2ss15TG20CA target HOX24 (E,F); tae-miR9774_L-2R-1_1ss11GT target carC (G,H); and ata-miR169a-3p target PAO2 (I,J).
Discussion
Agropyron mongolicum is a vital forage grass and has exceptional drought and cold resistance. It is grown in semiarid and arid desert regions where it is subjected to abiotic stresses, particularly drought stress. A. mongolicum has developed a robust set of mature regulatory mechanisms to manage the response to drought stress. The severity of drought is determined by various factors, such as rainfall, the ability of soils to store moisture, and evaporation demand. Drought has many effects on crops and can deleteriously affect metabolism, photosynthesis and defense mechanisms (Andrew et al., 2009). Plants respond to drought through root development, stomatal opening and closing, cell adaptation, ABA and reactive oxygen species (ROS) scavenging (Abid et al., 2017). The genes of exceptional drought resistance in A. mongolicum could be beneficial to improving the drought tolerance of gramineous crops, such as wheat and rice. Therefore, it is imperative to study the molecular mechanism of drought resistance in A. mongolicum.
Drought stress has been demonstrated to induce the particular expression of drought-related genes in plants (Bano et al., 2022). Three important high-throughput methods, the transcriptome, small RNA, and degradome sequencing, were applied to investigate the mechanism of drought resistance in A. mongolicum. In comparison with the miRNAs identified from the other plants, little research has been conducted on the role of miRNAs in A. mongolicum. To date, 114 miRNAs that are related to the drought resistance of A. mongolicum have been reported in our previous research group, and the functions of amo-miR21, amo-miR5 and amo-miR623 have been correlated with drought resistance (Zhang et al., 2019). The transcriptome dataset of A. mongolicum was used as a reference sequence for small RNA and degradome sequencing analyses to identify the miRNAs and their target genes that could be associated with drought stress. A total of 1,104 miRNAs, including 101 highly confident miRNAs (Supplementary Table 7), were identified based on the transcriptome data from A. mongolicum. Additionally, the miRNA lengths ranged from 18 to 25 nt, with a peak at 24 nt (Supplementary Figures 2C,D).
The results of the sRNA molecules of A. mongolicum match those of most plant miRNAs from prior studies (Zhao et al., 2010a; Niu et al., 2014; Bin et al., 2021). Some miRNAs that responded to drought in previous studies were also detected in our results, including miR156, miR159, miR162, miR171, miR444, and miR408. For example, rice tandem osa-miR156b and osa-miR156c were overexpressed in alfalfa (Medicago sativa). The expression of miR156 increased significantly in transgenic alfalfa, and the plants that overexpressed the gene were better able to tolerate salt and drought resistance (Wang et al., 2021b). The overexpression of miR171f in rice resulted in reduced drought symptoms in transgenic plants compared with the control plants, which were found to play a role in drought tolerance by targeting SCL6-I and SCL6-II (Taeyoung et al., 2022). Sly-miR159 targets SlMYB33 to regulate the accumulation of proline and putrescine to improve drought resistance (López et al., 2019). The data described above indicate that the sRNA sequencing results of A. mongolicum under drought stress are authentic and reliable.
In the WGCNA analysis, the unearthed potential drought-resistant hub genes (MADS47, CCX1, carC, PAO2, and HOX24) targeted five miRNAs, which were osa-miR444a-3p.2, bdi-miR408-5p_1ss19TA, tae-miR9774_L-2R-1_1ss11GT, ata-miR169a-3p, and bdi-miR528-p3_2ss15TG20CA. Further study of the function of these miRNA targets provides valuable information about the regulatory pathways available for any given gene. The bdi-miR528-p3_2ss15TG20CA expression of HOX24 may activated the ABA−activated signaling pathway. osa-miR444a-3p.2 was differentially expressed in A. mongolicum under drought stress (p ≤ 0.001) and predicted to regulate MADS47 target genes. The regulation of MADS47 by osa-miR444a-3p.2a may affect the brassinosteroid-mediated signaling pathway. Furthermore, the redox process may regulate by ata-miR169a-3p and miR9774, and sodium and potassium ion transport may regulate by bdi-miR408-5p_1ss19TA.
miR444 is specific to monocots and has been confirmed to target four MIKC-type MADS-box genes in rice (OsMADS23, OsMADS27a, OsMADS27b, and OsMADS57) (Sunkar et al., 2005; Lu et al., 2008; Wu et al., 2009; Li et al., 2010). Recent research provides evidence of a nitrate-dependent miR444-OsMADS27 signaling cascade involved in the regulation of root growth in rice, as well as its dramatic role in stress responses (Kannan et al., 2022). The overexpression of miR444 promotes the biosynthesis of brassinosteroids (BRs) and inhibits the elongation of roots in rice (Jiao et al., 2020). In previous research, few studies have reported on miR9774, which is found in the mixed tissues of wheat leaves, stems, roots, and young spikes (Wei et al., 2009). In the low Cd-accumulation of wheat (Chuanyu 17), tae-miR-9,774 downregulated the expression between the group treated with 100 μM CdCl2 and the control group (Zhou et al., 2020). miR408, a conserved and ancient miRNA found widely in plants, is involved in photosynthesis and controls the target genes of copper-binding proteins (Gao et al., 2022), which is vital for leaf growth. miR408 has been linked to drought and water shortage stress in A. thaliana, rice (Roseeta et al., 2013; Sonia et al., 2017), wheat (Guray et al., 2016), tomato (Lycopersicon esculentum; Bilgin et al., 2016), and perennial ryegrass (Lolium perenne; Nan et al., 2020). The antioxidant capability of plants can be improved by miR408, which enhances cellular antioxidants (Gao et al., 2022). In chickpea (Cicer arietinum), the expression of PLC (a target of miR408) decreased significantly with the overexpression of miR408 to provide drought tolerance (Hajyzadeh et al., 2015). When A. thaliana was exposed to drought stress, miR408 was upregulated, while its target genes PLC and LAC3 were downregulated (Chao et al., 2015). miR169 was identified for its involvement in drought stress in A. thaliana (Du et al., 2017b), poplar (Populus spp.), rape (Brassica napus), and potato (Solanum tuberosum; Yang et al., 2016) crops. For example, in response to drought and ABA treatment, Bna-miR169n targets BnaNF-YA9 in Brassica napus (Wang et al., 2022). PtmiR169o targets the PtNF-YA6 gene to regulate drought tolerance in poplar (Jiao et al., 2021). More research into miR169-targeted PAO2-regulated drought is required. miR528 is involved in biotic stresses in plants, such as drought tolerance and salt tolerance stress. In rice, the overexpression of TCONS_00021861 attenuated the repression of miR528-3p on YUCCA7, which, in turn, activated the indole acetic acid (IAA) biosynthetic pathway and conferred resistance to drought stress (Chen et al., 2021). Durum wheat ata-miR528-5p promotes redox homeostasis by targeting the F-box protein and Cu Zn superoxide dismutase (Cu Zn SOD) under stress (Liu et al., 2020). These findings suggest that the known miRNAs are likely to be engaged in cross-adaptation to control plant tolerance to abiotic conditions, such as drought. However, their specific duties and exact functions need to be verified in the future.
It is impossible to extrapolate the complete picture of a complex environmental component, such as drought regulation, from the effects of individual genes. A combination of transcriptome, degradome and miRNAs expression profiles is needed to elucidate the synergistic impact of drought-related genes (Liu et al., 2020). A total of 41,792 UniGenes (Table 2) were detected in the A. mongolicum transcriptome. Under specific abiotic stresses, such as drought stress, mRNAs have a particular expression in response to the pressure. Thus, there were 1,166 DEGs in the seven comparison groups under drought stress (Figure 2). The number of up- and downregulated genes also differed among the various comparison groups. The level of gene expression could differ owing to the varying drought treatment times. GO classification to drought-related GO terms include response to salt stress, response to ABA and response to wounding (Figure 1A). The degradome data identified 137 A. mongolicum-specific miRNAs that target 1,394 genes (Supplementary Table 9). The integrated analysis of transcriptome, sRNAs and degradome that focused on miRNAs and their target genes in negative regulatory mode, identified 91 miRNAs that negatively regulate 1,438 target genes. Some DEGs were commonly up- or downregulated under drought stress, while others exhibited unique patterns. In A. mongolicum, we observed that eight novel miRNAs with different expression patterns regulated 36 target genes. The 36 target genes that are controlled (Figure 4; Supplementary Table 10) were annotated under drought stress as uncharacterized oxidoreductase, magnesium-chelatase subunit CHLH chloroplastic, NADP-thioredoxin reductase C precursor, chloroplast ferredoxin-dependent glutamate synthase, and heat shock protein-interacting protein (Table 3). This study showed that all these functional annotations are involved in drought stress regulation. For example, the Chloroplastic, Magnesium Protoporphyrin-IX Chelatase H subunit (CHLH) receptor has been found to bind ABA, and CHLH is a crucial enzyme in chlorophyll production (Wasilewska et al., 2008; Zhang et al., 2019). NADP has been shown to regulate the maintenance of redox homeostasis and respond to environmental stresses in plants (Wang et al., 2020). According to the findings, drought stress is regulated in plants by a variety of pathways, including chlorophyll and ABA regulation. Plants that respond to drought stress could also activate pathways to other stresses, such as salt stress or heat stress, or some of the pathways could be shared by plants that respond to biotic stresses.
To identify the highly putative genes in drought responses in A. mongolicum, 41,792 mRNAs were analyzed using WGCNA technology based on transcriptome sequencing data to study the hub genes and co-expression networks in response to drought stress. A total of 39 co-expression modules were constructed, and four modules related to drought resistance were enriched for signal transduction, brassinosteroid mediated signaling pathway, and the ABA−activated signaling pathway (Figure 7). These enriched functions were closely related to drought stress. MADS47, CCX1, carC, PAO2, and HOX24 were five hub genes with putative drought tolerance (Figure 8). MADS47 is a MADS transcription factor, which plays essential functions in plant growth and development and response to adversity stress (Natalia et al., 2019; Zhao et al., 2020; Han et al., 2022a). OsMADS2, OsMADS30, and OsMADS55 were downregulated in expression under drought and salt stress in rice (Rita et al., 2007). However, the RT-qPCR results of A. mongolicum showed that the relative level of expression of MADS47 was upregulated in drought stress. In rice, OsMADS26 plays a negative regulatory role and reduces the drought resistance of the plant, which is the same regulatory pattern as MADS47 in A. mongolicum. MADS47 is a hub gene in the regulatory network and is functionally shown to be associated with the BR-mediated signaling pathway. BRs are plant hormones that promote growth, improve plant stress resistance, and regulate the level of expression of chlorophyll synthase genes (Ryo et al., 2022). BR can promote wound healing by activating reactive oxygen metabolism (Han et al., 2022b).
The HOX24 annotation information is related to ABA and water deficit regulation. A Homeo-Leucine Zipper (HD-Zip) is a class of transcription factors that is unique to higher plants and involved in stress response and the growth and development of plants. ATHB-6 is the HD-zip gene of maize (Zea mays), and the overexpression of ATHB-6 in maize activates the expression of critical genes in the ROS signaling pathway and ABA-dependent pathway under drought tolerance (Peng et al., 2022). The annotated function of HOX24 in sugarcane (Saccharum officinarum) under drought stress is related to antioxidants (Peiting et al., 2022). Both MADS47 and HOX24 in A. mongolicum are hub genes in the violet module, and they are mutually regulated and have genes that act together. Their functions are annotated as enzymatic activity, oxidation–reduction and cell surface receptor signaling pathway. The ABA hormone and BRs are the primary regulatory molecules that respond to drought signals and convey them down to the reciprocal genes that they regulate in the MADS47- and HOX24-mediated regulatory network, after which they exert drought regulation in the form of a network.
CCX1 is a member of the Cation/Ca2+ Exchanger family. The overexpression of CCX1 in A. thaliana increased the sensitivity to hydrogen peroxide (H2O2) treatment, suggesting that this gene is involved in ROS homeostasis (Li et al., 2016). Na+, K+, and Ca2+ can retard the senescence of broccoli (Brassica oleracea var. italica) buds. The CCX1 promoter was cloned in broccoli and found to inhibit its senescence. The functional annotation of CCX1 in A. mongolicum under drought stress is sodium-potassium ion transport (Yan et al., 2020). Previous studies have confirmed the regulatory function of CCX1 from the side. In the co-expression regulatory network of CCX1, which regulates genes with functions in oxidation–reduction and ion binding, protein ubiquitination regulation interacts synergistically in response to drought stress.
Polyamines (PA) are key components of plant growth and development and abiotic stress responses (EAlcázar and Tiburcio, 2014; Wimalasekera et al., 2015). Amine oxidases oxidatively catabolize PAs, and polyamine (PAO) oxidase is one of the breakdown products (Angelini et al., 2010). PAO2 is annotated as regulating the redox process and peroxisome in A. mongolicum. In A. thaliana, AtPAO2 is differentially expressed in the interaction of ABA, nitrate and ammonium ions, which can potentially improve the ability to regulate the growth of A. thaliana roots (Wimalasekera et al., 2015). carC is a regulatory gene and could play an active role in the production of carotene (Revuelta and Eslava, 1983). CarC is annotated as hydrolase activity in A. mongolicum. The carC coexpression network revealed that it primarily regulates chloroplast and redox genes. There are few reports about the role of carC gene in plants. As a result, more research is needed on the mechanism of the drought-resistant regulation of the carC gene in A. mongolicum. In conclusion, these studies show that MADS47, CCX1, carC, PAO2, and HOX24 genes in drought may play an essential role in A. mongolicum under drought stress and can be used as suitable hub genes for drought resistance in A. mongolicum.
Conclusion
This study provides the first integrated analysis of A. mongolicum miRNAs and mRNAs regulated at the transcriptional level in response to drought stress. The multi-layered stress-responsive networks mediated by miRNAs and their hub target genes are no doubt highly complex but are coordinated. Five potential drought tolerance hub genes (MADS47, CCX1, carC, PAO2, and HOX24) were targeted by the analysis and the regulatory network was mapped. The study provides a theory for the functional validation of drought resistance hub genes in A. mongolicum. At the same time, new findings would lay the foundations for improving drought tolerance in gramineous crops via molecular breeding strategies.
Data availability statement
The original contributions presented in the study are publicly available. This data can be found at: NCBI, PRJNA742257.
Author contributions
BF conducted the experiments. YM organized and supervised the overall project. BF, FS, ZY, XZ, XY, JW, XY, LN, and YF performed the editing of the manuscript. YZ provided the seeds of A. mongolicum. All authors contributed to the article and approved the submitted version.
Funding
This study was funded by the National Natural Science Foundation of China (No. 31860670).
Conflict of interest
The authors declare that the research was conducted in the absence of any commercial or financial relationships that could be construed as a potential conflict of interest.
Publisher’s note
All claims expressed in this article are solely those of the authors and do not necessarily represent those of their affiliated organizations, or those of the publisher, the editors and the reviewers. Any product that may be evaluated in this article, or claim that may be made by its manufacturer, is not guaranteed or endorsed by the publisher.
Supplementary material
The Supplementary material for this article can be found online at: https://www.frontiersin.org/articles/10.3389/fpls.2022.976684/full#supplementary-material
Footnotes
1. ^http://www.bioinformatics.babraham.ac.uk/projects/fastqc/ (Accessed August 6, 2022).
2. ^https://github.com/trinityrnaseq/trinityrnaseq/wiki (Accessed August 6, 2022).
3. ^http://www.ncbi.nlm.nih.gov/ (Accessed August 6, 2022).
4. ^http://www.geneontology.org (Accessed August 6, 2022).
5. ^http://www.expasy.ch/sprot/ (Accessed August 6, 2022).
6. ^http://www.genome.jp/kegg/ (Accessed August 6, 2022).
7. ^http://eggnogdb.embl.de/ (Accessed August 6, 2022).
8. ^https://horvath.genetics.ucla.edu/coexpression network/ (Accessed August 6, 2022).
References
Abid, U., Heng, S., Xiyan, Y., and Zhang, X. L. (2017). Drought coping strategies in cotton: increased crop per drop. Plant Biotechnol. J. 15, 271–284. doi: 10.1111/pbi.12688
Addo-Quaye, C., Snyder, J. A., Park, Y. B., Li, Y.-F., Sunkar, R., and Axtell, M. J. (2009). Sliced microRNA targets and precise loop-first processing of miR319 hairpins revealed by analysis of the physcomitrella patens degradome. RNA 15, 2112–2121. doi: 10.1261/rna.1774909
Ali, N., Shah, F., Muhammad, A., Usman, A., Amanullah,, Sumera, A., et al. (2017). miRNAs: major modulators for crop growth and development under abiotic stresses. Biotechnol. Lett. 39, 685–700. doi: 10.1007/s10529-017-2302-9
Allen, E., Xie, Z., Gustafson, A. M., and Carrington, J. C. (2005). microRNA-Directed Phasing during Trans-Acting siRNA Biogenesis in Plants. Cell 121, 207–221. doi: 10.1016/j.cell.2005.04.004
Andrew, D. B. L., Ainsworth Elizabeth, A., Bernacchi Carl, J., Rogers, A., and Ort Donald, O. D. (2009). Elevated CO2 effects on plant carbon, nitrogen, and water relations: six important lessons from face. J. Exp. Bot. 60, 2859–2876. doi: 10.1093/jxb/erp096
Angelini, R., Cona, A., Federico, R., Fincato, P., Tavladoraki, P., and Tisi, A. (2010). Plant amine oxidases “on the move”: an update. Plant Physiol. Biochem. 48, 560–564. doi: 10.1016/j.plaphy.2010.02.001
Bano, N., Fakhrah, S., Mohanty, C. S., and Bag, S. K. (2022). Transcriptome meta-analysis associated targeting hub genes and pathways of drought and salt stress responses in cotton (gossypium hirsutum): a network biology approach. Front. Plant Sci. 13:818472. doi: 10.3389/FPLS.2022.818472
Berninger, P., Gaidatzis, D., Nimwegen, E. V., and Zavolan, M. (2008). Computational analysis of small RNA cloning data. Methods 44, 13–21. doi: 10.1016/j.ymeth.2007.10.002
Bilgin, C. C., Ercan, A., and Baohong, Z. (2016). Small RNA and degradome deep sequencing reveals drought-and tissue-specific micrornas and their important roles in drought-sensitive and drought-tolerant tomato genotypes. Plant Biotechnol. J. 14, 1727–1746. doi: 10.1111/pbi.12533
Bin, T., Yusun, S., Aaron, N., Xia, L., Jie, Z., Aiqin, W., et al. (2021). Integration of small RNA and degradome sequencing reveals the regulatory network of al-induced programmed cell death in peanut. Int. J. Mol. Sci. 23, 246–264. doi: 10.3390/ijms23010246
Boyer, J. S. (1982). Plant productivity and environment. Science 218, 443–448. doi: 10.1126/science.218.4571.443
Buchfink, B., Chao, X., and Daniel, H. H. (2014). Fast and sensitive protein alignment using DIAMOND. Nat. Methods 12, 59–60. doi: 10.15496/publikation-1176
Carrington, J. C., and Ambros, V. (2003). Role of microRNAs in plant and animal development. Science 301, 336–338. doi: 10.1126/science.1085242
Chandra, P., Wunnava, A., Verma, P., Chandra, A., and Sharma, R. K. (2021). Strategies to mitigate the adverse effect of drought stress on crop plants-influences of soil bacteria: a review. Pedosphere 31, 496–509. doi: 10.1016/S1002-0160(20)60092-3
Chao, M., Shaul, B., and Amnon, L. (2015). miR408 is involved in abiotic stress responses in Arabidopsis. Plant J. 84, 169–187. doi: 10.1111/tpj.12999
Che, Y. H., and Li, L. H. (2007). Genetic diversity of prolamines in Agropyron mongolicum Keng indigenous to northern China. Genet. Resour. Crop. Evol. 54, 1145–1151. doi: 10.1007/s10722-006-9006-7
Chen, J., Zhong, Y., and Qi, X. (2021). LncRNA TCONS_00021861 is functionally associated with drought tolerance in rice (Oryza sativa L.) via competing endogenous RNA regulation. BMC Plant Biol. 21, 410–422. doi: 10.1186/s12870-021-03195-z
David, W., and Franck, V. (2011). miR393: integrator of environmental cues in auxin signaling plant signaling &. Behavior 6, 1672–1675. doi: 10.4161/psb.6.11.17900
Du, J., Li, X., Li, T., Yu, D., and Han, B. (2017a). Genome-wide transcriptome profiling provides overwintering mechanism of Agropyron mongolicum. BMC Plant Biol. 17, 138–151. doi: 10.1186/s12870-017-1086-3
Du, Q., Zhao, M., Gao, W., Sun, S., and Li, W. (2017b). microRNA/microRNA* complementarity is important for the regulation pattern of NFYA5 by miR169 under dehydration shock in Arabidopsis. Plant J. 91, 22–33. doi: 10.1111/tpj.13540
EAlcázar, R., and Tiburcio, A. F. (2014). Plant polyamines in stress and development: an emerging area of research in plant sciences. Front. Plant Sci. 5:319. doi: 10.3389/fpls.2014.00319
Elena, M., Virginie, J., Aurélie, H., Lokerse, A. S., Dolf, W., Herve, V., et al. (2010). miR390, Arabidopsis TAS3 tasiRNAs, and their auxin response factor targets define an autoregulatory network quantitatively regulating lateral root growth. Plant Cell 22, 1104–1117. doi: 10.1105/tpc.109.072553
Esfahanian, E., Nejadhashemi, A. P., Abouali, M., Adhikari, U., Zhang, Z., Daneshvar, F., et al. (2017). Development and evaluation of a comprehensive drought index. J. Environ. Manag. 185, 31–43. doi: 10.1016/j.jenvman.2016.10.050
Flynt, A., and Lai, E. (2008). Biological principles of microRNA-mediated regulation: shared themes amid diversity. Nat. Rev. Genet. 9, 831–842. doi: 10.1038/nrg2455
Frank, J. S., Michael, B., Zhongchi, L., Victor, A., Robert, H., and Ruvkun, G. (2000). The lin-41 RBCC gene acts in the C. elegans heterochronic pathway between the let-7 regulatory RNA and the LIN-29 transcription factor. Mol. Cell 5, 659–669. doi: 10.1016/S1097-2765(00)80245-2
Gao, Y., Baohua, F., Caixia, G., Huiquan, Z., Fengting, W., Longxing, T., et al. (2022). The evolution and functional roles of miR408 and its targets in plants. Int. J. Mol. Sci. 23, 530–555. doi: 10.3390/IJMS23010530
Gereziher, M. T., Yanchao, X., Jawad, U. M., Linyerera, S. M., Yuqing, H., Yuhong, W., et al. (2021). Multi-omics-based identification and functional characterization of Gh_A06G1257 proves its potential role in drought stress tolerance in gossypium hirsutum&13. Front. Plant Sci. 12:746771. doi: 10.3389/FPLS.2021.746771
Grabherr, M. G., Haas, B. J., Yassour, M., Levin, J. Z., Thompson, D. A., Amit, I., et al. (2011). Full-length transcriptome assembly from RNA-Seq data without a reference genome. Nat. Biotechnol. 29, 644–652. doi: 10.1038/nbt.1883
Guray, A., Derelli, T. E., Serkan, U., and Turgay, U. (2016). miRNA-based drought regulation in wheat. Funct. Integr. Genomics 16, 221–233. doi: 10.1007/s10142-015-0452-1
Hajyzadeh, M., Turktas, M., Khawar, K. M., and Unver, T. (2015). miR408 overexpression causes increased drought tolerance in chickpea. Gene 555, 186–193. doi: 10.1016/j.gene.2014.11.002
Han, Y. C., Liu, N., Li, C., Wang, S., Jia, L., Zhang, R., et al. (2022a). TaMADS2-3D, a MADS transcription factor gene, regulates phosphate starvation responses in plants. Crop J. 10, 243–253. doi: 10.1016/J.CJ.2021.03.020
Han, H., Liu, W., Lu, Y., Zhang, J., Yang, X., Li, X., et al. (2017). Isolation and application of P genome-specific DNA sequences of Agropyron Gaertn in Triticeae. Planta 245, 425–437. doi: 10.1007/s00425-016-2616-1
Han, Y., Yang, R., Zhang, X., Wang, Q., Wang, B., Zheng, X., et al. (2022b). Brassinosteroid accelerates wound healing of potato tubers by activation of reactive oxygen, metabolism and phenylpropanoid metabolism. Foods 11, 906–921. doi: 10.3390/FOODS11070906
Han, X., Yin, H., Song, X., Zhang, Y., Liu, M., Sang, J., et al. (2016). Integration of small RNAs, degradome and transcriptome sequencing in hyperaccumulator sedum alfredii uncovers a complex regulatory network and provides insights into cadmium phytoremediation. Plant Biotechnol. J. 14, 1470–1483. doi: 10.1111/pbi.12512
Hollender, C. A., Chunying, K., Omar, D., Aviva, G., Matthews, B. F., Janet, S., et al. (2014). Floral transcriptomes in woodland strawberry uncover developing receptacle and anther gene networks. Plant Physiol. 165, 1062–1075. doi: 10.1104/pp.114.237529
Jiang, D., Chen, W., Dong, J., Li, J., and Fen, Y. (2018). Overexpression of miR164b-resistant OsNAC2 improves plant architecture and grain yield in rice. J. Exp. Bot. 69, 1533–1543. doi: 10.1093/jxb/ery017
Jiao, Z., Lian, C., Han, S., Huang, M., Shen, C., Li, Q., et al. (2021). PtmiR169o plays a positive role in regulating drought tolerance and growth by targeting the PtNF-YA6 gene in poplar. Environ. Exp. Bot. 189, 104549–104563. doi: 10.1016/j.envexpbot.2021.104549
Jiao, X., Wang, H., Yan, J., Kong, X., Liu, Y., Chu, J., et al. (2020). Promotion of BR biosynthesis by miR444 is required for ammonium-triggered inhibition of root growth. Plant Physiol. 182, 1454–1466. doi: 10.1104/pp.19.00190
Kannan, P., Vivek, H. S., Anushree, N., Singh, R. R., Soumita, D., Avik Pal, H. C. Y., et al. (2022). Nitrate-dependent regulation of miR444-OsMADS27 signaling cascade controls root development in rice. J. Exp. Bot. 73, 3511–3530. doi: 10.1093/jxb/erac083
Langfelder, P., and Horvath, S. (2008). WGCNA: an R package for weighted correlation network analysis. BMC Bioinform. 9, 559–572. doi: 10.1186/1471-2105-9-559
Li, Z., Wang, X., Chen, J., Gao, J., Zhou, X., and Kuai, B. (2016). CCX1, a putative cation/Ca2+ exchanger, participates in regulation of reactive oxygen species homeostasis and leaf senescence. Plant Cell Physiol. 57, 2611–2619. doi: 10.1093/pcp/pcw175
Li, Y., Zheng, Y., Charles, A., Li, Z., Ajay, S., Guru, J., et al. (2010). Transcriptome-wide identification of microRNA targets in rice. Plant J. 62, 742–759. doi: 10.1111/J.1365-313X.2010.04187.X
Liu, H., Amanda, J., and Jason, A. (2020). Integrated analysis of small RNA, transcriptome, and degradome sequencing reveals the water-deficit and heat stress response network in durum wheat. Int. J. Mol. Sci. 21, 2260–2285. doi: 10.3390/ijms21176017
Livak, K. J., and Schmittgen, T. D. (2001). Analysis of relative gene expression data using real-time quantitative PCR and the 2(-Delta Delta C(T)) method. Methods 25, 402–408. doi: 10.1006/meth.2001.1262
Llave, C., Xie, Z., Kristin, D. K., and James, C. (2002). Cleavage of scarecrow-like mRNA targets directed by a class of Arabidopsis miRNA. Science 297, 2053–2056. doi: 10.1126/science.1076311
López, G. M. J., García, R. I., González-Hernández, A. I., Camañes, G., Vicedo, B., Real, M. D., et al. (2019). Expression of miR159 is altered in tomato plants undergoing drought stress. Plan. Theory 8, 201–212. doi: 10.3390/plants8070201
Lu, C., Donghoon, J., Karthik, K., Manoj, P., Kan, N., Rana, G., et al. (2008). Genome-wide analysis for discovery of rice microRNAs reveals natural antisense microRNAs (nat-miRNAs). Proc. Natl. Acad. Sci. U. S. A. 105, 4951–4956. doi: 10.1073/pnas.0708743105
Ma, Z., Coruh, C., and Axtell, M. J. (2010). Arabidopsis lyrata small RNAs: transient miRNA and small interfering RNA loci within the Arabidopsis genus. Plant Cell 22, 1090–1103. doi: 10.2307/25680120
Martin, M. (2011). Cut adapt removes adapter sequences from high-throughput sequencing reads. EMBnet J. 17, 10–12. doi: 10.14806/ej.17.1.200
Meng, Y., Shao, C., and Chen, M. (2011). Toward microRNA-mediated gene regulatory networks in plants. Brief. Bioinform. 12, 645–659. doi: 10.1093/bib/bbq091
Mortazavi, A., Williams, B. A., McCue, K., Schaeffer, L., and Wold, B. (2008). Mapping and quantifying mammalian transcriptomes by RNA-Seq. Nat. Methods 5, 621–628. doi: 10.1038/nmeth.1226
Mutz, K., Heilkenbrinker, A., Lönne, M., Walter, J., and Stahl, F. (2013). Transcriptome analysis using next-generation sequencing. Curr. Opin. Biotechnol. 24, 22–30. doi: 10.1016/j.copbio.2012.09.004
Nan, H., Tianran, S., Yanrong, L., Wenxin, Y., Geli, T., Yan, S., et al. (2020). Overexpression of Os-microRNA408 enhances drought tolerance in perennial ryegrass. Physiol. Plant. 172, 733–747. doi: 10.1111/ppl.13276
Natalia, C.-M., Joel, H., Wendy, C.-S., Maite, A., et al. (2019). MADS-box genes are key components of genetic regulatory networks involved in abiotic stress and plastic developmental responses in plants. Front. Plant Sci. 10:853. doi: 10.3389/fpls.2019.00853
Niu, S., Fan, G., Deng, M., Zhao, Z., and Dong, Y. (2014). Transcriptome/degradome-wide discovery of microRNAs and transcript targets in two Paulownia australis genotypes. PLoS One 9, 106736–106747. doi: 10.1371/journal.pone.0106736
Niu, J., Wang, J., An, J., Liu, L., and Lin, Z. (2016). Integrated mRNA and miRNA transcriptome reveal a cross-talk between developing response and hormone signaling for the seed kernels of Siberian apricot. Sci. Rep. 6, 35675–33587. doi: 10.1038/srep35675
Oladosu, Y., Rafii, M. Y., Samuel, C., Fatai, A., Magaji, U., Kareem, I., et al. (2019). Drought resistance in rice from conventional to molecular breeding: a review. Int. J. Mol. Sci. 20, 3519–3540. doi: 10.3390/ijms20143519
Patro, R., Duggal, G., Love, M. I., Irizarry, R. A., and Kingsford, C. (2017). Salmon provides fast and bias-aware quantification of transcript expression. Nat. Methods 14, 417–419. doi: 10.1038/nmeth.4197
Peiting, L., Pingping, L., Zhenli, Z., Zihong, L., Yanming, L., Chaohua, H., et al. (2022). Gene co-expression analysis reveals transcriptome divergence between wild and cultivated sugarcane under drought stress. Int. J. Mol. Sci. 23, 569–592. doi: 10.3390/IJMS23010569
Peng, J., Jiang, Z., Wei, X., Liu, S., Qu, J., Guan, S., et al. (2022). Overexpression of the homeobox-leucine zipper protein athb-6 improves the drought tolerance of maize (Zea mays L.). Plant Sci. 316, 111159–111171. doi: 10.1016/j.plantsci.2021.111159
Qiu, Z., Yan, Y., Zhang, Y., Guo, J., and Li, W. (2018). Characterization of miRNAs and their target genes in he-ne laser pretreated wheat seedlings exposed to drought stress. Ecotoxicol. Environ. Saf. 164, 611–617. doi: 10.1016/j.ecoenv.2018.08.077
Revuelta, J. L., and Eslava, A. P. (1983). A new gene (carC) involved in the regulation of carotenogenesis in Phycomyces. Mol. Genet. Genom. 192, 225–229. doi: 10.1007/BF00327670
Reyes, J. L., and Nam-Hai, C. (2007). ABA induction of miR159 controls transcript levels of two MYB factors during Arabidopsis seed germination. Plant J. 49, 592–606. doi: 10.1111/j.1365-313X.2006.02980.x
Rita, A., Pinky, A., Swatismita, R., Ashok, S., Vijay, S., Akhilesh, T., et al. (2007). MADS-box gene family in rice: genome-wide identification, organization and expression profiling during reproductive development and stress. BMC Genomics 8, 242–263. doi: 10.1186/1471-2164-8-242
Roseeta, D. M. C. B. S., Shivani, K., Preeti, A., Santosh, K., Mukesh, K., et al. (2013). Evolution of variety-specific regulatory schema for expression of osa-miR408 in indica rice varieties under drought stress. FEBS J. 280, 1717–1730. doi: 10.1111/febs.12186
Ryo, T., Ayumi, Y., Shino, M., Miki, N., Minami, M., Masaaki, S., et al. (2022). Brz-insensitive-pale green 1 is encoded by chlorophyll biosynthesis enzyme gene that functions in the downstream of brassinosteroid signaling. Biosci. Biotechnol. Biochem. 86, 1041–1048. doi: 10.1093/bbb/zbac071
Shi, G. Q., Jingying, F., Lingjie, R., Peiyue, Z., Chengjin, G., and Kai, X. (2018). TaMIR1119, a miRNA family member of wheat (Triticum aestivum), is essential in the regulation of plant drought tolerance. J. Integr. Agric. 17, 2369–2378. doi: 10.1016/S2095-3119(17)61879-3
Shubha, V., and Tyagi, A. K. (2010). Emerging trends in the functional genomics of the abiotic stress response in crop plants. Plant Biotechnol. J. 5, 361–380. doi: 10.1111/j.1467-7652.2007.00239.x
Smyth, G. K. (2010). Edge R: a bioconductor package for differential expression analysis of digital gene expression data. Bioinformatics 26, 139–140. doi: 10.1093/bioinformatics/btp616
Song, X., Yan, X., and Cao, Y. (2019). MicroRNAs and their regulatory roles in plant-environment interactions. Annu. Rev. Plant Biol. 70, 489–525. doi: 10.1146/annurev-arplant-050718-100334
Sonia, B., Mukesh, K., Devi, M. R., Utkarsh, R., Priyanka, A., Saloni, M., et al. (2017). Identification of miRNA-mediated drought responsive multi-tiered regulatory network in drought tolerant rice, Nagina 22. Sci. Rep. 7, 15446–15463. doi: 10.1038/s41598-017-15450-1
Sunkar, R., Girke, T., Kumar, J. P., and Zhu, J. K. (2005). Cloning and characterization of microRNAs from rice. Plant Cell 17, 1397–1411. doi: 10.1105/tpc.105.031682
Taeyoung, U., Joohee, C., Taehyeon, P., Joong, C. P., Eun, J. S., Sung, S. J., et al. (2022). Rice microRNA171f/SCL6 module enhances drought tolerance by regulation of flavonoid biosynthesis genes. Plant Direct. 6:e374. doi: 10.1002/PLD3.374
Taier, G., Hang, N., Shi, T., Liu, Y., Ye, W., Zhang, W., et al. (2021). Ectopic expression of Os-miR408 improves thermo-tolerance of perennial ryegrass. Agronomy 11, 1930–1943. doi: 10.3390/AGRONOMY11101930
Wang, M., Guo, W., Li, J., Pan, X., Pan, L., Zhao, J., et al. (2021b). The miR528-AO module confers enhanced salt tolerance in rice by modulating the ascorbic acid and abscisic acid metabolism and ROS scavenging. J. Agric. Food Chem. 69, 8634–8648. doi: 10.1021/ACS.JAFC.1C01096
Wang, X., Li, B., Ma, T., Sun, L., Li, T., Hu, C., et al. (2020). The NAD kinase OsNADK1 affects the intracellular redox balance and enhances the tolerance of rice to drought. BMC Plant Biol. 20, 11–30. doi: 10.1186/s12870-019-2234-8
Wang, X., Liu, Y., Han, Z., Chen, Y., Huai, D., Kang, Y., et al. (2021c). Integrated transcriptomics and metabolomics analysis reveal key metabolism pathways contributing to cold tolerance in Peanut&13. Front. Plant Sci. 12:752474. doi: 10.3389/FPLS.2021.752474
Wang, K., Liu, Y., Teng, F., Cen, H., Yan, J., Lin, S., et al. (2021a). Heterogeneous expression of Osa-MIR156bc increases abiotic stress resistance and forage quality of alfalfa. Crop J. 9, 1135–1144. doi: 10.1016/j.cj.2020.11.009
Wang, J., Zhou, Z., Tao, Q., Chen, S., Ren, X., Yu, L., et al. (2022). Brassica napus miR169 regulates BnaNF-YA in salinity, drought and ABA responses. Environ. Exp. Bot. 199:104882. doi: 10.1016/J.ENVEXPBOT.2022.104882
Wasilewska, A., Vlad, F., Sirichandra, C., Redko, Y., Jammes, F., Valon, C., et al. (2008). An update on abscisic acid signaling in plants and more. Molecular Plant 1, 198–217. doi: 10.1093/mp/ssm022
Wei, B., Tao, C., Zhang, R., Li, A., Huo, N., Li, S., et al. (2009). Novel microRNAs uncovered by deep sequencing of small RNA transcriptomes in bread wheat (Triticum aestivum L.) and Brachypodium distachyon (L.) Beauv. Funct. Integr. Genomics 9, 499–511. doi: 10.1007/s10142-009-0128-9
Wimalasekera, R., Schaarschmidt, F., Angelini, R., Cona, A., Tavladoraki, P., and Scherer, G. F. E. (2015). Polyamine oxidase2 of Arabidopsis contributes to ABA mediated plant developmental processes. Plant Physiol. Biochem. 96, 231–240. doi: 10.1016/j.plaphy.2015.08.003
Wu, L., Zhang, Q., Zhou, H., Ni, F., Wu, X., and Qi, Y. (2009). Rice MicroRNA effector complexes and targets. Plant Cell 21, 3421–3435. doi: 10.1105/tpc.109.070938
Xu, X., Yin, L., Ying, Q., Song, H., Xue, D., Lai, T., et al. (2013). High-throughput sequencing and degradome analysis identify miRNAs and their targets involved in fruit senescence of Fragaria ananassa. PLoS One 8, e70959–e70969. doi: 10.1371/journal.pone.0070959
Yan, K., Ran, M., Li, S., Zhang, J., Wang, Y., Wang, Z., et al. (2020). The delayed senescence of postharvest buds in salt ions was related to antioxidant activity, HDA9 and CCX1 in broccoli (Brassica oleracea L. var. italic planch). Food Chem. 324, 126887–126896. doi: 10.1016/j.foodchem.2020.126887
Yang, X., Wang, L., Yuan, D., Lindsey, K., and Zhang, X. (2013). Small RNA and degradome sequencing reveal complex miRNA regulation during cotton somatic embryogenesis. J. Exp. Bot. 64, 1521–1536. doi: 10.1093/jxb/ert013
Yang, J., Zhang, N., Zhou, X., Si, H., and Wang, D. (2016). Identification of four novel stu-miR169s and their target genes in Solanum tuberosum and expression profiles response to drought stress. Plant Syst. Evol. 302, 55–66. doi: 10.1007/s00606-015-1242-x
Yin, F. Q., Cheng, Q., Gao, J., Liu, M., Luo, X. R., Zhang, W. Y., et al. (2015). Genome-wide identification and analysis of drought-responsive genes and microRNAs in tobacco. Int. J. Mol. Sci. 16, 5714–5740. doi: 10.3390/ijms16035714
Zhang, X., Fan, B., Yu, Z., Nie, L., Zhao, Y., Yu, X., et al. (2019). Functional analysis of three miRNAs in Agropyron mongolicum Keng under drought stress. Agronomy 9, 661–686. doi: 10.3390/agronomy9100661
Zhang, B., Pan, X., Cox, S., Cobb, G., and Anderson, T. (2006). Evidence that miRNAs are different from other RNAs. Cellular and Molecular Life Sciences 63, 246–254. doi: 10.1007/s00018-005-5467-7
Zhao, C., Han, X., Taylor, F., Yao, Y., Bi, Y., Li, A., et al. (2010a). Deep sequencing identifies novel and conserved microRNAs in peanuts (Arachis hypogaea L.). BMC Plant Biol. 10, 3–12. doi: 10.1186/1471-2229-10-3
Zhao, P., Miao, Z., Zhang, J., Chen, S., Liu, Q., and Xiang, C. (2020). MADS-box factor AGL16 negatively regulates drought resistance via stomatal density and stomatal movement. J. Exp. Bot. 71, 6092–6106. doi: 10.1093/jxb/eraa303
Zhao, Y., Yun, J., Shi, F., Wang, J., Yang, Q., and Chao, Y. (2010b). Molecular cloning and characterization of a group 3 LEA gene from Agropyron mongolicum Keng. Afr. J. Biotechnol. 9, 69–82. doi: 10.1186/1475-2859-9-69
Zhou, M., Zheng, S., Li, Y., Liu, R., Zhang, L., and Wu, Y. (2020). Comparative profiling of roots small RNA expression and corresponding gene ontology and pathway analyses for low- and high-cadmium-accumulating genotypes of wheat in response to cadmium stress. Funct. Integr. Genomics 20, 177–190. doi: 10.1007/s10142-019-00710-2
Keywords: Agropyron mongolicum Keng, drought resistance, microRNAs, transcriptome, degradome, integration analysis, co-expression network
Citation: Fan B, Sun F, Yu Z, Zhang X, Yu X, Wu J, Yan X, Zhao Y, Nie L, Fang Y and Ma Y (2022) Integrated analysis of small RNAs, transcriptome and degradome sequencing reveal the drought stress network in Agropyron mongolicum Keng. Front. Plant Sci. 13:976684. doi: 10.3389/fpls.2022.976684
Edited by:
Jing Zhang, Nanjing Agricultural University, ChinaReviewed by:
Tushar Suhas Khare, Savitribai Phule Pune University, IndiaKe Teng, Beijing Academy of Agricultural and Forestry Sciences, China
Copyright © 2022 Fan, Sun, Yu, Zhang, Yu, Wu, Yan, Zhao, Nie, Fang and Ma. This is an open-access article distributed under the terms of the Creative Commons Attribution License (CC BY). The use, distribution or reproduction in other forums is permitted, provided the original author(s) and the copyright owner(s) are credited and that the original publication in this journal is cited, in accordance with accepted academic practice. No use, distribution or reproduction is permitted which does not comply with these terms.
*Correspondence: Yanhong Ma, bWF5YW5ob25nODBAMTI2LmNvbQ==