- 1Key Laboratory of Ecology and Environment in Minority Areas (Minzu University of China), National Ethnic Affairs Commission, Beijing, China
- 2College of Life and Environmental Sciences, Minzu University of China, Beijing, China
- 3Key Laboratory of Ecosystem Network Observation and Modeling, Institute of Geographic Sciences and Natural Resources Research, Chinese Academy of Sciences, Beijing, China
- 4Key Laboratory of Prevention and Control for Aquatic Invasive Alien Species, Pearl River Fisheries Research Institute, Chinese Academy of Fishery Sciences, Ministry of Agriculture and Rural Affairs, Guangzhou, China
- 5College of Grassland Science, Beijing Forestry University, Beijing, China
Introduction
The effects of climatic factors and soil nutrients in the determination of plant biomass production have long been of central interest to ecologists (Huxman et al., 2004; Fay et al., 2015). Recently, Radujković et al. (2021) found that soil micronutrients (particularly Zn and Fe) were important predictors of aboveground biomass production (Biomass). In their analyses, the combination of atmospheric factors that best explained the variation in Biomass was selected first; then, soil physicochemical properties, C-N-P nutrients, and other nutrients (K, Ca, Mg, Na, S, Zn, Fe, B, Cu, and Mn) were sequentially added to construct structural equation models (SEMs). However, the sequence of nutrient variable introduced to the model matters, and consequently influence the construction of the final best model. More importantly, although separately adding Zn and Fe to the SEM explained additional variations in Biomass, they grouped Zn and Fe into one composite variable and argued that both micronutrients were important predictors of Biomass given the significant effect of the composite variable. However, they did not examine whether it was appropriate to simultaneously include both Zn and Fe in the model. Here, we re-analyzed the dataset using multiple statistical methods and revealed that the SEM incorporating both micronutrients (including both Zn and Fe) as was done by the original paper was not the best model.
Data analysis
In the final model of Radujković et al. (2021) (Figure 1A), seven environmental variables were used to explain the variation in Biomass. We first used these seven variables to fit a multiple linear model: lm (Biomass ~ MAPgs + Ndep + CEC + SOM + C:N + Fe + Zn). We found that Fe and SOM had no significant effects on Biomass. To further demonstrate this, we used these seven variables to construct an SEM. This SEM was similar to Radujković et al. (2021) final SEM but without composite variables, which showed that Fe and SOM had no significant direct effects on Biomass (Figure 1B). After removing these two paths from the model, we found that the SEM was significantly improved, with a lower AICc value (Figure 1C). These results indicate that the final SEM of Radujković et al. (2021) is not the best model to explain the variation in grassland production. Finally, through model comparison and optimization (the R code is provided in the Supplementary Material), we obtained the best SEM to understand the key factors determining grassland production (Figure 1D). Different from their opinion that their results that zinc (Zn) and iron (Fe) were predictors of grassland production, our results indicated that only zinc (Zn) among micronutrients is a significant predictor.
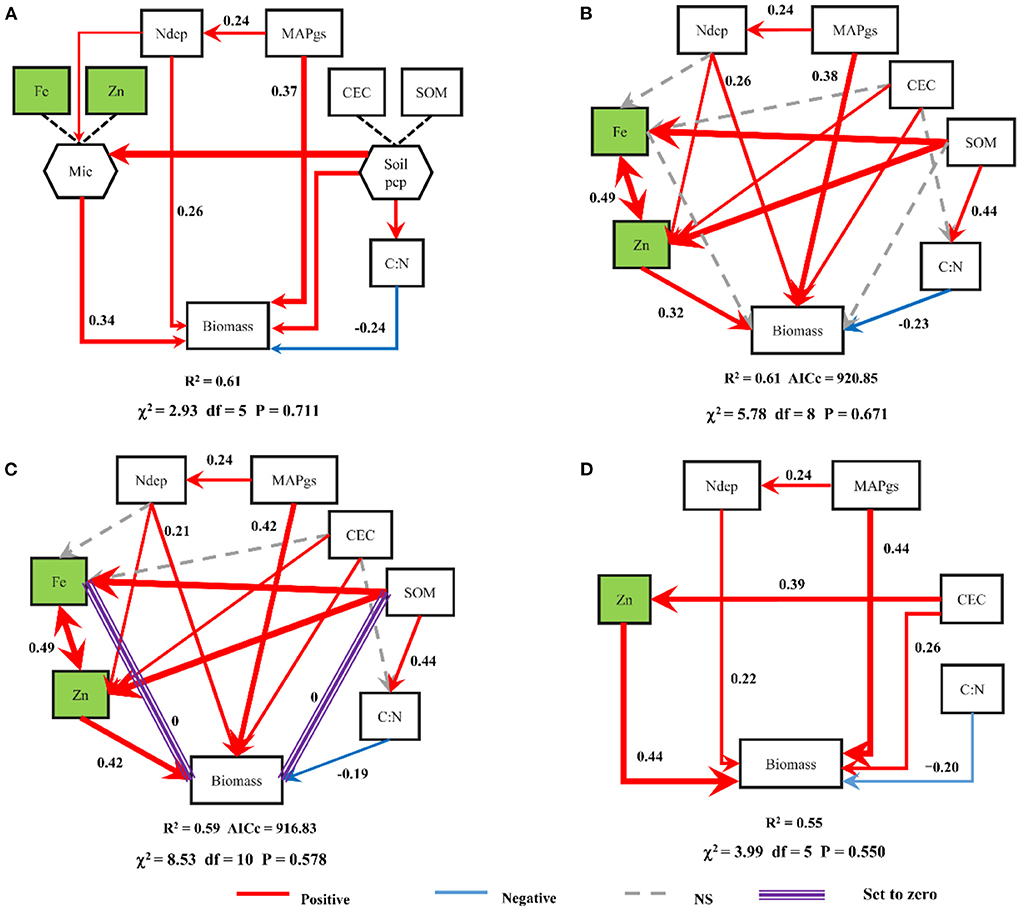
Figure 1. Comparison of structural equation models (SEMs). Panel (A), the SEM of Radujković et al. (2021); Panel (B), Radujković's (2021) SEM without composite variables; Panel (C) shows that the direct effects of both Fe and SOM on Biomass were set to zero. Panel (D), our best SEM. Numbers along the arrows, and the width of the arrows, indicate standardized path coefficients. MAPgs, mean annual precipitation during the growing season; Ndep, total atmospheric inorganic nitrogen deposition; Fe, extractable iron; Zn, extractable zinc; CEC, cation exchange capacity; SOM, soil organic matter; C:N, carbon-to-nitrogen ratio; Biomass, standing crop (live biomass and recently senescent material) at the peak of the growing season.
In addition, we used another method to construct a SEM to explain the factors underlying the variation in Biomass. Before SEM construction, we performed automated model selection using glmulti (Calcagno and De Mazancourt, 2010) based on AICc to determine the best combination of all environmental variables initially used in Radujković et al. (2021). This method allowed us to perform model selection by creating a set of models with all possible combinations of initial variables and sorting them according to the AICc. Five models with ΔAICc <1 were selected (Table 1). In these models, Zn was always retained, and Fe was always excluded. Based on the variables selected by these five linear models, we constructed five SEMs, of which three were consistent with our previous SEM (Figure 1D).
Discussion
In summary, we demonstrated that the SEM of Radujković et al. (2021) was not the best model for understanding the predictors of grassland production and that Fe was not a key soil micronutrient for predicting Biomass in their dataset. Even though it seems that the difference between Radujković's (2021) results and ours is minor, we argue that it should be highlighted. Our results have at least two important implications. First, the appropriate statistical procedures are the premise of sound scientific finding, and our study provides a paradigm for future SEM construction and optimization. Second, their study may motivate more experimental or observational studies focusing on soil Zn and Fe in the future, but our results indicate that, under the limited experimental resources, only Zn should be a priority for understanding the effects of soil micronutrients on grassland production.
Author contributions
CL and YL conceived of the comment. CL, MX, and NH performed statistical analyses. CL and YL drafted the manuscript. All authors contributed to the article and approved the submitted version.
Funding
This work was supported by National Natural Science Foundation of China (31988102, 42141004, and 32171544), the National Science and Technology Basic Resources Survey Program of China (2019FY101300), and the fellowship of China Postdoctoral Science Foundation (2020M680663 and 2021M693147).
Conflict of interest
The authors declare that the research was conducted in the absence of any commercial or financial relationships that could be construed as a potential conflict of interest.
Publisher's note
All claims expressed in this article are solely those of the authors and do not necessarily represent those of their affiliated organizations, or those of the publisher, the editors and the reviewers. Any product that may be evaluated in this article, or claim that may be made by its manufacturer, is not guaranteed or endorsed by the publisher.
Supplementary material
The Supplementary Material for this article can be found online at: https://www.frontiersin.org/articles/10.3389/fpls.2022.962513/full#supplementary-material
References
Calcagno, V., and De Mazancourt, C. (2010). glmulti: an R package for easy automated model selection with (generalized) linear models. J. Stat. Softw. 34, 1–29. doi: 10.18637/jss.v034.i12
Fay, P. A., Prober, S. M., Harpole, W. S., Knops, J. M. H., Bakker, J. D., et al. (2015). Grassland productivity limited by multiple nutrients. Nat. Plants 1, 15080. doi: 10.1038/nplants.2015.80
Huxman, T. E., Smith, M. D., Fay, P. A., Knapp, A. K., Shaw, M. R., et al. (2004). Convergence across biomes to a common rain-use efficiency. Nature 429, 651–654. doi: 10.1038/nature02561
Keywords: biomass production, micronutrients, zinc, iron, model selection
Citation: Liu C, Xu M, Li Y and He N (2022) Only zinc (Zn) among micronutrients is an important predictor of grassland production. Front. Plant Sci. 13:962513. doi: 10.3389/fpls.2022.962513
Received: 06 June 2022; Accepted: 07 July 2022;
Published: 22 July 2022.
Edited by:
Xiaoming Kang, Chinese Academy of Forestry, ChinaReviewed by:
Jie Yao, Chinese Academy of Forestry, ChinaAijun Xing, Institute of Botany (CAS), China
Yinzhan Liu, Henan University, China
Copyright © 2022 Liu, Xu, Li and He. This is an open-access article distributed under the terms of the Creative Commons Attribution License (CC BY). The use, distribution or reproduction in other forums is permitted, provided the original author(s) and the copyright owner(s) are credited and that the original publication in this journal is cited, in accordance with accepted academic practice. No use, distribution or reproduction is permitted which does not comply with these terms.
*Correspondence: Ying Li, bHlpbmdAaWdzbnJyLmFjLmNu