- 1Biosystematics Group, Wageningen University and Research, Wageningen, Netherlands
- 2Key Laboratory of Biology and Genetic Improvement of Oil Crops, Ministry of Agriculture, Oil Crops Research Institute, Chinese Academy of Agricultural Sciences, Wuhan, China
Black rot caused by the vascular pathogenic bacterium Xanthomonas campestris pv. campestris (Xcc) is widespread in Brassicaceae plants and an infectious disease that causes large yield losses in oil seed rape (Brassica napus L.). Improvement of resistance through breeding is a crucial strategy to prevent black rot disease in B. napus, but presently hampered by insufficient understanding of Xcc-Brassica interactions. This study compares two EMS-mutagenized B. napus lines that show contrasting resistance levels to their susceptible progenitor. Patterns of differential gene expression between these B. napus lines were evaluated at three time points post inoculation by comparative RNA-seq analysis. In line with the observed disease phenotypes, the susceptible line ZS9mXccS-1 displayed a steady amount of differentially expressed genes (DEGs) at different time points of infection, whereas the resistant line ZS9mXccR-1 displayed a gradual increase in DEGs throughout the course of infection. Weighted gene co-expression network analysis (WGCNA) pinpointed multiple defense-related hub genes with potential central roles in immunity, including the cell surface receptor genes CRK11 and BIR1, and the associated downstream regulatory genes WRKY11 and PBL30. KEGG analysis of DEGs belonging to two distinct co-expression modules revealed enriched pathways associated with defense, including Ca2+-signaling, receptor-mediated immunity, and phytohormone balance. Taken together, our comparative transcriptome analysis provides new avenues to unravel the mechanisms underlying black rot resistance in B. napus.
Introduction
Black rot caused by bacterial pathogen Xanthomonas campestris pv. campestris (Xcc) is one of the most destructive diseases in Brassica crops, including oil seed rape Brassica napus L. (Vicente and Holub, 2013). Typical black rot symptoms are V-shaped chlorotic lesions and blackening of leaf veins, which can lead to considerable yield losses—especially when infection occurs under favorable environmental conditions (Aires et al., 2011; Afrin et al., 2018). Currently grown cultivars do not provide adequate black rot resistance, and chemical control is not effective and hazardous to the environment (Vicente and Holub, 2013). As such, Brassica breeding for black rot resistance is considered one of the most effective and sustainable control methods.
Plants are subject to infection by a wide range of pathogens, including diverse pathogenic bacteria. Natural selection has driven plants to shape a sophisticated defense system to resist the attack of pathogenic microbes (Fu and Dong, 2013). Besides constitutive defenses, including structural barriers such as waxy epidermal cuticles and trichomes, plants evolved a multi-layered induced defense system to ward off pathogens. At the cell surface, pattern-recognition receptors (PRRs), either receptor-like kinases (RLKs) or receptor-like proteins (RLPs), recognize pathogen attack by perceiving diverse pathogen-associated molecular patterns (PAMPs). PAMP-triggered immunity (PTI) initiates a suite of downstream immune responses to prevent pathogen invasion, including accumulation of reactive oxygen species (ROS), induction of defense gene expression, Ca2+ influx, and plant hormone synthesis (Zipfel, 2014). Plant pathogens are capable to secrete effectors into host cells to suppress PTI by disrupting defense-associated signaling (Dodds and Rathjen, 2010). In turn, plants can detect these effectors or their activity, by intracellular resistance proteins composed of nucleotide−binding and leucine−rich repeat (NLR) domains. Effector-triggered immunity (ETI) generally leads to stronger and more damaging defense reactions, often manifested by a hypersensitive response (HR) leading to host cell death (Hatsugai et al., 2017). Recent studies show that PTI and ETI are interconnected and cross-potentiating each other, which is required to mount robust disease resistance (Ngou et al., 2021; Yuan et al., 2021).
Various studies have been performed to unravel the complexity of Brassica-Xcc interactions using QTL mapping and diverse omics approaches (Shaw et al., 2021). Diverse reports revealed correlations between black rot disease severity in Brassica and the accumulation of secondary metabolites, including glucosinolates, hydroxycinnamic acids (HAs), flavonoids, and various phenolics (Aires et al., 2011; Velasco et al., 2013; Barman et al., 2015; Islam et al., 2018). Such defensive metabolites were especially found to accumulate in incompatible interactions (Islam et al., 2017). Resistant B. napus cultivars also display high JA levels and enhanced expression of synthesis-related genes upon infection, eventually leading to the accumulation of JA-mediated metabolites functioning in host immunity. The opposite pattern was observed in susceptible cultivars that show enhanced levels of SA and ABA (Islam et al., 2019). Recent transcriptome analysis of two B. rapa cultivars with contrasting resistance levels identified the top 10 most differentially expressed genes (DEGs) upon Xcc infection, one of which encodes a NLR receptor protein (Song et al., 2020). Similar work by Sun et al. (2021), who characterized early transcriptional defense responses in B. oleracea, highlighted the importance of genes involved in the glucosinolate pathway, ROS scavenging, and photosynthetic metabolism in Xcc resistance. Despite recent achievements, our present understanding of the molecular mechanisms of black rot resistance in Brassica crops, especially oilseed rape, is still far from complete.
In this study, two ethyl methanesulfonate (EMS)-induced B. napus mutant lines representing contrasting levels of black rot resistance—one showing enhanced resistance (ZS9mXccR-1), the other enhanced susceptibility (ZS9mXccS-1), were compared with their progenitor B. napus ZS9. Whole transcriptome profile analyses were performed with these three lines at different time points of Xcc infection. Transcriptional dynamics between different lines post Xcc inoculation, such as the differences in GO enrichment and KEGG pathways, were systematically characterized. Candidate genes potentially playing key roles in resistance toward Xcc in B. napus were pinpointed. Overall, this study provides new insights into the molecular mechanisms underlying black rot resistance in B. napus and forms a valuable resource for future functional studies to inform oil seed resistance breeding.
Materials and methods
Plant material and growth conditions
This study makes use of B. napus cv. Zhongshuang 9 (ZS9), a widely cultivated semi-winter variety with improved traits, including Sclerotinia resistance and high yield (Wang et al., 2004). A set of 41 mutants was obtained by ethyl methanesulfonate mutagenesis (EMS) treatment using ZS9 as progenitor. Plant material was grown in a greenhouse under standard conditions [22/18°C (day/night), 16/8 h photoperiod, and > 60–70% relative humidity].
Inoculum preparation and plant infection assays
Xcc strains were grown on plates containing NYGA medium (5 g/L peptone, 3 g/L yeast extract, 20 g/L glycerol, 1.5% agar, pH 7.0) at 28°C for 48 h. Fresh bacterial cultures were dissolved in sterilized ddH2O and adjusted to an OD600 value of 0.4. Plants at a four-leaf stage were used in disease assays. Three leaves per plant were inoculated by clipping leaf tips (approximately 1–1.5 cm) with scissors dipped in bacterial suspension. Mock control leaves were clipped using scissors dipped in water. After inoculation, greenhouse conditions were set to facilitate Xcc infection [28/24°C (day/night), 14/10 h photoperiod, and > 90% relative humidity] (Lema et al., 2012).
Isolation of pathogenic bacteria from leaf tissue
Leaf disks (5 mm in diameter) sampled at inoculation sites or at the margin of lesions were surface sterilized according to Yang et al. (2019). Samples were grinded using a sterilized microcentrifuge pestle, and finally left to set for 15 min. Suspensions were diluted 100 times, of which 50 μl was plated on NYGA plates and incubated at 28°C. Experiments were conducted with three biological repetitions.
Tissue sampling and RNA isolation
At 5 and 8 days post inoculation (dpi), lesion areas including a margin of seemingly unaffected tissue were sampled using surgical scissors from each inoculated third true leaf. Leaf tissue of mock-inoculated plants was sampled approximately 5 mm from the point of inoculation. Samples were flash-frozen in liquid nitrogen and stored at –80°C. Total RNA was isolated using a TGuide plant RNA extraction kit (TIANGEN, Bei Jing, China) following the manufacturer’s instructions. RNA was purified by ethanol precipitation. Concentration and purity of RNA samples was measured with a NanoDrop 2000c spectrophotometer and a Qubit 4.0 fluorometer using the RNA Broad Range assay (Thermo Fisher Scientific, United States). Experiments were performed with three biological repetitions.
Comparative transcriptome analysis
Library preparation and RNA sequencing was performed at the Novogene Bioinformatics Institute (Beijing, China) according to Yang et al. (2018). Adapter sequences and low quality sequence reads were filtered from datasets using the NGSQC Toolkit (Patel and Jain, 2012). Clean reads were mapped to the B. napus reference genome Darmor-bzh by Hisat2 v2.0.5 (Chalhoub et al., 2014; Kim et al., 2015). Transcripts were quantified by stringtie v1.3.3b as Fragments Per Kilobase of exon model per million mapped reads (FPKM) (Pertea et al., 2015). Genes with expression differences meeting the thresholds |log2(FoldChange)| > 1 and padj < 0.05 were designated as DEGs by DESeq2 (Love et al., 2014). Principle component analysis (PCA) was conducted with the princomp function, and subsequent correlation analysis was performed using the corrplot package in R. K-means clustering was analyzed based on normalized FPKM by Genesis software (Sturn et al., 2002). Raw data of RNA-seq was available from the NCRI SRA database (PRJNA748871).
Functional annotation and enrichment analysis of differentially expressed genes
Functional annotation of DEGs was performed by similarity searches against the TAIR10 and NCBI non-redundant (nr) protein databases using blastx with an e-value of 10–5 as cut-off. GO enrichment analysis was implemented by clusterProfiler package in R (Yu et al., 2012). GO terms with a padj value < 0.05 were assigned as significantly enriched. KEGG pathways involved in these DEGs were analyzed using the webserver KOBAS v2.0 with p < 0.05 as cut-off criterion (Xie et al., 2011).
Gene co-expression network analysis
Weighted gene co-expression network analysis was implemented with the WGCNA package in R (Langfelder and Horvath, 2008; Supplementary Data 1). DEGs were preliminarily filtered using the FPKM threshold of 300 over all samples. Data clustering was performed to detect outliers. Weighted networks with scale-free topology were constructed using a soft thresholding power of β = 18 with a R2-value > 0.85. Obtained modules were color-coded, among which the gray module contains genes not belonging to any of the other modules. Heatmap and module clustering were used to visualize the co-expression network of 1,000 randomly selected genes. Color intensity represents the level of expression similarity among genes. Expression levels of DEGs within each module were depicted in a heatmap plot, and corresponding GO and KEGG enrichment analyses were performed as described above. Correlations between different modules and conditions were calculated to pinpoint the most correlated module-trait relationships. Selected modules were finally visualized by VisANT software (Hu et al., 2013).
Quantitative RT-PCR
Synthesis of B. napus cDNA was conducted using a PrimeScript RT reagent Kit with gDNA Eraser according to the manufacturer’s instructions. BnActin (GenBank: AF111812.1) was used as the reference gene to normalize and quantify target gene expression using the 2–ΔΔCt method (Livak and Schmittgen, 2001). Q-RT-PCR reactions were performed on a CFX Connect Real-time PCR system (Bio-Rad, United States) using SYBR Green Real-time PCR Master Mix. Relative expression levels are given as mean values ± standard deviation (SD) based on three biological repetitions. Gene-specific primers for Q-RT-PCR were designed with the online tool Primer3Plus and listed in Supplementary Table 1.
Results
Two ethyl methanesulfonate-mutagenized Brassica napus lines show contrasting phenotypes to black rot disease
A set of 41 EMS-mutagenized B. napus lines derived from accession ZS9 (TH26) was screened for disease resistance to Xcc race 3 (Supplementary Figure 1). Two lines showed contrasting phenotypes in resistance compared to its susceptible progenitor. TH316 displayed a strong resistance to race 3, whereas TH315 showed an enhanced disease susceptibility. These lines were hereafter named ZS9mXccR-1 and ZS9mXccS-1, respectively. To further evaluate and characterize this differential performance, additional disease assays were conducted on these three lines using lesion length and bacterial growth as measures of disease progression (Figure 1). Disease symptoms started to appear on ZS9mXccS-1 and ZS9 5 days after inoculation with Xcc race3. In contrast, no visual symptoms could be detected on inoculated ZS9mXccR-1 plant. At 8 dpi, clear V-shaped lesions could be observed on leaves of both ZS9 and ZS9mXccS-1 with mean lengths of 10 and 20 mm, respectively. Again, no lesions were found on ZS9mXccR-1 leaves, displaying only minor browning at the zone of inoculation (Figures 1A,B). In addition, leaf disks sampled from lesions on ZS9 plants were found to contain high bacterial numbers. Inoculated ZS9mXccR-1 leaves only contained few bacteria, underlining the observed resistance to race 3 of B. napus ZS9mXccR-1 (Figure 1C). To assess whether ZS9mXccR-1 displays broad-spectrum resistance to black rot, we performed diseases assays with different Xcc races. This revealed that ZS9mXccR-1 not only shows resistance to Xcc race 3, but also displays gain of resistance to races 2 and 4, but not to races 1, 6, and Xcc-15. In contrast, both ZS9 and ZS9mXccS-1 were found to be susceptible to all tested races (Supplementary Figure 2).
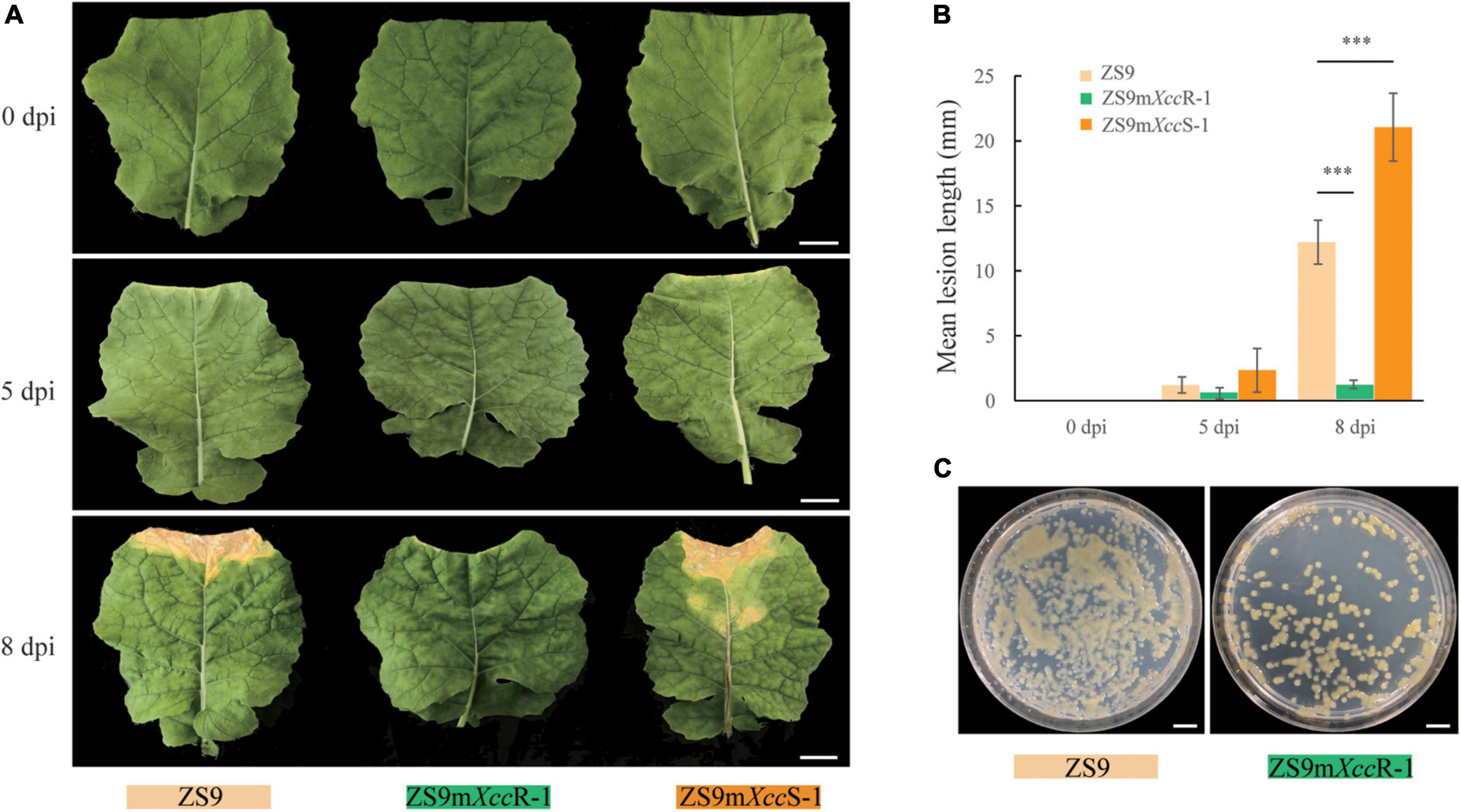
Figure 1. Two B. napus EMS lines showing contrasting levels of Xcc resistance. (A) Disease symptoms observed on the parental line ZS9 and the two EMS lines ZS9mXccR-1 and ZS9mXccS-1. Pictures were taken at 0, 5, and 8 days after Xcc inoculation. (B) Lesion lengths on ZS9, ZS9mXccR-1, and ZS9mXccS-1 after inoculation with Xcc. Bars represent mean lesion lengths ± SD based on three biological repetitions. *** indicate significant differences (p < 0.001) according to Student’s t-tests. (C) Extracts from ZS9mXccR-1 showing reduced bacterial titers compared to ZS9. Bars represent 20 mm in (A) and 10 mm in (C).
Comparative transcriptome profile analyses of two ethyl methanesulfonate mutants and the parental line Zhongshuang 9
To examine transcriptional dynamics upon Xcc infection in B. napus, RNA-seq analysis was performed on leaves of ZS9, ZS9mXccR-1, and ZS9mXccS-1 at 0, 5, and 8 dpi. Each sampling point contained three biological replicates, resulting in 27 libraries (Supplementary Table 2). A total of 1.2 billion raw reads was generated, with approximately 45 million reads per library. Clean reads after filtering were mapped to the B. napus reference genome Darmor-bzh (Chalhoub et al., 2014). The percentage of mapped reads was found to decrease gradually in the susceptible lines ZS9 and ZS9mXccS-1 during the time course of experiment. This observation is in line with the biological composition of the samples, in which the amount of Xanthomonas bacteria in the plant tissue increases over time. Detailed information on the quality of sequence reads, GC content, and the ratios of uniquely mapped reads are summarized in Supplementary Table 2.
To provide a comprehensive overview of the transcriptome data and to exclude noise and redundancy effects, a principal component analysis (PCA) was conducted based on gene read counts (Figure 2A). Replicates per treatment were found to cluster together both in PC1/2 and PC1/3. Subsequent, correlation analysis of FPKM values showed high correlation coefficients between different repetitions per treatment (Figure 2B). Additionally, the density distribution of gene expression levels showed a consistent tendency across different samples (Figure 2C). These results indicated high reproducibility of RNA-seq data allowing further in-depth analyses.
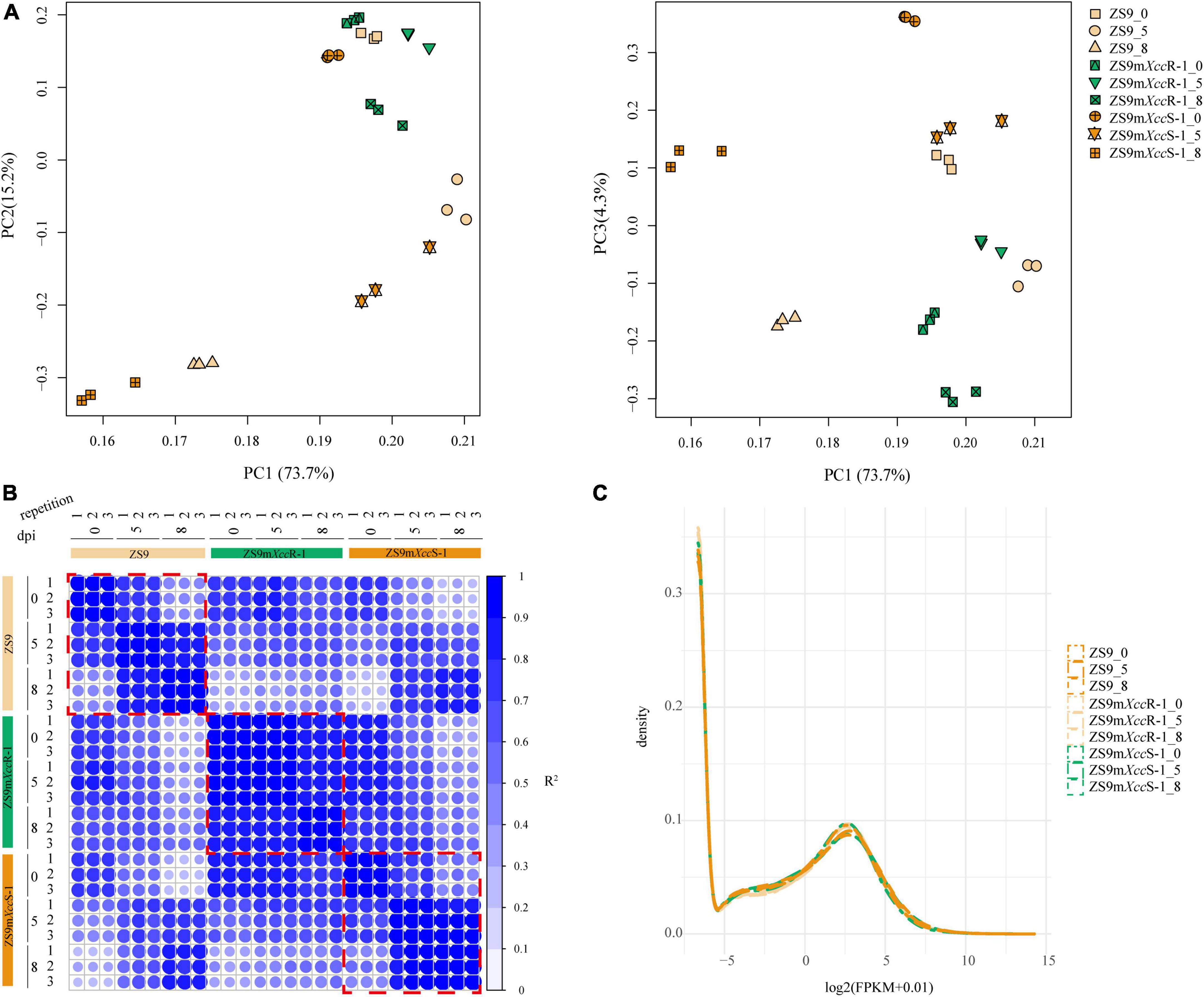
Figure 2. Quality evaluation of RNA-seq data. (A) PCA displaying the major composition of variance. Left panel: 73.7 and 15.2% of the variance referred to PC1 and PC2, respectively. Right panel: 73.7 and 4.3% of the variance referred to PC1 and PC3, respectively. (B) Representation of correlation matrix between RNA-seq samples based on Pearson correlation coefficients. Numbers represent biological repetitions. (C) Frequency histogram representing the distribution of gene expression levels across samples. Numbers 0, 5, and 8 indicate days post inoculation.
Transcriptional dynamics in Brassica napus after Xanthomonas campestris pv. campestris inoculation
To investigate expression changes in B. napus lines with different degrees of susceptibility to Xcc, we compared RNA-seq data of the EMS-mutant lines with that of the ZS9 parental line at same sampling time. This resulted in the comparison of six data pairs (ZS9mXccR-1_0 vs. ZS9_0, ZS9mXccR-1_5 vs. ZS9_5, ZS9mXccR-1_8 vs. ZS9_8, ZS9mXccS-1_0 vs. ZS9_0, ZS9mXccS-1_5 vs. ZS9_5, and ZS9mXccS-1_8 vs. ZS9_8). DEGs were defined as genes that were significantly different in at least one comparison pair after integrating all transcriptome profiles. In total, 37,744 DEGs were determined (Supplementary Table 3). The numbers of up-regulated and down-regulated DEGs detected at different time points in each B. napus line were counted and visualized in a bar plot (Figure 3A). Remarkably, ZS9mXccR-1 had both more up-regulated and down-regulated DEGs at every sampling time compared to ZS9mXccS-1, implying that the difference between ZS9mXccR-1 and ZS9 is larger than that between the susceptible lines ZS9mXccS-1 and ZS9. DEGs grouped according to their fold changes (FC) were found to be unevenly distributed across all samples, most of which had absolute FC values between 2 and 4 or higher than 8 (Figure 3B).
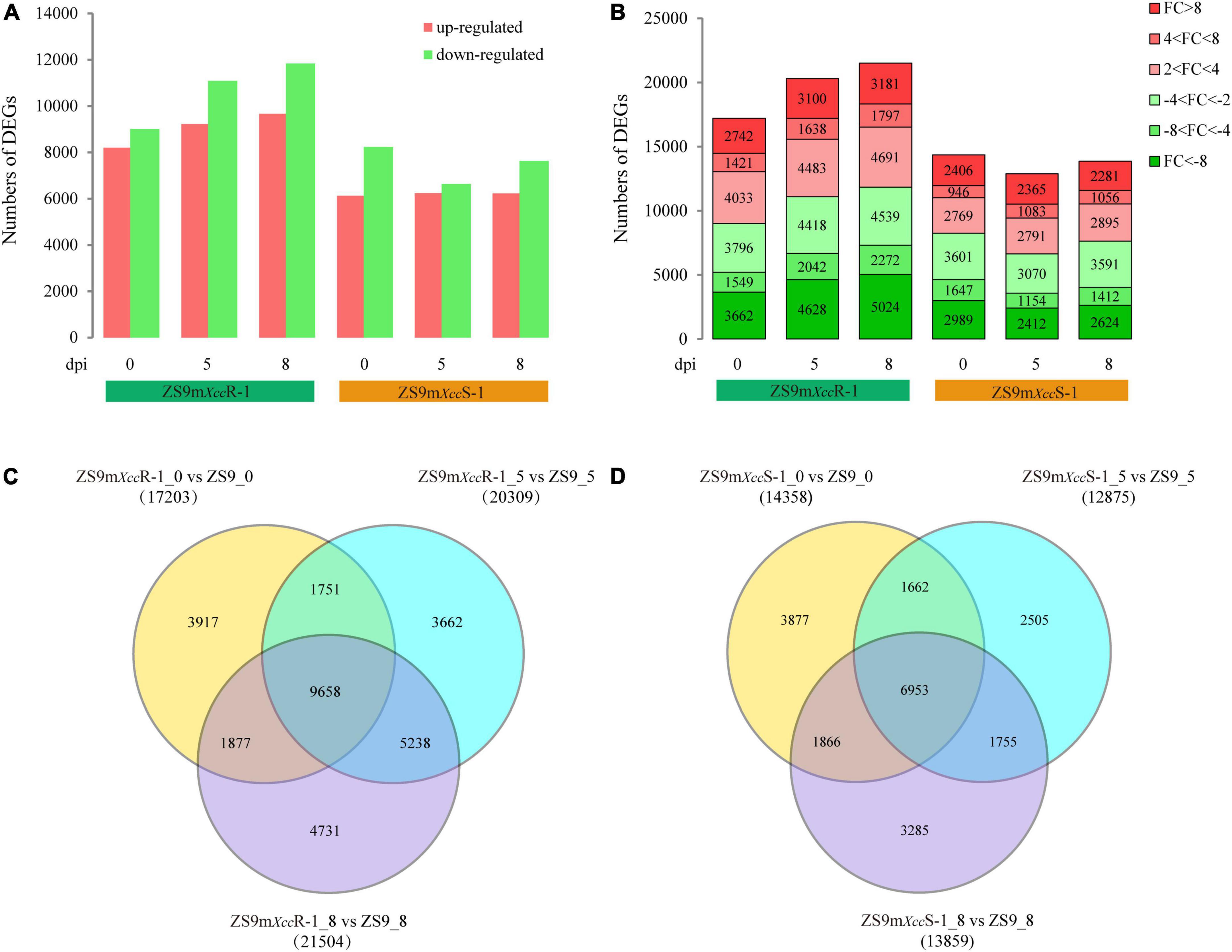
Figure 3. Comparative analysis of differentially expressed genes between B. napus ZS9 and the two EMS-lines ZS9mXccR-1 and ZS9mXccS-1 at different time points post inoculation. (A) Numbers of up-regulated and down-regulated DEGs in ZS9mXccR-1 and ZS9mXccS-1 at 0, 5, and 8 dpi. (B) Fold change (FC) distribution of DEGs in ZS9mXccR-1 and ZS9mXccS-1 at 0, 5, and 8 dpi. FC was calculated as the ratio of gene read counts between ZS9mXccR-1/ZS9mXccS-1 and ZS9. Venn diagrams displaying numbers of common and unique DEGs in the resistant ZS9mXccR-1 (C) and the susceptible ZS9mXccS-1 (D) at three time points post inoculation, respectively.
Comparative analysis of DEGs between ZS9mXccR-1 and ZS9 after Xcc inoculation showed strong overlap, in which almost half of the total DEGs were overlapping between the three sampling points (Figure 3C). This also accounted for the paired comparison of ZS9mXccS-1 and ZS9, which had 6,953 DEGs in common (Figure 3D). Notably, the total number of DEGs expressed at 5 and 8 dpi in ZS9mXccR-1 vs. ZS9 (5,238) was found to be almost three times higher as within the ZS9mXccS-1 vs. ZS9 comparison (1,755). These results revealed that the resistant line ZS9mXccR-1 and susceptible ZS9mXccS-1 show the most distinct patterns of gene expression upon Xcc infection.
To further differentiate DEGs in response to Xcc inoculation, K-means clustering was implemented to classify 37,744 genes based on their expression patterns, resulting into nine different clusters (Figure 4). Genes in cluster 1 display a rather stable expression in ZS9mXccR-1, but were found to be up-regulated at 5 dpi in ZS9mXccS-1 and at both 5 and 8 dpi in ZS9. Cluster 2 shows a quite similar pattern with genes that have higher expression levels in ZS9 at 8 dpi. Most genes grouped in clusters 3 and 9 were found to be down-regulated at all-time points in the three B. napus lines, except for DEGs in ZS9mXccR-1 at 5 dpi within cluster 9. This down-regulation of gene expression within the susceptible lines ZS9mXccS-1 and ZS9 was also detected in clusters 6 and 7, and clusters 5 and 7, respectively. Cluster 4 contains genes moderately down-regulated in ZS9mXccR-1 at 5 dpi and significantly up-regulated at 8 dpi, while the expression tendency in ZS9mXccS-1 was exactly the opposite, supplying valuable clues to understand the contrasting phenotypes of these B. napus lines in Xcc resistance.
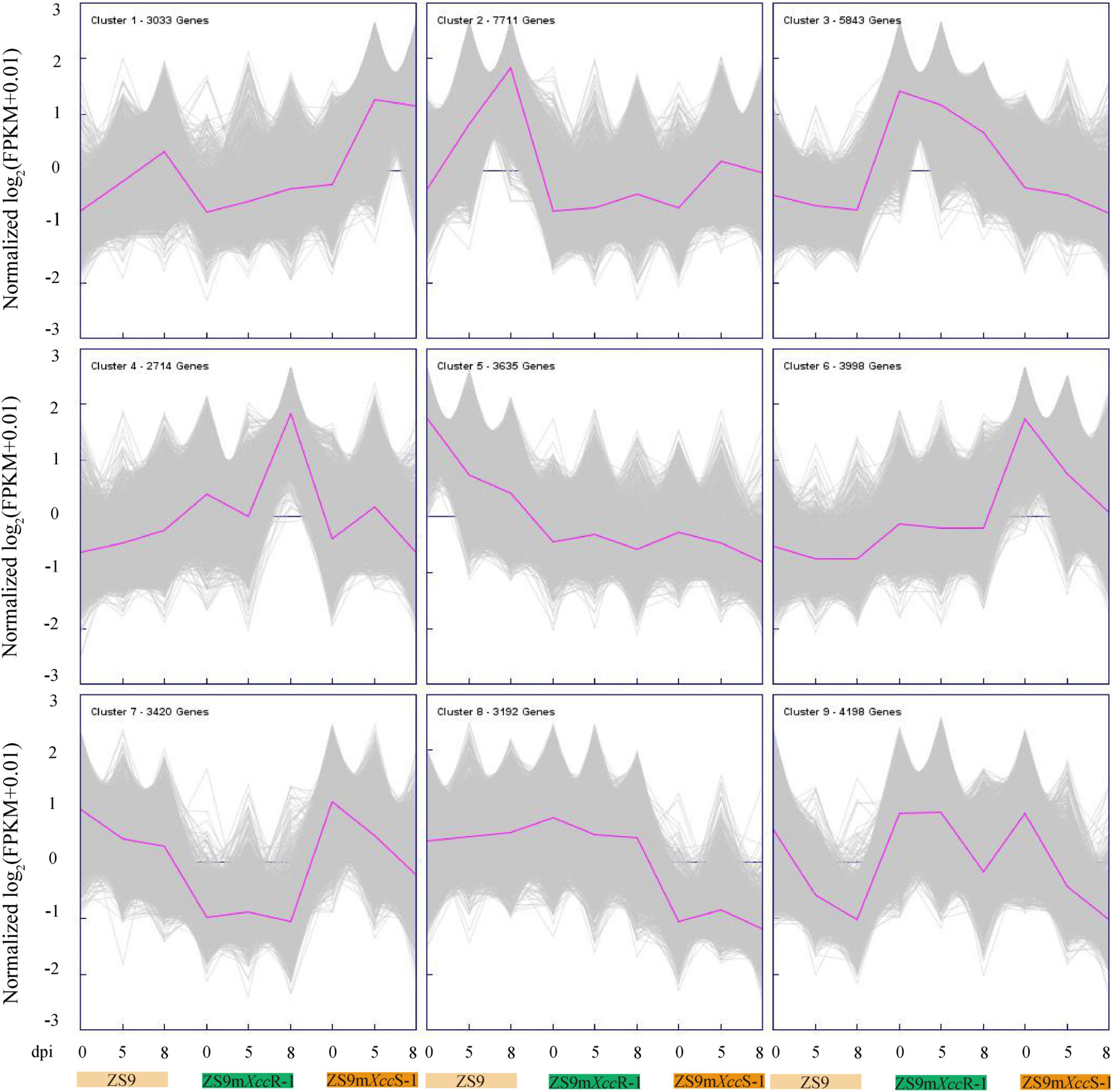
Figure 4. K-means cluster analysis of 37,744 DEGs. Purple lines indicate average expression levels of the subcluster. Gray lines represent expression levels of each gene within the cluster. The number of genes per cluster is indicated at the left corner of each panel.
Functional enrichment and comparative analysis of differentially expressed genes
Identified DEGs were functionally annotated by performing blastx against the TAIR10 and NCBI nr protein databases. Subsequently, these DEGs were subjected to functional GO enrichment analysis using the clusterProfiler package in R. With a threshold cutoff of padj < 0.05, DEGs could be clustered into 106 GO groups divided over the three functional categories (Supplementary Table 4). Most significantly enriched GO terms of DEGs were related to biosynthetic processes, such as the metabolism of polysaccharides (GO:0005976) and glucans (GO:0006073, GO:0044042), and cell wall organization (e.g., GO:00071554). GO terms related to oxidative stress, defense response, and cell wall modification also made up a considerable proportion, underlining the biotic stress conditions evoked in B. napus upon Xcc infection (Figure 5A). Enrichment analysis revealed that DEGs expressed in the resistant line ZS9mXccR-1 were mainly involved in cellular metabolic processes, cell wall organization and modification, cell recognition, and the response to oxidative stress (Table 1 and Supplementary Figure 3A). As for the susceptible line ZS9mXccS-1, unique GO terms of DEGs were found to be related to defense response, reaction to inorganic substances, and the carbohydrate metabolic process, but lacked categories involved in cell recognition, and cell wall organization and modification as enriched in the resistant line (Table 1 and Supplementary Figure 3B).
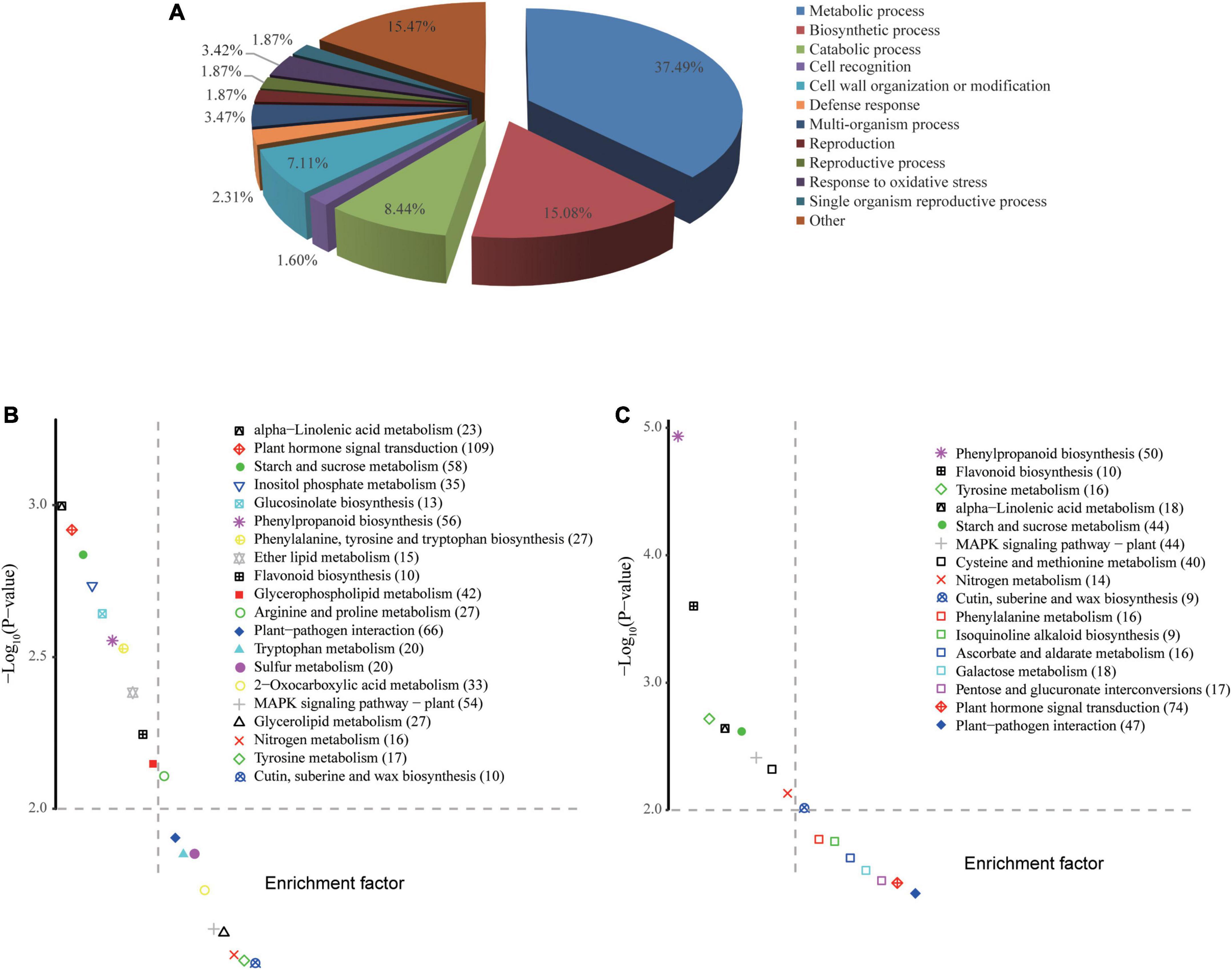
Figure 5. GO enrichment and KEGG pathway analyses of DEGs. (A) Biological classification of all DEGs by GO enrichment analysis. (B,C) KEGG pathways enriched in ZS9mXccR-1 and ZS9mXccS-1, respectively. The vertical and horizontal axes of KEGG scatter diagrams represent the –log10(P-value) and enrichment factor, respectively. Values in brackets are indicative of the number of DEGs enriched in each pathway.
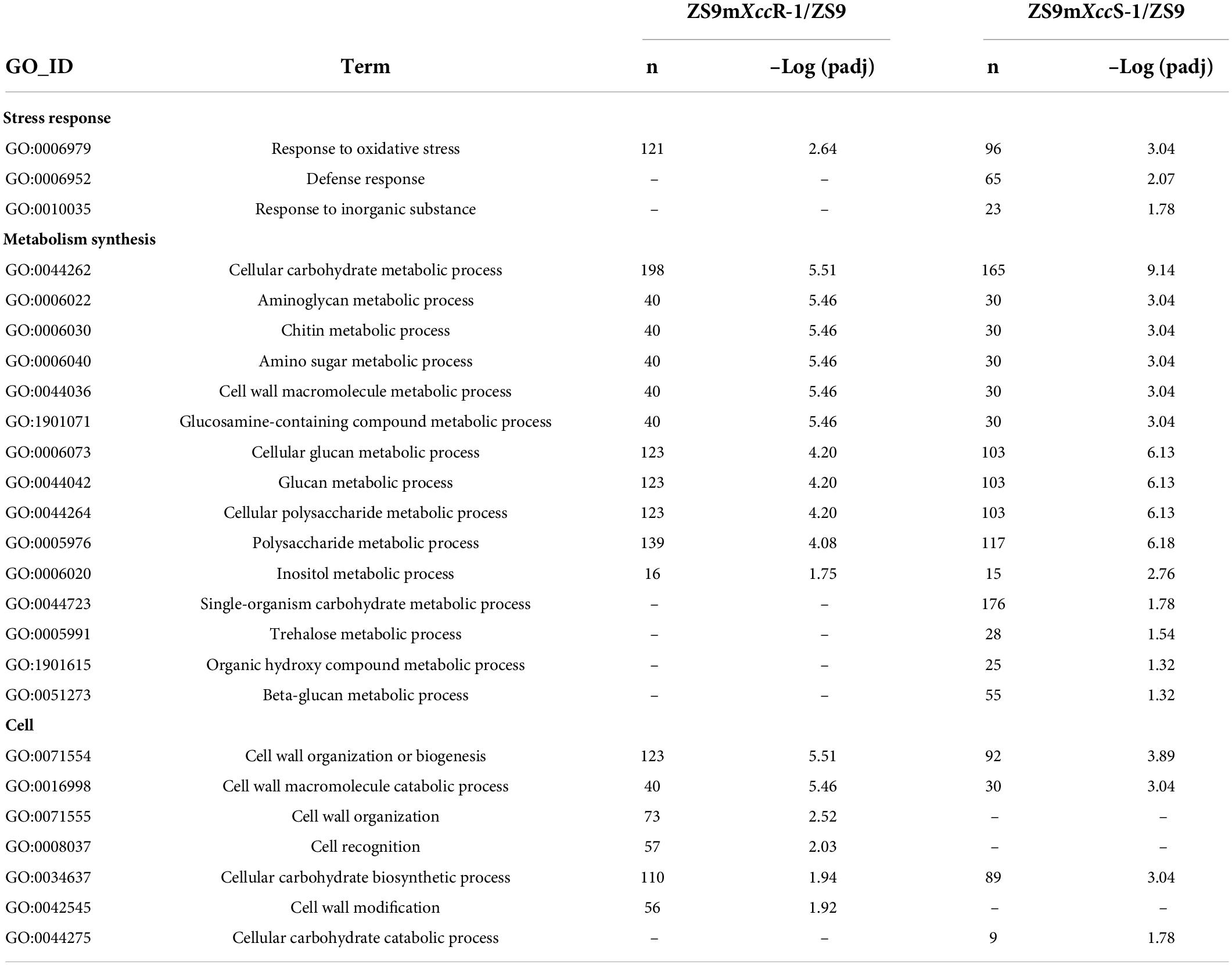
Table 1. Comparison of GO-terms (biological processes) enriched in ZS9mXccR-1 and ZS9mXccS-1 upon Xcc inoculation.
KEGG enrichment analyses were implemented to determine pathways associated with the identified DEGs. Comparing ZS9mXccR-1 with ZS9 resulted in the identification of 20 significant enriched KEGG pathways, whereas the pair-wise comparison of ZS9mXccS-1 and ZS9 resulted in 16 pathways (Figures 5B,C). Abundant pathways associated with plant defense were commonly detected in both ZS9mXccR-1 and ZS9mXccS-1 lines following Xcc inoculation, such as those involved in sucrose metabolism, phenylpropanoid biosynthesis, and plant-pathogen interactions. Pathways specifically assigned to the resistant line ZS9mXccR-1 were related to lipid metabolism and the biosynthesis of glucosinolates. Pathways unique to the susceptible line ZS9mXccS-1 were associated with metabolism of galactose, ascorbate, and various amino acids (Figures 5B,C).
Co-expression network analysis identifies hub genes associated with black rot resistance
To further mine potential genes related to black rot resistance, a weighted gene co-expression network analysis (WGCNA) was implemented using filtered DEG expression levels. A scale-free network was constructed with the soft threshold power of 18 under the threshold R2 > 0.85, resulting in 11 modules (Supplementary Figure 4A). Gene expression heatmaps and tree diagrams were used to represent a similarity matrix of gene co-expression. DEGs belonging to individual modules showed strong co-expression patterns, especially in the blue, turquoise, and brown modules (Figure 6A). Heatmap visualization of DEGs and GO functional enrichment classifications were performed for each individual module (Figure 6B, Supplementary Figure 4B, and Supplementary Table 5). Correlation analysis of module-treatment relationships showed that genes in black and purple modules are strongly associated with disease severity (ZS9mXccR-1_0, r = 0.49, P = 0.01; ZS9mXccR-1_8, r = 0.56, P = 0.002) (Supplementary Figure 4C). In addition, we also noticed that these DEGs were not only correlated with their corresponding modules, but also with their corresponding traits (Figure 6C), highlighting the significance to further study the genes within these modules. Genes showing this expression pattern potentially can explain the underlying mechanisms of black rot resistance. DEGs of the black module were found to be up- and down-regulated at 8 dpi in the resistant and susceptible EMS lines, respectively (Figure 6B). Network visualization revealed highly interconnected signatures of gene co-expression within the black module, identifying several defense-associated hub genes—including the cell surface receptors BIR1 and CRK11, the downstream regulatory proteins PBL30 and MEKK1, and the transcription factor WRKY11. Other hub genes with enhanced expression levels in the resistant line are encoding the exocyst complex protein EXO70B2, a Development and Cell Death (DCD)-domain containing protein, an inositol phosphorylceramide synthase, and an UDP-glucose 6-dehydrogenase (Figure 6D and Supplementary Figure 4D). In contrast, DEGs belonging to the purple module were found to be up-regulated in ZS9mXccR-1 and down-regulated in ZS9mXccS-1, or vice versa, during the course of infection. Co-expression analysis indicated that the highly interconnected genes were mainly involved in stress tolerance, including a Myb-like HTH transcription factor and multiple heat shock proteins. Two other hub genes within this cluster showing enhanced expression only in the resistant line are encoding the immune-related EIN3-binding F-box protein 2 and the Uridine diphosphate glycosyltransferase 74E2, which were suggested to play roles in ROS integration and hormone-response pathways (Figure 6E and Supplementary Figure 4D).
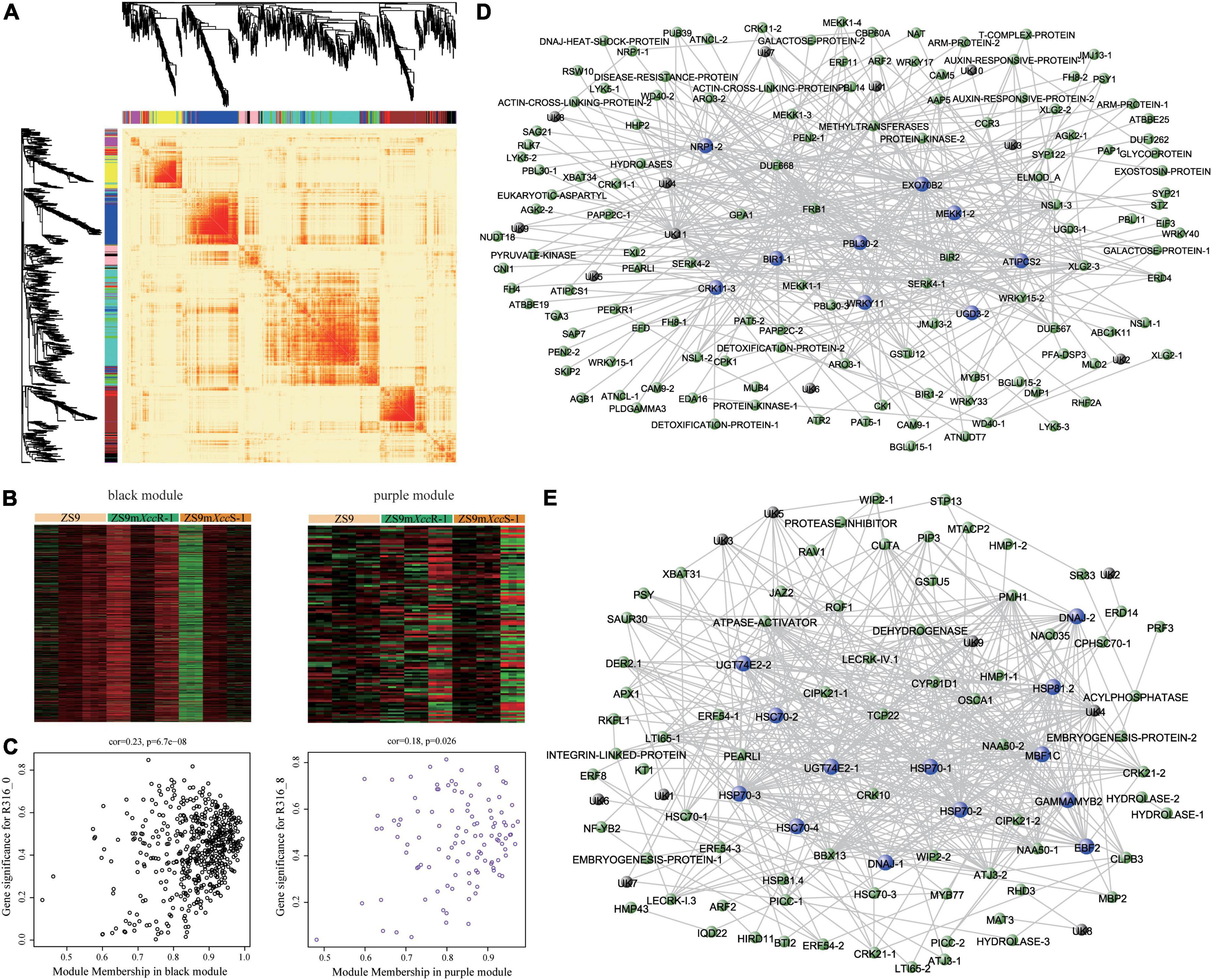
Figure 6. Weighted gene co-expression network analysis identifies hub genes functioning in Xcc resistance. (A) Heatmap plot showing patterns of differential gene expression across modules. Colored bars below dendrograms indicate respective modules. Color intensity represents the level of expression similarity among genes. (B) Expression heatmaps of DEGs clustered in black (left) and purple (right) modules. (C) Scatter plots displaying correlation relationships between gene-modules and gene-traits. Weighted gene co-expression networks of the black (D) and purple (E) modules. Highly connected hub genes are indicated by blue nodes. Black nodes represent genes of unknown function.
Pathways potentially involved in Brassica napus resistance against Xanthomonas campestris pv. campestris
As a next step, we performed KEGG pathway analyses on DEGs of the black and purple modules. This resulted in the identification of diverse biological pathways involved in black rot resistance, of which several are highlighted below (Figure 7 and Supplementary Table 6).
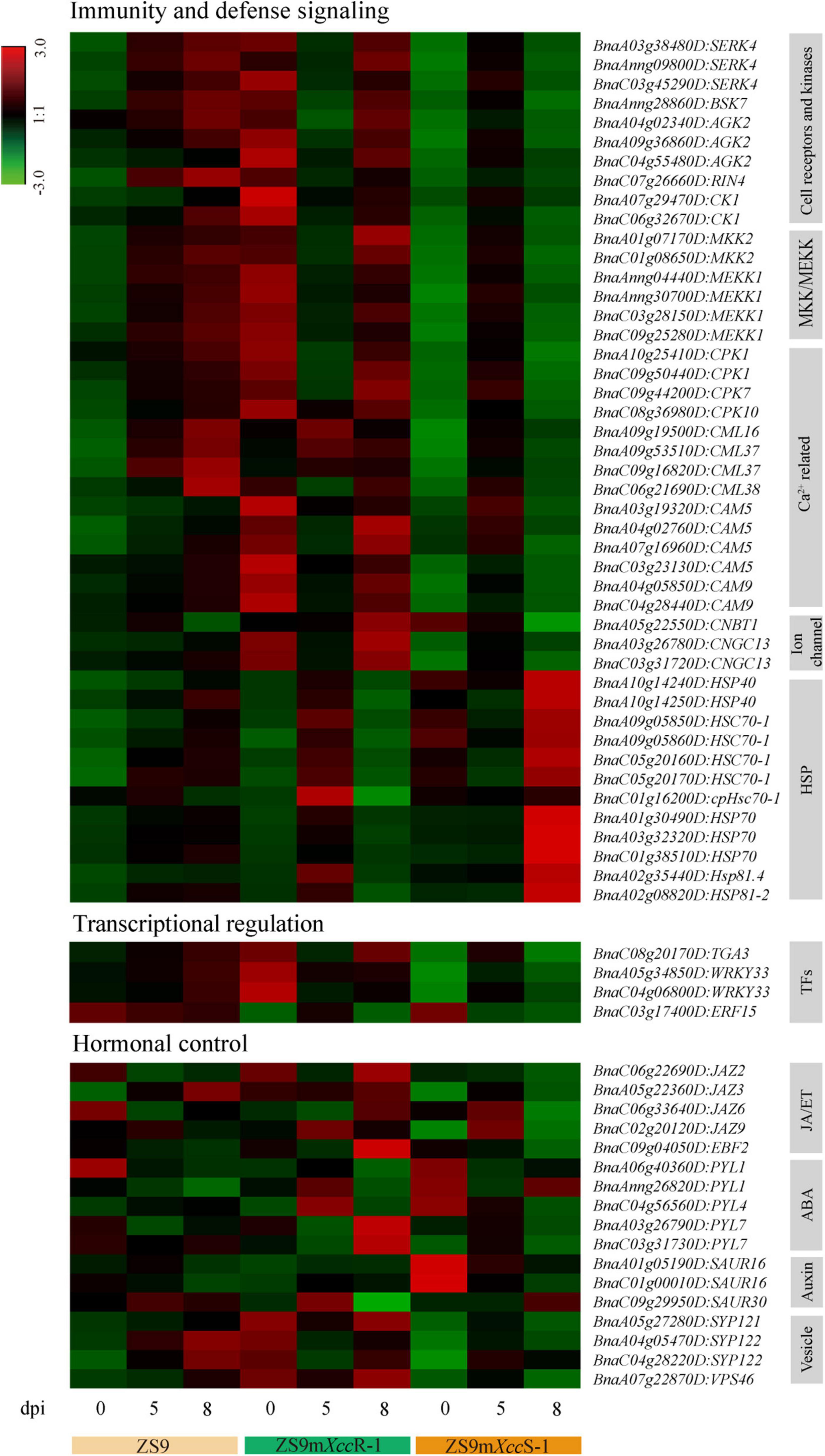
Figure 7. Heat map visualization of selected DEGs related to immunity and defense signaling, transcriptional regulation, and hormonal control.
Immunity and defense signaling
A large proportion of DEGs was found to be significantly enriched in pathways related to plant immunity, including components of both immune recognition and downstream signaling—such as the protein kinases SERK4 and BSK7. We also identified multiple copies of MKK2 and MEKK1 with differential expression levels in the susceptible and resistant B. napus lines. Besides DEGs in these pathways, multiple genes related to calcium (Ca2+) signaling, including several calcium-dependent protein kinases (CPKs) and calmodulin proteins (CaMs and CMLs) reported to be involved in immune homeostasis (Choi et al., 2009; Bredow et al., 2021), were identified and shown to be suppressed in expression in the hypersusceptible line at a late phase of infection. Furthermore, we found several cyclic nucleotide-gated ion channels (CNGCs), which are known to function in Ca2+ signaling, ROS accumulation, and PTI toward Xanthomonas oryzae in rice (Zhang et al., 2018). In contrast, several heat shock protein (HSP)-genes showed high transcript levels in ZS9mXccS-1 at 8 dpi, reflecting pathogenesis-related stress evoked by Xcc proliferation within the leaf tissue (Figure 7).
Transcriptional regulation
Transcriptional control of immune-responsive genes is crucial to mount robust immune responses. A multitude of transcription factor genes (TFs) was identified across the full dataset of DEGs, including members of the MYB, bZIP, and WRKY families (Supplementary Table 3). Only a few TFs, however, were found to be enriched in the black and purple modules (Figure 7). Among these, WRKY33 and TGA3—two known host immunity regulators (Wang et al., 2018; Cai et al., 2021)—showed relatively high levels of expression directly after inoculation in ZS9mXccR-1, but in ZS9mXccS-1 only at 5 dpi, implying a potential primed state of defense in the resistant line.
Hormonal control
Phytohormone signal transduction was also found to be significantly enriched pathway, including DEGs involved in abscisic acid (ABA), auxin, and jasmonic acid/ethylene (JA/ET) signaling. Multiple DEGs were found to encode different orthologs of the JAZ family that function as hormonal crosstalk hubs in JA-mediated plant immunity (Liu et al., 2017). Furthermore, several members of the PYL family that play crucial roles in ABA sensing and environmental stress signaling were detected, showing differential expression patterns during both compatible and incompatible interactions. Two of the three identified auxin-associated SAUR genes, known to regulate diverse plant developmental processes, were found to be down-regulated during infection in the hypersusceptible line ZS9mXccS-1.
Validating patterns of differential gene expression
To verify our transcriptome data, Q-RT-PCR was performed to validate the transcriptional expression of representative DEGs with different patterns across the biological samples (Supplementary Table 1). As a first step, we randomly selected three DEGs per plant line to verify patterns of differential gene expression. Expression of these selected genes was found to be largely consistent with their transcript profiles obtained by RNA-seq analysis (Figure 8A), such as DAA-1, PSAD-1 and ATHM2 that display a gradual reduction of gene expression in ZS9 across the time course of infection by both qRT-PCR and RNA-seq. Overall, these results imply strong technical and biological reproducibility between different replicates.
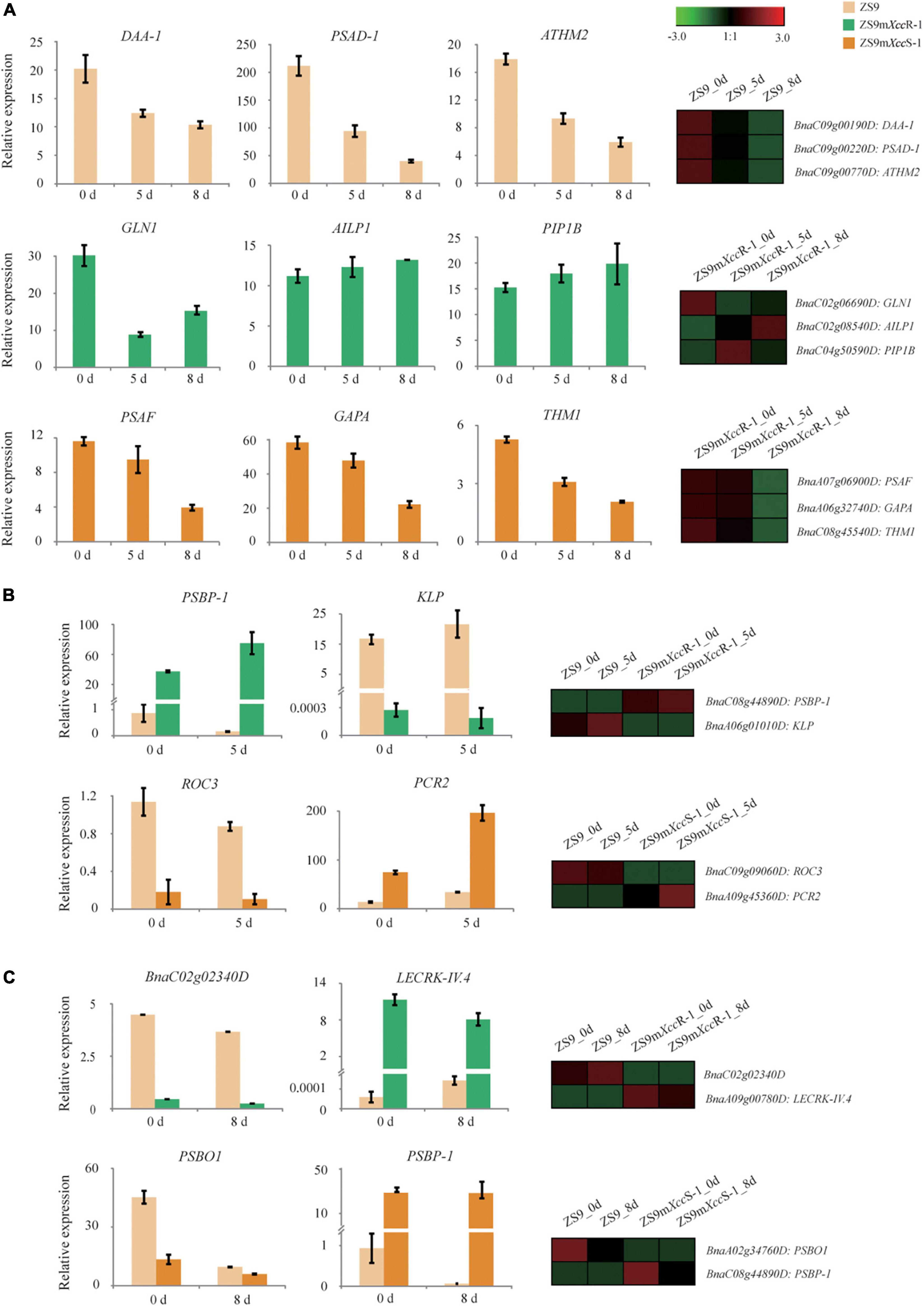
Figure 8. Validation of DEG expression patterns in B. napus upon Xcc inoculation using Q-RT-PCR. (A) Expression levels of representative DEGs with different patterns in ZS9 (top), ZS9mXccR-1 (middle), and ZS9mXccS-1 (lower panel) at 0, 5, and 8 dpi, respectively. (B) Expression levels of two selected DEGs in ZS9 vs. ZS9XccR-1 (top) and ZS9 vs. ZS9XccS-1 (down) at 0 and 5 dpi, respectively. (C) Expression levels of two selected DEGs in ZS9 vs. ZS9XccR-1 (top) and ZS9 vs. ZS9XccS-1 (down) at 0 and 8 dpi, respectively. Differential expression patterns of selected genes obtained by RNA-seq analysis are shown at the right margin.
In addition, we ranked DEGs based on levels of fold changes within both paired combination (ZS9mXccS-1/ZS9 and ZS9mXccR-1/ZS9), and selected DEGs from the top 50 up- and down-regulated for expression validation. Expression levels of the selected DEGs at 5 and 8 dpi, as measured by Q-RT-PCR, were found to match the patterns of gene expression generated by RNA-seq (Figures 8B,C). For example, BnaA09g00780D, an ortholog of the Arabidopsis lectin kinase gene LecRK-IV.4, was found to be one of most induced genes in the resistant line (Figure 8C). LecRK-IV.4 was previously shown to play an important role in immune responses to the bacterial pathogen Pseudomonas syringae in Arabidopsis (Wang et al., 2014). Correlation analysis of gene expression based on Q-RT-PCR and RNA-seq showed significant correlation, demonstrating the reproducibility and accuracy of our RNA-seq data (Supplementary Figure 5).
Discussion
Comparative transcriptome analyses provide insights into molecular Brassica napus-Xanthomonas campestris pv. campestris interactions
Black rot is one of the major vascular diseases in B. napus leading to large yield and economic losses. Although considerable efforts have been made to understand genetic mechanisms of black rot resistance in Brassica crops (Islam et al., 2017, 2019; Song et al., 2020; Sun et al., 2021), key genes underlying variation in black rot resistance are thus far largely unknown. Insights into the transcriptional dynamics of plant-pathogen interactions can help to potentially pinpoint genes underlying pathogen resistance (Meng et al., 2021). To elucidate the transcriptional response of B. napus to black rot infection, we performed comparative transcriptome sequencing of three B. napus lines with different levels of susceptibility at three time points after Xcc inoculation. Since Brassica-Xcc bioassays are prone to variability (Wei et al., 2016), considerable time and effort was invested to ensure proper biological replication under optimal conditions. This resulted in high correlation between RNA-seq samples, implicating reliability and accuracy of our B. napus-Xcc bioassays (Figure 2).
In this study, a total of 37,744 DEGs were subjected to subsequent GO and KEGG pathway enrichment analyses after transcript quantification. Gene set pair-wise comparison revealed both shared and unique terms and/or enriched pathways when comparing ZS9mXccS-1 and ZS9mXccR-1 with ZS9, respectively. Subsequently, DEGs were categorized into distinct modules based on their co-expression levels using WGCNA analysis, and modules correlating with the phenotypic trait,—including black and purple modules, were further analyzed in detail. We identified several hub genes in these modules with high interconnectivity to the other genes, including multiple cell surface receptors and downstream regulatory proteins involved in immune signaling (Figures 6D,E). Co-expression network and enrichment analyses pinpointed multiple biological processes underlying Xcc resistance in B. napus, including immunity and defense signaling, transcriptional regulation, and hormonal control (Figure 7).
Transcriptional insights into Xanthomonas campestris pv. campestris-induced defense signaling
Our transcriptomic analyses revealed that especially B. napus genes involved in early pathogen recognition and subsequent signaling are differentially expressed upon Xcc infection. This includes several genes encoding receptor-like kinases (RLKs) and cytoplasmic RLCKs functioning in plant immunity. Cysteine-rich receptor kinases (CRKs), such as CRK11—one of the identified hub genes, are known to play key roles in biotic stress responses and ROS signaling (Little et al., 2006; Bourdais et al., 2015). Overexpression of several CRKs in Arabidopsis was shown to enhance PTI responsiveness and resistance to P. syringae (Acharya et al., 2007; Yeh et al., 2015; Yadeta et al., 2017). Furthermore, several induced SERK4 genes were found in the resistant line ZS9mXccR-1. SERK family members are known to function as co-receptors of multiple ligand-induced PRRs functioning in plant immunity, such as FLS2 that mediates PTI by perceiving the bacterial PAMP flagellin (Ma et al., 2016). SERK4 was shown to interact with BAK1 (SERK3) to regulate the stability of the calcium channel CGNC20 to control ROS accumulation and cell death (Yu et al., 2019). Notably, CGNC13, a closely related homolog, was one of the identified DEGs in our study. CNGC1 and CGNC14 have been shown to negatively regulate non-host resistance to Xanthomonas oryzae in tomato (Zhang et al., 2018). Another key player in PRR-mediated defense found to be induced in expression upon Xcc infection in B. napus is the immune repressor gene BIR1. Overexpression of BIR1 in Arabidopsis leads to constitutive activation of defense responses, promoting resistance and cell death (Guzmán-Benito et al., 2019). This is in line with the expression pattern observed in the resistant and susceptible lines following Xcc inoculation in this study (Supplementary Figure 4D). Another hub gene identified is PBL30, a member of the larger RLCK VII subfamily (Rao et al., 2018). PBLs are known as central regulators of both PTI and ETI. One of these, BIK1, associates with the FLS2/BAK1 complex to activate PTI upon perception of bacterial flagellin (Lu et al., 2009). BIK1 is targeted by the Xcc effector AvrAC to enhance host susceptibility (Wang et al., 2015). PBL2, a paralog of BIK1, acts as a decoy and triggers ETI upon AvrAC binding via the interaction with ZAR1-mediated resistosome (Wang et al., 2015).
Vesicle-trafficking seems to be another important process during Xcc infection, emphasizing the importance of early recognition and defense responses at the host-pathogen interphase. We, for example, detected several closely related syntaxin (SYP121/122) genes known to play roles in vesicle-mediated pre- and post-invasive immunity to fungal and oomycete pathogens (Rubiato et al., 2022). Arabidopsis syp121/syp122 mutants show hypersensitivity to SA and display consecutive expression of multiple immune signaling pathways, both impacting PTI- and ETI-mediated defense (Zhang et al., 2008). Also one exocyst subunit gene, i.e., EXO70B2, was found to be differentially expressed in the resistant line. A recent study showed that EXO70B1 and EXO70B2 are required for proper trafficking of FLS2 and potential other immune receptors to the plant plasma membrane (Du et al., 2018; Wang et al., 2020).
Downstream genes involved in immune signaling were also found to be enhanced in expression, mostly in incompatible interactions. For example, multiple mitogen-activated protein kinase (MAPK) cascade genes, including MKK2 and MEKK1, were found relatively highly expressed in ZS9mXccR-1 at a late stage of infection, and much lower in compatible interactions (ZS9mXccS-1 and ZS9) (Figure 7). MKK2 and MEKK1 are known to play co-roles in downstream signaling cascades regulating PTI responses (Gao et al., 2008). Recently, MPK1 was shown to promote vascular defense to Xcc and Xoo in Arabidopsis and rice, respectively (Lin et al., 2022). Expression patterns positively correlating with resistance were also found for multiple genes involved in Ca2+-signaling, such as CaMs and CPKs (Figure 7). CaM expression was up-regulated to comparable levels in Xcc-resistant and susceptible B. napus cultivars (Mamun et al., 2020), while CaM genes of B. oleracea were found to display variable expression patterns upon Xcc infection (Song et al., 2020). These differential phenomena in different studies could be explained by the multifunctionality of CaMs in plants in a wide range of biological processes, including plant development and abiotic stress responses (Wang et al., 2009; Parvin et al., 2012). Observed differences are also likely affected by the level of infection and host-isolate interaction studied (Song et al., 2020). CPKs are known to play myriad roles in immune signaling, such as those mediating cell death, pathogen restriction, and ROS burst (Gao et al., 2013). Recently, CPK3 was identified as a key regulator of both PTI and ETI required for resistance to P. syringae in Arabidopsis, and to play an important role in immune-associated cytoskeleton organization (Lu et al., 2020). In addition, the downstream transcription factor gene WRKY11 was found to be up-regulated in the resistant B. napus mutant line upon Xcc infection. WRKY11 was shown to mediate Ca2+-dependent interactions with calmodulin and to act as a negative regulator of basal resistance to P. syringae in Arabidopsis (Park et al., 2005; Journot-Catalino et al., 2006).
Genes involved in hormone signaling displayed more variable and complex patterns of expression upon Xcc infection (Figure 7), which could be caused by the high degree of transcriptional crosstalk between the different phytohormone pathways. Strangely, no SA-related DEGs were detected among the two trait-correlated modules. This was somehow unexpected, given that SA was found to accumulate in a compatible Xcc-B. napus interaction as shown by Islam et al. (2019).
Roles of secondary metabolites in Xanthomonas campestris pv. campestris resistance
Multiple studies revealed the importance of several metabolites in response to Xcc, such as glucosinolates, HAs, flavonoids, and phenolic contents. Such defensive metabolites particularly tend to accumulate in incompatible Xcc-Brassica interactions (Aires et al., 2011; Velasco et al., 2013; Barman et al., 2015; Islam et al., 2018). Our study also revealed transcriptional activation of similar metabolic and biosynthetic processes upon Xcc infection (Figure 5). Multiple DEGs involved in the flavonoid and phenylpropanoid pathways, however, were not found to be up-regulated in the resistant line ZS9mXccR-1, but rather in the susceptible line ZS9mXccS-1 at an early stage of infection (Supplementary Figure 6). This is in contrast with Islam et al. (2017), which shows enhanced expression of genes within diverse secondary metabolism pathways in an incompatible Xcc-B. napus interaction. Notably, two recent RNA-seq analyses on Brassica-Xcc interactions did not highlight the role of secondary metabolites in response to Xcc infection (Song et al., 2020; Sun et al., 2021). KEGG analysis of DEGs from the black and purple modules also did not reveal enriched metabolic pathways. Due to this inconsistency across different studies, the exact role of plant secondary metabolites in response to Xcc infection needs to be further studied in detail.
Conclusion
Considerable efforts have been made to unravel the molecular mechanisms underlying Xcc resistance in Brassica crops in the last decade. In this study, we performed comparative transcriptome analyses on three B. napus lines with contrasting susceptibility using broader unbiased approaches to pinpoint pathways playing key functions in black rot resistance. Our results indicate that B. napus evades Xcc invasion and colonization by transcriptional activation of a multitude of genes involved in (i) early PTI-mediated defenses, including immune recognition and signaling, and (ii) in Ca2+ homeostasis and signaling. Together, this study provides novel insights into the molecular basis of black rot resistance in B. napus and may open new avenues in disease resistance breeding in Brassica crops.
Data availability statement
The original contributions presented in this study are publicly available. This data can be found here: NCBI, PRJNA748871.
Author contributions
SL, KB, and MS designed the research. LY performed the research and wrote the draft manuscript. CZ, ZB, and LLY assisted in experiments or data analysis. KB and SL edited the manuscript. All authors contributed to the article and approved the submitted version.
Funding
This work was supported by the National Key Research and Development Program of China (grant no. U20A2034). LY was supported by the China Scholarship Council (grant no. 201903250085).
Acknowledgments
Liu Fan at the Beijing Academy of Agriculture and Forestry Sciences (BAAFS) was acknowledged for kindly providing bacterial isolates.
Conflict of interest
The authors declare that the research was conducted in the absence of any commercial or financial relationships that could be construed as a potential conflict of interest.
Publisher’s note
All claims expressed in this article are solely those of the authors and do not necessarily represent those of their affiliated organizations, or those of the publisher, the editors and the reviewers. Any product that may be evaluated in this article, or claim that may be made by its manufacturer, is not guaranteed or endorsed by the publisher.
Supplementary material
The Supplementary Material for this article can be found online at: https://www.frontiersin.org/articles/10.3389/fpls.2022.960874/full#supplementary-material
Supplementary Figure 1 | Lesion lengths on 41 EMS-mutagenized B. napus lines at 8 days after Xcc inoculation.
Supplementary Figure 2 | Disease symptoms on B. napus ZS9, ZS9mXccR-1, and ZS9mXccS-1 at 8 days after inoculation with diverse Xcc races/strains. Bars represent 10 mm.
Supplementary Figure 3 | Bubble diagrams showing the distribution of GO enriched terms in ZS9mXccR-1 (A) and ZS9mXccS-1 (B). Color bars and dot sizes refer to padj values of enriched GO terms and numbers of involved DEGs, respectively.
Supplementary Figure 4 | Network topology analysis. (A) Topology and connectivity based on a multitude of soft thresholding powers. (B) Heatmaps of DEGs belonging to eight different modules. (C) Correlations between different modules and samples. Color bars represent negative (blue) and positive (red) correlations. (D) Expression of hub genes within black and purple modules at 0, 5, and 8 dpi, respectively.
Supplementary Figure 5 | Correlation analysis of gene expression obtained by Q-RT-PCR and RNA-seq analysis.
Supplementary Figure 6 | Heatmaps of DEGs involved in flavonoid (A) and phenylpropanoid (B) pathways.
Supplementary Data 1 | R script for WGCNA analysis.
References
Acharya, B. R., Raina, S., Maqbool, S. B., Jagadeeswaran, G., Mosher, S. L., Appel, H. M., et al. (2007). Overexpression of CRK13, an Arabidopsis cysteine-rich receptor-like kinase, results in enhanced resistance to Pseudomonas syringae. Plant J. 50, 488–499. doi: 10.1111/j.1365-313X.2007.03064.x
Afrin, K. S., Rahim, M. A., Park, J.-I., Natarajan, S., Kim, H.-T., and Nou, I.-S. (2018). Identification of NBS-encoding genes linked to black rot resistance in cabbage (Brassica oleracea var. capitata). Mol. Biol. Rep. 45, 773–785. doi: 10.1007/s11033-018-4217-5
Aires, A., Dias, C. S. P., Carvalho, R., Oliveira, M. H., Monteiro, A. A., Simões, M. V., et al. (2011). Correlations between disease severity, glucosinolate profiles and total phenolics and Xanthomonas campestris pv. campestris inoculation of different Brassicaceae. Scientia. Horticulturae. 129, 503–510.
Barman, A. R., Kamei, A., and Dutta, S. (2015). Defence-related enzymatic response in cabbage to Xanthomonas campestris pv. campestris. Arch. Phytopathol. Plant Protect. 48, 711–719.
Bourdais, G., Burdiak, P., Gauthier, A., Nitsch, L., Salojärvi, J., Rayapuram, C., et al. (2015). Large-scale phenomics identifies primary and fine-tuning roles for CRKs in responses related to oxidative stress. PLoS Genet. 11:e1005373. doi: 10.1371/journal.pgen.1005373
Bredow, M., Bender, K. W., Johnson Dingee, A., Holmes, D. R., Thomson, A., Ciren, D., et al. (2021). Phosphorylation-dependent subfunctionalization of the calcium-dependent protein kinase CPK28. Proc. Natl. Acad. Sci. U. S. A. 118:e2024272118. doi: 10.1073/pnas.2024272118
Cai, H., Huang, Y., Chen, F., Liu, L., Chai, M., Zhang, M., et al. (2021). ERECTA signaling regulates plant immune responses via chromatin-mediated promotion of WRKY33 binding to target genes. New Phytolog. 230, 737–756. doi: 10.1111/nph.17200
Chalhoub, B., Denoeud, F., Liu, S., Parkin, I. A., Tang, H., Wang, X., et al. (2014). Plant genetics. Early allopolyploid evolution in the post-Neolithic Brassica napus oilseed genome. Science 345, 950–953. doi: 10.1126/science.1253435
Choi, H. W., Lee, D. H., and Hwang, B. K. (2009). The pepper calmodulin gene CaCaM1 is involved in reactive oxygen species and nitric oxide generation required for cell death and the defense response. Mol. Plant Microbe. Interact. 22, 1389–1400. doi: 10.1094/MPMI-22-11-1389
Dodds, P. N., and Rathjen, J. P. (2010). Plant immunity: Towards an integrated view of plant–pathogen interactions. Nat. Rev. Genet. 11, 539–548. doi: 10.1038/nrg2812
Du, Y., Overdijk, E. J. R., Berg, J. A., Govers, F., and Bouwmeester, K. (2018). Solanaceous exocyst subunits are involved in immunity to diverse plant pathogens. J. Exp. Bot. 69, 655–666. doi: 10.1093/jxb/erx442
Fu, Z. Q., and Dong, X. (2013). Systemic acquired resistance: Turning local infection into global defense. Annu. Rev. Plant Biol. 64, 839–863. doi: 10.1146/annurev-arplant-042811-105606
Gao, M., Liu, J., Bi, D., Zhang, Z., Cheng, F., Chen, S., et al. (2008). MEKK1, MKK1/MKK2 and MPK4 function together in a mitogen-activated protein kinase cascade to regulate innate immunity in plants. Cell Res. 18, 1190–1198. doi: 10.1038/cr.2008.300
Gao, X., Chen, X., Lin, W., Chen, S., Lu, D., Niu, Y., et al. (2013). Bifurcation of Arabidopsis NLR Immune Signaling via Ca2+-dependent protein kinases. PLoS Pathog. 9:e1003127. doi: 10.1371/journal.ppat.1003127
Guzmán-Benito, I., Donaire, L., Amorim-Silva, V., Vallarino, J. G., Esteban, A., Wierzbicki, A. T., et al. (2019). The immune repressor BIR1 contributes to antiviral defense and undergoes transcriptional and post-transcriptional regulation during viral infections. New Phytol. 224, 421–438. doi: 10.1111/nph.15931
Hatsugai, N., Igarashi, D., Mase, K., Lu, Y., Tsuda, Y., Chakravarthy, S., et al. (2017). A plant effector-triggered immunity signalling sector is inhibited by pattern-triggered immunity. EMBO J. 36, 2758–2769.
Hu, Z., Chang, Y. C., Wang, Y., Huang, C. L., Liu, Y., Tian, F., et al. (2013). VisANT 4.0: Integrative network platform to connect genes, drugs, diseases and therapies. Nucleic Acids Res. 41, W225–W231. doi: 10.1093/nar/gkt401
Islam, M. T., Lee, B. R., Das, P. R., La, V. H., Jung, H. I., and Kim, T. H. (2018). Characterization of p-Coumaric acid-induced soluble and cell wall-bound phenolic metabolites in relation to disease resistance to Xanthomonas campestris pv. campestris in Chinese cabbage. Plant Physiol. Biochem. 125, 172–177. doi: 10.1016/j.plaphy.2018.02.012
Islam, M. T., Lee, B. R., Park, S. H., La, V. H., Bae, D. W., and Kim, T. H. (2017). Cultivar variation in hormonal balance is a significant determinant of disease susceptibility to Xanthomonas campestris pv. campestris in Brassica napus. Front. Plant Sci. 8:2121. doi: 10.3389/fpls.2017.02121
Islam, M. T., Lee, B.-R., Park, S.-H., La, V. H., Jung, W.-J., Bae, D.-W., et al. (2019). Hormonal regulations in soluble and cell-wall bound phenolic accumulation in two cultivars of Brassica napus contrasting susceptibility to Xanthomonas campestris pv. campestris. Plant Sci. 285, 132–140. doi: 10.1016/j.plantsci.2019.05.010
Journot-Catalino, N., Somssich, I. E., Roby, D., and Kroj, T. (2006). The transcription factors WRKY11 and WRKY17 act as negative regulators of basal resistance in Arabidopsis thaliana. Plant Cell 18, 3289–3302. doi: 10.1105/tpc.106.044149
Kim, D., Langmead, B., and Salzberg, S. L. (2015). HISAT: A fast spliced aligner with low memory requirements. Nat. Methods 12, 357–360. doi: 10.1038/nmeth.3317
Langfelder, P., and Horvath, S. (2008). WGCNA: An R package for weighted correlation network analysis. BMC Bioinform. 9:559. doi: 10.1186/1471-2105-9-559
Lema, M., Cartea, M. E., Sotelo, T., Velasco, P., and Soengas, P. (2012). Discrimination of Xanthomonas campestris pv. campestris races among strains from northwestern Spain by Brassica spp. genotypes and rep-PCR. Eur. J. Plant Pathol. 133, 159–169.
Lin, H., Wang, M., Chen, Y., Nomura, K., Hui, S., Gui, J., et al. (2022). An MKP-MAPK protein phosphorylation cascade controls vascular immunity in plants. Sci. Adv. 8:eabg8723. doi: 10.1126/sciadv.abg8723
Little, D., Gouhier-Darimont, C., Bruessow, F., and Reymond, P. (2006). Oviposition by Pierid butterflies triggers defense responses in Arabidopsis. Plant Physiol. 143, 784–800.
Liu, F., Sun, T., Wang, L., Su, W., Gao, S., Su, Y., et al. (2017). Plant jasmonate ZIM domain genes: Shedding light on structure and expression patterns of JAZ gene family in sugarcane. BMC Genom. 18:771. doi: 10.1186/s12864-017-4142-3
Livak, K. J., and Schmittgen, T. D. (2001). Analysis of relative gene expression data using real-time quantitative PCR and the 2(-Delta Delta C(T)) Method. Methods 25, 402–408.
Love, M. I., Huber, W., and Anders, S. (2014). Moderated estimation of fold change and dispersion for RNA-seq data with DESeq2. Genome Biol. 15:550. doi: 10.1186/s13059-014-0550-8
Lu, D., Wu, S., Gao, X., Zhang, Y., Shan, L., and He, P. (2009). A receptor-like cytoplasmic kinase, BIK1, associates with a flagellin receptor complex to initiate plant innate immunity. Proc. Natl. Acad. Sci. U. S. A. 107, 496–501.
Lu, Y. J., Li, P., Shimono, M., Corrion, A., Higaki, T., He, S. Y., et al. (2020). Arabidopsis calcium-dependent protein kinase 3 regulates actin cytoskeleton organization and immunity. Nat. Commun. 11:6234. doi: 10.1038/s41467-020-20007-4
Ma, X., Xu, G., He, P., and Shan, L. (2016). SERKing coreceptors for receptors. Trends Plant Sci. 21, 1017–1033. doi: 10.1016/j.tplants.2016.08.014
Mamun, M. A., Islam, M. T., Lee, B. R., La, V. H., Bae, D. W., and Kim, T. H. (2020). Genotypic variation in resistance gene-mediated calcium signaling and hormonal signaling involved in effector-triggered immunity or disease susceptibility in the Xanthomonas campestris pv. campestris–Brassica napus pathosystem. Plants 9:303. doi: 10.3390/plants9030303
Meng, H., Sun, M., Jiang, Z., Liu, Y., Sun, Y., Liu, D., et al. (2021). Comparative transcriptome analysis reveals resistant and susceptible genes in tobacco cultivars in response to infection by Phytophthora nicotianae. Sci. Rep. 11:809. doi: 10.1038/s41598-020-80280-7
Ngou, B. P. M., Ahn, H.-K., Ding, P., and Jones, J. D. G. (2021). Mutual potentiation of plant immunity by cell-surface and intracellular receptors. Nature 592, 110–115. doi: 10.1038/s41586-021-03315-7
Park, C. Y., Lee, J. H., Yoo, J. H., Moon, B. C., Choi, M. S., Kang, Y. H., et al. (2005). WRKY group IId transcription factors interact with calmodulin. FEBS Lett. 579, 1545–1550. doi: 10.1016/j.febslet.2005.01.057
Parvin, S., Lee, O. R., Sathiyaraj, G., Khorolragchaa, A., Kim, Y.-J., Devi, B. S. R., et al. (2012). Interrelationship between calmodulin (CaM) and H2O2 in abscisic acid-induced antioxidant defense in the seedlings of Panax ginseng. Mol. Biol. Rep. 39, 7327–7338. doi: 10.1007/s11033-012-1564-5
Patel, R. K., and Jain, M. (2012). NGS QC Toolkit: A toolkit for quality control of Next Generation Sequencing data. PLoS One 7:e30619. doi: 10.1371/journal.pone.0030619
Pertea, M., Pertea, G. M., Antonescu, C. M., Chang, T.-C., Mendell, J. T., and Salzberg, S. L. (2015). StringTie enables improved reconstruction of a transcriptome from RNA-seq reads. Nat. Biotechnol. 33, 290–295. doi: 10.1038/nbt.3122
Rao, S., Zhou, Z., Miao, P., Bi, G., Hu, M., Wu, Y., et al. (2018). Roles of receptor-like cytoplasmic kinase VII members in pattern-triggered immune signaling. Plant Physiol. 177, 1679–1690.
Rubiato, H. M., Liu, M., O’Connell, R. J., and Nielsen, M. E. (2022). Plant SYP12 syntaxins mediate an evolutionarily conserved general immunity to filamentous pathogens. ELife 11:e73487. doi: 10.7554/eLife.73487
Shaw, R. K., Shen, Y., Wang, J., Sheng, X., Zhao, Z., Yu, H., et al. (2021). Advances in multi-omics approaches for molecular breeding of black rot resistance in Brassica oleracea L. Front. Plant Sci. 12:742553. doi: 10.3389/fpls.2021.742553
Song, L., Tang, J., Yan, J., Zeng, A., Lv, S., Gao, B., et al. (2020). Transcriptomic analysis of resistant and susceptible cabbage lines reveals differential expressions and candidate genes involved in cabbage early responses to black rot. 3 Biotech 10:308. doi: 10.1007/s13205-020-02256-8
Sturn, A., Quackenbush, J., and Trajanoski, Z. (2002). Genesis: Cluster analysis of microarray data. Bioinformatics 18, 207–208.
Sun, Q., Zhang, E., Liu, Y., Xu, Z., Hui, M., Zhang, X., et al. (2021). Transcriptome analysis of two lines of Brassica oleracea in response to early infection with Xanthomonas campestris pv. campestris. Can. J. Plant Pathol. 43, 127–139.
Velasco, P., Lema, M., Francisco, M., Soengas, P., and Cartea, M. E. (2013). In vivo and in vitro effects of secondary metabolites against Xanthomonas campestris pv. campestris. Molecules 18, 11131–11143.
Vicente, J. G., and Holub, E. B. (2013). Xanthomonas campestris pv. campestris (cause of black rot of crucifers) in the genomic era is still a worldwide threat to brassica crops. Mol. Plant Pathol. 14, 2–18. doi: 10.1111/j.1364-3703.2012.00833.x
Wang, G., Roux, B., Feng, F., Guy, E., Li, L., Li, N., et al. (2015). The decoy substrate of a pathogen effector and a pseudokinase specify pathogen-induced modified-self recognition and immunity in plants. Cell Host Microbe. 18, 285–295. doi: 10.1016/j.chom.2015.08.004
Wang, H., Liu, G., Zheng, Y., Wang, X., and Yang, Q. (2004). Breeding of a Brassica napus cultivar Zhongshuang No. 9 with high-resistance to Sclerotinia sclerotiorum and dynamics of its important defense enzyme activity. Scientia. Agricultura. Sin. 37, 23–28.
Wang, L., Tsuda, K., Sato, M., Cohen, J. D., Katagiri, F., and Glazebrook, J. (2009). Arabidopsis CaM binding protein CBP60g contributes to MAMP-induced SA accumulation and is involved in disease resistance against Pseudomonas syringae. PLoS Pathogens. 5:e1000301. doi: 10.1371/journal.ppat.1000301
Wang, W., Liu, N., Gao, C., Cai, H., Romeis, T., and Tang, D. (2020). The Arabidopsis exocyst subunits EXO70B1 and EXO70B2 regulate FLS2 homeostasis at the plasma membrane. New Phytol. 227, 529–544. doi: 10.1111/nph.16515
Wang, Y., Bouwmeester, K., Beseh, P., Shan, W., and Govers, F. (2014). Phenotypic analyses of Arabidopsis T-DNA insertion lines and expression profiling reveal that multiple L-type lectin receptor kinases are involved in plant immunity. Mol. Plant Microbe. Interact. 27, 1390–1402. doi: 10.1094/MPMI-06-14-0191-R
Wang, Y., Schuck, S., Wu, J., Yang, P., Döring, A. C., Zeier, J., et al. (2018). A MPK3/6-WRKY33-ALD1-Pipecolic acid regulatory loop contributes to systemic acquired resistance. Plant Cell 30, 2480–2494. doi: 10.1105/tpc.18.00547
Wei, L., Jian, H., Lu, K., Filardo, F., Yin, N., Liu, L., et al. (2016). Genome-wide association analysis and differential expression analysis of resistance to Sclerotinia stem rot in Brassica napus. Plant Biotechnol. J. 14, 1368–1380. doi: 10.1111/pbi.12501
Xie, C., Mao, X., Huang, J., Ding, Y., Wu, J., Dong, S., et al. (2011). KOBAS 2.0: A web server for annotation and identification of enriched pathways and diseases. Nucleic Acids Res. 39, W316–W322. doi: 10.1093/nar/gkr483
Yadeta, K. A., Elmore, J. M., Creer, A. Y., Feng, B., Franco, J. Y., Rufian, J. S., et al. (2017). A cysteine-rich protein kinase associates with a membrane immune complex and the cysteine residues are required for cell death. Plant Physiol. 173, 771–787. doi: 10.1104/pp.16.01404
Yang, J., Wang, G., Ke, H., Zhang, Y., Ji, L., Huang, L., et al. (2019). Genome-wide identification of cyclophilin genes in Gossypium hirsutum and functional characterization of a CYP with antifungal activity against Verticillium dahliae. BMC Plant Biol. 19:272. doi: 10.1186/s12870-019-1848-1
Yang, L., Wu, Y., Zhang, M., Zhang, J., Stewart, J. M., Xing, C., et al. (2018). Transcriptome, cytological and biochemical analysis of cytoplasmic male sterility and maintainer line in CMS-D8 cotton. Plant Mol. Biol. 97, 537–551. doi: 10.1007/s11103-018-0757-2
Yeh, Y. H., Chang, Y. H., Huang, P., Huang, J., and Zimmerli, L. (2015). Enhanced Arabidopsis pattern-triggered immunity by overexpression of cysteine-rich receptor-like kinases. Front. Plant Sci. 6:322. doi: 10.3389/fpls.2015.00322
Yu, G., Wang, L. G., Han, Y., and He, Q. Y. (2012). clusterProfiler: An R package for comparing biological themes among gene clusters. Omics 16, 284–287. doi: 10.1089/omi.2011.0118
Yu, X., Xu, G., Li, B., de Souza Vespoli, L., Liu, H., Moeder, W., et al. (2019). The receptor kinases BAK1/SERK4 regulate Ca(2+) channel-mediated cellular homeostasis for cell death containment. Curr. Biol. 29, 3778–3790. doi: 10.1016/j.cub.2019.09.018
Yuan, M., Ngou, B. P. M., Ding, P., and Xin, X.-F. (2021). PTI-ETI crosstalk: An integrative view of plant immunity. Curr. Opin. Plant Biol. 62:102030. doi: 10.1016/j.pbi.2021.102030
Zhang, X.-R., Xu, Y.-P., and Cai, X.-Z. (2018). SlCNGC1 and SlCNGC14 suppress Xanthomonas oryzae pv. oryzicola-induced hypersensitive response and non-host resistance in tomato. Front. Plant Sci. 9:285. doi: 10.3389/fpls.2018.00285
Zhang, Z., Lenk, A., Andersson, M. X., Gjetting, T., Pedersen, C., Nielsen, M. E., et al. (2008). A lesion-mimic syntaxin double mutant in Arabidopsis reveals novel complexity of pathogen defense signalling. Mol. Plant 1, 510–527. doi: 10.1093/mp/ssn011
Keywords: Brassica napus, black rot resistance, RNA-seq, differential gene expression, Xanthomonas campestris pv. campestris
Citation: Yang L, Zhao C, Bai Z, Yang L, Schranz ME, Liu S and Bouwmeester K (2022) Comparative transcriptome analysis of compatible and incompatible Brassica napus—Xanthomonas campestris interactions. Front. Plant Sci. 13:960874. doi: 10.3389/fpls.2022.960874
Received: 03 June 2022; Accepted: 01 August 2022;
Published: 29 August 2022.
Edited by:
Ales Eichmeier, Mendel University in Brno, CzechiaReviewed by:
Sampath Perumal, University of Saskatchewan, CanadaVan Hien La, Chonnam National University, South Korea
Copyright © 2022 Yang, Zhao, Bai, Yang, Schranz, Liu and Bouwmeester. This is an open-access article distributed under the terms of the Creative Commons Attribution License (CC BY). The use, distribution or reproduction in other forums is permitted, provided the original author(s) and the copyright owner(s) are credited and that the original publication in this journal is cited, in accordance with accepted academic practice. No use, distribution or reproduction is permitted which does not comply with these terms.
*Correspondence: Shengyi Liu, bGl1c3lAb2lsY3JvcHMuY24=; Klaas Bouwmeester, a2xhYXMuYm91d21lZXN0ZXJAd3VyLm5s
†ORCID: Yang Li, orcid.org/0000-0002-9360-4617; Zhao Chuanji, https://orcid.org/0000-0002-2100-2510; M. Eric Schranz, orcid.org/0000-0001-6777-6565; Liu Shengyi, orcid.org/0000-0002-4955-4763; Bouwmeester Klaas, https://orcid.org/0000-0002-8141-3880