- 1State Key Laboratory of Tree Genetics and Breeding, Chinese Academy of Forestry, Hangzhou, China
- 2Key Laboratory of Tree Breeding of Zhejiang Province, Research Institute of Subtropical Forestry, Chinese Academy of Forestry, Hangzhou, China
- 3Forestry Faculty, Nanjing Forestry University, Nanjing, China
Phosphorus (P) is an indispensable macronutrient for plant growth and development, and it is involved in various cellular biological activities in plants. Camellia oleifera is a unique high-quality woody oil plant that grows in the hills and mountains of southern China. However, the available P content is deficient in southern woodland soil. Until now, few studies focused on the regulatory functions of microRNAs (miRNAs) and their target genes under low inorganic phosphate (Pi) stress. In this study, we integrated small RNA, degradome, and transcriptome sequencing data to investigate the mechanism of low Pi adaptation in C. oleifera. We identified 40,689 unigenes and 386 miRNAs by the deep sequencing technology and divided the miRNAs into four different groups. We found 32 miRNAs which were differentially expressed under low Pi treatment. A total of 414 target genes of 108 miRNAs were verified by degradome sequencing. Gene ontology (GO) functional analysis of target genes found that they were related to the signal response to the stimulus and transporter activity, indicating that they may respond to low Pi stress. The integrated analysis revealed that 31 miRNA–target pairs had negatively correlated expression patterns. A co-expression regulatory network was established based on the profiles of differentially expressed genes. In total, three hub genes (ARF22, WRKY53, and SCL6), which were the targets of differentially expressed miRNAs, were discovered. Our results showed that integrated analyses of the small RNA, degradome, and transcriptome sequencing data provided a valuable basis for investigating low Pi in C. oleifera and offer new perspectives on the mechanism of low Pi tolerance in woody oil plants.
Introduction
Phosphorus (P) is an indispensable macronutrient required for plant growth and development and is involved in energy transfer, metabolic regulation, protein activation, and other cellular biological activities in plants (Wang Y. et al., 2021; Wang Z. et al., 2021; Navarro and Munné-Bosch, 2022). Inorganic phosphate (Pi) in soil exists mainly in the form of organophosphate compounds and inorganic Pi (HPO42– and H2PO4–) (Lidbury et al., 2022). Although some soils contain high levels of Pi, the low solubility and high adherence of Pi in soil lead to a lack of available Pi, which is one of the factors that limits crop yield (Crombez et al., 2019). To maintain high crop production, an excessive amount of phosphate fertilizer is often applied to the soil, leading to severe water eutrophication (Conley et al., 2009; Johnston et al., 2014). Moreover, the already insufficient phosphate rock resources are declining and speculated to deplete within 50–100 years (Cordell et al., 2009).
Plants maintain cellular Pi homeostasis by coordinating Pi acquisition, storage, translocation, and remobilization (Fan X. et al., 2021; Jia et al., 2021; Wang Z. et al., 2021). In response to low Pi environments, plants produce various Pi starvation responses to survive. The Pi starvation response is modulated by gene regulatory networks that involve hundreds of Pi transporters and Pi starvation-induced genes (Rubio et al., 2001; He et al., 2015; Wang F. et al., 2018). Pi starvation-induced genes include transcription factors, signal molecules, and other upstream regulators. In Arabidopsis, nine phosphate transport 1 (Pht1) genes were involved in the absorption of Pi from the soil and the redistribution of Pi in plants (Smith et al., 2003). Phosphate starvation response 1 (AtPHR1) and other related transcription factors play vital roles in regulating the Pi starvation response. In the presence of Pi, SYG1/PHO81/XPR1 (SPX1) shows high binding affinity to PHR1, which inhibits the interaction of PHR1 with its conserved P1BS motif. However, in the absence of Pi, the binding affinity of SPX1 to PHR1 is reduced, allowing the PHR1–P1BS interaction and subsequent induction of transcription to take place (Liu N. et al., 2018; Li et al., 2021). For example, in rice (Oryza sativa), OsSPX1 and OsSPX2 interact with OsPHR2 and repress the activity of OsPHR2 in a similar way to that in Arabidopsis (Wang et al., 2014; Tyagi et al., 2021). The phosphorus-responsive transcription factors OsPHR1/2/3 are located in the core of the mycorrhizal symbiotic transcription regulatory network in plants under Pi starvation (Shi et al., 2021).
Recently, Pi starvation-induced genes, mainly those that encode signaling molecules and transcription factors, have been studied extensively in Arabidopsis and rice (Sun et al., 2012; Wang Q. et al., 2018). MicroRNAs (miRNAs) have also been shown to play crucial roles in regulating the Pi starvation response at the posttranscription level (Li et al., 2010; Wang S. et al., 2021). A large number of differentially expressed miRNAs involved in the response to low Pi stress have been identified by using small RNA (sRNA) sequencing. In soybean (Glycine max), 36 differentially expressed miRNAs involved in the response to Pi starvation were identified (Xu et al., 2013). In poplar (Populus tomentosa), three novel and 65 known miRNAs were found to respond dynamically to Pi fluctuations, and miR167, miR171, miR394, and miR857 participated in low Pi stress response (Bao et al., 2019). The functions of miRNAs in the response to Pi starvation have also been investigated. In Arabidopsis, miRNA399 was the first shown to regulate the Pi stress response. The expression of miRNA399 was upregulated when Pi was deficient and decreased quickly when Pi was sufficient (Fujii et al., 2005). Subsequently, six miRNA399 genes (miRNA399A–F) in Arabidopsis were found to be induced differently under low Pi stress, and three genes (phosphate transporter 1;7, DEAD box, and ubiquitin E2 conjugase) were predicted to be regulated by miRNA399, but only ubiquitin E2 conjugase (UBC24) was confirmed to be a target (Allen et al., 2005). In miRNA399-overexpressed Arabidopsis lines, the expression level of UBC24 mRNA was reduced significantly (Pegler et al., 2021). Several conserved miRNA families and some novel miRNAs have also been shown to respond to Pi starvation in various plant species. In wheat (Triticum aestivum), TaemiR408 was shown to be of great importance for adaptation to Pi starvation, and its overexpression in tobacco (Nicotiana tabacum) increased Pi accumulation upon Pi deprivation by improving Pi absorption (Bai et al., 2018). In Pi-deprived plants, TamiR1139 overexpression in wheat enhanced the phenotype, biomass, photosynthesis, and Pi acquisition, which suggested that TamiR1139 was vital in plant Pi starvation tolerance by transcriptionally regulating the target genes and modulating the Pi stress–defense processes (Liu Z. et al., 2018). In alfalfa (Medicago sativa), under Pi deficiency, the expression of miR399 and miR398 was upregulated and the expression of miR159, miR156, miR171, miR160, and miR2111 was downregulated (Li et al., 2018). In the woody tree Betula luminifera, the functions of miR395, miR397, miR169, and miR399 in response to Pi starvation were hypothesized to affect downstream biological processes (Zhang et al., 2021). In brief, the functions of Pi-responsive miRNAs have been studied mainly in herbaceous plants. Many of these miRNAs are species-specific and regulate biological processes by binding to their target genes.
Camellia oleifera is a unique high-quality woody oil plant that grows in the hills and mountains of southern China and has a long history of cultivation and consumption. Tea oil is a high-quality edible oil rich in nutrients. The unsaturated fatty acid content of the oil is approximately 80%, and it is easily absorbed and digested by the human body, thus giving it the name “Oriental Olive Oil [sic]” (Shi et al., 2020). Suitable distribution areas of C. oleifera have red, yellow, or yellow-brown soil with pH values of 4.5–6.5; however, mineral nutrient deficiencies are common in these areas (Li et al., 2019). Pi deficiency reduces C. oleifera biomass and Pi accumulation and also affects germination and fruiting (Wu et al., 2019). Until now, research on the adaptation of C. oleifera to low Pi has focused on the physiological aspects, and few reports focused on miRNAs (Zhou et al., 2020; Sun et al., 2021). The molecular mechanism of low Pi adaptation in C. oleifera requires further investigation to explain its resistance to low Pi. In this study, we aimed to identify miRNAs and their target genes that respond to under low Pi stress in the C. oleifera. For this, we combined the sRNA, degradome, and transcriptome sequencing data and generated an integrated resource for recognizing pivotal regulatory miRNA–target gene interactions in plants under low Pi stress. Our results will provide genetic resources for cultivating improved C. oleifera varieties that can adapt to low Pi environments and also provide a theoretical foundation for further alleviating the problems of Pi deficiency and environmental pollution.
Materials and methods
Plant materials and low phosphate stress treatment
Camellia oleifera “changlin166” cuttings (semi-lignified shoots and approximately 20 cm long) were collected from the Dongfanghong Forestry Farm in Jinhua City, Zhejiang Province, China, in June 2019. The cuttings from one single genotype were asexually propagated and cultivated and then grown in an artificial climate culture room under controlled conditions (25–28°C, 16-h light, 8-h dark). The experiment was performed in March 2021. First, individual plastic pots (19 × 11 cm) were filled with sand and placed in a climate chamber. Before beginning the treatment, the seedlings were irrigated every 3 days with a 1/2-strength Hoagland nutrient solution (Coolaber, China). Then, similarly developed plants were treated as follows: (1) control, 1/2-strength phosphorus-free Hoagland nutrient solution supplemented with 1 mM KH2PO4 and (2) low Pi treatment, 1/2-strength phosphorus-free Hoagland nutrient solution plus 5 μM KH2PO4. The pH of the nutrient solution was adjusted to 5.8 ± 0.1 by adding diluted HCl or NaOH. Then, 30 uniformly developed plants were divided into five groups, and a completely randomized block design with three replicates was used. Their roots were sampled at CK (control), 1, 3, 7, and 30 days, respectively. Fine powder (100 mg dry weight) was digested in a mixture of 5 mL 98% H2SO4 and 1 mL H2O2, and the P content was determined spectrophotometrically at 700 nm based on the molybdenum blue method (Sun et al., 2012). ATPase activity in the roots was analyzed using the modified p-nitrophenyl phosphate (NPP) method (Liu et al., 2004). Samples from the same batch were used for small RNA, transcriptome, and degradome high-throughput sequencing.
All collected root samples were frozen quickly in liquid nitrogen and stored at –80°C. Total RNA was extracted using the Total RNA Purification Kit (NORGEN, Thorold, ON, Canada). The RNA was treated with DNase I (Takara, Dalian, China) to remove any genomic DNA contamination. The integrity and concentration of RNA were measured by denaturing 1.2% (p/v) agarose gel electrophoresis and using a 2100 Bioanalyzer (Agilent Technologies, Santa Clara, CA, United States), respectively. The RNA concentrations of C. oleifera were 305.24–610.30 ng⋅μL–1. Each sample had an RNA integrity number > 8.0.
To construct cDNA libraries for transcriptome sequencing, the mRNA was obtained from the total RNA using Oligo (dT) magnetic beads and fragmented. First-strand cDNA was synthesized using six random hexamer primers and reverse transcriptase. Second-strand cDNA was synthesized using second-strand buffer, DNA polymerase I, RNase H, and RNase-free deionized water. This was followed by end-repairing of the double-stranded cDNA, and an “A” residue was added to the 3′ end. Then, specific sequencing adapters were attached to both ends of the DNA fragments. The ligated cDNA fragments were purified by gel-tipping (generally, 200 500-bp-long fragments are recovered), and high-fidelity enzymes were used to amplify the sequencing libraries. For sequencing libraries, quality control of raw data, adapters, and trimers and cleanup of low-quality reads were performed by FastQC software. The final products were loaded onto an Illumina HiSeq™ 2500 platform for transcriptome sequencing.
For sRNA sequencing, RNA was isolated from three replicates at five time periods and used to construct 15 sRNA libraries. Gel separation technology was used to collect 18- to 30-nt or 18- to 40-nt fragments in the samples, and adapters were ligated to their 5′ and 3′ ends. The fragments were used for cDNA synthesis, followed by PCR amplification and single-strand separation. The obtained single-stranded cDNA will be circularized and digested. The final products were sequenced on an Illumina HiSeq™ 2500 platform.
Library construction was performed as described previously (German et al., 2008). Raw reads (single-end; 50 bp) with adapter or primer contamination were removed using Trimmomatic V0.35 (Bolger et al., 2014) to obtain clean degradome reads, which were then mapped to the C. oleifera transcriptome sequencing data.
Analysis of transcriptome sequencing data
To ensure that the reads were of high enough quality, the low-quality raw sequencing data were removed and clean reads were obtained. Trinity1 with default parameters was used to evaluate the unigene sets obtained by assembling clean reads. Then, the unigenes were searched against the NCBI non-redundant (nr2), Kyoto Encyclopedia of Genes and Genomes (KEGG3), and Gene Ontology (GO4) databases to obtain protein functional annotations and metabolic pathway information (Ünlü et al., 2021). Using the assembled unigenes as the reference, the reads in each sample were mapped to the reference sequences to obtain the read coverage of each unigene in each sample, and then the fragments per kilobase of exon model per million mapped fragments (FPKM) normalization formula was applied to normalize the number of enriched fragments. Differentially expressed genes (DEGs) were identified between the different samples using DE-Seq software (Kvam et al., 2012). A gene was considered to be differentially expressed with |log2(foldchange)| > 1 and p-value ≤ 0.05.
Analysis of the sRNA sequencing data
Adapters, low-quality sequences, regions of low complexity, and common RNA families (tRNA, rRNA, snRNA, and snoRNA) were removed using RFam.5 Clean and unique reads were aligned to the miRBase database (v22.0)6 using BLAST to identify known miRNAs (Ling et al., 2014). The parameters for known and novel miRNAs and their precursor structure identification were referenced by Axtell and Meyers (2018). The sRNA sequencing data from 15 samples have been deposited in the NCBI SRA database7 under accession numbers SRR18672953–SRR18672967. Differentially expressed miRNAs with | log2(foldchange)| > 1 and adjusted p-values < 0.05 were selected for further analysis.
Analysis of degradome sequencing data
Adapter and low-quality sequences obtained by sequencing using the Illumina HiSeq™ 2500 platform were removed, and the adaptor and the clean reads were compared with the assembled unigene reference sequences to obtain the degraded mRNA fragments. Then, miRNA-mRNA degradation sites were analyzed using CleaveLand 4.08 (Addo-Quaye et al., 2009). The target genes were divided into five categories (0, 1, 2, 3, and 4) based on their transcript abundance levels. The predicted target genes were searched against the NCBI nr and nt databases and Swiss-Prot to annotate them. The candidate target genes were functionally annotated by GO and KEGG enrichment analyses.
cDNA and miRNA syntheses and analyses of gene transcription levels
In total, eight miRNA–target genes were randomly selected for fluorescent qPCR analysis. Reverse transcription was performed using an Mir-X™ miRNA First Strand Synthesis Kit (Takara, Dalian, China) and a PrimeScript™ RT Master Mix (Perfect Real Time) Kit (Takara, Dalian, China) according to the manufacturers’ instructions. The expression levels of the miRNAs and their target genes were determined using an Mir-X miRNA qRT-PCR TB Green® Kit (Takara, Dalian, China) and TB Green® Premix Ex Taq™ II (Tli RNaseH Plus) (Takara, Dalian, China), respectively, on a 7300 Real-Time PCR System (Applied Biosystems, CA, United States). Each sample was run in triplicate. The expression levels of the mRNAs and miRNAs were normalized to 18S rRNA and GAPDH, respectively (Zhou et al., 2013), and were calculated using the 2–ΔΔCt method (Livak and Schmittgen, 2001). The primers for miRNAs and their target genes are listed in Supplementary Table 1.
Co-expression network construction
Weighted gene co-expression network analysis (WGCNA) is the systematic biology method for analyzing the correlation patterns among differentially expressed genes across different samples (DiLeo et al., 2011; de Jong et al., 2012). A co-expression network analysis package is freely available at: https://horvath.genetics.ucla.edu/html/CoexpressionNetwork/Rpackages/WGCNA (Langfelder and Horvath, 2008). In brief, the expression profiles of all expressed genes under Pi stress conditions were used to construct a co-expression network. A matrix of pairwise correlation coefficients was used to construct a weighted adjacency matrix that contains pairwise connection strengths using the soft threshold method (power = 6) (de Jong et al., 2012). Rank clustering was performed based on the similarity between genes, and then, the genes with consistent expression in the gene cluster tree are partitioned into the same module (Zhang and Horvath, 2005). Each module is represented by a color, and gene expression profiles were summarized for each module using module signature genes. The gene expression profiles of each module were summarized by the module eigengene and defined as the first principal component of the module expression levels. The targets of differentially expressed miRNAs were selected as hub genes. To display the hub genes, we used Cytoscape software (v3.9.1)9 to produce a gene regulatory network diagram based on the data obtained from the transcriptome and degradome analyses (Zhang et al., 2020). Genes with the same or similar functions were classified into one group by GO annotation.
Results
Phosphate deprivation alters physiological characteristics
After 30 days of Pi starvation, the root/shoot ratio was increased significantly in roots, and the Pi content in shoots was decreased significantly. However, ATPase activity in roots increased significantly under Pi deprivation. The seedlings of C. oleifera showed attenuated growth and increased root biomass after 30 days of Pi starvation (Figure 1).
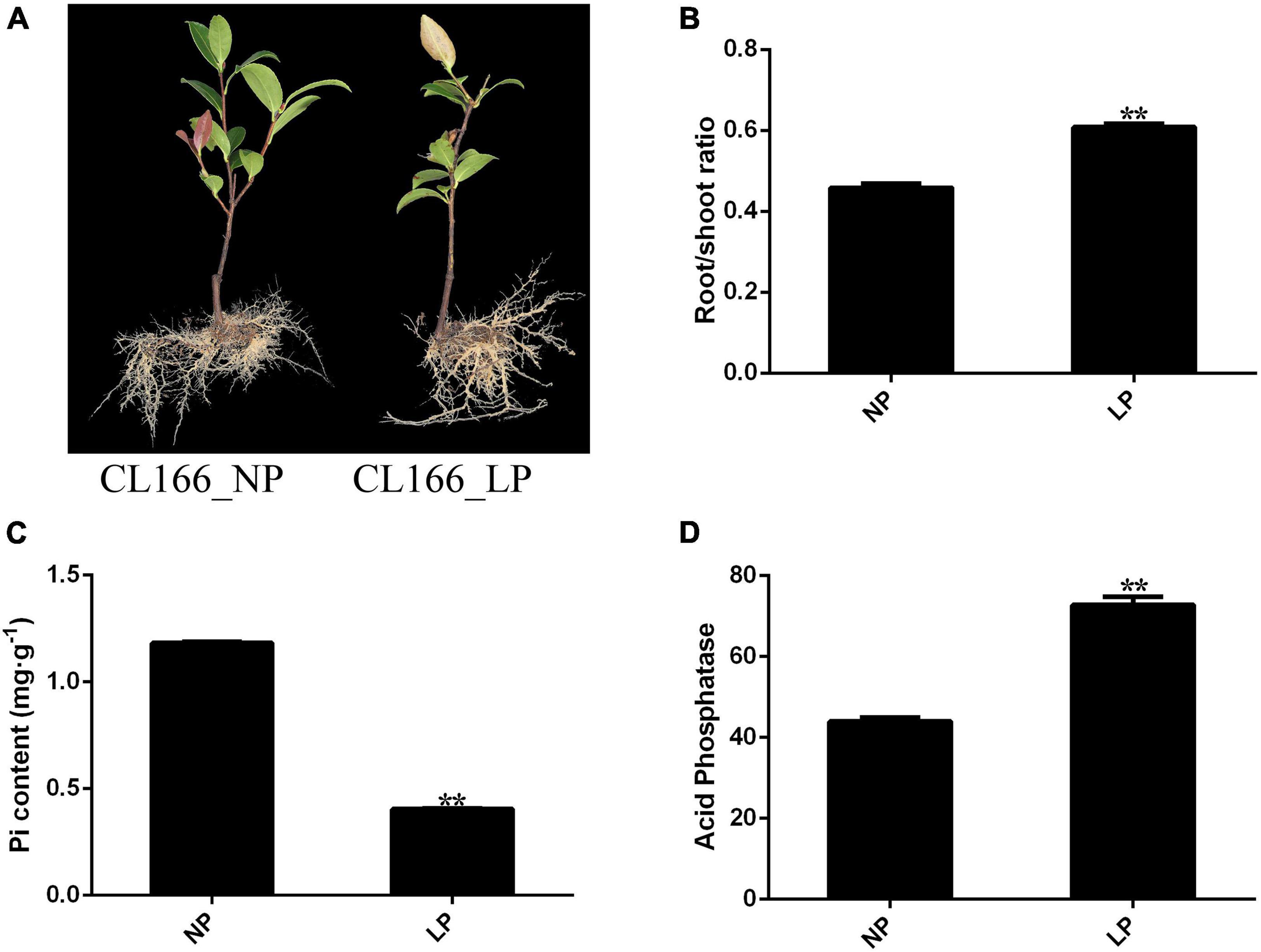
Figure 1. (A) Changes in phenotype. (B) Root/shoot ratio. (C) Pi content. (D) Acid phosphate. Bars indicate means ± SE (n = 4). P-values were obtained from t-tests between LP and NP condition. **P < 0.01.
Transcriptome sequencing in Camellia oleifera roots under low phosphate treatments
Transcriptome libraries were constructed using the extracted total RNA, and 29,389,514 assembled bases were generated. After removing low-quality reads, the remaining high-quality reads were assembled into 40,689 unigenes. Details of the transcriptome sequencing of C. oleifera are shown in Table 1. The unigenes were annotated by sequence alignments to a number of different databases. The BLASTX results identified 27,027 unigenes that were homologs of sequences in the nr database. A total of 23,423 and 18,713 genes were annotated with enriched GO terms and KEGG pathways, respectively. In the low Pi treatment groups, 2,787, 1,657, 1,413, and 4,548 genes were upregulated and 2,666, 1,845, 2,189, and 3,929 genes were downregulated at 1, 3, 7, and 30 days, respectively, compared with their expression levels in the corresponding control groups, and 1,586 differentially expressed mRNAs were common to all the time periods (Supplementary Figure 1). The heatmap of the gene expression level (top 3,000 genes with large expression variation) is shown in Supplementary Figure 2. The FPKM of all unigenes is shown in Supplementary Table 2.
Sequencing and identification of known and novel miRNAs
An overview of the obtained raw and clean sequences is given in Supplementary Table 3, and the 18- to 25-nt-long sequences obtained after deleting low-quality sequences are listed in Supplementary Table 4. The number distribution of the sRNAs is shown in Supplementary Figure 3. The length of C. oleifera sRNAs was 18–24 nt long, and the 21-nt sRNAs were the most abundant in all 15 libraries. A total of 386 miRNAs were found and divided into four separate groups (Figure 2). A total of 214 pre-miRNAs had the highest degree of similarity with 275 known unique mature miRNAs in 63 miRNA families (Supplementary Figure 4), which had a high degree of similarity compared to other known miRNAs. A total of 70 pre-miRNAs corresponding to 68 mature miRNAs were recognized as novel miRNAs (Supplementary Table 5). Among them, miRNAs of a length of 21 nt made up 38.99% of the known miRNAs, and miRNAs of a length of 24 nt made up 45.59% of the novel miRNAs (Supplementary Table 6). The free energy (dG) and minimum folding free energy index (MFEI) of predicted pre-miRNAs ranged from –17.1 to –93.4 kcal/mol and from 0.9 to 1.7 kcal/mol, respectively (Supplementary Table 7). These characteristics maintain the stability of the hairpin structures of the pre-miRNAs.
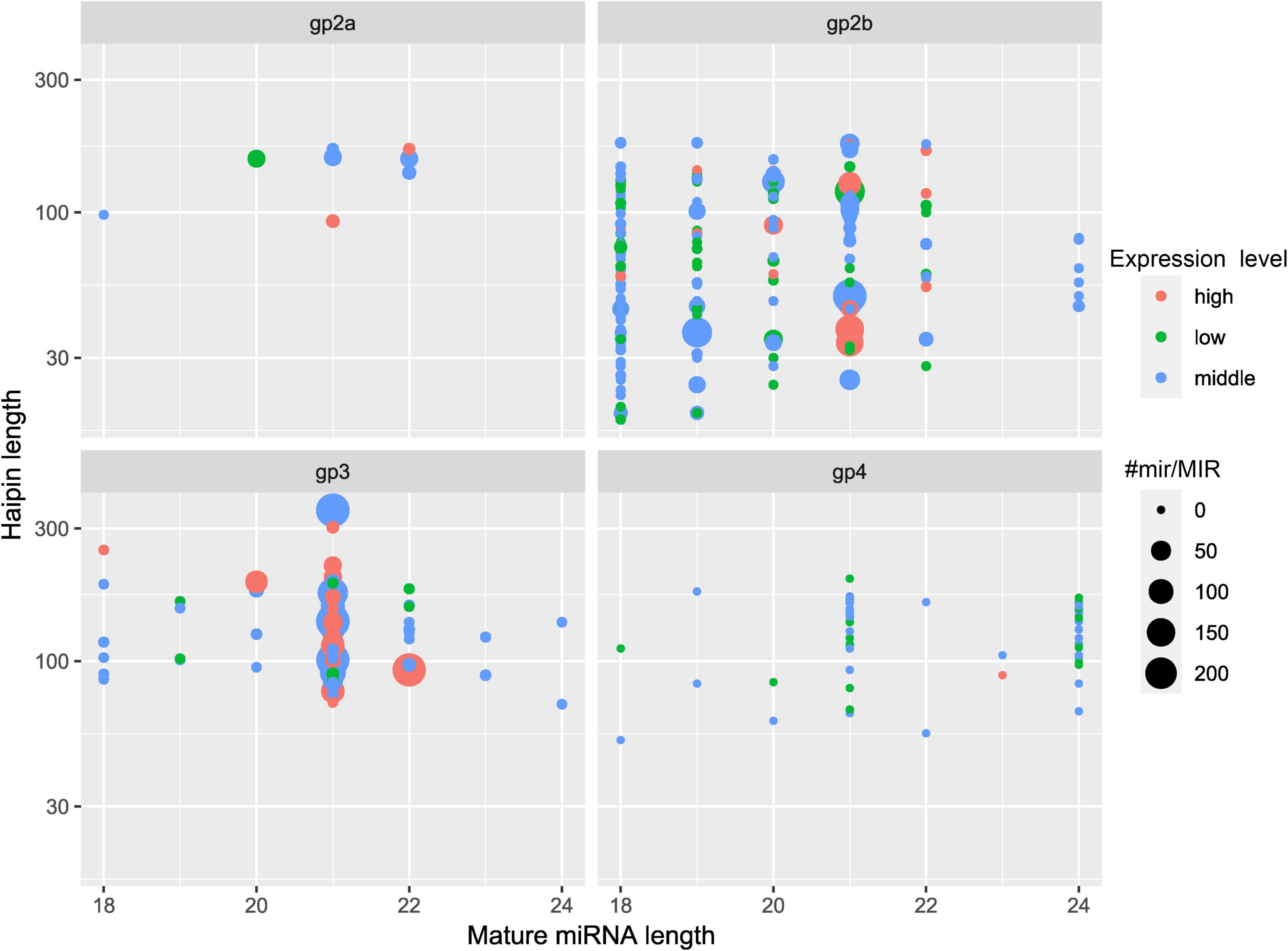
Figure 2. Summary of the classification of the four identified groups of miRNAs in C. oleifera. Counts MIRb, the counts of miRNAs from miRBase; expression level, low indicates < 10; middle indicates > 10 but less than average; high indicates over average.
miRNAs that respond to low phosphate in Camellia oleifera roots
To identify miRNAs involved in the response to Pi deficiency, the expression levels of miRNAs in the five time periods were analyzed and compared. A total of 32 miRNAs (p < 0.05) showed differential expression patterns; among them, 29 were known miRNAs and three were novel miRNAs. A heatmap of differentially expressed miRNAs is shown in Figure 3A. Some members of the same miRNA families had similar expression patterns. For example, two miRNA167s and three miRNA393 were significantly upregulated after low Pi stress for 7 days, and two of the novel miRNAs (PC-3p-24651_983 and PC-5p-174389_91) were upregulated, and one (PC-3p-132795_129) was downregulated (Figure 3A). We counted the distribution of 32 differential miRNAs between the control and treatments groups (Figure 3B). The largest number (17) of upregulated miRNAs was found after low Pi treatment for 1 day, and five miRNAs were significantly downregulated after low Pi treatment for 3 days compared with their expression levels in the corresponding controls (Figure 3B). These findings indicate that the duration of the low Pi treatment may have a short-run effect on the miRNA expression levels.
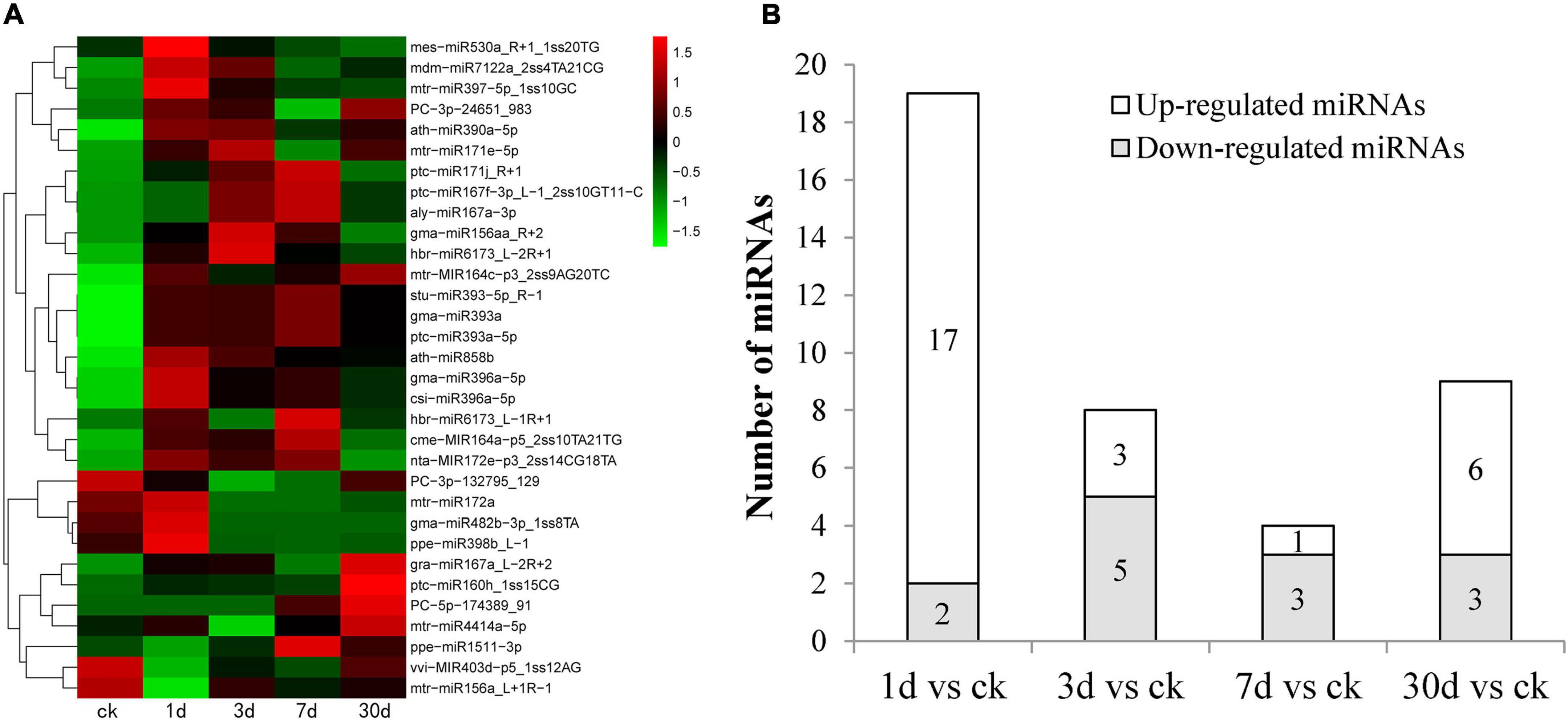
Figure 3. Phosphorus (Pi) deficiency and miRNAs in C. oleifera. (A) Differential expressed miRNAs in five different low Pi treatment durations (CK, 1, 3, 7, and 30 days) by miRNAs hierarchical clustering. Red indicates higher levels of miRNAs and green indicates lower levels of miRNAs. The names of the samples are shown at the bottom. The original expression values of the miRNAs were normalized using Z-score normalization. The absolute signal intensity ranges from −1.5 to +1.5, with corresponding color changes from green to red. (B) The number of differentially expressed miRNAs under Pi deficiency compared with the control.
Prediction of the target genes of the known and novel miRNAs by degradome sequencing
The degradome sequencing generated 61,520,868 raw reads, representing 19,066,404 unique reads from the mixed degradome pool. After removing the low-quality reads and the reads that lacked the adapters, 58,817,780 (95.61% of all reads) sequences were successfully mapped to the 40,689 unigenes. The candidate target genes were divided into five categories, namely, 0, 1, 2, 3, and 4, based on the abundance of degradation sites and transcripts (Addo-Quaye et al., 2009). To select the most credible genes for analysis, we set the alignment score ≤ 4 and category ≤ 2 and obtained 52, 2, and 360 target genes in categories 0, 1, and 2, respectively (Supplementary Table 8). Among the novel miRNAs, 13 miRNAs targeted 21 different genes. In order to predict their authenticity, the target sites of miRNAs and their targets were aligned by psRNATarget10 in Supplementary Table 9 (Dai and Zhao, 2011). In addition, t-plots were also constructed to show the cleavage sites for each miRNA–mRNA pair (Supplementary Figure 6).
A total of 414 target genes for 108 miRNAs were identified from the mixed degradome sequencing data; of the 414 target genes, 51 of the miRNAs were predicted to regulate a single gene and 56 of the miRNAs were predicted to regulate two or more genes (Supplementary Table 8). Target genes of 14 novel miRNAs were also obtained by degradome sequencing; eight of them were predicted to regulate a single gene and six novel miRNAs were predicted to regulate two or more genes (Table 2). The length distribution of the novel miRNAs was similar to that of the known miRNAs. We also found that the expression levels of different miRNAs in the same family were different. These results suggest that different miRNAs may have different mechanisms adapted to Pi deficiency in C. oleifera roots.
Annotation and enrichment analysis of the target genes for miRNAs
The 414 target genes were annotated with terms under the three main GO categories: biological process, cellular component, and molecular function. The enriched terms were visualized using REVIGO software (Liu et al., 2015) and plotted (Figure 4). Under biological processes, the plant hormone signaling pathways (GO:0009873 ethylene-activated signaling pathway, GO:0009734 auxin-activated signaling pathway, and GO:0009738 abscisic acid-activated signaling pathway) and their response to the stimulus (GO:2000306 positive regulation of photomorphogenesis, GO:0048768 root hair cell tip growth, and GO:0071281 cellular response to iron ion) were enriched, which are consistent with the current understanding of Pi deficiency. Under the cellular component, the nucleus and membrane components were the most enriched term. Under molecular function, DNA binding was the most enriched term, and enzymatic activities (GO:0004842 ubiquitin protein transferase activity, GO:0046522 S-methyl-5-thioribose kinase activity, and GO:0004721 phosphoprotein phosphatase activity) were also enriched, which suggests that these enzymes may play essential roles under low Pi conditions.
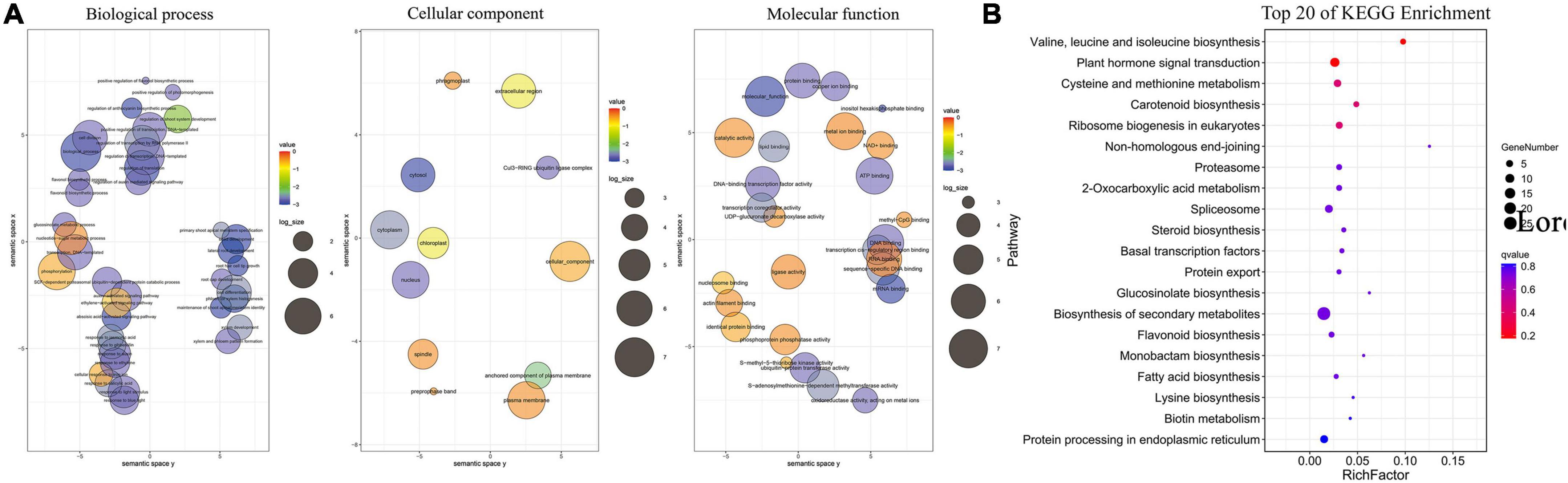
Figure 4. Gene ontology (GO) and Kyoto Encyclopedia of Genes and Genomes (KEGG) functional classification of identified target genes. (A) GO enrichment analysis for targets of DE miRNAs under conditions of phosphate (Pi) starvation. (B) KEGG Enrichment Analysis for Targets of DE miRNAs under conditions of Pi starvation.
The top 20 most enriched KEGG pathways are shown in Figure 4B. Among them, biosynthesis of secondary metabolites (ko01110) and plant hormone signal transduction (ko04075) were the most enriched pathways, which is consistent with GO enrichment results. Plant hormones, such as auxin, salicylic acid, jasmonic acid, ethylene, and gibberellin, are known to be involved in the response to Pi deficiency.
Correlation analysis of the expression profiles of the miRNAs and their target genes
To investigate changes in the whole transcriptome of C. oleifera roots under different Pi treatments, the assembled unigenes were used to analyze the differentially expressed genes. In a survey, 48 genes were the targets of 33 miRNAs, which responded to low Pi tolerance (Table 3), and 31 of the miRNA–target genes were negatively correlated with the absolute value of the negative correlation coefficient greater than 0.5 (Figure 5). For example, ptc-miR160h_1ss15CG was significantly downregulated and its target auxin response factor 22 (ARF22, TRINITY_ DN39928_c3_g4) was significantly upregulated and mtr-miR171d was upregulated and its target SCL6 (Scarecrow-like protein, TRINITY_DN38636_c0_g1) was downregulated under Pi deficiency.
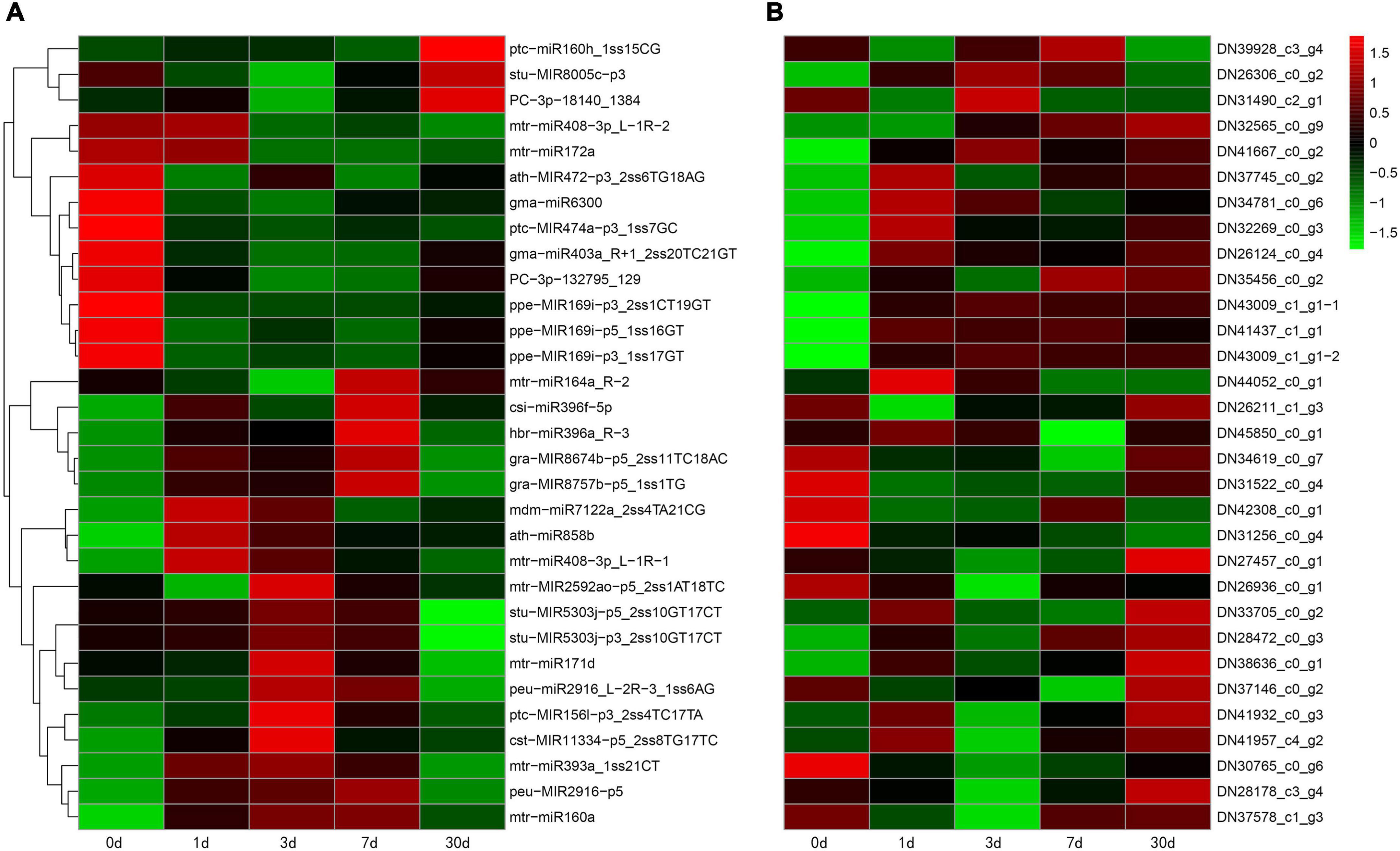
Figure 5. Combined view of expression levels between differentially expressed miRNAs (A) and their target genes (B) in C. oleifera at five different Pi treatment durations. The original expression values of miRNAs and their target genes were normalized by Z-score normalization.
A RT-qPCR analysis was conducted to verify the expression profile of eight key miRNA–target pairs. The results showed that the expression trends of the eight miRNAs and target genes were similar to those obtained by deep sequencing (Figure 6). The expression levels of the eight miRNA–target pairs were confirmed to be negatively correlated. For example, mtr-miR160a and ptc-miR160h_1ss15CG were upregulated and then downregulated at the longer time periods, and their target genes showed significant opposite expression patterns. These results suggest that transcription of the target mRNAs may be repressed by the corresponding miRNAs (Figure 6). These results suggest that transcription of the target miRNAs may be repressed by the corresponding miRNAs (Figure 6).
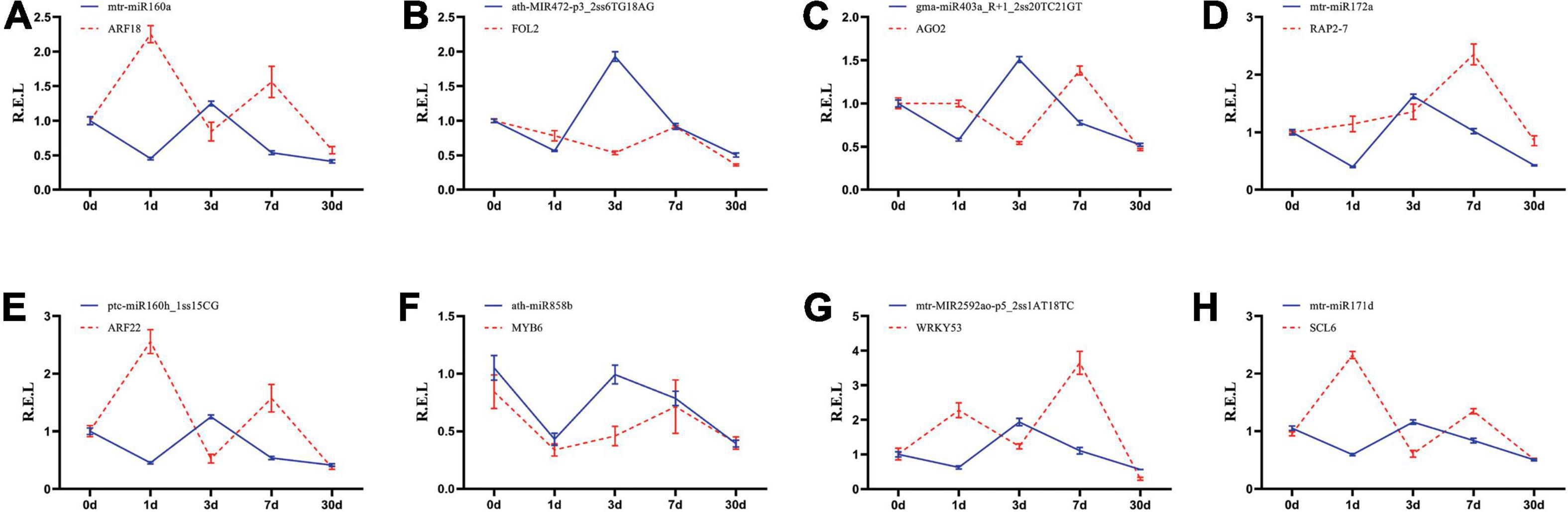
Figure 6. Expression correlation between miRNAs and their targets at five different Pi treatment durations. (A) mtr-miR160a/ARF18; (B) athMIR472p3_2ss6TG18AG/FOL2; (C) gmamiR403a_R + 1_2ss20TC21GT/AGO2; (D) mtr-miR172a/RAP2-7; (E) ptc-miR160h_1ss15CG/ARF22; (F) ath-miR858b/MYB6; (G) mtr-MIR2592ao-p5_2ss1AT18TC/WRKY53; (H) mtr-miR171d/SCL6; The blue and red lines indicate miRNAs and the target abundance, respectively, based on the RT-qPCR results.
Gene co-expression network analysis
After removing unexpressed and lowly expressed genes, we identified 34 gene co-expression modules that contained 40,689 unigenes (Supplementary Figure 5). We constructed a co-expression regulatory network based on different modules (Figure 7). To explore the network connections for the target genes, we focused on three of the differentially expressed target genes (ARF22, ptc-miR160h_1ss15CG target gene; WRKY53, mtr-miR2592ao-p5_2ss1AT18TC target gene; and SCL6, mtr-miR171d target gene), which were hub genes, which may play important roles in low Pi stress. A complete list of the module assignments for the three hub genes is provided in Supplementary Table 10. In the network, the three hub genes ARF22, WRKY53, and SCL6 are directly connected with 735, 46, and 272 edge genes, respectively. These hub genes will provide a foundation for future studies.
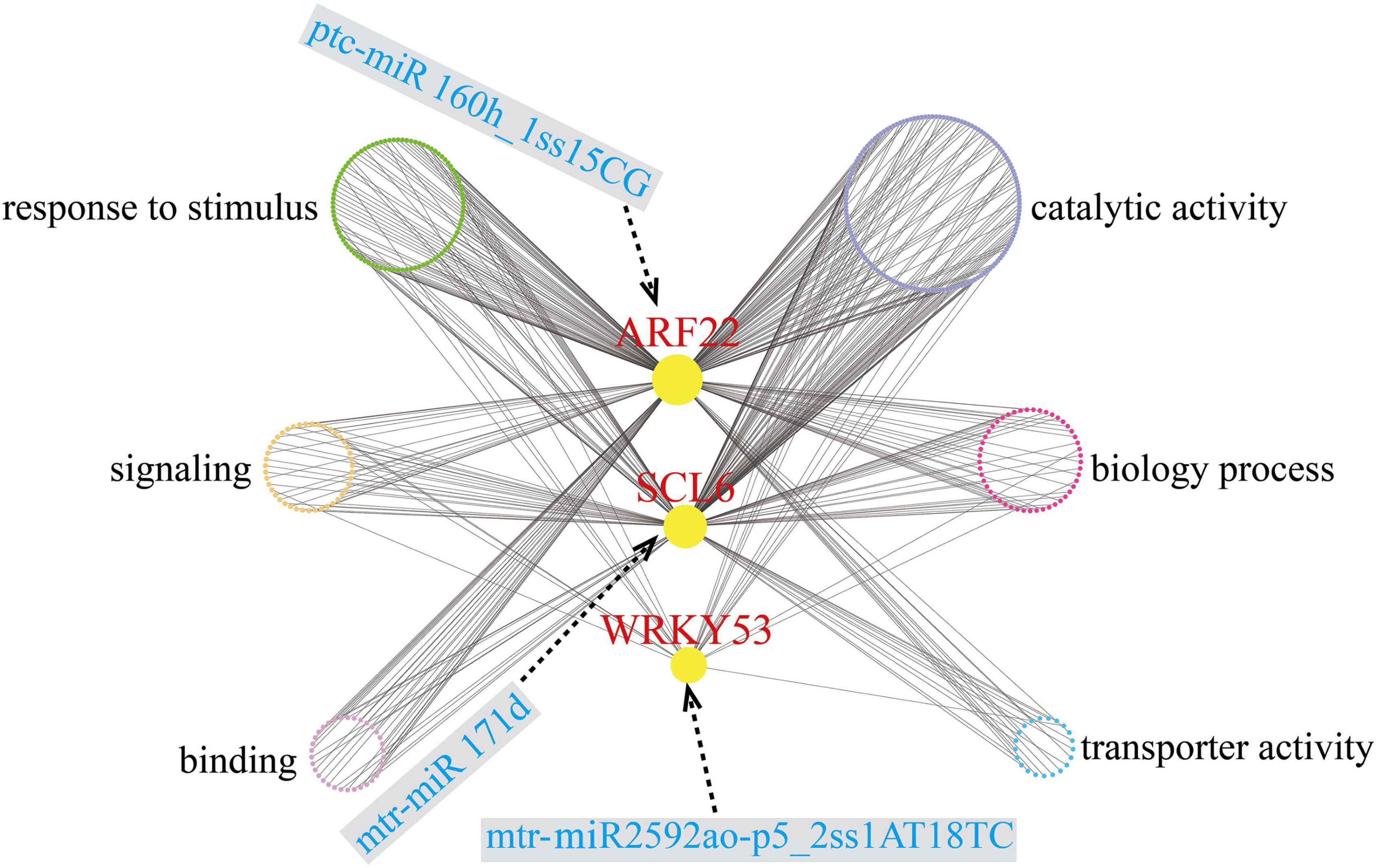
Figure 7. Co-expression subnetwork of ARF22, WRKY53, and SCL6. Red-colored letter denotes key hub gene; blue-colored letter denotes key miRNA; six different colored circles represent six different categories.
We also created a subnetwork containing 390 genes that were annotated with six GO terms related to the response to stimulus, signaling, binding, catalytic activity, biological process, and transporter activity (Figure 7); 94, 44, and 20 of these genes were annotated in the response to stimulus, signaling, and transporter activity terms, respectively (Supplementary Table 11). In the subnetwork, there were three signaling-related transcription factors as hub genes that may be involved in Pi uptake, transport, and homeostasis, and four transporter-related members of the ABC family also may promote Pi uptake and transport. When plants experience Pi deficiency, phosphate transporter and vacuolar H+ pyrophosphatase together maintain Pi balance.
Discussion
Camellia oleifera is a woody oil plant that grows in southern China. It is frequently exposed to low Pi availability in red soils and has evolved a suite of adaptive mechanisms to cope with conditions of Pi deficiency. Understanding the potential molecular mechanisms is essential for screening germplasm resources resistant to low Pi. The regulation of plant resistance to nutritional deficiency by the miRNA–target gene interactions has been described in several herb plants. However, the molecular mechanisms underlying the responses of woody plants to Pi deficiency are poorly understood. In this study, we performed an integrated analysis to investigate the regulatory network in the roots of C. oleifera under Pi deficiency based on miRNA, degradome, and transcriptome sequencing data. Our results showed that the genes targeted by miRNAs play important roles in response to Pi deficiency in the roots. Although our findings provide some understanding of the low Pi adaptation mechanisms, many more studies are needed to gain a comprehensive understanding of the miRNA–target genes of Pi deficiency.
miRNAs have been studied in many plants, but very few studies have been conducted on plants in the family Theaceae, which includes C. oleifera. In this study, the transcriptome sequences of C. oleifera were used as reference sequences for the miRNA and the degradome sequencing analysis. The relationship between miRNAs and target genes was obtained by degradome sequencing. Then, the miRNA–target gene network regulation map showed that the regulation of multiple target genes could be regulated by one miRNA and that one target gene could be regulated by multiple miRNAs. A total of 386 miRNAs were identified and divided into four groups (Figure 2), out of which 284 of them were highly confident miRNAs. Only 14% of them were relatively highly expressed, confirming that high-throughput sequencing can identify miRNAs with low expression levels in plants. The length of mature miRNAs was 18–24 nt (Supplementary Figure 3), which is consistent with previous results in plants such as alfalfa (Li et al., 2018), wheat (Bai et al., 2018), Betula luminifera (Zhang et al., 2021), poplar (Populus tomentosa) (Bao et al., 2019), and pine (Pinus massoniana) (Fan F. et al., 2021).
We identified 32 differentially expressed miRNAs (29 conserved and 3 novel miRNAs) under low Pi stress (Figure 3A). Different members of the same miRNA family had similar expression levels; for example, three miR393 and two miR171 family members were significantly upregulated under low-Pi conditions. Similar phenomena were also found in other species. In Arabidopsis, miR393s play important roles in the root system architecture of Pi deficiency and nitrogen deprivation (Vidal et al., 2010). In Populus tomentosa, the expression of miRNA171e decreased after 24 h of Pi deficiency and then increased dramatically when Piwas supplied (Bao et al., 2019). In maize (Zea mays), under low Pi stress, members of the miR393 family were differentially expressed in the leaves and roots (Gupta et al., 2017). In Medicago truncatula, miR171h is involved in integrating nutrient homeostasis by regulating the expression of NSP2 during arbuscular mycorrhizal and root nodule symbiosis (Hofferek et al., 2014). Therefore, miR393 and miR171 show different expression patterns in response to low Pi in different plants. In our study, we found two miR167 and two miR396 family members that were significantly upregulated by Pi deficiency. In maize, members of the miR396 family were differentially expressed by Pi starvation, zma-miR396a expression was continuously suppressed by Pi starvation, miR396c expression was slightly increased during the early phase of low Pi stress, and zma-miR396d was significantly upregulated in maize leaves (Nie et al., 2016). We also found two miR156 family members that showed contrary expression patterns in C. oleifera roots. miR156 is a highly conserved miRNA that plays key roles in many biological processes, including developmental and metabolic regulation, immune response, and abiotic stress. In Arabidopsis, members of the miR156 family responded to phosphate deprivation and played a potential role in phosphate homeostasis (Hsieh, 2010). Together, these results indicate that miRNAs have diverse functions in plants, and the complex mechanisms need to be further explored in future studies.
We used the transcriptome sequences as reference sequences and analyzed the correlation between miRNAs and their target genes by degradome sequencing. Many target transcripts were identified for the known and novel miRNAs (Han et al., 2016). Mature miRNAs regulate their target mRNAs by forming an miRNA-induced silencing complex that cleaves miRNA or inhibits its translation, thereby negatively regulating the target genes (Sun et al., 2019). miRNAs are known to be involved in abiotic and biotic stresses in plants, and miRNAs can be expressed to act on target genes under abiotic stresses (Cui et al., 2020; Li and Yu, 2021; Ma et al., 2021). We identified 414 genes that were predicted to be targeted by 108 corresponding miRNAs by degradome sequencing (Supplementary Table 8). We also identified 30 differentially expressed target genes that were targeted by 31 miRNAs, and most of these miRNA–target gene pairs had opposite expression patterns (Figure 4), including ptc-miR160h_1ss15CG, which was significantly downregulated under Pi deficiency, whereas its target gene ARF22 (TRINITY_DN39928_c3_g4) was significantly upregulated. However, in Arabidopsis, miR160 and its target genes ARF16, ARF10, and ARF17 regulate hypocotyl elongation in a light, brassinazole (BRZ, a BR biosynthesis inhibitor), or paclobutrazol (PAC, a GA biosynthesis inhibitor)-dependent manner (Dai et al., 2021). This finding also indicates that the same miRNA can regulate different target gene pairs and play different roles in different plants.
miR399 is responsive to Pi starvation and plays important regulatory roles in Pi homeostasis (Pegler et al., 2020, 2021; Peng et al., 2021). In our study, two miR399 members, miR399 and miR399c, which regulated six and 11 mRNAs, respectively, were detected by the analysis of our degradome sequencing data (Supplementary Table 12). However, miR399 and miR399c were not differentially expressed miRNAs, suggesting that C. oleifera may have evolved some other miRNAs in response to low Pi stress. In addition, miR827 was absent in the present sRNA libraries; the possible reason is the lack of its precursor sequence in the draft genome, which led to the loss of miR827 during evolution or the failed match during sRNA library construction. Similar phenomena were observed in B. luminifera and C. papaya, in which the miR827 locus was lost during evolution (Lin et al., 2018; Zhang et al., 2021).
There are 199 miRNA-target genes whose expression levels are negatively correlated (Supplementary Table 13). Overall, three transcription factors as hub genes, ARF22, WRKY53, and SCL6, may play pivotal roles in the regulation of low Pi-responsive genes through a co-expression regulatory network and are critical mediators in regulating various abiotic stresses (Wang X. et al., 2021). In durum wheat (Triticum turgidum subsp. Durum), ttu-miR160 was upregulated, and its target ARF22 was significantly downregulated in roots under chronic and short-term nitrogen stress (Zuluaga et al., 2018). In rice, several miR160s were downregulated in roots and shoots under heat stress (Sailaja et al., 2014). In our study, ARF22 was targeted by ptc-miR160h_1ss15CG, and ptc-miR160h_1ss15CG was slightly downregulated at the early stage and increased dramatically after 30 days under low Pi stress, whereas ARF22 showed opposite expression patterns (Figure 6). WRKY53 is involved in a complicated transcription factor signaling network that regulates senescence-specific gene expression and may participate in signal transduction in Arabidopsis (Miao et al., 2004). Several other WRKY family members were reported to be involved in the low Pi response, such as in an OsWRKY74-overexpressing plant that showed significantly enhanced tolerance to Pi starvation, whereas RNAi lines of OsWRKY74 were sensitive to Pi starvation (Dai et al., 2016). In soybean, WRKY46 showed an opposite expression pattern to that of OsWRKY74. The GmWRKY46-overexpressed plants were more sensitive to low Pi stress than the controls, whereas RNAi lines of GmWRKY46 had significantly enhanced Pi deficiency tolerance (Liu et al., 2022). Previous studies showed that miR171c-targeted SCL6 plays an important role in the control of shoot branch production in Arabidopsis (Wang et al., 2010). In soybean, the miR171–SCL6 pair regulated rhizobium symbiosis (Hossain et al., 2019). We found that the miR171d–SCL6 pair may respond to low Pi availability. We consider that these three hub genes and the miRNAs that target them may have major roles in the response to Pi deficiency in C. oleifera roots. However, the specific regulatory mechanism needs to be further verified.
Conclusion
In the study, we performed an integrated analysis of sRNA, degradome, and transcriptome sequencing data and generated a well-rounded resource centered on identifying key regulatory miRNA–target gene pairs that respond to low Pi stress. A total of 40,689 unigenes and 386 miRNAs were identified, and 32 significantly differentially expressed miRNAs were detected. The edge genes of the target hub genes for the low Pi-responsive miRNAs were involved in transporter activity, the response to the stimulus, catalytic activity, signaling, binding, and biological processes. Furthermore, 30 differentially expressed target genes of 31 differentially expressed miRNAs were identified by a concordant analysis of the expression patterns of the miRNA–target gene pairs. A total of 390 co-expressed genes formed a co-expression regulatory subnetwork in which three hub target genes, ARF22, WRKY53, and SCL6, which may play key roles in controlling transcriptomic regulation in response to low Pi stress, were identified. Our results provided a comprehensive understanding of Pi deficiency in C. oleifera and will help explain miRNA-mediated molecular mechanisms associated with plant responses to low Pi stress.
Data availability statement
The original contributions presented in the study are included in the article and Supplementary material. Transcriptome raw reads sequence data are available through the NCBI Sequence Read Archive (BioProject: PRJNA792896; BioSample: SAMN24471059; SRA Accession Number: SRR17365487-SRR17365501, 15 samples). Degradome raw reads sequence data are available through the NCBI Sequence Read Archive (BioProject: PRJNA795525; BioSample: SAMN24731130; SAMN24471059; SRA Accession Number SRR17494566). Small RNA raw reads sequence data are available through the NCBI Sequence Read Archive (BioProject: PRJNA824141; BioSample: SAMN27393800; SRA Accession Number: SRR18672953-SRR18672967, 15 samples).
Author contributions
JC and XY conceived this project. JC and XH designed experiments and interpreted the results. JC wrote the manuscript. XH provided technical guidance for the experiment. SY, LL, BY, and YC performed the experiments and analyzed the data. RZ and XY provided experimental materials and funds. All authors read and approved the submission of this manuscript.
Funding
This study was supported by the National Key R&D Program of China (2019YFD1001602).
Conflict of interest
The authors declare that the research was conducted in the absence of any commercial or financial relationships that could be construed as a potential conflict of interest.
Publisher’s note
All claims expressed in this article are solely those of the authors and do not necessarily represent those of their affiliated organizations, or those of the publisher, the editors and the reviewers. Any product that may be evaluated in this article, or claim that may be made by its manufacturer, is not guaranteed or endorsed by the publisher.
Supplementary material
The Supplementary Material for this article can be found online at: https://www.frontiersin.org/articles/10.3389/fpls.2022.932926/full#supplementary-material
Footnotes
- ^ http://trinityrnaseq.github.io/
- ^ https://www.ncbi.nlm.nih.gov/refseq/about/non-redundantproteins/
- ^ https://www.genome.jp/kegg/
- ^ http://geneontology.org/
- ^ http://rfam.janelia.org
- ^ http://www.mirbase.org/
- ^ https://www.ncbi.nlm.nih.gov/sra/?term=
- ^ https://github.com/MikeAxtell/CleaveLand4
- ^ https://cytoscape.org/download.html
- ^ https://www.zhaolab.org/psRNATarget/analysis?function=3
References
Addo-Quaye, C., Miller, W., and Axtell, M. J. (2009). CleaveLand: A pipeline for using degradome data to find cleaved small RNA targets. Bioinformatics 25, 130–131. doi: 10.1093/bioinformatics/btn604
Allen, E., Xie, Z., Gustafson, A. M., and Carrington, J. C. (2005). microRNA-directed phasing during trans-acting siRNA biogenesis in plants. Cell 121, 207–221. doi: 10.1016/j.cell.2005.04.004
Axtell, M. J., and Meyers, B. C. (2018). Revisiting criteria for plant microRNA annotation in the era of big data. Plant Cell 30, 272–284. doi: 10.1105/tpc.17.00851
Bai, Q., Wang, X., Chen, X., Shi, G., Liu, Z., Guo, C., et al. (2018). Wheat miRNA TaemiR408 acts as an essential mediator in plant tolerance to Pi deprivation and salt stress via modulating stress-associated physiological processes. Front. Plant Sci. 9:499. doi: 10.3389/fpls.2018.00499
Bao, H., Chen, H., Chen, M., Xu, H., Huo, X., Xu, Q., et al. (2019). Transcriptome-wide identification and characterization of microRNAs responsive to phosphate starvation in Populus tomentosa. Funct. Integr. Genom. 19, 953–972. doi: 10.1007/s10142-019-00692-1
Bolger, A. M., Marc, L., and Bjoern, U. (2014). Trimmomatic: A flexible trimmer for Illumina sequence data. Bioinformatics 30, 2114–2120. doi: 10.1093/bioinformatics/btu170
Conley, D. J., Paerl, H. W., Howarth, R. W., Boesch, D. F., Seitzinger, S. P., Havens, K. E., et al. (2009). Controlling eutrophication: Nitrogen and phosphorus. Science 323, 1014–1015. doi: 10.1126/science.1167755
Cordell, D., Drangert, J. O., and White, S. (2009). The story of phosphorus: Global food security and food for thought. Glob. Environ. Change 19, 292–305. doi: 10.1016/j.gloenvcha.2008.10.009
Crombez, H., Motte, H., and Beeckman, T. (2019). Tackling plant phosphate starvation by the roots. Dev. Cell 48, 599–615. doi: 10.1016/j.devcel.2019.01.002
Cui, C., Wang, J. J., Zhao, J. H., Fang, Y. Y., He, X. F., Guo, H. S., et al. (2020). A Brassica miRNA regulates plant growth and immunity through distinct modes of action. Mol. Plant 13, 231–245. doi: 10.1016/j.molp.2019.11.010
Dai, X., and Zhao, P. X. (2011). psRNATarget: A plant small RNA target analysis server. Nucleic Acids Res. 39:W155–W159. doi: 10.1093/nar/gkr319
Dai, X., Lu, Q., Wang, J., Wang, L., Xiang, F., and Liu, Z. (2021). MiR160 and its target genes ARF10, ARF16 and ARF17 modulate hypocotyl elongation in a light, BRZ, or PAC-dependent manner in Arabidopsis: MiR160 promotes hypocotyl elongation. Plant Sci. 303:110686. doi: 10.1016/j.plantsci.2020.110686
Dai, X., Wang, Y., and Zhang, W. H. (2016). OsWRKY74, a WRKY transcription factor, modulates tolerance to phosphate starvation in rice. J. Exp. Bot. 67, 947–960. doi: 10.1093/jxb/erv515
de Jong, S., Boks, M. P., Fuller, T. F., Strengman, E., Janson, E., de Kovel, C. G., et al. (2012). A gene co-expression network in whole blood of schizophrenia patients is independent of antipsychotic-use and enriched for brain-expressed genes. PLoS One 7:e39498. doi: 10.1371/journal.pone.0039498
DiLeo, M. V., Strahan, G. D., den Bakker, M., and Hoekenga, O. A. (2011). Weighted correlation network analysis (WGCNA) applied to the tomato fruit metabolome. PLoS One 6:e26683. doi: 10.1371/journal.pone.0026683
Fan, F., Shang, X., Ding, G., Zhou, Z., and Tan, J. (2021). Integrated mRNA and miRNA expression analyses of Pinus massoniana roots and shoots in long-term response to phosphate deficiency. J. Plant Growth Regul. 1–18. doi: 10.1007/s00344-021-10486-0
Fan, X., Zhou, X., Chen, H., Tang, M., and Xie, X. (2021). Cross-talks between macro-and micronutrient uptake and signaling in plants. Front. Plant Sci. 12:663477. doi: 10.3389/fpls.2021.663477
Fujii, H., Chiou, T. J., Lin, S. I., Aung, K., and Zhu, J. K. (2005). A miRNA involved in phosphate-starvation response in Arabidopsis. Curr. Biol. 15, 2038–2043. doi: 10.1016/j.cub.2005.10.016
German, M. A., Pillay, M., Jeong, D. H., Hetawal, A., Luo, S., Janardhanan, P., et al. (2008). Global identification of microRNA-target RNA pairs by parallel analysis of RNA ends. Nat. Biotechnol. 26, 941–946. doi: 10.1038/nbt1417
Gupta, S., Kumari, M., Kumar, H., and Varadwaj, P. K. (2017). Genome-wide analysis of miRNAs and Tasi-RNAs in Zea mays in response to phosphate deficiency. Funct. Integr. Genom. 17, 335–351. doi: 10.1007/s10142-016-0538-4
Han, X., Yin, H., Song, X., Zhang, Y., Liu, M., Sang, J., et al. (2016). Integration of small RNA s, degradome and transcriptome sequencing in hyperaccumulator Sedum alfredii uncovers a complex regulatory network and provides insights into cadmium phytoremediation. Plant Biotechnol. J. 14, 1470–1483. doi: 10.1111/pbi.12512
He, C., Liu, H., Su, S., Lu, Y., Luo, B., Nie, Z., et al. (2015). Genome-wide identification of candidate phosphate starvation responsive genes and the development of intron length polymorphism markers in maize. Plant Breed. 134, 11–16. doi: 10.1111/pbr.12230
Hofferek, V., Mendrinna, A., Gaude, N., Krajinski, F., and Devers, E. A. (2014). MiR171h restricts root symbioses and shows like its target NSP2 a complex transcriptional regulation in Medicago truncatula. BMC Plant Biol. 14:199. doi: 10.1186/s12870-014-0199-1
Hossain, M. S., Hoang, N. T., Yan, Z., Tóth, K., Meyers, B. C., and Stacey, G. (2019). Characterization of the spatial and temporal expression of two soybean miRNAs identifies SCL6 as a novel regulator of soybean nodulation. Front. Plant Sci. 10:475. doi: 10.3389/fpls.2019.00475
Hsieh, L. C. (2010). Uncovering small RNA-mediated responses to phosphate deficiency in Arabidopsis by deep sequencing. Plant Physiol. 151, 2120–2132. doi: 10.1104/pp.109.147280
Jia, X., Wang, L., Zeng, H., and Yi, K. (2021). Insights of intracellular/intercellular phosphate transport and signaling in unicellular green algae and multicellular land plants. New Phytol. 232, 1566–1571. doi: 10.1111/nph.17716
Johnston, A., Poulton, P. R., Fixen, P. E., and Curtin, D. (2014). Chapter Five-Phosphorus : Its efficient use in agriculture. Adv. Agron. 123, 177–228. doi: 10.1016/B978-0-12-420225-2.00005-4
Kvam, V. M., Liu, P., and Si, Y. (2012). A comparison of statistical methods for detecting differentially expressed genes from RNA-seq data. Am. J. Bot. 99, 248–256. doi: 10.3732/ajb.1100340
Langfelder, P., and Horvath, S. (2008). WGCNA: An R package for weighted correlation network analysis. BMC Bioinform. 9:559. doi: 10.1186/1471-2105-9-559
Li, A., Hu, B., and Chu, C. (2021). Epigenetic regulation of nitrogen and phosphorus responses in plants. J. Plant Physiol. 258:153363. doi: 10.1016/j.jplph.2021.153363
Li, J., Wu, Z., and Yuan, J. (2019). Impact of agro-farming activities on microbial diversity of acidic red soils in a Camellia Oleifera Forest. Rev. Bras. Cienc. Solo 43:0190044. doi: 10.1590/18069657rbcs20190044
Li, M., and Yu, B. (2021). Recent advances in the regulation of plant miRNA biogenesis. RNA Biol. 18, 2087–2096. doi: 10.1080/15476286.2021.1899491
Li, M., Xia, Y., Gu, Y., Zhang, K., Lang, Q., Chen, L., et al. (2010). MicroRNAome of porcine pre-and postnatal development. PLos One 5:e11541. doi: 10.1371/journal.pone.0011541
Li, Z., Xu, H., Li, Y., Wan, X., Ma, Z., Cao, J., et al. (2018). Analysis of physiological and miRNA responses to Pi deficiency in alfalfa (Medicago sativa L.). Plant Mol. Biol. 96, 473–492. doi: 10.1007/s11103-018-0711-3
Lidbury, I. D., Scanlan, D. J., Murphy, A. R., Christie-Oleza, J. A., Aguilo-Ferretjans, M. M., Hitchcock, A., et al. (2022). A widely distributed phosphate-insensitive phosphatase presents a route for rapid organophosphorus remineralization in the biosphere. Proc. Natl. Acad. Sci. U.S.A. 119:e2118122119. doi: 10.1073/pnas.2118122119
Lin, W. Y., Lin, Y. Y., Chiang, S. F., Syu, C., Hsieh, L. C., and Chiou, T. J. (2018). Evolution of micro RNA 827 targeting in the plant kingdom. New Phytol. 217, 1712–1725.
Ling, Y.-H., Ren, C.-H., Guo, X.-F., Xu, L.-N., Huang, Y.-F., Luo, J.-C., et al. (2014). Identification and characterization of microRNAs in the ovaries of multiple and uniparous goats (Capra hircus) during follicular phase. BMC Genom. 15:339. doi: 10.1186/1471-2164-15-339
Liu, N., Shang, W., Li, C., Jia, L., Wang, X., Xing, G., et al. (2018). Evolution of the SPX gene family in plants and its role in the response mechanism to phosphorus stress. Open Biol. 8:170231. doi: 10.1098/rsob.170231
Liu, Q., Zhou, Z., Wei, Y., Shen, D., Feng, Z., and Hong, S. (2015). Genome-wide identification of differentially expressed genes associated with the high yielding of oleoresin in secondary xylem of Masson pine (Pinus massoniana Lamb) by transcriptomic analysis. PLos One 10:e0132624. doi: 10.1371/journal.pone.0132624
Liu, X., Yang, Y., Wang, R., Cui, R., Xu, H., Sun, C., et al. (2022). GmWRKY46, a WRKY transcription factor, negatively regulates phosphorus tolerance primarily through modifying root morphology in soybean. Plant Sci. 315:111148. doi: 10.1016/j.plantsci.2021.111148
Liu, Y., Mi, G., Chen, F., Zhang, J., and Zhang, F. (2004). Rhizosphere effect and root growth of two maize (Zea mays L.) genotypes with contrasting P efficiency at low P availability. Plant Sci. 167, 217–223.
Liu, Z., Wang, X., Chen, X., Shi, G., Bai, Q., and Xiao, K. (2018). TaMIR1139: A wheat miRNA responsive to Pi-starvation, acts a critical mediator in modulating plant tolerance to Pi deprivation. Plant Cell Rep. 37, 1293–1309. doi: 10.1007/s00299-018-2313-6
Livak, K. J., and Schmittgen, T. D. (2001). Analysis of relative gene expression data using real-time quantitative PCR and the 2–ΔΔCt method. Methods 25, 402–408.
Ma, X., Denyer, T., Javelle, M., Feller, A., and Timmermans, M. C. (2021). Genome-wide analysis of plant miRNA action clarifies levels of regulatory dynamics across developmental contexts. Genome Res. 31, 811–822. doi: 10.1101/gr.270918.120
Miao, Y., Laun, T., Zimmermann, P., and Zentgraf, U. (2004). Targets of the WRKY53 transcription factor and its role during leaf senescence in Arabidopsis. Plant Mol. Biol. 55, 853–867. doi: 10.1007/s11103-004-2142-6
Navarro, M., and Munné-Bosch, S. (2022). Reduced phosphate availability improves tomato quality through hormonal modulation in developing fruits. J. Plant Growth Regul. 41, 153–162. doi: 10.1007/s00344-020-10290-2
Nie, Z., Ren, Z., Wang, L., Su, S., Wei, X., Zhang, X., et al. (2016). Genome-wide identification of microRNAs responding to early stages of phosphate deficiency in maize. Physiol. Plant. 157, 161–174. doi: 10.1111/ppl.12409
Pegler, J. L., Nguyen, D. Q., Oultram, J. M. J., Grof, C. P. L., and Eamens, A. L. (2021). Molecular manipulation of the miR396 and miR399 expression modules alters the response of Arabidopsis thaliana to phosphate stress. Plants 10:2570. doi: 10.3390/plants10122570
Pegler, J. L., Oultram, J. M., Grof, C. P., and Eamens, A. L. (2020). Molecular manipulation of the miR399/PHO2 expression module alters the salt stress response of Arabidopsis thaliana. Plants 10:73. doi: 10.3390/plants10010073
Peng, K., Tian, Y., Sun, X., Song, C., Ren, Z., Bao, Y., et al. (2021). Tae-miR399-UBC24 module enhances freezing tolerance in winter wheat via a CBF signaling pathway. J. Agric. Food Chem. 69, 13398–13415. doi: 10.1021/acs.jafc.1c04316
Rubio, V., Linhares, F., Solano, R., Martín, A. C., Iglesias, J., Leyva, A., et al. (2001). A conserved MYB transcription factor involved in phosphate starvation signaling both in vascular plants and in unicellular algae. Genes Dev. 15, 2122–2133. doi: 10.1101/gad.204401
Sailaja, B., Voleti, S., Subrahmanyam, D., Sarla, N., Prasanth, V. V., Bhadana, V., et al. (2014). Prediction and expression analysis of miRNAs associated with heat stress in Oryza sativa. Rice Sci. 21, 3–12. doi: 10.1016/S1672-6308(13)60164-X
Shi, J., Zhao, B., Zheng, S., Zhang, X., Wang, X., Dong, W., et al. (2021). A phosphate starvation response-centered network regulates mycorrhizal symbiosis. Cell 184:5527–5540.e18. doi: 10.1016/j.cell.2021.09.030
Shi, T., Wu, G., Jin, Q., and Wang, X. (2020). Camellia oil authentication: A comparative analysis and recent analytical techniques developed for its assessment. A review. Trends Food Sci. Technol. 97, 88–99. doi: 10.1016/j.tifs.2020.01.005
Smith, F. W., Mudge, S. R., Rae, A. L., and Glassop, D. (2003). Phosphate transport in plants. Plant Soil 248, 71–83. doi: 10.1023/A:1022376332180
Sun, Q., Liu, Y., Tang, Y., Zhang, P., Tong, Y., He, G., et al. (2021). The composition and phosphate-solubilizing capability of phosphate-solubilizing bacteria in the rhizosphere of wild Camellia oleifera in mountain Lushan. Am. J. Agric. For. 9:141.
Sun, S., Gu, M., Cao, Y., Huang, X., Zhang, X., Ai, P., et al. (2012). A constitutive expressed phosphate transporter, OsPht1; 1, modulates phosphate uptake and translocation in phosphate-replete rice. Plant Physiol. 159, 1571–1581. doi: 10.1104/pp.112.196345
Sun, X., Lin, L., and Sui, N. (2019). Regulation mechanism of microRNA in plant response to abiotic stress and breeding. Mol. Biol. Rep. 46, 1447–1457. doi: 10.1007/s11033-018-4511-2
Tyagi, W., Nongbri, E. N., and Rai, M. (2021). “Chapter 11 – Harnessing tolerance to low phosphorus in rice: Recent progress and future perspectives,” in Molecular breeding for rice abiotic stress tolerance and nutritional quality, eds M. A. Hossain, L. Hassan, K. M. Ifterkharuddaula, A. Kumar, and R. Henry (Hoboken, NJ: John Wiley & Sons, Ltd.), 215–233. doi: 10.1002/9781119633174.ch11
Ünlü, E. S., Kaya, Ö, Eker, I., and Gürel, E. (2021). Sequencing, de novo assembly and annotation of Digitalis ferruginea subsp. schischkinii transcriptome. Mol. Biol. Rep. 48, 127–137. doi: 10.1007/s11033-020-05982-7
Vidal, E. A., Araus, V., Lu, C., Parry, G., Green, P. J., Coruzzi, G. M., et al. (2010). Nitrate-responsive miR393/AFB3 regulatory module controls root system architecture in Arabidopsis thaliana. Proc. Natl. Acad. Sci. U.S.A. 107, 4477–4482. doi: 10.1073/pnas.0909571107
Wang, F., Deng, M., Xu, J., Zhu, X., and Mao, C. (2018). Molecular mechanisms of phosphate transport and signaling in higher plants. Semin. Cell Dev. Biol. 74, 114–122.
Wang, L., Mai, Y. X., Zhang, Y. C., Luo, Q., and Yang, H. Q. (2010). MicroRNA171c-targeted SCL6-II, SCL6-III, and SCL6-IV genes regulate shoot branching in Arabidopsis. Mol. Plant 3, 794–806. doi: 10.1093/mp/ssq042
Wang, Q., Kang, Y. S., Alowaifeer, A., Shi, K., Fan, X., Wang, L., et al. (2018). Phosphate starvation response controls genes required to synthesize the phosphate analog arsenate. Environ. Microbiol. 20, 1782–1793. doi: 10.1111/1462-2920.14108
Wang, S., Liu, L., Mi, X., Zhao, S., An, Y., Xia, X., et al. (2021). Multi-omics analysis to visualize the dynamic roles of defense genes in the response of tea plants to gray blight. Plant J. 106, 862–875. doi: 10.1111/tpj.15203
Wang, X., Niu, Y., and Zheng, Y. (2021). Multiple functions of MYB transcription factors in abiotic stress responses. Int. J. Mol. Sci. 22:6125. doi: 10.3390/ijms22116125
Wang, Y., Wang, F., Lu, H., Liu, Y., and Mao, C. (2021). Phosphate uptake and transport in plants: An elaborate regulatory system. Plant Cell Physiol. 62, 564–572. doi: 10.1093/pcp/pcab011
Wang, Z., Kuo, H. F., and Chiou, T. J. (2021). Intracellular phosphate sensing and regulation of phosphate transport systems in plants. Plant Physiol. 187, 2043–2055. doi: 10.1093/plphys/kiab343
Wang, Z., Ruan, W., Shi, J., Zhang, L., Xiang, D., Yang, C., et al. (2014). Rice SPX1 and SPX2 inhibit phosphate starvation responses through interacting with PHR2 in a phosphate-dependent manner. Proc. Natl. Acad. Sci. U. S. A. 111, 14953–14958. doi: 10.1073/pnas.1404680111
Wu, F., Li, J., Chen, Y., Zhang, L., Zhang, Y., Wang, S., et al. (2019). Effects of phosphate solubilizing bacteria on the growth, photosynthesis, and nutrient uptake of Camellia oleifera abel. Forests 10:348. doi: 10.3390/f10040348
Xu, F., Liu, Q., Chen, L., Kuang, J., Walk, T., Wang, J., et al. (2013). Genome-wide identification of soybean microRNAs and their targets reveals their organ-specificity and responses to phosphate starvation. BMC Genom. 14:66. doi: 10.1186/1471-2164-14-66
Zhang, B., and Horvath, S. (2005). A general framework for weighted gene co-expression network analysis. Stat. Appl. Genet. Mol. 4, Article17. doi: 10.2202/1544-6115.1128
Zhang, J., Lin, Y., Wu, F., Zhang, Y., Cheng, L., Huang, M., et al. (2021). Profiling of microRNAs and their targets in roots and shoots reveals a potential miRNA-mediated interaction network in response to phosphate deficiency in the forestry tree Betula luminifera. Front. Genet. 12:38. doi: 10.3389/fgene.2021.552454
Zhang, J., Miao, H., Xie, B., Wang, J., Jia, C., Zhang, J., et al. (2020). Genomic and transcriptional analysis of banana ovate family proteins reveals their relationship with fruit development and ripening. Biochem. Genet. 58, 412–429. doi: 10.1007/s10528-020-09951-4
Zhou, C., Lin, P., Yao, X., Wang, K., Chang, J., and Han, X. (2013). Selection of reference genes for quantitative real-time PCR in six oil-tea camellia based on RNA-seq. Mol. Biol. 47, 836–851.
Zhou, J., Lu, M., Zhang, C., Qu, X., Liu, Y., Yang, J., et al. (2020). Isolation and functional characterisation of the PHT1 gene encoding a high-affinity phosphate transporter in Camellia oleifera. J. Hortic. Sci. Biotechnol. 95, 553–564. doi: 10.1080/14620316.2019.1703562
Keywords: Camellia oleifera, low phosphorus stress, integration analysis, miRNA, co-expression network
Citation: Chen J, Han X, Ye S, Liu L, Yang B, Cao Y, Zhuo R and Yao X (2022) Integration of small RNA, degradome, and transcriptome sequencing data illustrates the mechanism of low phosphorus adaptation in Camellia oleifera. Front. Plant Sci. 13:932926. doi: 10.3389/fpls.2022.932926
Received: 30 April 2022; Accepted: 11 July 2022;
Published: 01 August 2022.
Edited by:
Weicong Qi, Jiangsu Academy of Agricultural Sciences (JAAS), ChinaReviewed by:
Shuai Li, Qingdao Agricultural University, ChinaSonia Balyan, University of Delhi South Campus, India
Copyright © 2022 Chen, Han, Ye, Liu, Yang, Cao, Zhuo and Yao. This is an open-access article distributed under the terms of the Creative Commons Attribution License (CC BY). The use, distribution or reproduction in other forums is permitted, provided the original author(s) and the copyright owner(s) are credited and that the original publication in this journal is cited, in accordance with accepted academic practice. No use, distribution or reproduction is permitted which does not comply with these terms.
*Correspondence: Renying Zhuo, emh1b3J5QGdtYWlsLmNvbQ==; Xiaohua Yao, eWFveGgxNjhAMTYzLmNvbQ==