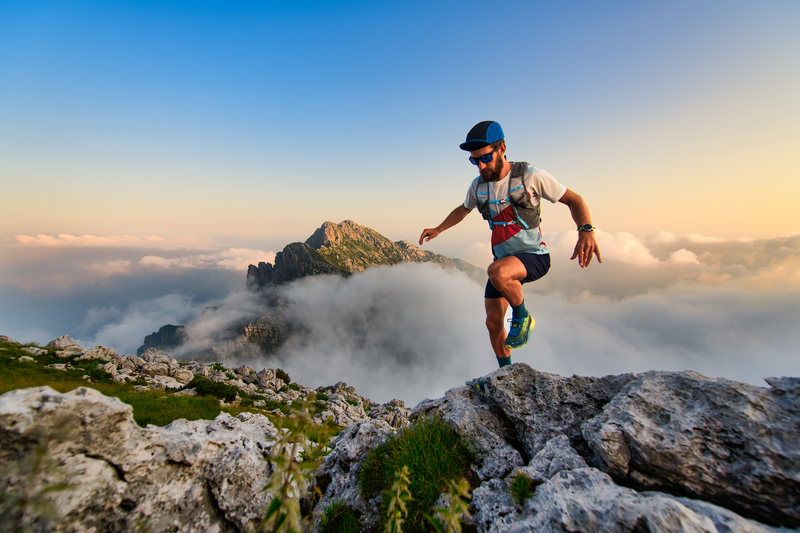
95% of researchers rate our articles as excellent or good
Learn more about the work of our research integrity team to safeguard the quality of each article we publish.
Find out more
ORIGINAL RESEARCH article
Front. Plant Sci. , 21 June 2022
Sec. Functional Plant Ecology
Volume 13 - 2022 | https://doi.org/10.3389/fpls.2022.920086
The Sanjiang Plain has the greatest concentration of freshwater marshes in China. Marshes in this area play a key role in adjusting the regional carbon cycle. As an important quality parameter of marsh ecosystems, vegetation aboveground biomass (AGB) is an important index for evaluating carbon stocks and carbon sequestration function. Due to a lack of in situ and long-term AGB records, the temporal and spatial changes in AGB and their contributing factors in the marsh of Sanjiang Plain remain unclear. Based on the measured AGB, normalized difference vegetation index (NDVI), and climate data, this study investigated the spatiotemporal changes in marsh AGB and the effects of climate variation on marsh AGB in the Sanjiang Plain from 2000 to 2020. Results showed that the marsh AGB density and annual maximum NDVI (NDVImax) had a strong correlation, and the AGB density could be accurately calculated from a power function equation between NDVImax and AGB density (AGB density = 643.57 × NDVI). According to the function equation, we found that the AGB density significantly increased at a rate of 2.47 g·C/m2/a during 2000–2020 in marshes of Sanjiang Plain, with the long-term average AGB density of about 282.05 g·C/m2. Spatially, the largest increasing trends of AGB were located in the north of the Sanjiang Plain, and decreasing trends were mainly found in the southeast of the study area. Regarding climate impacts, the increase in precipitation in winter could decrease the marsh AGB, and increased temperatures in July contributed to the increase in the marsh AGB in the Sanjiang Plain. This study demonstrated an effective approach for accurately estimating the marsh AGB in the Sanjiang Plain using ground-measured AGB and NDVI data. Moreover, our results highlight the importance of including monthly climate properties in modeling AGB in the marshes of the Sanjiang Plain.
Wetlands are one of the world's major ecosystems that play an important role in the global carbon cycle and ecological equilibrium (Hu et al., 2017; Liu et al., 2021). Marsh is a type of wetland ecosystem, which is crucial for regulating the regional climate and carbon cycle (Shen et al., 2020; Rietl et al., 2021; Wang et al., 2022a). As an important quality parameter of marsh ecosystems, vegetation aboveground biomass (AGB) is an important index for evaluating carbon stocks and carbon sequestration function in marshes (Shen et al., 2021a; Wang et al., 2021). Since climatic change remarkably influences marsh vegetation, understanding the impact of climatic change on marsh AGB is important for assessing the carbon storage of wetland ecosystems in the context of global climate change. Many studies have analyzed the spatiotemporal variations of vegetation AGB and their responses to climate change (Stegen et al., 2011; Shi et al., 2015; Castanho et al., 2020; Zhou et al., 2021). However, most of these studies focused on grassland and forest ecosystems, and only a few were conducted on marsh ecosystems (Flannigan et al., 2000; Shen et al., 2016, 2022a; Dai et al., 2021; Konings et al., 2021; Naik et al., 2021; Nandy et al., 2021; Qin et al., 2021; Wang et al., 2022b). Compared with other ecosystems, marsh ecosystems have unique environmental conditions, which may lead to different influences of climate change on AGB (Wang et al., 2021). In the context of climate change, clarifying the temporal and spatial variations of marsh AGB is very important for predicting the regional carbon cycle.
The Sanjiang Plain has the largest freshwater marsh in China (Wang et al., 2006). The marsh on the Sanjiang Plain plays a crucial role in regional biodiversity conservation and the carbon cycle (Sui et al., 2019). Some studies have analyzed the variations in marsh AGB and the effects of climatic change on marsh AGB in the Sanjiang Plain (Shi et al., 2015; Li et al., 2021). For example, Ni et al. (1996) studied the influences of hydrothermal conditions and the AGB of Deyeuxia angustifolia in the Sanjiang Plain marshes. Shi et al. (2015) analyzed the effects of different water levels on the AGB of vegetation in the freshwater marshes of the Sanjiang Plain and found that increasing water levels promoted the biomass of D. angustifolia. Li et al. (2021) analyzed the influences of temperature and precipitation on AGB and found that the increase of precipitation can increase the AGB in the Sanjiang Plain. However, these studies focused on analyzing changes in marsh AGB and their responses to climatic change at a species scale, and no research has been conducted over the entire Sanjiang Plain due to the limitations of field investigations. Climate change could induce different responses of different marsh vegetation in a region or the same marsh vegetation in different regions to climate changes (Shen et al., 2019a, 2021b). Thus, studying the variations in marsh AGB over the entire Sanjiang Plain and the influences of climate change on marsh AGB is necessary. Compared with measured sampling, remote sensing data have the advantage of comprehensive spatiotemporal coverage (Ozesmi and Bauer, 2002; Gerber et al., 2018; Yang et al., 2021; Shen et al., 2022a,b). Owing to the rapid development of remote sensing technology, many studies have used satellite remote sensing datasets combined with measured ground AGB datasets to estimate the vegetation AGB. Yang et al. (2018) established an effective model in the three-river headwaters region to estimate grassland AGB by combining the AGB field measurement and remote sensing data. Lumbierres et al. (2017) used the NDVI and measured AGB data to estimate the grassland AGB in three-river headwaters. Piao et al. (2004) suggested that a power function between the annual NDVImax and AGB density could be established to accurately estimate the AGB of grassland in China. Wang et al. (2021) have also constructed a power function to estimate AGB in the Tibetan Plateau marshes. Combined with ground-measured data, remote sensing is a useful tool for long-term monitoring of the AGB of ecosystems (Lumbierres et al., 2017). Therefore, it is necessary to analyze the change in AGB in the Sanjiang Plain by using a combination of remote sensing and measured data. In addition, Wang et al. (2021) reported that daytime and nighttime temperatures had an asymmetric impact on marsh AGB on the Tibetan Plateau. The positive impact of warming the minimum temperature at night was more significant. Compared with the Tibetan Plateau, the Sanjiang Plain has a lower altitude and more precipitation (Zhang et al., 2015). However, whether there is an asymmetric influence of warming daytime and nighttime temperatures on AGB in the Sanjiang Plain marshes remains unclear. Under asymmetric global day and night warming, exploring the effects of the maximum temperature in the daytime and the minimum temperature at night on the AGB in the Sanjiang Plain marshes is necessary.
In this study, an estimation model of marsh AGB was established by using measured biomass data and NDVI in the marshes of the Sanjiang Plain. Based on climate and NDVI datasets during 2000–2020, we investigated the spatiotemporal variation in marsh AGB and the influences of climate factors on marsh AGB. This study aimed to clarify the spatial and temporal changes in AGB and the impacts of climatic change on marsh AGB in the Sanjiang Plain. We put forward two hypotheses: (1) the AGB density of marsh vegetation can be estimated by the NDVImax in the Sanjiang Plain; (2) temperature is the main reason affecting the AGB in the marsh of the Sanjiang Plain. The results of this study can aid in protecting marsh vegetation and predicting the carbon cycle in the Sanjiang Plain marshes. As the marsh in the Sanjiang Plain is a typical temperate freshwater marsh, the findings of this study have important implications for the studies of freshwater marsh in temperate regions.
The Sanjiang Plain is located in the northeast of China, with a latitude of 43°49'N to 48°27'N and a longitude of 129°11'E to 135°05'E (Figure 1) (Luo et al., 2022). Sanjiang Plain has a low and flat terrain and is the largest marsh region in China (Mao et al., 2015). The Sanjiang Plain has a continental monsoonal climate, with more than half of the annual precipitation in July and August. The mean annual temperature is approximately 3.2°C, with January and July being the coldest and hottest months, respectively (Wu et al., 2021).
Figure 1. Distributions of the marsh, meteorological stations, and sample sites of aboveground biomass (ABG) at the Sanjiang Plain.
The climate data used in this study were the monthly minimum temperature (Tmin), maximum temperature (Tmax), mean temperature (Tmean), and precipitation data from 21 meteorological stations in the Sanjiang Plain during 2000–2020, obtained from the Chinese Meteorological Center (http://www.nmic.cn/). The marsh distribution data were the spatial distribution dataset of China's marsh wetlands in 2000 and 2015, which have been subjected to strict data quality control and verification (Mao et al., 2020). The spatial resolution of marsh distribution data was 30 m (Wang et al., 2015; Mao et al., 2019). The MODIS NDVI data used in this study were from the Goddard Space Flight Center's MOD13Q1 dataset from 2000 to 2020 (https://ladsweb.modaps.eosdis.nasa.gov/). The time resolution of MOD13Q1 NDVI was 16 days, and the spatial resolution was 250 m (Beck et al., 2006). The AGB data were field-observed marsh AGB data collected from 16 survey sites during 2013–2017 in the Sanjiang Plain (Shen et al., 2021a). The collection time of field biomass sampling sites was from July to September. Each sampling site was set up with three repeated quadrats of equal size (1 m × 1 m). To accurately reflect the AGB, the average value of three repeated quadrats was considered the sampling point's value based on the longitude and latitude of the sampling point (Wang et al., 2021). In this study, the marsh vegetation AGB (g) was converted to carbon (g·C) by using a conversion coefficient of 0.45 (Fang et al., 2007; Shen et al., 2021a; Wang et al., 2021).
According to the marsh distribution data in 2000 and 2015, we extracted the unchanged distribution of marshes in the Sanjiang Plain (marshes both in 2000 and 2015), to eliminate the interference of human activities on marsh wetlands (Shen et al., 2019b). In this study, we converted the 16-day NDVI into annual NDVImax data using the maximum value compositing method (Holben, 1986). The corresponding NDVImax of each sample site was obtained according to geographical position (Wang et al., 2021). We then approximated the AGB using the measured NDVImax and AGB density in the Sanjiang Plain marsh from 2000 to 2020. In this study, the regional averages of the variables are the averages of the corresponding pixels in the Sanjiang Plain marshes (Shen et al., 2016).
This study evaluated the equation based on the predicted root mean square error (RMSE) and relative error (Equations 1, 2) (Wang et al., 2021). The symbol n denotes the number of effective samples (n = 16 in this study).
In this study, we used the trend analysis method to calculate the trends of AGB and meteorological factors in the marshes of the Sanjiang Plain. The formula is included below (Shen et al., 2021b):
In Formula (3), AGBi is the value of AGB or the annual (or monthly) meteorological factor of year i, where, n represents the length of the study period, i.e., 21 years, i is the year serial number, and θslope is the slope of the meteorological factor or AGB change trend of each pixel. If θslope < 0, the change in AGB or meteorological factors in the growing season (or month) of the pixel is a decreasing trend; otherwise, it is an increasing trend.
In this study, we interpolated the meteorological data using the Kriging interpolation method, and the grid images of precipitation, Tmin, Tmax, and Tmean in the annual (or monthly) of marsh vegetation in the Sanjiang Plain with the same spatial resolution (250 m) as the AGB image were obtained (Shen et al., 2021b). The correlation between meteorological factors in the annual vegetation (or month) and AGB was calculated by using the correlation analysis method. The formula is as follows:
In Formula (4), Rxy represents the correlation coefficient, n is the length of the study period, n = 21 years in this study, xa is the annual (or monthly) average value of the meteorological factor in a certain year, is the multi-year average value of the annual (or monthly) average value of the meteorological factor in the past 21 years, ya is the AGB value of a year, and is the average value of the multi-year average AGB.
Based on the NDVImax and measured AGB dada, an optimal equation (power function, R2 = 0.86) was established for the Sanjiang Plain marsh (Figure 2A, Equation 5). We used the annual NDVImax dataset to calculate the marsh AGB in Sanjiang Plain from 2000 to 2020. Results showed that the average marsh AGB density was approximately 282.05 g·C/m2 over the entire Sanjiang Plain from 2000 to 2020. The area of the Sanjiang Plain marsh was about 6.0 × 108 m2, and thus the total marsh AGB of the whole Sanjiang Plain was about 0.17 Tg·C. Spatially, the long-term average marsh AGB density during 2000–2020 had a high value in the southeast and a low value in the northwest of the Sanjiang Plain (Figure 3A).
Figure 2. Equation between maximum normalized difference vegetation index (NDVImax) and observed AGB density (A), and comparison between estimated AGB density and observed AGB density (B) in the marsh of the Sanjiang Plain.
Figure 3. Distributions of long-term average AGB density (g·C/m2) (A) and change trends of AGB density (g·C/m2/a) (B) in the marsh of the Sanjiang Plain from 2000 to 2020.
To study the temporal variation in marsh AGB density, we analyzed the time series of AGB density. Marsh AGB density had a significant (p < 0.05) upward trend (2.47 g·C/m2/a) over the entire Sanjiang Plain during 2000–2020 (Figure 4). The annual variation trend of the AGB density was spatially heterogeneous in the Sanjiang Plain marsh. The most significant increase in AGB density was observed in the north of the Sanjiang Plain, whereas decreased AGB density was mainly documented in the southeast (Figure 3B).
To explore the relationship between meteorological variables and the AGB of marsh vegetation in the Sanjiang Plain, we calculated the correlations between meteorological variables and the AGB of marsh vegetation from 2000 to 2020. A weak positive correlation between the annual precipitation, Tmin, Tmax, and Tmean, and AGB (Table 1) was found in the study area. Spatially, the correlation between AGB and annual precipitation was positive in most regions of the study area (Figure 5A). The positive correlation with annual temperature was mainly distributed east of the Sanjiang Plain (Figures 5B–D). For the seasonal climate effects, the correlation between precipitation in January and the vegetation AGB in the Sanjiang Plain marshes was significantly (p < 0.05) negative. By contrast, the correlations between AGB and mean temperature, minimum temperature, and maximum temperature in January were weakly positive (Table 1), and the positive correlations were mainly distributed in the middle of the Sanjiang Plain (Figure 6). In addition, correlations between the AGB and temperatures (such as Tmean, Tmax, and Tmin) in July were significantly (p < 0.05) positive over the marshes of the Sanjiang Plain (Table 1), and the positive correlations were mainly concentrated in the north and middle of the Sanjiang Plain (Figure 7).
Table 1. Correlation coefficients between precipitation, mean temperature (Tmean), maximum temperature (Tmax), minimum temperature (Tmin), and aboveground biomass (AGB) of marsh vegetation in the Sanjiang Plain from 2000 to 2020.
Figure 5. Spatial patterns in relationships of marsh AGB with annual precipitation (A), Tmean (B), Tmax (C) and Tmin (D) on the Sanjiang Plain marshes from 2000 to 2020.
Figure 6. Spatial patterns in relationships of AGB with precipitation (A), Tmean (B), Tmax (C), and Tmin (D) in January on the Sanjiang Plain marshes from 2000 to 2020.
Figure 7. Spatial patterns in relationships of AGB with precipitation (A), mean temperature (Tmean) (B), maximum temperature (Tmax) (C), and minimum temperature (Tmin) (D) in July on the Sanjiang Plain marshes from 2000 to 2020.
In this study, we established an optimal equation (the value of R2 was the largest in the equation than in other equations) between the NDVImax and measured marsh AGB density (AGB density = 643.57 × NDVI). Our findings were similar to those reported by Piao et al. (2004) and Wang et al. (2021), who reported that the AGB density was related to NDVImax, and a power function could fit them well. However, the power function is not the same in these studies. It may be because Piao et al. (2004) and Wang et al. (2021) studied grasslands in China and the marsh in the Qinghai-Tibet Plateau, respectively, whereas our study focused on the Sanjiang Plain marshes. Different research regions and objects may explain the reasons for varied equations. For evaluating the accuracy of estimated AGB density based on the relation equation, the estimated and observed AGB of the marsh were compared (Figure 2B). The RMSE and relative error in this power function were 43.50 and 13.89%, respectively. These results confirm our hypothesis that the marsh AGB density in the Sanjiang Plain can be accurately estimated by the NDVImax in the Sanjiang Plain.
Based on the constructed power function equation, this study calculated that the long-term average marsh vegetation AGB density in the Sanjiang Plain from 2000 to 2020 was approximately 282.05 g·C/m2. Our result was similar to that documented by Zhang et al. (2020), where the average AGB density of the Dongsheng wetland was approximately 299.70 g·C/m2. We found a larger AGB density than that of Carex lasiocarpa in the Sanjiang Plain marsh (209.16 g·C/m2) documented by Song et al. (2003). This may be because Song et al. (2003) studied Carex lasiocarpa, which has a relatively small biomass, while this study studied all the marsh vegetation in the Sanjiang Plain. Spatially, the long-term average marsh AGB density during 2000–2020 was high in the southeast and low in the northwest of the Sanjiang Plain (Figure 3A), which may be related to the high (low) vegetation cover in the warm southeast (cold northwest) of the Sanjiang Plain (Shen et al., 2019a). The largest declining trend in marsh AGB was found in the east of the Sanjiang Plain (Figure 3B), which could be accounted for by the marsh degeneration in these regions due to the interference from human activities (Yan et al., 2016).
Annual meteorological factors (temperature and precipitation) and AGB in the marshes of the Sanjiang Plain had a weakly positive relationship, which was similar to the findings of Guo et al. (2008) who found that annual precipitation and annual temperature were not the main factors affecting marsh plant growth in the Sanjiang Plain. The marsh AGB was negatively correlated with winter precipitation and the correlation with precipitation in January was significant (p < 0.05) (Table 1), indicating that precipitation in winter, especially in January, could inhibit marsh vegetation growth in the Sanjiang Plain. The increase in precipitation during winter can lead to cooling and insufficient heat accumulation, resulting in a retarded plant growth (Rajput et al., 1987; Yun et al., 2018). The AGB of the marsh vegetation in the Sanjiang Plain had a significant positive relationship with temperature in July. This finding was consistent with Yan et al. (2021) who found that there was a positive correlation between temperature and vegetation biomass in the Sanjiang Plain but was different from the finding of Wang et al. (2022a), who found that the increase of temperature in summer would reduce the biomass of marsh vegetation in the Songnen Plain. This may be because the climate of the Songnen Plain was the semi-arid climate, while the climate of the Sanjiang Plain was a temperate monsoon climate. Therefore, summer precipitation is not the main factor affecting marsh AGB in the Sanjiang Plain (Shen et al., 2019a). To confirm the hypothesis that temperature is the main reason affecting the AGB in the marsh of the Sanjiang Plain, we further calculated the correlation coefficients between marsh AGB and precipitation in January and mean temperature in July in two different regions of the Sanjiang Plain (south and north of 47°N). The results showed that the positive correlation of AGB with July temperature and the negative correlation of AGB with January precipitation was larger north of 47°N than south of 47°N (Figure 8). It confirms that the marsh AGB was mainly affected by temperature in the Sanjiang Plain, and the positive effects of temperature and negative effects of precipitation on marsh AGB were more obvious in much colder regions of the Sanjiang Plain.
Figure 8. Correlation coefficients between marsh AGB and precipitation in January and mean temperature in July in two different regions of the Sanjiang Plain, south of 47°N ( ≤ 47°N) and north of 47°N (>47°N). ** and * mean significantly at the levels of p < 0.01 and p < 0.05, respectively.
Additionally, we found that warming at night-time and day-time had an asymmetric influence on marsh vegetation growth in the Sanjiang Plain, and warming at night had a greater effect on plant growth than warming during the day (Table 1). On the one hand, the temperature increase during the day promotes photosynthesis in plants and is conducive to the accumulation of organic matter (Xia et al., 2018), explaining that AGB has a positive correlation with Tmax in July. On the other hand, the increase in Tmin at night will bring a compensatory effect, which is beneficial to photosynthesis the next day (Hu and Kang, 2005; Xia et al., 2018). The over-compensation effect refers to the phenomenon that warming nighttime temperatures can promote photosynthesis and increase organic matter consumption during the next day to exceed the organic matter consumed due to enhanced respiration (Belsky, 1986; Peng et al., 2011; Chen et al., 2014). According to previous studies, when vegetation grows in sufficient nutrient and water environments, it has a high compensation effect and sometimes even shows an over-compensation effect (Belsky, 1986; Shen et al., 2021b). In July, owing to good hydrothermal conditions and sufficient nutrients (Wang et al., 2003), marsh vegetation in the Sanjian Plain has a strong photosynthetic capacity and it is easy to have an over-compensation effect (Wang et al., 2003). Therefore, the minimum temperature in July had a significant positive effect on the AGB in the marsh of the Sanjiang Plain.
To further explain the changes of marsh AGB, this study also analyzed the change trends in meteorological variables from 2000 to 2020 (Table 2). Annual precipitation increased significantly (1.14 mm/a) during the study period in the marsh of the Sanjiang Plain. Seasonally, the precipitation in January showed a significant decreasing trend (Table 2). Considering the significant negative correlation between the AGB and January precipitation (Table 1), the decrease in January precipitation (Table 2) may partly explain the increased AGB of marsh vegetation in the Sanjiang Plain. The annual temperatures (such as, Tmin, Tmax, and Tmean) showed no significant increasing trends, but the temperature in June increased significantly (Table 2). Considering the significant positive correlation between the marsh vegetation AGB and the temperature in July (Table 1), the increase in temperature in July may partly explain the increase in marsh vegetation AGB at the Sanjiang Plain. The marsh AGB in the Sanjiang Plain showed an overall increasing trend from 2000 to 2020. The area with the largest increasing trend was concentrated in the center of the Sanjiang Plain (Figure 9). In the middle region of the Sanjiang Plain, precipitation decreased the most in January (Figure 10), and the temperature increased the most in July (Figure 11). According to the spatial correlation between the AGB and climate factors (Figures 6, 7), the decrease in precipitation in January and the increase in temperature in July may account for the increasing AGB of marsh vegetation in the middle of the Sanjiang Plain.
Table 2. Temporal trends in precipitation (mm/a), Tmin (°C/a), Tmax (°C/a), and Tmean (°C/a) in marshes of the Sanjiang Plain from 2000 to 2020.
Figure 9. Change trends in annual precipitation (mm/a) (A), Tmean (°C/a) (B), Tmax (°C/a) (C), and Tmin (°C/a) (D) in marshes of the Sanjiang Plain from 2000 to 2020.
Figure 10. Change trends in precipitation (mm/a) (A), Tmean (°C/a) (B), Tmax (°C/a) (C), and Tmin (°C/a) (D) in January in marshes of the Sanjiang Plain from 2000 to 2020.
Figure 11. Change trends in precipitation (mm/a) (A), Tmean (°C/a) (B), Tmax (°C/a) (C), and Tmin (°C/a) (D) in July in marshes of the Sanjiang Plain from 2000 to 2020.
Although an effective method was provided for estimating the AGB density in the Sanjiang Plain marshes, this study may still have some limitations. First, the accuracy of NDVI data might be affected by factors, such as solar altitude angle, clouds, and atmosphere, which in turn could affect the results of this work. Second, the number of sampling points and meteorological stations was relatively limited, which may have caused some uncertainties in our results. Third, although this study extracted the unchanged marsh distribution in the Sanjiang Plain using two marsh distribution maps, it still could not rule out the impacts of anthropogenic activities on marshes in the study area. More marsh distribution data should be used to further analyze the marsh changes in the study area. Fourth, while numerous environmental factors affect AGB, we only analyzed the influences of temperature and precipitation on the marsh AGB of the Sanjiang Plain. Future research efforts should be directed toward understanding the influences of other factors on AGB in the marshes of the Sanjiang Plain.
The results showed that marsh vegetation AGB density and NDVImax had a good correlation, and the marsh AGB density in the Sanjiang Plain could be correctly calculated from a power function equation between NDVImax and marsh AGB density (AGB density = 643.57 × NDVI). According to the function equation, the total marsh AGB of the entire Sanjiang Plain was approximately 0.17 Tg·C. The AGB density demonstrated a significant increasing trend of 2.47 g·C/m2/a in the Sanjiang Plain from 2000 to 2020, with an average AGB density of 282.05 g·C/m2. Spatially, the largest increasing trends of AGB density were located in the north of the Sanjiang Plain, whereas the decreasing trends were found in the southeast. Regarding climate effects, changes in annual precipitation and temperature had no significant impacts on marsh AGB in the Sanjiang Plain. The increasing precipitation in January could significantly decrease the marsh AGB while the warming temperature in July increased the marsh AGB in the Sanjiang Plain. This work proposed an effective approach for accurately estimating the AGB density of marsh vegetation in the Sanjiang Plain by using NDVI data and highlighted the importance of including monthly climate properties in models of marsh AGB dynamics in the marsh of the Sanjiang Plain.
The original contributions presented in the study are included in the article/supplementary material, further inquiries can be directed to the corresponding author.
XS coordinated the project. YL carried out the data analysis and wrote the manuscript. YW, JZ, RM, XL, and MJ contributed to modify the manuscript. All authors contributed to the article and approved the submitted version.
This work was funded by the National Natural Science Foundation of China (41971065), Youth Innovation Promotion Association, CAS (2019235), the Natural Science Foundation of Jilin Province (20210101104JC), the Key Research Program of Frontier Sciences, CAS (ZDBS-LY-7019), and the Innovation Team Project of Northeast Institute of Geography and Agroecology, Chinese Academy of Sciences (2022CXTD02).
The authors declare that the research was conducted in the absence of any commercial or financial relationships that could be construed as a potential conflict of interest.
All claims expressed in this article are solely those of the authors and do not necessarily represent those of their affiliated organizations, or those of the publisher, the editors and the reviewers. Any product that may be evaluated in this article, or claim that may be made by its manufacturer, is not guaranteed or endorsed by the publisher.
Beck, P. S., Atzberger, C., Høgda, K. A., Johansen, B., and Skidmore, A. K. (2006). Improved monitoring of vegetation dynamics at very high latitudes: a new method using MODIS NDVI. Remote Sens. Environ. 100, 321–334. doi: 10.1016/j.rse.2005.10.021
Belsky, A. J. (1986). Does herbivory benefit plants? A review of the evidence. Am. Naturalist. 127, 870–892. doi: 10.1086/284531
Castanho, A. D., Coe, M. T., Brando, P., Macedo, M., Baccini, A., Walker, W., et al. (2020). Potential shifts in the aboveground biomass and physiognomy of a seasonally dry tropical forest in a changing climate. Environ. Res. Lett. 15, 034053. doi: 10.1088/1748-9326/ab7394
Chen, J., Tian, Y., Zhang, X., Zheng, C., Song, Z., Deng, A., et al. (2014). Nighttime warming will increase winter wheat yield through improving plant development and grain growth in North China. J. Plant Growth Regul. 33, 397–407. doi: 10.1007/s00344-013-9390-0
Dai, L., Fu, R., Guo, X., Ke, X., Du, Y., Zhang, F., et al. (2021). Effect of grazing management strategies on alpine grassland on the northeastern Qinghai-Tibet Plateau. Ecol. Eng. 173, 106418. doi: 10.1016/j.ecoleng.2021.106418
Fang, J., Guo, Z., Piao, S., and Chen, A. (2007). Terrestrial vegetation carbon sinks in China 1981–2000. Sci. China Ser. D Earth Sci. 50, 1341–1350. doi: 10.1007/s11430-007-0049-1
Flannigan, M. D., Stocks, B. J., and Wotton, B. M. (2000). Climate change and forest fires. Sci. Total Environ. 262, 221–229. doi: 10.1016/S0048-9697(00)00524-6
Gerber, F., de Jong, R., Schaepman, M. E., Schaepman-Strub, G., and Furrer, R. (2018). Predicting missing values in spatio-temporal remote sensing data. IEEE T. Geosci. Remote. 56, 2841–2853. doi: 10.1109/TGRS.2017.2785240
Guo, Z. X., Wang, Z. M., Song, K. S., Liu, D. W., and Zhang, B. (2008). Spatial features of productivity variability of Marsh in the Sanjiang plain. Wetland Sci. 6, 372–378.
Holben, B. N. (1986). Characteristics of maximum-value composite images from temporal AVHRR data. Int. J. Remote Sens. 7, 1417–1434. doi: 10.1080/01431168608948945
Hu, S., Niu, Z., Chen, Y., Li, L., and Zhang, H. (2017). Global wetlands: potential distribution, wetland loss, and status. Sci. Total Environ. 586, 319–327. doi: 10.1016/j.scitotenv.2017.02.001
Hu, T. T., and Kang, S. Z. (2005). The compensatory effect in drought resistance of plants and its application in water-saving agriculture. Acta Ecol. Sin. 25, 885–891. doi: 10.3321/j.issn:1000-0933.2005.04.034
Konings, A. G., Holtzman, N. M., Rao, K., Xu, L., and Saatchi, S. S. (2021). Interannual variations of vegetation optical depth are due to both water stress and biomass changes. Geophys. Res. Lett. 48, e2021GL095267. doi: 10.1029/2021GL095267
Li, H., Qu, Y., Zeng, X., Zhang, H., Cui, L., and Luo, C. (2021). Dynamic response of the vegetation carbon storage in the sanjiang plain to changes in land use/cover and climate. Herit Sci. 9, 1–12. doi: 10.1186/s40494-021-00605-1
Liu, M., Fu, B., Xie, S., He, H., Lan, F., Li, Y., et al. (2021). Comparison of multi-source satellite images for classifying marsh vegetation using DeepLabV3 Plus deep learning algorithm. Ecol. Indic. 125, 107562. doi: 10.1016/j.ecolind.2021.107562
Lumbierres, M., Méndez, P. F., Bustamante, J., Soriguer, R., and Santamaría, L. (2017). Modeling biomass production in seasonal wetlands using MODIS NDVI land surface phenology. Remote Sens Basel. 9, 392. doi: 10.3390/rs9040392
Luo, C., Fu, X., Zeng, X., Cao, H., Wang, J., Ni, H., et al. (2022). Responses of remnant wetlands in the Sanjiang Plain to farming-landscape patterns. Ecol. Indic. 135, 108542. doi: 10.1016/j.ecolind.2022.108542
Mao, D., He, X., Wang, Z., Tian, Y., Xiang, H., Yu, H., et al. (2019). Diverse policies leading to contrasting impacts on land cover and ecosystem services in Northeast China. J. Clean. Prod. 240, 117961. doi: 10.1016/j.jclepro.2019.117961
Mao, D., Wang, Z., Du, B., Li, L., Tian, Y., Jia, M., et al. (2020). National wetland mapping in China: a new product resulting from object based and hierarchical classification of Landsat 8 OLI images. ISPRS J. Photogramm. 164, 11–25. doi: 10.1016/j.isprsjprs.2020.03.020
Mao, D. H., Wang, Z. M., Li, L., Miao, Z. H., Ma, W. H., Song, C. C., et al. (2015). Soil organic carbon in the Sanjiang Plain of China: storage, distribution and controlling factors. Biogeosciences 12, 1635–1645. doi: 10.5194/bg-12-1635-2015
Naik, P., Dalponte, M., and Bruzzone, L. (2021). Prediction of forest aboveground biomass using multitemporal multispectral remote sensing data. Remote Sens Basel. 13, 1282. doi: 10.3390/rs13071282
Nandy, S., Srinet, R., and Padalia, H. (2021). Mapping forest height and aboveground biomass by integrating ICESat-2, Sentinel-1 and Sentinel-2 data using Random Forest algorithm in northwest Himalayan foothills of India. Geophys. Res. Lett. 48, e2021GL093799. doi: 10.1029/2021GL093799
Ni, H. W., Zang, S. Y., and andGao, Y. K. (1996). Study on seasonal dynamics of aboveground biomass and growth rate of Deyeuxia angustifolia population in marsh meadow on Sanjiang Plain. Plant Res. 16, 489–495.
Ozesmi, S. L., and Bauer, M. E. (2002). Satellite remote sensing of wetlands. Wetl Ecol. Manag. 10, 381–402. doi: 10.1023/A:1020908432489
Peng, S., Chen, A., Xu, L., Cao, C., and Piao, S. (2011). Recent change of vegetation growth trend in China. Environ. Res. Lett. 6, 044027. doi: 10.1088/1748-9326/6/4/044027
Piao, S. L., Fang, J. Y., He, J. S., and Xiao, Y. (2004). Spatial distribution of grassland biomass in China. Chin. J. Plant Ecol. 28, 491–498. doi: 10.17521/cjpe.2004.0067
Qin, Y., Xiao, X., Wigneron, J. P., Ciais, P., Brandt, M., Fan, L., et al. (2021). Carbon loss from forest degradation exceeds that from deforestation in the Brazilian Amazon. Nat. Clim. Change 11, 442–448. doi: 10.1038/s41558-021-01026-5
Rajput, R. P., Deshmukh, M. R., and Paradkar, V. K. (1987). Accumulated heat units and phenology relationships in wheat as influenced by planting dates under late sown conditions. J. Agron. Crop Sci. 159, 345–348. doi: 10.1111/j.1439-037X.1987.tb00112.x
Rietl, A. J., Megonigal, J. P., Herbert, E. R., and Kirwan, M. L. (2021). Vegetation type and decomposition priming mediate brackish marsh carbon accumulation under interacting facets of global change. Geophys. Res. Lett. 48, e2020GL092051. doi: 10.1029/2020GL092051
Shen, X., Jiang, M., Lu, X., Liu, X., Liu, B., Zhang, J., et al. (2021a). Aboveground biomass and its spatial distribution pattern of herbaceous marsh vegetation in China. Sci. China Earth Sci. 64, 1115–1125. doi: 10.1007/s11430-020-9778-7
Shen, X., Liu, B., Henderson, M., Wang, L., Jiang, M., and Lu, X. (2022a). Vegetation greening, extended growing seasons, and temperature feedbacks in warming temperate grasslands of China. J. Cim. 35, 1–51. doi: 10.1175/JCLI-D-21-0325.1
Shen, X., Liu, B., Jiang, M., and Lu, X. (2020). Marshland loss warms local land surface temperature in China. Geophys. Res. Lett. 47, e2020GL087648. doi: 10.1029/2020GL087648
Shen, X., Liu, B., Li, G., Yu, P., and Zhou, D. (2016). Impacts of grassland types and vegetation cover changes on surface air temperature in the regions of temperate grassland of China. Theor. Appl. Climatol. 126, 141–150. doi: 10.1007/s00704-015-1567-y
Shen, X., Liu, B., Xue, Z., Jiang, M., Lu, X., and Zhang, Q. (2019b). Spatiotemporal variation in vegetation spring phenology and its response to climate change in freshwater marshes of Northeast China. Sci. Total Environ. 666, 1169–1177. doi: 10.1016/j.scitotenv.2019.02.265
Shen, X., Liu, Y., Liu, B., Zhang, J., Wang, L., Lu, X., et al. (2022b). Effect of shrub encroachment on land surface temperature in semi-arid areas of temperate regions of the Northern Hemisphere. Agr. Forest Meteorol. 320, 108943. doi: 10.1016/j.agrformet.2022.108943
Shen, X., Xue, Z., Jiang, M., and Lu, X. (2019a). Spatiotemporal change of vegetation coverage and its relationship with climate change in freshwater marshes of Northeast China. Wetlands 39, 429–439. doi: 10.1007/s13157-018-1072-z
Shen, X. J., Liu, B. H., Jiang, M., Wang, Y. J., Wang, L., Zhang, J. Q., et al. (2021b). Spatiotemporal change of marsh vegetation and its response to climate change in China from 2000 to 2019. J. Geophys. Res. Biogeosci. 126, e2020JG006154. doi: 10.1029/2020JG006154
Shi, F., Song, C., Zhang, X., Mao, R., Guo, Y., and Gao, F. (2015). Plant zonation patterns reflected by the differences in plant growth, biomass partitioning and root traits along a water level gradient among four common vascular plants in freshwater marshes of the Sanjiang Plain, Northeast China. Ecol. Eng. 81, 158–164. doi: 10.1016/j.ecoleng.2015.04.054
Song, C. C., Wang, Y. Y., Wang, Y. S., Yan, B. X., Wang, D. X., Zhao, Z. C., et al. (2003). Carbon dynamics of wetland in the Sanjiang Plain. Chin. Geogr. Sci. 13, 228–231. doi: 10.1007/s11769-003-0021-8
Stegen, J. C., Swenson, N. G., Enquist, B. J., White, E. P., Phillips, O. L., Jørgensen, P. M., et al. (2011). Variation in above-ground forest biomass across broad climatic gradients. Global Ecol. Biogeogr. 20, 744–754. doi: 10.1111/j.1466-8238.2010.00645.x
Sui, X., Zhang, R., Frey, B., Yang, L., Li, M. H., and Ni, H. (2019). Land use change effects on diversity of soil bacterial, Acidobacterial and fungal communities in wetlands of the Sanjiang Plain, northeastern China. Sci. Rep. 9, 1–14. doi: 10.1038/s41598-019-55063-4
Wang, M., Lu, N., An, N., and Fu, B. (2022b). A trait-based approach for understanding changes in carbon sequestration in semi-arid grassland during succession. Ecosystems 25, 155–171. doi: 10.1007/s10021-021-00646-4
Wang, S. Y., Yang, Y. X., and Yang, B. (2003). Study on temperature of typical types of wetland soils and its influencing factors in the Sanjiang Plain. Geogr. Res. 22, 389–396. doi: 10.11821/yj2003030016
Wang, Y., Shen, X., Jiang, M., Tong, S., and Lu, X. (2021). Spatiotemporal change of aboveground biomass and its response to climate change in marshes of the Tibetan Plateau. Int J. Appl Earth Obs. 102, 102385. doi: 10.1016/j.jag.2021.102385
Wang, Y., Shen, X., Jiang, M., Tong, S., and Lu, X. (2022a). Daytime and nighttime temperatures exert different effects on vegetation net primary productivity of marshes in the western Songnen Plain. Ecol. Indic. 137, 108789. doi: 10.1016/j.ecolind.2022.108789
Wang, Z., Mao, D., Li, L., Jia, M., Dong, Z., Miao, Z., et al. (2015). Quantifying changes in multiple ecosystem services during 1992–2012 in the Sanjiang Plain of China. Sci. Total Environ. 514, 119–130. doi: 10.1016/j.scitotenv.2015.01.007
Wang, Z. M., Zhang, B., Zhang, S. Q., Li, X. Y., Liu, D. W., Song, K. S., et al. (2006). Changes of land use and of ecosystem service values in Sanjiang Plain, Northeast China. Environ. Monit. Assess. 112, 69–91. doi: 10.1007/s10661-006-0312-5
Wu, Y., Xu, N., Wang, H., Li, J., Zhong, H., Dong, H., et al. (2021). Variations in the diversity of the soil microbial community and structure under various categories of degraded wetland in Sanjiang Plain, northeastern China. Land Degrad. Dev. 32, 2143–2156. doi: 10.1002/ldr.3872
Xia, H., Li, A., Feng, G., Li, Y., Qin, Y., Lei, G., et al. (2018). The effects of asymmetric diurnal warming on vegetation growth of the Tibetan Plateau over the past three decades. Sust. Basel. 10, 1103. doi: 10.3390/su10041103
Yan, F., Zhang, S., Liu, X., Chen, D., Chen, J., Bu, K., et al. (2016). The effects of spatiotemporal changes in land degradation on ecosystem services values in Sanjiang Plain, China. Remote Sens Basel. 8, 917. doi: 10.3390/rs8110917
Yan, M., Xue, M., Zhang, L., Tian, X., Chen, B., and Dong, Y. (2021). A decade's change in vegetation productivity and its response to climate change over Northeast China. Plants 10, 821. doi: 10.3390/plants10050821
Yang, S., Feng, Q., Liang, T., Liu, B., Zhang, W., and Xie, H. (2018). Modeling grassland above-ground biomass based on artificial neural network and remote sensing in the Three-River Headwaters Region. Remote Sens. Environ. 204, 448–455. doi: 10.1016/j.rse.2017.10.011
Yang, X., Wu, J., Chen, X., Ciais, P., Maignan, F., Yuan, W., et al. (2021). A comprehensive framework for seasonal controls of leaf abscission and productivity in evergreen broadleaved tropical and subtropical forests. Innovation 2, 100154. doi: 10.1016/j.xinn.2021.100154
Yun, J., Jeong, S. J., Ho, C. H., Park, C. E., Park, H., and Kim, J. (2018). Influence of winter precipitation on spring phenology in boreal forests. Global Change Biol. 24, 5176–5187. doi: 10.1111/gcb.14414
Zhang, D. J., Qi, Q., and Tong, S. Z. (2020). Growth of carex tussocks as a response of flooding depth and tussock patterning and size in temperate sedge wetland. Northeast China Russ. J. Ecol. 51, 144–150. doi: 10.1134/S1067413620020137
Zhang, Z., Xing, W., Wang, G., Tong, S., Lv, X., and Sun, J. (2015). The peatlands developing history in the Sanjiang Plain, NE China, and its response to East Asian monsoon variation. Sci. Rep. 5, 1–10. doi: 10.1038/srep11316
Keywords: marsh wetland, biomass, NDVI, climatic change, Sanjiang Plain
Citation: Liu Y, Shen X, Wang Y, Zhang J, Ma R, Lu X and Jiang M (2022) Spatiotemporal Variation in Aboveground Biomass and Its Response to Climate Change in the Marsh of Sanjiang Plain. Front. Plant Sci. 13:920086. doi: 10.3389/fpls.2022.920086
Received: 14 April 2022; Accepted: 23 May 2022;
Published: 21 June 2022.
Edited by:
Xinsheng Chen, Anhui University, ChinaReviewed by:
Changliang Shao, Chinese Academy of Agricultural Sciences (CAAS), ChinaCopyright © 2022 Liu, Shen, Wang, Zhang, Ma, Lu and Jiang. This is an open-access article distributed under the terms of the Creative Commons Attribution License (CC BY). The use, distribution or reproduction in other forums is permitted, provided the original author(s) and the copyright owner(s) are credited and that the original publication in this journal is cited, in accordance with accepted academic practice. No use, distribution or reproduction is permitted which does not comply with these terms.
*Correspondence: Xiangjin Shen, c2hlbnhpYW5namluQGlnYS5hYy5jbg==
Disclaimer: All claims expressed in this article are solely those of the authors and do not necessarily represent those of their affiliated organizations, or those of the publisher, the editors and the reviewers. Any product that may be evaluated in this article or claim that may be made by its manufacturer is not guaranteed or endorsed by the publisher.
Research integrity at Frontiers
Learn more about the work of our research integrity team to safeguard the quality of each article we publish.