- 1Department of Plants, Soils, and Climate, College of Agriculture and Applied Sciences, Utah State University, Logan, UT, United States
- 2Bioinformatics Facility, Center for Integrated BioSystems, Utah State University, Logan, UT, United States
- 3Department of Computer Science, College of Science, Utah State University, Logan, UT, United States
Triticum aestivum (wheat), a major staple food grain, is affected by various biotic stresses. Among these, fungal diseases cause about 15–20% of yield loss, worldwide. In this study, we performed a comparative analysis of protein-protein interactions between two Puccinia graminis races (Pgt 21-0 and Pgt Ug99) that cause stem (black) rust in wheat. The available molecular techniques to study the host-pathogen interaction mechanisms are expensive and labor-intensive. We implemented two computational approaches (interolog and domain-based) for the prediction of PPIs and performed various functional analysis to determine the significant differences between the two pathogen races. The analysis revealed that T. aestivum-Pgt 21-0 and T. aestivum-Pgt Ug99 interactomes consisted of ∼90M and ∼56M putative PPIs, respectively. In the predicted PPIs, we identified 115 Pgt 21-0 and 34 Pgt Ug99 potential effectors that were highly involved in pathogen virulence and development. Functional enrichment analysis of the host proteins revealed significant GO terms and KEGG pathways such as O-methyltransferase activity (GO:0008171), regulation of signal transduction (GO:0009966), lignin metabolic process (GO:0009808), plastid envelope (GO:0009526), plant-pathogen interaction pathway (ko04626), and MAPK pathway (ko04016) that are actively involved in plant defense and immune signaling against the biotic stresses. Subcellular localization analysis anticipated the host plastid as a primary target for pathogen attack. The highly connected host hubs in the protein interaction network belonged to protein kinase domain including Ser/Thr protein kinase, MAPK, and cyclin-dependent kinase. We also identified 5,577 transcription factors in the interactions, associated with plant defense during biotic stress conditions. Additionally, novel host targets that are resistant to stem rust disease were also identified. The present study elucidates the functional differences between Pgt 21-0 and Pgt Ug99, thus providing the researchers with strain-specific information for further experimental validation of the interactions, and the development of durable, disease-resistant crop lines.
Introduction
Triticum aestivum L. (family Poaceae) is one of the highly cultivated staple food grains, and ranks third in terms of global production, owing to about 35% of the world’s food grain produce (Obembe et al., 2021). It contributes significantly to the daily nutrient intake of the human population, thus providing plant-derived proteins, carbohydrates, calories, vitamins, and a wide range of other nutrients (Shewry and Hey, 2015; Miransari and Smith, 2019). The gradual increase in the world’s human population leads to increased threat to global food security, which further demands to improve the crop yield substantially to meet the food supply of the world in the future (El Sabagh et al., 2021). Apart from the climate change, the global wheat production is also affected by various unpredictable biotic and abiotic stresses, which further leads to reduced genetic diversity of the crop (Afzal et al., 2015). Annually, the diseases caused by plant-pathogenic fungi lead to yield losses varying from 15 to 20%. Among these pathogenic fungi, the obligately biotrophic rust fungi emerge as a major threat to wheat production, leading to an economic loss of $4.3–5.0 billion dollars (Figueroa et al., 2018).
Stem (black) rust, caused by Puccinia graminis f. sp. tritici (Pgt), is considered as one of the highly destructive diseases of wheat. The occurrences of the disease have also been found in crops such as barley, rye, and other cereals (Dean et al., 2012). The disease can cause enormous yield losses ranging from 50 to 70% or more, depending on the environmental conditions (Saari and Prescott, 1985). Stem rust is also responsible for contraction of grain size, reduced photosynthetic area, diversion of photosynthetic assimilates, and water loss (Willocquet et al., 2021). Pgt consists of a wide range of strains, the most significant being the African strain “Ug99” (race TTKSK), which later evolved into variants of its own (Olivera et al., 2012; Li et al., 2019). Another Pgt isolate, “21-0,” was found in Australia, which has been used for the comparative study of stem rust in wheat. The draft genome of Pgt 21-0 was built using reference-based and de novo assembly (Upadhyaya et al., 2015). The infection by Pgt occurs in a series of steps, typically initiating by the germination of urediniospores on the surface of plant stem, followed by the formation of appressorium, mitosis of nuclei, and differentiation of haustorial mother cells into haustoria, which acquires nutrients from the plant cells (Leonard and Szabo, 2005).
The tremendous losses caused by the fungal pathogens have spurred the researchers to study the in-depth infection mechanism of the pathogen. Various studies have progressed the detection and genetic mapping of genes, and QTLs that confer resistance to Pgt in wheat (Duplessis et al., 2011), but a frequent resistance breakdown has been observed, owing to mutations in the Pgt isolates (Stokstad, 2007). The fungicides are an effective way against the fungal pathogens, but these pathogens develop resistance against the fungicides/chemicals, and also the fungicides have a negative impact on human health and environment (Van de Wouw et al., 2014). The protein-protein interactions (PPIs) in plant cells perform various functions, involving immune responses against biotic or abiotic stresses. The pathogens secrete effector proteins into the plant cell, sabotage the intercellular mechanisms of the host cell, and cause infection (Garbutt et al., 2014). Thus, the understanding of pathogen infection and the subsequent plant cell defense response is highly crucial. Computational prediction of PPIs reveals relationship among the proteins on a genome-wide scale. Various computational methods exist for the prediction of host-pathogen interactions (HPIs) such as protein sequence homology-based interolog approach, domain-based approach, gene co-expression, phylogenetic profiles, and others (Matthews et al., 2001; Ng et al., 2003; Sun et al., 2007; Piya et al., 2014; Kataria et al., 2022). In the present study, we delineated the PPIs between T. aestivum and Puccinia species proteins by employing two most widely used computational approaches, i.e., interolog (homology-based) and domain-based approach. Different molecular strategies for PPI detection are available, but those are expensive, time-consuming, and labor-intensive (Chen et al., 2008). Our research is mainly focused on elucidating genome-wide scale PPIs to unravel the complex intermolecular networks of T. aestivum-Puccinia interactome.
Materials and Methods
Data Source
The whole proteomes of T. aestivum, Pgt isolate 21-0, and Pgt isolate Ug99 were obtained from Ensembl Plants,1 National Center for Biotechnology Information (NCBI),2 and Ensembl Fungi,3 respectively. All the proteomes were analyzed with CD-HIT (Fu et al., 2012) at 100% to cluster the identical proteins. The total number of proteins are described in Table 1. In the research analysis, the proteins with prefixes “Traes,” “KAA,” and “GMQ” refer to T. aestivum, Pgt 21-0, and Pgt Ug99 proteins, respectively.
Interactome Prediction Between Triticum aestivum and Puccinia Species
The HPIs between T. aestivum and Puccinia species were predicted using two most widely implemented computational approaches: interolog-based, and domain-based. Interolog method is based on sequence homology that determines the conserved interactions between protein pairs of two species (Nourani et al., 2015). The interolog-based approach employs seven protein-protein interaction (PPI) databases, viz., BioGRID (Chatr-Aryamontri et al., 2017), DIP (Salwinski et al., 2004), HPIDB (Kumar and Nanduri, 2010), IntAct (Kerrien et al., 2012), MINT (Licata et al., 2012), PHI-base (Urban et al., 2020), and STRING (Szklarczyk et al., 2019). The interaction data from these databases was downloaded and implemented locally using SQL. The proteomes of host and pathogen species are aligned against these PPI databases using BLAST v2.7.1, followed by filtering of the results using random BLAST parameter combinations of sequence identity (30, 40, 50, and 60%), sequence coverage (40, 50, 60, and 80%), and e-value (1e-10, 1e-50, 1e-05, 1e-04, 1e-20, 1e-30, and 1e-25). In the past, there are no substantial reports for selecting an appropriate combination of BLAST parameters to predict PPIs https://academic.oup.com/bib/article/22/3/bbz162/5842243. A study on human and Escherichia coli HPIs determined the homologs using 30% sequence identity, 80% coverage, and e-value ≤1e-10 (Bose et al., 2017). In Arabidopsis-Pseudomonas system, the researchers identified homologs with 80% coverage, 1e-04 e-value, and 50% identity (Sahu et al., 2014). In our study, using different BLAST parameters (identity, coverage, and e-value), 112 combinations were generated, and an optimal combination was selected based on the maximum number of effectors.
On the other hand, in the domain-based approach, three domain-domain interaction (DDI) databases were implemented locally: 3did (Mosca et al., 2014), DOMINE (Raghavachari et al., 2008), and IDDI (Kim et al., 2012). In this method, the PPIs are predicted on the basis of Pfam domain composition. The proteins of host and pathogen were analyzed against Pfam database using “hmmscan” program in HMMER v3.3.1, which identified the significant domains. To filter the results of hmmscan, an e-value of 1e-23 and coverage 0.2 was used for host proteins, while those of pathogen proteins were filtered with e-value and coverage of 1e-17 and 0.45, respectively. The identified Pfam domains were then further used for the prediction of PPIs using local SQL queries. The details of number of sequences, and interaction pairs from each database are available in Supplementary Material 1, Sheet 1.
Effector and Secretory Proteins Prediction
Effector proteins, secreted by the fungi, interact with host proteins and manipulate the immune responses of host cell (Sonah et al., 2016). The secretory proteins contain a secretion signal peptide, less than 300 amino acids, that employs various cell wall degrading enzymes and phytotoxins to modulate the crucial host cell defense mechanisms (Kim et al., 2016). To identify the effector proteins, we analyzed the proteomes of Pgt 21-0 and Pgt Ug99 in EffectorP 2.04 (Sperschneider et al., 2018), while the secretory proteins were identified using SignalP-5.05 (Almagro Armenteros et al., 2019).
Functional Enrichment Analysis of the Proteins
The classification of the proteins into different functional categories such as molecular function, biological process, and cellular component was carried out by obtaining the functional annotation of the proteins. Gene Ontology (GO) and Kyoto Encyclopedia of Genes and Genomes (KEGG) analyses were conducted using the clusterProfiler (Yu et al., 2012) package in R. GO databases for T. aestivum and Puccinia species was created locally using makeOrgPackage function of the R package “AnnotationForge.” GO enrichment was then performed by implying Benjamini and Hochberg test correction method (Benjamini et al., 1995), followed by filtering the enriched terms on adjusted p-value cutoff of ≤0.05. Similarly, KEGG enrichment was also conducted at a p-value cutoff of 0.05.
Subcellular Localization
According to the studies, a high correlation is observed between the protein function and its subcellular localization, which provide more insights into the protein function (Chi, 2010). The pathogens secrete effector proteins into the host cell, which then translocate to various cellular compartments, and suppress the immune system of the host (Sperschneider et al., 2017). Thus, the prediction of subcellular localization of the host and pathogen proteins is an essential component of the plant-pathogen interaction studies. The subcellular localization of T. aestivum proteins was performed using standalone version of Support Vector Machine (SVM)-based tool, Plant-mSubP (Sahu et al., 2021). While for the subcellular localization of Puccinia proteins, we employed a deep learning-based tool, DeepLoc 1.0 (Almagro Armenteros et al., 2017).
Comparison Between Host-Pathogen Interactions of Pgt 21-0 and Pgt Ug99
Different races of P. graminis cause stem rust infection in wheat. We were interested in comparing the PPIs between two major strains (Pgt 21-0 and Pgt Ug99) to uncover the differences between the two fungal species. With regard to this, we identified the orthologs between both the fungal species using OrthoFinder, which implements phylogenetic-based prediction of the orthologs (Emms and Kelly, 2019). The interactions from the orthologs were referred to as common subnetwork. Further, we also focused on the strain-specific functional analysis of the Puccinia species. For this, we analyzed the “unique proteins,” i.e., the Puccinia species proteins that were not the orthologs of each other. This provided us more insights into the functionality of an individual strain.
Network Visualization and Analysis
The protein-protein interaction network is an extensively employed tool to study the functioning of cellular machinery by highlighting the crucial protein complexes and the relationship between the proteins, based on various network parameters such as node degree, centrality, etc. (Agapito et al., 2013). We analyzed the protein networks using the most widely used tool, Cytoscape v3.8.2 (Shannon et al., 1971). Various in-built layout algorithms and styles were used to analyze and enhance the visualization of the network.
Results and Discussion
To predict the protein-protein interactions, the proteomes of T. aestivum and Puccinia species were randomly paired, followed by the estimation of the interaction probability of an individual pair using interolog, and domain-based approaches. The interactome was predicted using the BLAST parameter combination of 30% sequence identity, 40% sequence coverage, and e-value of 1e-04. Using both the computational approaches, the predicted interactome for T. aestivum-Pgt 21-0 consisted of 90,493,282 interactions, whereby 84,125 host proteins interact with 9,022 pathogen proteins, of which 115 proteins were effectors (Table 2). While the T. aestivum-Pgt Ug99 interactome accounted for 56,755,414 interactions, involving 84,069 host and 5,863 pathogen proteins, consisting of 34 effectors (Table 3). The randomly employed (112) BLAST parameter combinations, and the resulting interactions from each combination for Pgt 21-0 and Pgt Ug99 have been described in Supplementary Material 1, Sheets 2, 3, respectively. For clarification, the term “effectors” has been used to represent the pathogen proteins that serve both as effector and secretory proteins.
Puccinia Orthologs Interactome
The ortholog analysis resulted in 1,958 proteins that are orthologs between Pgt 21-0 and Pgt Ug99. These orthologs were found to interact with 83,340 host proteins, involved in 21,901,125 interactions (referred to as “common subnetwork” throughout the analysis). For the subsequent functional analysis, the interactions from ortholog analysis were taken into consideration.
Highly Connected Protein Hubs
The host-pathogen protein-protein interaction network represents the functional clustering of the interacting proteins, which allows in-depth understanding of a specific protein with respect to the proteins in its surrounding (Wachi et al., 2005; Jonsson and Bates, 2006). The identification of the protein function helps gaining the knowledge of the disease infection mechanism by providing information about various biological processes and molecular mechanisms (Kuzmanov and Emili, 2013). In our study, the proteins hubs were determined from common subnetwork using the metric “node degree.” The average degree of host and pathogen proteins was found to be 263 and 11,185, respectively (Supplementary Material 2, Sheets 1, 2). The pathogen proteins have higher degree in comparison to host proteins, which is in-line with the host-pathogen protein ratio obtained by the researchers in the past (Li et al., 2012; Kurubanjerdjit et al., 2013). The top 20 protein hubs for each host and pathogen have been discussed below.
Triticum aestivum Protein Hubs
The protein network analysis revealed that majority of the host proteins belonged to protein kinase domain family, of which serine/threonine (Ser/Thr) protein kinase was found to form highly interconnected hubs (TraesCS4D02G250600.1.cds1, TraesCS7A02G437400.1, TraesCS5B02G254600.1.cds1, TraesCS 5A02G255500.1.cds1, TraesCS5D02G263800.1.cds1, TraesCS 2D02G120200.1, TraesCS3A02G247700.1.cds1, TraesCS4B02G2 60700.1.cds1, TraesCS3B02G271700.1.cds1, TraesCS4B02G1231 00.1, and TraesCS4A02G192300.1) (Figure 1). The physiological importance of Ser/Thr kinases play a crucial role in the regulation of various environmental stress responses, particularly in signaling pathway (País et al., 2009). SnRK Ser/Thr kinase is divided into 3 subgroups: SnRK1, SnRK2, and SnRK3 (Mao et al., 2010). In wheat, PKABA1 (a member of SnRK2) has been reported to be induced by ABA. The levels of ABA increase during stress conditions, thus showing its role in plant defense (Johnson et al., 2002). Another most interconnected protein (TraesCS6D02G339600.1), interacting with 1,497 pathogen proteins, belonged to heat shock protein 70 (hsp70) family. The members of hsp70 are thought to play a crucial role in different cellular processes during biotic and abiotic stress conditions (Usman et al., 2017). These proteins are also involved in R protein stability, and regulation of immune signaling pathways (Van Ooijen et al., 2010; Park and Seo, 2015). Five host proteins (TraesCS5A02G295800.1, TraesCS7A02G029700.1, TraesCS4A02G336800.2, TraesCS5B02G536500.1, and TraesCS5D02G534000.2) were identified as mitogen-activated protein kinases (MAPKs), which are known to be critical in response to pathogenic infection including the generation of hypersensitive response (HR), defense hormone responses, and ROS signaling (He et al., 2020). Another major hub was formed by the proteins (TraesCS5A02G521700.1, TraesCS4B02G353600.1, and TraesCS4D02G347600.1) that function as cyclin-dependent kinases (CDKs). A study shows the regulation of Arabidopsis resistance against Alternaria brassicicola by CDK8 that regulates the intermediates of the secondary metabolites, hydroxycinnamic acid amides (HCAAs), that play a role in fungal resistance. Also, an increased resistance against Botrytis cinerea was observed in the cdk8 mutant (Bessire et al., 2007; Zhu et al., 2014).
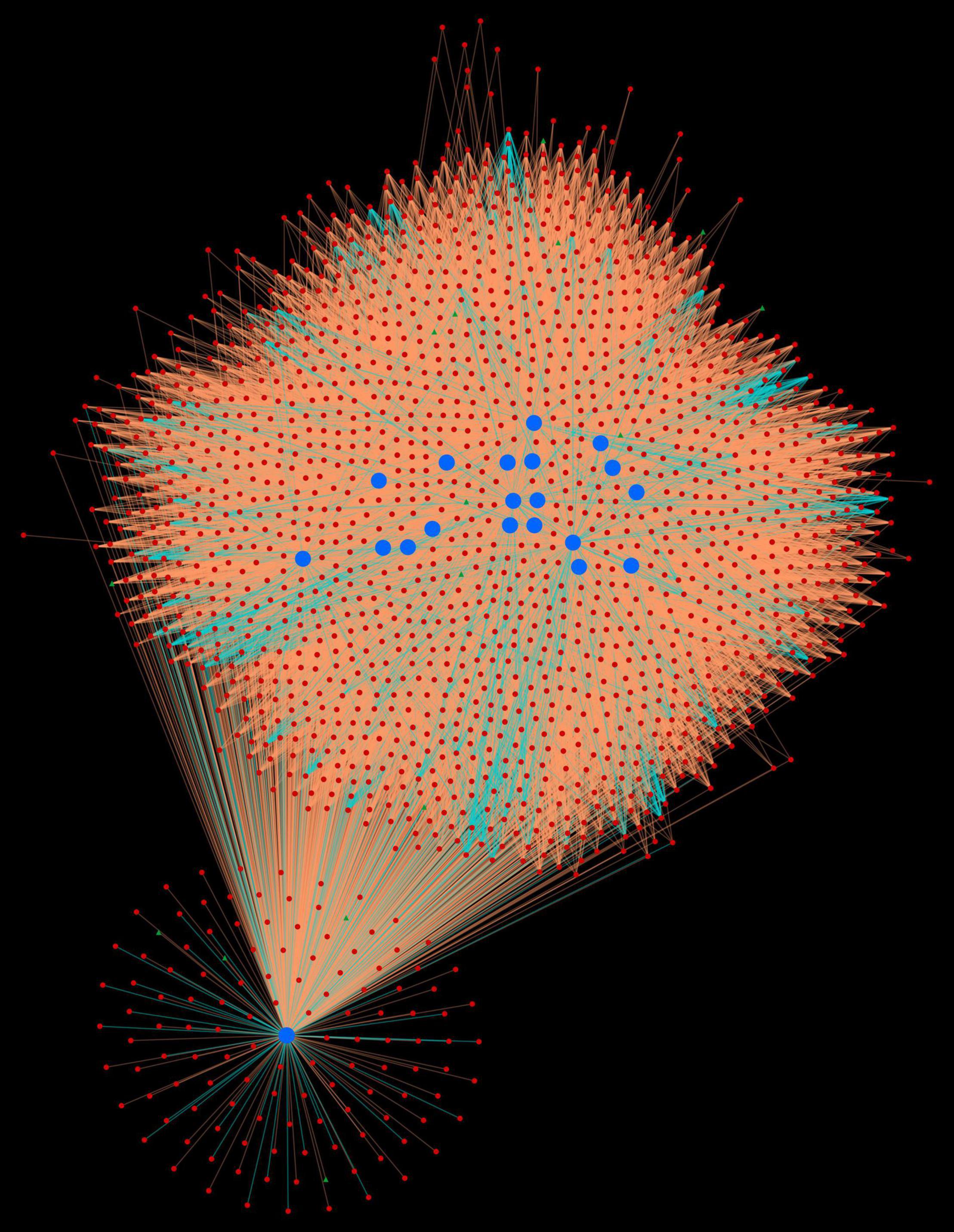
Figure 1. Protein-protein interaction network for top 20 host protein hubs. Blue nodes represent host proteins, red nodes are pathogen proteins, and green nodes are effector proteins. Orange edges depict the interactions from interolog-based approach, while cyan edges belong to domain-based approach.
Puccinia Protein Hubs
The common subnetwork analysis indicated that the largest hub was formed by aurora kinase that involves the Puccinia species protein GMQ_15838T0/KAA1117900.1, interacting with 57,744 host proteins. Various studies reveal the role of aurora kinase in mitotic processes such as chromosome segregation and cytokinesis, thus promoting the growth of fungal pathogens (Tückmantel et al., 2011; Bavetsias and Linardopoulos, 2015). Heat shock protein 70 superfamily forms another major hub involving seven pathogen proteins GMQ_09878T0/KAA1086976.1, GMQ_23673T0/KAA1112990.1, GMQ_10843T0/KAA107691 6.1, GMQ_14817T0/KAA1118905.1, GMQ_14422T0/KAA108 6735.1, GMQ_13441T0/KAA1119794.1, and GMQ_05646 T0/KAA1072403.1. Heat shock proteins are conserved molecular chaperones that play characteristic role in activating essential signal transducers in pathogenic fungi (Tiwari et al., 2015). In Fusarium pseudograminearum, 14 FpHsp70 genes were highly expressed at the time of crown rot infection in wheat. While the knockout of a Hsp70 homolog gene (FpLhs1) in ER lumenal resulted in reduction of fungal growth and virulence, implying the role of HSPs in pathogenicity (Chen et al., 2019). The pathogen proteins (GMQ_03421T0/KAA1111489.1, GMQ_12489T0/KAA1117418.1, GMQ_08991T0/KAA108750 2.1, GMQ_13869T0/KAA1085281.1, and GMQ_15253T0/KAA1 066088.1) were found to function as calcium/calmodulin-dependent kinases (CaMKs). Based on hidden Markov model, the fungal CaMKs are classified into different families (CAMK1, CAMKL, RAD53, and CAKM-Unique), and subfamilies (Kin4, Kin1, GIN4, PASK, AMPK, CHK1, and MARK) (Goldberg et al., 2013; Jiao et al., 2017). FgKin1 and FgKin4 in wheat fungal pathogen, Fusarium graminearum, are reported to be responsible for growth and pathogenesis (Wang et al., 2011; Luo et al., 2014). Similarly, the pathogen hubs formed by two Ser/Thr protein kinases: glycogen synthase kinase (GMQ_16722T0/KAA1117762.1), and AGC kinase (GMQ_11492T0/KAA1086570.1) are critical for pathogenicity and development in fungi (Qin et al., 2015; Fabri et al., 2019). The cluster of proteins (GMQ_05648T2/KAA1078421.1, GMQ_24430T0/KAA1114232.1, and GMQ_09263T0/KAA11 11598.1) served as cyclin-dependent kinases (CDKs). Researchers in the past established that the rice blast fungus, Magnaporthe oryzae, requires CDK subunit Cks1 for infection-associated development (Yue et al., 2017). Two proteins, GMQ_11353T0/KAA1076537.1 and GMQ_19044T0/KAA1114913.1, were associated with RNA-binding domain/RNA recognition motif. In Ustilago maydis, the causal agent of smut disease in corn, RNA-binding proteins were found to be involved in the fungal growth and development during the infection process (Becht et al., 2005).
The protein hubs analysis indicated that the pathogen proteins invade and subvert the host immune machinery, while the host activates various signaling cascades and hormones in response to the pathogen attack. Additionally, the hubs significantly revealed the crucial protein domain families that are involved in the disease infection and defense mechanisms in the pathogen and host, respectively, thus suggesting the cross-talks between the host and pathogen.
Gene Ontology Analysis: Unifying the Biology of Host and Pathogen Proteins
Gene Ontology enrichment analysis is an effective approach of deciphering the underlying biological process, molecular function and cellular component of the proteins of an organism (Tomczak et al., 2018). GO enrichment of the host and pathogen proteins was carried out using enrichment score [-log10(P-value)]. The enrichment analysis revealed that 83,340 host proteins in the common subnetwork are involved in 3,570 GO terms, categorized into biological process (2,167), cellular component (408), and molecular function (995) (Figure 2). The highly enriched GO terms in different categories involve gametophyte development (GO:0048229), regulation of response to stimulus (GO:0048583), plastid envelope (GO:0009526), chloroplast envelope (GO:0009941), O-methyltransferase activity (GO:0008171), xyloglucan:xyloglucosyl transferase activity (GO:0016762), and other significant GO terms (Supplementary Material 2, Sheet 3). Various studies have reported the direct or indirect involvement of the above-mentioned significant biological processes/cellular components/molecular functions in plant defense mechanisms. Ubiquitin-conjugating enzymes (E1, E2, and E3) are associated with ubiquitination, which regulates various plant immune signals. In Arabidopsis, UBC22 (E2 subfamily) showed its involvement in female gametophyte development, indicating its role in plant defense (Devoto et al., 2003; Wang et al., 2016). The enzyme hydroperoxide lyase (HPL) in plastid envelope is known to catalyze C6-aldehyde that play a role in plant defense. The attack of pathogenic fungi, B. cinerea, on Arabidopsis showed an upregulation of AtHPL expression, thus enhancing C6-aldehyde levels, which further inhibited the pathogen growth (Howe and Schilmiller, 2002; Kishimoto et al., 2008; Breuers et al., 2011). Caffeic acid 3-O-methyltransferases (COMT) are implicated in biosynthesis of lignin, which provides biotic/abiotic stress resistance to the plants (Bhuiyan et al., 2009; Kataria and Kaundal, 2021). In wheat, the COMT gene (TaCOMT-3D) showed significantly high expression level on infection with Rhizoctonia cerealis. TaCOMT-3D was localized in chromosome 3D (Wang et al., 2018). In our analysis, we identified six wheat proteins (TraesCS3D02G392500.1, TraesCS3D02G540200.1, TraesCS3D02G047700.1, 394TraesCS3D02G047800.1, TraesCS3D02G138700.1, and TraesCS3D02G292000.1) that belong to chromosome 3D and are associated with O-methyltransferase activity. These six host proteins were found interacting with 307 pathogen proteins, accounting to 1,611 interactions (Figure 3). This provides concrete evidence of the involvement of host proteins in plant defense against fungal attack. Furthermore, lignification also restricts the diffusion of nutrients from host to pathogen, thus suggesting the inability of haustoria to maintain the biotrophic relationship with the host, resulting in reduced pathogen infection.

Figure 2. Over-representation of top 15 GO terms from each category (molecular function, cellular component, and biological process) for the host proteins, based on enrichment score.
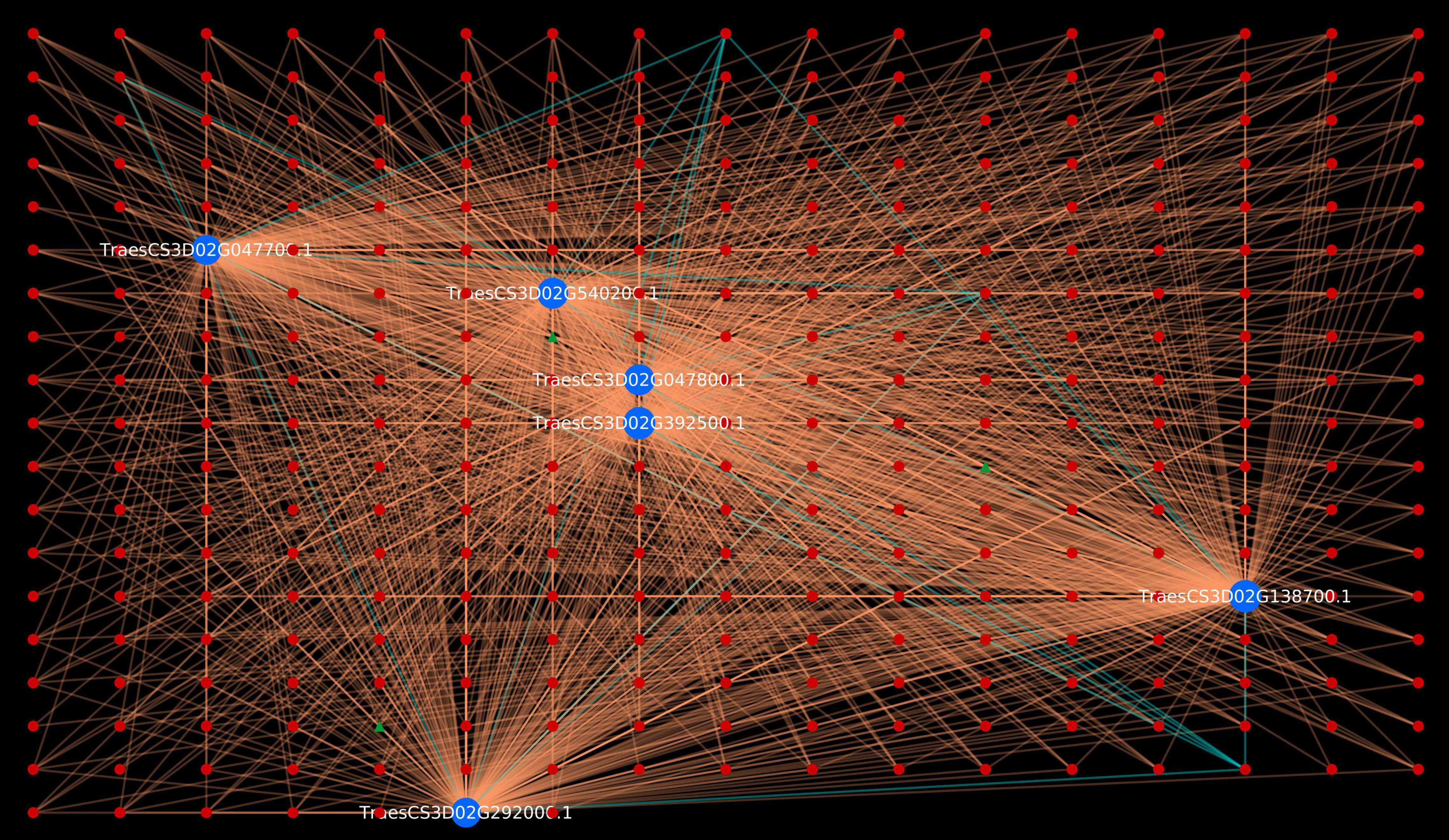
Figure 3. Visualization of six Triticum aestivum proteins belonging to chromosome 3D in GO:0008171 (O-methyltransferase activity). Blue nodes represent host proteins, red nodes are pathogen proteins, and green nodes are effector proteins. Orange edges depict the interactions from interolog-based approach, while cyan edges belong to domain-based approach.
On the other hand, 1958 pathogen proteins associated with the host proteins were involved in 1,362 GO terms. These include significant GO terms such as proteolysis (GO:0006508), protein peptidyl-prolyl isomerization (GO:0000413), endoplasmic reticulum (GO:0005783), GTPase activity (GO:0003924), and hydrolase activity (GO:0004553). These processes are known to be involved in pathogen virulence and development (Pogány et al., 2015; Pinter et al., 2019). The detailed GO enrichment of Puccinia species has been provided in Supplementary Material 2, Sheet 4.
Plant Defense and Immune Signaling Pathways During Biotic Stress
The in-depth knowledge of biological pathways of the proteins helps in better understanding of a PPI network. KEGG enrichment analysis of the proteins involved in PPIs was conducted. A total of 399 highly enriched KEGG pathways were obtained for the host proteins in the common subnetwork (Supplementary Material 2, Sheet 5). The over-represented pathways include NF-kappa B signaling pathway (ko04064), flavonoid biosynthesis (ko00941), biosynthesis of secondary metabolites (ko01110), plant-pathogen interaction (ko04626), MAPK signaling pathway (ko04016), and a few more significantly enriched pathways related to plant defense mechanism. The top 20 KEGG pathways have been represented in Figure 4. The nuclear factor kappa B (NF-κB) transcription factor helps in the regulation of cellular immune responses against diverse environmental stresses (Zhao et al., 2018). The interaction of protein NIM1 with transcription factor NF-κB has been reported to induce systemic acquired resistance (SAR) and gene-for-gene resistance against the disease in Arabidopsis (Ryals et al., 1997). The secondary metabolites are known to play a major role in plant immune responses to external stimuli. In our analysis, around 4,640 host proteins were found to be involved in biosynthesis of secondary metabolites pathway. Previous reports show the activation of secondary metabolites on recognition of the pathogen-secreted effectors by resistance proteins in the host (Ahuja et al., 2012; Piasecka et al., 2015). Flavonoids, a class of secondary metabolites, have been reported to account for plant development and defense responses against pathogens in various crops such as cotton (Mathesius, 2018). Among the aforementioned pathways, the most significant is mitogen-activated protein kinase (MAPK) signaling pathway, which is known to play a critical role in plant immune signaling during various stresses (Zhang and Klessig, 2001). A study revealed the induction of rice MAPKs, OsMKK3, and OsMPK7, during the infection process of Xanthomonas oryzae that causes leaf blight disease in rice. The overexpression of OsMKK3 and OsMPK7 genes during pathogenesis also suggested the probable disease resistance mechanism. Also, the silencing of overexpressed OsMPK7 lead to disease susceptible plants (Jalmi and Sinha, 2016). Also, transcription factors are known to be activated by MAPKs by the process of phosphorylation, thus regulating the immune response against the pathogens by integrating defense signals from various MAPKs (Nadal-Ribelles et al., 2019).
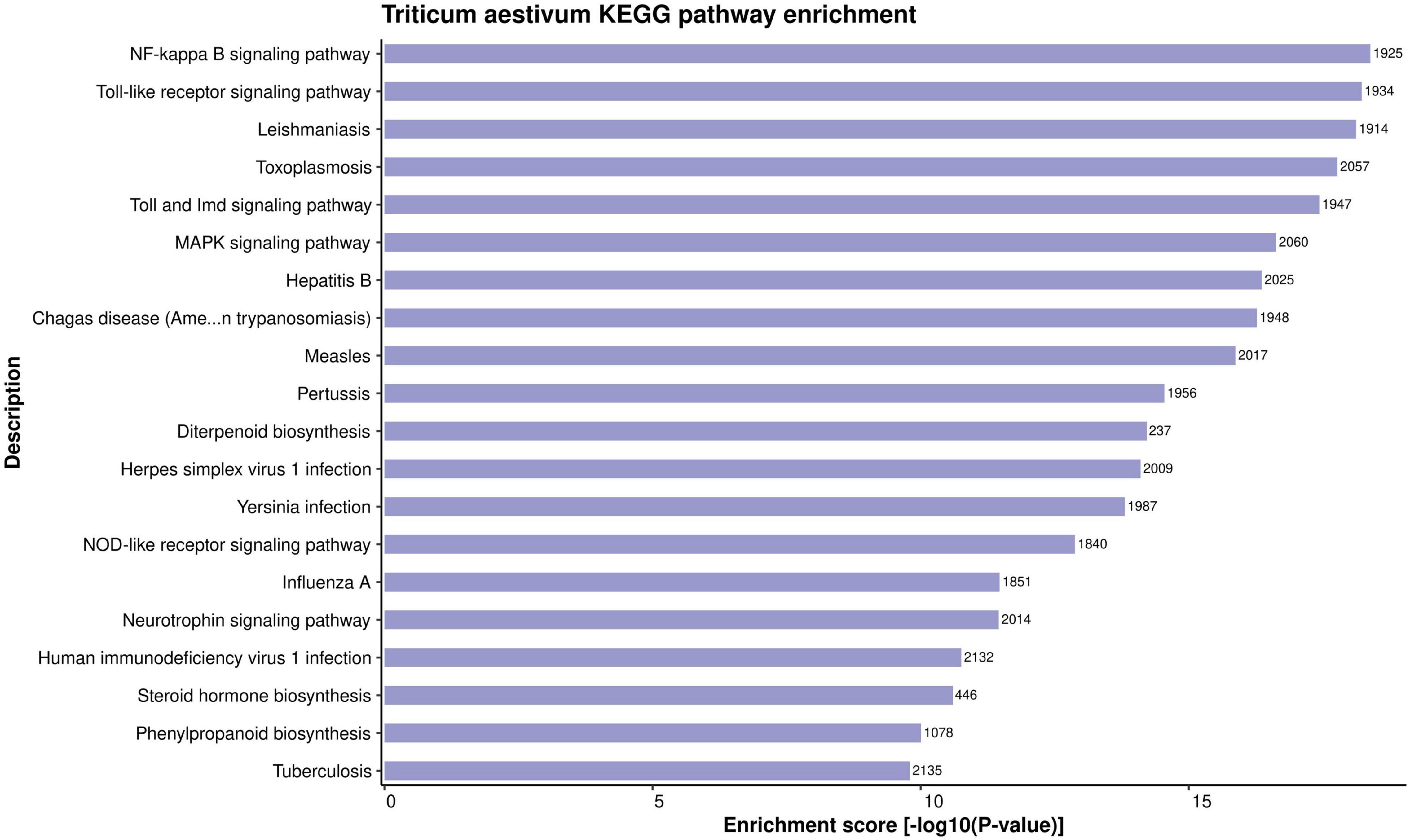
Figure 4. Representation of top 20 enriched KEGG pathways for the host proteins involved in HPIs, based on enrichment score.
We also performed the KEGG pathway enrichment of the pathogen proteins, which revealed important pathways such as MAPK signaling pathway (ko04016), biosynthesis of secondary metabolites (ko01110), and others, which have a direct or indirect relationship with pathogenicity (Supplementary Material 2, Sheet 6). The host proteins are also involved in these pathways, which suggests the potential interaction of host and pathogen proteins during plant defense response.
Thus, the functional enrichment analysis suggested significant molecular processes and biological pathways in which the host and pathogen proteins are involved. A comprehensive analysis of the enriched processes/pathways can further enhance the study of host-pathogen interaction mechanism, and other related biological processes occurring within the host cell.
Plastid: A Primary Target for Pathogen Attack in the Host
The proteins are translocated to various subcellular compartments, where they perform specific biological functions, thus revealing the physiology of the cell. Some proteins are also distributed to multiple cellular locations, depending on the sorting signal (Briesemeister et al., 2010). The subcellular localization is also known to be statistically correlated with the protein function, and its gene expression levels (Garapati et al., 2020). A significant number of novel proteins have been identified using sequencing technologies, but their subcellular location remains unknown.
We predicted the sequence-based subcellular localization of the T. aestivum and Puccinia proteins to have a better understanding of the occurrence of PPIs in a particular subcellular compartment. The subcellular localization analysis classified the T. aestivum proteins into 14 categories: plastid (29.56%), nucleus (23.96%), cell membrane (12.94%), endoplasm (7.07%), cytoplasm (6.91%), extracellular (6.23%), mitochondria (5.43%), golgi apparatus (2.74%), multi-target (2.66%), vacuole (1.75%), peroxisome (0.38%), cell wall (0.17%), endoplasmic reticulum (0.16%), and lysosome (0.03%) (Figure 5A). 2,218 proteins were found to be multi-target (moonlighting proteins), performing specific functions in various cellular organelles. 24,633 and 19,966 host proteins were localized in plastid and nucleus, respectively. Researchers in the past have reported the presence of host proteins in plastid, which plays an essential role in intracellular signaling pathways (de Dios Barajas-López et al., 2013; Caplan et al., 2015). Another study demonstrated the localization of rice OsVQ domain proteins in plastid and nucleus using rice protoplast system. OsVQ proteins are considered to be the co-regulators during immune response against biotic stress (Kim et al., 2013).
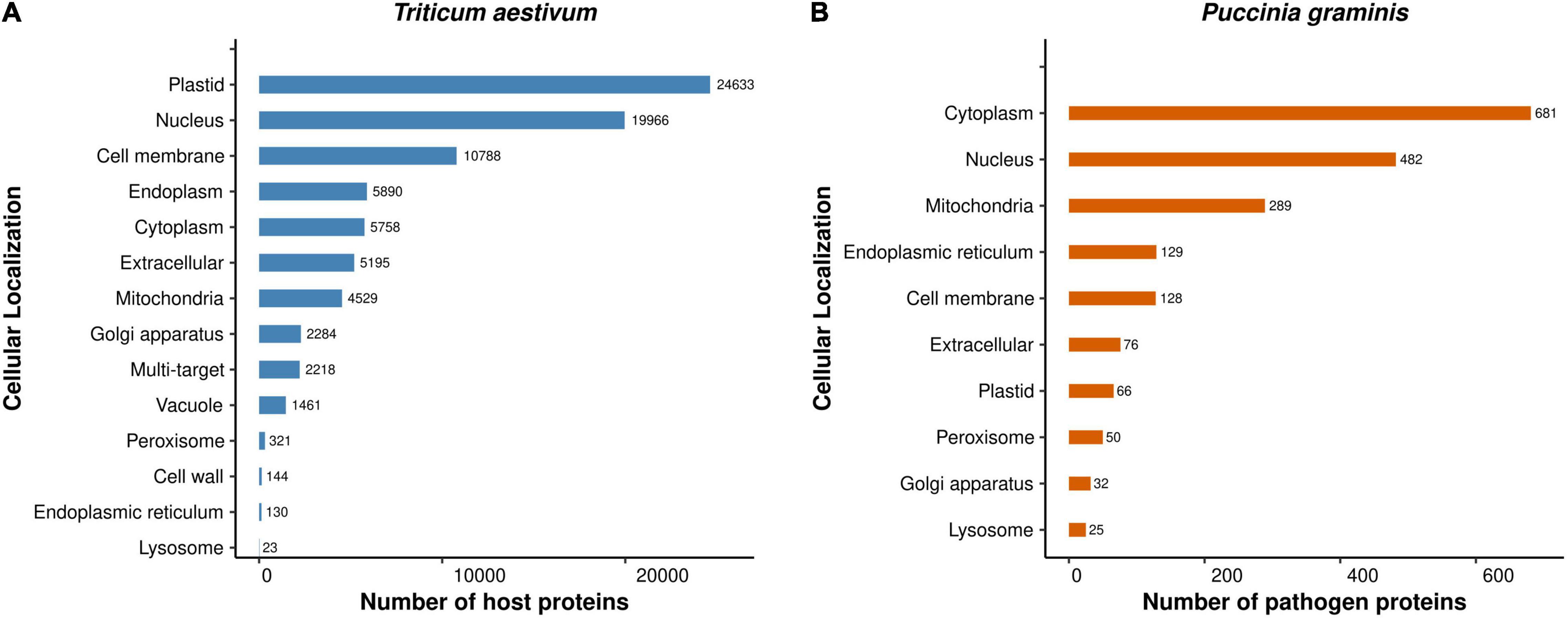
Figure 5. Subcellular Localization of the (A) host proteins, and (B) pathogen proteins involved in the common subnetwork.
On the other hand, the Puccinia species proteins were localized in cytoplasm (34.78%), nucleus (24.62%), mitochondria (14.76%), endoplasmic reticulum (6.54%), cell membrane (6.54%), extracellular (3.88%), plastid (3.37%), peroxisome (2.55%), golgi apparatus (1.63%), and lysosome (1.28%) (Figure 5B). In B. cinerea, two ubiquitin-like (UBL) activating enzymes, BcAtg3 (E2) and BcAtg7 (E1), were determined to be localized in cytoplasm (Ren et al., 2018), which is coherent to our localization prediction. The detailed information of the predicted subcellular localization of the host and pathogen proteins is available in Supplementary Material 2, Sheets 7, 8, respectively. Furthermore, we were also interested in predicting the location of interactions between the host and pathogen proteins. The analysis indicated that the host proteins are mostly targeted by the pathogen proteins in the plastid, whereby 24,237 host proteins interact with 674 pathogen proteins, accounting to 2,630,496 interactions (Supplementary Material 2, Sheet 9).
Pathogenicity of Effectors During Stem Rust Infection
The rust fungal pathogens secrete effectors (using specialized structures known as haustoria) which subvert the host cell immune machinery, followed by enhancing their pathogenicity in the host cell (Ramachandran et al., 2017). Therefore, understanding the behavior of the effector proteins is a crucial step in the HPI analysis. From the common subnetwork, we identified 18 effectors interacting with 43,054 host proteins, resulting in 156,529 interactions (Supplementary Material 3, Sheet 1). Among the 43,054 host proteins involved in interactions with effectors, 3367 were identified to be transcription factors.
The functional analysis suggested that the effector proteins in the interactions are highly enriched in superoxide metabolic process (GO:0006801), protein dephosphorylation (GO:0006470), vesicle (GO:0031982), phosphoric ester hydrolase activity (GO:0042578), and metabolic pathways (ko01100). These processes are involved in enhancing pathogenicity, development, and secretion during the host-pathogen interaction mechanism (Rodrigues et al., 2011; Tamayo et al., 2016; Rafiei et al., 2021), which helps in the survival of the pathogen under various stresses in the host cell. The host proteins associated with the effectors were found to be involved in secondary metabolic process (GO:0019748), cell wall polysaccharide metabolic process (GO:0010383), response to external biotic stimulus (GO:0043207), plant-pathogen interaction pathway (ko04626), and plant hormone signal transduction (ko04075). These processes are actively related to the plant defense and immune signaling against the biotic stresses. Thus, the predicted interactions of host proteins with the effectors can be considered of high confidence, and potential candidates for further studying the infection mechanism of stem rust in wheat.
Functional Differences Between Pgt 21-0 and Pgt Ug99
Furthermore, we were interested in deciphering the strain-specific functionalities of the Pgt 21-0 and Pgt Ug99 proteins involved in the PPIs. Since these proteins were not the orthologs of each other, hence these are referred to as unique proteins. The T. aestivum-Pgt 21-0 interactome predicted 68,465,557 PPIs, involving 83,962 host proteins and 7,063 unique Pgt 21-0 proteins, of which 100 proteins served as effectors. While the T. aestivum-Pgt Ug99 interactome involved 83,495 host proteins and 3,905 Pgt Ug99 proteins (16 effectors), accounting to 34,854,274 interactions. The low number of pathogens and predicted PPIs in Pgt Ug99 interactome as compared to that of Pgt 21-0 suggests the virulence of the pathogen, and that fewer pathogen proteins (mainly effectors) are capable of causing the infection.
Unique Puccinia graminis 21-0
The GO enrichment analysis of the unique pathogen proteins suggested that most of the GO terms were similar to that of the common subnetwork. But we also found 180 significant GO terms that were unique to Pgt 21-0 proteins. These included cell wall modification (GO:0042545), NADH dehydrogenase complex assembly (GO:0010257), cyclin-dependent protein kinase holoenzyme complex (GO:0000307), and SUMO ligase complex (GO:0106068). The unique KEGG pathways such as carotenoid biosynthesis (ko00906), and plant hormone signal transduction (ko4075) were found to be highly over-represented. Researchers in past have reported the direct or indirect role of these GO terms/KEGG pathways in pathogen virulence (Avalos et al., 2017). The detailed information of the significant GO terms and KEGG pathways for Pgt 21-0 is available in Supplementary Material 3, Sheets 2, 3, respectively. For the host proteins interacting with the unique Pgt 21-0 proteins, only 1 significant GO term (condensed chromosome kinetochore; GO:0000777) was identified. While no unique KEGG pathway was obtained for the host proteins.
Unique Puccinia graminis Ug99
In comparison with the common subnetwork, the unique Pgt Ug99 were highly enriched in 201 GO terms, including oxidoreductase activity (GO:0016491), regulation of response to stress (GO:0080134), snoRNA binding (GO:0030515), and GTPase complex (GO:1905360). The over-represented KEGG pathways obtained for these proteins involve monoterpenoid biosynthesis (ko00902), and polyketide sugar unit biosynthesis (ko00523), which have been shown to regulate the fungal growth and development (Chiang et al., 2009; Dallery et al., 2019; Noar et al., 2019). The significant GO terms and over-represented KEGG pathways have been detailed in Supplementary Material 3, Sheets 4, 5, respectively. While no significant GO terms or KEGG pathways were found for the host associated with unique Pgt Ug99 proteins.
Role of Transcription Factors in Plant Defense
Recent molecular studies have elucidated the role of transcription factors (TFs) in diverse cellular mechanisms such as regulating gene expression, act as transcriptional activators or repressors, and in plant defense (Seo and Choi, 2015). The plant immune signal activation is tightly controlled by the gene-specific transcription factors that bind to cis-elements in the promoter region (Li et al., 2016). In line with this, we predicted the wheat proteins that serve as transcription factors using PlantTFDB v5.0 (Jin et al., 2017). This resulted in 5,577 wheat proteins that served as transcription factors in the common subnetwork, involved in 1,311,301 interactions with 1,600 Puccinia ortholog proteins. These transcription factors were classified into 55 TF families, of which 28 families are significantly involved in biotic and abiotic stresses. The major TF families include basic helix-loop-helix (bHLH), ethylene responsive factor (ERF), myeloblastosis related (MYB), WRKY, basic leucine zipper (bZIP), and NAM, ATAF1/2, and CUC2 (NAM). The host proteins and their respective TF family has been described in Supplementary Material 4 (Sheet 1). Researchers in the past have extensively demonstrated the crucial role of various transcription factors in diverse biological processes, and significant immune signaling pathways in response to plant defense against pathogen attack (Asai et al., 2002; Wu et al., 2009; Zander et al., 2010; Pieterse et al., 2012; Zhao et al., 2012).
Novel Stem Rust-Resistant Host Targets
Our study on the host-pathogen interaction system focuses on understanding the disease infection mechanism, host immune response, and identifying the host targets that show resistance against stem rust disease. The resistance (R) genes in host are responsible for the recognition of effector proteins (secreted by pathogens), followed by the initiation of immune responses. According to gene-for-gene hypothesis, a successful resistant response requires two genes: R gene in the host and corresponding avirulence (Avr) effector gene in the pathogen, which makes resistance dependent on the specific pathogen strain (Flor, 1971). The mutations in Avr genes leads to the inability of the corresponding R genes to recognize the Avr genes, thus resulting in the pathogen to overcome host resistance (Ellis et al., 2014). The recent advancement in plant breeding techniques, in conjunction with increasing genomic resources, has accelerated the identification (and cloning) process of wheat resistance genes (Andersen et al., 2020). Scientists also created a wheat R-gene atlas to facilitate the research community with an efficient resource of resistance genes in wheat, aiming at reducing the pathogen co-evolution (Hafeez et al., 2021).
In wheat, a total of 46 R genes are officially designated to show resistance against stem rust, of which only 20 belong to T. aestivum (Leonard and Szabo, 2005). A few of the identified R genes in wheat include Sr5, Sr13, Sr23, Sr27, Sr36, Sr40, etc., which have varying effect on Pgt races. In wheat, R gene Sr5 is known to limit the growth of avirulent Puccinia strain, while Sr22 advances the development of Pgt races (Hatta et al., 2020; Wu et al., 2020). Various researchers mapped Sr genes on wheat chromosomes 1BS, 2B, 3B, 5DL, 6AL, 6DS, and 7AL (Table 4), conferring resistance against stem rust during adult-plant stage. A QTL-based study on wheat identified stable QTLs: QSr-sparc-2B, QSr-sparc-7A, QSr-sparc-5A, QSr-sparc-6A, and QSr-sparc-7B on chromosome 2BS, 7AL, 5AL, 6AS, and 7BL, respectively (Bokore et al., 2021). Further, we identified the PPIs associated with these chromosomes, which accounted to 9,387,396 PPIs, involving 35,809 host and 1,956 pathogen proteins. Of the 1,956 pathogen proteins in the interactions, 18 proteins were identified as effectors, involved in 66,772 PPIs (Supplementary Material 4, Sheet 2). The maximum number of interactions (1,305,326 PPIs) were identified on chromosome 2B, on which five Sr genes (Sr19, Sr23, Sr28, Sr36, and Sr40) have been mapped. The plant immune response against various pathogens is mediated by nucleotide-binding and leucine-rich repeat (NLR) domain proteins. Among the identified 35,809 host proteins, 2,123 proteins were found to be associated with NLR domain.
To have deeper insight into the resistance mechanism, we analyzed Sr22 gene located on chromosome 7A and cloned using MutRenSeq (Steuernagel et al., 2016). In the predicted interactome, the protein encoded by this gene was found to be interacting with 230 pathogen proteins (230 PPIs). The host protein was actively involved in various plant defense pathways such as MAPK signaling, plant-pathogen interaction, and plant hormone signal transduction pathway. Additionally, 4 effectors were also identified (Figure 6). It has also been reported that the stem rust resistance genes (Sr22, Sr33, Sr35, and Sr45) form a complex that effectively confers resistance against TTKSK in wheat (Hatta et al., 2021). Further, we identified the interactions associated with this complex in the predicted interactome, which resulted in 1,051 PPIs, involving 327 pathogen proteins, of which 4 were effectors (Figure 7). The host proteins involved in the interactions can be considered as the novel targets for the breeders for development of disease-resistant lines. Further, the interaction of these novel host proteins with the effectors are the potential candidate pairs to understand the immune responses against the fungal pathogen attack during stem rust disease, thus giving deeper insights to the infection mechanism and host defense responses.
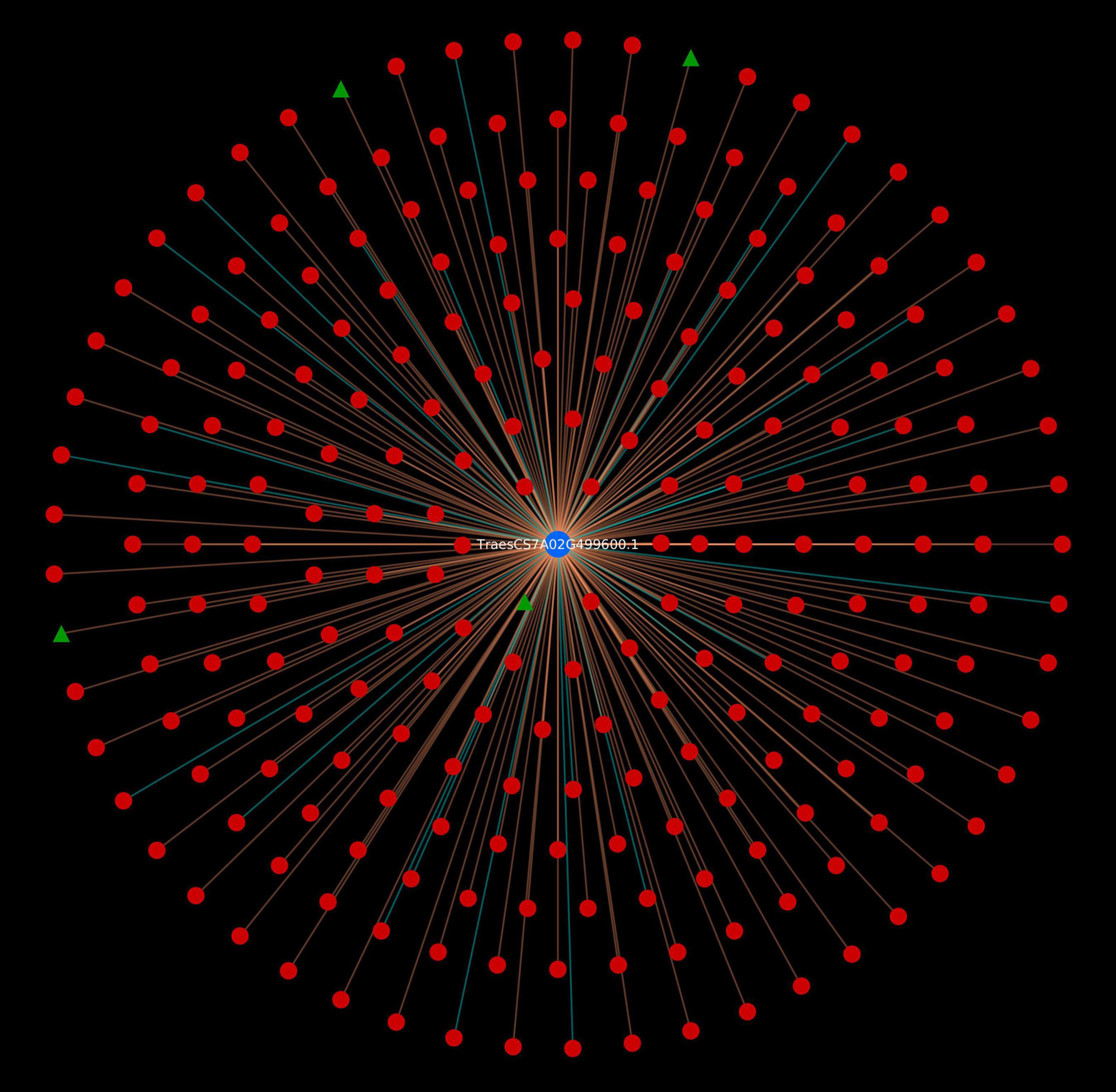
Figure 6. Wheat stem resistance gene Sr22 encoded protein. Blue nodes represent host protein, red nodes are pathogen proteins, and green nodes are effector proteins. Orange edges depict the interactions from interolog-based approach, while cyan edges belong to domain-based approach.
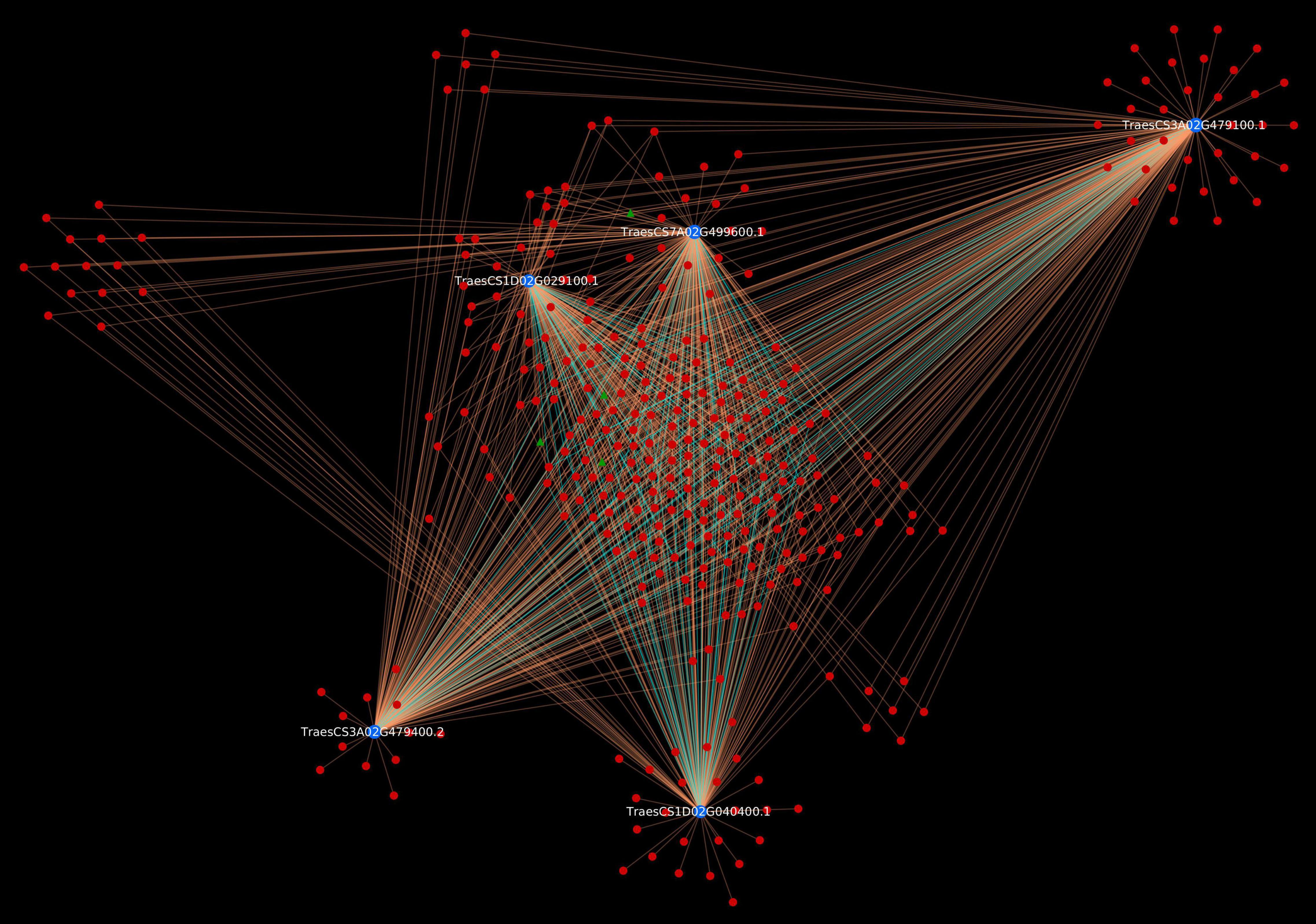
Figure 7. Stem rust resistance gene complex (Sr22, Sr33, Sr35, and Sr45) causing resistance against TTKSK race. Blue nodes represent host proteins, red nodes are pathogen proteins, and green nodes are effector proteins. Orange edges depict the interactions from interolog-based approach, while cyan edges belong to domain-based approach.
Data Availability Statement
The original contributions presented in this study are included in the article/Supplementary Material, further inquiries can be directed to the corresponding author.
Author Contributions
RKu formulated and designed the research and contributed to writing – review and editing, supervision, project administration, and funding acquisition. RKt analyzed the data, developed prediction models, performed functional analysis of the data, validations, literature mining, etc., and contributed to writing – original draft preparation. Both authors contributed to visualization, read, and agreed to the published version of the manuscript.
Funding
We acknowledged the support to this study from Utah Agricultural Experiment Station (UAES), Utah State University, seed grant #A48025 to RKu. This research was also partially supported by UAES office and approved as journal paper number 9587. The funding body did not play any role in the design of this study; the collection, analysis, or interpretation of data; or in the writing of this manuscript.
Conflict of Interest
The authors declare that the research was conducted in the absence of any commercial or financial relationships that could be construed as a potential conflict of interest.
Publisher’s Note
All claims expressed in this article are solely those of the authors and do not necessarily represent those of their affiliated organizations, or those of the publisher, the editors and the reviewers. Any product that may be evaluated in this article, or claim that may be made by its manufacturer, is not guaranteed or endorsed by the publisher.
Acknowledgments
We appreciate the team members of KAABiL (Kaundal Artificial intelligence & Advanced Bioinformatics Lab) for their valuable time and inputs at the time of research. We profoundly thank the referees for the suggestions to improve the research article.
Supplementary Material
The Supplementary Material for this article can be found online at: https://www.frontiersin.org/articles/10.3389/fpls.2022.895480/full#supplementary-material
Footnotes
- ^ https://plants.ensembl.org/index.html
- ^ https://www.ncbi.nlm.nih.gov/
- ^ https://fungi.ensembl.org/index.html
- ^ http://effectorp.csiro.au/
- ^ https://services.healthtech.dtu.dk/service.php?SignalP-5.0
References
Admassu, B., Perovic, D., Friedt, W., and Ordon, F. (2011). Genetic mapping of the stem rust (Puccinia graminis f. sp. tritici Eriks. & E. Henn) resistance gene Sr13 in wheat (Triticum aestivum L.). Theor. Appl. Genet. 122, 643–648. doi: 10.1007/s00122-010-1433-3
Afzal, F., Chaudhari, S. K., Gul, A., Farooq, A., Ali, H., Nisar, S., et al. (2015). “Bread wheat (Triticum aestivum L.) under biotic and abiotic stresses: an overview,” in Crop Production and Global Environmental Issues, ed. K. R. Hakeem (Cham: Springer International Publishing), 293–317. doi: 10.1007/978-3-319-23162-4_13
Agapito, G., Guzzi, P. H., and Cannataro, M. (2013). Visualization of protein interaction networks: problems and solutions. BMC Bioinformatics 14:S1. doi: 10.1186/1471-2105-14-S1-S1
Ahuja, I., Kissen, R., and Bones, A. M. (2012). Phytoalexins in defense against pathogens. Trends Plant Sci. 17, 73–90. doi: 10.1016/j.tplants.2011.11.002
Almagro Armenteros, J. J., Sønderby, C. K., Sønderby, S. K., Nielsen, H., and Winther, O. (2017). DeepLoc: prediction of protein subcellular localization using deep learning. Bioinformatics 33, 3387–3395. doi: 10.1093/bioinformatics/btx431
Almagro Armenteros, J. J., Tsirigos, K. D., Sønderby, C. K., Petersen, T. N., Winther, O., Brunak, S., et al. (2019). SignalP 5.0 improves signal peptide predictions using deep neural networks. Nat. Biotechnol. 37, 420–423. doi: 10.1038/s41587-019-0036-z
Andersen, E., Lindsey, L., and Nepal, M. (2020). Genome-wide identification of disease resistance genes (R Genes) in wheat. bioRxiv [Preprint] doi: 10.1101/2020.07.18.210286
Asai, T., Tena, G., Plotnikova, J., Willmann, M. R., Chiu, W. L., Gomez-Gomez, L., et al. (2002). Map kinase signalling cascade in Arabidopsis innate immunity. Nature 415, 977–983. doi: 10.1038/415977a
Avalos, J., Pardo-Medina, J., Parra-Rivero, O., Ruger-Herreros, M., Rodríguez-Ortiz, R., Hornero-Méndez, D., et al. (2017). Carotenoid biosynthesis in Fusarium. J. Fungi 3, 39. doi: 10.3390/jof3030039
Bavetsias, V., and Linardopoulos, S. (2015). Aurora kinase inhibitors: current status and outlook. Front. Oncol. 5:278. doi: 10.3389/fonc.2015.00278
Becht, P., Vollmeister, E., and Feldbrügge, M. (2005). Role for RNA-binding proteins implicated in pathogenic development of Ustilago maydis. Eukaryot. Cell 4, 121–133. doi: 10.1128/EC.4.1.121-133.2005
Benjamini, Y., Hochberg, Y., Benjamini, R., and Yoav, H. Y. (1995). Benjamini and Y FDR.pdf. J. R. Stat. Soc. Ser. B 57, 289–300.
Bessire, M., Chassot, C., Jacquat, A. C., Humphry, M., Borel, S., Petétot, J. M. D. C., et al. (2007). A permeable cuticle in Arabidopsis leads to a strong resistance to Botrytis cinerea. EMBO J. 26, 2158–2168. doi: 10.1038/sj.emboj.7601658
Bhuiyan, N. H., Selvaraj, G., Wei, Y., and King, J. (2009). Gene expression profiling and silencing reveal that monolignol biosynthesis plays a critical role in penetration defence in wheat against powdery mildew invasion. J. Exp. Bot. 60, 509–521. doi: 10.1093/jxb/ern290
Bokore, F. E., Cuthbert, R. D., Hiebert, C. W., Fetch, T. G., Pozniak, C. J., N’Diaye, A., et al. (2021). Mapping stem rust resistance loci effective in Kenya in Canadian spring wheat (Triticum aestivum L.) lines ‘AAC Prevail’ and ‘BW961.’. Can. J. Plant Pathol. 43, S263–S274. doi: 10.1080/07060661.2021.1966651
Bose, T., Venkatesh, K. V., and Mande, S. S. (2017). Computational analysis of host–pathogen protein interactions between humans and different strains of enterohemorrhagic Escherichia coli. Front. Cell. Infect. Microbiol. 7:128. doi: 10.3389/fcimb.2017.00128
Breuers, F. K. H., Bräutigam, A., and Weber, A. P. M. (2011). The plastid outer envelope - A highly dynamic interface between plastid and cytoplasm. Front. Plant Sci. 2:97. doi: 10.3389/fpls.2011.00097
Briesemeister, S., Rahnenführer, J., and Kohlbacher, O. (2010). Going from where to why-interpretable prediction of protein subcellular localization. Bioinformatics 26, 1232–1238. doi: 10.1093/bioinformatics/btq115
Caplan, J. L., Kumar, A. S., Park, E., Padmanabhan, M. S., Hoban, K., Modla, S., et al. (2015). Chloroplast stromules function during innate immunity. Dev. Cell 34, 45–57. doi: 10.1016/j.devcel.2015.05.011
Chatr-Aryamontri, A., Oughtred, R., Boucher, L., Rust, J., Chang, C., Kolas, N. K., et al. (2017). The BioGRID interaction database: 2017 update. Nucleic Acids Res. 45, D369–D379. doi: 10.1093/nar/gkw1102
Chen, L., Geng, X., Ma, Y., Zhao, J., Chen, W., Xing, X., et al. (2019). The ER lumenal Hsp70 protein FpLhs1 is important for conidiation and plant infection in Fusarium pseudograminearum. Front. Microbiol. 10:1401. doi: 10.3389/fmicb.2019.01401
Chen, P. Y., Deane, C. M., and Reinert, G. (2008). Predicting and validating protein interactions using network structure. PLoS Comput. Biol. 4:e1000118. doi: 10.1371/journal.pcbi.1000118
Chi, S. M. (2010). Prediction of protein subcellular localization by weighted gene ontology terms. Biochem. Biophys. Res. Commun. 399, 402–405. doi: 10.1016/j.bbrc.2010.07.086
Chiang, Y. M., Szewczyk, E., Davidson, A. D., Keller, N., Oakley, B. R., and Wang, C. C. C. (2009). A gene cluster containing two fungal polyketide synthases encodes the biosynthetic pathway for a polyketide, asperfuranone, in Aspergillus nidulans. J. Am. Chem. Soc. 131, 2965–2970. doi: 10.1021/ja8088185
Crossa, J., Burgueño, J., Dreisigacker, S., Vargas, M., Herrera-Foessel, S. A., Lillemo, M., et al. (2007). Association analysis of historical bread wheat germplasm using additive genetic covariance of relatives and population structure. Genetics 177, 1889–1913. doi: 10.1534/genetics.107.078659
Dallery, J. F., Adelin, É, Le Goff, G., Pigné, S., Auger, A., Ouazzani, J., et al. (2019). H3K4 trimethylation by CclA regulates pathogenicity and the production of three families of terpenoid secondary metabolites in Colletotrichum higginsianum. Mol. Plant Pathol. 20, 831–842. doi: 10.1111/mpp.12795
de Dios Barajas-López, J., Blanco, N. E., and Strand, Å (2013). Plastid-to-nucleus communication, signals controlling the running of the plant cell. Biochim. Biophys. Acta Mol. Cell Res. 1833, 425–437. doi: 10.1016/j.bbamcr.2012.06.020
Dean, R., Van Kan, J. A. L., Pretorius, Z. A., Hammond-Kosack, K. E., Di Pietro, A., Spanu, P. D., et al. (2012). The Top 10 fungal pathogens in molecular plant pathology. Mol. Plant Pathol. 13, 414–430. doi: 10.1111/j.1364-3703.2011.00783.x
Devoto, A., Muskett, P. R., and Shirasu, K. (2003). Role of ubiquitination in the regulation of plant defence against pathogens. Curr. Opin. Plant Biol. 6, 307–311. doi: 10.1016/S1369-5266(03)00060-8
Duplessis, S., Cuomo, C. A., Lin, Y. C., Aerts, A., Tisserant, E., Veneault-Fourrey, C., et al. (2011). Obligate biotrophy features unraveled by the genomic analysis of rust fungi. Proc. Natl. Acad. Sci. U.S.A. 108, 9166–9171. doi: 10.1073/pnas.1019315108
El Sabagh, A., Islam, M. S., Skalicky, M., Ali Raza, M., Singh, K., Anwar Hossain, M., et al. (2021). Salinity stress in wheat (Triticum aestivum L.) in the changing climate: adaptation and management strategies. Front. Agron. 3:1661932. doi: 10.3389/fagro.2021.661932
Ellis, J. G., Lagudah, E. S., Spielmeyer, W., and Dodds, P. N. (2014). The past, Present and future of breeding rust resistant wheat. Front. Plant Sci. 5:641. doi: 10.3389/fpls.2014.00641
Emms, D. M., and Kelly, S. (2019). OrthoFinder: phylogenetic orthology inference for comparative genomics. Genome Biol. 20, 1–14. doi: 10.1186/s13059-019-1832-y
Fabri, J. H. T. M., Godoy, N. L., Rocha, M. C., Munshi, M., Cocio, T. A., Von Zeska Kress, M. R., et al. (2019). The AGC kinase YpkA regulates sphingolipids biosynthesis and physically interacts with SakA MAP kinase in Aspergillus fumigatus. Front. Microbiol. 10:3347. doi: 10.3389/fmicb.2018.03347
Figueroa, M., Hammond-Kosack, K. E., and Solomon, P. S. (2018). A review of wheat diseases—a field perspective. Mol. Plant Pathol. 19, 1523–1536. doi: 10.1111/mpp.12618
Flor, H. H. (1971). Current status of the gene-fob-gene concept. Annu. Rev. Phytopathol. 9, 275–296.
Fu, L., Niu, B., Zhu, Z., Wu, S., and Li, W. (2012). CD-HIT: accelerated for clustering the next-generation sequencing data. Bioinformatics 28, 3150–3152. doi: 10.1093/bioinformatics/bts565
Garapati, H. S., Male, G., and Mishra, K. (2020). Predicting subcellular localization of proteins using protein-protein interaction data. Genomics 112, 2361–2368. doi: 10.1016/j.ygeno.2020.01.007
Garbutt, C. C., Bangalore, P. V., Kannar, P., and Mukhtar, M. S. (2014). Getting to the edge: protein dynamical networks as a new frontier in plant-microbe interactions. Front. Plant Sci. 5:312. doi: 10.3389/fpls.2014.00312
Ghazvini, H., Hiebert, C. W., Zegeye, T., Liu, S., Dilawari, M., Tsilo, T., et al. (2012). Inheritance of resistance to Ug99 stem rust in wheat cultivar Norin 40 and genetic mapping of Sr42. Theor. Appl. Genet. 125, 817–824. doi: 10.1007/s00122-012-1874-y
Goldberg, J. M., Griggs, A. D., Smith, J. L., Haas, B. J., Wortman, J. R., and Zeng, Q. (2013). Kinannote, a computer program to identify and classify members of the eukaryotic protein kinase superfamily. Bioinformatics 29, 2387–2394. doi: 10.1093/bioinformatics/btt419
Hafeez, A. N., Arora, S., Ghosh, S., Gilbert, D., Bowden, R. L., and Wulff, B. B. H. (2021). Creation and judicious application of a wheat resistance gene atlas. Mol. Plant 14, 1053–1070. doi: 10.1016/j.molp.2021.05.014
Hatta, M. A. M., Arora, S., Ghosh, S., Matny, O., Smedley, M. A., Yu, G., et al. (2021). The wheat Sr22, Sr33, Sr35 and Sr45 genes confer resistance against stem rust in barley. Plant Biotechnol. J. 19, 273–284. doi: 10.1111/pbi.13460
Hatta, M. A. M., Ghosh, S., Athiyannan, N., Richardson, T., Steuernagel, B., Yu, G., et al. (2020). Extensive genetic variation at the sr22 wheat stem rust resistance gene locus in the grasses revealed through evolutionary genomics and functional analyses. Mol. Plant Microbe Interact. 33, 1286–1298. doi: 10.1094/MPMI-01-20-0018-R
He, X., Wang, C., Wang, H., Li, L., and Wang, C. (2020). The function of MAPK cascades in response to various stresses in horticultural plants. Front. Plant Sci. 11:952. doi: 10.3389/fpls.2020.00952
Hiebert, C. W., Fetch, T. G., and Zegeye, T. (2010). Genetics and mapping of stem rust resistance to Ug99 in the wheat cultivar Webster. Theor. Appl. Genet. 121, 65–69. doi: 10.1007/s00122-010-1291-z
Howe, G. A., and Schilmiller, A. L. (2002). Oxylipin metabolism in response to stress. Curr. Opin. Plant Biol. 5, 230–236. doi: 10.1016/S1369-5266(02)00250-9
Jalmi, S. K., and Sinha, A. K. (2016). Functional involvement of a mitogen activated protein kinase module, OsMKK3-OsMPK7-OsWRK30 in mediating resistance against Xanthomonas oryzae in rice. Sci. Rep. 6:37974. doi: 10.1038/srep37974
Jiao, M., Yu, D., Tan, C., Guo, J., Lan, D., Han, E., et al. (2017). Basidiomycete-specific PsCaMKL1 encoding a CaMK-like protein kinase is required for full virulence of Puccinia striiformis f. sp. Tritici. Environ. Microbiol. 19, 4177–4189. doi: 10.1111/1462-2920.13881
Jin, J., Tian, F., Yang, D. C., Meng, Y. Q., Kong, L., Luo, J., et al. (2017). PlantTFDB 4.0: toward a central hub for transcription factors and regulatory interactions in plants. Nucleic Acids Res. 45, D1040–D1045. doi: 10.1093/nar/gkw982
Johnson, R. R., Wagner, R. L., Verhey, S. D., and Walker-Simmons, M. K. (2002). The abscisic acid-responsive kinase PKABA1 interacts with a seed-specific abscisic acid response element-binding factor, TaABF, and phosphorylates TaABF peptide sequences. Plant Physiol. 130, 837–846. doi: 10.1104/pp.001354
Jonsson, P. F., and Bates, P. A. (2006). Global topological features of cancer proteins in the human interactome. Bioinformatics 22, 2291–2297. doi: 10.1093/bioinformatics/btl390
Kataria, R., Duhan, N., and Kaundal, R. (2022). Computational systems biology of alfalfa – bacterial blight host-pathogen interactions: uncovering the complex molecular networks for developing durable disease resistant crop. Front. Plant Sci. 12:807354. doi: 10.3389/fpls.2021.807354
Kataria, R., and Kaundal, R. (2021). alfaNET: a database of alfalfa-bacterial stem blight protein–protein interactions revealing the molecular features of the disease-causing bacteria. Int. J. Mol. Sci. 22:8342. doi: 10.3390/ijms22158342
Kerrien, S., Aranda, B., Breuza, L., Bridge, A., Broackes-Carter, F., Chen, C., et al. (2012). The IntAct molecular interaction database in 2012. Nucleic Acids Res. 40, 841–846. doi: 10.1093/nar/gkr1088
Kim, D. Y., Kwon, S. I., Choi, C., Lee, H., Ahn, I., Park, S. R., et al. (2013). Expression analysis of rice VQ genes in response to biotic and abiotic stresses. Gene 529, 208–214. doi: 10.1016/j.gene.2013.08.023
Kim, K. T., Jeon, J., Choi, J., Cheong, K., Song, H., Choi, G., et al. (2016). Kingdom-wide analysis of fungal small secreted proteins (SSPs) reveals their potential role in host association. Front. Plant Sci. 7:186. doi: 10.3389/fpls.2016.00186
Kim, Y., Min, B., and Yi, G. S. (2012). IDDI: integrated domain-domain interaction and protein interaction analysis system. Proteome Sci. 10:S9. doi: 10.1186/1477-5956-10-S1-S9
Kishimoto, K., Matsui, K., Ozawa, R., and Takabayashi, J. (2008). Direct fungicidal activities of C6-aldehydes are important constituents for defense responses in Arabidopsis against Botrytis cinerea. Phytochemistry 69, 2127–2132. doi: 10.1016/j.phytochem.2008.04.023
Kumar, R., and Nanduri, B. (2010). HPIDB - a unified resource for host-pathogen interactions. BMC Bioinformatics 11:S16. doi: 10.1186/1471-2105-11-16
Kurubanjerdjit, N., Tsai, J. J. P., Sheu, C. Y., and Ng, K. L. (2013). The prediction of protein-protein interaction of A. thaliana and X. campestris pv. campestris based on protein domain and interolog approaches. Plant Omics 6, 388–398.
Kuzmanov, U., and Emili, A. (2013). Protein-protein interaction networks: probing disease mechanisms using model systems. Genome Med. 5, 1–12. doi: 10.1186/gm441
Leonard, K. J., and Szabo, L. J. (2005). Stem rust of small grains and grasses caused by Puccinia graminis. Mol. Plant Pathol. 6, 99–111. doi: 10.1111/j.1364-3703.2005.00273.x
Li, B., Meng, X., Shan, L., and He, P. (2016). Transcriptional regulation of pattern-triggered immunity in plants. Cell Host Microbe 19, 641–650. doi: 10.1016/j.chom.2016.04.011
Li, F., Upadhyaya, N. M., Sperschneider, J., Matny, O., Nguyen-Phuc, H., Mago, R., et al. (2019). Emergence of the Ug99 lineage of the wheat stem rust pathogen through somatic hybridisation. Nat. Commun. 10:5068. doi: 10.1038/s41467-019-12927-7
Li, Z. G., He, F., Zhang, Z., and Peng, Y. L. (2012). Prediction of protein-protein interactions between Ralstonia solanacearum and Arabidopsis thaliana. Amino Acids 42, 2363–2371. doi: 10.1007/s00726-011-0978-z
Licata, L., Briganti, L., Peluso, D., Perfetto, L., Iannuccelli, M., Galeota, E., et al. (2012). MINT, the molecular interaction database: 2012 Update. Nucleic Acids Res. 40, 857–861. doi: 10.1093/nar/gkr930
Luo, Y., Zhang, H., Qi, L., Zhang, S., Zhou, X., Zhang, Y., et al. (2014). FgKin1 kinase localizes to the septal pore and plays a role in hyphal growth, ascospore germination, pathogenesis, and localization of Tub1 beta-tubulins in Fusarium graminearum. New Phytol. 204, 943–954. doi: 10.1111/nph.12953
Mao, X., Zhang, H., Tian, S., Chang, X., and Jing, R. (2010). TaSnRK2.4, an SNF1-type serine/threonine protein kinase of wheat (Triticum aestivum L.), confers enhanced multistress tolerance in Arabidopsis. J. Exp. Bot. 61, 683–696. doi: 10.1093/jxb/erp331
Mathesius, U. (2018). Flavonoid functions in plants and their interactions with other organisms. Plants 7, 7–9. doi: 10.3390/plants7020030
Matthews, L. R., Vaglio, P., Reboul, J., Ge, H., Davis, B. P., Garrels, J., et al. (2001). Identification of potential interaction networks using sequence-based searches for conserved protein-protein interactions or “interologs.”. Genome Res. 11, 2120–2126. doi: 10.1101/gr.205301
Miransari, M., and Smith, D. (2019). Sustainable wheat (Triticum aestivum L.) production in saline fields: a review. Crit. Rev. Biotechnol. 39, 999–1014. doi: 10.1080/07388551.2019.1654973
Mosca, R., Céol, A., Stein, A., Olivella, R., and Aloy, P. (2014). 3did: a catalog of domain-based interactions of known three-dimensional structure. Nucleic Acids Res. 42, 374–379. doi: 10.1093/nar/gkt887
Nadal-Ribelles, M., Solé, C., Martínez-Cebrián, G., Posas, F., and de Nadal, E. (2019). “Shaping the transcriptional landscape through MAPK signaling,” in Gene Expression and Control, ed. F. Uchiumi (Rijeka: IntechOpen), doi: 10.5772/intechopen.80634
Ng, S. K., Zhang, Z., and Tan, S. H. (2003). Integrative approach for computationally inferring protein domain interactions. Bioinformatics 19, 923–929. doi: 10.1093/bioinformatics/btg118
Noar, R. D., Thomas, E., Xie, D. Y., Carter, M. E., Ma, D., and Daub, M. E. (2019). A polyketide synthase gene cluster associated with the sexual reproductive cycle of the banana pathogen, Pseudocercospora fijiensis. PLoS One 14:e0220319. doi: 10.1371/journal.pone.0220319
Nourani, E., Khunjush, F., and Durmus, S. (2015). Computational approaches for prediction of pathogen-host protein-protein interactions. Front. Microbiol. 6:94. doi: 10.3389/fmicb.2015.00094
Obembe, O. S., Hendricks, N. P., and Tack, J. (2021). Decreased wheat production in the USA from climate change driven by yield losses rather than crop abandonment. PLoS One 16:e0252067. doi: 10.1371/journal.pone.0252067
Olivera, P. D., Badebo, A., Xu, S. S., Klindworth, D. L., and Jin, Y. (2012). Resistance to race TTKSK of Puccinia graminis f. sp. tritici in Emmer wheat. Crop Sci. 52, 2234–2242. doi: 10.2135/cropsci2011.12.0645
País, S. M., Téllez-Iñón, M. T., and Capiati, D. A. (2009). Serine/threonine protein phosphatases type 2A and their roles in stress signaling. Plant Signal. Behav. 4, 1013–1015. doi: 10.4161/psb.4.11.9783
Park, C. J., and Seo, Y. S. (2015). Heat shock proteins: a review of the molecular chaperones for plant immunity. Plant Pathol. J. 31, 323–333. doi: 10.5423/PPJ.RW.08.2015.0150
Piasecka, A., Jedrzejczak-Rey, N., and Bednarek, P. (2015). Secondary metabolites in plant innate immunity: conserved function of divergent chemicals. New Phytol. 206, 948–964. doi: 10.1111/nph.13325
Pieterse, C. M. J., Van Der Does, D., Zamioudis, C., Leon-Reyes, A., and Van Wees, S. C. M. (2012). Hormonal modulation of plant immunity. Annu. Rev. Cell Dev. Biol. 28, 489–521. doi: 10.1146/annurev-cellbio-092910-154055
Pinter, N., Andrea, C., Id, H., Id, M. H., Rekhter, D., Id, K. Z., et al. (2019). Signal peptide peptidase activity connects the unfolded protein response to plant defense suppression by Ustilago maydis. PLoS Pogthog. 15:e10 07734.
Piya, S., Shrestha, S. K., Binder, B., Neal Stewart, C., and Hewezi, T. (2014). Protein-protein interaction and gene co-expression maps of ARFs and Aux/IAAs in Arabidopsis. Front. Plant Sci. 5:744. doi: 10.3389/fpls.2014.00744
Pogány, M., Dankó, T., Kámán-Tóth, E., Schwarczinger, I., and Bozsó, Z. (2015). Regulatory proteolysis in Arabidopsis-Pathogen interactions. Int. J. Mol. Sci. 16, 23177–23194. doi: 10.3390/ijms161023177
Qin, J., Wang, G., Jiang, C., Xu, J. R., and Wang, C. (2015). Fgk3 glycogen synthase kinase is important for development, pathogenesis, and stress responses in Fusarium graminearum. Sci. Rep. 5:8504. doi: 10.1038/srep08504
Rafiei, V., Vélëz, H., and Tzelepis, G. (2021). The role of glycoside hydrolases in phytopathogenic fungi and oomycetes virulence. Int. J. Mol. Sci. 22:9359. doi: 10.3390/ijms22179359
Raghavachari, B., Tasneem, A., Przytycka, T. M., and Jothi, R. (2008). DOMINE: a database of protein domain interactions. Nucleic Acids Res. 36, 656–661. doi: 10.1093/nar/gkm761
Ramachandran, S. R., Yin, C., Kud, J., Tanaka, K., Mahoney, A. K., Xiao, F., et al. (2017). Effectors from wheat rust fungi suppress multiple plant defense responses. Phytopathology 107, 75–83. doi: 10.1094/PHYTO-02-16-0083-R
Ren, W., Sang, C., Shi, D., Song, X., Zhou, M., and Chen, C. (2018). Ubiquitin-like activating enzymes BcAtg3 and BcAtg7 participate in development and pathogenesis of Botrytis cinerea. Curr. Genet. 64, 919–930. doi: 10.1007/s00294-018-0810-3
Rodrigues, M. L., Nosanchuk, J. D., Schrank, A., Vainstein, M. H., Casadevall, A., and Nimrichter, L. (2011). Vesicular transport systems in fungi. Future Microbiol. 6, 1371–1381. doi: 10.2217/fmb.11.112
Rouse, M. N., Nava, I. C., Chao, S., Anderson, J. A., and Jin, Y. (2012). Identification of markers linked to the race Ug99 effective stem rust resistance gene Sr28 in wheat (Triticum aestivum L.). Theor. Appl. Genet. 125, 877–885. doi: 10.1007/s00122-012-1879-6
Ryals, J., Weymann, K., Lawton, K., Friedrich, L., Ellis, D., Steiner, H. Y., et al. (1997). The arabidopsis NIM1 protein shows homology to the mammalian transcription factor inhibitor IκB. Plant Cell 9, 425–439. doi: 10.1105/tpc.9.3.425
Saari, E. E., and Prescott, J. M. (1985). World Distribution in Relation to Economic Losses. Cambridge, MA: Academic Press, Inc, doi: 10.1016/b978-0-12-148402-6.50017-1
Sahu, S. S., Loaiza, C. D., and Kaundal, R. (2021). Plant-mSubP: a computational framework for the prediction of single- And multi-target protein subcellular localization using integrated machine-learning approaches. AoB Plants 12, 1–10. doi: 10.1093/AOBPLA/PLZ068
Sahu, S. S., Weirick, T., and Kaundal, R. (2014). Predicting genome-scale Arabidopsis-Pseudomonas syringae interactome using domain and interolog-based approaches. BMC Bioinformatics 15:S13. doi: 10.1186/1471-2105-15-S11-S13
Salwinski, L., Miller, C. S., Smith, A. J., Pettit, F. K., Bowie, J. U., and Eisenberg, D. (2004). The database of interacting proteins: 2004 update. Nucleic Acids Res. 32, 449–451. doi: 10.1093/nar/gkh086
Seo, E., and Choi, D. (2015). Functional studies of transcription factors involved in plant defenses in the genomics era. Brief. Funct. Genomics 14, 260–267. doi: 10.1093/bfgp/elv011
Shannon, P., Markiel, A., Ozier, O., Baliga, N. S., Wang, J. T., Ramage, D., et al. (1971). Cytoscape: a software environment for integrated models. Genome Res. 13:426. doi: 10.1101/gr.1239303.metabolite
Shewry, P. R., and Hey, S. J. (2015). The contribution of wheat to human diet and health. Food Energy Secur. 4, 178–202. doi: 10.1002/FES3.64
Sonah, H., Deshmukh, R. K., and Bélanger, R. R. (2016). Computational prediction of effector proteins in fungi: opportunities and challenges. Front. Plant Sci. 7:126. doi: 10.3389/fpls.2016.00126
Sperschneider, J., Catanzariti, A. M., Deboer, K., Petre, B., Gardiner, D. M., Singh, K. B., et al. (2017). LOCALIZER: subcellular localization prediction of both plant and effector proteins in the plant cell. Sci. Rep. 7:44598. doi: 10.1038/srep44598
Sperschneider, J., Dodds, P. N., Gardiner, D. M., Singh, K. B., and Taylor, J. M. (2018). Improved prediction of fungal effector proteins from secretomes with EffectorP 2.0. Mol. Plant Pathol. 19, 2094–2110. doi: 10.1111/mpp.12682
Spielmeyer, W., Sharp, P. J., and Lagudah, E. S. (2003). Identification and validation of markers linked to broad-spectrum stem rust resistance gene Sr2 in wheat (Triticum aestivum L.). Crop Sci. 43, 333–336. doi: 10.2135/cropsci2003.3330
Steuernagel, B., Periyannan, S. K., Hernández-Pinzón, I., Witek, K., Rouse, M. N., Yu, G., et al. (2016). Rapid cloning of disease-resistance genes in plants using mutagenesis and sequence capture. Nat. Biotechnol. 34, 652–655. doi: 10.1038/nbt.3543
Stokstad, E. (2007). Plant pathology. Deadly wheat fungus threatens world’s breadbaskets. Science 315, 1786–1787. doi: 10.1126/science.315.5820.1786
Sun, J., Li, Y., and Zhao, Z. (2007). Phylogenetic profiles for the prediction of protein-protein interactions: how to select reference organisms? Biochem. Biophys. Res. Commun. 353, 985–991. doi: 10.1016/j.bbrc.2006.12.146
Szklarczyk, D., Gable, A. L., Lyon, D., Junge, A., Wyder, S., Huerta-Cepas, J., et al. (2019). STRING v11: protein-protein association networks with increased coverage, supporting functional discovery in genome-wide experimental datasets. Nucleic Acids Res. 47, D607–D613. doi: 10.1093/nar/gky1131
Tamayo, D., Muñoz, J. F., Lopez, Á, Urán, M., Herrera, J., Borges, C. L., et al. (2016). Identification and Analysis of the role of superoxide dismutases isoforms in the Pathogenesis of Paracoccidioides spp. PLoS Negl. Trop. Dis. 10:e0004481. doi: 10.1371/journal.pntd.0004481
Tiwari, S., Thakur, R., and Shankar, J. (2015). Role of heat-shock proteins in cellular function and in the biology of fungi. Biotechnol. Res. Int. 2015, 1–11. doi: 10.1155/2015/132635
Tomczak, A., Mortensen, J. M., Winnenburg, R., Liu, C., Alessi, D. T., Swamy, V., et al. (2018). Interpretation of biological experiments changes with evolution of the Gene Ontology and its annotations. Sci. Rep. 8:5115. doi: 10.1038/s41598-018-23395-2
Tückmantel, S., Greul, J. N., Janning, P., Brockmeyer, A., Grütter, C., Simard, J. R., et al. (2011). Identification of Ustilago maydis Aurora kinase as a novel antifungal target. ACS Chem. Biol. 6, 926–933. doi: 10.1021/cb200112y
Upadhyaya, N. M., Garnica, D. P., Karaoglu, H., Sperschneider, J., Nemri, A., Xu, B., et al. (2015). Comparative genomics of australian isolates of the wheat stem rust pathogen puccinia graminis f. Sp. Tritici reveals extensive polymorphism in candidate effector genes. Front. Plant Sci. 5:759. doi: 10.3389/fpls.2014.00759
Upadhyaya, N. M., Mago, R., Staskawicz, B. J., Ayliffe, M. A., Ellis, J. G., and Dodds, P. N. (2014). A bacterial type III secretion assay for delivery of fungal effector proteins into wheat. Mol. Plant Microbe Interact. 27, 255–264. doi: 10.1094/MPMI-07-13-0187-FI
Urban, M., Cuzick, A., Seager, J., Wood, V., Rutherford, K., Venkatesh, S. Y., et al. (2020). PHI-base: the pathogen-host interactions database. Nucleic Acids Res. 48, D613–D620. doi: 10.1093/nar/gkz904
Usman, M. G., Rafii, M. Y., Martini, M. Y., Yusuff, O. A., Ismail, M. R., and Miah, G. (2017). Molecular analysis of Hsp70 mechanisms in plants and their function in response to stress. Biotechnol. Genet. Eng. Rev. 33, 26–39. doi: 10.1080/02648725.2017.1340546
Van de Wouw, A. P., Marcroft, S. J., Ware, A., Lindbeck, K., Khangura, R., and Howlett, B. J. (2014). Breakdown of resistance to the fungal disease, blackleg, is averted in commercial canola (Brassica napus) crops in Australia. Field Crop. Res. 166, 144–151. doi: 10.1016/j.fcr.2014.06.023
Van Ooijen, G., Lukasik, E., Van Den Burg, H. A., Vossen, J. H., Cornelissen, B. J. C., and Takken, F. L. W. (2010). The small heat shock protein 20 RSI2 interacts with and is required for stability and function of tomato resistance protein I-2. Plant J. 63, 563–572. doi: 10.1111/j.1365-313X.2010.04260.x
Wachi, S., Yoneda, K., and Wu, R. (2005). Interactome-transcriptome analysis reveals the high centrality of genes differentially expressed in lung cancer tissues. Bioinformatics 21, 4205–4208. doi: 10.1093/bioinformatics/bti688
Wang, C., Zhang, S., Hou, R., Zhao, Z., Zheng, Q., Xu, Q., et al. (2011). Functional analysis of the kinome of the wheat scab fungus Fusarium graminearum. PLoS Pathog. 7:e1002460. doi: 10.1371/journal.ppat.1002460
Wang, M., Zhu, X., Wang, K., Lu, C., Luo, M., Shan, T., et al. (2018). A wheat caffeic acid 3-O-methyltransferase TaCOMT-3D positively contributes to both resistance to sharp eyespot disease and stem mechanical strength. Sci. Rep. 8:6543. doi: 10.1038/s41598-018-24884-0
Wang, S., Cao, L., and Wang, H. (2016). Arabidopsis ubiquitin-conjugating enzyme UBC22 is required for female gametophyte development and likely involved in Lys11-linked ubiquitination. J. Exp. Bot. 67, 3277–3288. doi: 10.1093/jxb/erw142
Willocquet, L., Rossi, V., and Savary, S. (2021). Simulation modelling of yield losses caused by wheat stem rust. Plant Pathol. 71, 544–555. doi: 10.1111/ppa.13488
Wu, X. X., Lin, Q. J., Ni, X. Y., Sun, Q., Chen, R. Z., Xu, X. F., et al. (2020). Characterization of wheat monogenic lines with known sr genes and wheat lines with resistance to the ug99 race group for resistance to prevalent races of puccinia graminis f. sp. tritici in China. Plant Dis. 104, 1939–1943. doi: 10.1094/PDIS-12-19-2736-RE
Wu, Y., Deng, Z., Lai, J., Zhang, Y., Yang, C., Yin, B., et al. (2009). Dual function of Arabidopsis ATAF1 in abiotic and biotic stress responses. Cell Res. 19, 1279–1290. doi: 10.1038/cr.2009.108
Yu, G., Wang, L. G., Han, Y., and He, Q. Y. (2012). ClusterProfiler: an R package for comparing biological themes among gene clusters. Omi. A J. Integr. Biol. 16, 284–287. doi: 10.1089/omi.2011.0118
Yue, X., Que, Y., Deng, S., Xu, L., Oses-Ruiz, M., Talbot, N. J., et al. (2017). The cyclin dependent kinase subunit Cks1 is required for infection-associated development of the rice blast fungus Magnaporthe oryzae. Environ. Microbiol. 19, 3959–3981. doi: 10.1111/1462-2920.13796
Zander, M., La Camera, S., Lamotte, O., Métraux, J. P., and Gatz, C. (2010). Arabidopsis thaliana class-II TGA transcription factors are essential activators of jasmonic acid/ethylene-induced defense responses. Plant J. 61, 200–210. doi: 10.1111/j.1365-313X.2009.04044.x
Zhang, S., and Klessig, D. F. (2001). MAPK cascades in plant defense signaling. Trends Plant Sci. 6, 520–527. doi: 10.1016/S1360-1385(01)02103-3
Zhao, M., Joy, J., Zhou, W., De, S., Wood, W. H., Becker, K. G., et al. (2018). Transcriptional outcomes and kinetic patterning of gene expression in response to NF-κB activation. PLoS Biol. 16:e2006347. doi: 10.1371/journal.pbio.2006347
Zhao, Y., Wei, T., Yin, K. Q., Chen, Z., Gu, H., Qu, L. J., et al. (2012). Arabidopsis RAP2.2 plays an important role in plant resistance to Botrytis cinerea and ethylene responses. New Phytol. 195, 450–460. doi: 10.1111/j.1469-8137.2012.04160.x
Zhu, Y., Schluttenhoffer, C. M., Wang, P., Fu, F., Thimmapuram, J., Zhu, J. K., et al. (2014). CYCLIN-DEPENDENT KINASE8 differentially regulates plant immunity to fungal pathogens through kinase-dependent and -independent functions in Arabidopsis. Plant Cell 26, 4149–4170. doi: 10.1105/tpc.114.128611
Keywords: wheat, stem rust, computational modeling, effectors, interolog method, domain-based approach, disease resistance
Citation: Kataria R and Kaundal R (2022) Deciphering the Crosstalk Mechanisms of Wheat-Stem Rust Pathosystem: Genome-Scale Prediction Unravels Novel Host Targets. Front. Plant Sci. 13:895480. doi: 10.3389/fpls.2022.895480
Received: 13 March 2022; Accepted: 31 May 2022;
Published: 21 June 2022.
Edited by:
Xiaoli Fan, Chengdu Institute of Biology (CAS), ChinaCopyright © 2022 Kataria and Kaundal. This is an open-access article distributed under the terms of the Creative Commons Attribution License (CC BY). The use, distribution or reproduction in other forums is permitted, provided the original author(s) and the copyright owner(s) are credited and that the original publication in this journal is cited, in accordance with accepted academic practice. No use, distribution or reproduction is permitted which does not comply with these terms.
*Correspondence: Rakesh Kaundal, rkaundal@usu.edu