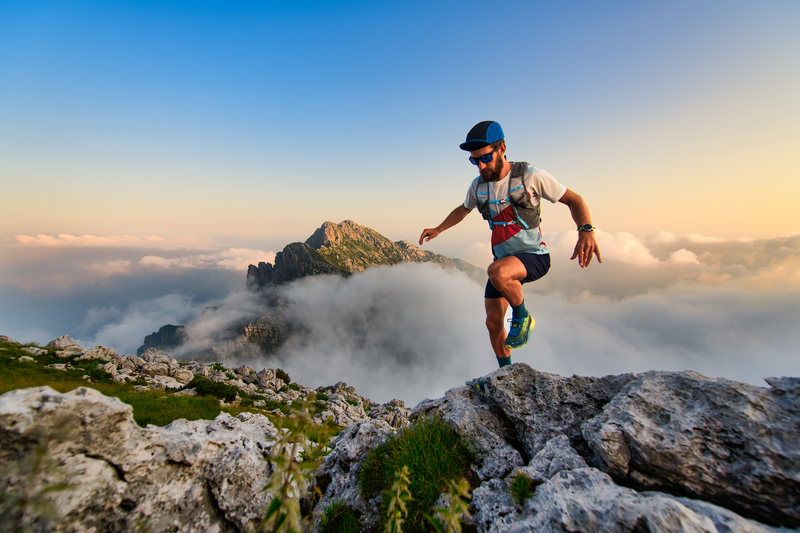
94% of researchers rate our articles as excellent or good
Learn more about the work of our research integrity team to safeguard the quality of each article we publish.
Find out more
REVIEW article
Front. Plant Sci. , 29 April 2022
Sec. Plant Metabolism and Chemodiversity
Volume 13 - 2022 | https://doi.org/10.3389/fpls.2022.881856
This article is part of the Research Topic Plant Secondary Metabolic Regulation and Engineering View all 27 articles
A correction has been applied to this article in:
Corrigendum: Research progress and trends in metabolomics of fruit trees
Metabolomics is an indispensable part of modern systems biotechnology, applied in the diseases’ diagnosis, pharmacological mechanism, and quality monitoring of crops, vegetables, fruits, etc. Metabolomics of fruit trees has developed rapidly in recent years, and many important research results have been achieved in combination with transcriptomics, genomics, proteomics, quantitative trait locus (QTL), and genome-wide association study (GWAS). These research results mainly focus on the mechanism of fruit quality formation, metabolite markers of special quality or physiological period, the mechanism of fruit tree’s response to biotic/abiotic stress and environment, and the genetics mechanism of fruit trait. According to different experimental purposes, different metabolomic strategies could be selected, such as targeted metabolomics, non-targeted metabolomics, pseudo-targeted metabolomics, and widely targeted metabolomics. This article presents metabolomics strategies, key techniques in metabolomics, main applications in fruit trees, and prospects for the future. With the improvement of instruments, analysis platforms, and metabolite databases and decrease in the cost of the experiment, metabolomics will prompt the fruit tree research to achieve more breakthrough results.
To comprehensively and quantitatively study key metabolites in a biological system, “metabolomics,” which refers to the qualitative and quantitative analysis of all metabolites with molecular weight < 1,000 Da in organisms or cells, was proposed by Nicholson in 1999 (Nicholson et al., 1999).
Unlike DNA, RNA, and proteins, which consist of several fixed and known structural units, metabolites are reactive, are structurally diverse, and have a wide range of concentrations. Metabolites do not follow a fixed structural module and have different physical properties (Sumner et al., 2003). The Human Metabolome Database (HMDB) has identified more than 40,000 human metabolites. The environment in which plants grow and reproduce is far more complex than those of humans and animals and often disturbed by a variety of biotic and abiotic factors. Considering their own factors, plants can only adjust the whole process of their life cycle in a more flexible and diverse way, with the total number of metabolites produced by plants ranging from 200,000 to 1 million (Dixon and Strack, 2003).
Fruits provide irreplaceable nutrition to human and have an increasingly important impact on human health. Compared with animals and main crops, metabolomics of fruit trees starts later. But fruits are beneficial to human health due to their diverse secondary metabolites, including organic acids, flavonoids, anthocyanins, terpenes, etc., which are very suitable for the study by metabolomics technology. Thus, a rapid increase of research articles appears from 2017 to 2021 (Figure 1) according to the Web of Science1. Many important research results have been achieved in metabolomics of a fruit tree in recent years. In this article, main achievements in metabolomics of fruit tree will be introduced.
Metabolites in plants can generally be divided into primary and secondary metabolites. Primary metabolites are the basic material conditions necessary for the growth and development of organisms, while secondary metabolites are metabolites produced only at a certain stage or period of growth of an organism (Koch, 2004; Carreno-Quintero et al., 2013). Depending on the purpose of the experiment, researchers can choose individual metabolomics strategies, including targeted metabolomics, non-targeted metabolomics, pseudo-targeted metabolomics, and widely targeted metabolomics.
Targeted metabolomics assays are performed on known compounds with targeted extraction methods and high-purity standards to quantify the target metabolism. Generally, targeted metabolomics focuses on fewer compounds and can be searched directly in classified databases, for example, databases for glycans and lipids such as LipidMaps and LipidBank (Cajka and Fiehn, 2016). Non-targeted metabolomics assays are performed on unknown compounds. It covers as many compounds of different properties and classes as possible in the extraction and detection, with a relatively broad coverage of substances. However, both the qualitative and quantitative properties of compounds are compromised by the lack of standards. Generally, non-targeted metabolomics studies are used to screen for differential metabolites in different treatments, and targeted metabolomics studies are used for the next more precise study.
To overcome the disadvantages of non-targeted metabolomics, widely targeted metabolomics, also known as second-generation targeted metabolomics, allows for the simultaneous detection of thousands of substances by the establishment of a database of thousands of metabolite specimens (Wei et al., 2013). Pseudo-targeted metabolomics is a quantitative ion selection algorithm based on untargeted metabolomics. It is used to perform quantitative ion selection for all detected metabolites. The ion-pair information of metabolites is obtained by high-resolution mass spectrometry (HR-MS), and the abundance of metabolites is measured by targeted multiple reaction monitoring (MRM). The method has high coverage, good linearity, and reproducibility and does not require standards to limit the metabolites’ detection. Both known and unknown metabolites in the sample can be measured (Zheng et al., 2020).
Spatial metabolomics integrates traditional metabolomics technology and MS imaging technology, which can not only identify what substances are contained in a sample and their contents as traditional metabolomics but also detect the spatial distribution information of compounds in a single experiment to achieve qualitative and quantitative positioning at the same time (Li et al., 2018).
The comparison of advantages and disadvantages of different metabolomic strategies is shown in Table 1.
The exploration of the diversity of fruit tree metabolism and potential molecular mechanisms by which fruit tree cells control their own chemical composition depends on the advances of analytical methods.
Samples should be collected representatively, with priority given to normally developing plants. Furthermore, 3–6 individuals should be selected as a source of biological replicates to avoid differences due to excessive individual differences. Ice boxes or ice packs should be used to collect samples in the field to reduce the degradation of metabolites by enzymes that are still active after sample collection. At the same time, samples should be collected with certain accuracy, and the samples of the experimental and control groups should be consistent in terms of time (Urbanczyk-Wochniak et al., 2005), site, and processing conditions, as far as the experiment allows. If the kernels are not studied, it should be removed with a scalpel in a low-temperature metal bath on an RNAase reagent-treated bench. For the study of the peel, the pulp should be taken as less as possible (Montefiori et al., 2009; de Godoy et al., 2013; Rudell et al., 2017). Freezing of the whole fruit should be avoided if possible during the sampling process, which will make the subsequent freeze-drying and grinding work difficult, and once the whole fruit is freeze-thawed, the whole experimental results will be affected. Then, the sample will be stored in a suitable volume of a centrifuge tube, marked and quickly put into liquid nitrogen for precooling, and then put into −80°C refrigerator for storage. Note that the samples should not be stored in tin foil, self-sealing bags, Ziploc bags, plastic bags, etc.; such packaging is easy to break under low temperature or in the process of transportation and easy to make the markings blurred, resulting in sample confusion.
The metabolites are usually extracted separately with water or organic solvents (e.g., methanol, chloroform, etc.) to obtain aqueous and organic solvent extracts, thus separating the non-polar lipophilic phase from the polar phase. To enrich the concentration of metabolites during the study, the process of vacuum concentration and spin-drying of the extracts before redissolution is often added. In the process of sample analysis method establishment, the optimal method can be figured out by trying different combination of extraction solvents, extraction temperatures, ratios of sample and solvent, redissolved solvent, mobile phase gradients, and mobile phases and by the controlled variable method (Kim and Verpoorte, 2010). The detection of non-targeted metabolites should include as many compound peaks as possible, and the detection of targeted metabolites should ensure that all concerned metabolites can be detected. The sample sequence requires the insertion of quality control samples at intervals of 10 or 20 during the detection by liquid chromatography-MS (LC-MS), which generally includes all compounds of the sample being tested or a mixture of a representative batch of samples. In addition, compounds not contained in the fruit trees are added as internal standards during the extraction of the samples to calibrate the loss of sample compounds during the extraction and detection process and thus to correct the peak areas of other compounds. In vivo direct-immersion solid-phase microextraction followed by gas chromatography-time-of-flight mass spectrometry method (SPME GC × GC-TOF-MS) can detect volatile compounds directly without extracting the compounds (Risticevic et al., 2016, 2020).
The flowchart of metabolomics sample preparation, analysis, and data processing is shown in Figure 2.
Chromatography is used for the purpose of separating metabolites according to the different affinities of compounds and columns and is generally divided into LC and gas chromatography (GC). LC is used to detect non-volatile compounds, polar compounds, thermally unstable compounds, and high-molecular-weight compounds (including proteins, peptides, and polymers). The mobile phase generally uses methanol, acetonitrile, water, or isopropanol; the added acid is generally acetic acid or formic acid, inorganic acid cannot be used (inorganic acid may corrode the pipeline); the added base is generally ammonium hydroxide or ammonia, generally does not use metal bases (will corrode the pipeline). Trifluoroacetic acid (TFA) helps to improve the separation of the liquid phase but will produce ion suppression in the positive and negative ion modes of MS. Triethylamine/trimethylamine (TEA/TMA) contributes to the formation of negative ions. GC can be used for the detection of volatile compounds, the mobile phase is an inert gas, and samples with a certain vapor pressure and good stability at column temperature can be detected. Substances with low volatility and easily decomposed by heat can be transformed by derivatization into derivatives with high volatility and good thermal stability for detection. N-methyl-N-trimethylsilyl trifluoroacetamide (MSTFA) and methoxamine hydrochloride are often used as derivatization reagents, as preliminary studies have shown that these compounds are the most suitable for the derivatization of plant metabolites (Fiehn et al., 2000).
Mass spectrometry separates some of the ions based on the mass to charge ratio. The ions pass through the analyzer and are separated according to different mass to charge ratios. Ions with the same mass to charge ratio are clustered together to form a mass spectrogram. Commonly used mass spectrometers are triple quadrupole mass analyzer (Yost and Enke, 1978), time-of-flight mass analyzer, ion trap mass analyzer, and electrostatic field orbital trap. The triple quadrupole mass analyzer consists of four straight rod electrodes suspended equidistantly parallel to the axis. Under the action of a certain DC/VC, ions with m/z meeting certain requirements can pass through the quadrupole to the detector, while other ions are filtered out. The advantages of the triple quadrupole are light weight, small size, and low cost. The time-of-flight mass analyzer uses pulses to extract ions from an ion source. The ions are accelerated by an accelerating voltage, have the same kinetic energy, and enter the drift tube. Ions with a small mass to charge ratio are the fastest and reach the detector first. Larger ions reach the detector last. The advantages of TOF MS are a wide range of mass to charge ratio of the detected ions, high sensitivity, and suitability for secondary tandem MS. A fast scanning speed is suitable for studying very fast processes. The principle of an ion trap mass analyzer is similar to that of quadrupole mass analyzer. When the HF voltage amplitude and HF voltage frequency are fixed to a certain value, only ions with a certain mass to charge ratio can be stabilized on a certain track in the trap. By rotating and changing the electrode voltage, different m/z ions fly out of the trap and reach the detector. The advantage of ion trap mass spectrometer is that a single ion trap can realize multistage “time” tandem MS, simple structure, low price, high cost performance, high sensitivity, and large mass range. The electrostatic field ion orbitrap is where analytes are ionized in an ion source and then sequentially enter a quadrupole, a C-trap, and an Orbitrap. The electrostatic field ion orbitrap mass spectrometer can perform high precision mass scans, and it is more stable than other mass analyzers.
Mass spectrometry characterizes compounds by different characteristic ions of different compounds. Chromatographic methods enhance the coverage of metabolites and improve the quantitative accuracy of MS. The most commonly used separation and analysis methods are LC-MS (Vos et al., 2007), GC-MS (Lisec et al., 2015). For LC-MS and GC-MS methods, internal standards are added before sample processing to correct errors caused by sample pretreatment and instrument response.
Data processing in metabolomics generally consists of two major steps, namely, data preprocessing and statistical analysis (Ma and Qi, 2021). Data preprocessing includes steps such as removing system noise signals, removing disturbing signals caused by system instability, and removing operational errors to provide a more reliable dataset for the next statistical analysis. Prior to formal data processing, tools such as XCMS, MZmine, and MarkerView are used to process the original peak area and other data for non-targeted metabolomics data analysis. The first step of the statistical analysis is unsupervised multivariate statistical analysis, usually using the principal component analysis (PCA). The second step may be the univariate statistical analysis to screen for variables with statistically significant differences in different groups. The third step is a supervised multivariate statistical analysis such as partial least squares discriminant analysis (PLS-DA) to select the variables that contribute more to the classification of the sample, i.e., screen for markers.
The purpose of the PCA analysis (Shaffer, 2002) is to find specific values that produce differences in a large amount of sample data. PLS-DA analysis will classify samples into groups, which is good for finding the similarities and differences between sample groups; orthogonal partial least squares discriminant analysis (OPLS-DA) can also distinguish the group differences between samples, but this analysis can better focus on some positive correlation data. The results of data analysis are also subject to the t-test and variable importance in projection (VIP) ranking values to screen for differential metabolites. It is generally accepted that variables meeting both P < 0.05 and VIP > 1.0 are differential metabolites. Metabolic pathways can be resolved using databases such as HMDB, KEGG, Reactome, BioCyc, and MetaCyc.
Fruit tree metabolomics has developed rapidly in recent years, and many important research results have been achieved in combination with transcriptomics, genomics, proteomics, quantitative trait locus (QTL), and genome-wide association study (GWAS) (Tables 2, 3). These research results mainly focus on the mechanism of fruit quality formation, metabolite markers of special quality or physiological period, key genes and metabolites of fruit tree resistance to biotic/abiotic stress, the influence of environment on the fruit tree, and fruit tree population genetic basis by combining with QTL and GWAS to narrow down QTL regions, predict candidate genes, construct the regulatory network, etc. (Figure 3).
Fruit quality, such as fruit color, sweet/acid ratio, size, firmness, aroma, and special nutrients, is very important trait for consumers and is the main factor determining market competitiveness. Research on the mechanisms of fruit quality formation can reveal new information about fruit physiology, provide new biomarkers for variety or producing area identification, and accelerate the improvement of the fruit quality.
From a flower to a mature fruit, undergoing complex changes, metabolomics reveals the composition and changes of the compounds that form the fruit quality. A widely targeted metabolomic analysis in pineapple (Ananas comosus L.) young fruits (YF), mature fruits (MF), and fully mature fruits (FMF) identified 466 metabolites. In comparison, lysophospholipids (LPLs) were found to be the highest in YF, and their content decreased gradually with fruit development and remained almost constant in FMF (Hong et al., 2021). During the ripening of ginseng berry berries, the content of malate gradually increases, and most amino acids and organic acids gradually decrease. Compounds that could distinguish different cultivation ages and maturity stages of ginseng were obtained (Mamat et al., 2020). Content variation in amino acid and isoprenoid in ASR1-antisense transgenic tomato (Solanum lycopersicum) fruits and normal tomato was examined by targeted metabolomics. Amino acids and isoprenoids were found to be significantly different in these two species. The combined analysis with transcriptome data demonstrates that the Asr1 [i.e., abscisic acid (ABA), stress, and ripening 1] transcription factor is involved in the cascade regulation of red tomato fruit maturation (Dominguez et al., 2021). Special metabolites also accumulate in mangosteen (Mamat et al., 2020), ginseng berry (Park et al., 2019), starfruit (Ramadan et al., 2020), avocado (Hurtado-Fernández et al., 2015), strawberry (Zhang et al., 2011; Wang et al., 2021e), apple (Rudell and Mattheis, 2009; Risticevic et al., 2016, 2020; Jing and Malladi, 2020; Xu et al., 2020), grape berry (Dai et al., 2013), mulberry (Lee et al., 2016), cupressaceae (Falasca et al., 2014), olea (Olmo-García et al., 2018), walnuts (Wang et al., 2021b), and African baobab (Baky et al., 2021) during fruit maturing.
Fruit color not only affects appetite but also affects nutrients. By targeting carotenoids in white fleshed apricots (Prunus armeniaca L.) “Kuchebaixing” and orange fleshed apricots “Shushangganxing” at different developmental stages (i.e., S1–S4), 14 carotenoids and 27 carotenoid lipids were identified in apricot flesh. The comparison revealed significant differences in the carotenoid content between these two different apricot cultivars during S3 and S4 development and finally identified β-carotene and (E/Z)-octahydro lycopene as the key metabolites responsible for the differences in total carotenoid content in the different cultivars. Combination of metabolome and transcriptome reveals PSY, NCED1, and CCD4 as key genes responsible for differential accumulation of the total carotenoid content in apricot fruit (Zhou et al., 2021). The red pericarp longan (Dimocarpus longan Lour.) (RP) and white longan (SX) were analyzed using widely targeted metabolomics; a total of 597 substances were identified in both white and red longan. Among them, 33 compounds, mainly flavonoids, were present in red-skinned longan and 23 compounds, mainly phenolic acids, were present in white-skinned longan. Cyanidin 3-O-glucoside, cyanidin 3-O-6″-malonyl-glucoside, and cyanidin O-syringic acid specially existed in RP longan. Delphinin 3-O-glucoside specially existed in SX longan. Accordingly, genes in the biosynthetic pathway of anthocyanin, such as F3H, F3’H, UFGT, GST, MYB, bHLH, NAC, and MADS, were detected significantly upregulated in RP longan using the transcriptome analysis (Yi et al., 2021). The analysis of six Tuscan sweet cherries (Prunus avium L.) endemic to Italy by non-targeted metabolomics successfully identified 15 metabolites in the positive ion mode and 14 metabolites in the anion mode. Most of these metabolites belong to flavonoids, including a highly potent antioxidant, cinchonain, while the highest flavonoid content was found in two varieties, namely, Crognola and Morellona (Berni et al., 2021). Anthocyanins also have important effects on fruit coloration in jujube (Zhang et al., 2020), fig (Wang et al., 2017), and Cerasus humilis (Ji et al., 2020).
The taste and aroma of the fruit are important intrinsic qualities of the fruit. A variation of metabolites in kiwifruit (Actinidia chinensis) flavor formation was revealed by widely targeted metabolomics to detect the content of sugar, organic matter, and volatile compounds in the flesh of kiwifruit during six developmental and six postharvest periods. A total of 34 flavor-related metabolites were identified. Further studies showed that the accumulation of metabolites in kiwifruit differed between the immature and mature stages. In the immature stage, the accumulated metabolites will tend to accumulate esters and sugars, and in the mature stage, they will tend to accumulate aldehydes, alcohols, ketones, and organic acids. In addition, a regulatory network that can regulate the production and accumulation of soluble sugars, organic acids, and volatiles was constructed by combining metabolomic and transcriptomic data analysis. This regulatory network can identify key structural and regulatory genes associated with flavor metabolism, key transcription factors that regulate the metabolism of soluble sugars and esters, and moreover, reveal their role in regulating the transcription of key structural genes involved in these metabolic pathways (Wang et al., 2022). Lipid compounds and phenylpropane derivatives were identified as the main components of the dates’ aroma by detecting 13 volatile compounds of dates (Phoenix dactylifera L.). In addition, 2,3-butanediol, hexaldehyde, hexol, and cinnamaldehyde can be used to distinguish between the different dates varieties (Khalil et al., 2017). Methyl hexatate, which was not detected in two other producing regions, is a volatile unique to Averrhoa carambola L. in Egypt (Ramadan et al., 2020). Special metabolites were differentially accumulated in different cultivar and wild persimmon varieties (Ramírez-Briones et al., 2019; Ryu et al., 2019), Citrus L. (Wang et al., 2016; Fayek et al., 2019; Villa-Ruano et al., 2019), and peach varieties (Karagiannis et al., 2021).
After the fruit is picked, the quality may change depending on the storage condition before entering the consumer’s table (Karagiannis, 2018; Wu et al., 2019, 2021; Lwin and Lee, 2020; Fuentealba, 2021). Comparing the changes of volatile compounds before and after 7 days of storage of 3 hairy peaches (Prunus persica L.) and 3 nectarines, it was found that the distribution of volatile compounds in fruits tended to be similar after storage. Using random forest analysis, it was possible to identify 15 volatile organic compounds, namely, 6 terpenes, 6 esters, 2 lactones, and 1 aromatic VOC, which differed between varieties before and after storage. Of which, 4 terpenes and 4 esters were positively correlated with nectarine. Another 16 VOCs highly correlated with 11 key VOC pathway genes (Muto et al., 2020). In ethephon-treated sweet cherries, fruit ripening and ethylene-related metabolites, such as malate and monosaccharides, have changed (Smith et al., 2011).
The development of metabolomics has made it possible to identify the active components of special fruit trees with medicinal value. Fifteen compounds were identified from the Kigelia africana fruit using UHPLC-TOF-MS and GC-MS. In which, physostigmine was demonstrated with an excellent anticancer activity (Fagbohun et al., 2021). In addition, nine antiaging compounds in Nephelium lappaceum (rambutan) seeds were identified (Lee et al., 2020). Genipap (Genipa americana L.) is a known fruit with medicinal value. Biomarkers after the administration of genipap in human urine is identified by LC-MS (Dickson et al., 2020).
Biotic stress and abiotic stress are great challenges to the fruit tree with impact on production, quality, and survival. The biotic/abiotic stress causes physiological changes, which eventually causes corresponding metabolites changes. By metabolomics analysis and in comparison with normal fruit tree, differential metabolites will be identified as new biomarkers of the biotic/abiotic stress and serve for disease diagnosis. Key metabolites pathways responding to biotic/abiotic stress can reveal molecular mechanism about resistance to biotic/abiotic stress.
Several researches have been carried out to find biomarkers and pathology of biotic stress, especially in microbe infection. Huanglongbing (HLB) (Candidatus Liberibacter sp.) is a common disease on citrus. Infested sugar orange (Citrus reticulata) is divided into one normal type and three abnormal types based on fruit peel color. Non-targeted metabolomics was used, and 215 significant differences metabolites were found between abnormal and normal fruit. Two unique metabolites (i.e., O-caffeoyl maltotriose and prunetin) were detected only in normal pericarp. Besides, it is also revealed that “phenylpropanoid biosynthesis” pathway exhibited obvious enrichment in all comparison groups, according to the metabolic pathway enrichment analysis of differential metabolites (Wang et al., 2020). Penicillium spp. is a citrus fruits susceptible pathogen. The contents of sugars, organic acids, vitamin C, and D-citmonene decreased in the citrus peel after infection, and the amount of ethanol and beta-terpene alcohol increased (Tang et al., 2018). Amino acids and their derivatives were significantly higher in the citrus peel of roughing disorder (Lu et al., 2017). In mulberry and apple, pathogen infection altered the levels of abscisic acid and cytokinin (Gai et al., 2014; Klee et al., 2019). Bird foraging flower buds will affect the survival of fruit trees. Salvianolic acid A is more abundant in peach buds where birds are prone to forage and may be a potential metabolite that attracts birds to forage (Zhang et al., 2019).
The study on mechanism of resistance to disease is important for breed improvement. Scanning electron microscopy (SEM), transcriptome, and metabolomics analysis revealed that exogenous application of NaH2PO42H2O (P) and abscisic acid (ABA) increased the expression of “Huangguan” pear (Pyrus bretschneideri × Pyrus pyrifolia) wax-related and calcium-regulated genes, as well as increased “Huangguan” pear resistance to brown spot disease during harvest and storage (Wang et al., 2021f). Widely targeted metabolomics analysis was used to analyze leaves of HLB-tolerant and -sensitive citrus (Citrus reticulata) varieties, covering primary and secondary metabolic pathways including carbohydrate metabolism, nucleotide metabolism, amino acid metabolism, energy metabolism, and biosynthesis of secondary metabolites. In which, aspartate and glutamate metabolism, purine metabolism, biosynthesis of plant hormones, and catabolic pathways were upregulated in the tolerant group. A total of 50 metabolites related to HLB tolerance were identified, and these metabolites were considered as potential markers for the identification of citrus HLB (Suh et al., 2021). It was found that phenylpropanes and their derivatives play an important role in citrus fruits to resistant Penicillium infection (Ballester et al., 2013). In addition, fruit trees also produce special metabolites to affect the microbial populations in the rhizosphere to help resist the invasion of pathogens (Padhi et al., 2019).
Improper fertilization and abnormal temperature are common abiotic stresses on fruit trees. Nitrogen fertilizer is often over-applied in apple (Malus × domestica Borkh) cultivation in China (Approx. 600–800 kg N ha–1). Although high nitrogen fertilizer application promoted apple yield and fruit weight per unit, at the same time, the carbon-to-nitrogen ratio, soluble sugars, flavonoids, and other fruit quality indicators decreased. The combined analysis of transcriptome, proteomics, and metabolomics revealed the global pattern of high nitrogen effect on apple fruits. High nitrogen significantly inhibited the accumulation of carbohydrates (sucrose, glucose, and alginose) and flavonoids (rutin, rhamnolipid-3-O-rutinoside, and trihydroxyisoflavone-7-O-galactoside) in the fruits. More carbon groups are used in the synthesis of amino acids (especially arginine) and their derivatives under high nitrogen conditions to enhance the nitrogen metabolism process (Wang et al., 2021a). Under low phosphorus stress, the content of flavonoids and anthocyanin in leaves increased, and the amino acid and their derivatives, organic acids, and flavonoids in roots increased. In contrast, under high phosphorus stress, a higher flavone content in the leaves and lower anthocyanins in the roots were found. Thus, apple seedlings respond to phosphorus stress by regulating the flavonoid pathway (Sun et al., 2021). Different concentrations of phosphate fertilizer were also found leading to changes in flavonoids in Chinese wolfberry (Lycium barbarum L.) (Wei et al., 2020).
The growth and development of fruit trees need light, temperature, air, water, and other non-nature environment. The change of environment will affect the physiology of fruit tree and quality of fruit. Metabolomics provides new method and new insight to reveal the mechanism of environmental regulation on fruit tree.
Light plays an important role in fruit growth. Bagging apple fruit reduces insect infestation, but it also reduces light exposure and increases the risk of browning. As found by Wang et al. (2021c), the bagging resulted in the production of 50 differential metabolites, in which a significant decrease in flavonoids was positively correlated with peel browning. In general, the inner fruit of turmeric trees received less light. The LC-MS and GC-MS analysis showed that sucrose and sorbitol contents were higher in the outer fruit, while glucose and malic acid contents were higher in the inner fruit (Rudell et al., 2017).
Persistent low temperature favors fruit trees to break dormancy, and changing temperature has an adverse effect on germination. Research of flower buds of pears treated at different temperatures showed that metabolites associated with the pentose phosphate pathway, energy production, and the tricarboxylic acid cycle (TCA) cycle may have reduced the germination rate of pear buds (Horikoshi et al., 2018). In contrast, [H2CN2 (HC)] breaking of blueberry dormancy is associated with increases in soluble sugars, organic acids, and amino acids (aspartate, glutamate, and phenylalanine) (Wang et al., 2021d). Treated with low temperature after harvesting leads to decrease of xylose, galactose, galacturonic acid, glucuronate, glycine, and rhamnose (R. Parijadi et al., 2019). Studies on Butia spp. (Arecaceae) growing at different latitude, longitude, and altitude in Brazil have found that fruits at lower altitude are sweeter, and fruits at higher altitude contain more carotenoids and phenols (Hoffmann et al., 2017).
Metabolomics was used to analyze the variation in fruit quality of four main Spanish grape cultivars RJ43, CL306, VN31, and 1084 under simulated climatic conditions [i.e., expected temperature, CO2 content, and relative humidity (RH)] and different irrigation patterns (i.e., water sufficiency/water deficit) for 2,100 years. The results indicate that CO2 levels in 2100 will increase sugar accumulation and reduce acidity in the grapes, but this effect is partially mitigated by insufficient water. In addition, climate change and moisture conditions significantly affected the concentration and intensity of amino acid accumulation in grapes, but these effects were different in different varieties. Besides, CO2 content and water deficiency combined to reduce anthocyanin and anthocyanin/total soluble solids ratio in grapes. In general, although global climate change and moisture conditions can have a significant impact on grape quality, that is limited by the grape varieties. For example, RJ43 and CL306 are more affected by global changes, while 1,084 is relatively less affected (Arrizabalaga-Arriazu et al., 2021).
In addition, the biological environment can also affect the physiological and biochemical characteristics of fruit trees. Banana fruits near natural forests (near-NF) are very different from that distant from natural forests (distant-NF) in color, ripening characteristics, and susceptibility. Mature banana fruits harvested in near-NF contain more organic acids, GBAB (a four-carbon non-proteinogenic amino acid) and unsaturated fatty acids, and these metabolites increase the flavor and nutritional composition of the banana, while also enhancing the ability of the banana fruit to cope with biotic and abiotic stresses. Fruits harvested in distant-NF contain more glutamate and putrescine, which makes bananas have an unpleasant smell after harvest and is also bad for storage (Nascimento et al., 2019).
Rootstocks and interstocks are generally used to dwarf fruit trees to achieve light and simplified management of the orchard. Comparison of the metabolome of pears grafted to different stocks revealed a significant increase in the concentrations of flavonoids and phenolic acids in scions grafted to dwarf stocks, along with significantly decreased concentrations of D-sorbitol and D-mannitol in the roots. It indicates that flavonoids and phenolic acids are key compounds involved in reducing scion growth and dwarf rootstock may control tree growth by regulating carbohydrate partitioning from shoot to roots (Cui et al., 2021). Training can also have effects on apple tree metabolism (D’Abrosca et al., 2017; Anthony et al., 2020). Girdling improves the quality of the sweet cherry fruit. The anthocyanin content in the peel varied significantly between pruning systems, and the slender spindle training system was the most favorable for fruit growth (Michailidis et al., 2020).
While the inheritance and variation of metabolites are controlled by smaller loci, traditional phenotypic traits are often located at larger loci. The addition of metabolomics can accelerate the research progress and candidate genes screening in fruit trees. By considering the metabolite levels as quantitative traits, the metabolic traits can be analyzed by QTL, i.e., metabolic QTL (mQTL) analysis (Carreno-Quintero et al., 2012). Combining metabolomics and GWAS, named mGWAS (Luo, 2015). Most of the mQTL work has been focused on Arabidopsis thaliana, S. Lycopersicum (Toubiana et al., 2015; Knoch et al., 2017), Triticum aestivum, Oryza sativa, and Zea mays (Gong et al., 2013; Jiang et al., 2013; Chen et al., 2021), and recently mQTL have also been applied in study the mechanism of fruit genetic basis.
To obtain hybrid plants with high-soluble solids content (SSC)/titratable acidity (TA) ratios in strawberries (Fragaria × ananassa), Vallarino et al. (2019) used GC-MS to detect primary metabolites in “232,” “1,392,” and their hybrid population F1 for 2 years. A total of 50 compounds were detected and successfully identified, including soluble sugars, organic acids, amino acids, soluble alcohols, etc. Pearson correlation analysis revealed possible synergistic regulation of fructose and glucose, glucose and fructose 6-phosphate, succinic acid and fumaric acid, and raffinose and succinic acid. The mQTL analysis was performed for these 50 major metabolites, SSC, TA, pH, and L-AA data using the constructed “232” × “1,392” linkage maps. The final results of 133 QTL localizations were obtained, and QTL for organic acids and sugars (glucuronide and succinate, raffinose and sucrose) were found in LG V-4. It was speculated that a common gene with pleiotropy for sucrose, raffinose, and succinate might cause these changes. The expression level of FvH4_5g03890 (glucose-6 -β 1-episomerase) gene was found to be negatively correlated with sucrose and raffinose contents by glucose biosynthesis, metabolism-related annotation and quantitative RT-PCR (Vallarino et al., 2019).
A hotspot of QTLs of phenolic compounds, including procyanidins and flavan-3-ols, in ripe apple fruits were mapped by mQTLs at the top of LG16 (Khan et al., 2012a). Higher expression of leucoanthocyanidin reductase gene (MdLAR1), which located on the LG16, was associated with a fourfold increase of procyanidin. Combining with expression analysis of structural and regulation genes of the phenylpropanoid and flavonoid pathways, MdLAR1 was identified the candidate gene for procyanidins and flavan-3-ols (Khan et al., 2012b).
A total of metabolic profiling of 101 F2-generation grape stems were analyzed by LC-HR MS, and 1,317 characteristic ions were detected. Among them, 19 were related to stilbene compounds, and 5 mQTLs were finally identified by QTL localization. Two large-effect mQTLs, corresponding to a stilbenoid dimer and a trimer, were jointly localized in the 25.0–27.8 Mbps of LG18. This range also included 48 genes of bio-resistance, 15 genes in flavonoid biosynthesis pathway, and 6 genes of triterpenoid biosynthesis. In addition, haplotype dosage effects of 3 mQTLs were statistically significant. Fruit trees with high quality and disease resistance can be screened simultaneously (Teh et al., 2019).
Two hundred and seven jujube varieties, including 25 wild and 182 cultivated jujubes (Ziziphus jujuba Mill.), were used to study domestication-driven changes of fruit bioactive molecules by a combination of genome-sequencing, widely targeted metabolomics, and mGWAS. Genome-sequencing revealed 109 domestication-relative sweeps, which mainly distributed on chromosomes 1, 4, and 10. A total of 2,985 metabolites were annotated by a metabolome library with various bioactive metabolites. In which, 187 metabolites were associated with domestication. Totally, 1,080 associated loci for 986 metabolites were identified by mGWAS. Among them, 15 triterpenoids, which were significantly reduced in cultivated jujube, existed at six major loci. Mutations in the promoter of an OSC gene associated with triterpenes content reduction during domestication. In addition, by comparing the metabolites of 24 dry and 31 fresh types of jujube, 203 significantly different metabolites were identified, of which 7 cyclopeptide alkaloids (CPA) were highly accumulated in the dry variety. An N-methyltransferase were identified the CPA candidate gene by divergence sweep identification and its genomic sequence variations were responsible for content of CPAs in 39 jujube accessions (Zhang et al., 2021).
In recent years, fruit tree metabolomics-related research has increased rapidly, revealing the mechanism of fruit quality formation, biotic/abiotic stress, and environmental influence on fruit tree. Alternatively, the obtained differential metabolites can be used as novel biomarkers for the identification of variety, origin, and pathogen infection. Metabolomics-transcriptomic combined analysis, further revealed the gene/metabolic regulatory network and candidate genes leading to trait. Metabolomics, combining with GWAS/QTL, narrows the range of candidate genes and increases the odds of acquiring key genes.
However, the development of metabolomics in fruit trees is still in its initial stage, and most studies are the method exploration and simple repetition of metabolomics application to fruit trees, obtaining differential metabolites but lacking in-depth mechanistic research. If the changes in the metabolome are combined with the changes in the organ, tissue, cellular, and molecular levels, the mechanism of trait changes should be even more systematically revealed. In addition, non-targeted metabolome is widely used in fruit tree metabolomics research, but only a few dozen compounds in thousands obtained can be identified, which not only leads to low metabolomics efficiency, but also the differential metabolites obtained may not real represent differences. The current metabolic library is mainly prepared for human, animal, and drug experiments and rarely covers the unique metabolites of fruit trees. The construction of metabolomics database of fruit tree will greatly promote the application of metabolomics in fruit tree. Widely targeted and pseudo-targeted metabolomes allow the quantitative and qualitative acquisition of thousands of compounds and are alternative solutions if the cost is reduced. Then, extreme metabolite diversity not only makes the fruit tree metabolomics study particularly required but also brings challenges to the various stages of metabolomics experiments, analysis, and library building. To further promote the application of metabolomics to fruit trees, the following problems or bottlenecks should be solved. Firstly, a more broad-spectrum and universal detection method should to be constructed. Secondly, a really unbiased, high-sensitive, and high-throughput metabolism analysis platform with the ability to parallel analyze huge amounts of data is needed. Thirdly, accurate and efficient identification or annotation of metabolites database or tools should be developed.
With the improvement of instruments and metabolite database and decrease of cost of experiment, metabolomics will prompt the fruit tree research to achieve more breakthrough results.
JL, ST, GY, XD, XH, and JW provided suggestions for the frame structure and content of the manuscript. JL wrote the first draft. ST assisted in the translation of the manuscript. GY and XD provided help in the arrangement of the table. KZ, GY, XD, XMZ, YZ, CW, and XZ contributed to the revision of the manuscript. All authors contributed to the article and approved the submitted version.
This study was supported by the Promotion and Innovation of Beijing Academy of Agriculture and Forestry Sciences (KJCX201910), the technological innovation ability project of Beijing Academy of Agriculture and Forestry Sciences (KJCX20210403), Heilongjiang Touyan Innovation Team Program (Tree Genetics and Breeding Innovation Team), the National Natural Science Foundation of China (32072536), and National Key R&D Program of China from the Ministry of Science and Technology of China (Grant No. 2019YFC1711100), Youth Research Fund of the Beijing Academy of Forestry and Fruit Sciences (LGYJJ202006 and LGYJJ202005).
The authors declare that the research was conducted in the absence of any commercial or financial relationships that could be construed as a potential conflict of interest.
All claims expressed in this article are solely those of the authors and do not necessarily represent those of their affiliated organizations, or those of the publisher, the editors and the reviewers. Any product that may be evaluated in this article, or claim that may be made by its manufacturer, is not guaranteed or endorsed by the publisher.
Anthony, B. M., Chaparro, J. M., Prenni, J. E., and Minas, I. S. (2020). Early metabolic priming under differing carbon sufficiency conditions influences 2 peach fruit quality development. Plant Physiol. Biochem. 157, 416–431. doi: 10.1016/j.plaphy.2020.11.004
Arrizabalaga-Arriazu, M., Gomès, E., Morales, F., Irigoyen, J. J., Pascual, I., and Hilbert, G. (2021). Impact of 2100-projected air temperature, carbon dioxide, and water scarcity on grape primary and secondary metabolites of different Vitis vinifera cv. Tempranillo Clones. J. Agric. Food Chem. 69, 6172–6185. doi: 10.1021/acs.jafc.1c01412
Baky, M. H., Badawy, M. T., Bakr, A. F., Hegazi, N. M., Abdellatif, A., and Farag, M. A. (2021). Metabolome-based profiling of African baobab fruit (Adansonia digitata L.) using a multiplex approach of MS and NMR techniques in relation to its biological activity. RSC Adv. 11, 39680–39695. doi: 10.1039/D1RA08277A
Ballester, A.-R., Lafuente, M. T., de Vos, R. C. H., Bovy, A. G., and González-Candelas, L. (2013). Citrus phenylpropanoids and defence against pathogens. Part I: metabolic profiling in elicited fruits. Food Chem. 136, 178–185. doi: 10.1016/j.foodchem.2012.07.114
Berni, R., Charton, S., Planchon, S., Legay, S., Romi, M., Cantini, C., et al. (2021). Molecular investigation of Tuscan sweet cherries sampled over three years: gene expression analysis coupled to metabolomics and proteomics. Hortic. Res. 8:12. doi: 10.1038/s41438-020-00445-3
Cajka, T., and Fiehn, O. (2016). Toward merging untargeted and targeted methods in mass spectrometry-based metabolomics and lipidomics. Anal. Chem. 88, 524–545. doi: 10.1021/acs.analchem.5b04491
Carreno-Quintero, N., Acharjee, A., Maliepaard, C., Bachern, C., Mumm, R., Bouwmeester, H., et al. (2012). Untargeted metabolic quantitative trait loci analyses reveal a relationship between primary metabolism and potato tuber quality. Plant Physiol. 158, 1306–1318. doi: 10.1104/pp.111.188441
Carreno-Quintero, N., Bouwmeester, H. J., and Keurentjes, J. J. B. (2013). Genetic analysis of metabolome–phenotype interactions: from model to crop species. Trends Genet. 29, 41–50. doi: 10.1016/j.tig.2012.09.006
Chen, J., Xue, M., Liu, H., Fernie, A. R., and Chen, W. (2021). Exploring the genic resources underlying metabolites through mGWAS and mQTL in wheat: from large-scale gene identification and pathway elucidation to crop improvement. Plant Commun. 2:100216. doi: 10.1016/j.xplc.2021.100216
Cui, Z., Zhang, H., Galarneau, E. R. -A., Yang, Y., Li, D., Song, J., et al. (2021). Metabolome analysis reveals important compounds related to dwarfing effect of interstock on scion in pear. Ann. Appl. Biol. 179, 108–122. doi: 10.1111/aab.12684
D’Abrosca, B., Scognamiglio, M., Corrado, L., Chiocchio, I., Zampella, L., Mastrobuoni, F., et al. (2017). Evaluation of different training systems on Annurca apple fruits revealed by agronomical, qualitative and NMR-based metabolomic approaches. Food Chem. 222, 18–27. doi: 10.1016/j.foodchem.2016.11.144
Dai, Z. W., Léon, C., Feil, R., Lunn, J. E., Delrot, S., and Gomès, E. (2013). Metabolic profiling reveals coordinated switches in primary carbohydrate metabolism in grape berry (Vitis vinifera L.), a non-climacteric fleshy fruit. J. Exp. Bot. 64, 1345–1355. doi: 10.1093/jxb/ers396
de Godoy, F., Bermúdez, L., Lira, B. S., de Souza, A. P., Elbl, P., Demarco, D., et al. (2013). Galacturonosyltransferase 4 silencing alters pectin composition and carbon partitioning in tomato. J. Exp. Bot. 64, 2449–2466. doi: 10.1093/jxb/ert106
Dickson, L., Tenon, M., Svilar, L., Fança-Berthon, P., Martin, J. C., Rogez, H., et al. (2020). Genipap (Genipa americana L.) juice intake biomarkers after medium-term consumption. Food Res. Int. 137:109375. doi: 10.1016/j.foodres.2020.109375
Dixon, R. A., and Strack, D. (2003). Phytochemistry meets genome analysis, and beyond. Phytochemistry 62, 815–816. doi: 10.1016/S0031-9422(02)00712-4
Dominguez, P. G., Conti, G., Duffy, T., Insani, M., Alseekh, S., Asurmendi, S., et al. (2021). Multiomics analyses reveal the roles of the ASR1 transcription factor in tomato fruits. J. Exp. Bot. 72, 6490–6509. doi: 10.1093/jxb/erab269
Fagbohun, O. F., Olawoye, B., Ademakinwa, A. N., Oriyomi, O. V., Fagbohun, O. S., Fadare, O. A., et al. (2021). UHPLC/GC-TOF-MS metabolomics, MTT assay, and molecular docking studies reveal physostigmine as a new anticancer agent from the ethyl acetate and butanol fractions of Kigelia africana (Lam.) Benth. fruit extracts. Biomed. Chromatogr. 35:e4979. doi: 10.1002/bmc.4979
Falasca, A., Melck, D., Paris, D., Saviano, G., Motta, A., and Iorizzi, M. (2014). Seasonal changes in the metabolic fingerprint of Juniperus communis L. berry extracts by 1H NMR-based metabolomics. Metabolomics 10, 165–174. doi: 10.1007/s11306-013-0566-1
Fayek, N. M., Farag, M. A., Abdel Monem, A. R., Moussa, M. Y., Abd-Elwahab, S. M., and El-Tanbouly, N. D. (2019). Comparative Metabolite Profiling of Four Citrus Peel Cultivars via ultra-performance liquid chromatography coupled with quadrupole-time-of-flight-mass spectrometry and multivariate data analyses. J. Chromatogr. Sci. 57, 349–360. doi: 10.1093/chromsci/bmz006
Fiehn, O., Kopka, J., Trethewey, R. N., and Willmitzer, L. (2000). Identification of uncommon plant metabolites based on calculation of elemental compositions using gas chromatography and quadrupole mass spectrometry. Anal. Chem. 72, 3573–3580. doi: 10.1021/ac991142i
Fuentealba, C. (2021). Cell wall and metabolite composition of sweet cherry fruits from two cultivars with contrasting susceptibility to surface pitting during storage. Food Chem. 342:128307. doi: 10.1016/j.foodchem.2020.128307
Gai, Y. P., Han, X. J., Li, Y. Q., Yuan, C. Z., Mo, Y. Y., Guo, F. Y., et al. (2014). Metabolomic analysis reveals the potential metabolites and pathogenesis involved in mulberry yellow dwarf disease: phytoplasma invasion impaired mulberry metabolism. Plant Cell Environ. 37, 1474–1490. doi: 10.1111/pce.12255
Gong, L., Chen, W., Gao, Y., Liu, X., Zhang, H., Xu, C., et al. (2013). Genetic analysis of the metabolome exemplified using a rice population. Proc. Natl. Acad. Sci. U.S.A. 110, 20320–20325. doi: 10.1073/pnas.1319681110
Hoffmann, J. F., Carvalho, I. R., Barbieri, R. L., Rombaldi, C. V., and Chaves, F. C. (2017). Butia spp. (Arecaceae) LC-MS-based metabolomics for species and geographical origin discrimination. J. Agric. Food Chem. 65, 523–532. doi: 10.1021/acs.jafc.6b03203
Hong, K., Chen, L., Gu, H., Zhang, X., Chen, J., Nile, S. H., et al. (2021). Novel insight into the relationship between metabolic profile and fatty acid accumulation altering cellular lipid content in pineapple fruits at different stages of maturity. J. Agric. Food Chem. 69, 8578–8589. doi: 10.1021/acs.jafc.1c02658
Horikoshi, H. M., Sekozawa, Y., Kobayashi, M., Saito, K., Kusano, M., and Sugaya, S. (2018). Metabolomics analysis of “Housui” Japanese pear flower buds during endodormancy reveals metabolic suppression by thermal fluctuation. Plant Physiol. Biochem. 126, 134–141. doi: 10.1016/j.plaphy.2018.02.028
Hurtado-Fernández, E., Pacchiarotta, T., Mayboroda, O. A., Fernández-Gutiérrez, A., and Carrasco-Pancorbo, A. (2015). Metabolomic analysis of avocado fruits by GC-APCI-TOF MS: effects of ripening degrees and fruit varieties. Anal. Bioanal. Chem. 407, 547–555. doi: 10.1007/s00216-014-8283-9
Ji, X., Ren, J., Lang, S., Wang, D., Zhu, L., and Song, X. (2020). Differential regulation of Anthocyanins in Cerasus humilis fruit color revealed by combined transcriptome and metabolome analysis. Forests 11:1065. doi: 10.3390/f11101065
Jiang, P. S., Zhang, H. X., Lü, X. L., Hao, Z. F., Li, B., Li, M. S., et al. (2013). Analysis of Meta-QTL and candidate genes related to yield components in maize. Acta Agron. Sin. 39:969. doi: 10.3724/SP.J.1006.2013.00969
Jing, S., and Malladi, A. (2020). Higher growth of the apple (Malus × domestica Borkh.) fruit cortex is supported by resource intensive metabolism during early development. BMC Plant Biol. 20:75. doi: 10.1186/s12870-020-2280-2
Karagiannis, E. (2018). Postharvest responses of sweet cherry fruit and stem tissues revealed by metabolomic profiling. Plant Physiol. Biochem. 127, 478–484. doi: 10.1016/j.plaphy.2018.04.029
Karagiannis, E., Sarrou, E., Michailidis, M., Tanou, G., Ganopoulos, I., Bazakos, C., et al. (2021). Fruit quality trait discovery and metabolic profiling in sweet cherry genebank collection in Greece. Food Chem. 342:128315. doi: 10.1016/j.foodchem.2020.128315
Khalil, M. N. A., Fekry, M. I., and Farag, M. A. (2017). Metabolome based volatiles profiling in 13 date palm fruit varieties from Egypt via SPME GC–MS and chemometrics. Food Chem. 217, 171–181. doi: 10.1016/j.foodchem.2016.08.089
Khan, S. A., Chibon, P. Y., de Vos, R. C. H., Schipper, B. A., Walraven, E., Beekwilder, J., et al. (2012a). Genetic analysis of metabolites in apple fruits indicates an mQTL hotspot for phenolic compounds on linkage group 16. J. Exp. Bot. 63, 2895–2908. doi: 10.1093/jxb/err464
Khan, S. A., Schaart, J. G., Beekwilder, J., Allan, A. C., Tikunov, Y. M., Jacobsen, E., et al. (2012b). The mQTL hotspot on linkage group 16 for phenolic compounds in apple fruits is probably the result of a leucoanthocyanidin reductase gene at that locus. BMC Res. Notes 5:618. doi: 10.1186/1756-0500-5-618
Kim, H. K., and Verpoorte, R. (2010). Sample preparation for plant metabolomics. Phytochem. Anal. 21, 4–13. doi: 10.1002/pca.1188
Klee, S. M., Sinn, J. P., Finley, M., Allman, E. L., Smith, P. B., Aimufua, O., et al. (2019). Erwinia amylovora auxotrophic mutant exometabolomics and virulence on apples. Appl. Environ. Microbiol. 85:e00935-19. doi: 10.1128/AEM.00935-19
Knoch, D., Riewe, D., Meyer, R. C., Boudichevskaia, A., Schmidt, R., and Altmann, T. (2017). Genetic dissection of metabolite variation in Arabidopsis seeds: evidence for mQTL hotspots and a master regulatory locus of seed metabolism. J. Exp. Bot. 68, 1655–1667. doi: 10.1093/jxb/erx049
Koch, K. (2004). Sucrose metabolism: regulatory mechanisms and pivotal roles in sugar sensing and plant development. Curr. Opin. Plant Biol. 7, 235–246. doi: 10.1016/j.pbi.2004.03.014
Lee, K.-M., Oh, T.-J., Kim, S.-H., Kim, H.-Y., Chung, H., Min, D. S., et al. (2016). Comprehensive metabolic profiles of mulberry fruit (Morus alba Linnaeus) according to maturation stage. Food Sci. Biotechnol. 25, 1035–1041. doi: 10.1007/s10068-016-0167-7
Lee, Y. R., Cho, H. M., Park, E. J., Zhang, M., Doan, T. P., Lee, B. W., et al. (2020). Metabolite Profiling of Rambutan (Nephelium lappaceum L.) seeds using UPLC-qTOF-MS/MS and senomorphic effects in aged human dermal fibroblasts. Nutrients 12:1430. doi: 10.3390/nu12051430
Li, B., Neumann, E. K., Ge, J., Gao, W., Hua, Y., Ping, L., et al. (2018). Interrogation of spatial metabolome of ginkgo biloba with High-resolution MALDI and LDI mass spectrometry imaging. Plant Cell Environ. 41, 2693–2703. doi: 10.1111/pce.13395
Lisec, J., Schauer, N., Kopka, J., Willmitzer, L., and Fernie, A. R. (2015). Gas chromatography mass spectrometry-based metabolite profiling in plants. Nat Protoc. 1, 387–396. doi: 10.1038/nprot.2006.59
Lu, X.-P., Li, F. F., Xiong, J., Cao, X. J., Ma, X. C., Zhang, Z. M., et al. (2017). Transcriptome and metabolome analyses provide insights into the occurrence of peel roughing disorder on Satsuma Mandarin (Citrus unshiu Marc.) Fruit. Front. Plant Sci. 8:1907. doi: 10.3389/fpls.2017.01907
Luo, J. (2015). Metabolite-based genome-wide association studies in plants. Curr. Opin. Plant Biol. 24, 31–38. doi: 10.1016/j.pbi.2015.01.006
Lwin, H. P., and Lee, J. (2020). Fruit quality and major metabolites in cold-stored ‘Wonhwang’ Asian pears are differentially affected by fruit size. J. Sci. Food Agric. 100, 5117–5125. doi: 10.1002/jsfa.10422
Ma, A., and Qi, X. (2021). Mining plant metabolomes: methods, applications, and perspectives. Plant Commun. 2:100238. doi: 10.1016/j.xplc.2021.100238
Mamat, S. F., Azizan, K. A., Baharum, S. N., Noor, N. M., and Aizat, W. M. (2020). GC-MS and LC-MS analyses reveal the distribution of primary and secondary metabolites in mangosteen (Garcinia mangostana Linn.) fruit during ripening. Sci. Hortic. 262:109004. doi: 10.1016/j.scienta.2019.109004
Michailidis, M., Karagiannis, E., Tanou, G., Samiotaki, M., Sarrou, E., Karamanoli, K., et al. (2020). Proteomic and metabolic analysis reveals novel sweet cherry fruit development regulatory points influenced by girdling. Plant Physiol. Bioch. 149, 233–244. doi: 10.1016/j.plaphy.2020.02.017
Montefiori, M., McGhie, T. K., Hallett, I. C., and Costa, G. (2009). Changes in pigments and plastid ultrastructure during ripening of green-fleshed and yellow-fleshed kiwifruit. Sci. Hortic. 119, 377–387. doi: 10.1016/j.scienta.2008.08.022
Muto, A., Müller, C. T., Bruno, L., McGregor, L., Ferrante, A., Chiappetta, A. A. C., et al. (2020). Fruit volatilome profiling through GC × GC-ToF-MS and gene expression analyses reveal differences amongst peach cultivars in their response to cold storage. Sci. Rep. 10:18333. doi: 10.1038/s41598-020-75322-z
Nascimento, T. P., Castro-Alves, V. C., Castelan, F. P., Calhau, M. F. N. S., Saraiva, L. A., Agopian, R. G., et al. (2019). Metabolomic profiling reveals that natural biodiversity surrounding a banana crop may positively influence the nutritional/sensorial profile of ripe fruits. Food Res. Int. 124, 165–174. doi: 10.1016/j.foodres.2018.07.050
Nicholson, J. K., Lindon, J. C., and Holmes, E. (1999). “Metabonomics”: understanding the metabolic responses of living systems to pathophysiological stimuli via multivariate statistical analysis of biological NMR spectroscopic data. Xenobiotica 29, 1181–1189. doi: 10.1080/004982599238047
Olmo-García, L., Kessler, N., Neuweger, H., Wendt, K., Olmo-Peinado, J., Fernández-Gutiérrez, A., et al. (2018). Unravelling the distribution of secondary metabolites in Olea europaea L.: exhaustive characterization of eight olive-tree derived matrices by complementary platforms (LC-ESI/APCI-MS and GC-APCI-MS). Molecules 23:2419. doi: 10.3390/molecules23102419
Padhi, E. M. T., Maharaj, N., Lin, S.-Y., Mishchuk, D. O., Chin, E., Godfrey, K., et al. (2019). Metabolome and microbiome signatures in the roots of citrus affected by huanglongbing. Phytopathology 109, 2022–2032. doi: 10.1094/PHYTO-03-19-0103-R
Park, S. E., Seo, S. H., Kim, E. J., Park, D. H., Park, K. M., Cho, S. S., et al. (2019). Metabolomic approach for discrimination of cultivation age and ripening stage in ginseng berry using gas chromatography-mass spectrometry. Molecules 24:3837. doi: 10.3390/molecules24213837
R. Parijadi, A. A., Ridwani, S., Dwivany, F. M., Putri, S. P., and Fukusaki, E. (2019). A metabolomics-based approach for the evaluation of off-tree ripening conditions and different postharvest treatments in mangosteen (Garcinia mangostana). Metabolomics 15:73. doi: 10.1007/s11306-019-1526-1
Ramadan, N. S., Wessjohann, L. A., Mocan, A., Vodnar, D. C., El-Sayed, N. H., El-Toumy, S. A., et al. (2020). Nutrient and sensory metabolites profiling of Averrhoa Carambola L. (Starfruit) in the context of its origin and ripening stage by GC/MS and chemometric analysis. Molecules 25:2423. doi: 10.3390/molecules25102423
Ramírez-Briones, E., Rodríguez Macías, R., Casarrubias Castillo, K., del Río, R. E., Martínez-Gallardo, N., Tiessen, A., et al. (2019). Fruits of wild and semi-domesticated Diospyros tree species have contrasting phenological, metabolic, and antioxidant activity profiles. J. Sci. Food Agric. 99, 6020–6031. doi: 10.1002/jsfa.9878
Risticevic, S., Souza-Silva, E. A., DeEll, J. R., Cochran, J., and Pawliszyn, J. (2016). Capturing plant metabolome with direct-immersion in vivo solid phase microextraction of plant tissues. Anal. Chem. 88, 1266–1274. doi: 10.1021/acs.analchem.5b03684
Risticevic, S., Souza-Silva, E. A., Gionfriddo, E., DeEll, J. R., Cochran, J., Hopkins, W. S., et al. (2020). Application of in vivo solid phase microextraction (SPME) in capturing metabolome of apple (Malus × domestica Borkh.) fruit. Sci. Rep. 10:6724. doi: 10.1038/s41598-020-63817-8
Rivera-Mondragón, A., Tuenter, E., Ortiz, O., Sakavitsi, M. E., Nikou, T., Halabalaki, M., et al. (2020). UPLC-MS/MS-based molecular networking and NMR structural determination for the untargeted phytochemical characterization of the fruit of Crescentia cujete (Bignoniaceae). Phytochemistry 177:112438. doi: 10.1016/j.phytochem.2020.112438
Rudell, D. R., and Mattheis, J. P. (2009). Superficial scald development and related metabolism is modified by postharvest light irradiation. Postharvest Biol. Technol. 51, 174–182. doi: 10.1016/j.postharvbio.2008.07.008
Rudell, D. R., Serra, S., Sullivan, N., Mattheis, J. P., and Musacchi, S. (2017). Survey of ‘d’Anjou’ pear metabolic profile following harvest from different canopy positions and fruit tissues. HortScience 52, 1501–1510. doi: 10.21273/HORTSCI12375-17
Ryu, S., Muramatsu, T., Furihata, K., Wei, F., Koda, M., Miyakawa, T., et al. (2019). NMR-based metabolic profiling and comparison of Japanese persimmon cultivars. Sci. Rep. 9:15011. doi: 10.1038/s41598-019-51489-y
Shaffer, R. E. (2002). Multi- and Megavariate Data Analysis. Principles and applications, I. Eriksson, E. Johansson, N. Kettaneh-Wold and S. Wold, Umetrics Academy, Ume, 2001, ISBN 91-973730-1-X, 533pp. J. Chemom. 16, 261–262. doi: 10.1002/cem.713
Smith, E. D., Whiting, M. D., and Rudell, D. R. (2011). Metabolic profiling of ethephon-treated sweet cherry (Prunus avium L.). Metabolomics 7, 126–133. doi: 10.1007/s11306-010-0238-3
Suh, J. H., Tang, X., Zhang, Y., Gmitter, F. G., and Wang, Y. (2021). Metabolomic analysis provides new insight into tolerance of Huanglongbing in Citrus. Front. Plant Sci. 12:710598. doi: 10.3389/fpls.2021.710598
Sumner, L. W., Mendes, P., and Dixon, R. A. (2003). Plant metabolomics: large-scale phytochemistry in the functional genomics era. Phytochemistry 62, 817–836. doi: 10.1016/S0031-9422(02)00708-2
Sun, T., Zhang, J., Zhang, Q., Li, X., Li, M., Yang, Y., et al. (2021). Transcriptome and metabolome analyses revealed the response mechanism of apple to different phosphorus stresses. Plant Physiol. Biochem. 167, 639–650. doi: 10.1016/j.plaphy.2021.08.040
Tang, N., Chen, N., Hu, N., Deng, W., Chen, Z., and Li, Z. (2018). Comparative metabolomics and transcriptomic profiling reveal the mechanism of fruit quality deterioration and the resistance of citrus fruit against Penicillium digitatum. Postharvest Biol. Technol. 145, 61–73. doi: 10.1016/j.postharvbio.2018.06.007
Teh, S. L., Rostandy, B., Awale, M., Luby, J. J., Fennell, A., and Hegeman, A. D. (2019). Genetic analysis of stilbenoid profiles in grapevine stems reveals a major mQTL hotspot on chromosome 18 associated with disease-resistance motifs. Hortic. Res. 6:121. doi: 10.1038/s41438-019-0203-x
Toubiana, D., Batushansky, A., Tzfadia, O., Scossa, F., Khan, A., Barak, S., et al. (2015). Combined correlation-based network and mQTL analyses efficiently identified loci for branched-chain amino acid, serine to threonine, and proline metabolism in tomato seeds. Plant J. 81, 121–133. doi: 10.1111/tpj.12717
Urbanczyk-Wochniak, E., Baxter, C., Kolbe, A., Kopka, J., Sweetlove, L. J., and Fernie, A. R. (2005). Profiling of diurnal patterns of metabolite and transcript abundance in potato (Solanum tuberosum) leaves. Planta 221, 891–903. doi: 10.1007/s00425-005-1483-y
Vallarino, J. G., Pott, D. M., Cruz-Rus, E., Miranda, L., Medina-Minguez, J. J., Valpuesta, V., et al. (2019). Identification of quantitative trait loci and candidate genes for primary metabolite content in strawberry fruit. Hortic. Res. 6:4. doi: 10.1038/s41438-018-0077-3
Villa-Ruano, N., Pérez-Hernández, N., Zepeda-Vallejo, L. G., Quiroz-Acosta, T., Mendieta-Moctezuma, A., Montoya-García, C., et al. (2019). 1 H-NMR based metabolomics profiling of citrus juices produced in veracruz, México. Chem. Biodivers. 16:e1800479. doi: 10.1002/cbdv.201800479
Vos, R., Moco, S., Lommen, A., Keurentjes, J. J., Bino, R. J., and Hall, R. D. (2007). Untargeted large-scale plant metabolomics using liquid chromatography coupled to mass spectrometry. Nat. Protoc. 2, 778–791. doi: 10.1038/nprot.2007.95
Wang, F., Huang, Y., Wu, W., Zhu, C., Zhang, R., Chen, J., et al. (2020). Metabolomics analysis of the peels of different colored citrus fruits (Citrus reticulata cv. ‘Shatangju’) during the maturation period based on UHPLC-QQQ-MS. Molecules 25:396. doi: 10.3390/molecules25020396
Wang, F., Ge, S., Xu, X., Xing, Y., Du, X., Zhang, X., et al. (2021a). Multiomics analysis reveals new insights into the apple fruit quality decline under high nitrogen conditions. J. Agric. Food Chem. 69, 5559–5572. doi: 10.1021/acs.jafc.1c01548
Wang, H., Asker, K., Zhan, C., and Wang, N. (2021b). Transcriptomic and metabolic analysis of fruit development and identification of genes involved in raffinose and hydrolysable tannin biosynthesis in walnuts. J. Agric. Food Chem. 69, 8050–8062. doi: 10.1021/acs.jafc.1c02434
Wang, H., Wang, S., Fan, M. M., Zhang, S. H., Sun, L. L., and Zhao, Z. Y. (2021c). Metabolomic insights into the browning of the peel of bagging ‘Rui Xue’ apple fruit. BMC Plant Biol. 21:209. doi: 10.1186/s12870-021-02974-y
Wang, H., Xia, X., and An, L. (2021d). Metabolomics analysis reveals the mechanism of hydrogen cyanamide in promoting flower bud break in blueberry. Agronomy 11:102. doi: 10.3390/agronomy11010102
Wang, J., Yang, E., Chaurand, P., and Raghavan, V. (2021e). Visualizing the distribution of strawberry plant metabolites at different maturity stages by MALDI-TOF imaging mass spectrometry. Food Chem. 345:128838. doi: 10.1016/j.foodchem.2020.128838
Wang, Q., Wu, X., Liu, L., Yao, D., Li, J., Fang, J., et al. (2021f). Transcriptome and metabolomic analysis to reveal the browning spot formation of ‘Huangguan’ pear. BMC Plant Biol. 21:321. doi: 10.1186/s12870-021-03049-8
Wang, R., Shu, P., Zhang, C., Zhang, J., Chen, Y., Zhang, Y., et al. (2022). Integrative analyses of metabolome and genome-wide transcriptome reveal the regulatory network governing flavor formation in kiwifruit (Actinidia chinensis). New Phytol. 233, 373–389. doi: 10.1111/nph.17618
Wang, S., Tu, H., Wan, J., Chen, W., Liu, X., Luo, J., et al. (2016). Spatio-temporal distribution and natural variation of metabolites in citrus fruits. Food Chem. 199, 8–17. doi: 10.1016/j.foodchem.2015.11.113
Wang, Z., Cui, Y., Vainstein, A., Chen, S., and Ma, H. (2017). Regulation of Fig (Ficus carica L.) Fruit Color: metabolomic and transcriptomic analyses of the flavonoid biosynthetic pathway. Front. Plant Sci. 8:1990. doi: 10.3389/fpls.2017.01990
Wei, C., Liang, G., Guo, Z., Wang, W., Zhang, H., Liu, X., et al. (2013). A novel integrated method for large-scale detection, identification, and quantification of widely targeted metabolites: application in the study of rice metabolomics. Mol. Plant 6, 1769–1780. doi: 10.1093/mp/sst080
Wei, F., Shi, Z., Wan, R., Li, Y., Wang, Y., An, W., et al. (2020). Impact of phosphorus fertilizer level on the yield and metabolome of goji fruit. Sci. Rep. 10:14656. doi: 10.1038/s41598-020-71492-y
Wu, T., Hu, D., and Wang, Q. (2021). Noni fruit’s water spot appearance on the second day of harvest: a trade-off between resistance and energy. Chem. Biol. Technol. Agric. 8:8. doi: 10.1186/s40538-020-00207-2
Wu, T., Li, M., and Lan, Z. (2019). Reveal the variation patterns of chemical composition in the fruit of Morinda citrifolia (noni) during postharvest storage through metabolomic characterization. Trop. Plant Biol. 12, 85–97. doi: 10.1007/s12042-019-09222-6
Xu, J., Yan, J., Li, W., Wang, Q., Wang, C., Guo, J., et al. (2020). Integrative analyses of widely targeted metabolic profiling and transcriptome data reveals molecular insight into metabolomic variations during apple (Malus domestica) fruit development and ripening. Int. J. Mol. Sci. 21:4797. doi: 10.3390/ijms21134797
Yazdanpanah, P., Jonoubi, P., Zeinalabedini, M., Rajaei, H., Ghaffari, M. R., Vazifeshenas, M. R., et al. (2021). Seasonal metabolic investigation in pomegranate (Punica granatum L.) highlights the role of amino acids in genotype- and organ-specific adaptive responses to freezing stress. Front. Plant Sci. 12:699139. doi: 10.3389/fpls.2021.699139
Yi, D., Zhang, H., Lai, B., Liu, L., Pan, X., Ma, Z., et al. (2021). Integrative analysis of the coloring mechanism of red longan pericarp through metabolome and transcriptome analyses. J. Agric. Food Chem. 69, 1806–1815. doi: 10.1021/acs.jafc.0c05023
Yost, R. A., and Enke, C. G. (1978). Selected ion fragmentation with a tandem quadrupole mass spectrometer. J. Am. Chem. Soc. 100, 2274–2275. doi: 10.1021/ja00475a072
Zhang, J., Wang, X., Yu, O., Tang, J., Gu, X., Wan, X., et al. (2011). Metabolic profiling of strawberry (Fragaria × ananassa Duch.) during fruit development and maturation. J. Exp. Bot. 62, 1103–1118. doi: 10.1093/jxb/erq343
Zhang, Q., Wang, L., Liu, Z., Zhao, Z., Zhao, J., Wang, Z., et al. (2020). Transcriptome and metabolome profiling unveil the mechanisms of Ziziphus jujuba Mill. peel coloration. Food Chem. 312:125903. doi: 10.1016/j.foodchem.2019.125903
Zhang, S., Ying, H., Pingcuo, G., Wang, S., Zhao, F., Cui, Y., et al. (2019). Identification of potential metabolites mediating bird’s selective feeding on Prunus mira flowers. BioMed. Res. Int. 2019:1395480. doi: 10.1155/2019/1395480
Zhang, Z., Shi, Q., Wang, B., Ma, A., Wang, Y., Xue, Q., et al. (2021). Jujube metabolome selection determined the edible properties acquired during domestication. Plant J. 109, 1116–1133. doi: 10.1111/tpj.15617
Zheng, F., Zhao, X., Zeng, Z., Wang, L., Lv, W., Wang, Q., et al. (2020). Development of a plasma pseudotargeted metabolomics method based on ultra-high-performance liquid chromatography–mass spectrometry. Nat. Protoc. 15, 2519–2537. doi: 10.1038/s41596-020-0341-5
Keywords: fruit tree, metabolomics, quality, resistance, mQTL, mGWAS
Citation: Li J, Yan G, Duan X, Zhang K, Zhang X, Zhou Y, Wu C, Zhang X, Tan S, Hua X and Wang J (2022) Research Progress and Trends in Metabolomics of Fruit Trees. Front. Plant Sci. 13:881856. doi: 10.3389/fpls.2022.881856
Received: 23 February 2022; Accepted: 01 April 2022;
Published: 29 April 2022.
Edited by:
Fangyuan Zhang, Southwest University, ChinaReviewed by:
Ge Bai, Yunnan Academy of Tobacco Agricultural Sciences, ChinaCopyright © 2022 Li, Yan, Duan, Zhang, Zhang, Zhou, Wu, Zhang, Tan, Hua and Wang. This is an open-access article distributed under the terms of the Creative Commons Attribution License (CC BY). The use, distribution or reproduction in other forums is permitted, provided the original author(s) and the copyright owner(s) are credited and that the original publication in this journal is cited, in accordance with accepted academic practice. No use, distribution or reproduction is permitted which does not comply with these terms.
*Correspondence: Shengnan Tan, c2hlbmduYW50YW5AMTI2LmNvbQ==; Xin Hua, aHVheGluQG5lZnUuZWR1LmNu; Jing Wang, c2R1d2pAMTI2LmNvbQ==
†These authors share first authorship
Disclaimer: All claims expressed in this article are solely those of the authors and do not necessarily represent those of their affiliated organizations, or those of the publisher, the editors and the reviewers. Any product that may be evaluated in this article or claim that may be made by its manufacturer is not guaranteed or endorsed by the publisher.
Research integrity at Frontiers
Learn more about the work of our research integrity team to safeguard the quality of each article we publish.