- 1Biological Systems Engineering, University of Wisconsin-Madison, Madison, WI, United States
- 2Key Laboratory of Spectral Imaging Technology, Xi’an Institute of Optics and Precision Mechanics, Xi’an, China
- 3School of Statistics and Data Science, Nankai University, Tianjin, China
Potato is one of the most significant food crops globally due to its essential role in the human diet. The growing demand for potato, coupled with severe environmental losses caused by extensive farming activities, implies the need for better crop protection and management practices. Precision agriculture is being well recognized as the solution as it deals with the management of spatial and temporal variability to improve agricultural returns and reduce environmental impact. As the initial step in precision agriculture, the traditional methods of crop and field characterization require a large input in labor, time, and cost. Recent developments in remote sensing technologies have facilitated the process of monitoring crops and quantifying field variations. Successful applications have been witnessed in the area of precision potato farming. Thus, this review reports the current knowledge on the applications of remote sensing technologies in precision potato trait characterization. We reviewed the commonly used imaging sensors and remote sensing platforms with the comparisons of their strengths and limitations and summarized the main applications of the remote sensing technologies in potato. As a result, this review could update potato agronomists and farmers with the latest approaches and research outcomes, as well as provide a selective list for those who have the intentions to apply remote sensing technologies to characterize potato traits for precision agriculture.
Introduction
Potato (Solanum tuberosum L.) is one of the most essential and commonly consumed non-grain food in the world. Due to its importance in the food industry supply chain, potato has become a dominant crop in many countries (Ortiz and Mares, 2017). For example, more than 23 million tons of potato tubers were harvested from about 940 thousand acres in the United States in 2019 (U. S. Department of Agriculture, 2020). Also, recently, the planting area and the total yield of potatoes have increased more than those of any other food crop in Africa. However, the continuously enhanced use of agricultural inputs (e.g., fertilizer and pesticide) could lead an environmental degradation, including the depletion of natural resources, polluted surface water and underground flows, and eutrophication (Kleinman et al., 2011; Konikow, 2015; Sishodia et al., 2017). Moreover, the over and/or inefficient use of water, fertilizers, pesticides, and herbicides for potato crops over the growing season causes economic losses and negative impacts on the environment (Hendricks et al., 2019). To constantly and sustainably meet the increasing needs for potato production, reducing environmental losses with improved potato productivity is the key topic in precision agricultural activities for potatoes (Delgado et al., 2019).
Traditionally, the effects of precision field activities, such as irrigation timing and frequency, fertilizer applications, planting dates, and population density, were determined through field surveying and/or biophysical modeling on crop growth and yield under different environmental conditions (Molahlehi et al., 2013; Al-Gaadi et al., 2016). Such methods commonly require visual examinations and destructive sampling and thus can be time-consuming with poor scalability. In the past few decades, the development of technologies, such as remote sensing, global positioning system, geographic information systems, artificial intelligence, has significantly promoted the applications in precision agriculture for enhancing crop production and quality while reducing the negative impacts on the environment in a more efficient way (Mulla, 2013). Precision agriculture has been gradually applied to monitor the crop growth status, generate auto-irrigation systems and disease detection systems in both developed and developing countries. Based on the interaction of electromagnetic radiation with soil and canopy reflectance, the remote sensing technique is considered as an effective tool in precision management for optimizing the decision-making process, which helps to improve profitable tuber yield and quality and minimize the negative impacts on the natural resources (Banerjee et al., 2020; Sanchez et al., 2020; Sun et al., 2020). Also, the data processing approaches, such as machine learning and image processing, have been widely used for extracting useful information from remotely sensed data (Dutta Gupta and Pattanayak, 2017; Oppenheim and Shani, 2017; Suh et al., 2018). Besides, cloud computing systems have been successfully used in data storing and processing for precision agriculture (Zhou et al., 2016b). Remote sensing systems, integrating advances in imaging, data processing, and computing technologies, thus have the potential to monitor the crop growth status and help make the decisions for crop management (Pavón-Pulido et al., 2017; Say et al., 2017).
In recent years, information-based precision agriculture has been successfully applied to explore potato crop traits and field phenotyping (Fortin et al., 2011; Sugiura et al., 2016; Franceschini et al., 2017a; Pei et al., 2019). Potatoes are stem tubers that are concealed in soils over the growing season. After being seeded in the soil, the top sides of the tuber produce shoot that grow into typical stems and leaves, and the undersides produce roots (Khayatnezhad et al., 2011). By establishing the relationship between traits of the aboveground parts and the underground tubers, remote sensing techniques have great potential in estimating the tuber growth conditions and predicting tuber yield prior to harvest (Shayanowako et al., 2014). Compared with traditional sampling methods, remote sensing is capable of providing a non-destructive and efficient way to collect the multi-temporal reflectance data of the aboveground plants for monitoring the biomarkers of potato crops (Nikolakopoulos et al., 2017; Feng et al., 2020). Past studies have focused on the specific applications of potatoes in precision agriculture using remote sensing approaches, such as tuber yield prediction (Akhand et al., 2016; Zaeen et al., 2020), above-ground biomass (AGB) estimation (Li B. et al., 2020; ten Harkel et al., 2020), water stress detection (Rud et al., 2014), nitrogen stress assessment (Bohman et al., 2019), and disease detection (Nebiker et al., 2016; Franceschini et al., 2017b,2019; Polder et al., 2019). Most of these studies demonstrated the strengths of remote sensing techniques along with their limitations and future challenges for the applications in potato production.
The primary purpose of this review is to provide a comprehensive background and knowledge on applications of remote sensing technologies in potato trait characterization in the context of precision agriculture. Both Web of Science and Google Scholar databases were used for literature review with keywords or topics of potato remote sensing from 2010 to the present. This paper is organized in the following main aspects: (1) compare the strengths and limitations of a variety of imaging sensors and remote sensing platforms and (2) summarize the remote sensing techniques adopted for the specific applications of potatoes in precision agriculture.
Imaging sensors
Remote sensing for crop trait characterization broadly involves a series of techniques in sensing, recording, analyzing, and/or modeling. When incident radiation (light source or natural light) hits plant surface, the radiation will either be absorbed, transmitted, or reflected. The reflected radiation is expressed as the electromagnetic spectrum with a range of wavelengths. The sensing technologies include developing devices to capture the reflected spectrum and convert them into readable signals for further analysis (Xie and Yang, 2020).
When choosing sensors for a specific purpose, image temporal, spatial, and spectral resolutions are the three main factors to consider. Temporal resolution refers to the time window between two images over the same place. Spatial resolution refers to the size of the smallest objects that can be captured and displayed in the imagery. Spectral resolution refers to the ability of a sensor to measure specific wavelengths of the electromagnetic spectrum and it is mainly decided by the sensor types.
Within the context of potato trait characterization, a variety of sensors has been developed and applied for different purposes. The sensors, varying in their spatial and temporal resolutions, mainly include visible Red-Green-Blue (RGB) cameras, multi- and hyper-spectral cameras, thermal infrared (TIR) cameras, and light detection and ranging (LiDAR) sensors. In this section, we summarized the sensors that have been applied in characterizing potato traits with comparisons of their strengths and limitations.
Visible red-green-blue cameras
Visible RGB cameras receive lights in red (564–580 nm), green (534–545 nm), and blue (420–440 nm) board spectral bands in the visible spectrum regions to emulate human vision. Due to the broad applicability of RGB cameras in all the fields, they are well-developed products by private sectors with a large selective range in price, sensor sizes, lens availability, data storage, and transmission. For characterizing crop traits, the RGB cameras generally have the best performance in providing high spatial-resolution images with the ability to be associated with various sensing environments, which makes them the most commonly adopted sensors in the areas of remote sensing.
Applications in potato trait characterization have been leveraging the advantages of RGB images in different ways. In potato, studies have been conducted on quantifying disease severity using the RGB images by detecting pixels of diseased leaves with that of healthy leaves and soil. It was shown that the diseased leaves could be clearly distinguished from the transformed color spaces (Sugiura et al., 2016) or spectral indices (Gibson-Poole et al., 2017; Siebring et al., 2019) from the RGB color space. Also due to the contrast between plant and soil background captured by the RGB image, promising applications have been seen in detecting potato emergence (Gibson-Poole et al., 2018; Li et al., 2019) and calculating ground vegetation coverage (Gibson-Poole et al., 2018). Other than the color-based features, high resolution images from the RGB cameras can be used to reconstruct three-dimensional (3D) dense point models using photogrammetry methods. Plant height is then obtained by subtracting the digital elevation model (DEM) which represents the absolute elevation of the bare ground from the digital surface model (DSM) which represents the absolute height of the plant canopies. Li B. et al. (2020) compared the performance in estimating potato plant height from the RGB 3D models with the plant height regressed from the hyperspectral reflectance and the results showed that the RGB 3D models lead to a higher correlation with the ground truth data [Coefficient of determination R2 = 0.93, root mean square error (RMSE) = 6.39 cm] than the full spectra regression (R2 = 0.85, RMSE = 7.24 cm).
Compared with other sensors, the RGB cameras provide an intuitive way to visualize and quantify crop traits. RGB cameras are typically low-cost, lightweight, and can be easily mounted on various platforms for data collection. However, three spectral bands and the broad bandwidth of the RGB cameras limit their ability to receive crop traits other than human perceptions and consequently their applications in sensing more complicated crop traits, such as yield and nutrient deficit. Besides, it requires extra efforts to remove background noises from the RGB images under certain situations when the plants have similar visual colors to the background or when plants are covered with shadows and other plants (Li et al., 2014).
Multi- and hyper-spectral cameras
Multi- and hyper-spectral cameras provide information with higher spectral resolutions than the RGB cameras and spectral bands additional to the visible range. The multispectral cameras usually consist of a small number (less than 10) of discrete wavebands in the visible-near infrared range from 400 to 1,000 nm. The spectral wavebands usually are narrower RGB bands and those with prior knowledge of their sensitivity in receiving important crop information (Hunt et al., 2013), such as red edge (700–730 nm) and NIR (760–900 nm). There are two types of multispectral cameras currently used in precision agriculture, that is, customized and commercial off-the-shelf cameras. As suggested by the name, the customized camera has the internal NIR filter removed from a visible RGB camera and is then replaced with an external filter to alter the camera spectral sensitivity (Zhou et al., 2016a), for example, from R-G-B to R-G-NIR. The commercial multispectral cameras are explicitly made with an interference filter to block or transmit specific lights (Xie and Yang, 2020). Compared with the customized camera, the commercial ones can provide more than three wavebands with other selectable spectral regions, but with lower spatial resolutions (Table 1).
By integrating both spectroscopic and imaging techniques into one system, hyperspectral cameras provide 3D hyperspectral cubes that contain both contiguous spectral information and two-dimensional spatial information. There are four types of hyperspectral cameras based on their image acquisition methods, namely point scanning, line scanning, area scanning, and snapshot cameras (Wu and Sun, 2013). The line scanning, also known as the pushbroom cameras, records a line of an image at each time and then forms the whole image by scanning along the other direction. The line scanning cameras are particularly suitable for being carried on imaging platforms that follow certain moving patterns (e.g., zigzag paths) to cover a large field and thus are the most popular ones in precision agricultural applications (Xie and Yang, 2020). Compared with the multispectral camera, hyperspectral cameras provide a real spectrum in every image pixel, allowing to calculate advanced vegetation indices (VIs) and estimate unperceived crop phenotypes. However, hyperspectral cameras have more restrictions on reflectance calibration procedures and moving patterns and they are more costly both in the devices and data storage (Li et al., 2014).
The spectral cameras have been widely used to predict potato leave biomass (Li B. et al., 2020; Peng et al., 2021)/tube yield (Sankaran et al., 2015; Tanabe et al., 2019; Li B. et al., 2020; Sun et al., 2020), detect canopy damage/disease (Nebiker et al., 2016; Hunt and Rondon, 2017; Duarte-Carvajalino et al., 2018; Franceschini et al., 2019), and estimate potato biophysical properties, such as chlorophyll content (Elarab et al., 2015; Franceschini et al., 2017a; Li C. et al., 2020), nitrogen concentrations (Nigon et al., 2014, 2015; Hunt and Rondon, 2017; Hunt et al., 2018), and leaf area index (LAI, Franceschini et al., 2017a). Among them, disease detection is the common application of visible RGB and spectral cameras. Unlike separating the healthy and unhealthy leaf areas in RGB images, spectral cameras are used to investigate the relationship between spectral measurements and disease severity at plot/field level. For example, the normalized difference vegetation index (NDVI) combined with spectral reflectance values in NIR and R was able to highlight sites infested by blight disease in a large potato field (Nebiker et al., 2016). Atherton et al. (2015) also reported that heavily diseased plants had significantly different spectral profiles from healthy plants.
Thermal infrared cameras
Radiation emitted by objects in the longwave infrared range (8–1.3 μm) is proportional to their surface temperature, which can be exploited for remote measurements of plant canopy temperature using TIR cameras (Kuenzer and Dech, 2013; Williams et al., 2018). Plant canopy temperature has been used as an indicator of plant stomatal traits (e.g., stomatal conductance, stomatal aperture, or leaf porosity) because leaves surfaces are cooled by evaporation, and stomatal opening or higher transpiration rates lead to high evaporation rates (i.e., decreased temperatures; Deery et al., 2019). In plant science and agronomy, measuring plant leaf temperature using thermal imaging has long been used to study the mechanisms behind plant water relations (Chaerle and Van Der Straeten, 2000), plant physiology processes (Jones et al., 2002; Aldea et al., 2006a; Grant et al., 2010), plant responses to pathogens (Chaerle et al., 1999) or environmental stressors (Jones et al., 2009), and plant photosynthetic rate (Aldea et al., 2006b; Vítek et al., 2020). In the area of precision agriculture, substantial interests have been witnessed in irrigation scheduling (Li et al., 2022), yield prediction (Bellis et al., 2022), evapotranspiration estimation (Lu et al., 2022; Wolff et al., 2022), pre-symptomatic diagnosis (Cohen et al., 2022), and stress tolerance (Zhou et al., 2020; Degani et al., 2022; Rippa et al., 2022).
In potato applications, TIR cameras are primarily used to evaluate potato water status. Plant water stress indicators, for example the Crop water stress index (CWSI), were calculated using TIR image-derived temperature and validated by comparing with biophysical measurements of plant water status. Related studies showed that the TIR image-based indices highly agreed with the ground measurements and were able to reflect the effects of different irrigation treatments (Rud et al., 2014; Gerhards et al., 2016; Cucho-Padin et al., 2020; Elsayed et al., 2021a). In some other applications, the TIR cameras were used as an aid tool for spectral cameras for detecting pest stress (Théau et al., 2020) or assessing chlorophyll content (Elarab et al., 2015).
The TIR cameras are the most effective tool in remotely sensing plant leaf/canopy temperature and water-related status. In practical, pre-calibration and post-correction procedures are required for receiving reliable temperature readings from the TIR cameras especially under changing ambient environmental factors such as air temperature, humidity, and wind speed (Jones et al., 2009; Jin et al., 2021). Additionally, the low spatial resolutions of the TIR cameras (Table 1) make them easily affected by image background temperature. It is also reported by Théau et al. (2020) that spectral indices (including visible ones) would be preferred over the TIR indices when both performed similarly in detecting various plant characteristics.
Light detection and ranging system
Light detection and ranging (or laser scanner) sensors are used to obtain depth information of targeted objects. A LiDAR device has a single-beam laser (narrowband in 600–1,000 nm) that emits a light pulse and a receiver measures the propagation time of the light pulse from emission to reflection. The time is then converted into a distance given the known speed of light (ten Harkel et al., 2020). LiDAR devices are lightweight with compact sizes and thus can be easily integrated on different platforms. Unmanned aerial vehicle (UAV)-based LiDAR systems have been applied to map the 3D structure of the plant canopy and topography of the landscape (Lin et al., 2019; ten Harkel et al., 2020). UAV-based LiDAR devices were reported to deliver accurate estimations of plant height with the RMSE of 0.02, 0.07, and 0.12 m compared with the manual measurements on snap bean crops (Zhang et al., 2021), sugar beet, and potato (ten Harkel et al., 2020), respectively. However, LiDAR devices can be expensive and require longer imaging times, compared to other stereo vision methods from images for 3D mapping.
Remote sensing platforms for data collection
In precision agriculture, a variety of remote sensing platforms have been developed and applied for different purposes, mainly including ground and aerial platforms. The ground platforms provide higher spatial and temporal resolution imagery than the aerial platforms and generally do not require a specific certificate to operate. Depending on the structural differences, four types of ground platforms are often seen, namely pole-based, cable-suspended, tractor, and gantry platforms (Li et al., 2021b). However, due to the limited spatial coverage, vibrations, and causes of soil compaction, ground platforms are challenged to be used in large-scale applications (Sankaran et al., 2015), such as potato precision management. Thus in this section, we focused on the aerial platforms that have been largely applied in precision potato management, that is, satellite-based and UAV-based platforms, with comparisons of their strengths and limitations.
Satellite-based remote sensing platforms
Satellite imagery, as one of the remote sensing means, began more than half a century ago and has been used efficiently in multiple agricultural applications (Ma et al., 2021; Wang Y. et al., 2021). Its major benefits include non-destructive capture of the earth surface at wide geographical regions (hundreds of square kilometers in a single image), selectable revisit time (1 day–2 weeks), and sensors with high spatial and spectral resolutions (Zhang et al., 2020). In recent years, a number of studies have investigated the potential of using satellite-based remote sensing platforms for precision potato management activities. The specifications for eleven representative platforms and their corresponding applications in precision potato management have been summarized in Table 2.
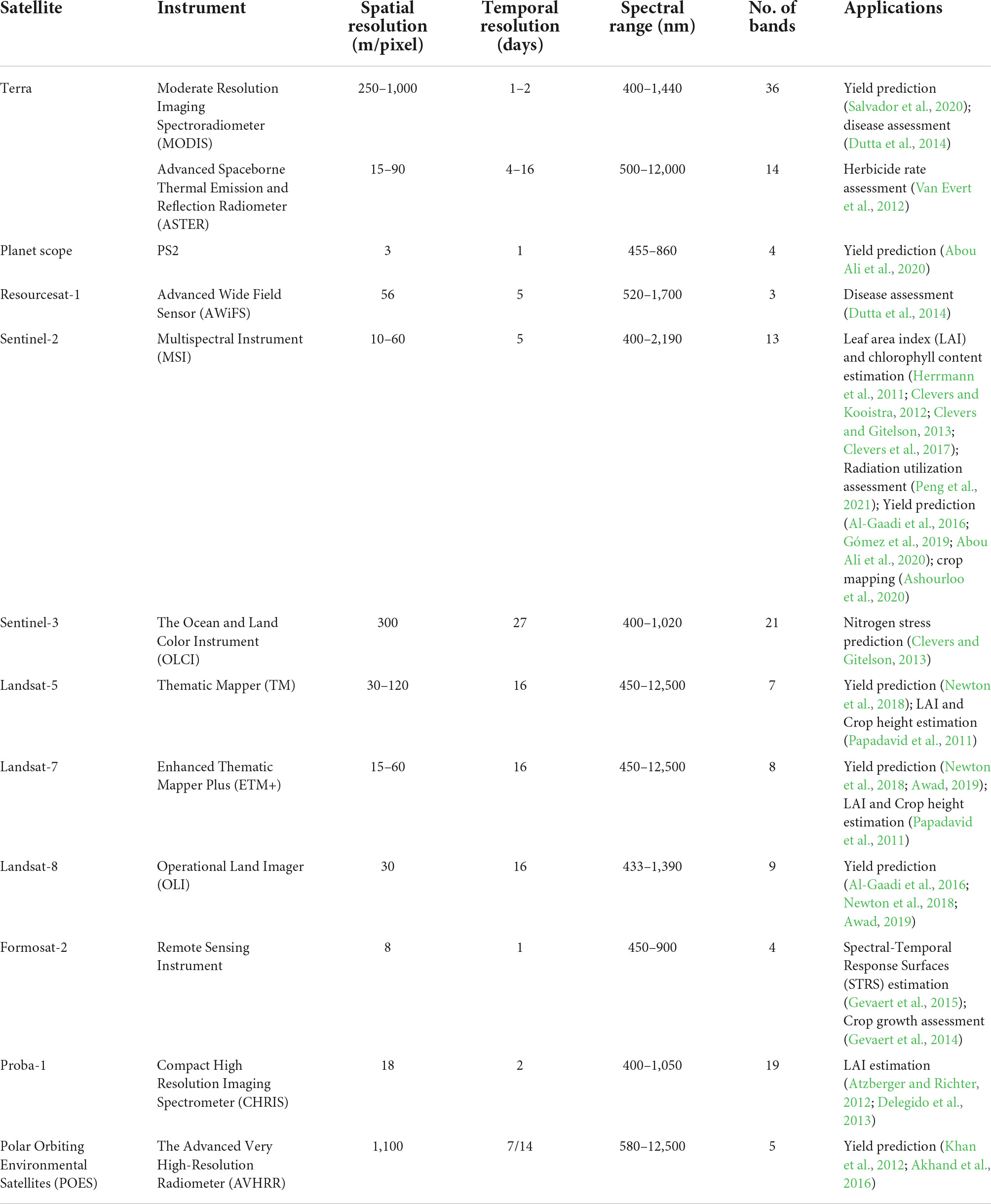
Table 2. Satellite-based remote sensing platforms and their applications in potato trait characterization.
Among all the selected satellite missions, the Landsat program involves a series of satellites that have been successfully used in agricultural monitoring because of the availability of historical data of the Earth’s surface for over 40 years. The most recent Landsat-8 was launched in 2013 with two imagers (Operational Land Imager and Thermal Infrared Sensor) that provide spectral observations in nine spectral bands at a 30-m spatial resolution. Several studies thus adapted the high-resolution observations from Landsat-8 for potato yield prediction (Al-Gaadi et al., 2016; Newton et al., 2018; Awad, 2019).
Besides the Landsat missions, Sentinel-2 is another popular platform for agriculture monitoring. For the potato management, Sentinel-2 imagery has been used for LAI and chlorophyll content estimation (Herrmann et al., 2011; Clevers and Kooistra, 2012; Clevers and Gitelson, 2013; Clevers et al., 2017), radiation utilization assessment (Peng et al., 2021), yield prediction (Al-Gaadi et al., 2016; Gómez et al., 2019; Abou Ali et al., 2020), and crop mapping (Ashourloo et al., 2020). Launched in 2015 by Copernicus Programme, Sentinel-2 systematically acquires optical imagery with global coverage. It is equipped with a push-broom multi-spectral instrument (MSI) which provides optical observations at 13 spectral bands ranging from visible (VIS) to short-wave infrared (SWIR) at high spatial resolutions (10–60 m/pixel). Like Landsat-8, Sentinel-2 also provides a global coverage but with a much higher temporal resolution (Table 1), which makes Sentinel-2 the most widely used satellite platform in potato precision management.
Other satellite platforms with unique specifications in one of the resolutions have been used to address specific purposes in potato management. For example, Terra Moderate Resolution Imaging Spectroradiometer has the highest spectral resolution of 36 bands in the range between 400 and 1,440 nm and thus the benefits for detecting the abnormal reflectance from leaves in the narrow bands for potato disease assessment (Dutta et al., 2014). Planet Scope provides images with the highest spatial resolution (3 m/pixel) every day through a constellation of about 130 low-orbit satellites. Though costly (∼$ 218 per 100 km2) compared to the publicly launched satellites, its imagery has been used as a good source for the decision-making at field-level and plot-level potato management on a daily basis (Abou Ali et al., 2020).
Unmanned aerial vehicle-based remote sensing platforms
Over the decades, UAV-based platforms have been a popular approach in precision agriculture. UAVs refer to devices with flying capacity without humans onboard (Eisenbeiss, 2004; Sankaran et al., 2015). Other than the UAV and its accessories (e.g., batteries and remote controller), UAV-based remote sensing platforms for agricultural purposes generally include onboard sensors for collecting data, a gimbal for motion correction and stabilization, and a flight planning App installed in a smart mobile device for automated flight control. To deliver precisely geo-referenced data, UAV platforms are typically coupled with ground control points (GCPs) with known global navigation satellite system positions.
The UAV platforms are available in several types with distinguishing features. The most widely used UAVs in precision agriculture are multi-rotor copters or fixed-wings (Li et al., 2021b). Multi-rotor copters can be quadcopters, hexacopters, or octocopters depending on the number of propellers they have. Multi-rotor copters are driven by the pairs of propellers to create thrust downward and directed by changing the thrust of one or more propellers. Thus, they are able to hover, take off and land vertically and have no lower limits on flight altitude. Fixed-wing UAVs, like airplanes, have a pair of rigid wings and a thruster at back. The lift of fixed-wing UAVs comes from the air pressure differences between the upper and lower sides that are generated passively as its wings cut through the air at a specific angle when being pushed forward. Fixed-wing UAVs are fast and energy-efficient, and thus allow much larger ground coverage than the multi-rotor copters. On the other hand, they do not have the capability to hover and fly close to the ground. A list of popular UAV models and their applications in potato applications are summarized in Table 3.
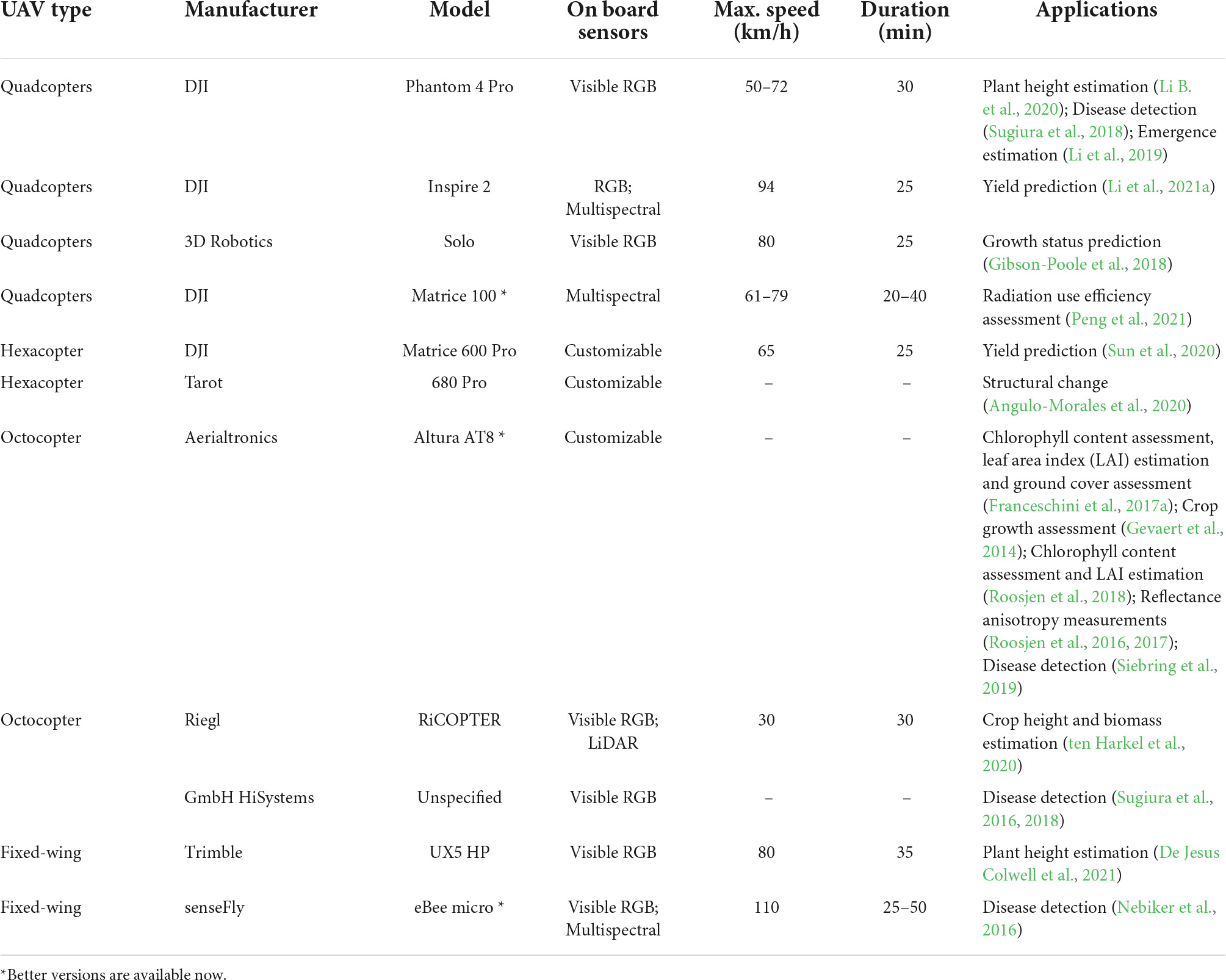
Table 3. UAV-based remote sensing platforms and their applications in potato traits characterization.
Selection of UAVs is conditional on specific tasks, including field area, spatial resolution, flexibility for carrying various cameras, and costs. Among all UAV types, quadcopters are the most popular as they are light weight and easy to operate. The DJI Phantom series with high-resolution motion RGB cameras have been used in applications for obtaining plant structural traits (Li B. et al., 2020) and recently they have an updated multispectral version for those who favor spectral measurements. Hexacopters generally are not sold with onboard sensors but offer interfaces to power and control third-party cameras. Thus the hexacopters are often used to carry multi- or hyper-spectral cameras for perceiving complicated crop traits such as yield responses (Feng et al., 2020; Zhou et al., 2021b) or stress (Zhou et al., 2020, 2021a). Similar to the hexacopter, octocopters are flexible in carrying multiple sensors and other devices for durable flight. The most widely accepted octocopter is the Altura AT8 (Aerialtronics, South Holland, Netherlands) which was used to carry a hyperspectral imaging system (2.0 kg in total) as the original version of the Hyperspectral Mapping System (HYMSY, Suomalainen et al., 2014). The HYMSY system costs around $12,769 and is largely used in potato studies conducted in European. On the opposite to multi-rotor UAVs, the fixed-wings offer a solution to the need of covering large fields because of their nature of high flight speed and altitude. Cameras on fixed-wings should have high frame rates and short exposure time to avoid blurred images caused by the high flight speed (Li et al., 2021b). As products evolve, fixed-wings vendors now offer a variety of versions with dual or triple cameras for agricultural purposes.
Applications
Precision agricultural management is the key to reducing environmental impact and enhancing agricultural sustainability (Zhang et al., 2021). Within the context of potato management, a variety of activities are employed to ensure its productivity and quality throughout the growing cycle. Some of these activities heavily rely on in situ field monitoring of potato growing conditions, field environmental conditions and diseases, followed by in-season decision-making on irrigation, fertilization, pesticides and off-season guidance on storage, sell, and post-harvest field management. Remote sensing techniques have been explored and applied to directly measure crop yield, disease damages, and some key indicators of potato growth. In this section, we reviewed the applications of these techniques in potato management, including tuber yield prediction, AGB estimation, water deficit stress assessment, nitrogen concentration estimation, and disease detection. Some key traits that are closely related to potato growth were also summarized, namely plant height, LAI, chlorophyll, and emergence detection and plant counting. The details of the applications can be found in Table 4.
Tuber yield prediction
Achieving the maximum crop yield at the lowest investment is an ultimate goal for farmers (Al-Gaadi et al., 2016). Early detection of problems associated with crop yield can greatly help with the decision-making for field management and reduce profit loss. In addition, yield mapping pre- or post-harvest can be used as spatial references for implementing variable rate technologies to achieve precise applications of field-level inputs and thus optimize production across entire fields (Al-Gaadi et al., 2016).
Potato tuber yield is jointly affected by genetic variation, environmental conditions (soil and weather), seed quality, and crop management practices (Li et al., 2021a). Classical potato yield prediction models are often used to estimate yields within the growing season by considering nitrogen fertilizer, temperature, and daylight or the incidence of solar radiation (Wang X. et al., 2021). Recent studies showed higher prediction accuracies by using machine learning models with remote sensing data at various levels, providing target-specific insights and recommendations for precision agriculture practices.
Salvador et al. (2020) predicted potato tuber yield at a municipal level in Mexica using machine learning models with satellite remote sensing imagery. The models were trained with NDVI values calculated from the TERRA satellite images combined with meteorological data on a monthly basis. The best testing performance was from the Random Forest model for the winter cycle (R2 = 0.76 and RMSE% = 18.9) and the polynomial Support Vector Machine for the summer cycle (R2 = 0.86 and RMSE% = 14.9). There are a few similar studies using satellite imagery and machine learning models for predicting potato yield at large scales in Spain (Gómez et al., 2019), Saudi Arabia (Al-Gaadi et al., 2016), and Bangladesh (Bala and Islam, 2009).
Unmanned aerial vehicle-based imagery is largely used for predicting potato yield in site-specific studies. Multi- (Tanabe et al., 2019; Li et al., 2021a) and hyper-spectral (Li B. et al., 2020; Sun et al., 2020) have been used to establish and validate a variety of machine learning models for predicting potato yield. The prediction performance of images collected at different growth stages was compared. It was found that images at earlier growth stages, i.e., the tuber initiation stage (Li et al., 2021a) and vegetative growth stage (Tanabe et al., 2019), tuber bulking stage (Li B. et al., 2020) had better prediction performances than those at the later stage when close to the end of seasons. Even though, Sun et al. (2020) reported a decent prediction accuracy with R2 = 0.63, RMSE = 3.03 ton/ha, and RMSE% = 5.8 when using hyperspectral image features at tuber maturation stage and machine learning models to predict potato tuber yield under four irrigation levels.
Potato above-ground biomass estimation
Above-ground biomass is a basic agronomic trait in field investigations and is often used to indicate crop growth status, the effectiveness of agricultural management measures, and the carbon sequestration ability of crops (Li B. et al., 2020). Particularly for potatoes, AGB is one of the most important indicators for calculating the nitrogen nutrition index and diagnosing the potato nitrogen status for precise nitrogen fertilizer management (Jin et al., 2021; Yang et al., 2021). Manual measurement of AGB requires cutting culms at the ground level for a defined portion of the experimental plot, and then weighing before (AGB fresh weight) and after drying (AGB dry weight) in an oven until constant weight (Pask et al., 2012), which is laborious and subject to experimental errors (Jimenez-Berni et al., 2018).
Estimations of potato AGB using remote sensing methods have been explored in a variety of ways, by integrating spectral-based VIs, plant height (manual- or sensor-based) with machine learning, classical and empirical models. Ground-based data acquired on potato canopies by spectroradiometers (Pei et al., 2019; Elsayed et al., 2021b; Yang et al., 2021), RGB, and thermal cameras (Elsayed et al., 2021a) were used as predictors in machine learning models and the studies showed that the agreements between the estimated and measured biomass were up to R2 of 0.7–0.8. Pei et al. (2019) also compared the estimation performance using the ground spectral data at different stages and the best result was given by the spectral features at the tube growth stage with R2 = 0.71 and RMSE% = 19.3.
In addition to the spectral features, plant height was considered as an important contributor to potato biomass. ten Harkel et al. (2020) derived the potato plant height from a UAV-LiDAR device and imported it to an empirical model originated from (Jimenez-Berni et al., 2018) for the estimation of biomass from LiDAR. The biomass solely based on plant height had an R2 of 0.24 with the RMSE of 22.1 g/m2. In another case, the UAV image-based plant height (from RGB images using the Structure from Motion method) was integrated with UAV hyperspectral VIs into a Random Forest model. The model accuracy for the biomass fresh weight was up to R2 = 0.90 with RMSE% = 20.6, and for the biomass dry weight up to R2 = 0.92 with RMSE% = 17.4 (Li B. et al., 2020).
An interesting comparison in the estimation performance of potato biomass using ground, UAV, and satellite data was conducted by Peng et al. (2021). Data from each source were independently used as variables in a classical model (the Carnegie–Ames–Stanford approach). The results showed that the ground spectral data reached the highest accuracy with R2 = 0.76 and RMSE% = 22.2, while the UAV and the Satellite multispectral image features had the similar performance with R2 = 0.70 (RMSE% = 22.4) and R2 = 0.72 (RMSE% = 24.4), respectively.
Potato water deficit stress assessment
Potato plants are sensitive to water stress due to their shallow root systems. Approximately 70% of the total water needed for potato growth comes from the upper 30 cm of the soil (Ahmadi et al., 2011). Potato commonly grows in coarse-textured soil with low water holding capacity (Rud et al., 2014) or in arid and semiarid countries (Elsayed et al., 2021a). Therefore, inappropriate water management practices or water deficit stress in potato plants could cause significant reductions in tuber yield (Karam et al., 2014) and degraded tuber quality (Djaman et al., 2021).
The plant water deficit is conventionally detected by measuring plant water statuses, such as leaf water potential and stomatal conductance, or by measuring soil gravimetric water content. All of the measurements are direct, destructive, labor-intensive, and unable for large-scale cultivation or tracking intra-field variability (Rud et al., 2014). CWSI, accounting for the differences between canopy and air temperatures at a fixed air vapor pressure deficit, has been extensively validated to represent plant water status, after it was designed by Idso et al. (1981). The use of leaf or canopy temperature to detect the water-related stresses is based on the principle that a plant’s stomatal closure takes place during water stress, which results in a decrease of energy dissipation and an increase in plant temperature (Idso et al., 1981; Elsayed et al., 2021a). The CWSI has been proposed as a good indicator of plant water stress (Cucho-Padin et al., 2020) and proved relating to stomatal conductance (Rud et al., 2014) and yield (Ghazouani et al., 2017).
The computation of CWSI requires the knowledge of plant canopy temperature which can be acquired through remote sensing technologies. Handheld infrared cameras have been used to obtain temperature values of plant canopy and soil to calculate the CSWI values for potato plants under irrigation treatments. Cucho-Padin et al. (2020) found that the CWSI values derived from a TIR camera had a high correlation (the Pearson’s correlation coefficient r = 0.84) with those from ground proximal sensors. The results also showed that no significant reduction in tuber yield was observed by using CWSI < 0.4 as a threshold for irrigation in potato crops, compared to the irrigation amount with CWSI > 0.4, which agrees with other works (Ramírez et al., 2016; Rinza et al., 2019) and provides a reference for saving irrigation water in arid and semiarid regions (Cucho-Padin et al., 2020).
The effectiveness of aerial TIR images in representing potato water status was evaluated in Rud et al. (2014) by comparing the image-derived CWSI with the biophysical measurements of leaf stomatal conductance values. In this study, RGB images were acquired simultaneously with the TIR images and used to create masks for the TIR images to distinguish between vegetation and soil. The CWSI was calculated based on the difference between leaf and air temperatures normalized to the variation in environmental meteorological conditions and showed high correlations (0.64 ≤ R2 ≤ 0.99) with the stomatal conductance measurements.
A few studies have tried to combine spectral indices with the CWSI and canopy temperature to indicate potato water stress. Elsayed et al. (2021a) reported a good alignment (R2 = 0.89) between the estimated and measured water content of the potato AGB by using the spectral indices derived from RGB and thermal images combined with machine learning models. Similarly, Gerhards et al. (2016) compared the performance of detecting water content in potato plants between spectral and thermal-based indices. The results showed that the CWSI was most suitable for water stress detection among all the thermal-based indices. Additionally, it was found that the NIR/SWIR reflectance-based indices and the spectral emissivity (non-imaging devices) were equally sensitive to plant water content and responded quicker than the indices from imaging devices (such as the NDVI from a hyperspectral TIR camera; Gerhards et al., 2016).
Potato nitrogen concentration estimation
Potato growth depends largely on the nitrogen availability in the soil (Nigon et al., 2014). However, the shallow-root system coupled with their common cultivation in coarse-textured soils leads to poor nitrogen use efficiency in potato plants (Nigon et al., 2014). Excessive applications of nitrogen fertilizers, on one hand, might have negative impacts on potato productivity by inducing a greater growth of aboveground biomass but reducing the root growth. On the other hand, it raises the health risks associated with nitrate-contaminated groundwater caused by the high rates of nitrate leaching in irrigated potato fields (Zebarth and Rosen, 2007; Lynch et al., 2012). Therefore, precise spatial and temporal nitrogen management is important to optimize production and minimize environmental nitrogen losses (Nigon et al., 2014).
One of the typical guidance on the timing and amount of nitrogen applications on potato is nitrogen nutrition index, defined as the ratio of the actual nitrogen concentration (Nc) over the minimum crop nitrogen concentration allowing for maximum biomass production (Ncri). While Ncri is an estimated value depending on the potato AGB, Nc needs to be determined along with the potato growth and traditionally by wet tests in laboratory. However, Nc values from the sampled potato plants might not accurately represent nitrogen status for an entire field and potato nitrogen status might change considerably by the time of analysis (Zhou et al., 2018). Thus, fast and accurate estimation of potato Nc values is urgently needed to assist the precision nitrogen management in potato productions.
Canopy spectral reflectance has been used extensively in agriculture to measure the actual nitrogen status of crop growth in order to guide nitrogen fertilization (Raun et al., 2002; Van Evert et al., 2012; Zhou et al., 2016c). The principle that the canopy spectral reflectance can indicate the canopy Nc values is based on the fact that the light reflected in certain wavebands is sensitive to leaf chlorophyll concentration, which in turn closely depends on leaf nitrogen concentration (Lawlor et al., 1989; Baret and Fourty, 1997; Hansen and Schjoerring, 2003). Studies have been conducted to compare the performances in estimating the potato Nc among canopy spectral reflectance obtained at different scales (handheld/UAV/Satellite) with narrow or wide spectral bandwidths and various ranges in spectral wavelength. The commonly used spectral features and their details have been summarized in Table 3.
Potato disease detection
Potato production is threatened by several diseases resulting in considerable yield losses, causing decreases in the quality and increases in the price of potatoes (Taylor et al., 2008). In-season early detection is particularly important for disease management decisions to control disease severity and prevent the spread of diseases (Oppenheim and Shani, 2017). Besides, detecting the overall disease grade of potato fields allows growers to make decisions regarding the best way to store, sell, and manage the fields for future generations (Gibson-Poole et al., 2018). Traditional potato disease inspection requires visual identification of the signs of disease. However, the disease plants can be difficult to identify, especially when symptoms are not yet developed or at the early stages. The remote sensing technique provides an opportunity to overcome the limitations, and they have already been widely used to detect diseases of potatoes for many years.
Among all the diseases that occurred during potato growth, Potato Virus Y (Potyviridae, PVY) is the most common reason for the rejection of seed lots from certification and one of the most common potato diseases (Griffel et al., 2018). The foliar symptoms of PVY include mosaic, leaf drop, and interveinal necrosis, and this virus can also cause necrotic rings on potato tubers (Gray et al., 2010). However, the recently prevalent PVY strains exhibit transient and milder foliar symptoms, making it less effective the visual inspection of seed lots with high PVY incidence (Frost et al., 2013). Spectral reflections in the NIR and SWIR regions have provided differentiable profiles in the underlying responses to the PVY stress. Couture et al. (2018) assessed the efficacy of hyperspectral information (a handheld device with narrow bands between 350 to 2,500 nm) in detecting the presence of PVY in potato leaves prior to the onset of the visual symptoms and observed a significant difference in the whole spectral profile between the PVY-negative and PVY-positive plants, especially in the NIR and SWIR spectral regions. A partial-least square discriminate analysis model trained with normalized spectral indices derived from the hyperspectral bands correctly identified the positive PVY infection status in vivo with a mean cross-validation accuracy of Kappa = 0.73. Similarly, high accuracy of 89.8% was reported by Griffel et al. (2018) in classifying the PVY-infected and non-infected potato plants using the UAV-based spectral information in the NIR and SWIR regions. Another example was given by Polder et al. (2019) that the healthy potato plants were distinguished from PVY infected ones (the classification Precision = 0.78) by a deep learning model directly using the hyperspectral images (400–1,000 nm).
Potato late blight (Phytophthora infestans) is another serious disease affecting potato production worldwide (Hwang et al., 2014). The disease rapidly destroys leaves, and it consequently leads to yield losses and tuber quality deterioration. Symptoms of late blight on potato plants mostly appear on the leaves, developing from small, dark green, irregular-shaped water-soaked spots to large, dark brown or black lesions surrounded by a yellow chlorotic halo. Compared to the PVY, late blight affections are more easily detectable from potato canopies using remote sensing techniques. Various sensing combinations have been evaluated with promising potential to estimate the late blight severity, from ground-based spectrometer (Ray et al., 2011; Franceschini et al., 2017a; Gold et al., 2020) to UAV-based RGB (Sugiura et al., 2016), multi-/hyper-spectral (Nebiker et al., 2016; Franceschini et al., 2017b; Duarte-Carvajalino et al., 2018) cameras and to satellite-based MSI (Dutta et al., 2014). Despite using considerably different prediction models, the general idea of quantifying the late blight severity is first to differentiate the infected and the healthy leaves parts and then obtain as the estimations the percentage of the damaged part over the healthy part (Dutta et al., 2014; Sugiura et al., 2016; Duarte-Carvajalino et al., 2018).
Another potato disease that has been detected using remote sensing techniques is early blight (Alternaria solani). Atherton et al. (2015) analyzed the spectral profile of potato plants with the early blight and noticed a significant difference in the spectral reflectance between heavily diseased plants and healthy plants. Van De Vijver et al. (2020) developed a ground-based proximal sensing platform-Hypercart for acquiring hyperspectral images in the potato field. The machine learning models highly agreed (R2 = 0.92) with the visual assessments. Additionally, significant differences between healthy leaf and injected leaf tissues were observed in the green (550 nm), red (680 nm), and NIR (720–750 nm) regions and the NIR was recognized as the most helpful region for detecting the early blight lesions.
Other traits
Potato plant height
Plant height is one of the most important traits for precision agricultural management. Plant height usually had a significantly positive correlation with yield in many types of crops, thus it is often used as an indicator of crop yield potential (Li et al., 2016; Feng et al., 2019). Besides, plant height can be used to estimate crop AGB and crop lodging. Remote sensing of plant height commonly adopts two different techniques, one directly obtaining the depth information from sensors, for example, the LiDAR devices. The other one is to estimate the 3D information from highly overlapped 2D images using photogrammetric methods such as stereo vision or structure from motion.
The effectiveness and accuracy of plant height measurement in remote sensing depend on the joint effects of the device type, measuring distances, algorithms, and plant variations. For estimating the potato plant height, ten Harkel et al. (2020) used a UAV-LiDAR system at 40 m above ground level and obtained the estimation accuracy of R2 = 0.50 and RMSE = 12 cm. By using photogrammetric methods, a less expensive way than the LiDAR devices is to take the difference between DSM (representing the absolute elevation of the object in image pixels) and DEMs (representing the absolute elevation of the bare ground under the canopy) derived from RGB imagery. Estimated potato heights often showed high correlations with the R2 = 0.93 and RMSE = 6.39 cm using a high-resolution RGB camera at a flight height of 30 m (Li B. et al., 2020) and R2 = 0.52 at a flight height of 75 m with more than 200 potato varieties (De Jesus Colwell et al., 2021).
Leaf area index
Leaf area index is one of the most fundamental vegetation biophysical parameters, defined as a dimensionless measure of the one-sided leaf area (m2) per unit ground surface area (m2). LAI has long been reported as a good indicator of vegetation status (Reyes-González et al., 2019), photosynthesis rate (Clevers et al., 2017), biomass accumulation (Viña et al., 2011), evapotranspiration (Jung et al., 2010), yield estimation (Luo et al., 2020), etc. In potato growth, LAI is also crucial in estimating plant nitrogen concentration. Zhou et al. (2017) developed a ratio vegetation index/LAI-reference curve defining the optimal nitrogen-nutrition for potatoes throughout the season and it could be used as a diagnostic tool to detect nitrogen stress in potato plants during the season.
A variety of handheld instruments have been developed specifically for measuring plant LAI in field conditions, such as the LAI-2000/LAI-2200C instrument (Li-Cor, Inc, Lincoln, NE, United States) the SS1 Sun-Scan canopy analyzer (Delta-T Devices Ltd, Cambridge, United Kingdom), and AccuPAR LP-80 ceptometer (Decagon Devices, Inc). Multi-/hyper-spectral spectrometers are also commonly used to estimate LAI through empirical models that were derived from historical data between LAI measurements (by the LAI instruments) and spectral indices (Herrmann et al., 2011; Papadavid et al., 2011; Clevers et al., 2017). Satellite and UAV-based LAI estimates were validated for measuring at larger scales by observing significant linear relationships with the measurements of the handheld LAI instruments (Herrmann et al., 2011; Clevers et al., 2017; Roosjen et al., 2018).
Leaf chlorophyll content
Leaf chlorophyll content (LCC) plays a significant role in plant growth, helping crops to absorb and store the energy to build their tissues. Combining carbon dioxide (absorbed from the air) and water, the stored energy can be converted into glucose through the photosynthesis process (Zheng et al., 2018). Then, the plants utilize the glucose and the nutrients (such as fertilizers and water) from the soil to generate new leaves and other parts, and release the produced oxygen back into the air (Yadav et al., 2010). The LCC is one essential indicator of plant health, and can be used to assess the photosynthetic rate, plant productivity, and nutrient level (Clevers and Kooistra, 2012; Vesali et al., 2017).
A portable non-imaging device, the Soil Plant Analysis Development chlorophyll meter, provides a rapid, accurate, and non-destructive approach to measure the LCC for different crops. Readings from this device have been successfully used as an indicator of plant health status and nitrogen concentrations in potato remote sensing (Giletto et al., 2010; Ramírez et al., 2014, 2015) and often used as ground truth for investigating the effectiveness of RGB and spectral images (in lab conditions) in estimating the LCC, providing the basis for monitoring the LCC in situ with the remote sensing platforms for potato management (Dutta Gupta et al., 2013; Borhan et al., 2017). Under field conditions, handheld hyperspectral spectrometer (Liu et al., 2020), satellite multispectral imagery (Kooistra and Clevers, 2016), and UAV hyperspectral imagery (Gevaert et al., 2015) have been explored with promising potential in estimating the potato LLC.
Potato emergence detection and plant counting
Potato emergence rate and uniformity play important roles in potato field management (Moran et al., 1997) and yield prediction (Ciuberkis et al., 2007). Poor potato emergence and canopy uniformity lead to variations in the amount of canopy sunlight interception and hence varied photosynthetic efficiency (Moran et al., 1997). Thus, consistent potato emergence is always desirable at early growth stages and is a key trait being routinely assessed in potato agronomy and potato improvement research. Potato emergence can be affected by many factors, such as seed quality, dormancy period, soil temperature, water stress, and nutrient deficiency (Sankaran et al., 2017; Li et al., 2019). The standard method for assessing crop emergence is to visually count the number of plants that emerged at frequent intervals during the establishment phase of development, whereas the uniformity is estimated by subjective and inaccurate manual scoring (Knowles and Knowles, 2006; Herman et al., 2016). Remote sensing techniques can greatly enhance the efficiency in the emergence evaluation. There have been a number of studies leveraging UAV-based imagery and advanced machine learning methods for detecting potato emergence.
Sankaran et al. (2017) observed a significant correlation (r = 0.82) between the image-based and manual plant count. Image pixels representing plant canopies were separated (by a color threshold) from the UAV-based multispectral images collected at 15 m in a potato field and then the number of image polygons were estimated as the plant count. Similarly, Li et al. (2019) obtained the image polygons from the UAV-based RGB images using the Otsu thresholding method. The potato emergence rate of a pixel polygon was then estimated from six morphological features of the polygon by a trained machine learning model. The estimations showed a high correlation (R2 = 0.96) with the manual count. Despite the excellent performance, the image plant counts in these two studies required the accurate separation of pixels between plant and background, which limits its applications for situations with non-uniform backgrounds and changing illuminations. A more adaptable way was proposed by Machefer et al. (2020) for potato plant counting by directly inputting UAV-based RGB images to a transferred deep learning architecture. The model was previously trained on low-density crops so a much smaller number of labeled images was needed to train the model for potato plants. Besides, no pre-processing step was required on the UAV images, which helps to keep the stable performance under varying UAV image collection conditions.
Conclusion
Remote sensing has great potential in characterizing potato traits for precision agricultural applications, as a promising alternative to conventional field scouting and visual examination for farmers. This review fills a critical gap in precision agriculture literature by providing a comprehensive review of the applications of remote sensing technologies specifically used in potato trait characterization. We reviewed the commonly used imaging sensors and remote sensing platforms with the comparisons of their strengths and limitations in characterizing potato traits. The main applications that are benefited from the development of advanced remote sensing technologies have been summarized with the scientific logic behind the scenes and the current research status. The applications include tuber yield prediction, AGB estimation, water stress detection, nitrogen stress assessment, and disease detection. Four other agronomic traits that were widely investigated relating to potato growth were listed as well, namely plant height, LAI, LCC, and emergence rate and plant counting. This review will update potato agronomists and farmers with the latest approaches and research outcomes, as well as provide a selective list for those who have the intentions to apply remote sensing technologies to characterize potato traits for precision agriculture.
Author contributions
CS: conceptualization and writing—original draft preparation. JZ, YM, YX, and BP: writing—reviewing and editing. ZZ: conceptualization, resources, and writing—reviewing and editing. All authors contributed to the article and approved the submitted version.
Funding
This work was supported by the USDA National Institute of Food and Agriculture, Hatch project WIS03081, University of Wisconsin–Madison, Office of the Vice Chancellor for Research, and Graduate Education with funding from the Wisconsin Alumni Research Foundation.
Conflict of Interest
The authors declare that the research was conducted in the absence of any commercial or financial relationships that could be construed as a potential conflict of interest.
Publisher’s Note
All claims expressed in this article are solely those of the authors and do not necessarily represent those of their affiliated organizations, or those of the publisher, the editors and the reviewers. Any product that may be evaluated in this article, or claim that may be made by its manufacturer, is not guaranteed or endorsed by the publisher.
References
Abou Ali, H., Delparte, D., and Griffel, L. M. (2020). From pixel to yield: forecasting potato productivity in Lebanon and Idaho. Int. Arch. Photogramm. Remote Sens. Spatial Inf. Sci. XLII-3-W11, 1–7. doi: 10.5194/isprs-archives-XLII-3-W11-1-2020
Ahmadi, S. H., Plauborg, F., Andersen, M. N., Sepaskhah, A. R., Jensen, C. R., and Hansen, S. (2011). Effects of irrigation strategies and soils on field grown potatoes: root distribution. Agric. Water Manage. 98, 1280–1290.
Akhand, K., Nizamuddin, M., Roytman, L., and Kogan, F. (2016). “Using remote sensing satellite data and artificial neural network for prediction of potato yield in Bangladesh,” in Proceedings of the Remote Sensing and Modeling of Ecosystems for Sustainability XIII, Vol. 9975. (Bellingham, WA: International Society for Optics and Photonics), 52–66. doi: 10.1117/12.2237214
Aldea, M., Frank, T. D., and DeLucia, E. H. (2006a). A method for quantitative analysis of spatially variable physiological processes across leaf surfaces. Photosynth. Res. 90, 161–172. doi: 10.1007/s11120-006-9119-z
Aldea, M., Hamilton, J. G., Resti, J. P., Zangerl, A. R., Berenbaum, M. R., Frank, T. D., et al. (2006b). Comparison of photosynthetic damage from arthropod herbivory and pathogen infection in understory hardwood saplings. Oecologia 149, 221–232. doi: 10.1007/s00442-006-0444-x
Al-Gaadi, K. A., Hassaballa, A. A., Tola, E., Kayad, A. G., Madugundu, R., Alblewi, B., et al. (2016). Prediction of potato crop yield using precision agriculture techniques. PLoS One 11:e0162219. doi: 10.1371/journal.pone.0162219
Angulo-Morales, V., Rodríguez-Galvis, J., Lizarazo-Salcedo, I., and Gaona-García, E. (2020). A novel method for estimation of structural changes in potato crops from UAV-based digital surface models. ISPRS Ann. Photogramm. Remote Sens. Spat. Inf. Sci. V-1-2020, 269–276. doi: 10.5194/isprs-annals-V-1-2020-269-2020
Ashourloo, D., Shahrabi, H. S., Azadbakht, M., Rad, A. M., Aghighi, H., and Radiom, S. (2020). A novel method for automatic potato mapping using time series of Sentinel-2 images. Comput. Electron. Agric. 175:105583. doi: 10.1016/j.compag.2020.105583
Atherton, D., Watson, D. G., Zhang, M., Qin, Z., and Liu, X. (2015). “Hyperspectral spectroscopy for detection of early blight (Alternaria solani) disease in potato (Solanum tuberosum) plants at two different growth stages,” in Proceedings of the 2015 ASABE Annual International Meeting, Vol. 1. (St. Joseph, MI: ASABE). doi: 10.13031/aim.20152186976
Atzberger, C., and Richter, K. (2012). Spatially constrained inversion of radiative transfer models for improved LAI mapping from future Sentinel-2 imagery. Remote Sens. Environ. 120, 208–218. doi: 10.1016/j.rse.2011.10.035
Awad, M. M. (2019). Toward precision in crop yield estimation using remote sensing and optimization techniques. Agriculture 9:54. doi: 10.3390/agriculture9030054
Bala, S. K., and Islam, A. S. (2009). Correlation between potato yield and MODIS-derived vegetation indices. Int. J. Remote Sens. 30, 2491–2507. doi: 10.1080/01431160802552744
Banerjee, B. P., Joshi, S., Thoday-Kennedy, E., Pasam, R. K., Tibbits, J., Hayden, M., et al. (2020). High-throughput phenotyping using digital and hyperspectral imaging-derived biomarkers for genotypic nitrogen response. J. Exp. Bot. 71, 4604–4615. doi: 10.1093/jxb/eraa143
Baret, F., and Fourty, T. H. (1997). “Radiometric estimates of nitrogen status of leaves and canopies,” in Diagnosis of the Nitrogen Status in Crops, ed. G. Lemaire (Berlin: Springer), 201–227. doi: 10.1007/978-3-642-60684-7_12
Bellis, E. S., Hashem, A. A., Causey, J. L., Runkle, B. R. K., Moreno-García, B., Burns, B. W., et al. (2022). Detecting intra-field variation in rice yield with unmanned aerial vehicle imagery and deep learning. Front. Plant Sci. 13:716506. doi: 10.3389/fpls.2022.716506
Bohman, B. J., Rosen, C. J., and Mulla, D. J. (2019). Evaluation of variable rate nitrogen and reduced irrigation management for potato production. Agron. J. 111, 2005–2017. doi: 10.2134/agronj2018.09.0566
Borhan, M. S., Panigrahi, S., Satter, M. A., and Gu, H. (2017). Evaluation of computer imaging technique for predicting the SPAD readings in potato leaves. Inf. Process. Agric. 4, 275–282. doi: 10.1016/j.inpa.2017.07.005
Chaerle, L., Caeneghem, W. V., Messens, E., Lambers, H., Van Montagu, M., and Van Der Straeten, D. (1999). Presymptomatic visualization of plant–virus interactions by thermography. Nat. Biotechnol. 17, 813–816. doi: 10.1038/11765
Chaerle, L., and Van Der Straeten, D. (2000). Imaging techniques and the early detection of plant stress. Trends Plant Sci. 5, 495–501. doi: 10.1016/S1360-1385(00)01781-7
Ciuberkis, S., Bernotas, S., Raudonius, S., and Felix, J. (2007). Effect of weed emergence time and intervals of weed and crop competition on potato yield. Weed Technol. 21, 213–218. doi: 10.1614/WT-04-210.1
Clevers, J. G. P. W., and Gitelson, A. A. (2013). Remote estimation of crop and grass chlorophyll and nitrogen content using red-edge bands on Sentinel-2 and -3. Int. J. Appl. Earth Obs. Geoinf. 23, 344–351. doi: 10.1016/j.jag.2012.10.008
Clevers, J. G. P. W., and Kooistra, L. (2012). Using hyperspectral remote sensing data for retrieving canopy chlorophyll and nitrogen content. IEEE J. Sel. Top. Appl. Earth Obs. Remote Sens. 5, 574–583. doi: 10.1109/JSTARS.2011.2176468
Clevers, J. G. P. W., Kooistra, L., and Van den Brande, M. M. M. (2017). Using Sentinel-2 data for retrieving LAI and leaf and canopy chlorophyll content of a potato crop. Remote Sens. 9:405. doi: 10.3390/rs9050405
Cohen, B., Edan, Y., Levi, A., and Alchanatis, V. (2022). Early detection of grapevine (Vitis vinifera) downy mildew (Peronospora) and diurnal variations using thermal imaging. Sensors 22:3585. doi: 10.3390/s22093585
Couture, J. J., Singh, A., Charkowski, A. O., Groves, R. L., Gray, S. M., Bethke, P. C., et al. (2018). Integrating spectroscopy with potato disease management. Plant Dis. 102, 2233–2240. doi: 10.1094/PDIS-01-18-0054-RE
Cucho-Padin, G., Rinza, J., Ninanya, J., Loayza, H., Quiroz, R., and Ramírez, D. A. (2020). Development of an open-source thermal image processing software for improving irrigation management in potato crops (Solanum tuberosum L.). Sensors 20:472. doi: 10.3390/s20020472
De Jesus Colwell, F., Souter, J., Bryan, G. J., Compton, L. J., Boonham, N., and Prashar, A. (2021). Development and validation of methodology for estimating potato canopy structure for field crop phenotyping and improved breeding. Front. Plant Sci. 12:612843. doi: 10.3389/fpls.2021.612843
Deery, D. M., Rebetzke, G. J., Jimenez-Berni, J. A., Bovill, W. D., James, R. A., Condon, A. G., et al. (2019). Evaluation of the phenotypic repeatability of canopy temperature in wheat using continuous-terrestrial and airborne measurements. Front. Plant Sci. 10:875. doi: 10.3389/fpls.2019.00875
Degani, O., Chen, A., Dor, S., Orlov-Levin, V., Jacob, M., Shoshani, G., et al. (2022). Remote evaluation of maize cultivars susceptibility to late wilt disease caused by Magnaporthiopsis maydis. J. Plant Pathol. 104, 509–525. doi: 10.1007/s42161-022-01039-9
Delegido, J., Verrelst, J., Meza, C. M., Rivera, J. P., Alonso, L., and Moreno, J. (2013). A red-edge spectral index for remote sensing estimation of green LAI over agroecosystems. Eur. J. Agron. 46, 42–52. doi: 10.1016/j.eja.2012.12.001
Delgado, J. A., Short, N. M., Roberts, D. P., and Vandenberg, B. (2019). Big data analysis for sustainable agriculture on a geospatial cloud framework. Front. Sustain. Food Syst. 3:54. doi: 10.3389/fsufs.2019.00054
Djaman, K., Irmak, S., Koudahe, K., and Allen, S. (2021). Irrigation management in potato (Solanum tuberosum L.) production: a review. Sustainability 13:1504. doi: 10.3390/su13031504
Duarte-Carvajalino, J. M., Alzate, D. F., Ramirez, A. A., Santa-Sepulveda, J. D., Fajardo-Rojas, A. E., and Soto-Suárez, M. (2018). Evaluating late blight severity in potato crops using unmanned aerial vehicles and machine learning algorithms. Remote Sens. 10:1513. doi: 10.3390/rs10101513
Dutta, S., Singh, S. K., and Panigrahy, S. (2014). Assessment of late blight induced diseased potato crops: a case study for west Bengal district using temporal AWiFS and MODIS data. J. Indian Soc. Remote Sens. 42, 353–361. doi: 10.1007/s12524-013-0325-9
Dutta Gupta, S., Ibaraki, Y., and Pattanayak, A. K. (2013). Development of a digital image analysis method for real-time estimation of chlorophyll content in micropropagated potato plants. Plant Biotechnol. Rep. 7, 91–97. doi: 10.1007/s11816-012-0240-5
Dutta Gupta, S., and Pattanayak, A. (2017). Intelligent image analysis (IIA) using artificial neural network (ANN) for non-invasive estimation of chlorophyll content in micropropagated plants of potato. In Vitro Cell. Dev. Biol. Plant 53, 520–526. doi: 10.1007/s11627-017-9825-6
Eisenbeiss, H. (2004). A mini unmanned aerial vehicle (UAV): system overview and image acquisition. Int. Arch. Photogramm. Remote Sens. Spat. Inf. Sci. 36, 1–7.
Elarab, M., Ticlavilca, A. M., Torres-Rua, A. F., Maslova, I., and McKee, M. (2015). Estimating chlorophyll with thermal and broadband multispectral high resolution imagery from an unmanned aerial system using relevance vector machines for precision agriculture. Int. J. Appl. Earth Obs. Geoinf. 43, 32–42. doi: 10.1016/j.jag.2015.03.017
Elsayed, S., El-Hendawy, S., Khadr, M., Elsherbiny, O., Al-Suhaibani, N., Alotaibi, M., et al. (2021a). Combining thermal and RGB imaging indices with multivariate and data-driven modeling to estimate the growth, water status, and yield of potato under different drip irrigation regimes. Remote Sens. 13:1679. doi: 10.3390/rs13091679
Elsayed, S., El-Hendawy, S., Khadr, M., Elsherbiny, O., Al-Suhaibani, N., Dewir, Y. H., et al. (2021b). Integration of spectral reflectance indices and adaptive neuro-fuzzy inference system for assessing the growth performance and yield of potato under different drip irrigation regimes. Chemosensors 9:55. doi: 10.3390/chemosensors9030055
Feng, A., Zhang, M., Sudduth, K. A., Vories, E. D., and Zhou, J. (2019). Cotton yield estimation from UAV-based plant height. Trans. ASABE 62, 393–404. doi: 10.13031/trans.13067
Feng, L., Zhang, Z., Ma, Y., Du, Q., Williams, P., Drewry, J., et al. (2020). Alfalfa yield prediction using UAV-based hyperspectral imagery and ensemble learning. Remote Sens. 12:2028. doi: 10.3390/rs12122028
Fortin, J. G., Anctil, F., Parent, L.-É, and Bolinder, M. A. (2011). Site-specific early season potato yield forecast by neural network in Eastern Canada. Precis. Agric. 12, 905–923. doi: 10.1007/s11119-011-9233-6
Franceschini, M. H. D., Bartholomeus, H., Van Apeldoorn, D., Suomalainen, J., and Kooistra, L. (2017a). Intercomparison of unmanned aerial vehicle and ground-based narrow band spectrometers applied to crop trait monitoring in organic potato production. Sensors 17:1428. doi: 10.3390/s17061428
Franceschini, M. H. D., Bartholomeus, H., van Apeldoorn, D., Suomalainen, J., and Kooistra, L. (2017b). Assessing changes in potato canopy caused by late blight in organic production systems through UAV-based pushbroom imaging spectrometer. Int. Arch. Photogramm. Remote Sens. Spat. Inf. Sci. XLII-2-W6, 109–112. doi: 10.5194/isprs-archives-XLII-2-W6-109-2017
Franceschini, M. H. D., Bartholomeus, H., van Apeldoorn, D. F., Suomalainen, J., and Kooistra, L. (2019). Feasibility of unmanned aerial vehicle optical imagery for early detection and severity assessment of late blight in potato. Remote Sens. 11:224. doi: 10.3390/rs11030224
Frost, K. E., Groves, R. L., and Charkowski, A. O. (2013). Integrated control of potato pathogens through seed potato certification and provision of clean seed potatoes. Plant Dis. 97, 1268–1280. doi: 10.1094/PDIS-05-13-0477-FE
Gerhards, M., Rock, G., Schlerf, M., and Udelhoven, T. (2016). Water stress detection in potato plants using leaf temperature, emissivity, and reflectance. Int. J. Appl. Earth Obs. Geoinf. 53, 27–39. doi: 10.1016/j.jag.2016.08.004
Gevaert, C. M., Suomalainen, J., Tang, J., and Kooistra, L. (2015). Generation of spectral–temporal response surfaces by combining multispectral satellite and hyperspectral UAV imagery for precision agriculture applications. IEEE J. Sel. Top. Appl. Earth Obs. Remote Sens. 8, 3140–3146. doi: 10.1109/JSTARS.2015.2406339
Gevaert, C. M., Tang, J., García-Haro, F. J., Suomalainen, J., and Kooistra, L. (2014). “Combining hyperspectral UAV and multispectral Formosat-2 imagery for precision agriculture applications,” in Proceedings of the 2014 6th Workshop on Hyperspectral Image and Signal Processing: Evolution in Remote Sensing (WHISPERS), Lausanne, 1–4. doi: 10.1109/WHISPERS.2014.8077607
Ghazouani, H., Capodici, F., Ciraolo, G., Maltese, A., Rallo, G., and Provenzano, G. (2017). Potential of thermal images and simulation models to assess water and salt stress: application to potato crop in Central Tunisia. Chem. Eng. Trans. 58, 709–714. doi: 10.3303/CET1758119
Gibson-Poole, S., Fenton, B., Griffin-Walker, R., and Hamilton, A. (2018). “Getting down to the plant level: potato trials analysis using a UAV equipped with un-modified and modified commercial off-the-shelf digital cameras,” in Proceedings of the Dundee Conference. Crop Production in Northern Britain 2018, 27-28 February 2018, Dundee, 231–236.
Gibson-Poole, S., Humphris, S., Toth, I., and Hamilton, A. (2017). Identification of the onset of disease within a potato crop using a UAV equipped with un-modified and modified commercial off-the-shelf digital cameras. Adv. Anim. Biosci. 8, 812–816. doi: 10.1017/S204047001700084X
Giletto, C. M., Díaz, C., Rattín, J. E., Echeverría, H. E., and Caldiz, D. O. (2010). Green index to estimate crop nitrogen status in potato processing varieties. Chil. J. Agric. Res. 70, 141–149. doi: 10.4067/S0718-58392010000100015
Gold, K. M., Townsend, P. A., Herrmann, I., and Gevens, A. J. (2020). Investigating potato late blight physiological differences across potato cultivars with spectroscopy and machine learning. Plant Sci. 295:110316. doi: 10.1016/j.plantsci.2019.110316
Gómez, D., Salvador, P., Sanz, J., and Casanova, J. L. (2019). Potato yield prediction using machine learning techniques and Sentinel 2 data. Remote Sens. 11:1745. doi: 10.3390/rs11151745
Grant, O. M., Tronina, Ł, Ramalho, J. C., Kurz Besson, C., Lobo-do-Vale, R., Santos Pereira, J., et al. (2010). The impact of drought on leaf physiology of Quercus suber L. trees: comparison of an extreme drought event with chronic rainfall reduction. J. Exp. Bot. 61, 4361–4371. doi: 10.1093/jxb/erq239
Gray, S., De Boer, S., Lorenzen, J., Karasev, A., Whitworth, J., Nolte, P., et al. (2010). Potato virus Y: an evolving concern for potato crops in the United States and Canada. Plant Dis. 94, 1384–1397. doi: 10.1094/PDIS-02-10-0124
Griffel, L. M., Delparte, D., and Edwards, J. (2018). Using support vector machines classification to differentiate spectral signatures of potato plants infected with potato virus Y. Comput. Electron. Agric. 153, 318–324. doi: 10.1016/j.compag.2018.08.027
Hansen, P. M., and Schjoerring, J. K. (2003). Reflectance measurement of canopy biomass and nitrogen status in wheat crops using normalized difference vegetation indices and partial least squares regression. Remote Sens. Environ. 86, 542–553. doi: 10.1016/S0034-4257(03)00131-7
Hendricks, G. S., Shukla, S., Roka, F. M., Sishodia, R. P., Obreza, T. A., Hochmuth, G. J., et al. (2019). Economic and environmental consequences of overfertilization under extreme weather conditions. J. Soil Water Conserv. 74, 160–171. doi: 10.2489/jswc.74.2.160
Herman, D. J., Knowles, L. O., and Knowles, N. R. (2016). Differential sensitivity of genetically related potato cultivars to treatments designed to alter apical dominance, tuber set and size distribution. Am. J. Potato Res. 93, 331–349. doi: 10.1007/s12230-016-9507-7
Herrmann, I., Pimstein, A., Karnieli, A., Cohen, Y., Alchanatis, V., and Bonfil, D. J. (2011). LAI assessment of wheat and potato crops by VENμS and Sentinel-2 bands. Remote Sens. Environ. 115, 2141–2151. doi: 10.1016/j.rse.2011.04.018
Hunt, E. R., Doraiswamy, P. C., McMurtrey, J. E., Daughtry, C. S. T., Perry, E. M., and Akhmedov, B. (2013). A visible band index for remote sensing leaf chlorophyll content at the canopy scale. Int. J. Appl. Earth Obs. Geoinf. 21, 103–112. doi: 10.1016/j.jag.2012.07.020
Hunt, E. R. J., Horneck, D. A., Spinelli, C. B., Turner, R. W., Bruce, A. E., Gadler, D. J., et al. (2018). Monitoring nitrogen status of potatoes using small unmanned aerial vehicles. Precis. Agric. 19, 314–333. doi: 10.1007/s11119-017-9518-5
Hunt, E. R. J., and Rondon, S. I. (2017). Detection of potato beetle damage using remote sensing from small unmanned aircraft systems. J. Appl. Remote Sens. 11:026013. doi: 10.1117/1.JRS.11.026013
Hunt, E. R. J., Rondon, S. I., Hamm, P. B., Turner, R. W., Bruce, A. E., and Brungardt, J. J. (2016). Insect detection and nitrogen management for irrigated potatoes using remote sensing from small unmanned aircraft systems. Auton. Air Ground Sens. Syst. Agric. Optim. Phenotyping 9866, 122–127. doi: 10.1117/12.2224139
Hwang, Y. T., Wijekoon, C., Kalischuk, M., Johnson, D., Howard, R., Prüfer, D., et al. (2014). Evolution and management of the Irish potato famine pathogen Phytophthora Infestans in Canada and the United States. Am. J. Potato Res. 91, 579–593. doi: 10.1007/s12230-014-9401-0
Idso, S. B., Jackson, R. D., Pinter, P. J., Reginato, R. J., and Hatfield, J. L. (1981). Normalizing the stress-degree-day parameter for environmental variability. Agric. Meteorol. 24, 45–55. doi: 10.1016/0002-1571(81)90032-7
Jimenez-Berni, J. A., Deery, D. M., Rozas-Larraondo, P., Condon, A. T. G., Rebetzke, G. J., James, R. A., et al. (2018). High throughput determination of plant height, ground cover, and above-ground biomass in wheat with LiDAR. Front. Plant Sci. 9:237. doi: 10.3389/fpls.2018.00237
Jin, X., Zarco-Tejada, P. J., Schmidhalter, U., Reynolds, M. P., Hawkesford, M. J., Varshney, R. K., et al. (2021). High-throughput estimation of crop traits: a review of ground and aerial phenotyping platforms. IEEE Geosci. Remote Sens. Mag. 9, 200–231. doi: 10.1109/MGRS.2020.2998816
Jones, H. G., Serraj, R., Loveys, B. R., Xiong, L., Wheaton, A., and Price, A. H. (2009). Thermal infrared imaging of crop canopies for the remote diagnosis and quantification of plant responses to water stress in the field. Funct. Plant Biol. 36, 978–989. doi: 10.1071/FP09123
Jones, H. G., Stoll, M., Santos, T., de Sousa, C., Chaves, M. M., and Grant, O. M. (2002). Use of infrared thermography for monitoring stomatal closure in the field: application to grapevine. J. Exp. Bot. 53, 2249–2260. doi: 10.1093/jxb/erf083
Jung, M., Reichstein, M., Ciais, P., Seneviratne, S. I., Sheffield, J., Goulden, M. L., et al. (2010). Recent decline in the global land evapotranspiration trend due to limited moisture supply. Nature 467, 951–954. doi: 10.1038/nature09396
Karam, F., Amacha, N., Fahed, S., El Asmar, T., and Domínguez, A. (2014). Response of potato to full and deficit irrigation under semiarid climate: agronomic and economic implications. Agric. Water Manage. 142, 144–151. doi: 10.1016/j.agwat.2014.05.007
Khan, K., Krakauer, N. Y., and Rahman, L. (2012). Using AVHRR-based vegetation health indices for estimation of potato yield in Bangladesh. J. Civil Environ. Eng. 2:111. doi: 10.4172/2165-784X.1000111
Khayatnezhad, M., Shahriari, R., Gholamin, R., Jamaati-e-Somarin, S., and Zabihi-e-Mahmoodabad, R. (2011). Correlation and path analysis between yield and yield components in potato (Solanum tubersum L.). Middle East J. Sci. Res. 7, 17–21.
Kleinman, P. J. A., Sharpley, A. N., McDowell, R. W., Flaten, D. N., Buda, A. R., Tao, L., et al. (2011). Managing agricultural phosphorus for water quality protection: principles for progress. Plant Soil 349, 169–182. doi: 10.1007/s11104-011-0832-9
Knowles, N. R., and Knowles, L. O. (2006). Manipulating stem number, tuber set, and yield relationships for northern- and southern-grown potato seed lots. Crop Sci. 46, 284–296. doi: 10.2135/cropsci2005.05-0078
Konikow, L. F. (2015). Long-term groundwater depletion in the United States. Groundwater 53, 2–9. doi: 10.1111/gwat.12306
Kooistra, L., and Clevers, J. G. P. W. (2016). Estimating potato leaf chlorophyll content using ratio vegetation indices. Remote Sens. Lett. 7, 611–620. doi: 10.1080/2150704X.2016.1171925
Kuenzer, C., and Dech, S. (2013). Thermal Infrared Remote Sensing: Sensors, Methods, Applications, Vol. 17. Dordrecht: Springer. doi: 10.1007/978-94-007-6639-6
Lawlor, D. W., Kontturi, M., and Young, A. T. (1989). Photosynthesis by flag leaves of wheat in relation to protein, ribulose bisphosphate carboxylase activity and nitrogen supply. J. Exp. Bot. 40, 43–52. doi: 10.1093/jxb/40.1.43
Li, B., Xu, X., Han, J., Zhang, L., Bian, C., Jin, L., et al. (2019). The estimation of crop emergence in potatoes by UAV RGB imagery. Plant Methods 15:15. doi: 10.1186/s13007-019-0399-7
Li, B., Xu, X., Zhang, L., Han, J., Bian, C., Li, G., et al. (2020). Above-ground biomass estimation and yield prediction in potato by using UAV-based RGB and hyperspectral imaging. ISPRS J. Photogramm. Remote Sens. 162, 161–172. doi: 10.1016/j.isprsjprs.2020.02.013
Li, C., Chen, P., Ma, C., Feng, H., Wei, F., Wang, Y., et al. (2020). Estimation of potato chlorophyll content using composite hyperspectral index parameters collected by an unmanned aerial vehicle. Int. J. Remote Sens. 41, 8176–8197. doi: 10.1080/01431161.2020.1757779
Li, D., Quan, C., Song, Z., Li, X., Yu, G., Li, C., et al. (2021b). High-throughput plant phenotyping platform (HT3P) as a novel tool for estimating agronomic traits from the lab to the field. Front. Bioeng. Biotechnol. 8:623705. doi: 10.3389/fbioe.2020.623705
Li, D., Miao, Y., Gupta, S. K., Rosen, C. J., Yuan, F., Wang, C., et al. (2021a). Improving potato yield prediction by combining cultivar information and UAV remote sensing data using machine learning. Remote Sens. 13:3322. doi: 10.3390/rs13163322
Li, L., Zhang, Q., and Huang, D. (2014). A review of imaging techniques for plant phenotyping. Sensors 14, 20078–20111. doi: 10.3390/s141120078
Li, W., Liu, C., Yang, Y., Awais, M., Li, W., Ying, P., et al. (2022). A UAV-aided prediction system of soil moisture content relying on thermal infrared remote sensing. Int. J. Environ. Sci. Technol. 1–14. doi: 10.1007/s13762-022-03958-7
Li, W., Niu, Z., Chen, H., Li, D., Wu, M., and Zhao, W. (2016). Remote estimation of canopy height and aboveground biomass of maize using high-resolution stereo images from a low-cost unmanned aerial vehicle system. Ecol. Indic. 67, 637–648. doi: 10.1016/j.ecolind.2016.03.036
Lin, Y.-C., Cheng, Y.-T., Zhou, T., Ravi, R., Hasheminasab, S. M., Flatt, J. E., et al. (2019). Evaluation of UAV LiDAR for mapping coastal environments. Remote Sens. 11:2893. doi: 10.3390/rs11242893
Liu, N., Xing, Z., Zhao, R., Qiao, L., Li, M., Liu, G., et al. (2020). Analysis of chlorophyll concentration in potato crop by coupling continuous wavelet transform and spectral variable optimization. Remote Sens. 12:2826. doi: 10.3390/rs12172826
Lu, S., Xuan, J., Zhang, T., Bai, X., Tian, F., and Ortega-Farias, S. (2022). Effect of the shadow pixels on evapotranspiration inversion of vineyard: a high-resolution UAV-based and ground-based remote sensing measurements. Remote Sens. 14:2259. doi: 10.3390/rs14092259
Luo, S., He, Y., Li, Q., Jiao, W., Zhu, Y., and Zhao, X. (2020). Nondestructive estimation of potato yield using relative variables derived from multi-period LAI and hyperspectral data based on weighted growth stage. Plant Methods 16:150. doi: 10.1186/s13007-020-00693-3
Lynch, J., Marschner, P., and Rengel, Z. (2012). “Effect of internal and external factors on root growth and development,” in Marschner’s Mineral Nutrition of Higher Plants, 3rd Edn. ed. P. Marschner (London: Academic Press.), 331–346. doi: 10.1016/B978-0-12-384905-2.00013-3
Ma, Y., Zhang, Z., Kang, Y., and Özdoğan, M. (2021). Corn yield prediction and uncertainty analysis based on remotely sensed variables using a Bayesian neural network approach. Remote Sens. Environ. 259:112408.
Machefer, M., Lemarchand, F., Bonnefond, V., Hitchins, A., and Sidiropoulos, P. (2020). Mask R-CNN refitting strategy for plant counting and sizing in UAV imagery. Remote Sens. 12:3015. doi: 10.3390/rs12183015
Molahlehi, L., Steyn, J. M., and Haverkort, A. J. (2013). Potato crop response to genotype and environment in a subtropical highland agro-ecology. Potato Res. 56, 237–258. doi: 10.1007/s11540-013-9241-1
Moran, M. S., Inoue, Y., and Barnes, E. M. (1997). Opportunities and limitations for image-based remote sensing in precision crop management. Remote Sens. Environ. 61, 319–346. doi: 10.1016/S0034-4257(97)00045-X
Mourad, R., Jaafar, H., Anderson, M., and Gao, F. (2020). Assessment of leaf area index models using harmonized Landsat and Sentinel-2 surface reflectance data over a semi-arid irrigated landscape. Remote Sens. 12:3121. doi: 10.3390/rs12193121
Mulla, D. J. (2013). Twenty five years of remote sensing in precision agriculture: key advances and remaining knowledge gaps. Biosyst. Eng. 114, 358–371. doi: 10.1016/j.biosystemseng.2012.08.009
Nebiker, S., Lack, N., Abächerli, M., and Läderach, S. (2016). Light-weight multispectral UAV sensors and their capabilities for predicting grain yield and detecting plant diseases. Int. Arch. Photogramm. Remote Sens. Spat. Inf. Sci. XLI-B1, 963–970. doi: 10.5194/isprs-archives-XLI-B1-963-2016
Newton, I. H., Tariqul Islam, A. F. M., Saiful Islam, A. K. M., Tarekul Islam, G. M., Tahsin, A., and Razzaque, S. (2018). Yield prediction model for potato using landsat time series images driven vegetation indices. Remote Sens. Earth Syst. Sci. 1, 29–38. doi: 10.1007/s41976-018-0006-0
Nigon, T. J., Mulla, D. J., Rosen, C. J., Cohen, Y., Alchanatis, V., Knight, J., et al. (2015). Hyperspectral aerial imagery for detecting nitrogen stress in two potato cultivars. Comput. Electron. Agric. 112, 36–46. doi: 10.1016/j.compag.2014.12.018
Nigon, T. J., Mulla, D. J., Rosen, C. J., Cohen, Y., Alchanatis, V., and Rud, R. (2014). Evaluation of the nitrogen sufficiency index for use with high resolution, broadband aerial imagery in a commercial potato field. Precis. Agric. 15, 202–226. doi: 10.1007/s11119-013-9333-6
Nikolakopoulos, K. G., Soura, K., Koukouvelas, I. K., and Argyropoulos, N. G. (2017). UAV vs classical aerial photogrammetry for archaeological studies. J. Archaeol. Sci. Rep. 14, 758–773. doi: 10.1016/j.jasrep.2016.09.004
Oppenheim, D., and Shani, G. (2017). Potato disease classification using convolution neural networks. Adv. Anim. Biosci. 8, 244–249. doi: 10.1017/S2040470017001376
Ortiz, O., and Mares, V. (2017). “The historical, social, and economic importance of the potato crop,” in The Potato Genome, eds S. Kumar Chakrabarti, C. Xie, and J. Kumar Tiwari (Cham: Springer International Publishing), 1–10. doi: 10.1007/978-3-319-66135-3_1
Papadavid, G. C., Hadjimitsis, D. G., Toulios, L., and Michaelides, S. (2011). Mapping potato crop height and leaf area index through vegetation indices using remote sensing in Cyprus. J. Appl. Remote Sens. 5:053526. doi: 10.1117/1.3596388
Pask, A., Pietragalla, J., Mullan, D., and Reynolds, M. P. (2012). Physiological Breeding II: A Field Guide to Wheat Phenotyping. Mexico: CIMMYT.
Pavón-Pulido, N., López-Riquelme, J. A., Torres, R., Morais, R., and Pastor, J. A. (2017). New trends in precision agriculture: a novel cloud-based system for enabling data storage and agricultural task planning and automation. Precis. Agric. 18, 1038–1068. doi: 10.1007/s11119-017-9532-7
Pei, H., Feng, H., Li, C., Yang, G., Wu, Z., and Liu, M. (2019). “Estimation of aboveground biomass of potato based on ground hyperspectral,” in Proceedings of the 2019 8th International Conference on Agro-Geoinformatics (Agro-Geoinformatics), Istanbul, 1–4. doi: 10.1109/Agro-Geoinformatics.2019.8820542
Peng, J., Manevski, K., Kørup, K., Larsen, R., Zhou, Z., and Andersen, M. N. (2021). Environmental constraints to net primary productivity at northern latitudes: a study across scales of radiation interception and biomass production of potato. Int. J. Appl. Earth Obs. Geoinf. 94:102232. doi: 10.1016/j.jag.2020.102232
Polder, G., Blok, P. M., De Villiers, H. A. C., Van Der Wolf, J. M., and Kamp, J. (2019). Potato virus Y detection in seed potatoes using deep learning on hyperspectral images. Front. Plant Sci. 10:209. doi: 10.3389/fpls.2019.00209
Ramírez, D. A., Rolando, J. L., Yactayo, W., Monneveux, P., Mares, V., and Quiroz, R. (2015). Improving potato drought tolerance through the induction of long-term water stress memory. Plant Sci. 238, 26–32. doi: 10.1016/j.plantsci.2015.05.016
Ramírez, D. A., Yactayo, W., Gutiérrez, R., Mares, V., De Mendiburu, F., Posadas, A., et al. (2014). Chlorophyll concentration in leaves is an indicator of potato tuber yield in water-shortage conditions. Sci. Hortic. 168, 202–209. doi: 10.1016/j.scienta.2014.01.036
Ramírez, D. A., Yactayo, W., Rens, L. R., Rolando, J. L., Palacios, S., De Mendiburu, F., et al. (2016). Defining biological thresholds associated to plant water status for monitoring water restriction effects: stomatal conductance and photosynthesis recovery as key indicators in potato. Agric. Water Manage. 177, 369–378. doi: 10.1016/j.agwat.2016.08.028
Raun, W. R., Solie, J. B., Johnson, G. V., Stone, M. L., Mullen, R. W., Freeman, K. W., et al. (2002). Improving nitrogen use efficiency in cereal grain production with optical sensing and variable rate application. Agron. J. 94, 815–820.
Ray, S. S., Jain, N., Arora, R. K., Chavan, S., and Panigrahy, S. (2011). Utility of hyperspectral data for potato late blight disease detection. J. Indian Soc. Rem. Sens. 39:161. doi: 10.1007/s12524-011-0094-2
Reyes-González, A., Kjaersgaard, J., Trooien, T., Reta-Sánchez, D. G., Sánchez-Duarte, J. I., Preciado-Rangel, P., et al. (2019). Comparison of leaf area index, surface temperature, and actual evapotranspiration estimated using the metric model and in situ measurements. Sensors 19:1857. doi: 10.3390/s19081857
Rinza, J., Ramírez, D. A., García, J., De Mendiburu, F., Yactayo, W., Barreda, C., et al. (2019). Infrared radiometry as a tool for early water deficit detection: insights into its use for establishing irrigation calendars for potatoes under humid conditions. Potato Res. 62, 109–122. doi: 10.1007/s11540-018-9400-5
Rippa, M., Battaglia, V., Cermola, M., Sicignano, M., Lahoz, E., and Mormile, P. (2022). Monitoring of the copper persistence on plant leaves using pulsed thermography. Environ. Monit. Assess. 194:160. doi: 10.1007/s10661-022-09807-x
Roosjen, P. P. J., Brede, B., Suomalainen, J. M., Bartholomeus, H. M., Kooistra, L., and Clevers, J. G. P. W. (2018). Improved estimation of leaf area index and leaf chlorophyll content of a potato crop using multi-angle spectral data – potential of unmanned aerial vehicle imagery. Int. J. Appl. Earth Obs. Geoinf. 66, 14–26. doi: 10.1016/j.jag.2017.10.012
Roosjen, P. P. J., Suomalainen, J. M., Bartholomeus, H. M., and Clevers, J. G. P. W. (2016). Hyperspectral reflectance anisotropy measurements using a pushbroom spectrometer on an unmanned aerial vehicle—results for barley, winter wheat, and potato. Remote Sens. 8:909. doi: 10.3390/rs8110909
Roosjen, P. P. J., Suomalainen, J. M., Bartholomeus, H. M., Kooistra, L., and Clevers, J. G. P. W. (2017). Mapping reflectance anisotropy of a potato canopy using aerial images acquired with an unmanned aerial vehicle. Remote Sens. 9:417. doi: 10.3390/rs9050417
Rud, R., Cohen, Y., Alchanatis, V., Levi, A., Brikman, R., Shenderey, C., et al. (2014). Crop water stress index derived from multi-year ground and aerial thermal images as an indicator of potato water status. Precis. Agric. 15, 273–289. doi: 10.1007/s11119-014-9351-z
Salvador, P., Gómez, D., Sanz, J., and Casanova, J. L. (2020). Estimation of potato yield using satellite data at a municipal level: a machine learning approach. ISPRS Int. J. Geoinf. 9:343. doi: 10.3390/ijgi9060343
Sanchez, P. D. C., Hashim, N., Shamsudin, R., and Mohd Nor, M. Z. (2020). Applications of imaging and spectroscopy techniques for non-destructive quality evaluation of potatoes and sweet potatoes: a review. Trends Food Sci. Technol. 96, 208–221. doi: 10.1016/j.tifs.2019.12.027
Sankaran, S., Khot, L. R., Espinoza, C. Z., Jarolmasjed, S., Sathuvalli, V. R., Vandemark, G. J., et al. (2015). Low-altitude, high-resolution aerial imaging systems for row and field crop phenotyping: a review. Eur. J. Agron. 70, 112–123. doi: 10.1016/j.eja.2015.07.004
Sankaran, S., Quirós, J. J., Knowles, N. R., and Knowles, L. O. (2017). High-resolution aerial imaging based estimation of crop emergence in potatoes. Am. J. Potato Res. 94, 658–663. doi: 10.1007/s12230-017-9604-2
Say, S., Keskin, M., Sehri, M., and Sekerli, Y. (2017). Adoption of precision agriculture technologies in developed and developing countries. Online J. Sci. Technol. 8, 7–15. doi: 10.1093/jxb/err064
Shayanowako, A., Mangani, R., Mtaita, T., and Mazarura, U. (2014). Influence of main stem density on Irish potato growth and yield: a review. Annu. Res. Rev. Biol. 5, 229–237. doi: 10.9734/ARRB/2015/9973
Siebring, J., Valente, J., Domingues Franceschini, M. H., Kamp, J., and Kooistra, L. (2019). Object-based image analysis applied to low altitude aerial imagery for potato plant trait retrieval and pathogen detection. Sensors 19:5477. doi: 10.3390/s19245477
Sishodia, R. P., Shukla, S., Graham, W. D., Wani, S. P., Jones, J. W., and Heaney, J. (2017). Current and future groundwater withdrawals: effects, management and energy policy options for a semi-arid Indian watershed. Adv. Water Resour. 110, 459–475. doi: 10.1016/j.advwatres.2017.05.014
Sugiura, R., Tsuda, S., Tamiya, S., Itoh, A., Nishiwaki, K., Murakami, N., et al. (2016). Field phenotyping system for the assessment of potato late blight resistance using RGB imagery from an unmanned aerial vehicle. Biosyst. Eng. 148, 1–10. doi: 10.1016/j.biosystemseng.2016.04.010
Sugiura, R., Tsuda, S., Tsuji, H., and Murakami, N. (2018). “Virus-infected plant detection in potato seed production field by UAV imagery,” in Proceedings of the 2018 ASABE Annual International Meeting, Detroit, MI. doi: 10.13031/aim.201800594
Suh, H. K., Hofstee, J. W., IJsselmuiden, J., and Van Henten, E. J. (2018). Sugar beet and volunteer potato classification using bag-of-visual-words model, scale-invariant feature transform, or speeded up robust feature descriptors and crop row information. Biosyst. Eng. 166, 210–226. doi: 10.1016/j.biosystemseng.2017.11.015
Sun, C., Feng, L., Zhang, Z., Ma, Y., Crosby, T., Naber, M., et al. (2020). Prediction of end-of-season tuber yield and tuber set in potatoes using in-season UAV-based hyperspectral imagery and machine learning. Sensors 20:5293. doi: 10.3390/s20185293
Suomalainen, J., Anders, N., Iqbal, S., Roerink, G., Franke, J., Wenting, P., et al. (2014). A lightweight hyperspectral mapping system and photogrammetric processing chain for unmanned aerial vehicles. Remote Sens. 6, 11013–11030. doi: 10.3390/rs61111013
Tanabe, D., Ichiura, S., Nakatsubo, A., Kobayashi, T., and Katahira, M. (2019). “Yield prediction of potato by unmanned aerial vehicle,” in Proceedings of the 2019 International Conference on Trends in Agricultural Engineering, Prague.
Taylor, R. J., Pasche, J. S., and Gudmestad, N. C. (2008). Susceptibility of eight potato cultivars to tuber infection by Phytophthora erythroseptica and Pythium ultimum and its relationship to mefenoxam-mediated control of pink rot and leak. Ann. Appl. Biol. 152, 189–199. doi: 10.1111/j.1744-7348.2007.00203.x
ten Harkel, J., Bartholomeus, H., and Kooistra, L. (2020). Biomass and crop height estimation of different crops using UAV-based Lidar. Remote Sens. 12:17. doi: 10.3390/rs12010017
Théau, J., Gavelle, E., and Ménard, P. (2020). Crop scouting using UAV imagery: a case study for potatoes. J. Unmanned Veh. Syst. 8, 99–118. doi: 10.1139/juvs-2019-0009
U. S. Department of Agriculture (2020). National Agricultural Statistics Service. Available online at: https://www.nass.usda.gov/Statistics_by_State/Washington/Publications/Potatoes/2020/PT09_1.pdf (accessed June 1, 2022).
Van De Vijver, R., Mertens, K., Heungens, K., Somers, B., Nuyttens, D., Borra-Serrano, I., et al. (2020). In-field detection of Alternaria solani in potato crops using hyperspectral imaging. Comput. Electron. Agric. 168:105106.
Van Evert, F. K., Van Der Voet, P., Van Valkengoed, E., Kooistra, L., and Kempenaar, C. (2012). Satellite-based herbicide rate recommendation for potato haulm killing. Eur. J. Agron. 43, 49–57. doi: 10.1016/j.eja.2012.05.004
Vesali, F., Omid, M., Mobli, H., and Kaleita, A. (2017). Feasibility of using smart phones to estimate chlorophyll content in corn plants. Photosynthetica 55, 603–610. doi: 10.1007/s11099-016-0677-9
Viña, A., Gitelson, A. A., Nguy-Robertson, A. L., and Peng, Y. (2011). Comparison of different vegetation indices for the remote assessment of green leaf area index of crops. Remote Sens. Environ. 115, 3468–3478. doi: 10.1016/j.rse.2011.08.010
Vítek, P., Veselá, B., and Klem, K. (2020). Spatial and temporal variability of plant leaf responses cascade after PSII inhibition: Raman, chlorophyll fluorescence and infrared thermal imaging. Sensors 20:1015. doi: 10.3390/s20041015
Wang, X., Miao, Y., Dong, R., Zha, H., Xia, T., Chen, Z., et al. (2021). Machine learning-based in-season nitrogen status diagnosis and side-dress nitrogen recommendation for corn. Eur. J. Agron. 123:126193. doi: 10.1016/j.eja.2020.126193
Wang, Y., Zhang, Z., Feng, L., Ma, Y., and Du, Q. (2021). A new attention-based CNN approach for crop mapping using time series Sentinel-2 images. Comput. Electron. Agric. 184:106090.
Williams, D., Aitkenhead, M., Karley, A. J., Graham, J., and Jones, H. G. (2018). “Use of imaging technologies for high throughput phenotyping,” in Raspberry: Breeding, Challenges and Advances, eds J. Graham and R. Brennan (Cham: Springer International Publishing), 145–158. doi: 10.1007/978-3-319-99031-6_9
Wolff, W., Francisco, J. P., Flumignan, D. L., Marin, F. R., and Folegatti, M. V. (2022). Optimized algorithm for evapotranspiration retrieval via remote sensing. Agric. Water Manage. 262:107390. doi: 10.1016/j.agwat.2021.107390
Wu, D., and Sun, D.-W. (2013). Advanced applications of hyperspectral imaging technology for food quality and safety analysis and assessment: a review—part I: fundamentals. Innov. Food Sci. Emerg. Technol. 19, 1–14. doi: 10.1016/j.ifset.2013.04.014
Xie, C., and Yang, C. (2020). A review on plant high-throughput phenotyping traits using UAV-based sensors. Comput. Electron. Agric. 178:105731. doi: 10.1016/j.compag.2020.105731
Yadav, S. P., Ibaraki, Y., and Dutta Gupta, S. (2010). Estimation of the chlorophyll content of micropropagated potato plants using RGB based image analysis. Plant Cell Tissue Organ Cult. 100, 183–188. doi: 10.1007/s11240-009-9635-6
Yang, H., Li, F., Wang, W., and Yu, K. (2021). Estimating above-ground biomass of potato using random forest and optimized hyperspectral indices. Remote Sens. 13:2339. doi: 10.3390/rs13122339
Zaeen, A. A., Sharma, L., Jasim, A., Bali, S., Buzza, A., and Alyokhin, A. (2020). In-season potato yield prediction with active optical sensors. Agrosyst. Geosci. Environ. 3:e20024. doi: 10.1002/agg2.20024
Zebarth, B. J., and Rosen, C. J. (2007). Research perspective on nitrogen BMP development for potato. Am. J. Potato Res. 84, 3–18. doi: 10.1007/BF02986294
Zhang, C., Marzougui, A., and Sankaran, S. (2020). High-resolution satellite imagery applications in crop phenotyping: an overview. Comput. Electron. Agric. 175:105584. doi: 10.1016/j.compag.2020.105584
Zhang, F., Hassanzadeh, A., Kikkert, J., Pethybridge, S. J., and van Aardt, J. (2021). Comparison of UAS-based structure-from-motion and LiDAR for structural characterization of short broadacre crops. Remote Sens. 13:3975. doi: 10.3390/rs13193975
Zheng, T., Liu, N., Wu, L., Li, M., Sun, H., Zhang, Q., et al. (2018). Estimation of chlorophyll content in potato leaves based on spectral red edge position. IFAC PapersOnline 51, 602–606. doi: 10.1016/j.ifacol.2018.08.131
Zhou, J., Zhou, J., Ye, H., Ali, M. L., Chen, P., and Nguyen, H. T. (2021b). Yield estimation of soybean breeding lines under drought stress using unmanned aerial vehicle-based imagery and convolutional neural network. Biosyst. Eng. 204, 90–103. doi: 10.1016/j.biosystemseng.2021.01.017
Zhou, J., Mou, H., Zhou, J., Ali, M. L., Ye, H., Chen, P., et al. (2021a). Qualification of soybean responses to flooding stress using uav-based imagery and deep learning. Plant Phenomics 2021, 9892570. doi: 10.34133/2021/9892570
Zhou, J., Zhou, J., Ye, H., Ali, M. L., Nguyen, H. T., and Chen, P. (2020). Classification of soybean leaf wilting due to drought stress using UAV-based imagery. Comput. Electron. Agric. 175:105576. doi: 10.1016/j.compag.2020.105576
Zhou, L., Chen, N., Chen, Z., and Xing, C. (2016b). ROSCC: an efficient remote sensing observation-sharing method based on cloud computing for soil moisture mapping in precision agriculture. IEEE J. Sel. Top. Appl. Earth Obs. Remote Sens. 9, 5588–5598. doi: 10.1109/JSTARS.2016.2574810
Zhou, J., Pavek, M. J., Shelton, S. C., Holden, Z. J., and Sankaran, S. (2016a). Aerial multispectral imaging for crop hail damage assessment in potato. Comput. Electron. Agric. 127, 406–412.
Zhou, Z., Andersen, M. N., and Plauborg, F. (2016c). Radiation interception and radiation use efficiency of potato affected by different N fertigation and irrigation regimes. Eur. J. Agron. 81, 129–137. doi: 10.1016/j.eja.2016.09.007
Zhou, Z., Jabloun, M., Plauborg, F., and Andersen, M. N. (2018). Using ground-based spectral reflectance sensors and photography to estimate shoot N concentration and dry matter of potato. Comput. Electron. Agric. 144, 154–163. doi: 10.1016/j.compag.2017.12.005
Keywords: precision agriculture, remote sensing, satellite imagery, potato, unmanned aerial system, sensors
Citation: Sun C, Zhou J, Ma Y, Xu Y, Pan B and Zhang Z (2022) A review of remote sensing for potato traits characterization in precision agriculture. Front. Plant Sci. 13:871859. doi: 10.3389/fpls.2022.871859
Received: 08 February 2022; Accepted: 29 June 2022;
Published: 18 July 2022.
Edited by:
Zhihan Lv, Uppsala University, SwedenReviewed by:
Ankush Prashar, Newcastle University, United KingdomPenghao Wang, Murdoch University, Australia
Copyright © 2022 Sun, Zhou, Ma, Xu, Pan and Zhang. This is an open-access article distributed under the terms of the Creative Commons Attribution License (CC BY). The use, distribution or reproduction in other forums is permitted, provided the original author(s) and the copyright owner(s) are credited and that the original publication in this journal is cited, in accordance with accepted academic practice. No use, distribution or reproduction is permitted which does not comply with these terms.
*Correspondence: Zhou Zhang, zzhang347@wisc.edu
†These authors have contributed equally to this work