- 1Department of Biotechnology, College of Life Science and Technology, Huazhong University of Science and Technology, Wuhan, China
- 2School of Agricultural Sciences, Zhengzhou University, Zhengzhou, China
Rapeseed is the second most important oil crop in the world. Improving seed yield and seed oil content are the two main highlights of the research. Unfortunately, rapeseed development is frequently affected by different diseases. Extensive research has been made through many years to develop elite cultivars with high oil, high yield, and/or disease resistance. Quantitative trait locus (QTL) analysis has been one of the most important strategies in the genetic deciphering of agronomic characteristics. To comprehend the distribution of these QTLs and to uncover the key regions that could simultaneously control multiple traits, 4,555 QTLs that have been identified during the last 25 years were aligned in one unique map, and a quantitative genomic map which involved 128 traits from 79 populations developed in 12 countries was constructed. The present study revealed 517 regions of overlapping QTLs which harbored 2,744 candidate genes and might affect multiple traits, simultaneously. They could be selected to customize super-rapeseed cultivars. The gene ontology and the interaction network of those candidates revealed genes that highly interacted with the other genes and might have a strong influence on them. The expression and structure of these candidate genes were compared in eight rapeseed accessions and revealed genes of similar structures which were expressed differently. The present study enriches our knowledge of rapeseed genome characteristics and diversity, and it also provided indications for rapeseed molecular breeding improvement in the future.
Introduction
Rapeseed (Brassica napus, AACC = 38) is a tetraploid species derived from the natural hybridization between turnip rape (B. rapa, AA = 20) and cabbage (B. oleracea, CC = 18) (Nagaharu, 1935; Al-Shehbaz et al., 2006; Chalhoub et al., 2014). Both Brassica species and the model plant Arabidopsis thaliana belong to the Brassicaceae family; their separation took place about 14–20 million years ago (Yang et al., 1999; Beilstein et al., 2006). Rapeseed is the second most important oil crop in the world, which could supply 13% of the world’s vegetable oil (Hajduch et al., 2006; Amar et al., 2009).
Rapeseed utilization is not limited to oil sources, it also can be used for food and energy production, remediation, and as sightseeing attraction (Raboanatahiry et al., 2021). To fulfill the global high demand for oil, the main objectives of researchers are to discover ways to increase the oil content and to develop high-yielding varieties, to succeed in sustainable manufacture in the future. Unfortunately, abiotic and biotic factors frequently weaken the rapeseed development, such as the invasion of Sclerotinia sclerotiorum (steam rot) and Plasmodiophora brassicae (blackleg disease), which resulted in yield losses of 10–80% (Wang Z. et al., 2014) and 20–30% (Wang et al., 2011), respectively, in China. Besides, drought is one of the most devastating abiotic stresses for seed yield, which affects 40% of the worldwide land area (Zhang et al., 2014).
It would be of great interest to find the genetic loci that could control the traits associated with seed yield and quality, and disease traits, simultaneously, for artificial selection breeding. Rapeseed has experienced selection which contributed to the diversification of winter and spring types. The selection also caused region restructuring where genes controlling agronomically important traits are located. Thus, intensive breeding allowed to optimize those important traits such as oil content, flowering time, and pathogen resistance (Chalhoub et al., 2014). Diversity in the same species is because every individual has their uniqueness starting from their genome and it is reflected into their trait characteristics. Diversity among different species could be understood by analyzing their genomes. Variations within the genome are a reflection of breeding events. Genome diversity might be exploited to detect beneficial phenotypes associated with specific loci on the genome and linked to environmental conditions.
Quantitative trait loci (QTLs) are correlated with variations of phenotype and are extensively used for agronomic trait analysis and in plant breeding. Lots of phenotypic traits are usually responsible for the improvement of most crops, they are quantitative in nature, and are influenced both by many genes and environmental conditions (Zhu and Zhao, 2007). QTL mapping could be used to decipher regulatory loci and genetic mechanism of traits (Paran and Zamir, 2003) to identify the genomic regions which are responsible for trait variation, and to establish a link between phenotype and polymorphic markers in random biparental populations (Cao et al., 2010; Jiang et al., 2014). Several research works have revealed that the phenotypic effect of QTLs for one character in one genetic background might produce a different phenotypic effect in another genetic background. For example, KN (KenC-8 × N53-2) and TN (Tapidor × Ningyou7) are two populations that were both cultivated in China, the oil content (OC) and detected QTLs were not similar: 41 QTLs for OC were found with a maximum OC of 50.9% in the 202 TN-DH lines (Jiang et al., 2014), whereas in the 348 KN-DH lines, 63 OC-QTLs were found with maximum OC of 54.8% (Wang et al., 2013), and in 300 KN-DH lines, 67 QTLs were detected with a maximum OC of 57.17% (Chao et al., 2017). To uncover the similarities and differences between the discovered QTLs, a consensus map that displays multiple QTLs from different genetic and environmental backgrounds is indispensable.
Earlier, building a consensus map was possible, but it was limited by the difference of markers that were used in different studies (Raman et al., 2013). Now, it can be overcome with a QTL alignment map which has been used for seed oil content and seed yield QTLs by using B. napus Darmor-bzh as the reference genome (Liu S. et al., 2016; Chao et al., 2017; Raboanatahiry et al., 2017, 2018). The advantage of QTL alignment is to allow the easy comparison between QTLs and the regions of overlapping QTLs and can be used to uncover the “stable” or “specified” regions for a trait or an environment, but also to detect the pleiotropic loci, i.e., regions that control multiple traits, simultaneously. In our previous studies, regions of overlapping QTLs were displayed: on one hand, the regions involved QTLs of the same traits but originated from different populations (e.g., OC-QTL from KN and TN which were both cultivated in China and overlapped in the same region), these regions can be qualified as “stable” for Chinese environment, despite the change in populations, or if the QTLs were from two populations which were developed in two different environments (e.g., KN in China, and PT in Canada), these regions were “stable” for the studied trait (e.g., oil content). On another hand, QTLs of different traits which overlapped in the same region were also found, and they might have a pleiotropic effect for those multiple traits (Raboanatahiry et al., 2017, 2018).
Quantitative trait loci (QTLs) investigation and the discovery of related candidate genes can be done together, this strategy helps to comprehend the authority of these genes over traits (Remington and Purugganan, 2003; Zhu and Zhao, 2007). The identification of candidate genes implies the detection of important genes for agricultural and economic quantitative traits. Candidate genes are present within the QTL regions and are responsible for phenotype variation (Tabor et al., 2002). The effect of these genes on the variation of phenotype could be elucidated via investigation on the gene arrangement and the interaction of loci affecting this variation (Zhu and Zhao, 2007). This technique has already been used to identify potential candidate genes in B. napus Darmor-bzh, for instance, Chao et al. (2017) used this technique to identify potential candidate genes for seed oil content traits, and found 448 genes underlying 41 oil content QTLs. Moreover, 76 candidate genes were found for 57 QTLs for oil content and fatty acids (Raboanatahiry et al., 2017), and 147 candidate genes were discovered inside a region where 131 yield QTLs were overlapping (Raboanatahiry et al., 2018). Candidate genes can be manipulated to get the most beneficial gene combination to get the maximum profit, especially those genes which were found in the region of overlapping QTLs involving many traits. For instance, LPAT2 and LPAT5 were identified as candidate genes in the QTL interval for oil content in the KN population of B. napus (Wang et al., 2013; Chao et al., 2017), and the seeds of the mutants lpat2 and lpat5 lines displayed a decrease in oil content (Zhang K. et al., 2019).
Additionally, the release of various B. napus genome sequences: Darmor-bzh (Chalhoub et al., 2014), Tapidor (Bayer et al., 2017), Zhongshuang11—ZS11 (Sun et al., 2017), Gangan, Shengli, Zheyou7, Quinta, No2127, Westar, and 1689 other accessions (Song et al., 2021), has represented a precious resource, which will have a tremendous impact on understanding rapeseed accessions diversity, notably the structural variation of regions which are associated with agronomic traits.
In our previous study, regions of overlapping QTLs for a single trait were detected (e.g., oil content or seed yield). In this study, the purpose was to construct a quantitative genomic map and to detect genomic regions that might control multiple traits associated with seed, yield, hormones level, and diseases, simultaneously, and the related candidate genes were also identified. Moreover, the structural variation and gene expression levels of those candidate genes were studied in eight different accessions. Consequently, the ultimate objectives were: (1) to build a quantitative genomic map of QTLs associated with agronomic and disease-related traits to display overlapping regions with multiple traits; (2) to reveal the candidate genes within those regions of overlapping QTLs, to find genes that might have pleiotropic effects on seed composition, seed yield, hormones, and disease, and to analyze their interaction; (3) to identify the homologous of these candidate genes in eight rapeseed accessions and to analyze the genes expression and the structural variation. The present study would enhance the knowledge of rapeseed genome characteristics and diversity, the findings can be used to develop molecular markers associated with the studied traits, and also can provide some guidance for molecular design for breeding. Identified candidate genes might be used to target genomics-based improvement and better seed yield, seed composition, and disease resistance in the future.
Results
A Quantitative Genomic Map of Quantitative Trait Locus Controlling Seed Yield, Seed Components, Hormone Level, and Disease-Related Traits
A total of 4,555 QTLs of 128 agronomic and disease-related traits, developed from 79 different populations of three different ecotypes and grown in 12 different countries (Supplementary Table 1), were gathered and combined in one unique map (Figure 1). A total distance of 978.4 Mb on the physical map of Darmor-bzh was covered (Figure 2A, Supplementary Figure 1, and Supplementary Table 2). Further observation revealed that 2,695 and 1,860 QTLs were located on A and C genomes, respectively. A3 and C3 chromosomes contained the highest number of QTLs, with 430 and 399 QTLs, respectively. A9, A3, and C3 chromosomes contained the highest number of traits (80, 71, and 69 traits, respectively) (Figure 2A). Most QTLs for seed components, seed yield, hormones level, and disease-related traits were found in the A genome rather than in the C genome.
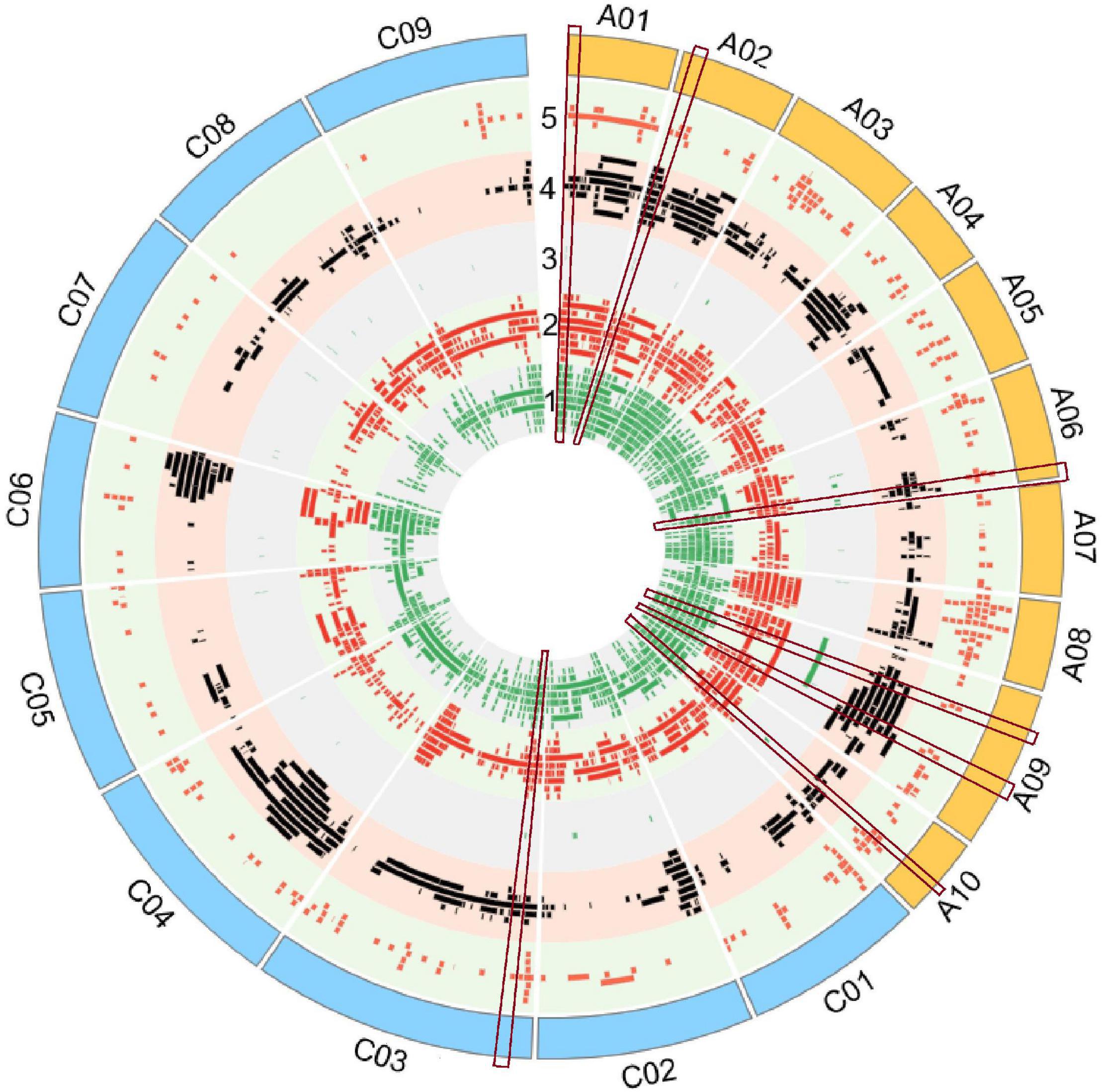
Figure 1. Quantitative trait loci (QTLs) alignment of agronomic and disease-related traits on the physical map of Darmor-bzh. QTLs were arranged in each track, from inner to outer circle according to their apparition on the physical map of Darmor-bzh. Track 1: Yield-related traits, track 2: Seed composition, track 3: hormone level, track 4: biotic factor, track 5: abiotic factor. The region of overlapping QTLs with five categories of traits is shown inside the red rectangular label. The map was built using Circos software.
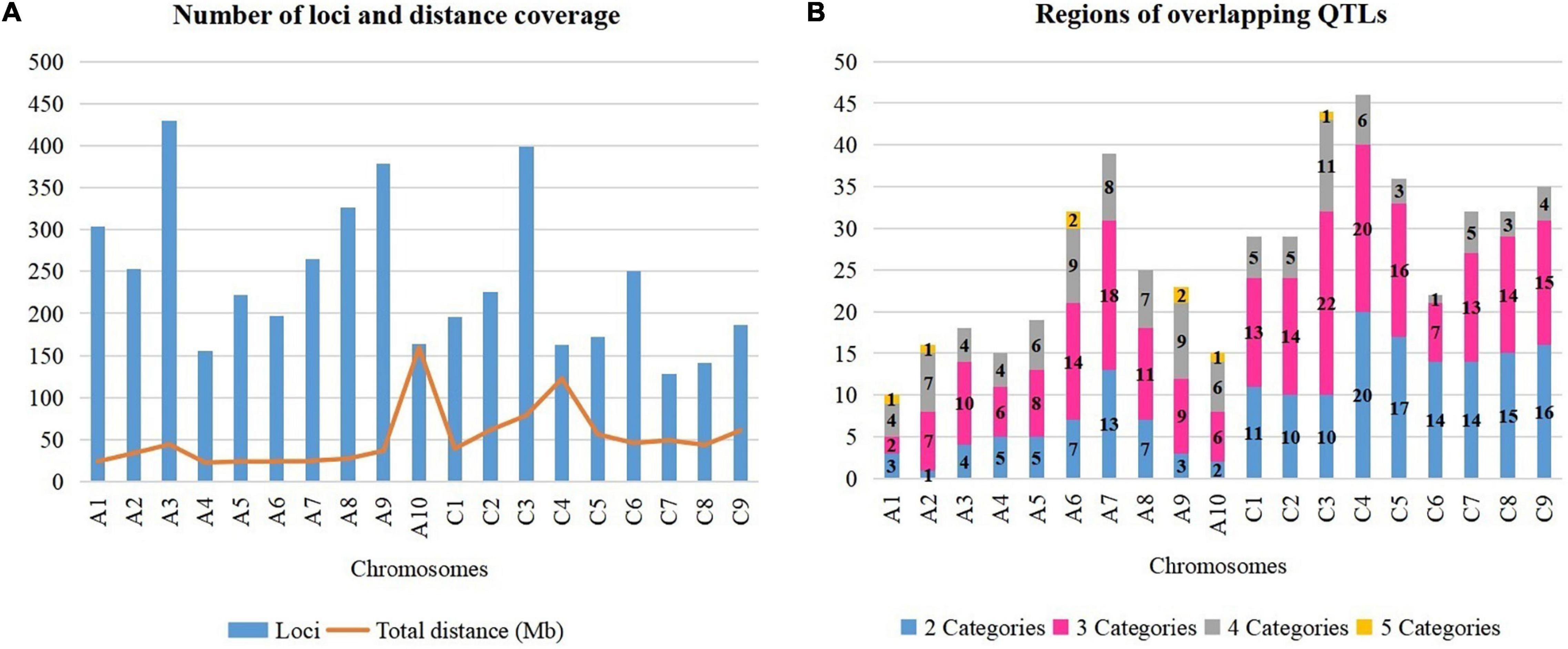
Figure 2. Dissection of rapeseed genome. (A) Number of loci and total distance. (B) Regions on chromosomes with overlapping QTLs.
It is crucial to locate regions of the genome where multiple traits overlapped the most. Thereby, the above-mentioned 128 traits were subdivided into five categories: 10 abiotic factor traits (A), nine biotic factor traits (B), four hormones related traits (H), 26 seed components traits (S), and 79 yield-related traits (Y). The total number of QTLs in each category were 349 (A), 334 (B), 42 (H), 1392 (S), and 2,438 (Y). Each region on Darmor-bzh genome was carefully observed to detect the regions where QTLs of more than one category of trait could overlap, i.e., regions with two, three, four, or five categories of traits, which were present in one region, simultaneously. A total of 517 regions that hosted overlapping QTLs were observed (Figure 2B and Supplementary Figure 1). The region of overlapping QTLs on each chromosome, the number of QTLs, and the categories of traits are summarized in Supplementary Table 3. First, eight regions were found to harbor all the five categories of studied traits (A, B, H, S, and Y) (Supplementary Table 4). Those eight regions were located on six chromosomes: one region was found on each of A1 (1.71–1.71 Mb, with 40 QTLs), A2 (2.31–2.31 Mb, with 20 QTLs), A10 (11.78–11.87 Mb, with 14 QTLs), and C3 (5.09–5.33 Mb, with 11 QTLs), and two regions were found on A6 (21.68–21.95 Mb with 15 QTLs and 22–22.30 Mb with 13 QTLs), and A9 (8.12–9.87 and 20.76–22.51 Mb, with 34 QTLs on each of them). Second, 107 regions that contained four categories of traits were found in all 19 chromosomes. The number of regions in each chromosome was, respectively, 11 on C3, nine on each of A6 and A9, eight on A7, seven on each of A2 and A8, six on each of A6, A10, and C4, five on C1 and C2, four on each of A1, A3, A4, and C9, three on each of C5 and C8, and one on C6. For example, 28 QTLs of four categories of traits (1A, 12B, 5S, 10Y) overlapped on A2 (1.49–2.31 Mb). Note that the region on A2 (1.71–22.04 Mb) included 288 overlapping QTLs (12A, 22B, 63S, and 191Y), which was the richest region of overlapping QTLs in B. napus genome. Third, 225 regions on all 19 chromosomes were found to have overlapping QTLs involving three categories of traits: 22 on C3, 20 on C4, 18 on A7, 16 on C5, 15 on C9, 14 on each of A6, C2, and C8, 13 on each of C1 and C7, 11 on A8, 10 on A3, nine on A9, eight on A5, seven on each of A2 and C6, six on each of A4 and A10, and two on A1. For instance, on a region of A5 (3.49–5.29 Mb), 40 QTLs of three categories of traits (5B, 12S, 23Y) overlapped. Fourth, 177 regions were found to contain overlapping QTLs which involved two categories of traits: 20 on C4, 17 on C5, 16 on C9, 15 on C8, 14 on each of C6 and C7, 13 on A7, 11 on C1, 10 on each of C2 and C3, seven on each of A6 and A8, five on each of A4 and A5, four on A3, three on A1 and A9, two on A10, and one on A2. As an example, 13 QTLs of two categories of traits (10B, 3Y) overlapped on C4 (20.66–20.70 Mb).
Note that some QTLs might overlap multiple times with other QTLs in different regions because of their extended length, for example, a QTL for C16:0 was located on A1 (2.25–19.86 Mb) and it could overlap two times with QTLs in the region which involved five and four categories (1.71–1.71 and 1.71–22.04 Mb, respectively). Then, the most abundant and the most overlapping categories of traits were S and Y categories, they were found in 403 among the 517 regions of overlapping QTLs detected in this study. OC and seed yield (SY) are the two most important traits in rapeseed, and the seed yield is determined by the number of seeds per siliques (SNP), the number of siliques per plant, the seed weight (Qzer et al., 1999; Quarrie et al., 2006; Chen et al., 2007), and the overlapping QTLs for OC and SY traits were observed in 82 regions (Supplementary Table 5), where the chromosome A9 had the richest regions with 11 regions of overlapping QTLs for OC and SY. The H category of the trait was found rarely in the overlapping region since the identified QTLs in early published papers were few (42 QTLs), so far, this H category was found in 39 among the 517 regions of the overlapping QTLs of this study. Otherwise, the regions of overlapping QTLs which involved one environment or one population were observed. No specified region was found exclusively for one population. Also, only specified regions of overlapping QTLs, which involved some populations developed exclusively in China, were found in 11 areas of the genome: four areas on C3 (36.94–37.27, 37.27–38.94, 39.94–40.21, and 41.40–46.52 Mb), two areas on A8 (16.87–17.37 and 17.37–18.00 Mb), and one area on each of A7 (17.48–18.48 Mb), A10 (0.14–1.64 Mb), C4 (42.73–44.22 Mb), C6 (8.43–9.43 Mb), and C7 (24.87–25.45 Mb) (Supplementary Table 3). For instance, the region on A8 (16.87–17.37 Mb) had four QTLs (3S, 1Y), which were all found with the Chinese experimental field. Besides, “hot QTL regions” had been detected in four locations on the rapeseed genome. Those regions were enriched with more than 100 QTLs which were aligned: on chromosome A1 (1.71–22.04 Mb), a total of 228 QTLs of four categories of traits (A, B, S, Y) was found. Chromosome A3 (0.63–6.75 Mb) had 139 QTLs of three categories of traits (B, S, Y), and A8 (9.63–11.12 Mb) contained 111 QTLs of four categories of traits (A, B, S, Y). Then, chromosome C6 (29.29–36.52 Mb) was enriched with 144 QTLs of three categories of traits (B, S, Y). Ultimately, the rapeseed genome had been finely dissected to unveil regions that harbored multiple traits, simultaneously. It would be crucial to couple those findings with the identification of genes that were located within those regions to understand the influence of those genes over those traits.
Candidate Genes Identified Within Regions of Overlapping Quantitative Trait Locus, and Their Interaction Network
Previous studies identified 439 genes that were related to oil formation (Raboanatahiry et al., 2017), 1,398 genes that were related to yield traits (Raboanatahiry et al., 2018), and 1,344 genes which were resistance genes (Dolatabadian et al., 2017). Thus, a total of 3,181 genes were selected because they correlated with the studied traits, and they were aligned to the physical map of Darmor-bzh to detect the candidate genes. A total of 2,744 candidate genes were found within overlapping QTLs of two to five categories of traits (Figure 3A and Supplementary Table 6). A total number of genes of 26 (1%), 729 (47%), 1,291 (27%), and 700 (25%) were found for five, four, three, and two categories of traits, respectively (Figure 3B).
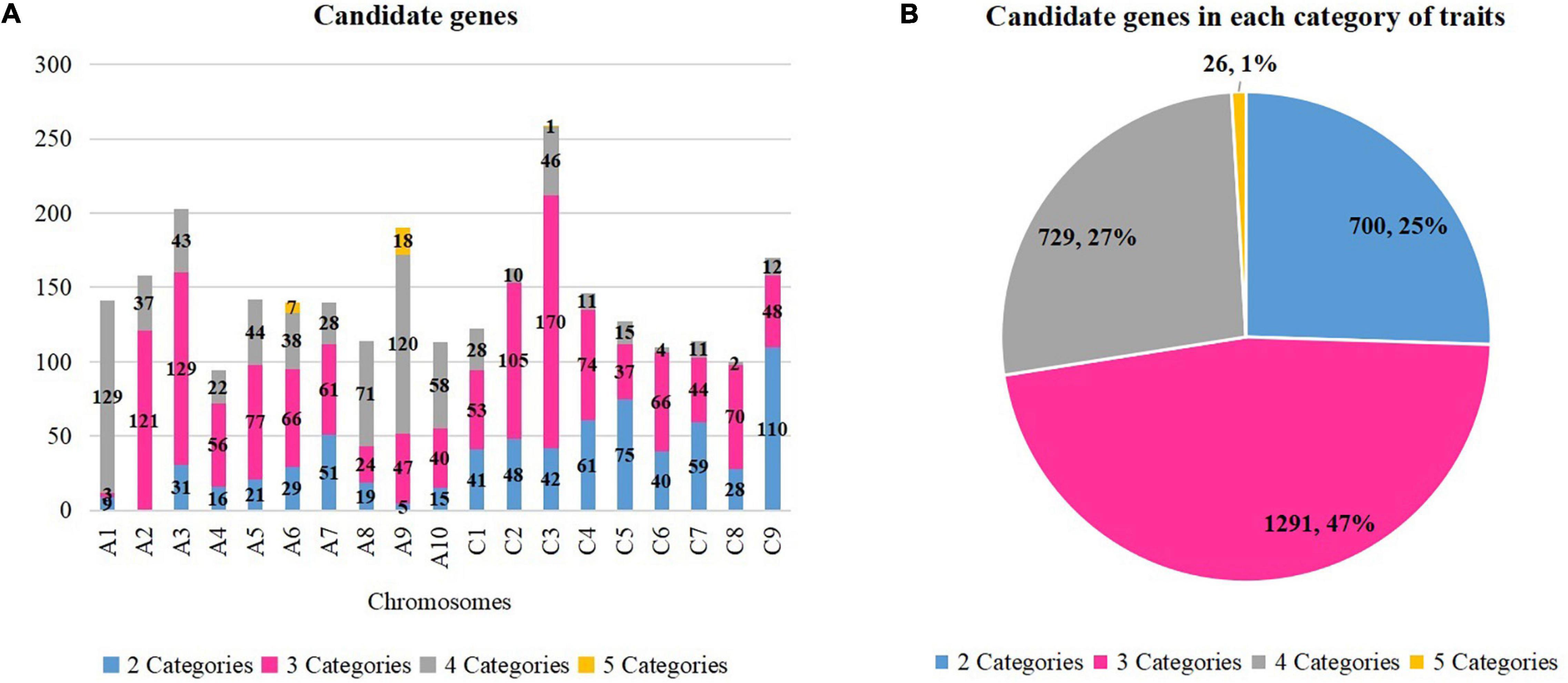
Figure 3. Candidate genes in each category of traits. (A) Candidate genes in each chromosome. (B) Candidate genes in each category of traits. The number and percentage of genes in each category are displayed. Different colors correspond to different categories.
Eight regions of the overlapping QTLs of five categories of traits (A, B, H, S, and Y) were found in six chromosomes (A1, A2, A6, A9, A10, and C3). A total of 26 candidate genes were found on three among those six chromosomes: seven genes on A6 (four on 21.68–21.95 Mb and three on 22–22.30 Mb), 18 genes on A9 (six on 8.12–9.87 Mb and 12 on 20.76–22.51 Mb), and one gene on C3 (5.09–5.33 Mb). For example, three candidates were found on A6 (22–22.30 Mb) which were a DHLAT gene (BnaA06g33300D), an RLK gene (BnaA06g33320D), and an AAPPT gene (BnaA06g33540D). Meanwhile, 729 candidate genes were found within overlapping QTLs of four categories of traits in all 19 chromosomes, and they were, respectively, of 129 (A1), 120 (A9), 71 (A8), 58 (A10), 46 (C3), 44 (A5), 43 (A3), 38 (A6), 37 (A2), 28 (A7 and C1, each), 22 (A4), 15 (C5), 12 (C9), 11 (C4 and C7, each), 10 (C2), four (C6), and two (C8). For example, three candidate genes (CCT BnaA03g14860D, RLK BnaA03g15210D, and KAT2 BnaA03g15290D) on A3 (6.84–7.12 Mb) were found within 19 overlapping QTLs (2B, 1H, 5S, 11Y). Moreover, 1,289 candidate genes were located within overlapping QTLs of three categories of traits, and they were found on all 19 chromosomes: 169 (C3), 129 (A3), 121 (A2), 104 (C2), 77 (A5), 74 (C4), 70 (C8), 66 (A6 and C6), 61 (A7), 56 (A4), 53 (C1), 48 (C9), 47 (A9), 44 (C7), 40 (A10), 37 (C5), 24 (A8), and three (A1). For instance, two candidate genes (ADC2 BnaC01g03710D, and FAE BnaC01g04130D) were found on C1 (1.93–2.16 Mb) involving 13 overlapping QTLs (4B, 1S, 8Y). At last, overlapping QTLs of 2 categories of traits contained 700 candidate genes in 18 chromosomes (excluding A2): 110 (C9), 75 (C5), 61 (C4), 59 (C7), 51 (A7), 48 (C2), 42 (C3), 41 (C1), 40 (C6), 31 (A3), 29 (A6), 28 (C8), 21 (A5), 19 (A8), 16 (A4), 15 (A10), 9 (A1), and 5 (A9). For example, two candidate genes (RLK BnaC07g13860D and RN BnaC07g14020D) were found on C7 (19.60–19.79 Mb) involving two overlapping QTLs (1 A and 1 B). In assumption from those findings, important genes which were located within regions of overlapping QTL with multiple traits were identified. They might influence more than one category of traits, and they could be selected according to the desired improvement of two or multiple traits.
The interaction network analysis of the 2,744 candidate genes was made with their 1,555 orthologous genes in A. thaliana because B. napus are not available on the String database. Gene ontology (GO) analysis indicated that the 1,555 genes could be classified into 16 categories, according to Panther GO-slim biological process’s classification (Supplementary Table 7): it included the cellular process, biological phase, reproductive process, multi-organism process, localization, interspecies interaction between organisms, reproduction, biological regulation, response to stimulus, signaling, developmental process, rhythmic process, multicellular organismal process, metabolic process, growth, immune system process. Other genes which could not fit into those categories were classified as “Others.”
The interaction network was visualized with Cytoscape and 1,271 nodes and 10,101 edges were displayed (Figure 4). In this network, 34 genes might be more influential over other genes (degree layout, DL≧60) (Supplementary Table 8). Those genes belonged to the GO categories of the cellular process, metabolic process, multicellular organismal process, rhythmic process, and “others” category. ACP, DGAT, KASI, KASII, KASIII, LPAAT, and MCMT had functions related to oil biosynthesis (Li-Beisson et al., 2013), AGL20, AP2, AUX1, CO, COP1, EMB, FLC, FLD, FRI, FT, FVE, GI, LPAAT, PHYA, PHYB, RGA, SVP, TFL1, and TFL2 were related to yield traits, while AP40, ARP, ERD, GMP synthase, HEME, SH3, and WUS were involved in plant resistance to disease (Poole, 2007). The 26 candidate genes detected in the region of overlapping QTLs involving five categories of traits had less influence over the other genes DL < 46 (Supplementary Table 9), in comparison to the 11 above-mentioned genes. The most influential genes had different functions and were involved in different metabolism pathways, yet they might have a higher effect over other genes, this might indicate that the simultaneous control of multiple categories of traits might be affected at different paths of metabolisms.
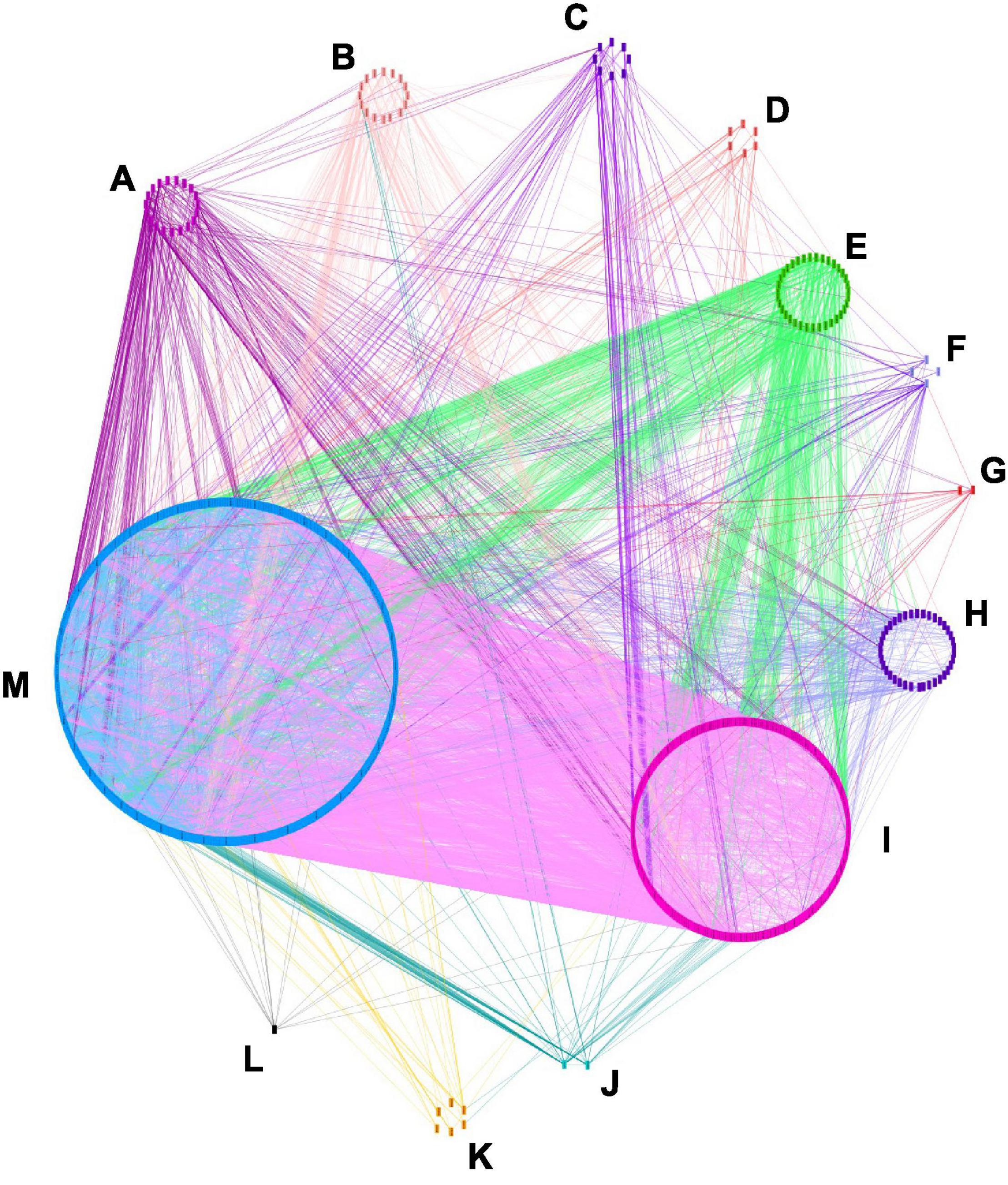
Figure 4. Candidate genes interaction network. The interaction analysis was made with orthologous A. thaliana genes by using STRING (http://string-db.org/) and visualized with Cytoscape_V3.8.2. 1271 nodes and 10101 edges are shown. Eleven categories of genes are displayed according to their GO term enrichment. (A) Signaling, (B) multicellular organism, (C) growth, (D) biological regulation, (E) cellular process, (F) developmental process, (G) immune system process, (H) localization, (I) metabolic process, (J) rythmic process, (K) response to stimulus, (L) reproduction, (M) others.
Gene Expression and Structural Variation of Candidate Genes in Eight Rapeseed Accessions
The 34 most influential genes of the interaction network in B. napus were selected for the current gene expression analysis. Additionally, the 26 candidate genes which were found within QTLs of five categories (A, B, H, S, and Y) were also added to the analysis (Supplementary Table 10). Two groups of genes could be observed, the first group was the genes that were mainly expressed in the seeds and siliques, including FUS3, PRP4 KINASE B, KR, RCN1, KASI, KASIII, MCAT, FLC, FVE, and EMB (Figure 5A and Supplementary table 11), with higher expression in the seeds rather than in the siliques, except for the EMB gene. The second group had the genes expressed in different tissues and the genes with very low expression levels (Figure 5B and Supplementary Table 11).
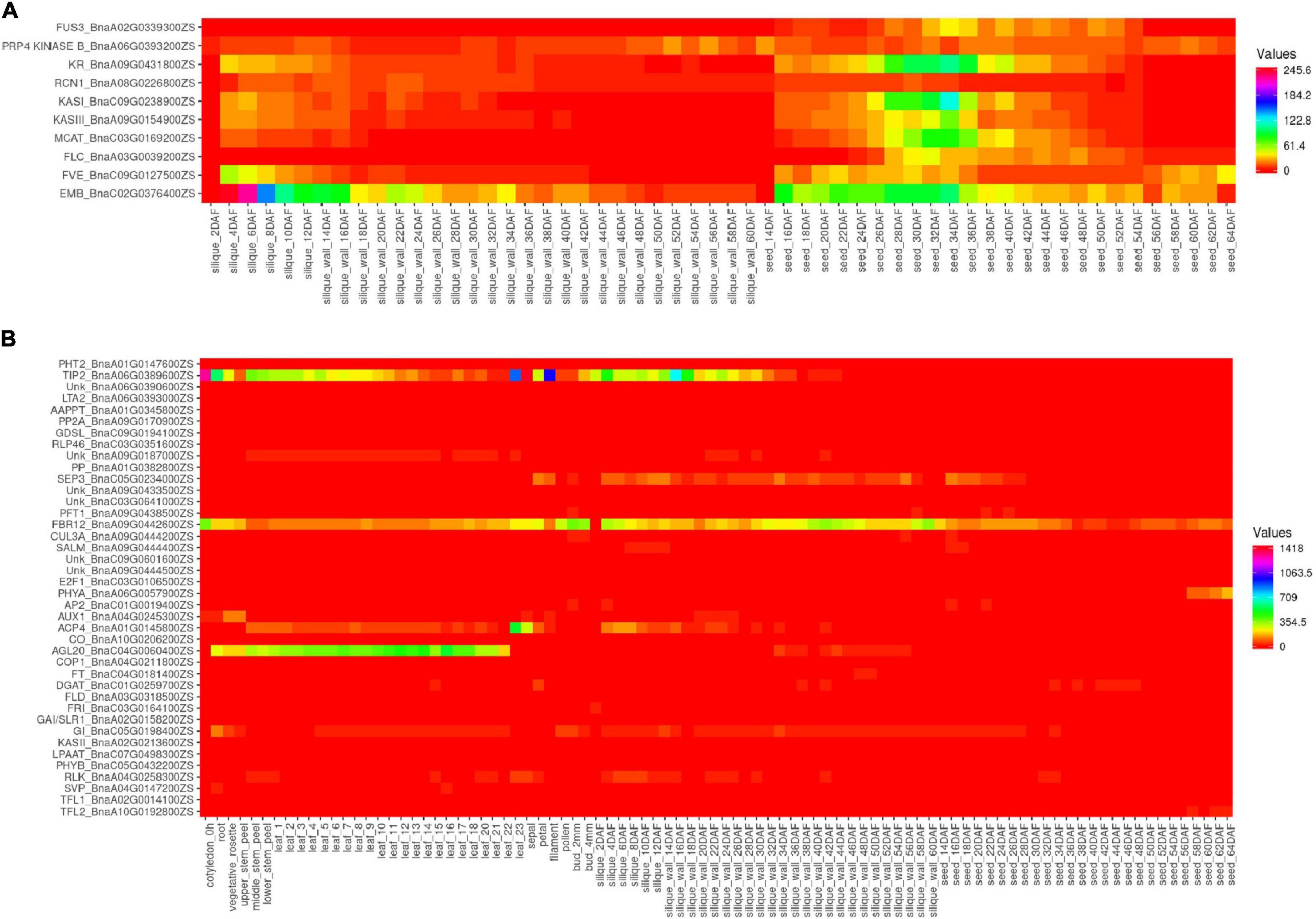
Figure 5. Expression level of the candidate genes in ZS11. (A) Ten genes exclusively with higher expression in the siliques and seeds. (B) Expression of other genes in different tissues. The heatmap was built using Heatmapper (http://www2.heatmapper.ca/).
A quantitative PCR (qPCR) was performed using the complementary DNA (cDNA) from the seeds and siliques of KenC-8. It was observed that the genes had higher expression in the seeds than in the siliques, except for the PRP4 KINASE B gene which had higher expression in the siliques than in the seeds. Moreover, the EMB gene which had a higher expression in the siliques than in the seeds of ZS11, had a higher expression in the seeds than in the siliques of KenC-8 (Figure 6).
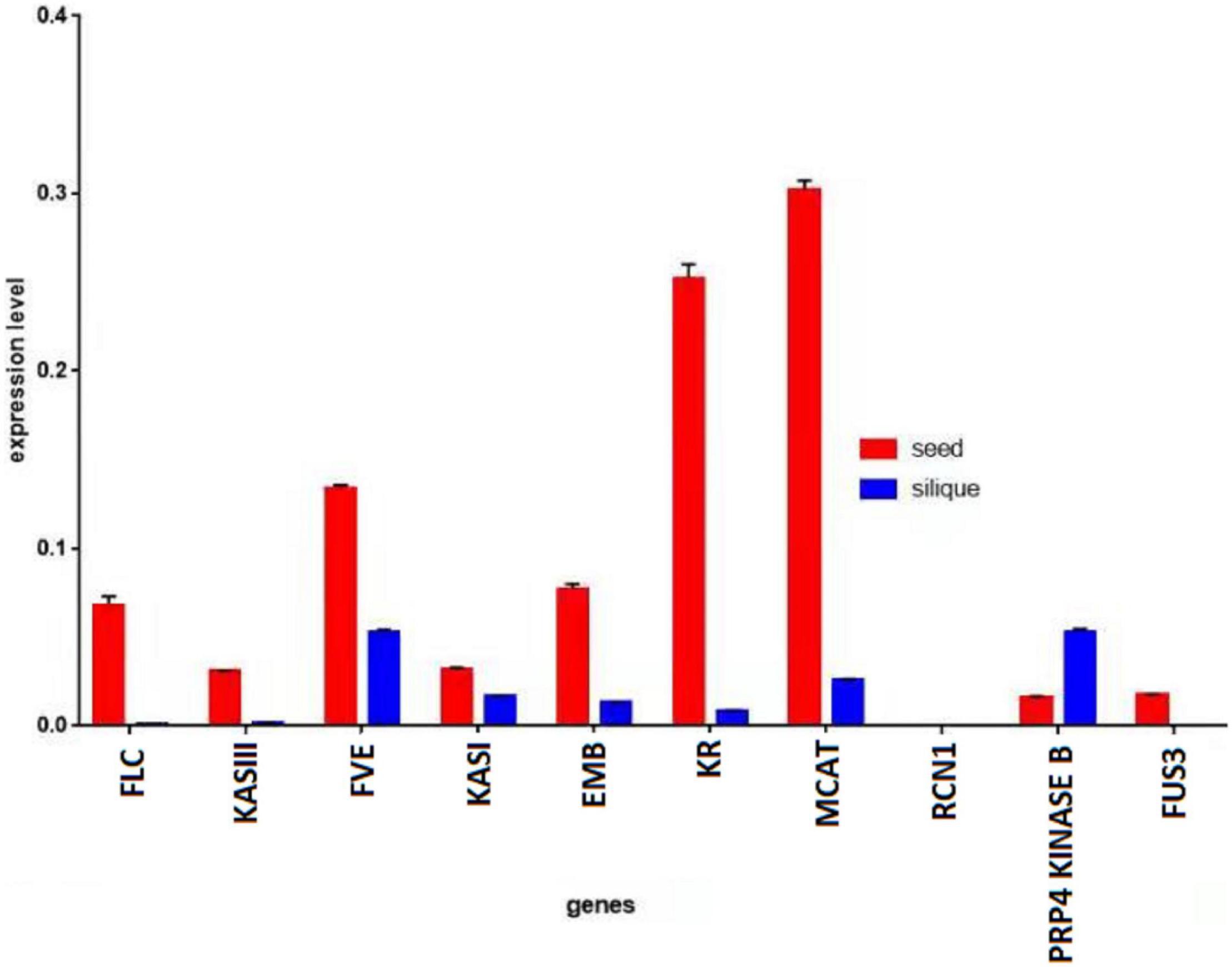
Figure 6. Expression level of genes in seeds vs. siliques of KenC-8. Actin was used as reference gene.
Besides, the gene expression and the structural variation of the above-mentioned 10 genes were analyzed in eight rapeseed varieties, including two winter-types (Quinta and Tapidor), two spring-types (Westar and No2127), and four semi-winter types (ZS11, Zheyou7, Shengli and Gangan). The synteny of the genes in the nine rapeseed varieties is shown in Figure 7. The genes were located in five chromosomes in Darmor-bzh, and in eight chromosomes in the other eight rapeseed varieties. The length of the genes was almost similar in the eight varieties but they were much longer in size in Darmor-bzh (Supplementary Table 12). The expression of those 10 genes was compared in the eight rapeseed varieties (Supplementary Figure 2). The nucleotide sequence identity was analyzed (Supplementary Table 13). Genes with a higher rate of sequences identity had similar gene expressions. It was the case of the KASIII genes in Zheyou and ZS11. However, genes that displayed 100% of sequence identity could also have different expression profiles, such as the KR genes of the eight varieties that shared 100% of sequence identity but displayed a different expression level. More, some other genes with different sequences identity showed similar expression profiles, like the case of FLC genes in Gangan and No2127.
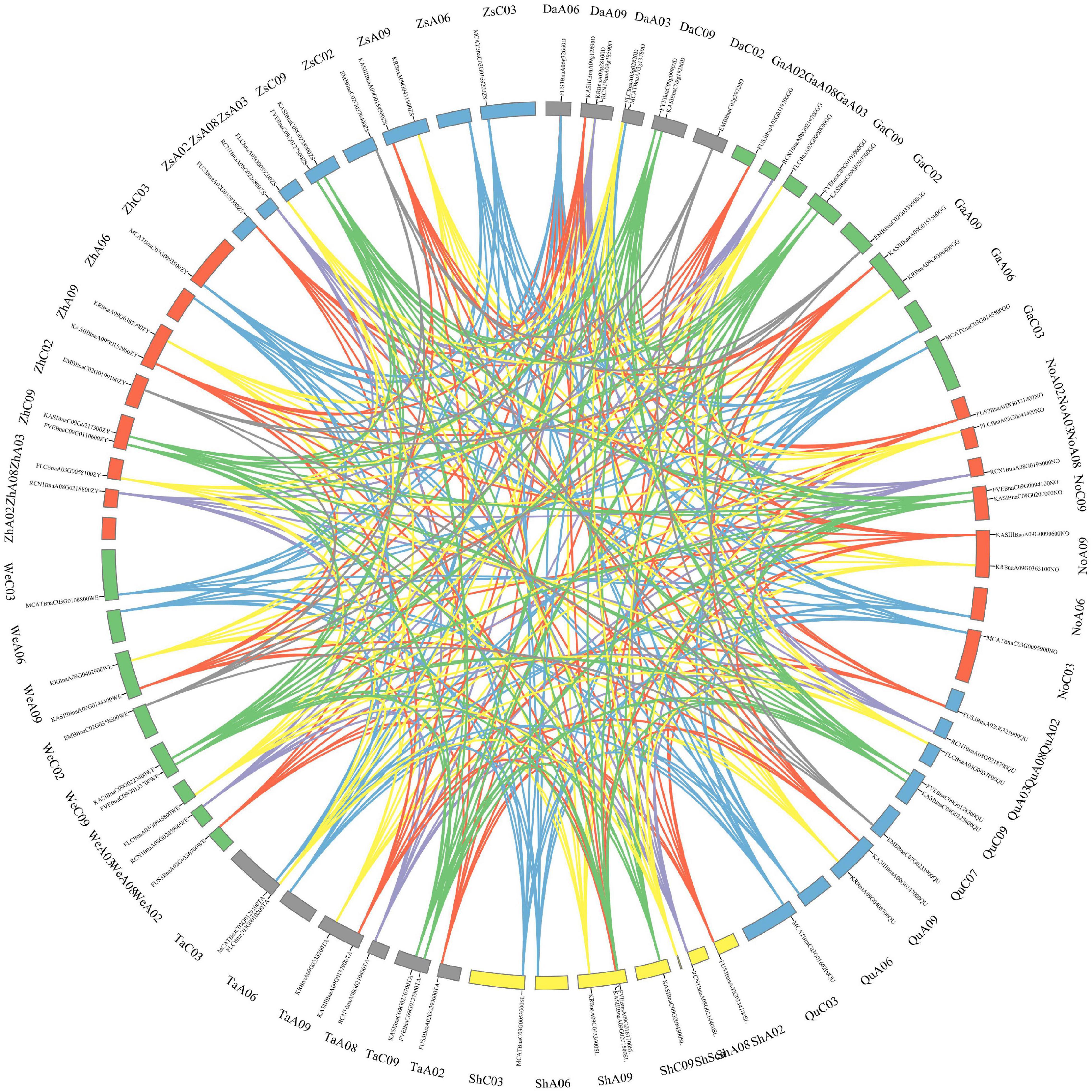
Figure 7. Synteny of the ten genes in nine rapeseed varieties. The map was built with TBtools software, with Darmor-bzh (Da), Gangan (Ga), No2127 (No), Quinta (Qu), Shengli (Sh), Tapidor (Ta), Westar (We), Zheyou (Zh), Zhongshuang 11 (Zs). Chromosome location is displayed near the genome name.
Discussion
The current study aimed to combine QTLs for seed component, seed yield, hormones, and disease-related traits, which were detected in previous studies, in one physical map in rapeseed, to identify the related candidate genes and to analyze their expression and structural variation in different rapeseed accessions. The same strategy was used to find the regions that might control multiple traits of one category, i.e., seed oil (Raboanatahiry et al., 2017) and seed yield (Raboanatahiry et al., 2018). In those two studies, some regions were suggested to possibly contribute to the improvement of one trait or multiple traits of one category, and some regions were supposed to be stable for one given environment. For example, a region on A1 (2.50–2.99 Mb) had overlapping QTLs for plant height, which was from two populations developed in China (Tapidor × Ningyou7 and Express617 × V8), so this region might affect the plant height and it is a stable region for the Chinese environment (Raboanatahiry et al., 2018). More, QTLs for C16:0, C18:0, C18:1, C18:2, C20:0, and C22:1 were overlapping on C3 (53.75–58.29 Mb), thus, this region might control those six traits, simultaneously (Raboanatahiry et al., 2017). The current study was made at a higher level because it was not limited to one category of trait as in the previous studies, but five categories which involved almost all the studied traits in rapeseed.
Increasing seed oil and seed yield is among the main focus of researchers on rapeseed, to cater to the increasing demand for
oil. However, the usage of rapeseed is not limited to biomass for oil, but also multiple purposes, such as protein, carbohydrate, vitamins sources, and many more (Raboanatahiry et al., 2021). Despite the effort in improving seed components and seed yield, rapeseed crops are under attack from various diseases that resulted in huge crop loss. For example, Leptosphaeria maculans causes blackleg disease (West et al., 2001), which has created an economic loss of $900 million per growing season globally (Fitt et al., 2008). Although resistant cultivars have been developed and cultivated since the 1990s in Canada (Kutcher et al., 2010), which has decreased the yield loss by 1% (Gugel and Petrie, 1992), abiotic stresses have also caused about 50% yield reduction in major crops (Bray et al., 2000). Extensive research is still undertaken to take total control of those biotic and abiotic diseases, via selective breeding. Otherwise, phytohormones play important roles in plant growth and development, such as IAA (Grossmann, 2010), but also on plant adaptation to assure survival to face the environmental fluctuation. Abscisic acid (ABA) responds to both biotic and abiotic stresses (Cao et al., 2011), which have an influence on one another (Fujita et al., 2006). Those phytohormones support agronomic trait improvement and response to disease. Therefore, all the five categories of traits analyzed in the current study are correlated and are pivotal for rapeseed crop improvement.
Dissection of Rapeseed Genome Revealed Regions Controlling Multiple Traits
The current study is the first study to gather all the QTLs of important agronomic and disease-related traits discovered in B. napus over 25 years, to construct a quantitative genomic map, which is crucial to uncover similarities and differences in QTLs detected from different populations and environments, but also to reveal the regions that might control multiple beneficial traits, simultaneously.
It was obvious that most of the QTLs were found on the A rather than C genome (2,695 vs. 1,860 QTLs, respectively). Selection has played important role in improving B. napus. It was reported that the C genome rather than the A genome, contained extended breeding regions (51.15 Mb on C vs. 16.80 Mb on A) which might contribute more to alleles producing elite traits (Wang Z. et al., 2014). However, a recent investigation on the origin of B. napus and the genetic loci that contributed to its improvement had revealed that the parallel selection of the A and C genomes had led to seed quality improvement in B. napus (Lu et al., 2019). “A” genome-specific selection greatly enhanced disease resistance, oil accumulation, and environment adaptation of B. napus during its first stage of improvement, while the C genome had improved developmental traits. This might explain the fact that most of the QTLs of studied traits in the current study could be found on the A genome. Particularly, for Asian B. napus varieties, it was reported that they have experienced strong artificial selection from the A genome which contributed to their adaptation following their introduction from Europe (Zou et al., 2019).
Apart from that, 517 regions were found with overlapping QTLs involving at least two categories of traits. Those regions might be suitable for selection to improve two or more desired traits, simultaneously, for example, to improve both abiotic stress response, seed component, and seed yield (A4:3.07–4.11 Mb). Several studies have already investigated on the co-location of QTLs from a different category of traits (Shi et al., 2012; Zhao J. et al., 2012; Ding et al., 2014; Bouchet et al., 2016; Körber et al., 2016; Stein et al., 2017; Wan et al., 2017; Rahaman et al., 2018; Jan et al., 2019; Wrucke et al., 2019; Wu et al., 2019; Zhang K. et al., 2019). Those studies demonstrated the importance of analyzing multiple traits, at the same time, to target the loci for breeding cultivars with the most advantageous profile. For example, a study that focused on flowering time (FT) and Sclerotinia stem rot resistance (SSR) reported that early FT might increase susceptibility to S. sclerotiorum, and regions of co-location of FT and SSR resistance traits were found which were crucial for breeding early maturing and SSR resistance cultivars. Moreover, four co-localized QTL hotspots of SSR resistance and FT on A2 (0–7.7 Mb), A3 (0.8–7.5 Mb), C2 (–15.2 Mb), C6 (20.2–36.6 Mb), which were consensual with previous studies (Wu et al., 2019). In the current study, the QTL of SSR and DIF (FT) were also co-localized in those regions.
Particularly, seed components and seed yield traits often overlapped in this study. In earlier studies, yield traits such as the flowering time, the morphology of the root, and the plant growth environment could affect seed quality traits such as erucic acid, oil, protein, and glucosinolate contents (Paran and Zamir, 2003; Si et al., 2003; Quijada et al., 2006; Shi et al., 2013; Wang X. et al., 2017). In oil crops, QTLs that could influence both seed quality and yield traits had already been discovered in several studies, positively or negatively. For instance, oil and protein contents were positively correlated with seed weight in 11 B. carinata lines developed in Canada (Getinet et al., 1996). Zhao et al. (2006) found evidence of a positive correlation between oil content and seeds per silique while evaluating 282 DH lines from a cross between Sollux and Gaoyou (B. napus), and developed in Germany and China. In a study performed by Chen et al. (2010) in a DH population derived from a cross between high and low oil content B. napus and developed in Canada, oil content and flowering time were negatively correlated. In that study, QTLs for oil content, flowering time, and seed yield were co-localized on a small region of LG7 where alleles of low oil content, early flowering time, and higher seed yield were found together. However, QTLs for high oil content and early flowering time were found in the co-location on LG2. Otherwise, since oil content and seed yield are the most important trait in rapeseed, 82 QTL regions were discovered, where QTLs for these two traits overlapped. They could be selected to improve the two traits, simultaneously.
Overlapping QTLs of multiple traits might happen when gene alteration frequencies at nearly linked loci occur, but also, it might be caused by the pleiotropic effect when an appropriate substitution of genes occurs (Smith and Haigh, 1974). Also, pleiotropy or/and linked genes might have caused this phenomenon. Hot QTLs regions were also discovered with more than 100 aligned QTLs, which is interesting for rapeseed molecular breeding, because the region with the QTLs of diverse traits could be targeted to improve multiple traits, simultaneously, and the more the number of QTLs increased in that region, the more the locus was stable because, despite the diversity in population and environment background, it did not alter the location of the QTL on the genome.
The region on the chromosome which was exclusively for QTLs from one population was not found, and those exclusively for one environment were for China only (fixed environment for China). This indicated that those QTLs remained unchanged, despite the variation of population and environment.
Identified Candidates Genes Might Be Pleiotropic or Linked Genes?
In previous studies, 110 genes in Arabidopsis were identified to be involved in oil formation (Li-Beisson et al., 2013) and 439 homologous genes were found in B. napus (Raboanatahiry et al., 2017). Moreover, 425 yield genes which were related to branch number, flowering time, maturity time, plant height, pod number, seed number, seed weight, and seed yield in Arabidopsis were identified (Shi et al., 2009) and 1,398 homologous genes were detected in B. napus (Raboanatahiry et al., 2018). Dolatabadian et al. (2017) found 1,344 resistance genes in B. napus. Those genes had a relationship with the currently studied traits, thus, they were selected to uncover the candidate genes.
The function of those selected genes correlated with the studied traits. Since many genes could be found within a region of a genome, a preselection is necessary, so that the candidates have a close connection to the studied traits, and have a functional outcome on the process (Zhu and Zhao, 2007). A total of 2,744 genes related to oil, yield, and resistance were found within overlapping QTLs involving two to five categories of traits. As mentioned above, overlapping QTLs might be caused by pleiotropy or linked genes. Pleiotropy is when one gene can control multiple unrelated phenotypic traits (Stearns, 2010; Conner et al., 2011; Wagner and Zhang, 2011; Solovieff et al., 2013). Pleiotropy is largely distributed due to biochemical and developmental systems and it affects development and evolution and creates correlations between genes and phenotype, and it affects selection and imposes the accessibility of the evolution extent (Gromko, 1987; Lynch and Walsh, 1998). The pleiotropic organization of traits (dominant or epistatic) can be modified by selection and inbreeding (Goodnight, 1988; Cheverud and Routman, 1996; Hansen and Wagner, 2001). Linked genes are genes located close to each other on the same chromosome and are inherited together during meiosis. Genes might separately control different phenotypes but are found closely located on the same region of a chromosome.
Candidates found within the region of overlapping QTLs with five categories of traits attracted more attention since they might be more influential than the others over multiple traits. A total of 26 candidates were found on five regions distributed on A6, A9, and C3 chromosomes, and they would be the most recommended in this study, for genomic selection to target multiple traits simultaneously (Figure 8). They belonged to different families and might have different distinct roles, but the way they act to influence each other or to affect the studied traits still needs a deep investigation. Functional investigation of each gene over the studied traits would be indispensable to comprehend their influence on the traits and would reveal whether they were pleiotropic genes or linked genes.
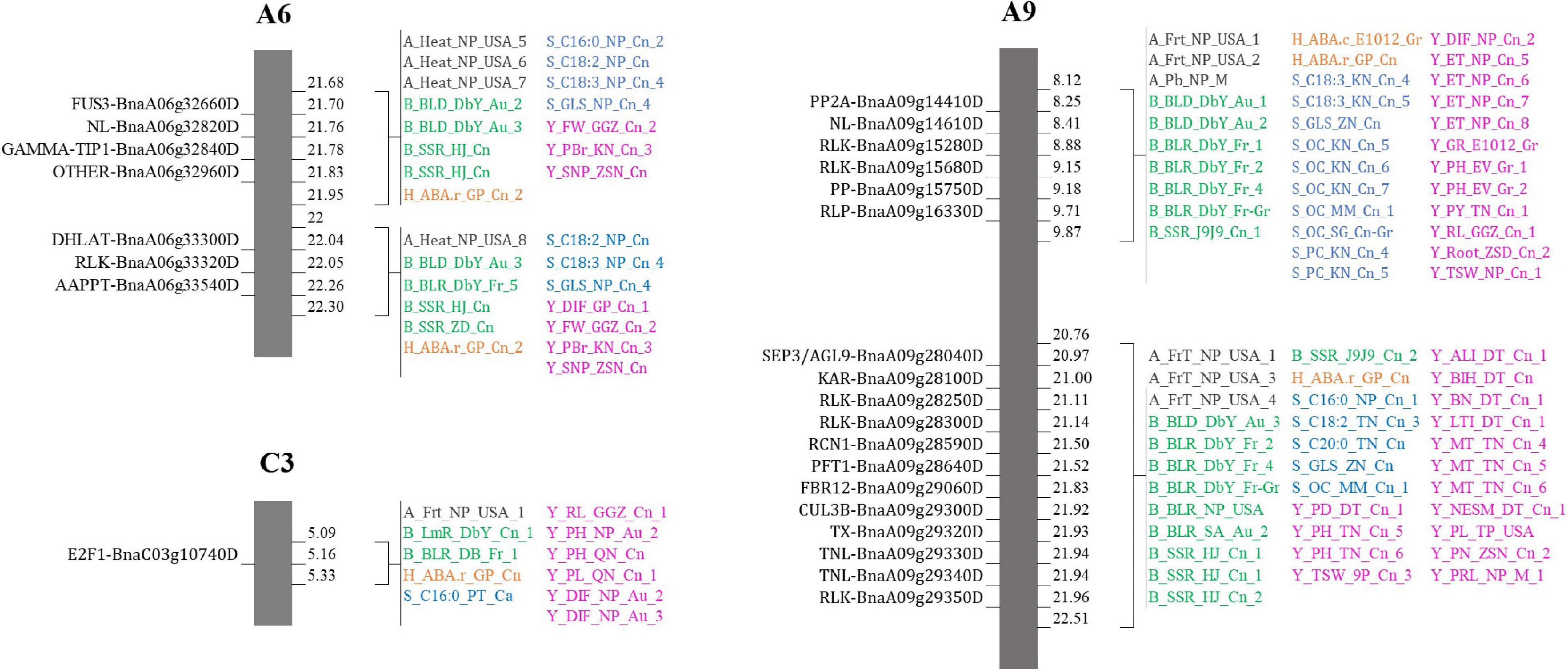
Figure 8. Suggestions for selective breeding. Regions on A6, A9, and C3 chromosomes where QTLs of five categories overlapped.
Gene interaction network revealed that 34 genes might have more influence over the other genes. Genes are responsible for the genetic variation of traits (Tabor et al., 2002), and the structure and dynamism of the genetic regulatory network have an impact on quantitative traits (Frank, 2003). In this study, KAS, ACP, AUX1, CO, FT, PHYA, and AGL20 were also identified as the most influential genes in our previous studies (Raboanatahiry et al., 2017, 2018). Despite the number of genes identified in this study being far larger than those of the previous study, and the gene function were also broader, those seven genes of different functions still had higher influence over the other genes, indicating that simultaneous control of multiple traits might be affected at different metabolism pathways.
The expression analysis of the candidate genes revealed an exclusive increase for 10 genes (FUS3, PRP4 KINASE B, KR, RCN1, KASI, KASIII, MCAT, FLC, FVE, and EMB) in the seeds and siliques of ZS11, with higher expression in the seeds than in the siliques, except for EMB. Reversely, the PRP4 KINASE B of KenC-8 had increased expression in the siliques in comparison to the seeds. Previous studies reported the function of those 10 genes: MCAT, KASI, and KASIII (β-ketoacyl-ACP synthase I and III), and KR (β-ketoacyl- ACP reductase) are enzymes of fatty acid biosynthesis. MCAT (Malonyl-CoA:ACP transacylase) was suggested to be essential for a high oil content B. napus, and might be used to improve the seed oil contents (Qu et al., 2014). Plastidial KASIII genes could alter the fatty acid profile of B. napus seeds, by increasing the C18:2 and C18:3 contents at the expense of C18:1 (Verwoert et al., 1995). KASI played a positive role in Arabidopsis morphology and fertility, and in polar lipid composition. Moreover, KASI disruption affected embryo development and decreased the fatty acid contents (Wu and Xue, 2010). FUS3 (FUSCA3) is a regulator of seed development and seed oil content. It induces the genes of fatty acid biosynthesis during development in Arabidopsis (Wang et al., 2007). Likewise, the oil production decreased in fus3 of B. napus (Elahi et al., 2015). In Arabidopsis, RCN1 (roots curl in naphthylphthalamic acid1) encodes a regulatory α-subunit of protein phosphatase 2A. RCN1 modulates auxin responses in roots (Garbers et al., 1996), and defection resulted in increased basipetal auxin transport and a significant delay in gravitropism (Rashotte et al., 2001). Moreover, rcn1 roots had a reduced elongation in seedlings and hypocotyl elongation (Muday et al., 2006). FLC (FLOWERING LOCUS C) is a vernalization regulator with a high expression level in winter-type rapeseeds (Schiessl et al., 2019). It induces a delayed flowering time in Arabidopsis and Brassica rapa (Kim et al., 2007), but also in B. napus (Tadege et al., 2001). However, FLC could be repressed by FVE genes which act on the regulation of flowering time (Baek et al., 2008; Yu et al., 2016). EMB (EMBRYO DEFECTIVE) is required for growth and development in Arabidopsis (Devic, 2008; Meinke, 2020). In the current study, the particular presence of those genes in the seeds indicated their importance at an early stage of plant formation.
The expression and structural variation analysis of the genes in eight rapeseed varieties showed that some genes which had 100% sequence identity displayed different expression profiles, and some other genes with different sequences identity showed similar expression profiles. Note that Vector NTI software (Invitrogen) was used to calculate the sequence identity, and it was observed that even with a large structural variation, the software still displayed a 100% of sequence identity between the genes, which was unexpected. However, genes with several SNPs had less than 100% of sequence identity. Several SNPs (case of KASI) and a large structure variation (case of MCAT genes) in the candidate genes were observed, which might explain the difference in expression. This probably implied that trait variations possibly occur because of the structure and expression variation of the candidate genes, which can be verified in future studies.
Epigenetics is one of the factors which might cause an alteration in gene expression while preserving the primary DNA sequence or genotype (Bird, 2002; Tchurikov, 2005). Epigenetic mechanisms include DNA methylation which commonly induces gene silencing by blocking the transcription binding sites, histone modification which alters chromatin structure and accessibility of genes for transcription, and non-coding RNA-associated gene silencing which targets mRNA transcripts for destruction induce and preserve epigenetic change (Egger et al., 2004; Tirado, 2014). Even if an epigenetic change is natural and regular, it can also be influenced by environmental factors (Aguilera et al., 2010; Feil and Fraga, 2012). In the case of our study, further analysis is needed to conclude about epigenetic via comparison of promoter sequence between genes. Because the full genomic sequences are absent in Brassica napus pan-genome information resource (BnPIR), the analysis could not be done in the current study. Also, the eight accessions were produced with different genetic and environmental backgrounds, and the age of plant materials also plays a role in gene expression. Thus, the difference in the gene expression even with a similar sequence was expected.
Breeding a Super-Rapeseed Cultivar That Meets Expectations
The current study uncovered regions, with two, three, four, or five categories of traits that can be chosen and used for marker-assisted selection, to produce a customized rapeseed cultivar with desired traits. For instance, stresses imposed by heat are detrimental to seed yield and quality (reviewed by Sehgal et al., 2018). To control these traits at once, the region on A3 (11.40–12.47 Mb) could be selected for fine-mapping, since it contained overlapping QTLs for heat, seed yield, and seed composition. Candidate genes included in this region could be cloned and validated through functional analysis, to understand the related molecular mechanism.
Another innovation of the current study is the usage of the rapeseed pan-genome of BnPIR to compare the gene expression and gene structure of candidate genes. This strategy aimed to comprehend how the same genes of different accessions would be expressed, and how their structures are different. This might serve later to explain their functions. Since numerous rapeseed accessions have been sequenced, performing the same study as our current study is now feasible in those other accessions. It would enhance our understanding of rapeseed genome variation. In the future, it would be interesting to know whether the QTLs of multiple traits could also be found overlapping in the same region of the other rapeseed varieties, as found in Darmor-bzh of this study. It is important to discover if the regions were maintained in all the varieties of rapeseed. The characterization of haplotypes is also needed to understand if those regions could be inherited together.
Besides, compared to rice, the rapeseed breeding program needs more effort and innovation. Until now, rapeseed research focused on QTLs and the studied traits were repetitive. However, the rice breeding program already focuses on QTG (quantitative trait gene) and QTN (quantitative trait nucleotide) for the improvement of this crop (Wei et al., 2021). This effort was made to further close the gap between genomic studies and practical breeding, and to facilitate the localization of causative variants of all known traits. A collection of rice varieties with those variations was made and a genome navigation system was established for breeding. Thus, research on rapeseed should switch progressively into those QTG and QTN analyses. Multiple rapeseed accessions are also available and a collection of variations should be implanted for breeding.
Finally, the current study has enhanced our knowledge of rapeseed genome characteristics and diversity. Co-localized QTLs might have an ally or antagonistic effect. For the usage in practical breeding, identification of the most favorable alleles combinations which will produce maximum profits is still crucial.
Materials and Methods
Alignment of Quantitative Trait Locus on the Physical Map of Darmor-bzh
Extensive literature inquiry allowed us to identify more than 350 papers that reported on genome-wide association studies (GWAS) and QTLs analyses in B. napus over the last 25 years (1995–2020). They were manually sorted according to data availability. Research articles with missing information were removed (absence of flanking markers, marker sequence, or physical position of QTLs on Darmor-bzh). QTLs/GWAS with just one flanking marker were kept and given an area of 1 cm from the unique marker as loci. A total of 4,555 QTLs for seed, yield, hormones, and disease-related traits were collected from 145 research articles, involving 79 different populations of three different ecotypes and grown in 12 different countries (Supplementary Table 1). They were aligned in the physical map of Darmor-bzh. The location of QTLs flanking markers on the physical map was detected via e-PCR (Schuler, 1997; Rotmistrovsky et al., 2004), and the method of alignment was as similar as our previous studies (Raboanatahiry et al., 2017, 2018). The map was built using Circos software (Krzywinski et al., 2009).
Identification of Candidate Genes
Genes in B. napus which were reported in three different pieces of literature were selected as a basis for the identification of candidate genes in the current study: 439 genes were related to oil formation (Raboanatahiry et al., 2017), 1,398 genes were related to yield traits (Raboanatahiry et al., 2018), and 1,344 genes were resistance genes (Dolatabadian et al., 2017). They were aligned into the physical map of Darmor-bzh, and the genes located within overlapping QTLs were identified as candidates for the traits.
Construction of Gene Interaction Network
The gene interaction network was predicted using STRING (Szklarczyk et al., 2015).1 Orthologous genes in A. thaliana were used to perform the analysis (Chao et al., 2017; Raboanatahiry et al., 2017, 2018). The genes were clustered using Panther GO-slim biological process (Mi et al., 2021),2 and the interaction was visualized with Cytoscape_V3.6.0 (Shannon et al., 2003).
Gene Expression and Structural Variation Analyses of Candidate Genes
The gene expression was obtained from BnTIR (Liu et al., 2021), because ZS11 is the only rapeseed variety available in the BnTIR database, it was used as a reference for this analysis. ZS11 genes were acquired from the BnPIR database (Song et al., 2021) and the genes expression analysis was obtained from the BnTIR database (Liu et al., 2021). The heatmap was built using Heatmapper (Babicki et al., 2016).3 The developing siliques and the seeds of KenC-8 were collected 30 days after flowering for the q-PCR analysis, and Actin7 was used as an internal control.
The identification of homologous candidate genes in Gangan, No2127, Quinta, Shengli, Tapidor, Westar, Zheyou7, and ZS11 was made with a blast tool in the BnPIR database (Song et al., 2021),4 using Darmor-bzh gene sequences as a query sequence. In silico gene expression analysis was made using the “gene expression” tool of BnPIR. The identity percentage between CDS sequences was calculated using Vector NTI Advance 11.5.1.
Data Availability Statement
The original contributions presented in the study are included in the article/Supplementary Material, further inquiries can be directed to the corresponding author/s.
Author Contributions
NR wrote the manuscript. NR and HC performed the analysis of the QTL alignment map and candidate genes identification. NR and JH made the synteny analysis and the gene interaction network. NR, HL, and YY analyzed the gene expression. ML supervised the work and revised the manuscript. All authors contributed to the article and approved the submitted version.
Funding
This work was funded by the National Natural Science Foundation of China (31871656, 32072098, 31701456, and 32172087) and the China Postdoctoral Science Foundation (2016M602309).
Conflict of Interest
The authors declare that the research was conducted in the absence of any commercial or financial relationships that could be construed as a potential conflict of interest.
Publisher’s Note
All claims expressed in this article are solely those of the authors and do not necessarily represent those of their affiliated organizations, or those of the publisher, the editors and the reviewers. Any product that may be evaluated in this article, or claim that may be made by its manufacturer, is not guaranteed or endorsed by the publisher.
Supplementary Material
The Supplementary Material for this article can be found online at: https://www.frontiersin.org/articles/10.3389/fpls.2022.862363/full#supplementary-material
Footnotes
- ^ http://string-db.org/
- ^ http://pantherdb.org/
- ^ http://www2.heatmapper.ca/
- ^ http://cbi.hzau.edu.cn/bnapus/
References
Aguilera, O., Fernández, A. F., Muñoz, A., and Fraga, M. F. (2010). Epigenetics and environment: a complex relationship. J. Appl. Physiol. 109, 243–251. doi: 10.1152/japplphysiol.00068.2010
Al-Shehbaz, P. I. A., Beilstein, M. A., and Kellogg, E. (2006). Systematics and phylogeny of the Brassicaceae (Cruciferae): an overview. Plant Systemat. Evolut. 259, 89–120. doi: 10.1007/s00606-006-0415-z
Amar, S., Becker, H. C., and Möllers, C. (2009). Genetic variation in phytosterol content of winter rapeseed (Brassica napus L.) and development of NIRS calibration equations. Plant Breed. 128, 78–83. doi: 10.1111/j.1439-0523.2008.01531
Arifuzzaman, M., Mamidi, S., McClean, P., and Rahman, M. (2016). QTL mapping for root vigor and days to flowering in Brassica napus L. Can. J. Plant Sci. 97, 99–109. doi: 10.1139/cjps-2016-0048
Asghari, A., Asghar Fathi, A., Mohammadi, S. A., and Mohammaddust, H. (2009). QTL analysis for diamondback moth resistance in canola (Brassica napus L.). Int. J. Plant Product. 3, 29–34. doi: 10.22069/IJPP.2012.649
Babicki, S., Arndt, D., Marcu, A., Liang, Y., Grant, J. R., Maciejewski, A., et al. (2016). Heatmapper: web-enabled heat mapping for all. Nucleic Acids Res. 44, W147–W153. doi: 10.1093/nar/gkw419
Baek, I. S., Park, H. Y., You, M. K., Lee, J. H., and Kim, J. K. (2008). Functional conservation and divergence of FVE genes that control flowering time and cold response in rice and Arabidopsis. Mol. Cells 26, 368–372.
Bao, B., Chao, H., Wang, H., Zhao, W., Zhang, L., Raboanatahiry, N., et al. (2018). Stable, environmental specific and novel QTL identification as well as genetic dissection of fatty acid metabolism in Brassica napus. Front. Plant Sci. 9:1018. doi: 10.3389/fpls.2018.01018
Basnet, R. K., Duwal, A., Tiwari, D. N., Xiao, D., Monakhos, S., Bucher, J., et al. (2015). Quantitative Trait Locus Analysis of Seed Germination and Seedling Vigor in Brassica rapa Reveals QTL Hotspots and Epistatic Interactions. Front. Plant Sci. 6:1032. doi: 10.3389/fpls.2015.01032
Basunanda, P., Radoev, M., Ecke, W., Friedt, W., Becker, H. C., and Snowdon, R. J. (2010). Comparative mapping of quantitative trait loci involved in heterosis for seedling and yield traits in oilseed rape (Brassica napus L.). Theoret. Appl. Genet. 120:271. doi: 10.1007/s00122-009-1133-z
Basunanda, P., Spiller, T. H., Hasan, M., Gehringer, A., Schondelmaier, J., Lühs, W., et al. (2007). Marker-assisted increase of genetic diversity in a double-low seed quality winter oilseed rape genetic background. Plant Breed. 126, 581–587. doi: 10.1111/j.1439-0523.2007.01404.x
Bayer, P. E., Hurgobin, B., Golicz, A. A., Chan, C. K., Yuan, Y., Lee, H., et al. (2017). Assembly and comparison of two closely related Brassica napus genomes. Plant Biotechnol. J. 15, 1602–1610. doi: 10.1111/pbi.12742
Behnke, N., Suprianto, E., and Mollers, C. (2018). A major QTL on chromosome C05 significantly reduces acid detergent lignin (ADL) content and increases seed oil and protein content in oilseed rape (Brassica napus L.). Theoret. Appl. Genet 131, 2477–2492. doi: 10.1007/s00122-018-3167-6
Beilstein, M. A., Al-Shehbaz, I. A., and Kellogg, E. A. (2006). Brassicaceae phylogeny and trichome evolution. Am. J. Bot. 93, 607–619. doi: 10.3732/ajb.93.4.607
Bird, A. (2002). DNA methylation patterns and epigenetic memory. Genes Devel. 16, 6–21. doi: 10.1101/gad.947102
Bouchet, A. S., Laperche, A., Bissuel-Belaygue, C., Baron, C., Morice, J., Rousseau-Gueutin, M., et al. (2016). Genetic basis of nitrogen use efficiency and yield stability across environments in winter rapeseed. BMC Genetics 17:131. doi: 10.1186/s12863-016-0432-z
Bray, E. A., Bailey-Serres, J., and Weretilnyk, E. (2000). “Responses to abiotic stress,” in Biochemistry and Molecular Biology of Plants, eds W. Gruissem and R. Jones (Rockville: American Society of Plant Physiologists), 1158–1203.
Butruille, D. V., Guries, R. P., and Osborn, T. C. (1999). Linkage analysis of molecular markers and quantitative trait loci in populations of inbred backcross lines of Brassica napus L. Genetics 153, 949–964. doi: 10.1093/genetics/153.2.949
Cao, F. Y., Yoshioka, K., and Desveaux, D. (2011). The roles of ABA in plant–pathogen interactions. J. Plant Res. 124, 489–499. doi: 10.1007/s10265-011-0409-y
Cao, Z., Tian, F., Wang, N., Jiang, C., Lin, B., Xia, W., et al. (2010). Analysis of QTLs for erucic acid and oil content in seeds on A8 chromosome and the linkage drag between the alleles for the two traits in Brassica napus. J. Genet. Genom. 37, 231–240. doi: 10.1016/S1673-8527(09)60041-2
Chalhoub, B., Denoeud, F., Liu, S., Parkin, I. A., Tang, H., Wang, X., et al. (2014). Early allopolyploid evolution in the post-Neolithic Brassica napus oilseed genome. Science 345, 950–953. doi: 10.1126/science.1253435
Chao, H., Raboanatahiry, N., Wang, X., Zhao, W., Chen, L., Guo, L., et al. (2019). Genetic dissection of harvest index and related traits through genome-wide quantitative trait locus mapping in Brassica napus L. Breed. Sci. 69, 104–116. doi: 10.1270/jsbbs.18115
Chao, H., Wang, H., Wang, X., Guo, L., Gu, J., Zhao, W., et al. (2017). Genetic dissection of seed oil and protein content and identification of network associated with oil content in Brassica napus. Scient. Rep. 7:46295. doi: 10.1038/srep46295
Chen, F., Zhang, W., Yu, K., Sun, L., Gao, J., Zhou, X., et al. (2018). Unconditional and conditional QTL analyses of seed fatty acid composition in Brassica napus L. BMC Plant Biol. 18:49. doi: 10.1186/s12870-018-1268-7
Chen, G., Geng, J., Rahman, M., Liu, X., Tu, J., Fu, T., et al. (2010). Identification of QTL for oil content, seed yield, and flowering time in oilseed rape (Brassica napus). Euphytica 175, 161–174. doi: 10.1007/s10681-010-0144-9
Chen, W., Zhang, Y., Liu, X., Chen, B., Tu, J., and Tingdong, F. (2007). Detection of QTL for six yield-related traits in oilseed rape (Brassica napus) using DH and immortalized F2 populations. Theoret. Appl. Genet. 115, 849–858. doi: 10.1007/s00122-007-0613-2
Chen, Y., Qi, L., Zhang, X., Huang, J., Wang, J., Chen, H., et al. (2013). Characterization of the quantitative trait locus OilA1 for oil content in Brassica napus. Theoret. Appl. Genet. 126, 2499–2509. doi: 10.1007/s00122-013-2150-5
Cheng, X., Xia, S., Zeng, X., Gu, J., Yang, Y., Xu, J., et al. (2016). Identification of quantitative trait loci associated with oil content and development of near isogenic lines for stable qOC-A10 in Brassica napus L. Can. J. Plant Sci. 96, 423–432. doi: 10.1139/cjps-2014-0442
Cheverud, J. M., and Routman, E. J. (1996). Epistasis as a source of increased additive genetic variance at population bottlenecks. Evolution 50, 1042–1051. doi: 10.1111/j.1558-5646.1996.tb02345.x
Conner, J. K., Karoly, K., Stewart, C., Koelling, V. A., Sahli, H. F., and Shaw, F. H. (2011). Rapid independent trait evolution despite a strong pleiotropic genetic correlation. Am. Natural. 178, 429–441. doi: 10.1086/661907
Delourme, R., Falentin, C., Huteau, V., Clouet, V., Horvais, R., Gandon, B., et al. (2006). Genetic control of oil content in oilseed rape (Brassica napus L.). Theoret. Appl. Genet. 113, 1331–1345. doi: 10.1007/s00122-006-0386-z
Delourme, R., Piel, N., Horvais, R., Pouilly, N., Domin, C., Vallée, P., et al. (2008). Molecular and phenotypic characterization of near isogenic lines at QTL for quantitative resistance to Leptosphaeria maculans in oilseed rape (Brassica napus L.). Theory Appl. Genet. 117, 1055–1067. doi: 10.1007/s00122-008-0844-x
Devic, M. (2008). The importance of being essential: EMBRYO-DEFECTIVE genes in Arabidopsis. Compt. Rendus Biol. 331, 726–736. doi: 10.1016/j.crvi.2008.07.014
Di, F., Wang, T., Ding, Y., Chen, X., Wang, H., Li, J., et al. (2020). Genetic mapping combined with a transcriptome analysis to screen for candidate genes responsive to abscisic acid treatment in Brassica napus embryos during seed germination. DNA Cell Biol. 39, 533–547. doi: 10.1089/dna.2019.5169
Ding, G. D., Zhao, Z. K., Wang, L., Zhang, D., Shi, L., and Xu, F. (2014). Identification and multiple comparisons of QTL and epistatic interaction conferring high yield under boron and phosphorus deprivation in Brassica napus. Euphytica 198, 337–351. doi: 10.1007/s10681-014-1110-8
Ding, X. Y., Xu, J. S., Huang, H., Ding, X., Xu, J., Huang, H., et al. (2020). Unraveling waterlogging tolerance-related traits with QTL analysis in reciprocal intervarietal introgression lines using genotyping by sequencing in rapeseed (Brassica napus L.). J. Integr. Agricult. 19, 1974–1983. doi: 10.1016/S2095-3119(19)62783-8
Dolatabadian, A., Patel, D. A., Edwards, D., and Batley, J. (2017). Copy number variation and disease resistance in plants. Theor. Appl. Genet. 130, 2479–2490. doi: 10.1007/s00122-017-2993-2
Duan, X., Wang, X., Jin, K., Wang, W., Liu, H., Liu, L., et al. (2019). Genetic dissection of root architectural traits by QTL and genome-wide association mapping in rapeseed (Brassica napus). Plant Breed. 138, 184–192. doi: 10.1111/pbr.12665
Egger, G., Liang, G., Aparicio, A., and Jones, P. A. (2004). Epigenetics in human disease and prospects for epigenetic therapy. Nature 429, 457–463. doi: 10.1038/nature02625
Elahi, N., Duncan, R. W., and Stasolla, C. (2015). Decreased seed oil production in FUSCA3 Brassica napus mutant plants. Plant Physiol. Biochem. 96, 222–230. doi: 10.1016/j.plaphy.2015.08.002
Fan, C., Cai, G., Qin, J., Li, Q., Yang, M., Wu, J., et al. (2010). Mapping of quantitative trait loci and development of allele-specific markers for seed weight in Brassica napus. Theoret. Appl. Genet. 121, 1289–1301. doi: 10.1007/s00122-010-1388-4
Feil, R., and Fraga, M. (2012). Epigenetics and the environment: emerging patterns and implications. Nat. Rev. Genet. 13, 97–109. doi: 10.1038/nrg3142
Fitt, B. D. L., Hu, B. C., Li, Z. Q., Liu, S. Y., Lange, R. M., Kharbanda, P. D., et al. (2008). Strategies to prevent spread of Leptosphaeria maculans (phoma stem canker) onto oilseed rape crops in China, costs and benefits. Plant Pathol. 57, 652–664. doi: 10.1111/j.1365-3059.2008.01841.x
Frank, S. A. (2003). Genetic variation of polygenic characters and the evolution of genetic degeneracy. J. Evolut. Biol. 16, 138–142. doi: 10.1046/j.1420-9101.2003.00485.x
Fu, Y., Wei, D., Dong, H., He, Y., Cui, Y., Mei, J., et al. (2015). Comparative quantitative trait loci for silique length and seed weight in Brassica napus. Scient. Rep. 5:14407. doi: 10.1038/srep14407
Fu, Y., Zhang, D. Q., Gleeson, M., Zhang, Y., Lin, B., Hua, S., et al. (2017). Analysis of QTL for seed oil content in Brassica napus by association mapping and QTL mapping. Euphytica 17:123. doi: 10.1007/s10681-016-1817-9
Fujita, M., Fujita, Y., Noutoshi, Y., Takahashi, F., Narusaka, Y., Yamaguchi-Shinozaki, K., et al. (2006). Crosstalk between abiotic and biotic stress responses: a current view from the points of convergence in the stress signaling networks. Curr. Opin. Plant Biol. 9, 436–442. doi: 10.1016/j.pbi.2006.05.014
Gabur, I., Chawla, H. S., Lopisso, D. T., von Tiedemann, A., Snowdon, R. J., and Obermeier, C. (2020). Gene presence-absence variation associates with quantitative Verticillium longisporum disease resistance in Brassica napus. Scient. Rep. 10:4131. doi: 10.1038/s41598-020-61228-3
Gan, L., Zhang, C. Y., Wang, X. D., Wang, H., Long, Y., Yin, Y. T., et al. (2013). Proteomic and comparative genomic analysis of two Brassica napus lines differing in oil content. J. Proteom. Res. 12, 4965–4978. doi: 10.1021/pr4005635
Garbers, C., DeLong, A., Deruére, J., Bernasconi, P., and Söll, D. (1996). A mutation in protein phosphatase 2A regulatory subunit A affects auxin transport in Arabidopsis. EMBO J. 15, 2115–2124. doi: 10.1002/j.1460-2075.1996.tb00565.x
Getinet, A., Rakow, G., and Downey, R. K. (1996). Agronomic performance and seed quality of Ethiopian mustard in Saskatchewan. Can. J. Plant Sci. 76, 387–392. doi: 10.4141/cjps96-069
Goodnight, C. J. (1988). Epistasis and the effect of founder events on the additive genetic variance. Evolution 42, 441–454. doi: 10.1111/j.1558-5646.1988.tb04151.x
Gromko, M. H. (1987). Genetic constraint on the evolution of courtship behavior in Drosophila melanogaster. Heredity 3:58. doi: 10.1038/hdy.1987.72
Grossmann, K. (2010). Auxin herbicides: current status of mechanism and mode of action. Pest Manage Sci. 66, 113–120. doi: 10.1002/ps.1860
Guan, M., Huang, X., Xiao, Z., Jia, L., Wang, S., Zhu, M., et al. (2019). Association mapping analysis of fatty acid content in different ecotypic rapeseed using mrMLM. Front. Plant Sci. 9:1872. doi: 10.3389/fpls.2018.01872
Gugel, P. K., and Petrie, G. A. (1992). History, occurrence, impact and control of blackleg of rapeseed. Can. J. Plant Pathol. 14, 36–45. doi: 10.1080/07060669209500904
Gyawali, S., Harrington, M., Durkin, J., Horner, K., Parkin, I. A., Hegedus, D. D., et al. (2016). Microsatellite markers used for genome-wide association mapping of partial resistance to Sclerotinia sclerotiorum in a world collection of Brassica napus. Mol. Breed. 36:72. doi: 10.1007/s11032-016-0496-5
Hackenberg, D., Asare-Bediako, E., Baker, A., Walley, P., Jenner, C., Greer, S., et al. (2020). Identification and QTL mapping of resistance to Turnip yellows virus (TuYV) in oilseed rape Brassica napus. Theory Appl. Genet. 133, 383–393. doi: 10.1007/s00122-019-03469-z
Hajduch, M., Casteel, J. E., Hurrelmeyer, K. E., Song, Z., Agrawal, G. K., and Thelen, J. J. (2006). Proteomic analysis of seed filling in Brassica napus. Plant Physiol. 141, 32–46. doi: 10.1104/pp.105.075390
Hansen, T. F., and Wagner, G. P. (2001). Modeling genetic architecture: a multilinear theory of gene interaction. Theoret. Populat. Biol. 59, 61–86. doi: 10.1006/tpbi.2000.1508
He, Y., Wu, D., Wei, D., Fu, Y., Cui, Y., Dong, H., et al. (2017). GWAS, QTL mapping and gene expression analyses in Brassica napus reveal genetic control of branching morphogenesis. Scient. Rep. 7:15971. doi: 10.1038/s41598-017-15976-4
Hua, Y., Zhang, D., Zhou, T., He, M., Ding, G., Shi, L., et al. (2016a). Transcriptomics-assisted quantitative trait locus fine mapping for the rapid identification of a nodulin 26-like intrinsic protein gene regulating boron efficiency in allotetraploid rapeseed. Plant Cell Environ. 39, 1601–1618. doi: 10.1111/pce.12731
Hua, Y., Zhou, T., Ding, G., Yang, Q., Shi, L., and Xu, F. (2016b). Physiological, genomic and transcriptional diversity in responses to boron deficiency in rapeseed genotypes. J. Exp. Bot. 67, 5769–5784. doi: 10.1093/jxb/erw342
Huang, C., Yang, M., Shao, D., Wang, Y., Wan, S., He, J., et al. (2020). Fine mapping of the BnUC2 locus related to leaf up-curling and plant semi-dwarfing in Brassica napus. BMC Genomics 21:530. doi: 10.1186/s12864-020-06947-7
Huang, J., Chen, F., Zhang, H., Ni, X., Wang, Y., Liu, H., et al. (2017). Dissection of additive, epistatic and QTL × environment effects involved in oil content variations in rapeseed. Plant Breed. 136, 728–737. doi: 10.1111/pbr.12522
Huang, Y. J., Jestin, C., Welham, S. J., King, G. J., Manzanares-Dauleux, M. J., Fitt, B. D., et al. (2016). Identification of environmentally stable QTL for resistance against Leptosphaeria maculans in oilseed rape (Brassica napus). Theory Appl. Genet. 129, 169–180. doi: 10.1007/s00122-015-2620-z
Huang, Y. J., Paillard, S., Kumar, V., King, G. J., Fitt, B. D. L., and Delourme, R. (2019). Oilseed rape (Brassica napus) resistance to growth of Leptosphaeria maculans in leaves of young plants contributes to quantitative resistance in stems of adult plants. PLoS One 14:e0222540. doi: 10.1371/journal.pone.0222540
Jan, H. U., Guan, M., Yao, M., Liu, W., Wei, D., Abbadi, A., et al. (2019). Genome-wide haplotype analysis improves trait predictions in Brassica napus hybrids. Plant Sci. 283, 157–164. doi: 10.1016/j.plantsci.2019.02.007
Javed, N., Geng, J., Tahir, M., McVetty, P. B. E., Li, G., and Duncan, R. W. (2016). Identification of QTL influencing seed oil content, fatty acid profile and days to flowering in Brassica napus L. Euphytica 191:207. doi: 10.1007/s10681-015-1565-2
Jian, H., Zhang, A., Ma, J., Wang, T., Yang, B., Shuang, L. S., et al. (2019). Joint QTL mapping and transcriptome sequencing analysis reveal candidate flowering time genes in Brassica napus L. BMC Genomics 20:21. doi: 10.1186/s12864-018-5356-8
Jiang, C., Shi, J., Li, R., Long, Y., Wang, H., Li, D., et al. (2014). Quantitative trait loci that control the oil content variation of rapeseed (Brassica napus L.). Theoret. Appl. Genet. 127, 957–968. doi: 10.1007/s00122-014-2271-5
Kaur, S., Cogan, N. O., Ye, G., Baillie, R. C., Hand, M. L., Ling, A. E., et al. (2009). Genetic map construction and QTL mapping of resistance to blackleg (Leptosphaeria maculans) disease in Australian canola (Brassica napus L.) cultivars. Theory Appl. Genet. 120, 71–83. doi: 10.1007/s00122-009-1160-9
Kebede, B., and Rahman, H. (2019). QTL mapping of root and aboveground biomass in the Brassica C genome using a B. napus population carrying genome content introgressed from B. oleracea. Mol. Breed. 39:169. doi: 10.1007/s11032-019-1064-6
Khan, S. U., Jiao, Y. M., Liu, S., Zhang, K., Khan, M. H. U., Zhai, Y., et al. (2019). Genome-wide association studies in the genetic dissection of ovule number, seed number, and seed weight in Brassica napus L. Industr. Crops Products 142:111877. doi: 10.1016/j.indcrop.2019.111877
Khanzada, H., Wassan, G. M., He, H., Mason, A. S., Keerio, A. A., Khanzada, S., et al. (2020). Differentially evolved drought stress indices determine the genetic variation of Brassica napus at seedling traits by genome-wide association mapping. J. Adv. Res. 24, 447–461. doi: 10.1016/j.jare.2020.05.019
Kim, S. Y., Park, B. S., Kwon, S. J., Kim, J., Lim, M. H., Park, Y. D., et al. (2007). Delayed flowering time in Arabidopsis and Brassica rapa by the overexpression of FLOWERING LOCUS C (FLC) homologs isolated from Chinese cabbage (Brassica rapa L.: ssp Pekinensis). Plant Cell Rep. 26, 327–336. doi: 10.1007/s00299-006-0243-1
Knoch, D., Abbadi, A., Grandke, F., Meyer, R. C., Samans, B., Werner, C. R., et al. (2020). Strong temporal dynamics of QTL action on plant growth progression revealed through high-throughput phenotyping in canola. Plant Biotechnol. J. 18, 68–82. doi: 10.1111/pbi.13171
Körber, N., Bus, A., Li, J., Parkin, I. A., Wittkop, B., Snowdon, R. J., et al. (2016). Agronomic and seed quality traits dissected by genome-wide association mapping in Brassica napus. Front. Plant Sci. 7:386. doi: 10.3389/fpls.2016.00386
Krzywinski, M., Schein, J., Birol, I., Connors, J., Gascoyne, R., Horsman, D., et al. (2009). Circos: An information aesthetic for comparative genomics. Genome Res. Adv. 19, 1639–1645. doi: 10.1101/gr.092759.109
Kumar, V., Paillard, S., Fopa-Fomeju, B., Falentin, C., Deniot, G., Baron, C., et al. (2018). Multi-year linkage and association mapping confirm the high number of genomic regions involved in oilseed rape quantitative resistance to blackleg. Theory Appl. Genet. 131, 1627–1643. doi: 10.1007/s00122-018-3103-9
Kutcher, H. R., Yu, F., and Brun, H. (2010). Improving blackleg disease management of Brassica napus from knowledge of genetic interactions with Leptosphaeria maculans. Can. J. Plant Pathol. 32, 29–34. doi: 10.1080/07060661003620961
Larkan, N. J., Raman, H., Lydiate, D. J., Robinson, S. J., Yu, F., Barbulescu, D. M., et al. (2016). Multi-environment QTL studies suggest a role for cysteine-rich protein kinase genes in quantitative resistance to blackleg disease in Brassica napus. BMC Plant Biol. 16:183. doi: 10.1186/s12870-016-0877-2
Li, B., Gao, J., Chen, J., Wang, Z., Shen, W., Yi, B., et al. (2020). Identification and fine mapping of a major locus controlling branching in Brassica napus. Theory Appl. Genet. 133, 771–783. doi: 10.1007/s00122-019-03506-x
Li, B., Zhao, W., Li, D., Chao, H., Zhao, X., Ta, N., et al. (2018). Genetic dissection of the mechanism of flowering time based on an environmentally stable and specific QTL in Brassica napus. Plant Sci. 277, 296–310. doi: 10.1016/j.plantsci.2018.10.005
Li, F., Chen, B., Xu, K., Gao, G., Yan, G., Qiao, J., et al. (2016b). A genome-wide association study of plant height and primary branch number in rapeseed (Brassica napus). Plant Sci. 242, 169–177. doi: 10.1016/j.plantsci.2015.05.012
Li, J. Q., Zhao, Z. K., Hayward, A., Cheng, H., and Fu, D. (2015). Integration analysis of quantitative trait loci for resistance to Sclerotinia sclerotiorum in Brassica napus. Euphytica 205, 483–489. doi: 10.1007/s10681-015-1417-0
Li, L., Luo, Y., Chen, B., Xu, K., Zhang, F., Li, H., et al. (2016a). A Genome-Wide Association Study Reveals New Loci for Resistance to Clubroot Disease in Brassica napus. Front. Plant Sci. 7:1483. doi: 10.3389/fpls.2016.01483
Li-Beisson, Y., Shorrosh, B., Beisson, F., Andersson, M. X., Arondel, V., Bates, P. D., et al. (2013). Acyl-lipid metabolism. Arabidopsis Book 11:e0161. doi: 10.1199/tab.0161
Liu, D., Yu, L., Wei, L., Yu, P., Wang, J., Zhao, H., et al. (2021). BnTIR: an online transcriptome platform for exploring RNA-seq libraries for oil crop Brassica napus. Plant Biotechnol. J. 19, 1895–1897. doi: 10.1111/pbi.13665
Liu, J., Wang, J., Wang, H., Wang, W., Zhou, R., Mei, D., et al. (2016). Multigenic control of pod shattering resistance in Chinese rapeseed germplasm revealed by genome-wide association and linkage analyses. Front. Plant Sci. 7:1058. doi: 10.3389/fpls.2016.01058
Liu, J., Wang, W., Mei, D., Wang, H., Fu, L., Liu, D., et al. (2016). Characterizing variation of branch angle and genome-wide association mapping in rapeseed (Brassica napus L.). Front. Plant Sci. 7:21. doi: 10.3389/fpls.2016.00021
Liu, S., Fan, C., Li, J., Cai, G., Yang, Q., Wu, J., et al. (2016). A genome wide association study reveals novel elite allelic variations in seed oil content of Brassica napus. Theoret. Appl. Genet. 129, 1203–1215. doi: 10.1007/s00122-016-2697-z
Liu, Y., Zhou, X., Yan, M., Wang, P., Wang, H., Xin, Q., et al. (2020). Fine mapping and candidate gene analysis of a seed glucosinolate content QTL, qGSL-C2, in rapeseed (Brassica napus L.). Theory Appl. Genet. 133, 479–490. doi: 10.1007/s00122-019-03479-x
Lu, K., Peng, L., Zhang, C., Lu, J., Yang, B., Xiao, Z., et al. (2017). Genome-wide association and transcriptome analyses reveal candidate genes underlying yield-determining traits in Brassica napus. Front. Plant Sci. 8:206. doi: 10.3389/fpls.2017.00206
Lu, K., Wei, L., Li, X., Wang, Y., Wu, J., Liu, M., et al. (2019). Whole-genome resequencing reveals Brassica napus origin and genetic loci involved in its improvement. Nat. Commun. 10:1154. doi: 10.1038/s41467-019-09134-9
Luo, Z., Wang, M., Long, Y., Huang, Y., Shi, L., Zhang, C., et al. (2017). Incorporating pleiotropic quantitative trait loci in dissection of complex traits: seed yield in rapeseed as an example. Theoret. Appl. Genet. 130, 1569–1585. doi: 10.1007/s00122-017-2911-7
Lynch, M., and Walsh, B. (1998). Genetics and Analysis of Quantitative Traits. Sunderland, MA: Sinauer Associates, .
Meinke, D. W. (2020). Genome-wide identification of EMBRYO-DEFECTIVE (EMB) genes required for growth and development in Arabidopsis. New Phytol. 226, 306–325. doi: 10.1111/nph.16071
Mi, H., Ebert, D., Muruganujan, A., Mills, C., Albou, L. P., Mushayamaha, T., et al. (2021). PANTHER version 16: a revised family classification, tree-based classification tool, enhancer regions and extensive API. Nucleic Acids Res. 49, D394–D403. doi: 10.1093/nar/gkaa1106
Muday, G. K., Brady, S. R., Argueso, C., Deruère, J., Kieber, J. J., and DeLong, A. (2006). RCN1-regulated phosphatase activity and EIN2 modulate hypocotyl gravitropism by a mechanism that does not require ethylene signaling. Plant Physiol. 141, 1617–1629. doi: 10.1104/pp.106.083212
Nguyen, T. C. T., Abrams, S. R., Friedt, W., and Snowdon, R. J. (2018). Quantitative trait locus analysis of seed germination, seedling vigour and seedling-regulated hormones in Brassica napus. Plant Breed. 137, 388–401. doi: 10.1111/pbr.12576
Paran, I., and Zamir, D. (2003). Quantitative traits in plants: beyond the QTL. Trends Genet. 19, 303–306. doi: 10.1016/S0168-9525(03)00117-3
Poole, R. L. (2007). The TAIR database. Methods Mol. Biol. 406, 179–212. doi: 10.1007/978-1-59745-535-0_8
Qasim, M. U., Zhao, Q., Shahid, M., Samad, R. A., Ahmar, S., Wu, J., et al. (2020). Identification of QTLs Containing resistance genes for Sclerotinia stem rot in Brassica napus using comparative transcriptomic studies. Front. Plant Sci. 11:776. doi: 10.3389/fpls.2020.00776
Qi, L., Mao, L., Sun, C., Pu, Y., Fu, T., Ma, C., et al. (2014). Interpreting the genetic basis of silique traits in Brassica napus using a joint QTL network. Plant Breed. 133, 52–60. doi: 10.1111/pbr.12131
Qian, L., Qian, W., and Snowdon, R. J. (2014). Sub-genomic selection patterns as a signature of breeding in the allopolyploid Brassica napus genome. BMC Genomics 15:1170. doi: 10.1186/1471-2164-15-1170
Qu, C., Jiang, H., and Zhang, L. (2014). Cloning and Enzymatic Activity Analysis of the Malonyl-CoA:acyl Carrier Protein Transacylase in Brassica napus Cultivars with Different Oil Content. Am. J. Plant Sci. 5, 2599–2610. doi: 10.4236/ajps.2014.518274
Qu, C., Jia, L., Fu, F., Zhao, H., Lu, K., Wei, L., et al. (2017). Genome-wide association mapping and Identification of candidate genes for fatty acid composition in Brassica napus L. using SNP markers. BMC Genomics 18:232. doi: 10.1186/s12864-017-3607-8
Quarrie, S. A., Pekic Quarrie, S., Radosevic, R., Rancic, D., Kaminska, A., Barnes, J. D., et al. (2006). Dissecting a wheat QTL for yield present in a range of environments: from the QTL to candidate genes. J. Exp. Bot. 57, 2627–2637. doi: 10.1093/jxb/erl026
Quijada, P. A., Udall, J. A., Lambert, B., and Osborn, T. C. (2006). Quantitative trait analysis of seed yield and other complex traits in hybrid spring rapeseed (Brassica napus L.): 1. Theoret. Appl. Genet. 113, 549–561. doi: 10.1007/s00122-006-0323-1
Qzer, H., Oral, E., and Dogru, U. (1999). Relationships between yield and yield components on currently improved spring rapeseed cultivars. Turkish. J. Agricult. Forest. 53, 603–607.
Raboanatahiry, N., Chao, H., Dalin, H., Pu, S., Yan, W., Yu, L., et al. (2018). QTL alignment for seed yield and yield related traits in Brassica napus. Front. Plant Sci. 9:1127. doi: 10.3389/fpls.2018.01127
Raboanatahiry, N., Chao, H., Guo, L., Gan, J., Xiang, J., Yan, M., et al. (2017). Synteny analysis of genes and distribution of loci controlling oil content and fatty acid profile based on QTL alignment map in Brassica napus. BMC Genomics 18:776. doi: 10.1186/s12864-017-4176-6
Raboanatahiry, N., Li, H., Yu, L., and Li, M. (2021). Rapeseed (Brassica napus): Processing Utilization, and Genetic Improvement. Agronomy 11:1776. doi: 10.3390/agronomy11091776
Radoev, M., Becker, H. C., and Ecke, W. (2008). Genetic analysis of heterosis for yield and yield components in rapeseed (Brassica napus L.) by quantitative trait locus mapping. Genetics 179, 1547–1558. doi: 10.1534/genetics.108.089680
Rahaman, M., Mamidi, S., and Rahman, M. (2018). Genome-wide association study of heat stresstolerance traits in spring-type Brassica napus L. under controlled conditions. Crop J. 6, 115–125. doi: 10.1016/j.cj.2017.08.003
Rahman, M., Mamidi, S., del Rio, L., Ross, A., Kadir, M. M., Rahaman, M. M., et al. (2016). Association mapping in Brassica napus (L.) accessions identifies a major QTL for blackleg disease resistance on chromosome A01. Mol. Breed. 36:90. doi: 10.1007/s11032-016-0513-8
Raman, H., Raman, R., Diffey, S., Qiu, Y., McVittie, B., Barbulescu, D. M., et al. (2018). Stable Quantitative Resistance Loci to Blackleg Disease in Canola (Brassica napus L.) Over Continents. Front. Plant Sci. 9:1622. doi: 10.3389/fpls.2018.01622
Raman, H., Raman, R., Kilian, A., Detering, F., Long, Y., Edwards, D., et al. (2013). A consensus map of rapeseed (Brassica napus L.) based on diversity array technology markers: applications in genetic dissection of qualitative and quantitative traits. BMC Genomics 14:277. doi: 10.1186/1471-2164-14-277
Raman, H., Raman, R., McVittie, B., Orchard, B., Qiu, Y., and Delourme, R. (2017). A Major Locus for Manganese Tolerance Maps on Chromosome A09 in a Doubled Haploid Population of Brassica napus L. Front. Plant Sci. 8:1952. doi: 10.3389/fpls.2017.01952
Raman, H., Raman, R., Qiu, Y., Yadav, A. S., Sureshkumar, S., Borg, L., et al. (2019). GWAS hints at pleiotropic roles for FLOWERING LOCUS T in flowering time and yield-related traits in canola. BMC Genomics 20:636. doi: 10.1186/s12864-019-5964-y
Raman, R., Diffey, S., Barbulescu, D. M., Coombes, N., Luckett, D., Salisbury, P., et al. (2020). Genetic and physical mapping of loci for resistance to blackleg disease in canola (Brassica napus L.). Scientific Rep. 10:4416. doi: 10.1038/s41598-020-61211-y
Raman, R., Diffey, S., Barbulescu, D. M., Coombes, N., Luckett, D., Salisbury, P., et al. (2012). Molecular mapping of qualitative and quantitative loci for resistance to Leptosphaeria maculans causing blackleg disease in canola (Brassica napus L.). Theory Appl. Genet. 125, 405–418. doi: 10.1007/s00122-012-1842-6
Rashotte, A. M., DeLong, A., and Muday, G. K. (2001). Genetic and chemical reductions in protein phosphatase activity alter auxin transport, gravity response, and lateral root growth. Plant Cell 13, 1683–1697. doi: 10.1105/tpc.010158
Remington, D. L., and Purugganan, M. D. (2003). Candidate gene, quantitative loci, and functional trait evolution in plants. Int. J. Plant Sci. 164, S7–S20. doi: 10.1086/367812
Rotmistrovsky, K., Jang, W., and Schuler, G. D. (2004). A web server for performing electronic PCR. Nucleic Acids Res. 32, W108–W112. doi: 10.1093/nar/gkh450
Schiessl, S. V., Quezada-Martinez, D., Tebartz, E., Snowdon, R. J., and Qian, L. (2019). The ernalization regulator FLOWERING LOCUS C is differentially expressed in biennial and annual Brassica napus. Scient. Rep. 9:14911. doi: 10.1038/s41598-019-51212-x
Schuler, G. D. (1997). Sequence mapping by electronic PCR. Genome Res. 7, 541–550. doi: 10.1101/gr.7.5.541
Sehgal, A., Sita, K., Siddique, K. H. M., Kumar, R., Bhogireddy, S., Varshney, R. K., et al. (2018). Drought or/and heat-stress effects on seed filling in food crops: impacts on functional biochemistry, seed yields, and nutritional quality. Front. Plant Sci. 9:1705. doi: 10.3389/fpls.2018.01705
Shannon, P., Markiel, A., Ozier, O., Baliga, N. S., Wang, J. T., Ramage, D., et al. (2003). Cytoscape: A software environment for integrated models of biomolecular interaction networks. Genome Res. 13, 2498–2504. doi: 10.1101/gr.1239303
Shen, W., Qin, P., Yan, M., Li, B., Wu, Z., Wen, J., et al. (2019). Fine mapping of a silique length and seed weight-related gene in Brassica napus. Theory Appl. Genet. 132, 2985–2996. doi: 10.1007/s00122-019-03400-6
Shen, Y., Yang, Y., Xu, E., Ge, X., Xiang, Y., and Li, Z. (2018). Novel and major QTL for branch angle detected by using DH population from an exotic introgression in rapeseed (Brassica napus L.). Theory Appl. Genet. 131, 67–78. doi: 10.1007/s00122-017-2986-1
Shi, J., Li, R., Qiu, D., Jiang, C., Long, Y., Morgan, C., et al. (2009). Unraveling the complex trait of crop yield with quantitative trait loci mapping in Brassica napus. Genetics 182, 851–861. doi: 10.1534/genetics.109.101642
Shi, J., Li, R., Zou, J., Long, Y., and Meng, J. (2011). A dynamic and complex network regulates the heterosis of yield-correlated traits in rapeseed (Brassica napus L.). PLoS One 6:e21645. doi: 10.1371/journal.pone.0021645
Shi, J., Zhan, J., Yang, Y., Ye, J., Huang, S., Li, R., et al. (2015). Linkage and regional association analysis reveal two new tightly-linked major-QTLs for pod number and seed number per pod in rapeseed (Brassica napus L.). Scient. Rep. 5:14481. doi: 10.1038/srep14481
Shi, L., Shi, T., Broadley, M. R., White, P. J., Long, Y., Meng, J., et al. (2013). High-throughput root phenotyping screens identify genetic loci associated with root architectural traits in Brassica napus under contrasting phosphate availabilities. Anna. Bot. 112, 381–389. doi: 10.1093/aob/mcs245
Shi, L., Yang, J. P., Liu, J., Li, R., Long, Y., Xu, F., et al. (2012). Identification of quantitative trait loci associated with low boron stress that regulate root and shoot growth in Brassica napus seedlings. Mol. Breed. 30, 393–406. doi: 10.1007/s11032-011-9629-z
Si, P., Mailer, R. J., Galwey, N., and Turner, D. (2003). Influence of genotype and environment on oil and protein concentrations of canola (Brassica napus L.) grown across southern Australia. Austral. J. Agricult. Res. 54, 397–407. doi: 10.1071/AR01203
Smith, J. M., and Haigh, J. (1974). The hitch-hiking effect of a favourable gene. Genet. Res. 23, 23–35. doi: 10.1017/s0016672300014634
Solovieff, N., Cotsapas, C., Lee, P. H., Purcell, S. M., and Smoller, J. W. (2013). Pleiotropy in complex traits: challenges and strategies. Nat. Rev. Genet. 14, 483–495. doi: 10.1038/nrg3461
Song, J. M., Liu, D. X., Xie, W. Z., Yang, Z., Guo, L., Liu, K., et al. (2021). BnPIR: Brassica napus pan-genome information resource for 1689 accessions. Plant Biotechnol. J. 19, 412–414. doi: 10.1111/pbi.13491
Stearns, F. W. (2010). One hundred years of pleiotropy: a retrospective. Genetics 186, 767–773. doi: 10.1534/genetics.110.122549
Stein, A., Coriton, O., Rousseau-Gueutin, M., Samans, B., Schiessl, S. V., Obermeier, C., et al. (2017). Mapping of homoeologous chromosome exchanges influencing quantitative trait variation in Brassica napus. Plant Biotechnol. 15, 1478–1489. doi: 10.1111/pbi.12732
Sun, F., Fan, G., Hu, Q., Zhou, Y., Guan, M., Tong, C., et al. (2017). The high-quality genome of Brassica napus cultivar ‘ZS11’ reveals the introgression history in semi-winter morphotype. Plant J. 92, 452–468. doi: 10.1111/tpj.13669
Sun, F., Liu, J., Hua, W., Sun, X., Wang, X., and Wang, H. (2016). Identification of stable QTLs for seed oil content by combined linkage and association mapping in Brassica napus. Plant Sci. 252, 388–399. doi: 10.1016/j.plantsci.2016.09.001
Sun, M., Hua, W., Liu, J., Huang, S., Wang, X., Liu, G., et al. (2012). Design of new genome- and gene-sourced primers and identification of QTL for seed oil content in a specially high-oil Brassica napus cultivar. PLoS One 7:e47037. doi: 10.1371/journal.pone.0047037
Szklarczyk, D., Franceschini, A., Wyder, S., Forslund, K., Heller, D., Huerta-Cepas, J., et al. (2015). STRING v10: protein-protein interaction networks, integrated over the tree of life. Nucleic Acids Res. 43, D447–D452. doi: 10.1093/nar/gku1003
Tabor, H. K., Risch, N. J., and Myers, R. M. (2002). Candidate-gene approaches for studying complex genetic traits: practical considerations. Nat. Rev. Genet. 3, 391–397. doi: 10.1038/nrg796
Tadege, M., Sheldon, C. C., Helliwell, C. A., Stoutjesdijk, P., Dennis, E. S., and Peacock, W. J. (2001). Control of flowering time by FLC orthologues in Brassica napus. Plant J. 28, 545–553. doi: 10.1046/j.1365-313x.2001.01182.x
Tchurikov, N. A. (2005). Molecular mechanisms of epigenetics. Biochem. Moscow 70, 406–423. doi: 10.1007/s10541-005-0131-2
Had, L., and Möllers, C. (2016). Genetic variation and inheritance of phytosterol and oil content in a doubled haploid population derived from the winter oilseed rape Sansibar × Oase cross. Theoret. Appl. Genet. 129, 181–199. doi: 10.1007/s00122-015-2621-y
Tirado, C. A. (2014). In book: Pathobiology of Human Disease Chapter: Epigenetics. Netherland: Elsevier doi: 10.1016/B978-0-12-386456-7.06601-6
Tudor, E. H., Jones, D. M., He, Z., Bancroft, I., Trick, M., Wells, R., et al. (2020). QTL-seq identifies BnaFT.A02 and BnaFLC.A02 as candidates for variation in vernalization requirement and response in winter oilseed rape (Brassica napus). Plant Biotechnol. J. 18, 2466–2481. doi: 10.1111/pbi.13421
Nagaharu, U. (1935). Genome analysis in Brassica with special reference to the experimental formation of B. napus and peculiar mode of fertilization. Jap. J. Bot. 7, 389–452.
Udall, J. A., Quijada, P. A., Lambert, B., and Osborn, T. C. (2006). Quantitative trait analysis of seed yield and other complex traits in hybrid spring rapeseed (Brassica napus L.): 2. Theoret. Appl. Genet. 113, 597–609. doi: 10.1007/s00122-006-0324-0
Verwoert, I. I., van der Linden, K. H., Walsh, M. C., Nijkamp, H. J., and Stuitje, A. R. (1995). Modification of Brassica napus seed oil by expression of the Escherichia coli fabH gene, encoding 3-ketoacyl-acyl carrier protein synthase III. Plant Mol. Biol. 27, 875–886. doi: 10.1007/BF00037016
Wagner, G. P., and Zhang, J. (2011). The pleiotropic structure of the genotype-phenotype map: the evolvability of complex organisms. Nat. Rev. Genet. 12, 204–213. doi: 10.1038/nrg2949
Wan, H., Chen, L., Guo, J., Li, Q., Wen, J., Yi, B., et al. (2017). Genome-wide association study reveals the genetic architecture underlying salt tolerance-related traits in rapeseed (Brassica napus L.). Front. Plant Sci. 8:593. doi: 10.3389/fpls.2017.00593
Wan, H., Wei, Y., Qian, J., Gao, Y., Wen, J., Yi, B., et al. (2018). Association mapping of salt tolerance traits at germination stage of rapeseed (Brassica napus L.). Euphytica 214:190. doi: 10.1007/s10681-018-2272-6
Wang, X., Zhang, C., Li, L., Fritsche, S., Endrigkeit, J., Zhang, W., et al. (2012). Unraveling the genetic basis of seed tocopherol content and composition in rapeseed (Brassica napus L.). PLoS One 7:e50038. doi: 10.1371/journal.pone.0050038
Wang, B., Wu, Z., Li, Z., Zhang, Q., Hu, J., Xiao, Y., et al. (2018). Dissection of the genetic architecture of three seed-quality traits and consequences for breeding in Brassica napus. Plant Biotechnol. J. 16, 1336–1348. doi: 10.1111/pbi.12873
Wang, F., and Guan, C. Y. (2010). Molecular mapping and identification of quantitative trait loci for yield components in rapeseed (Brasscia napus L.). Yi Chuan 32, 271–277. (In Chinese). doi: 10.3724/sp.j.1005.2010.00271
Wang, H., Cheng, H., Wang, W., Liu, J., Hao, M., Mei, D., et al. (2016a). Identification of BnaYUCCA6 as a candidate gene for branch angle in Brassica napus by QTL-seq. Scient. Rep. 6:38493. doi: 10.1038/srep38493
Wang, H., Guo, J., Lambert, K. N., and Lin, Y. (2007). Developmental control of Arabidopsis seed oil biosynthesis. Planta 226, 773–783. doi: 10.1007/s00425-007-0524-0
Wang, H., Zaman, Q. U., Huang, W., Mei, D., Liu, J., Wang, W., et al. (2019). QTL and Candidate Gene Identification for Silique Length Based on High-Dense Genetic Map in Brassica napus L. Front. Plant Sci. 10:1579. doi: 10.3389/fpls.2019.01579
Wang, J., Huang, Y., Zhang, Y., and Yao, J. (2011). Control of rapeseed clubroot by screened antagonistic microorganisms against Plasmodiophora brassicae. Chin. J. Oil Crop Sci. 33, 169–174.
Wang, J., Jian, H., Wei, L., Qu, C., Xu, X., Lu, K., et al. (2015). Genome-Wide Analysis of Seed Acid Detergent Lignin (ADL) and Hull Content in Rapeseed (Brassica napus L.). PLoS One 10:e0145045. doi: 10.1371/journal.pone.0145045
Wang, J., Kuang, L., Wang, X., Liu, G., Dun, X., and Wang, H. (2019). Temporal genetic patterns of root growth in Brassica napus L. revealed by a low-cost, high-efficiency hydroponic system. Theory Appl. Genet. 132, 2309–2323. doi: 10.1007/s00122-019-03356-7
Wang, J., Xian, X., Xu, X., Qu, C., Lu, K., Li, J., et al. (2017). Genome-wide association mapping of seed coat color in Brassica napus. J. Agricult. Food Chem. 65, 5229–5237. doi: 10.1021/acs.jafc.7b01226
Wang, N., Chen, B., Xu, K., Gao, G., Li, F., Qiao, J., et al. (2016). Association mapping of flowering time QTLs and insight into their contributions to rapeseed growth habits. Front. Plant Sci. 7:338. doi: 10.3389/fpls.2016.00338
Wang, N., Li, F., Chen, B., Xu, K., Yan, G., Qiao, J., et al. (2014). Genome-wide investigation of genetic changes during modern breeding of Brassica napus. Theoret. Appl. Genet. 127, 1817–1829. doi: 10.1007/s00122-014-2343-6
Wang, T., Wei, L., Wang, J., Xie, L., Li, Y. Y., Ran, S., et al. (2020). Integrating GWAS, linkage mapping and gene expression analyses reveals the genetic control of growth period traits in rapeseed (Brassica napus L.). Biotechnol. Biofuels 13:134. doi: 10.1186/s13068-020-01774-0
Wang, W., Zhang, Y., Ding, G. D., White, P. J., Broadley, M. R., Hammond, J. P., et al. (2019). Identification of QTLs for relative root traits associated with phosphorus efficiency in two culture systems in Brassica napus. Euphytica 215:192. doi: 10.1007/s10681-019-2512-4
Wang, X., Chen, L., Wang, A., Wang, H., Tian, J., Zhao, X., et al. (2016). Quantitative trait loci analysis and genome-wide comparison for silique related traits in Brassica napus. BMC Plant Biol. 16:71. doi: 10.1186/s12870-016-0759-7
Wang, X., Chen, Y., Thomas, C. L., Ding, G., Xu, P., Shi, D., et al. (2017). Genetic variants associated with the root system architecture of oilseed rape (Brassica napus L.) under contrasting phosphate supply. DNA Res. 24, 407–417. doi: 10.1093/dnares/dsx013
Wang, X., Long, Y., Yin, Y., Zhang, C., Gan, L., Liu, L., et al. (2015). New insights into the genetic networks affecting seed fatty acid concentrations in Brassica napus. BMC Plant Biol. 15:91. doi: 10.1186/s12870-015-0475-8
Wang, X., Wang, H., Long, Y., Li, D., Yin, Y., Tian, J., et al. (2013). Identification of QTLs associated with oil content in a high-oil Brassica napus cultivar and construction of a high-density consensus map for QTLs comparison in B. napus. PLoS One 8:e80569. doi: 10.1371/journal.pone.0080569
Wang, Y., Chen, W., Chu, P., Wan, S., Yang, M., Wang, M., et al. (2016). Mapping a major QTL responsible for dwarf architecture in Brassica napus using a single-nucleotide polymorphism marker approach. BMC Plant Biol. 16:178. doi: 10.1186/s12870-016-0865-6
Wang, Z., Fang, H., Chen, Y., Chen, K., Li, G., Gu, S., et al. (2014). Overexpression of BnWRKY33 in oilseed rape enhances resistance to Sclerotinia sclerotiorum. Mol. Plant Pathol. 15, 677–689. doi: 10.1111/mpp.12123
Wei, D. Y., Mei, J. Q., Fu, Y., Disi, J. O., Li, J., and Qian, W. (2014). Quantitative trait loci analyses for resistance to Sclerotinia sclerotiorum and flowering time in Brassica napus. Mol. Breed. 34, 1797–1804. doi: 10.1007/s11032-014-0139-7
Wei, L., Jian, H., Lu, K., Filardo, F., Yin, N., Liu, L., et al. (2016). Genome-wide association analysis and differential expression analysis of resistance to Sclerotinia stem rot in Brassica napus. Plant Biotechnol. J. 14, 1368–1380. doi: 10.1111/pbi.12501
Wei, X., Qiu, J., Yong, K., Fan, J., Zhang, Q., Hua, H., et al. (2021). A quantitative genomics map of rice provides genetic insights and guides breeding. Nat. Genet. 53, 243–253. doi: 10.1038/s41588-020-00769-9
West, J. S., Kharbanda, P. D., Barbetti, M. J., and Fitt, B. D. L. (2001). Epidemiology and management of Leptosphaeria maculans (phoma stem canker) on oilseed rape in Australia Canada and Europe. Plant Pathol. 50, 10–27. doi: 10.1046/j.1365-3059.2001.00546.x
Wrucke, D. F., Mamidi, S., and Rahman, M. (2018). Genome-wide association study for frost tolerance in canola (Brassica napus L.) under field conditions. J. Plant Biochem. Biotechnol. 28, 211–222. doi: 10.1007/s13562-018-0472-8
Wrucke, D. F., Talukder, Z. I., and Rahman, M. (2019). Genome-wide association study for frost tolerance in rapeseed/canola (Brassica napus) under simulating freezing conditions. Plant Breed. 139, 356–367. doi: 10.1111/pbr.12771
Wu, G. Z., and Xue, H. W. (2010). Arabidopsis β-ketoacyl-[acyl carrier protein] synthase I is crucial for fatty acid synthesis and plays a role in chloroplast division and embryo development. Plant Cell 22, 3726–3744. doi: 10.1105/tpc.110.075564
Wu, J., Cai, G., Tu, J., Li, L., Liu, S., Luo, X., et al. (2013). Identification of QTLs for resistance to Sclerotinia stem rot and BnaC.IGMT5.a as a candidate gene of the major resistant QTL SRC6 in Brassica napus. PLoS One 8:e67740. doi: 10.1371/journal.pone.0067740
Wu, J., Chen, P., Zhao, Q., Cai, G., Hu, Y., Xiang, Y., et al. (2019). Co-location of QTL for Sclerotinia stem rot resistance and flowering time in Brassica napus. Crop J. 7, 227–237. doi: 10.1016/j.cj.2018.12.007
Wu, J., Zhao, Q., Liu, S., Shahid, M., Lan, L., Cai, G., et al. (2016). Genome-wide association study identifies new loci for resistance to Sclerotinia stem rot in Brassica napus. Front. Plant Sci. 7:1418. doi: 10.3389/fpls.2016.01418
Xu, L., Hu, K., Zhang, Z., Guan, C., Chen, S., Hua, W., et al. (2016). Genome-wide association study reveals the genetic architecture of flowering time in rapeseed (Brassica napus L.). DNA Res. 23, 43–52. doi: 10.1093/dnares/dsv035
Yan, X. Y., Li, J. N., Fu, F. Y., Jin, M. Y., Chen, L., and Liu, L. Z. (2009). Co-location of seed oil content, seed hull content and seed coat color QTL in three different environments in Brassica napus L. Euphytica 170, 355–364. doi: 10.1007/s10681-009-0006-5
Yang, P., Shu, C., Chen, L., Xu, J., Wu, J., and Liu, K. (2012). Identification of a major QTL for silique length and seed weight in oilseed rape (Brassica napus L.). Theoret. Appl. Genet. 125, 285–296. doi: 10.1007/s00122-012-1833-7
Yang, S., Zhang, B., Liu, G., Hong, B., Xu, J., Chen, X., et al. (2018). A comprehensive and precise set of intervarietal substitution lines to identify candidate genes and quantitative trait loci in oilseed rape (Brassica napus L.). Theory Appl. Genet. 13, 2117–2129. doi: 10.1007/s00122-018-3140-4
Yang, Y., Shi, J., Wang, X., Liu, G., and Wang, H. (2016). Genetic architecture and mechanism of seed number per pod in rapeseed: elucidated through linkage and near-isogenic line analysis. Scient. Rep. 6:24124. doi: 10.1038/srep24124
Yang, Y. W., Lai, K. N., Tai, P. Y., and Li, W. H. (1999). Rates of nucleotide substitution in angiosperm mitochondrial DNA sequences and dates of divergence between Brassica and other angiosperm lineages. J. Mol. Evolut. 48, 597–604. doi: 10.1007/PL00006502
Ye, J., Yang, Y., Chen, B., Shi, J., Luo, M., Zhan, J., et al. (2017). An integrated analysis of QTL mapping and RNA sequencing provides further insights and promising candidates for pod number variation in rapeseed (Brassica napus L.). BMC Genomics 18:71. doi: 10.1186/s12864-016-3402-y
Yu, C. W., Chang, K. Y., and Wu, K. (2016). Genome-Wide Analysis of Gene Regulatory Networks of the FhadHDA6-FLD Complex in Arabidopsis. Front. Plant Sci. 7:555. doi: 10.3389/fpls.2016.00555
Yu, K. J., Wang, X. D., Li, W. J., Sun, L., Peng, Q., Chen, F., et al. (2019). Identification and physical mapping of QTLs associated with flowering time in Brassica napus L. Euphytica 215:152. doi: 10.1007/s10681-019-2480-8
Zhang, F., Huang, J., Tang, M., Cheng, X., Liu, Y., Tong, C., et al. (2019). Syntenic quantitative trait loci and genomic divergence for Sclerotinia resistance and flowering time in Brassica napus. J. Integr. Plant Biol. 61, 75–88. doi: 10.1111/jipb.12754
Zhang, F., Xiao, X., Xu, K., Cheng, X., Xie, T., Hu, J., et al. (2020). Genome-wide association study (GWAS) reveals genetic loci of lead (Pb) tolerance during seedling establishment in rapeseed (Brassica napus L.). BMC Genomics 21:139. doi: 10.1186/s12864-020-6558-4
Zhang, F. G., Xiao, X., Yan, G. X., Hu, J., Cheng, X., Li, L., et al. (2018). Association mapping of cadmium-tolerant QTLs in Brassica napus L. and insight into their contributions to phytoremediation. Environ. Exp. Bot. 155, 420–428. doi: 10.1016/j.envexpbot.2018.07.014
Zhang, K., Nie, L., Cheng, Q., Yin, Y., Chen, K., Qi, F., et al. (2019). Effective editing for lysophosphatidic acid acyltransferase 2/5 in allotetraploid rapeseed (Brassica napus L.) using CRISPR-Cas9 system. Biotechnol. Biofuels 12:225. doi: 10.1186/s13068-019-1567-8
Zhang, L., Li, S., Chen, L., and Yang, G. (2012). Identification and mapping of a major dominant quantitative trait locus controlling seeds per silique as a single Mendelian factor in Brassica napus L. Theoret. Appl. Genet. 125:695. doi: 10.1007/s00122-012-1861-3
Zhang, L., Yang, G., Liu, P., Hong, D., Li, S., and He, Q. (2011). Genetic and correlation analysis of silique-traits in Brassica napus L. by quantitative trait locus mapping. Theoret. Appl. Genet. 122, 21–31. doi: 10.1007/s00122-010-1419-1
Zhang, X., Lu, G., Long, W., Zou, X., Li, F., and Nishio, T. (2014). Recent progress in drought and salt tolerance studies in Brassica crops. Breed. Sci. 64, 60–73. doi: 10.1270/jsbbs.64.60
Zhang, Y., Li, X., Chen, W., Wen, J., Shen, J., Ma, C., et al. (2011). Identification of two major QTL for yellow seed color in two crosses of resynthesized Brassica napus line No. 2127-17. Mol. Breed. 28, 335–342. doi: 10.1007/s11032-010-9486-1
Zhao, J., Huang, J., Chen, F., Xu, F., Ni, X., Xu, H., et al. (2012). Molecular mapping of Arabidopsis thaliana lipid-related orthologous genes in Brassica napus. Theoret. Appl. Genet. 124, 407–421. doi: 10.1007/s00122-011-1716-3
Zhao, J., Udall, J. A., Quijada, P. A., Grau, C. R., Meng, J., and Osborn, T. C. (2006). Quantitative trait loci for resistance to Sclerotinia sclerotiorum and its association with a homeologous non-reciprocal transposition in Brassica napus L. Theory Appl. Genet. 112, 509–516. doi: 10.1007/s00122-005-0154-5
Zhao, J. Y., Becker, H. C., Ding, H. D., Zhang, Y. F., Zhang, D. Q., and Ecke, W. (2005). QTL of three agronomically important traits and their interactions with environment in a European x Chinese rapeseed population. Yi Chuan Xue Bao 32, 969–978.
Zhao, Q., Wu, J., Cai, G., Yang, Q., Shahid, M., Fan, C., et al. (2019). A novel quantitative trait locus on chromosome A9 controlling oleic acid content in Brassica napus. Plant Biotechnol. J. 17, 2313–2324. doi: 10.1111/pbi.13142
Zhao, W., Wang, X., Wang, H., Tian, J., Li, B., Chen, L., et al. (2016). Genome-wide identification of QTL for seed yield and yield-related traits and construction of a high-density consensus map for QTL comparison in Brassica napus. Front. Plant Sci. 7:17. doi: 10.3389/fpls.2016.00017
Zhao, W., Zhang, L., Chao, H., Wang, H., Ta, N., Li, H., et al. (2019). Genome-wide identification of silique-related traits based on high-density genetic linkage map in Brassica napus. Mol. Breed. 39:86. doi: 10.1007/s11032-019-0988-1
Zhao, Z., Wu, L., Nian, F., Ding, G., Shi, T., Zhang, D., et al. (2012). Dissecting quantitative trait loci for boron efficiency across multiple environments in Brassica napus. PLoS One 7:e45215. doi: 10.1371/journal.pone.0045215
Zhao, Z., Wu, L., Nian, F., Ding, G., Shi, T., Zhang, D., et al. (2019). QTL analysis of four yield-related traits for Brassica napus L. in multiple environments. Mol. Breed. 39:166. doi: 10.1007/s11032-019-1067-3
Zhou, Q., Han, D., Mason, A. S., Zhou, C., Zheng, W., Li, Y., et al. (2018). Earliness traits in rapeseed (Brassica napus): SNP loci and candidate genes identified by genome-wide association analysis. DNA Res. 25, 229–244. doi: 10.1093/dnares/dsx052
Zhu, M., and Zhao, S. (2007). Candidate gene identification approach: progress and challenges. Int. J. Biol. Sci. 3, 420–427. doi: 10.7150/ijbs.3.420
Zhu, Q., King, G. J., Liu, X., Shan, N., Borpatragohain, P., Baten, A., et al. (2019). Identification of SNP loci and candidate genes related to four important fatty acid composition in Brassica napus using genome wide association study. PLoS One 14:e0221578. doi: 10.1371/journal.pone.0221578
Zhu, Y., Ye, J., Zhan, J., Zheng, X., Zhang, J., Shi, J., et al. (2020). Validation and characterization of a seed number per silique quantitative trait locus qSN.A7 in rapeseed (Brassica napus L.). Front. Plant Sci. 11:68. doi: 10.3389/fpls.2020.00068
Zou, J., Mao, L., Qiu, J., Wang, M., Jia, L., Wu, D., et al. (2019). Genome-wide selection footprints and deleterious variations in young Asian allotetraploid rapeseed. Plant Biotechnol. J. 17, 1998–2010. doi: 10.1111/pbi.13115
Keywords: Brassica napus, quantitative genomic map, oil content, seed yield, disease, candidate genes, gene expression, structural variation
Citation: Raboanatahiry N, Chao H, He J, Li H, Yin Y and Li M (2022) Construction of a Quantitative Genomic Map, Identification and Expression Analysis of Candidate Genes for Agronomic and Disease-Related Traits in Brassica napus. Front. Plant Sci. 13:862363. doi: 10.3389/fpls.2022.862363
Received: 25 January 2022; Accepted: 15 February 2022;
Published: 11 March 2022.
Edited by:
Yong Xiao, Chinese Academy of Tropical Agricultural Sciences, ChinaReviewed by:
Zaiyun Li, Huazhong Agricultural University, ChinaNian Wang, Huazhong Agricultural University, China
Copyright © 2022 Raboanatahiry, Chao, He, Li, Yin and Li. This is an open-access article distributed under the terms of the Creative Commons Attribution License (CC BY). The use, distribution or reproduction in other forums is permitted, provided the original author(s) and the copyright owner(s) are credited and that the original publication in this journal is cited, in accordance with accepted academic practice. No use, distribution or reproduction is permitted which does not comply with these terms.
*Correspondence: Maoteng Li, limaoteng426@hust.edu.cn