- 1Centro de Ciências Biológicas, State University of Londrina, Londrina, Brazil
- 2Laboratório de Biotecnologia, Instituto de Desenvolvimento Rural do Paraná, Embrapa Café, Londrina, Brazil
- 3Tropical Melhoramento e Genética (TMG), Cambé, Brazil
Although Brazil is currently the largest soybean producer in the world, only a small number of studies have analyzed the genetic diversity of Brazilian soybean. These studies have shown the existence of a narrow genetic base. The objectives of this work were to analyze the population structure and genetic diversity, and to identify selection signatures in the genome of soybean germplasms from different companies in Brazil. A panel consisting of 343 soybean lines from Brazil, North America, and Asia was genotyped using genotyping by sequencing (GBS). Population structure was assessed by Bayesian and multivariate approaches. Genetic diversity was analyzed using metrics such as the fixation index, nucleotide diversity, genetic dissimilarity, and linkage disequilibrium. The software BayeScan was used to detect selection signatures between Brazilian and Asian accessions as well as among Brazilian germplasms. Region of origin, company of origin, and relative maturity group (RMG) all had a significant influence on population structure. Varieties belonging to the same company and especially to the same RMG exhibited a high level of genetic similarity. This result was exacerbated among early maturing accessions. Brazilian soybean showed significantly lower genetic diversity when compared to Asian accessions. This was expected, because the crop’s region of origin is its main genetic diversity reserve. We identified 7 genomic regions under selection between the Brazilian and Asian accessions, and 27 among Brazilian varieties developed by different companies. Associated with these genomic regions, we found 96 quantitative trait loci (QTLs) for important soybean breeding traits such as flowering, maturity, plant architecture, productivity components, pathogen resistance, and seed composition. Some of the QTLs associated with the markers under selection have genes of great importance to soybean’s regional adaptation. The results reported herein allowed to expand the knowledge about the organization of the genetic variability of the Brazilian soybean germplasm. Furthermore, it was possible to identify genomic regions under selection possibly associated with the adaptation of soybean to Brazilian environments.
Introduction
Soybean [Glycine max (L.) Merril] is one of the crops of most economic importance in the world. Brazil is currently the largest producer in the world, responsible for nearly 40% of the global soybean supply (FAOSTAT, 2019; Instituto Brasileiro de Geografia e Estatística [IBGE], 2021). This Brazilian leadership in world soybean production has been possible through the efficient exploration of genetic diversity by different breeding programs. Thus, a better understanding of how to preserve and increase this diversity as well as identify selection signatures in the soybean genome associated with its adaptation to Brazilian growing environments is paramount to allowing further genetic gains in yield.
Although the genetic diversity present in soybean germplasms all over the world have been studied extensively, in Brazil, these studies have been scarce. Brazilian germplasms present a particularly narrow genetic base when compared to Chinese, European, and North American breeding programs (Priolli et al., 2013; Gwinner et al., 2017; Liu et al., 2017; Žulj Mihaljević et al., 2020). In their seminal paper of 1986, Hiromoto and Vello (1986) found that the genetic base of Brazilian soybean largely came from only 26 common ancestors, with only 4 (CNS, Tokyo, Roanoke, and S-100) contributing approximately half of this base. The genetic diversity of soybean germplasms in Brazilian breeding programs stayed relatively stable from 1970 to 2000 (Priolli et al., 2004). More recently, a study of the genetic base of 444 Brazilian cultivars found that while the number of common ancestors had risen to 60, a small subset of these were responsible for most of the genetic base (Wysmierski and Vello, 2013).
Crop domestication and founding events contribute to a reduction in the available genetic diversity of several crops (Hyten et al., 2006; Liu et al., 2019). Soybean was domesticated approximately 5,000 years ago in China (Xu et al., 2002; Guo et al., 2010; Li et al., 2010; Han et al., 2016; Wang J. et al., 2016; Jeong et al., 2019) and has since been introduced to countries all over the world. Soybean was introduced in North America and Europe in the nineteenth century, and until approximately 1940, most soybean varieties grown in the United States were introduced materials. Soybean breeding programs then began producing cultivars adapted to North American growing environments, establishing the North American soybean’s genetic base (Carter et al., 2004).
Around 20 years after the United States started its breeding programs, a small number of North American cultivars were brought from the United States to Brazil. Breeders started crossing these materials among themselves and with other sources to generate cultivars adapted to the growing environments in Brazil. This sequence of founding events formed genetic diversity bottlenecks that culminated with the narrow genetic base now seen in Brazilian soybean germplasms (Miranda et al., 2007; Contreras-Soto et al., 2017a; Gwinner et al., 2017). An increase in the genetic diversity available to breeders could be achieved by using genotypes from different origins that have a high level of genetic dissimilarity or divergence (de Almeida et al., 1999). Thus, a quantitative analysis of the genetic dissimilarity between pairs or potential parentals becomes an important resource for breeding programs, allowing for a more efficient choice of parentals to generate progenies with high genetic variance (Vieira et al., 2005; de Almeida et al., 2012), which could potentially increase the selection gain.
While founding events and domestication cause a decrease in the genetic diversity of a crop, this reduction isn’t uniform throughout the entire genome. Genomic regions associated with traits of agronomic importance and adaptation to the environment are invariably under greater selection pressure. Thus, events such as domestication as well as artificial selection by breeding programs can cause a sharp reduction in the genetic diversity in specific parts of a crop’s genome (Maynard Smith and Haigh, 1974; Vigouroux et al., 2002). These signatures of selection have been used to study several crops’ domestication and breeding history and to direct introgression efforts aimed at increasing a germplasms’ genetic diversity at specific genomic regions (Tanksley and McCouch, 1997; Jun et al., 2011; Ge et al., 2012; Wang J. et al., 2016; Grainger et al., 2018; Saleem et al., 2021; Zhang et al., 2021).
The last decade has seen the advent of techniques that combine next generation sequencers with multiplex sequencing approaches to allow for the high throughput genotyping of large diversity panels at a relatively low cost. Approaches such as genotyping by sequencing (GBS) (Elshire et al., 2011) greatly reduced genotyping costs by focusing the sequencing and variant calling on euchromatic genomic regions with a high concentration of coding sequences, which makes it a useful tool for studying the genetic diversity present in soybean germplasms (Bruce et al., 2019).
We used GBS to assess a diverse collection of soybean accessions from different breeding companies and geographic regions of origin to (1) investigate the population structure and genetic diversity among soybean cultivars from different Brazilian breeding programs, (2) identify genomic regions under selection among cultivars developed by different companies as well as between different geographic regions, and (3) identify quantitative trait loci (QTLs) present in those genomic regions.
Materials and Methods
Plant Materials and Single Nucleotide Polymorphism Genotyping
A panel consisting of 343 soybean accessions was used in this study. Of those, 263 were Brazilian cultivars developed by several different companies in the last two decades, 54 were accessions collected on the Asian continent in the twentieth century, and 26 were North American genotypes developed between 1956 and 1995 (Supplementary Table 1).
Genomic DNA was extracted from leaf tissue from a single plant per genotype using the DNA-easy Plant Kit (Qiagen) following the manufacturer protocols. The GBS libraries were constructed at the Plate-Forme d’Analyses Génomiques (Université Laval, Québec, Canada) following a protocol established by Elshire et al. (2011) using the ApeKI restriction enzyme. Library sequencing was performed with the Illumina HiSeq2000 system.
Briefly, after demultiplexing was performed based on barcodes, a sample of 1,000,000 reads was used for quality control (QC) using the FastQC platform. Reads with invalid barcodes or containing adapter or primer sequences were filtered. Furthermore, low quality bases (PHRED score < 15) were removed from the 3’-end of each sequence; reads shorter than 20 bp at the end of this process were also filtered.
Single nucleotide polymorphism (SNP) calling was performed using Platypus (Rimmer et al., 2014) and bcftools (Li, 2011) on sequences aligned to the soybean reference genome Gmax 2.01 using the Bowtie package (Langmead and Salzberg, 2012). The parameters used in both pipelines were similar. In brief, to be considered in the Platypus pipeline, SNPs needed to present a PHRED score higher than 15 and have a sequencing coverage value higher than 5 × in at least 20% of the reads that contained the polymorphism. In the bcftools pipeline, the minimum QUAL and PHRED scores were maintained at 15, while minimum coverage was set to 15×.
The resulting set of markers underwent further QC using TASSEL software (Bradbury et al., 2007). The SNPs with a minor allele frequency less than 5%, call rate below 75%, and heterozygosity higher than 50% were filtered. Subsequently, missing data was imputed using the LD-kNNi method (Money et al., 2015).
Population Structure and Genetic Diversity Analysis
Population structure was assessed by Bayesian and multivariate approaches. We performed a population structure analysis using a model-based Bayesian approach in Structure v.2.3.4 (Pritchard et al., 2000) through the Structure_threader plug-in (Pina-Martins et al., 2017). The SNPs used in the analysis were filtered based on linkage disequilibrium (LD; R2< 0.25) using PLINK software (Purcell et al., 2007), which resulted in a set of 959 SNPs. The K-values varied from 1 to 8, with 10 runs for each K-value. Burn-in time and replication number were set to 50,000 and 75,000, respectively. The K-values that best fit the data were determined using the Evanno approach (Evanno et al., 2005). This method uses a ΔK-value to compare variations in the log probability of the data for successive K-values. Population stratification was also explored using a principal component analysis in the TASSEL software (Bradbury et al., 2007).
An LD decay analysis was performed using PLINK (Purcell et al., 2007) based on 2,175 SNPs. Average LD decay was computed for each chromosome using the R2-value for all pairwise comparisons between SNPs in 1 Mb windows. This analysis was conducted for the Brazilian set of cultivars as well as for the Asian set of accessions. To visualize the average LD decay profile, a cubic spline regression approach was adopted using the ggplot2 package in R (Wickham, 2016). Genome-wide LD decay was estimated using the spline method with the LD output from all chromosomes.
Diversity estimates were computed for the Brazilian and Asian accessions separately. The nucleotide diversity index (π) and fixation indexes (FST) were calculated for each SNP site using VCFtools v0.1.16 (Danecek et al., 2011). Genetic distance among Brazilian cultivars was calculated using the IBS (Identity by State) metric in TASSEL. The genetic distances were used in the construction of a dendrogram using the UPGMA (unweighted pair group method with arithmetic mean) algorithm. The grouping pattern was then analyzed as a function of RMG and company of origin to better understand the influence of those factors on population stratification.
Identification of Selection Signatures in the Soybean Genome
To detect selection footprints between the Brazilian and Asian accessions as well as among different Brazilian companies’ germplasms, we used an outlier locus detection approach through BayeScan (Foll and Gaggiotti, 2008). We performed 20 pilot runs of 5,000 iterations each, followed by 550,000 iterations on a sample size of 50,000 with a thinning interval of 10. The prior odds were set to 10, and SNPs with a q-value < 0.1 were considered statistically significant.
Identification of Quantitative Trait Loci Present in Genomic Regions Under Selection
To identify QTLs located in genomic regions under selection, we used soybean QTL data from the Soybase database (see text footnote 1). All SNPs under selection as detected by BayeScan were mapped to the soybean reference genome. A SNP was within a QTL’s genomic region if its distance to the QTL was shorter than the average LD decay distance previously defined for the panel.
Results
Genotyping by Sequencing
The SNP calling by Platypus and bcftools identified 346,919 and 498,931 polymorphisms, respectively. Upon applying the QC steps, 33,359 and 24,865 SNPs were left, of which 12,757 were identified by both pipelines. Further filtering by TASSEL software resulted in a set of 2,175 high quality markers that were used in the subsequent analyses.
The SNP distribution throughout the genome was not uniform. Heterochromatic regions presented a markedly lower number of markers, with 87% of the sequenced polymorphisms concentrated in euchromatic regions. On average, 109 SNPs were identified per chromosome. Chromosome 18 harbored the highest number of SNPs (231), while chromosome 12 had the lowest number (68).
As expected from highly endogamic lines, on average, the SNPs presented with low heterozygosity (5.47%). The average minor allele frequency value after QC was 0.171, with a median of 0.136 and standard deviation of 0.115. Among the SNPs sequenced in this panel, 59.8% were transitions, and 40.2% were transversions.
Population Structure and Genetic Diversity
The best K-values chosen by the Evanno method were 2 and 3 (Figure 1A). Given the accessions’ geographic regions of origin, K = 3 was further explored. Each subpopulation concentrated individuals from a different geographic region (Figure 1B). Subpopulation 1 was formed mostly by Asian genotypes. Subpopulation 2 corresponded mainly to late maturing Brazilian genotypes and a single North American genotype. Subpopulation 3 concentrated most of the North American genotypes and early maturing Brazilian genotypes.
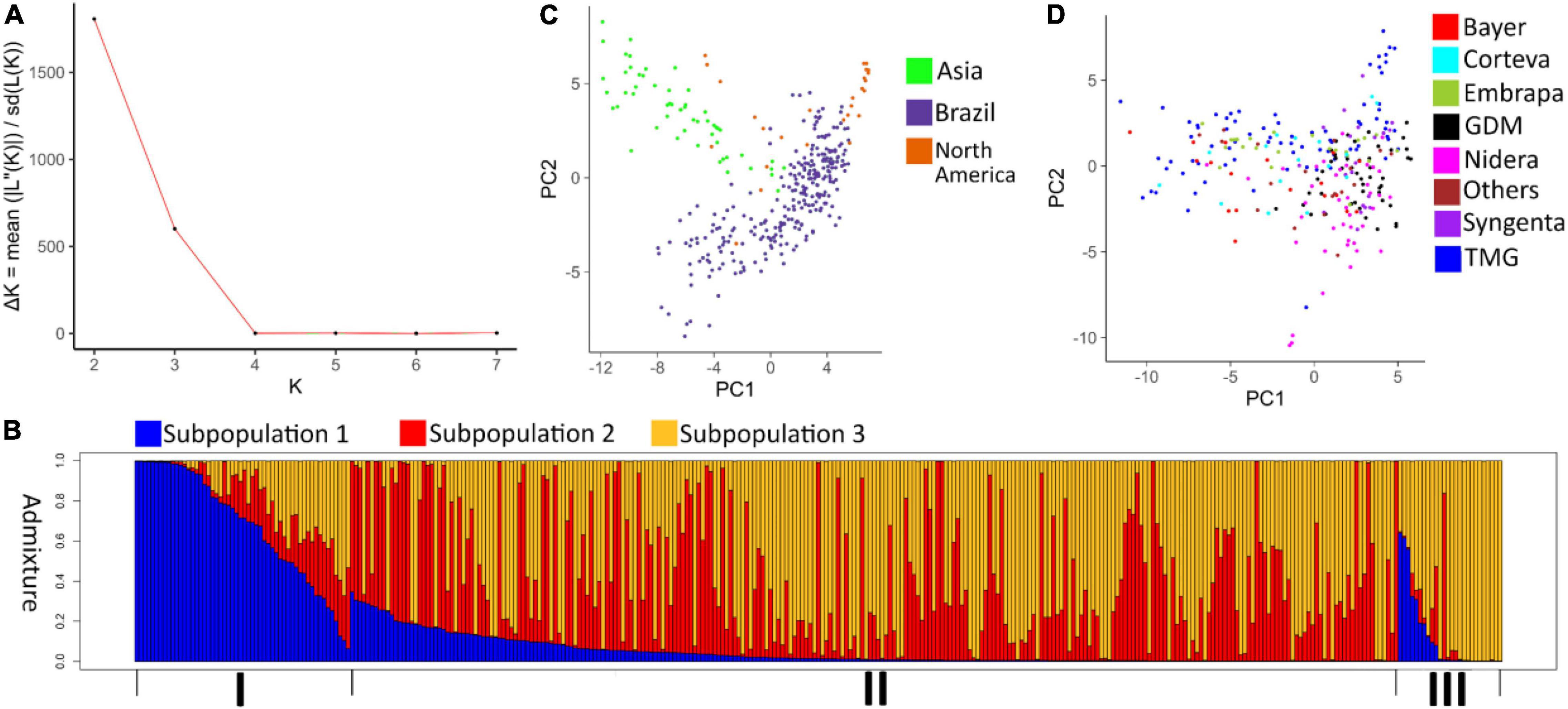
Figure 1. (A) Delta K-values for k = 1–8. (B) Analysis of the population structure using 343 soybean accessions with K = 3. (I) corresponds to the group of soybeans of Asian origin; (II) formed by Brazilian accessions; (III) formed by North American accessions. (C) Principal component analysis of 343 soybean accessions classified by geographic origin. (D) Principal component analysis of 247 Brazilian soybean cultivars classified by company of origin.
The population structure was also assessed by a principal component analysis. Using the first 2 principal components (explaining 7.6 and 3.8% of the total variation, respectively) as a basis, the genotypes’ distribution indicated the formation of 3 clusters, each formed primarily by accessions from a different geographic region (Figure 1C). A principal component analysis was also used to assess the stratification and diversity among Brazilian genotypes from different companies. In this analysis, the first two components explained 7 and 3.1% of the total variation. Cultivars from companies such TMG and Nidera showed a wider genetic base compared to companies such as GDM and Syngenta (Figure 1D).
A smaller average FST value was observed among Brazilian genotypes from different companies (0.0417), while a larger value was obtained when comparing Brazilian genotypes to Asian genotypes (0.102). This indicates a high level of genetic similarity among varieties developed by Brazilian companies, especially when compared to Asian genotypes. Average π among Brazilian genotypes was markedly lower than that for the Asian genotypes, 2.53 × 10–6 vs. 3.00 × 10–6.
The LD decayed as a function of the physical distance between markers in both the Brazilian and Asian genotypes. The average R2 was 0.237 among Brazilian genotypes and 0.161 among Asian genotypes. The distance over which the LD decayed to below 0.2 was around 230 kb in the Brazilian genotypes, whereas in the Asian genotypes, this value was much lower, only 94 kb (Figure 2).
We observed two grouping patterns. First, cultivars belonging to the same RMG grouped together, with early maturing cultivars presenting a higher degree of genetic similarity among themselves. Second, materials developed by the same company showed higher levels of similarity, which drove the grouping pattern (Supplementary Figure 1).
Identification of Selection Signatures in the Soybean Genome
BayeScan was used to detect selection signatures in the Brazilian × Asian genotypes and among Brazilian genotypes from different companies. In the Brazilian × Asian genotypes, we used 2,099 SNPs and 317 accessions, of which 263 were Brazilian cultivars and 54 were Asian genotypes. We identified 7 SNPs under selection in five different chromosomes, 4, 8, 10, 16, and 19 (Figure 3A and Supplementary Table 2). All SNPs except for SNP 1.7 were fixed among the Brazilian genotypes. In the second analysis, 260 Brazilian cultivars from 10 different companies and 1,850 SNPs were used. In total, 27 SNPs under selection in 9 different chromosomes were identified (Figure 3B and Supplementary Table 3).
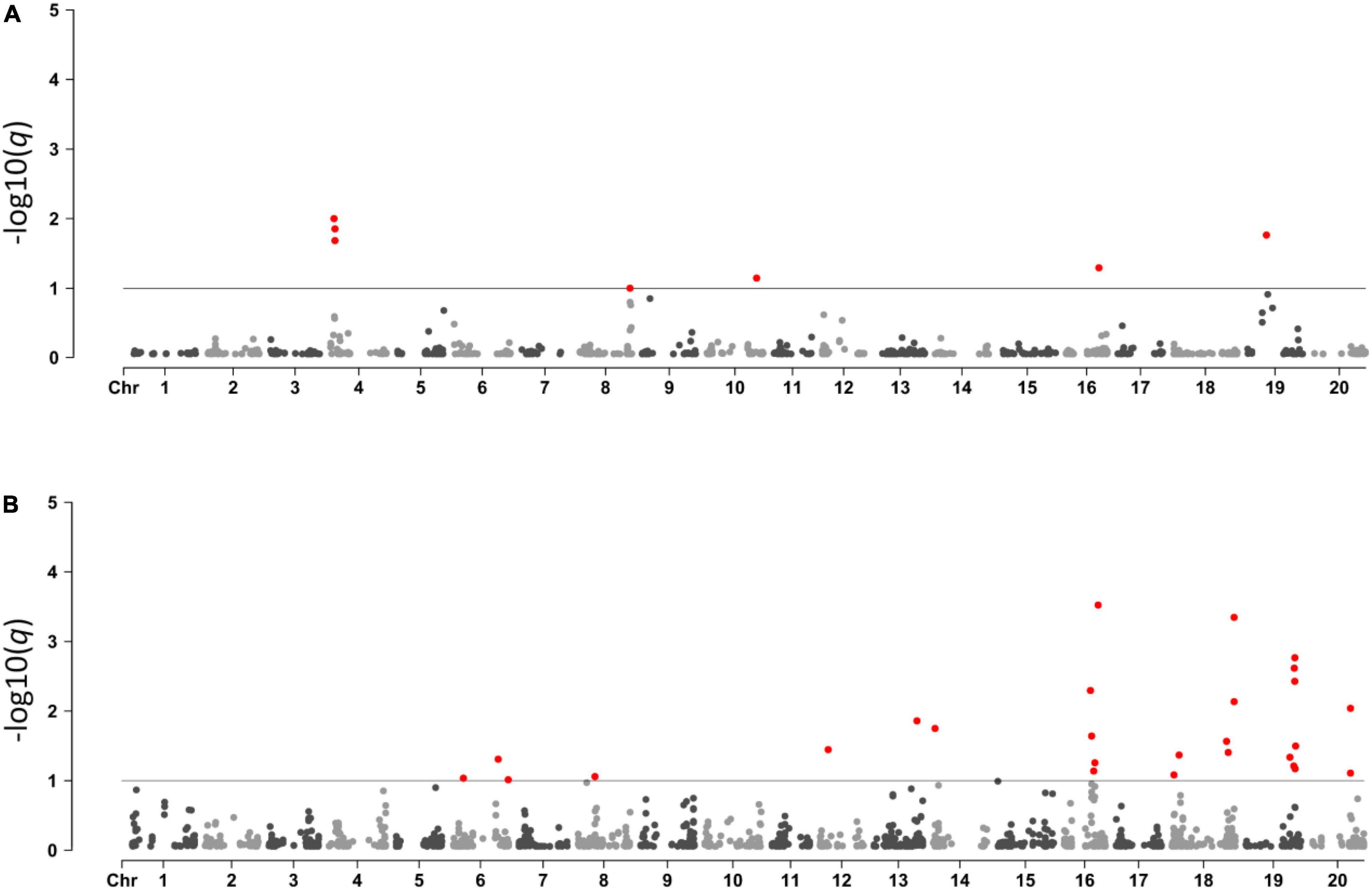
Figure 3. Manhattan plots showing SNPs under selection between Brazilian and Asian lines (A) and among different Brazilian breeding programs (B).
Candidate Genes and Associated Quantitative Trait Loci Under Selection
The upstream and downstream genomic regions within the LD decay distance (230 kb) of each SNP under selection were analyzed. Previously described QTLs (available at the Soybase’s QTL database) present in those regions are reported in Supplementary Table 4. Fourteen QTLs under selection were identified between the Brazilian and Asian genotypes (Figure 4 and Supplementary Table 4). We found QTLs associated with characteristics of relevance to the adaptation and commercial use of soybean in Brazil, for example, the number of days to flowering, number of days to maturity, plant height, and pod shattering.
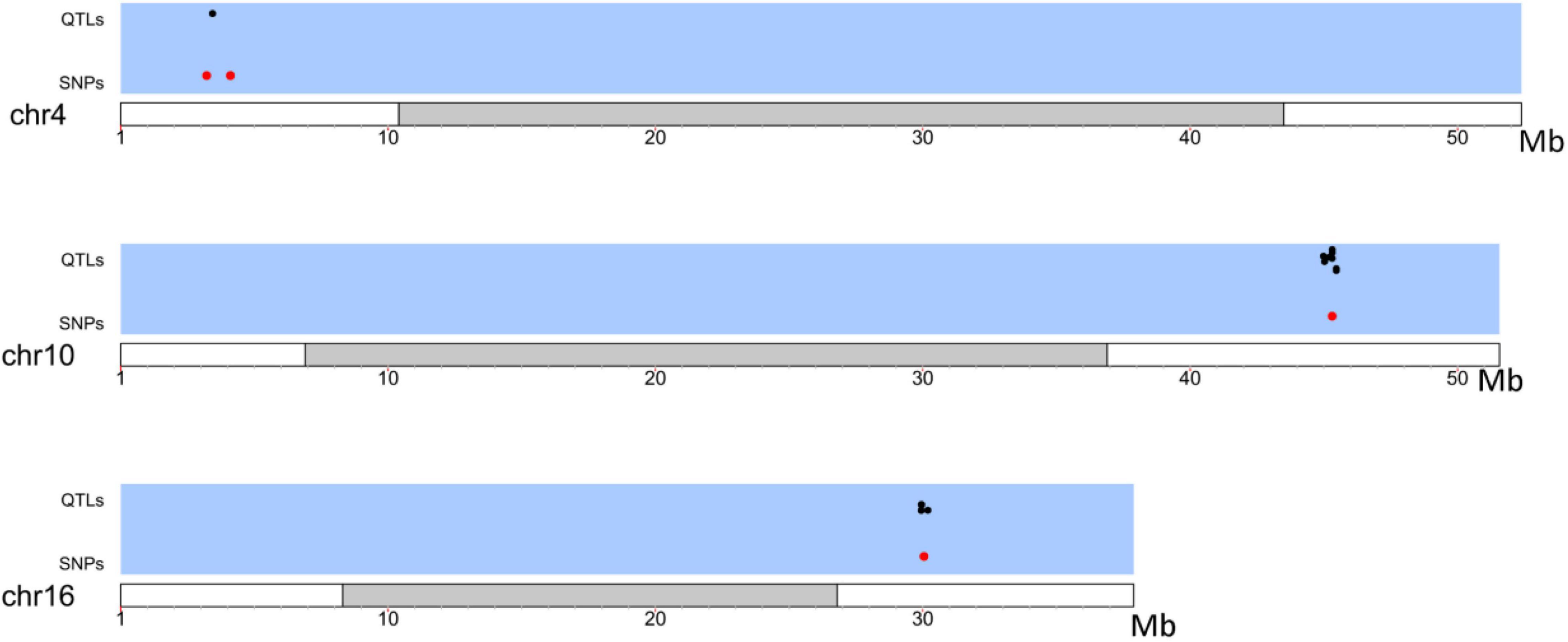
Figure 4. Distribution of QTLs (black) in LD with SNPs under selection (Red) between Brazilian and Asian Genotypes.
SNP 1.5 is located inside the E2 gene, a GIGANTEA (GI) homologue. E2 has a large impact on the number of days to flowering and maturity in soybean, with few functional differences to its homologue in Arabidopsis thaliana (Watanabe et al., 2011; Wang Y. et al., 2016). The GI gene mediates the interaction between the circadian oscillator and CONSTANS (CO) to promote flowering. There is evidence of the conservation of this mechanism in several plant species, including soybean, thus making E2 one of the main agents controlling flowering and maturity in this crop (Fowler et al., 1999; Suárez-López et al., 2001; Mizoguchi et al., 2005; Nogueira, 2011; Haider, 2014; Lin et al., 2020; Miranda et al., 2020).
Eighty-two QTLs under selection were identified among different Brazilian companies’ portfolios (Supplementary Table 5 and Figure 5). QTLs involved in the number of days to flowering and maturity; protein, oil, and lipid content; resistance to several pathogens; productivity components (seed weight, number of seeds per plant); and plant architecture (height, node number, stem shape, and determinacy) were identified within the genomic regions surrounding the SNPs under selection.
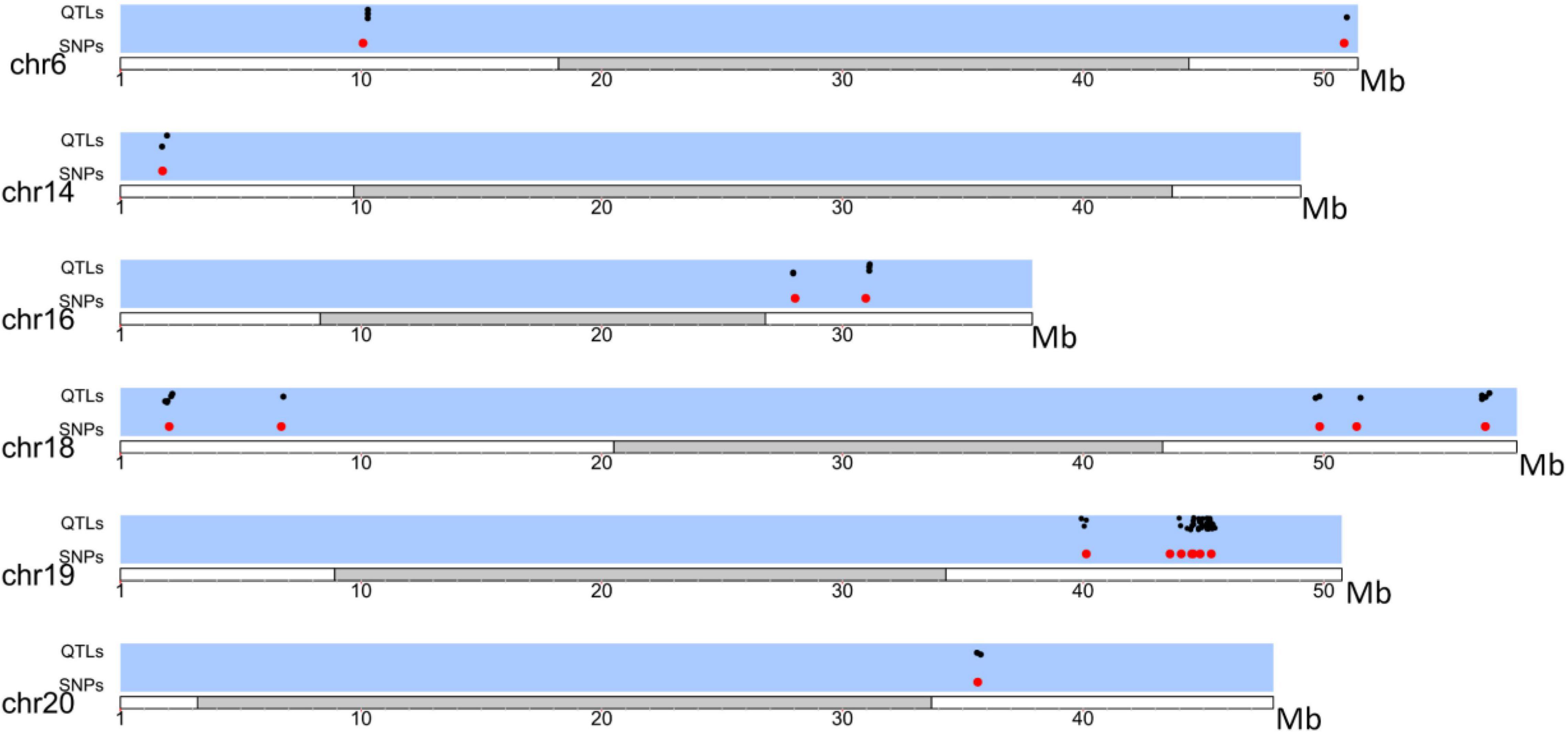
Figure 5. Distribution of QTLs (black) in LD with SNPs under selection (Red) among Brazilian companies.
Several genes known to play important roles in the control of these phenotypes can also be found in the same genomic regions. Three genes controlling cycle duration in soybean can be found in the regions under selection. The gene GmFT2a is 141,137 bp away from SNP 2.12. GmFT2a is one of the 12 FLOWERING LOCUS T (FT) genes found in soybean and is the causal gene for the E9 locus, which controls flowering in soybean (Kong et al., 2010, 2014; Li et al., 2014; Nan et al., 2014; Zhao et al., 2016; Wu et al., 2017; Cai et al., 2018; Lin et al., 2020). The FT gene is conserved in several plant species. In A. thaliana, it is the gene to which several signaling pathways converge to, integrating the photoperiod, temperature, vernalization, and light quality signaling pathways to regulate flowering (Amasino, 2010; Wickland and Hanzawa, 2015). In soybean, GmFT2a interacts with the FDL19 transcription factor to stimulate the expression of APETALA1 homologues, promoting flowering (Nan et al., 2014).
The SNP 2.19 under selection is located 197,494 bp from Glyma.19g13800, which is a homologue of AT2G41710, an endogenous gene in A. thaliana that belongs to the APETALA 2 (AP) family. This gene family is directly involved in the control of flowering and seed development in A. thaliana. Various authors have already demonstrated that the roles of this gene family are conserved in several species, including soybean (Jofuku et al., 1994; Yant et al., 2010; Lei et al., 2019; Jiang et al., 2020).
SNPs 2.22, 2.23, 2.24, and 2.25 are located inside regions associated with cycle duration and several plant architecture components such as growth habit, plant height, and node number. Previous works that detected such QTLs in this region point to Dt1 as a causal gene (Zhang et al., 2015; Contreras-Soto et al., 2017b; Mao et al., 2017). Dt1 is a TERMINAL FLOWER 1 (TFL-1) homologue; TFL1 regulates growth habit and flowering in A. thaliana (Ratcliffe et al., 1998; Liu et al., 2010; Hanano and Goto, 2011; Goretti et al., 2020). Although some authors indicate that Dt1 is involved in flowering regulation in soybean (Zhang et al., 2015; Mao et al., 2017), several studies have shown that this gene is sub-functionalized in soybean (Liu et al., 2010; Tian et al., 2010). However, a recent work from Yue et al. (2021) contradicts this notion by demonstrating that Dt1 can interact with GmFT5a to control the number of days to flowering in soybean.
In chromosome 14, SNP 2.7 is inside a gene that codes for a toll-like interleukin receptor. This gene has been considered a causal gene of the QTL associated with Diaporthe phaseolorum resistance (Chang et al., 2016). In chromosome 18, SNP 2.13 is in a region that contains a QTL associated with Fusarium virguliforme and Heterodera glycines resistance (Wen et al., 2014; Chang et al., 2016). This QTL is a gene complex that includes GmRLK18-1, a leucine-rich repeat receptor-like protein kinase involved in resistance to sudden death syndrome (F. virguliforme) and the soybean cyst nematode (H. glycines) (Srour et al., 2012; Wen et al., 2014).
SNP 2.15 is 53,313 bp away from Glyma.18g211100, which codes for a peroxidase. Peroxidases play an important role in pathogen resistance, taking part in lignin, suberin, phytoalexin, and reactive oxygen species synthesis. These substances participate in the hypersensitivity response that causes controlled cell death to limit an area of infection and pathogen development (Almagro et al., 2009).
A gene associated with the number of seeds per pod is in one of the genomic regions under selection surrounding SNP 2.27. Gm-JAG1 was identified by Fang et al. (2017) as the probable causal gene for Seed-set1-g53.1. GM-JAG1, positioned 206,523 bp from SNP 2.27, is a JAG homologue that in A. thaliana encodes a zinc finger-like protein that regulates organ growth and development (Ohno et al., 2004; Jeong et al., 2013; Sayama et al., 2017).
Discussion
Genotyping by Sequencing
Most of the polymorphic SNPs detected are concentrated in euchromatic regions. This is expected because the GBS technique is based on reducing genome complexity using methylation-sensitive restriction enzymes to focus sequencing to low-methylated regions, with higher gene concentration (Elshire et al., 2011; Sonah et al., 2013). Soybean is an autogamous plant, which explains the high level of homozygosity detected in the markers. This has been widely documented by other authors who have worked with soybean and other autogamous crops (Cockram et al., 2010; Mesquita, 2017; Sherman-Broyles et al., 2017; Li et al., 2020). The prevalence of SNPs classified as transitions corroborates values found in the literature (Van et al., 2005; Shu et al., 2011; dos Santos et al., 2016). Transversions more frequently change the translated amino acid sequence in comparison to transitions. This leads to transitions being under weaker purifying selection pressure, increasing their frequency in the genome relative to transversions (Guo et al., 2017).
Population Structure and Genetic Diversity
The population structure analysis indicated the existence of three subpopulations originating from different geographic regions. The Asian genotypes were the most distinct group compared to the rest of the panel, with higher π-values as well as smaller linkage blocks, indicating higher genetic diversity than the Brazilian genotypes. These results corroborate previous authors’ findings that Asian genotypes have higher genetic diversity than cultivars from other regions (Li et al., 2008; Liu et al., 2017; Bruce et al., 2019). This high diversity is mainly because this is the region of domestication and where the center of origin of the crop is located (Xu et al., 2002; Guo et al., 2010; Li et al., 2010; Han et al., 2016; Wang J. et al., 2016; Jeong et al., 2019).
Average FST values among germplasms from different Brazilian breeding programs were markedly smaller than those between Asian and Brazilian genotypes, indicating low genetic divergence among Brazilian genotypes. This low genetic diversity compared to Asian genotypes corroborates previous works that have assessed genetic diversity in the Brazilian soybean (Priolli et al., 2013; Wysmierski and Vello, 2013; Gwinner et al., 2017). Founding events are strong diversity bottlenecks, and soybean went through several of these before being introduced into Brazil in the 1960s (Hiromoto and Vello, 1986; Hyten et al., 2006; Banks et al., 2013).
The distance at which LD decays is in accordance with previous works in soybean (Wen et al., 2014; Mao et al., 2017; Wang et al., 2018), with higher values observed for Brazilian genotypes. This was expected, given that these accessions went through rigorous artificial selection processes and more diversity bottlenecks in comparison to the Asian genotypes. The presence of larger linkage blocks in populations developed by modern breeding programs has been described by several authors (Hyten et al., 2007; Song et al., 2015; Wang J. et al., 2016). Brazilian germplasms have a narrow genetic base due to the low number of ancestors contributing to a large part of the allelic diversity present in soybean cultivars (Hiromoto and Vello, 1986; Priolli et al., 2013; Wysmierski and Vello, 2013). This small effective population size leads to the formation of large linkage blocks, resulting in a longer LD decay distance (Song et al., 2015).
Factors such as RMG and company of origin also had a significant influence on population stratification when the Brazilian genotypes were analyzed separately. We observed the formation of distinct groups of early maturing and late maturing cultivars. The influence of RMG on population structure has already been described (Žulj Mihaljević et al., 2020). Contreras-Soto et al. (2017a) also observed a link between RMG and population stratification in a Brazilian soybean panel. These authors also demonstrated the importance of the company of origin on population structure. The formation of a subpopulation comprised of late maturing cultivars was also observed by Gwinner et al. (2017) using a panel of 77 Brazilian soybean genotypes.
The RMG of a cultivar defines the latitude the genotype is adapted to Alliprandini et al. (2009), Cavassim et al. (2013), and Zdziarski et al. (2018). Thus, panel stratification between cultivars adapted to southern growing environments and cultivars better adapted to the environmental conditions at lower latitudes is expected. The growing environments in different latitudes differ not only in photoperiod length, but also in soil pH, nutrient availability, average temperature, and precipitation (Kaster and Farias, 2012; Lopes and Guimarães Guilherme, 2016). Therefore, breeding is directed differently depending on the latitude for which the program aims to develop cultivars, which could explain the stratification observed here.
We also observed that cultivars developed by the same company showed higher genetic similarity among themselves. The effect of company of origin on population stratification has previously been demonstrated in tropical soybean cultivars (Contreras-Soto et al., 2017a). Cultivars developed by the same company frequently originate from the same breeding program, and therefore, a lower level of genetic dissimilarity is expected.
When analyzed by company, the population structure revealed interesting patterns. A significant portion of the portfolios from companies such as TMG, Bayer, Corteva, and Embrapa belong to the same subpopulation as PI559369 (Lee 68), indicating high genetic similarity. Lee 68 originated from the backcrossing of Lee × Arksoy into Lee. Lee, in turn, is a progeny from the cross between S-100 and C.N.S, the two main ancestors responsible for the genetic base of Brazilian soybeans (Wysmierski and Vello, 2013).
Genotypes from companies such as GDM, Nidera, and Syngenta are notably similar in genetic constitution to North American lines from crosses involving Williams. Although Williams isn’t among the main ancestors contributing to the Brazilian genetic base, it is possibly through Williams that relevant ancestors such as Dunfield, Mandarin, Manchu, Peking, Richland, and Mukden contribute the base (Wysmierski, 2010; Priolli et al., 2013). These cultivars also tend to present with a shorter cycle duration and adaptation to higher latitudes.
Selection Signatures in the Soybean Genome
A Bayesian approach was adopted through BayeScan software to detect genomic regions under selection between Brazilian and Asian genotypes, North American and Brazilian genotypes, and among Brazilian genotypes from different breeding programs. No SNPs under selection were detected between the North American and Brazilian genotypes. Seven SNPs under selection distributed in six genomic regions were detected in the Brazilian × Asian genotypes test. Genomic regions under selection between populations from different geographic origins are often associated with phenotypes controlling the species adaptability to different growing environments (Günther and Coop, 2013; Li et al., 2020; Saleem et al., 2021).
That six of the seven SNPs under selection were monomorphic in the Brazilian subpopulation suggests that these markers are associated with characteristics of relevance to the crop’s adaptability to Brazilian growing environments, although the possibility that random genetic drift is causing the fixation of these markers cannot be ignored. However, the SNPs under selection are not randomly distributed throughout the genome, but instead are located close to QTLs and genes specifically associated with phenotypes of great relevance to regional adaptation such as the number of days to flowering and maturity. This is strong evidence that the fixation in these loci wasn’t caused by random genetic drift (Hartl and Clark, 2007).
A larger set of SNPs under selection was detected among the Brazilian breeding programs, probably due to a higher number of groups being included in the analysis. Outlier SNPs are in LD with the QTLs associated with phenotypes of great relevance to breeders such as cycle duration, pathogen resistance, water use efficiency, and tolerance to nutrient deficiency. Brazil is a country where soybean growing environments span several latitudes, which demands from the crop the ability to adapt to environments with variable photoperiods, pathogen incidence, fertility, and precipitation. Studying the genomic regions under selection among breeding programs allows us to identify the QTLs relevant to regional adaptation (Jun et al., 2011).
It’s important to note that genomic regions of interest to breeders are constantly under severe selection pressure, leading to a reduction of diversity in those regions, which could culminate with their fixation. This reduction in diversity can be detrimental to the breeding programs’ ability to continually develop highly productive and adaptable cultivars (Hyten et al., 2006). There is a notable lack of studies that have looked at selection signatures in Brazilian soybean cultivars. Here, we identified 34 SNPs under selection which are in LD with 96 QTLs; many of these are of agronomic importance to soybean’s productivity and adaptation in Brazil. The genomic regions we identified can be explored by breeders aiming to increase the useful genetic diversity in Brazilian soybean germplasms and to develop cultivars able to adapt to the many Brazilian growing environments.
Data Availability Statement
The data used in the study has been uploaded to a repository and can be found here: https://doi.org/10.5281/zenodo.6362858.
Author Contributions
HM: conceptualization, data curation, formal analysis, investigation, methodology, software, visualization, writing—original draft, and writing—review and editing. LP: conceptualization, supervision, validation, and writing—review and editing. AM, GS, and JM: conceptualization, supervision, validation, writing—review and editing, resources, project administration, and funding acquisition. All authors contributed to the article and approved the submitted version.
Funding
This work was supported by the Tropical Melhoramento e Genética company and the Coordenação de Aperfeiçoamento de Pessoal de Nível Superior (CAPES—Brazil).
Conflict of Interest
The authors declare that the research was conducted in the absence of any commercial or financial relationships that could be construed as a potential conflict of interest.
Publisher’s Note
All claims expressed in this article are solely those of the authors and do not necessarily represent those of their affiliated organizations, or those of the publisher, the editors and the reviewers. Any product that may be evaluated in this article, or claim that may be made by its manufacturer, is not guaranteed or endorsed by the publisher.
Acknowledgments
We would like to thank the Tropical Melhoramento e Genética for support this research and the Coordenação de Aperfeiçoamento de Pessoal de Nível Superior—Brasil (CAPES) for the scholarship in Brazil.
Supplementary Material
The Supplementary Material for this article can be found online at: https://www.frontiersin.org/articles/10.3389/fpls.2022.842571/full#supplementary-material
Supplementary Figure 1 | Cluster analysis using the UPGMA method on genetic distances based on IBS among 247 Brazilian soybean cultivars, branches are colored by RMG whereas Cultivar labels are colored based on company of origin.
Footnotes
References
Alliprandini, L. F., Abatti, C., Bertagnolli, P. F., Cavassim, J. E., Gabe, H. L., Kurek, A., et al. (2009). Understanding soybean maturity groups in brazil: environment, cultivar classification, and stability. Crop Sci. 49, 801–808. doi: 10.2135/cropsci2008.07.0390
Almagro, L., Gómez Ros, L. V., Belchi-Navarro, S., Bru, R., Ros Barceló, A., and Pedreño, M. A. (2009). Class III peroxidases in plant defence reactions. J. Exp. Bot. 60, 377–390. doi: 10.1093/jxb/ern277
Amasino, R. (2010). Seasonal and developmental timing of flowering. Plant J. 61, 1001–1013. doi: 10.1111/j.1365-313X.2010.04148.x
Banks, S. C., Cary, G. J., Smith, A. L., Davies, I. D., Driscoll, D. A., Gill, A. M., et al. (2013). How does ecological disturbance influence genetic diversity? Trends Ecol. Evol. 28, 670–679. doi: 10.1016/j.tree.2013.08.005
Bradbury, P. J., Zhang, Z., Kroon, D. E., Casstevens, T. M., Ramdoss, Y., and Buckler, E. S. (2007). TASSEL: software for association mapping of complex traits in diverse samples. Bioinformatics 23, 2633–2635. doi: 10.1093/bioinformatics/btm308
Bruce, R. W., Torkamaneh, D., Grainger, C., Belzile, F., Eskandari, M., and Rajcan, I. (2019). Genome-wide genetic diversity is maintained through decades of soybean breeding in Canada. Theor. Appl. Genet. 132, 3089–3100. doi: 10.1007/s00122-019-03408-y
Cai, Y., Chen, L., Liu, X., Guo, C., Sun, S., Wu, C., et al. (2018). CRISPR/Cas9-mediated targeted mutagenesis of GmFT2a delays flowering time in soya bean. Plant Biotechnol. J. 16, 176–185. doi: 10.1111/pbi.12758
Carter, T. E., Nelson, R. L., Sneller, C. H., and Cui, Z. (2004). “Genetic diversity in soybean,” in Soybeans: Improvement, Production, and Uses, Vol. 16, eds R. M. Shibles, J. E. Harper, R. F. Wilson, and R. C. Shoemaker (Hoboken, NJ: John Wiley & Sons, Ltd), 304–416. doi: 10.2134/agronmonogr16.3ed.c8
Cavassim, J. E., Bespalhok Filho, J. C., Alliprandini, L. F., de Oliveira, R. A., Daros, E., and Guerra, E. P. (2013). Stability of soybean genotypes and their classification into relative maturity groups in Brazil. Am. J. Plant Sci. 4, 2060–2069. doi: 10.4236/ajps.2013.411258
Chang, H. X., Lipka, A. E., Domier, L. L., and Hartman, G. L. (2016). Characterization of disease resistance Loci in the USDA soybean germplasm collection using genome-wide association studies. Am. Phytopathol. Soc. 106, 1139–1151. doi: 10.1094/PHYTO-01-16-0042-FI
Cockram, J., White, J., Zuluaga, D. L., Smith, D., Comadran, J., MacAulay, M., et al. (2010). Genome-wide association mapping to candidate polymorphism resolution in the unsequenced barley genome. Proc. Natl. Acad. Sci. U.S.A. 107, 21611–21616. doi: 10.1073/pnas.1010179107
Contreras-Soto, R. I., de Oliveira, M. B., Costenaro-da-Silva, D., Scapim, C. A., and Schuster, I. (2017a). Population structure, genetic relatedness and linkage disequilibrium blocks in cultivars of tropical soybean (Glycine max). Euphytica 213:173. doi: 10.1007/s10681-017-1966-5
Contreras-Soto, R. I., Mora, F., Lazzari, F., de Oliveira, M. A. R., Scapim, C. A., and Schuster, I. (2017b). Genome-wide association mapping for flowering and maturity in tropical soybean: implications for breeding strategies. Breed. Sci. 67, 435–449. doi: 10.1270/jsbbs.17024
Danecek, P., Auton, A., Abecasis, G., Albers, C. A., Banks, E., DePristo, M. A., et al. (2011). The variant call format and VCFtools. Bioinformatics 27, 2156–2158. doi: 10.1093/bioinformatics/btr330
De Almeida, L. A., Afonso, R., Kiihl, D. S., Albino, M., De Miranda, C., Jesus, G., et al. (1999). “Melhoramento da soja para regiões de baixas latitudes.,” in Recursos Genéticos e Melhoramento de Plantas para o Nordeste Brasileiro, eds M. A. de Queiroz, C. O. Goedert, and S. R. R. Ramos (Brasília: Embrapa Recursos Geneticos e Biotecnologia).
de Almeida, R. D., Peluzio, J. M., Pires, L. P. M., Cancellier, L. L., Afférri, F. S., Colombo, G. A., et al. (2012). Divergencia genética entre cultivares de soja em várzea irrigada no estado do tocantins. Cienc. Rural 42, 395–400. doi: 10.1590/S0103-84782012000300002
dos Santos, J. V. M., Valliyodan, B., Joshi, T., Khan, S. M., Liu, Y., Wang, J., et al. (2016). Evaluation of genetic variation among Brazilian soybean cultivars through genome resequencing. BMC Genomics 17:110. doi: 10.1186/s12864-016-2431-x
Elshire, R. J., Glaubitz, J. C., Sun, Q., Poland, J. A., Kawamoto, K., Buckler, E. S., et al. (2011). A robust, simple genotyping-by-sequencing (GBS) approach for high diversity species. PLoS One 6:e19379. doi: 10.1371/journal.pone.0019379
Evanno, G., Regnaut, S., and Goudet, J. (2005). Detecting the number of clusters of individuals using the software structure: a simulation study. Mol. Ecol. 14, 2611–2620. doi: 10.1111/j.1365-294X.2005.02553.x
Fang, C., Ma, Y., Wu, S., Liu, Z., Wang, Z., Yang, R., et al. (2017). Genome-wide association studies dissect the genetic networks underlying agronomical traits in soybean. Genome Biol. 18:161. doi: 10.1186/s13059-017-1289-9
FAOSTAT (2019). Food and Agriculture Organization ofthe United Nations (FAO). FAOSTATDatabase. Rome: FAO.
Foll, M., and Gaggiotti, O. (2008). A genome-scan method to identify selected loci appropriate for both dominant and codominant markers: a Bayesian perspective. Genetics 180, 977–993. doi: 10.1534/genetics.108.092221
Fowler, S., Lee, K., Onouchi, H., Samach, A., Richardson, K., Morris, B., et al. (1999). GIGANTEA: a circadian clock-controlled gene that regulates photoperiodic flowering in Arabidopsis and encodes a protein with several possible membrane-spanning domains. EMBO J. 18, 4679–4688. doi: 10.1093/emboj/18.17.4679
Ge, H., You, G., Wang, L., Hao, C., Dong, Y., Li, Z., et al. (2012). Genome selection sweep and association analysis shed light on future breeding by design in wheat. Crop Sci. 52, 1218–1228. doi: 10.2135/cropsci2010.12.0680
Goretti, D., Silvestre, M., Collani, S., Langenecker, T., Méndez, C., Madueño, F., et al. (2020). TERMINAL FLOWER1 functions as a mobile transcriptional cofactor in the shoot apical meristem. Plant Physiol. 182, 2081–2095. doi: 10.1104/pp.19.00867
Grainger, C. M., Letarte, J., and Rajcan, I. (2018). Using soybean pedigrees to identify genomic selection signatures associated with long-term breeding for cultivar improvement. Can. J. Plant Sci. 98, 1176–1187. doi: 10.1139/cjps-2017-0339
Günther, T., and Coop, G. (2013). Robust identification of local adaptation from allele frequencies. Genetics 195, 205–220. doi: 10.1534/genetics.113.152462
Guo, C., McDowell, I. C., Nodzenski, M., Scholtens, D. M., Allen, A. S., Lowe, W. L., et al. (2017). Transversions have larger regulatory effects than transitions. BMC Genomics 18:394. doi: 10.1186/s12864-017-3785-4
Guo, J., Wang, Y., Song, C., Zhou, J., Qiu, L., Huang, H., et al. (2010). A single origin and moderate bottleneck during domestication of soybean (Glycine max): implications from microsatellites and nucleotide sequences. Ann. Bot. 106, 505–514. doi: 10.1093/aob/mcq125
Gwinner, R., Alemu Setotaw, T., Pasqual, M., Dos Santos, J. B., Zuffo, A. M., Zambiazzi, E. V., et al. (2017). Genetic diversity in Brazilian soybean germplasm. Crop Breed. Appl. Biotechnol. 17, 373–381. doi: 10.1590/1984-70332017v17n4a56
Haider, W. (2014). Exploring Flowering Gene Networks in Soybean And Arabidopsis Through Transcriptome Analysis. Doctoral dissertation. Chicago, IL: University of Illinois.
Han, Y., Zhao, X., Liu, D., Li, Y., Lightfoot, D. A., Yang, Z., et al. (2016). Domestication footprints anchor genomic regions of agronomic importance in soybeans. New Phytologist 209, 871–884. doi: 10.1111/nph.13626
Hanano, S., and Goto, K. (2011). Arabidopsis terminal flower1 is involved in the regulation of flowering time and inflorescence development through transcriptional repression. Plant Cell 23, 3172–3184. doi: 10.1105/tpc.111.088641
Hartl, D. L., and Clark, A. G. (2007). Principles of Population Genetics, 4th Edn. New York, NY: Oxford University Press.
Hiromoto, D. M., and Vello, N. A. (1986). The genetic base of Brazilian soybean Glycine Max (L.) Merril cultivars. Rev. Brasil. Genét. 9, 295–306.
Hyten, D. L., Choi, I. Y., Song, Q., Shoemaker, R. C., Nelson, R. L., Costa, J. M., et al. (2007). Highly variable patterns of linkage disequilibrium in multiple soybean populations. Genetics 175, 1937–1944. doi: 10.1534/genetics.106.069740
Hyten, D. L., Song, Q., Zhu, Y., Choi, I. Y., Nelson, R. L., Costa, J. M., et al. (2006). Impact of genetic bottlenecks on soybean genome diversity. Proc. Natl. Acad. Sci. U.S.A. 103, 16666–16671. doi: 10.1073/pnas.0604379103
Instituto Brasileiro de Geografia e Estatística [IBGE] (2021). Levantamento Sistemático da Produção Agrícola (LSPA). Rio de Janeiro: Instituto Brasileiro de Geografia e Estatística.
Jeong, N., Suh, S. J., Kim, M. H., Lee, S., Moon, J. K., Kim, H. S., et al. (2013). Ln is a key regulator of leaflet shape and number of seeds per pod in soybean. Plant Cell 24, 4807–4818. doi: 10.1105/tpc.112.104968
Jeong, S. C., Moon, J. K., Park, S. K., Kim, M. S., Lee, K., Lee, S. R., et al. (2019). Genetic diversity patterns and domestication origin of soybean. Theor. Appl. Genet. 132, 1179–1193. doi: 10.1007/s00122-018-3271-7
Jiang, W., Zhang, X., Song, X., Yang, J., and Pang, Y. (2020). Genome-wide identification and characterization of APETALA2/Ethylene-responsive element binding factor superfamily genes in soybean seed development. Front. Plant Sci. 11:566647. doi: 10.3389/fpls.2020.566647
Jofuku, K. D., den Boer, B. G. W., Van Montagu, M., and Okamuro, J. K. (1994). Control of Arabidopsis flower and seed development by the homeotic gene APETALA2. Plant Cell 6, 1211–1225. doi: 10.1105/tpc.6.9.1211
Jun, T. H., Van, K., Kim, M. Y., Kwak, M., and Lee, S. H. (2011). Uncovering signatures of selection in the soybean genome using SSR diversity near QTLs of agronomic importance. Genes Genom. 33, 391–397. doi: 10.1007/s13258-010-0159-6
Kaster, M., and Farias, J. R. (2012). Regionalização dos Testes de Valor de Cultivo e Uso e da Indicação de Cultivares de soja - terceira Aproximação: Comissão de Genética e Melhoramento. Rodovia Carlos João Strass: Embrapa Soja, 231–235.
Kong, F., Liu, B., Xia, Z., Sato, S., Kim, B. M., Watanabe, S., et al. (2010). Two coordinately regulated homologs of FLOWERING LOCUS T are involved in the control of photoperiodic flowering in soybean. Plant Physiol. 154, 1220–1231. doi: 10.1104/pp.110.160796
Kong, F., Nan, H., Cao, D., Li, Y., Wu, F., Wang, J., et al. (2014). A new dominant gene E9 conditions early flowering and maturity in soybean. Crop Sci. 54, 2529–2535. doi: 10.2135/cropsci2014.03.0228
Langmead, B., and Salzberg, S. L. (2012). Fast gapped-read alignment with Bowtie 2. Nat. Methods 9, 357–359. doi: 10.1038/nmeth.1923
Lei, M., Li, Z. Y., Wang, J. B., Fu, Y. L., and Xu, L. (2019). Ectopic expression of the Aechmea fasciata APETALA2 gene AfAP2-2 reduces seed size and delays flowering in Arabidopsis. Plant Physiol. Biochem. 139, 642–650. doi: 10.1016/j.plaphy.2019.03.034
Li, H. (2011). A statistical framework for SNP calling, mutation discovery, association mapping and population genetical parameter estimation from sequencing data. Bioinformatics 27, 2987–2993. doi: 10.1093/bioinformatics/btr509
Li, Y. H., Li, D., Jiao, Y. Q., Schnable, J. C., Li, Y. F., Li, H. H., et al. (2020). Identification of loci controlling adaptation in Chinese soya bean landraces via a combination of conventional and bioclimatic GWAS. Plant Biotechnol. J. 18, 389–401. doi: 10.1111/pbi.13206
Li, Y. H., Li, W., Zhang, C., Yang, L., Chang, R. Z., Gaut, B. S., et al. (2010). Genetic diversity in domesticated soybean (Glycine max) and its wild progenitor (Glycine soja) for simple sequence repeat and single-nucleotide polymorphism loci. New Phytol. 188, 242–253. doi: 10.1111/j.1469-8137.2010.03344.x
Li, Y. H., Zhou, G., Ma, J., Jiang, W., Jin, L. G., Zhang, Z., et al. (2014). De novo assembly of soybean wild relatives for pan-genome analysis of diversity and agronomic traits. Nat. Biotechnol. 32, 1045–1052. doi: 10.1038/nbt.2979
Li, Y., Guan, R., Liu, Z., Ma, Y., Wang, L., Li, L., et al. (2008). Genetic structure and diversity of cultivated soybean (Glycine max (L.) Merr.) landraces in China. Theor. Appl. Genet. 117, 857–871. doi: 10.1007/s00122-008-0825-0
Lin, X., Liu, B., Weller, J. L., Abe, J., and Kong, F. (2020). Molecular mechanisms for the photoperiodic regulation of flowering in soybean. J. Integr. Plant Biol. 63, 981–994. doi: 10.1111/jipb.13021
Liu, B., Watanabe, S., Uchiyama, T., Kong, F., Kanazawa, A., Xia, Z., et al. (2010). The soybean stem growth habit gene Dt1 is an ortholog of Arabidopsis TERMINAL FLOWER1. Plant Physiol. 153, 198–210. doi: 10.1104/pp.109.150607
Liu, W., Chen, L., Zhang, S., Hu, F., Wang, Z., Lyu, J., et al. (2019). Decrease of gene expression diversity during domestication of animals and plants. BMC Evol. Biol. 19:19. doi: 10.1186/s12862-018-1340-9
Liu, Z., Li, H., Wen, Z., Fan, X., Li, Y., Guan, R., et al. (2017). Comparison of genetic diversity between Chinese and american soybean (Glycine max (L.)) accessions revealed by high-density SNPs. Front. Plant Sci. 8:2014. doi: 10.3389/fpls.2017.02014
Lopes, A. S., and Guimarães Guilherme, L. R. (2016). “A career perspective on soil management in the Cerrado region of Brazil,” in Advances in Agronomy, Vol. 137, ed. D. L. Sparks (Amsterdam: Elsevier Inc), doi: 10.1016/bs.agron.2015.12.004
Mao, T., Li, J., Wen, Z., Wu, T., Wu, C., Sun, S., et al. (2017). Association mapping of loci controlling genetic and environmental interaction of soybean flowering time under various photo-thermal conditions. BMC Genomics 18:415. doi: 10.1186/s12864-017-3778-3
Maynard Smith, J., and Haigh, J. (1974). The hitch-hiking effect of a favourable gene. Genetics Res. 89, 391–403. doi: 10.1017/S0016672308009579
Mesquita, A. C. O. (2017). Mapeamento por Associação Genômica Ampla para Identificação de Resistência ao Mofo Branco em Soja. Master’s thesis. Uberlândia: Uberlândia Federal University.
Miranda, C., Scaboo, A., Cober, E., Denwar, N., and Bilyeu, K. (2020). The effects and interaction of soybean maturity gene alleles controlling flowering time, maturity, and adaptation in tropical environments. BMC Plant Biol. 20:65. doi: 10.1186/s12870-020-2276-y
Miranda, Z. D. F. S., Arias, C. A. A., Prete, C. E. C., Kiihl, R. A. D. S., De Almeida, L. A., De Toledo, J. F. F., et al. (2007). Genetic characterization of ninety elite soybean cultivars using coefficient of parentage. Pesqui. Agropecu. Bras. 42, 363–369. doi: 10.1590/S0100-204X2007000300009
Mizoguchi, T., Wright, L., Fujiwara, S., Cremer, F., Lee, K., Onouchi, H., et al. (2005). Distinct roles of GIGANTEA in promoting flowering and regulating circadian rhythms in Arabidopsis. Plant Cell 17, 2255–2270. doi: 10.1105/tpc.105.033464
Money, D., Gardner, K., Migicovsky, Z., Schwaninger, H., Zhong, G. Y., and Myles, S. (2015). LinkImpute: fast and accurate genotype imputation for nonmodel organisms. G3 5, 2383–2390. doi: 10.1534/g3.115.021667
Nan, H., Cao, D., Zhang, D., Li, Y., Lu, S., Tang, L., et al. (2014). GmFT2a and GmFT5a redundantly and differentially regulate flowering through interaction with and upregulation of the bZIP transcription factor GmFDL19 in soybean. PLoS One 9:e97669. doi: 10.1371/journal.pone.0097669
Nogueira, A. P. O. (2011). Correlações, Análise De Trilha E Diversidade Fenotípica E Molecular De Soja. Doctoral dissertation. Viçosa: Viçosa Federal University.
Ohno, C. K., Reddy, G. V., Heisler, M. G. B., and Meyerowitz, E. M. (2004). The Arabidopsis JAGGED gene encodes a zinc finger protein that promotes leaf tissue development. Development 131, 1111–1122. doi: 10.1242/dev.00991
Pina-Martins, F., Silva, D. N., Fino, J., and Paulo, O. S. (2017). Structure_threader: an improved method for automation parallelization of programs STRUCTURE, FASTSTRUCTURE and MavericK on Multi Core CPU systems. Mol. Ecol. 17, e268–e274. doi: 10.1111/1755-0998.12702
Priolli, R. H. G., Mendes-Junior, C. T., Sousa, S. M. B., Sousa, N. E. A., and Contel, E. P. B. (2004). Diversidade genética da soja entre períodos e entre programas de melhoramento no Brasil. Pesqui. Agropecu. Bras. 39, 967–975. doi: 10.1590/s0100-204x2004001000004
Priolli, R. H. G., Wysmierski, P. T., da Cunha, C. P., Pinheiro, J. B., and Vello, N. A. (2013). Genetic structure and a selected core set of Brazilian soybean cultivars. Genet. Mol. Biol. 36, 382–390. doi: 10.1590/S1415-47572013005000034
Pritchard, J. K., Stephens, M., and Donnelly, P. (2000). Inference of population structure using multilocus genotype data. Genetics 155, 945–959. doi: 10.1093/genetics/155.2.945
Purcell, S., Neale, B., Todd-Brown, K., Thomas, L., Ferreira, M. A. R., Bender, D., et al. (2007). PLINK: a tool set for whole-genome association and population-based linkage analyses. Am. J. Hum. Genet. 81, 559–575. doi: 10.1086/519795
Ratcliffe, O. J., Amaya, I., Vincent, C. A., Rothstein, S., Carpenter, R., Coen, E. S., et al. (1998). A common mechanism controls the life cycle and architecture of plants. Development 125, 1609–1615. doi: 10.1242/dev.125.9.1609
Rimmer, A., Phan, H., Mathieson, I., Iqbal, Z., Twigg, S. R. F., Wilkie, A. O. M., et al. (2014). Integrating mapping-, assembly- and haplotype-based approaches for calling variants in clinical sequencing applications. Nat. Genet. 46, 912–918. doi: 10.1038/ng.3036
Saleem, A., Muylle, H., Aper, J., Ruttink, T., Wang, J., Yu, D., et al. (2021). A genome-wide genetic diversity scan reveals multiple signatures of selection in a european soybean collection compared to chinese collections of wild and cultivated soybean accessions. Front. Plant Sci. 12:631767. doi: 10.3389/fpls.2021.631767
Sayama, T., Tanabata, T., Saruta, M., Yamada, T., Anai, T., Kaga, A., et al. (2017). Confirmation of the pleiotropic control of leaflet shape and number of seeds per pod by the Ln gene in induced soybean mutants. Breed. Sci. 67, 363–369. doi: 10.1270/jsbbs.16201
Sherman-Broyles, S., Bombarely, A., and Doyle, J. (2017). Characterizing the allopolyploid species among the wild relatives of soybean: utility of reduced representation genotyping methodologies. J. Syst. Evol. 55, 365–376. doi: 10.1111/jse.12268
Shu, Y., Li, Y., Zhu, Z., Bai, X., Cai, H., Ji, W., et al. (2011). SNPs discovery and CAPS marker conversion in soybean. Mol. Biol. Rep. 38, 1841–1846. doi: 10.1007/s11033-010-0300-2
Sonah, H., Bastien, M., Iquira, E., Tardivel, A., Légaré, G., Boyle, B., et al. (2013). An improved genotyping by sequencing (GBS) approach offering increased versatility and efficiency of SNP discovery and genotyping. PLoS One 8:e54603. doi: 10.1371/journal.pone.0054603
Song, Q., Hyten, D. L., Jia, G., Quigley, C. V., Fickus, E. W., Nelson, R. L., et al. (2015). Fingerprinting soybean germplasm and its utility in genomic research. G3 5, 1999–2006. doi: 10.1534/g3.115.019000
Srour, A., Afzal, A. J., Blahut-Beatty, L., Hemmati, N., Simmonds, D. H., Li, W., et al. (2012). The receptor like kinase at Rhg1-a/Rfs2 caused pleiotropic resistance to sudden death syndrome and soybean cyst nematode as a transgene by altering signaling responses. BMC Genomics 13:368. doi: 10.1186/1471-2164-13-368
Suárez-López, P., Wheatley, K., Robson, F., Onouchi, H., Valverde, F., and Coupland, G. (2001). CONSTANS mediates between the circadian clock and the control of flowering in Arabidopsis. Nature 410, 1116–1120. doi: 10.1038/35074138
Tanksley, S. D., and McCouch, S. R. (1997). Seed banks and molecular maps: unlocking genetic potential from the wild. Science 277, 1063–1066. doi: 10.1126/science.277.5329.1063
Tian, Z., Wang, X., Lee, R., Li, Y., Specht, J. E., Nelson, R. L., et al. (2010). Artificial selection for determinate growth habit in soybean. Proc. Natl. Acad. Sci. U.S.A. 107, 8563–8568. doi: 10.1073/pnas.1000088107
Van, K., Hwang, E. Y., Kim, M. Y., Park, H. J., Lee, S. H., and Cregan, P. B. (2005). Discovery of SNPs in soybean genotypes frequently used as the parents of mapping populations in the United States and Korea. J. Hered. 96, 529–535. doi: 10.1093/jhered/esi069
Vieira, E. A., de Carvalho, F. I. F., de Oliveira, A. C., Benin, G., Zimmer, P. D., da Silva, J. A. G., et al. (2005). Comparação entre medidas de distância genealógica, morfológica e molecular em aveia em experimentos com e sem a aplicação de fungicida. Bragantia 64, 51–60. doi: 10.1590/s0006-87052005000100006
Vigouroux, Y., McMullen, M., Hittinger, C. T., Houchins, K., Schulz, L., Kresovich, S., et al. (2002). Identifying genes of agronomic importance in maize by screening microsatellites for evidence of selection during domestication. Proc. Natl. Acad. Sci. U.S.A. 99, 9650–9655. doi: 10.1073/pnas.112324299
Wang, J., Chu, S., Zhang, H., Zhu, Y., Cheng, H., and Yu, D. (2016). Development and application of a novel genome-wide SNP array reveals domestication history in soybean. Sci. Rep. 6:20728. doi: 10.1038/srep20728
Wang, Y. Y., Li, Y., Wu, H., Hu, B., Zheng, J., Zhai, H., et al. (2018). Genotyping of soybean cultivars with medium-density array reveals the population structure and QTNs underlying maturity and seed traits. Front. Plant Sci. 9:610. doi: 10.3389/fpls.2018.00610
Wang, Y., Gu, Y., Gao, H., Qiu, L., Chang, R., Chen, S., et al. (2016). Molecular and geographic evolutionary support for the essential role of GIGANTEAa in soybean domestication of flowering time. BMC Evol. Biol. 16:79. doi: 10.1186/s12862-016-0653-9
Watanabe, S., Xia, Z., Hideshima, R., Tsubokura, Y., Sato, S., Yamanaka, N., et al. (2011). A map-based cloning strategy employing a residual heterozygous line reveals that the GIGANTEA gene is involved in soybean maturity and flowering. Genetics 188, 395–407. doi: 10.1534/genetics.110.125062
Wen, Z., Tan, R., Yuan, J., Bales, C., Du, W., Zhang, S., et al. (2014). Genome-wide association mapping of quantitative resistance to sudden death syndrome in soybean. BMC Genomics 15:809. doi: 10.1186/1471-2164-15-809
Wickland, D. P., and Hanzawa, Y. (2015). The FLOWERING LOCUS T/TERMINAL FLOWER 1 gene family: functional evolution and molecular mechanisms. Mol. Plant 8, 983–997. doi: 10.1016/j.molp.2015.01.007
Wu, F., Sedivy, E. J., Price, W. B., Haider, W., and Hanzawa, Y. (2017). Evolutionary trajectories of duplicated FT homologues and their roles in soybean domestication. Plant J. 90, 941–953. doi: 10.1111/tpj.13521
Wysmierski, P. T. (2010). Contribuição Genética dos Ancestrais da soja às Cultivares Brasileiras. Master’s thesis. Piracicaba: ESALQ.
Wysmierski, P. T., and Vello, N. A. (2013). The genetic base of Brazilian soybean cultivars: evolution over time and breeding implications. Genet. Mol. Biol. 36, 547–555. doi: 10.1590/S1415-47572013005000041
Xu, D. H., Abe, J., Gai, J. Y., and Shimamoto, Y. (2002). Diversity of chloroplast DNA SSRs in wild and cultivated soybeans: evidence for multiple origins of cultivated soybean. Theor. Appl. Genet. 105, 645–653. doi: 10.1007/s00122-002-0972-7
Yant, L., Mathieu, J., Dinh, T. T., Ott, F., Lanz, C., Wollmann, H., et al. (2010). Orchestration of the floral transition and floral development in arabidopsis by the bifunctional transcription factor APETALA2. Plant Cell 22, 2156–2170. doi: 10.1105/tpc.110.075606
Yue, L., Li, X., Fang, C., Chen, L., Yang, H., Yang, J., et al. (2021). FT5a interferes with the Dt1-AP1 feedback loop to control flowering time and shoot determinacy in soybean. J. Integr. Plant Biol. 63, 1004–1020. doi: 10.1111/jipb.13070
Zdziarski, A. D., Todeschini, M. H., Milioli, A. S., Woyann, L. G., Madureira, A., Stoco, M. G., et al. (2018). Key soybean maturity groups to increase grain yield in Brazil. Crop Sci. 58, 1155–1165. doi: 10.2135/cropsci2017.09.0581
Zhang, J., Song, Q., Cregan, P. B., Nelson, R. L., Wang, X., Wu, J., et al. (2015). Genome-wide association study for flowering time, maturity dates and plant height in early maturing soybean (Glycine max) germplasm. BMC Genomics 16:217. doi: 10.1186/s12864-015-1441-4
Zhang, W., Xu, W., Zhang, H., Liu, X., Cui, X., Li, S., et al. (2021). Comparative selective signature analysis and high-resolution GWAS reveal a new candidate gene controlling seed weight in soybean. Theor. Appl. Genet. 134, 1329–1341. doi: 10.1007/s00122-021-03774-6
Zhao, C., Takeshima, R., Zhu, J., Xu, M., Sato, M., Watanabe, S., et al. (2016). A recessive allele for delayed flowering at the soybean maturity locus E9 is a leaky allele of FT2a, a FLOWERING LOCUS T ortholog. BMC Plant Biol. 16:20. doi: 10.1186/s12870-016-0704-9
Keywords: Brazilian Soybean, adaptation, selection signatures in the genome, population structure, genotyping by sequencing
Citation: Mendonça HC, Pereira LFP, Maldonado dos Santos JV, Meda AR and Sant’ Ana GC (2022) Genetic Diversity and Selection Footprints in the Genome of Brazilian Soybean Cultivars. Front. Plant Sci. 13:842571. doi: 10.3389/fpls.2022.842571
Received: 23 December 2021; Accepted: 14 February 2022;
Published: 30 March 2022.
Edited by:
Viktor Korzun, KWS SAAT SE & Co. KGaA, GermanyReviewed by:
Tomohiro Ban, Yokohama City University, JapanSanu Arora, John Innes Centre, United Kingdom
Copyright © 2022 Mendonça, Pereira, Maldonado dos Santos, Meda and Sant’ Ana. This is an open-access article distributed under the terms of the Creative Commons Attribution License (CC BY). The use, distribution or reproduction in other forums is permitted, provided the original author(s) and the copyright owner(s) are credited and that the original publication in this journal is cited, in accordance with accepted academic practice. No use, distribution or reproduction is permitted which does not comply with these terms.
*Correspondence: Gustavo César Sant’ Ana, Z3VzdGF2b3NhbnRhbmFAdG1nLmFnci5icg==