Corrigendum: Landscape Genomics in Tree Conservation Under a Changing Environment
- 1School of Pharmacy, Xi’an Jiaotong University, Xi’an, China
- 2School of Ecology and Nature Conservation, Beijing Forestry University, Beijing, China
Understanding the genetic basis of how species respond to changing environments is essential to the conservation of species. However, the molecular mechanisms of adaptation remain largely unknown for long-lived tree species which always have large population sizes, long generation time, and extensive gene flow. Recent advances in landscape genomics can reveal the signals of adaptive selection linking genetic variations and landscape characteristics and therefore have created novel insights into tree conservation strategies. In this review article, we first summarized the methods of landscape genomics used in tree conservation and elucidated the advantages and disadvantages of these methods. We then highlighted the newly developed method “Risk of Non-adaptedness,” which can predict the genetic offset or genomic vulnerability of species via allele frequency change under multiple scenarios of climate change. Finally, we provided prospects concerning how our introduced approaches of landscape genomics can assist policymaking and improve the existing conservation strategies for tree species under the ongoing global changes.
Introduction
Forest trees cover ca. 30% of the terrestrial surface of the earth from boreal to tropical latitudes and contain approximately three-quarters of the terrestrial biomass of the earth, which tightly links them with the global carbon cycle (Holliday et al., 2017; Isabel et al., 2020). They generally have higher levels of genetic diversity and experience rapid microevolution, which often show distinguishable adaptation to local environments (Hamrick et al., 1992; Petit and Hampe, 2006; Neale and Kremer, 2011). In addition, quantitative traits with high heritability make trees exhibit stronger signals of local adaptation (clinal variation); however, a large genome, long generation time makes it not suitable for quantitative trait loci (QTL) and related analysis even though great progress have been achieved on quantitative genetics study on trees (Savolainen et al., 2013; Milesi et al., 2019 and references therein). Therefore, understanding the genetic basis of adaptation to the environment via landscape genomics studies is essential for management interventions of tree species related to conservation and reforestation under climate change (Allendorf et al., 2010; Savolainen et al., 2013; Anderson and Song, 2020).
Empirical studies had already suggested that adaptation in tree species primarily arises from standing genetic variations, facilitating more rapid adaptation to climate change than that via new mutations (Barrett and Schluter, 2008; Alberto et al., 2013; Savolainen et al., 2013). However, rapid climate change can break this association and create a mismatch between population climatic optima and current climate (Jump and Peñuelas, 2005; Aitken et al., 2008). Additional challenges such as gene flow, eco-evolutionary dynamics on the species range margins, and variation in climate changes across the landscape may also impact the adaptation of species (Savolainen et al., 2007; Alberto et al., 2013; Aitken and Bemmels, 2016). Still, in practice, very few conservation strategies consider genetic resources, especially for forest species (Lefèvre et al., 2013), with some applications in forest restoration program, e.g., it is recommended that Quercus mongolica seeds should not be transferred from their provenances because the genetic cline was determined between the northeastern and southwestern Japan by neutral genetic markers (Nagamitsu and Shuri, 2021).
Great progress had been achieved on the topic of the adaptive potential of natural populations (Hoffmann et al., 2017; Gaitán-Espitia and Hobday, 2021). In recent years, integrated interdisciplinary methods such as landscape genetics or genomics which are used to disentangle the impacts of environmental conditions on forest trees might provide guides for forest conservations (Rellstab et al., 2015; Isabel et al., 2020). The classical method for detecting the genetic basis of adaptation relies on population genetics (Wright and Gaut, 2004). This method attempts to find out outlier single-nucleotide polymorphisms (SNPs) by the comparisons of the genetic differentiation (FST) between populations and to hypothesize that these outliers are most likely to be affected by natural selection (Excoffier et al., 2009; Hohenlohe et al., 2010). However, this method suffers a high ratio of false-positive results due to the ignorance of environmental heterogeneity (Eveno et al., 2008; Hoban et al., 2016). Another approach is landscape genomics, which uncovers the molecular mechanism of adaptation on the basis of the genotype-environment associations (GEAs) by integrating genetic variation and spatial models (Holderegger et al., 2006; Sork et al., 2013; Sork, 2017; De Lafontaine et al., 2018). Recently, the evaluation of the genomic vulnerability (Bay et al., 2018), genetic offset (Fitzpatrick and Keller, 2015), or risk of non-adaptedness (RONA) (Rellstab et al., 2016) was used to predict the climate-driven population shifts. At present, a growing number of studies focus on tree species already utilized the population-level genomic data to evaluate the genomic vulnerability of the species in a changing climate.
The next-generation sequencing makes landscape genomic studies currently possible for detecting adaptive signals and uncovering the genomic basis of adaptation in many organisms. Although landscape genomics has been pursued for a decade and the advances of theoretical frameworks and applications are promising (Schoville et al., 2012; Joost et al., 2013; Bragg et al., 2015; Ćalić et al., 2015; Rellstab et al., 2015; Capblancq et al., 2020), molecular ecologists and evolutionists are currently awash with data, and the analytical methods in landscape genomics have lagged behind.
As an emerging approach used for the conservation genetics of trees, it is essential to understand its advanced trend. However, the existing approaches belong to landscape genomics for detecting adaptive signatures and predicting genetic offset of adaptive allelic frequencies under multiple climates have different assumptions, advantages, and limitations. An effective integrative framework shortage and how to utilize the results from these variable methods to improve management interventions of forest trees are big challenges for landscape genomics studies in the genomic era. Therefore, we first surveyed recent literature on the landscape genomics approach used for tree conservation study. We checked the Original Journal articles in the Molecular Ecology, Evolutionary Applications, Global Change Biology, New Phytologist, Ecology Letters, and Nature Climate Change from 2015 to 2021 (Table 1). Publications were selected based on four criteria: (i) the research was performed on forest tree species; (ii) an SNP dataset was used; (iii) adaptive SNPs were detected, and (iv) articles must predict the optimal composition to the future climate to evaluate genetic offset. Second, we summarized and depicted the advantages and disadvantages of utilizing related methods and genomic tools involved in detecting GEAs (Table 2) to quantify and/or map the disruption in local adaptation of forest trees under climate change. Then, we established a general framework (Figure 1) integrated methods of landscape genomics and population genomics for local adaptation analysis in forest trees. Finally, we provided suggestions on how these approaches can be used in making conservation strategies for tree species under climate change.
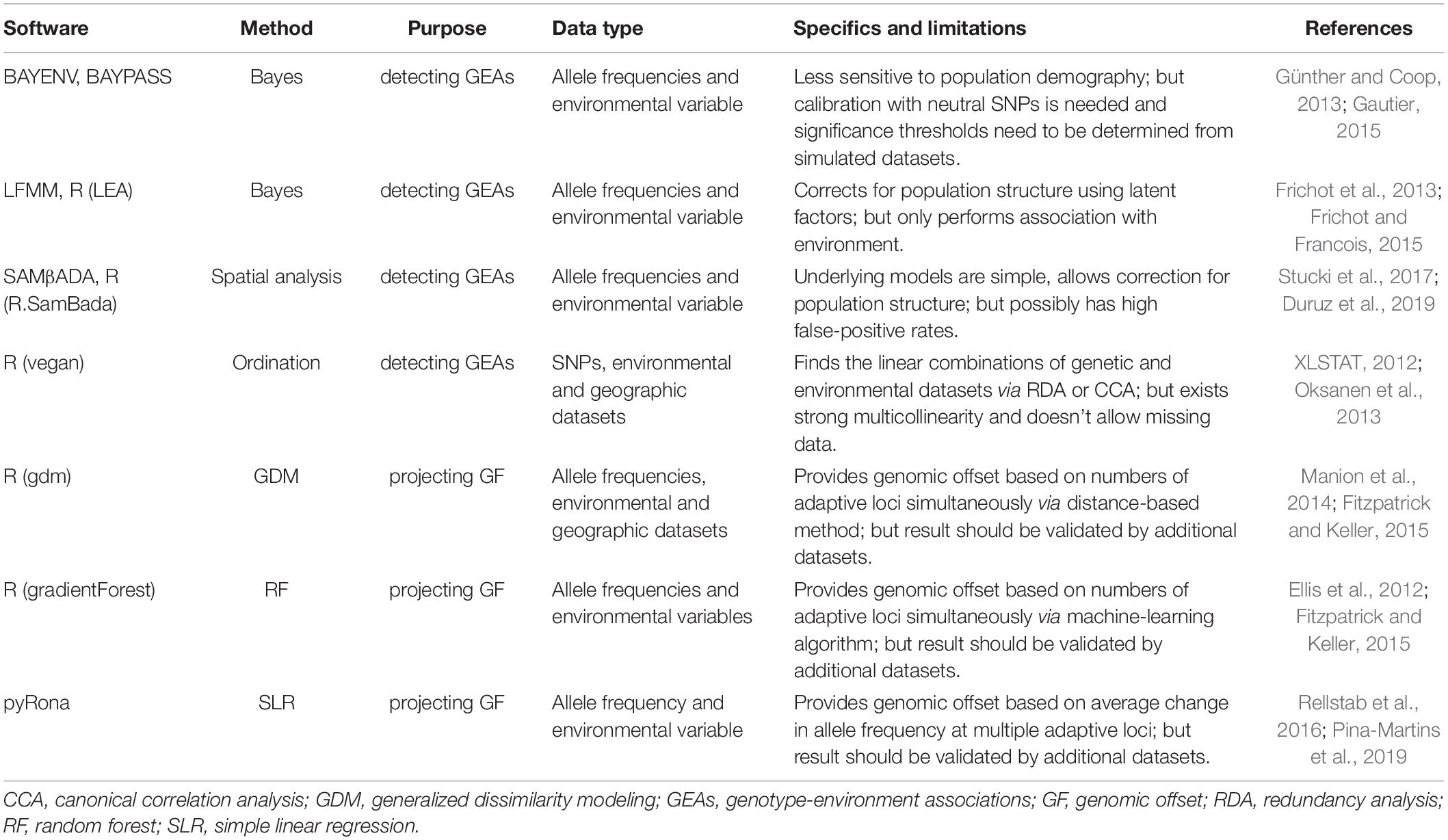
Table 2. Overview of methods and software available for environmental associations and genomic offset analyses in landscape genomics.
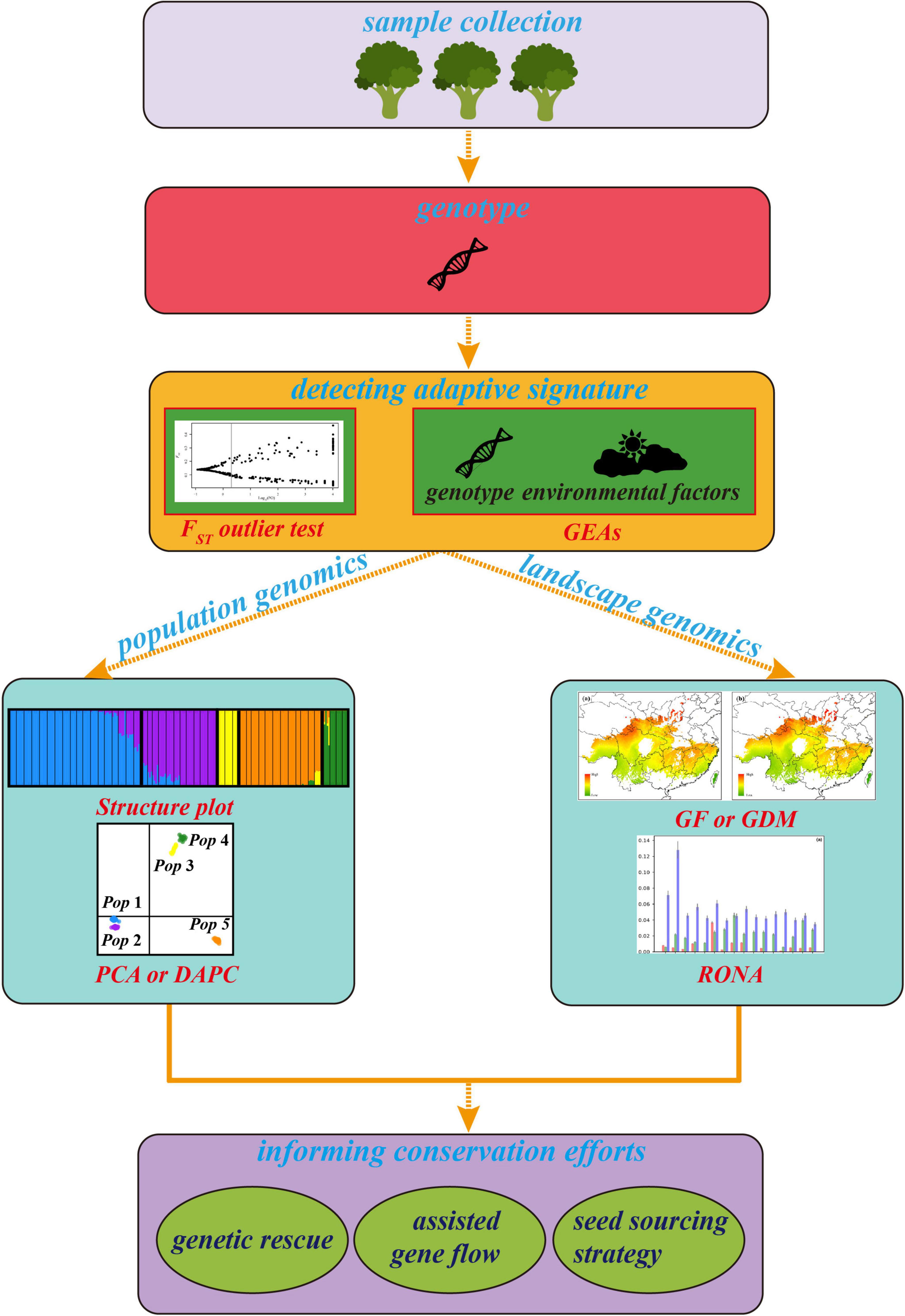
Figure 1. The general framework of landscape genomics for tree conservation. The plots of cluster, FST outlier test and RONA are modified from Du et al. (2020) and Feng et al. (2020), respectively.
Existing Approaches of Landscape Genomics
Mixed-Effects Models
Mixed-effects models provide a unified analytical framework to indicate robust and powerful evidence for adaptation (Rellstab et al., 2015). The advantage of the mixed-effects model is that it can reduce false-positive results by considering the influence of pairwise genetic distances and population structure. In mixed models, the genetic structure is incorporated as a random factor, allele frequencies are defined as response variables, and environmental factors are used as fixed factors. In this “Mixed-effects models” section, we illuminated the principles and methodologies using mixed models to detect signals of local adaptation based on BAYENV (Coop et al., 2010), Bayesian population association analysis (BayPass) (Gautier, 2015; Olazcuaga et al., 2020), latent factor mixed models (LFMMs) (Frichot et al., 2013), and spatial analysis method (SAM) (Joost et al., 2007, 2008).
The BAYENV is a method under the Bayesian framework employed to evaluate correlations between loci and environmental variables, and it can incorporate the uncertainty of allele frequencies from uneven sample sizes (Coop et al., 2010). The advantage of this program is that it applies a covariance matrix to take account for population structure, which is similar to an FST or kinship matrix. BAYENV requires a null model based on neutral loci and then determines the covariance matrix of estimated allele frequencies across populations. The significance test of each locus-variable combination utilizes Bayes factors calculated automatically by the program. However, cautions should be noticed here that these factors may not be directly compared across environmental variables due to variable-specific value ranges. In 2013, Günther and Coop developed an updated program called BAYENV2, which added non-parametric tests in the options and could be robustly applied for the Pool-Seq data (Günther and Coop, 2013).
BayPass (Gautier, 2015) is an extension of the Bayesian outlier detection model implemented in BAYENV to execute GEAs or environmental association analysis (EAA). It takes demographic effects into account by the estimation of the covariance matrix of allele frequency between populations. The core model (i.e., multivariate generalization model) in the BayPass reports locus XtX that is analogous to FST but explicitly corrected for this covariance matrix, accounting for the neutral correlations of allelic frequencies. Simulation studies suggested that the BayPass provided a robust framework to detect adaptive SNP signals (Gautier, 2015). However, a recent study revealed that the assumed linear relationships between allele frequencies utilized in EAA in line with the algorithm proposed by Gautier (2015) are unsatisfactory and even problematic when dealing with small datasets (Olazcuaga et al., 2020). Hence, they proposed a new approach that does not necessitate considering the uncertainty of the allele frequency estimation but assumes the exchangeability of SNPs both across the populations and along the genome. It is effective for gaining well-behaved P-values, avoiding intensively computational calibration, and providing reasonable numbers of SNPs analyzed (Olazcuaga et al., 2020). The advantage of BayPass is that it improves test performances by the estimations of the covariance matrix Ω (Olazcuaga et al., 2020).
The LFMMs rely on the Markov Chain Monte Carlo algorithms and integrate fixed effects to model environmental variables (Frichot et al., 2013). This algorithm is an extension of principal component analysis (PCA). LFMMs incorporate fixed effects to model environmental variables, and natural genetic structure is introduced as a random factor (i.e., latent factor). The computational speed is fast, and in addition, this approach does not need any a priori knowledge, making it attractive for determining adaptive signals with genomic data (Frichot et al., 2013; Rellstab et al., 2015). LFMMs can be implemented by the software LFMM (Frichot et al., 2013; Caye et al., 2019) or the R package LEA (Frichot and Francois, 2015).
The SAM is developed to assess putative associations between molecular markers and environmental variables using multiple univariate logistic regressions (Joost et al., 2007). It detects signatures of selection based on an integrative application of geographical information systems (GIS), environmental variables, and molecular data (Joost et al., 2007, 2008) implemented in MATLAB. The significance is determined by the likelihood ratio and Wald tests. Simulation studies implied that SAM might provide false-positive results if tested species endure complicated demography (De Mita et al., 2013; Frichot et al., 2013). Recently, an improved version of SAM called SAMβADA was developed (Stucki et al., 2017). This new approach allows for rapidly analyzing large genomics datasets by parallel processing. Compared with the early analysis method (i.e., SAM), the advantages of this new algorithm include that it (i) incorporates multivariate analyses to assess the impacts of many environmental predictor variables, (ii) allows to split the datasets and merges the results via parallel processing of SAMβADA, and (iii) enables the inclusion of explanatory variables representing population structure into the models to decrease false-positive results. However, pre- and post-processing of data will be labor-intensive when using the SAMβADA. In view of these facts, Duruz et al. (2019) published the R. SamBada landscape genomics pipeline to ease the identification and interpretation of candidate genes underlying local adaptation.
Multivariate Statistical Analysis
The multivariate statistical analysis usually integrates environmental variables and spatial genetic structure into the analytical framework to detect the adaptive variation. Traditionally, isolation by environment (IBE) is commonly used to detect selection signatures (Wright and Gaut, 2004; Wang and Bradburd, 2014; Manthey and Moyle, 2015). However, this Mantel-based method had poor performance in detecting true-positive results (Harmon and Glor, 2010; Hardy and Pavoine, 2012; Legendre et al., 2015), and its estimation bias might be amplified in the genomic era. Instead, the multivariate statistical analysis such as canonical correlation analysis (CCA) (Ter Braak, 1986) and redundancy analysis (RDA) (Van Den Wollenberg, 1977; Legendre and Legendre, 2012) may be more realistic for detecting selection signatures than univariate methods (Forester et al., 2016, 2018), because the selection is always a polygenic process driven by multiple environmental factors.
The CCA aimed to find a linear relationship between multiple loci and environmental factors. The loadings consist of loci and environmental variables indicate which loci respond to which environmental factors. However, we need caution to infer the outcomes if strong patterns of multicollinearity exist within datasets (Rellstab et al., 2015; Fenderson et al., 2020). RDA is another ordination approach that is effective to detect adaptive variation based on allele frequency data (Legendre and Legendre, 2012; Capblancq et al., 2018). First, RDA produces a matrix of fitted values based on the multivariate linear regression between genetic and environmental data, and then, the PCA of the fitted values produces canonical axes that are linear combinations of the original explanatory variables (Forester et al., 2016). In addition, partial RDA (pRDA) that stems from RDA also allows for constructing and testing complicated models to avoid the impacts of neutral genetic structure or spatial effects on detecting loci underlying adaptive variation (Legendre and Legendre, 2012). Another analogous approach, called the distance-based redundancy analysis (dbRDA), which differs in associations between genetic data and principal coordinate analysis and the procedure of emerging response variables compared with the RDA, can also enable to detect the adaptive evolution (Legendre and Anderson, 1999). However, when using the abovementioned methods, an important caveat exists is that the explanatory variables within these methods are uncorrelated and the number of loci examined might be at least three times as large as the number of putative explanatory variables (Jombart et al., 2009).
Simulation and empirical studies suggested that the RDA-based method could detect lower false-positive and higher true-positive rates when compared with generalized linear models (GLM) or LFMM (Forester et al., 2018). Even the powers to identify adaptive loci associated with environmental variables are similar via RDA and LFMM, the former has the advantage to identify the main selective gradients as a combination of environmental variables (Capblancq et al., 2018). Additionally, the constrained ordination methods have robust performance and enable to avoid spurious GEAs when the tested species has isolation-by-distance (IBD) pattern or low dispersal capability (Forester et al., 2016, 2018; Capblancq et al., 2018).
Predicting Genomic Vulnerability Under Alternative Climate Scenarios
Traditionally, the vulnerability of species mainly relied on the prediction of species distribution models (SDMs) (Elith and Leathwick, 2009) or its extensions, such as climate-niche factor analysis (CNFA) (Rinnan and Lawler, 2019). However, the abovementioned methods are unable to account for the continuous, multidimensional nature of genomic variation (Fitzpatrick and Keller, 2015). The expanding omics and statistical tools enable us to generate robust predictions of plant adaptive potential under climate change. In this study, we introduced three new methods used for predicting genomic vulnerability under alternative climate scenarios based on the linear or non-linear functions: generalized dissimilarity modeling (GDM) (Ferrier et al., 2007), gradient forests (GFs) (Ellis et al., 2012), and RONA (Rellstab et al., 2016).
Predicting Genomic Vulnerability Using Non-linear Regressions
The GDM is used for estimating and predicting the spatial pattern of turnover in community composition (Ferrier et al., 2002, 2007). Fitzpatrick and Keller (2015) extended the application of GDM to forecast the genetic offset of Populus balsamifera, and they concluded that the changes of genetic composition are required if it tries to mitigate maladaptation and maintain genetic diversity in the future. GDM accounts for spatial patterns in genetic data caused by demographic processes, accommodates varied factors (e.g., geographic or ecological separation, barriers to dispersal) as predictors, and also enables to deal with numerous SNP loci (Fitzpatrick and Keller, 2015). The functions can be implemented via the R package gdm (Manion et al., 2014). A recent study evaluated the local, forward, and reverse genetic offsets (Figure 2) of balsam poplar using the GDM and incorporated migration and dispersal into predictive genomic models to show the adaptive potential of balsam poplar in future climates (Gougherty et al., 2021). This study provides a new way to assess population-level risk at alternative climate scenarios that accounts for local adaptation and breaks through the prediction limitations at the species level.
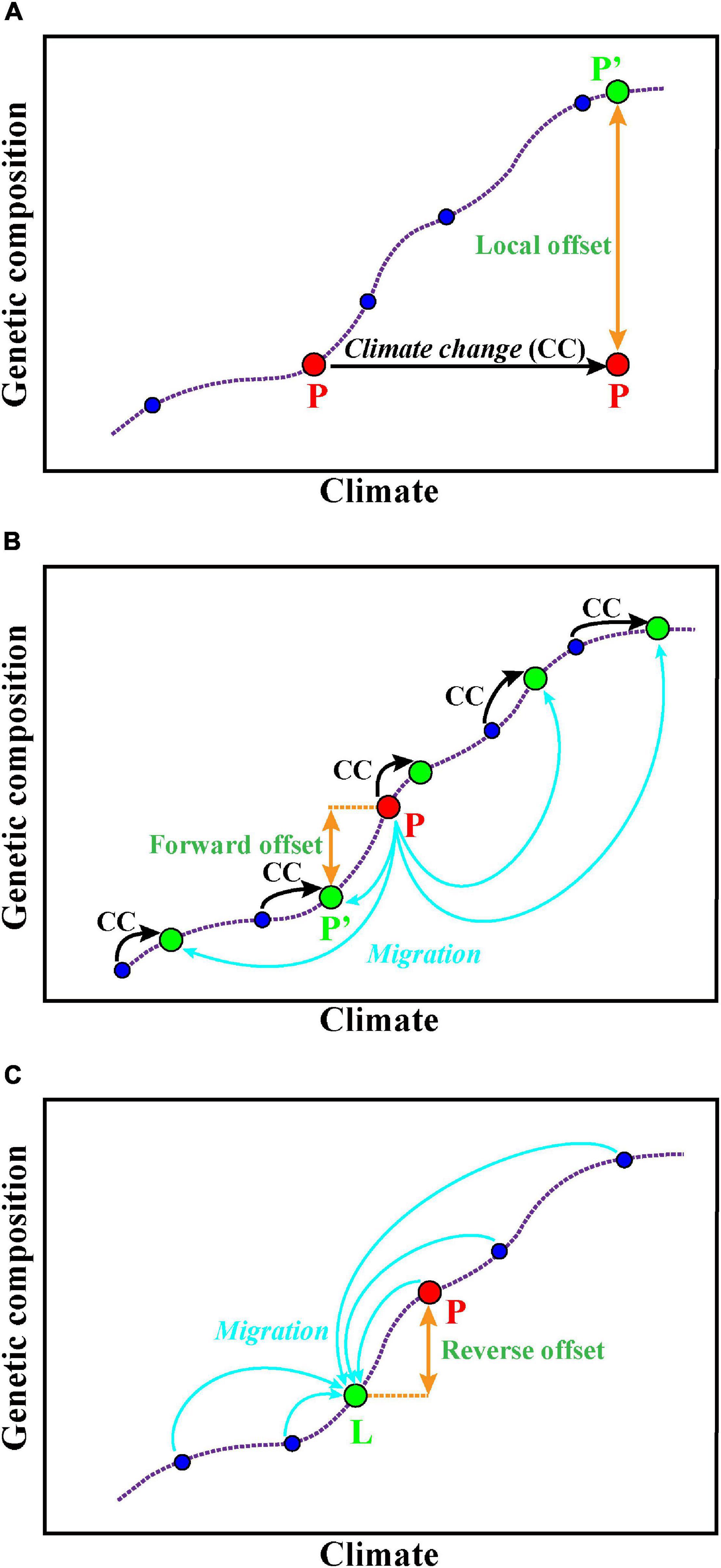
Figure 2. Predictions of potential adaptation to alternative climate scenarios. (A) Local offset means the specific population P (red color) to the theoretically required changes of allele frequency under a future climate in situ [P′ (green)]. (B) Forward genetic offset means that a specific contemporary population P (red color) can migrate (blue arrows) to the habitat whose future climate best matches its genetic composition [P′ (green)]. (C) Reverse genetic offset means for a specific location L (green color) and its future climate, the minimum genetic distance of a contemporary population P (red color) to the theoretically required population for location L. The purple dotted line represents the association between the genetic composition of several populations (blue circles) and their local, contemporary climate. These figures are modified from Rellstab (2021).
The GF is an extension of random forests based on the non-parametric, machine-learning regression tree approach (Ellis et al., 2012). This method enables to estimate and map the frequency changes of SNPs associated with environmental tolerance at different spatial-temporal scales (Fitzpatrick and Keller, 2015). It can be executed via the R package gradientForest (Ellis et al., 2012). Unlike GDM, GF can handle complicated associations between predictors and accommodate these correlated predictors, providing a means to determine the response of individual SNPs to environmental gradients.
Both GDM and GF can handle large genomic datasets that include numerous rare alleles and accommodate pronounced non-linearities in the exploration of GEAs, providing unprecedented insights into genome regions under local selection and predicting the changes of adaptive genomic diversity across landscape. For instance, Martins et al. (2018) revealed a strong association between the genetic variation of Quercus rugosa and the precipitation seasonality in Mexico via the GDM and GF, and they predicted that future populations of Q. rugosa might be at risk due to the high rate of climate change. However, considering that the actual evolutionary responses of populations to climate change will be more complex than the simplified projections based on the two abovementioned approaches, we must consider caveats when explaining the result of genetic offset arising from the GDM and GF.
Predicting Genomic Vulnerability Using Linear Regression
Rellstab et al. (2016) developed a method called RONA to evaluate genomic vulnerabilities of populations under alternative climate scenarios based on linear regressions inspired by the study of assessing the relative risk of maladaptation in Douglas fir (Bradley St Clair and Howe, 2007). RONA represents the average change in allele frequency at adaptive SNPs required to keep pace with the change of a given environmental factor in future (Figure 3). The average absolute difference of the changes in allele frequencies of these loci between the current and future climate conditions represents RONA under a given environmental variable (Rellstab et al., 2016; Pina-Martins et al., 2019). Recently, Borrell et al. (2020) utilized the current RONA (c-RONA, Figure 3) to define the average change in allele frequency at climate-associated loci required to match the estimation of the optimum for a given environmental factor, using the future RONA (f-RONA; Figure 3) to define the original concept of RONA proposed by Rellstab et al. (2016).
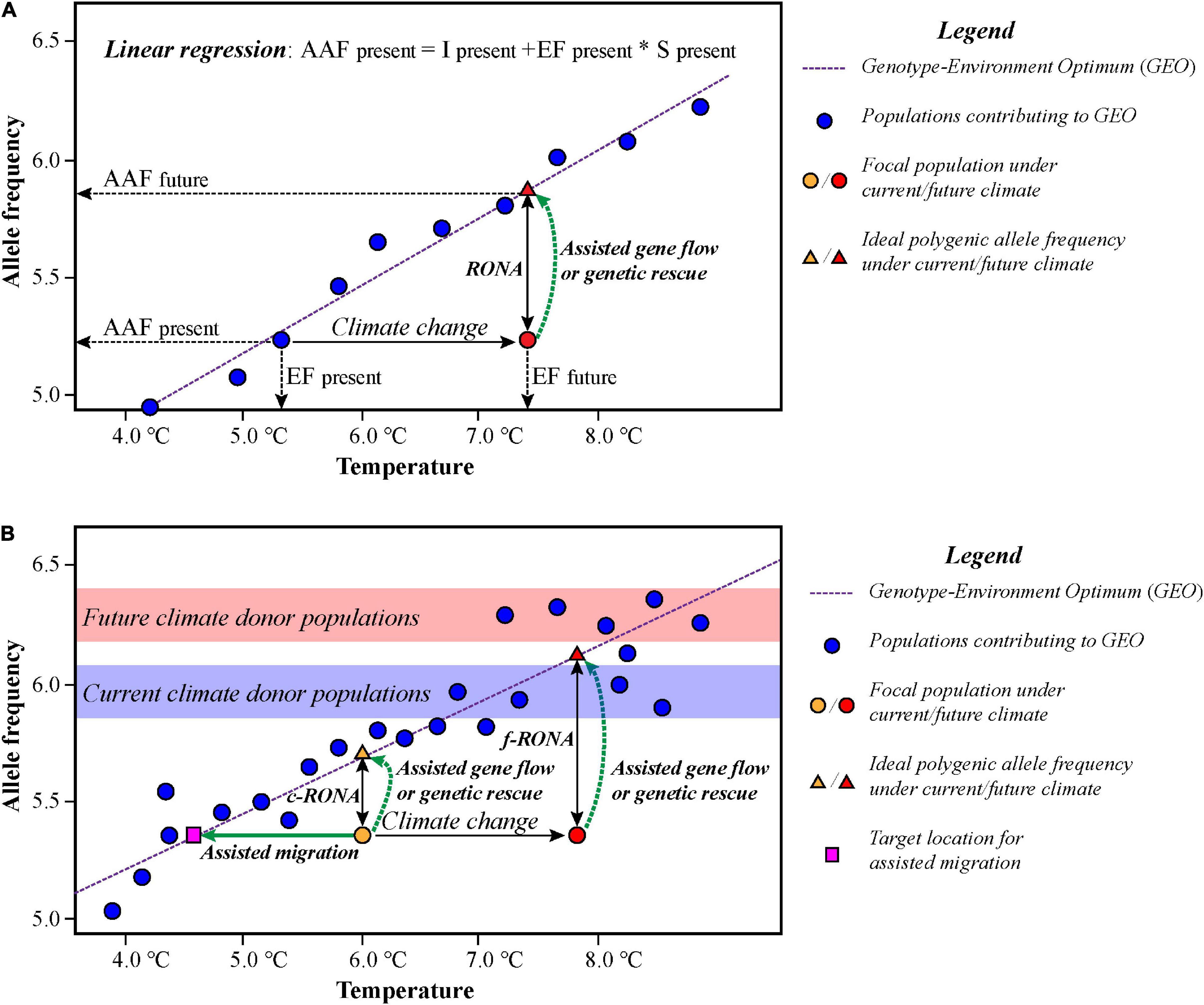
Figure 3. Schematic illustration of the risk of non-adaptedness (RONA) to alternative climate change. (A) RONA is the average change of allele frequency in a set of adaptive loci that are required under future climate scenario according to a simple linear regression of the relationship of allele frequency and environments. AAF, alternative allele frequency; EF, environmental factor; I, intercept of the regression; S, slope of the regression. (B) The current and future RONA (c-RONA and f-RONA); c-RONA/f-RONA is the average change in allele frequency required under current environmental conditions. Blue and red bands indicate suitable candidate donor populations for assisted gene flow under current and future scenarios, respectively. The figures (A,B) are modified from Rellstab et al. (2016) and Borrell et al. (2020), respectively.
Theoretically, if the difference between the current and the prediction values is high, then more conservation efforts are needed for persisting of focal species. Empirical studies in trees and invertebrates show that if the expected allele frequency changes are less than 0.1 per decade, it might keep pace with climate change, while if the changes are greater than 0.1–0.2 per decade, it may cause a lag between allele frequency and climate adaptation (Jump, 2006; Egan et al., 2015; Jump et al., 2017). However, this simplified approach does not take gene flow and migration into account and assumes that the best model profiling the GEAs is resulted from local adaptation. Furthermore, the predictions of RONA for adaptive loci based on this method have multiple values, and each RONA arises from a given environmental variable, which will not account for the interactive effects of loci contributing to climate adaptation (Rellstab et al., 2016; Capblancq et al., 2020). Therefore, we must keep in mind that the genomic vulnerability approaches are still in their infancy and face numerous challenges and uncertainties, and they have yet to be tested and validated in real conservation applications (Rellstab, 2021).
Applications of Landscape Genomics in Tree Conservation
Landscape genomics significantly improves our understanding of ecological and evolutionary processes in tree species and offers guidelines for conservation efforts and management applications. The potential of landscape genomics for forest management is discussed in the following sections.
Using Landscape Genomics to Inform Genetic Rescue
Genetic rescue aims to increase population fitness and avoid population declines by introducing immigration of new alleles (Tallmon et al., 2004; Whiteley et al., 2015; Bell et al., 2019; Fitzpatrick and Funk, 2021). Landscape genomics studies will increase the effectiveness of genetic rescue by identifying which populations are most likely to increase fitness and population growth rate (Whiteley et al., 2015). The population with the lowest level of adaptive differentiation would be chosen in order to minimize outbreeding impression. A recent study on dwarf birch suggested that the genetic rescue should be applied for the populations with small population sizes that occurred in the margins of the distribution of species (Borrell et al., 2020). However, previous studies had indicated that genetic rescue can only improve fitness and increase population sizes in the short term rather than save imperiled populations over the long term (Whiteley et al., 2015). Additionally, if the inbreeding depression in small populations resulted from the recent effect of human-caused fragmentation, assisted migration is more appropriate than genetic rescue (Hohenlohe et al., 2021).
Using Landscape Genomics to Inform Assisted Gene Flow
Assisted gene flow (AGF) means managed translocation of individuals within the current species range to mitigate local maladaptation (Aitken and Whitlock, 2013; Aitken and Bemmels, 2016). AGF is equivalent to the genetic rescue when target populations are small and maladapted, with genetic diversity therein dominantly threatened by drift (Aitken and Whitlock, 2013). Compared with genetic rescue, AGF emphasizes the introduced alleles that are preadapted to new local environments and thus increase the frequency of these adaptive loci in existing populations. AGF has already been applied for some forest trees. For example, Browne et al. (2019) suggested that AGF might be applied to mitigate adaptation lag of temperature for California oak according to a landscape genomic survey of the species. Another fascinating case is dissecting the associations of GEAs in balsam poplar (Gougherty et al., 2021). Gougherty et al. found that the eastern populations of the balsam poplar might face the greatest vulnerability and risk of future extirpation to climate change, and the conservation efforts via AGF are needed for those populations by estimating the local, forward, and reverse genetic offsets of the species. However, outbreeding depression might occur if source and recipient populations are isolated for a long time (Aitken and Whitlock, 2013). Additionally, high levels of gene flow introduced by AGF might result in biotic homogenization between source and target populations and consequently prevent them from adapting to novel climate conditions (Gaitán-Espitia and Hobday, 2021).
Using Landscape Genomics to Inform Seed Sourcing Strategy
Seed sourcing strategy aims to capture the adaptive diversity and improve the adaptive potential of species under climate change and has been proposed for ecological restoration during past decades (Broadhurst et al., 2008; Breed et al., 2013, 2019). Landscape genomics is an ideal approach to inform seed sourcing strategies for species persisting. Jordan et al. (2017) detected 81 putatively adaptive SNPs in Eucalyptus microcarpa, and 62 of which are associated with mean annual temperature by a combination of four FST outlier tests and one EAA (i.e., BAYENV2) as the general framework of landscape genomics (Figure 1). They found that the expected allelic frequency changes of these adaptive SNPs in the New South Wales (NSW) populations were greater than that of other sites, suggesting that the warmer, northern end of the range (i.e., NSW) of E. microcarpa might not suitable for seed source. Recently, a provenance decision-making framework proposed by Carvalho et al. (2021) offers a comprehensive perspective for seed source guidelines based on the information that arises from neutral and adaptive variation via integrative analyses of population genomics and landscape genomics, which can also be applied for informing seed sourcing strategy of forest species.
Challenges and Future Directions
Landscape genomics provides unprecedented insights into understanding the mechanism of adaptive variation of tree species by dissecting the impacts of environmental variables and landscape characteristics on their adaptive evolution (Browne et al., 2019; Pina-Martins et al., 2019; Borrell et al., 2020; Du et al., 2020; Zhao et al., 2020). Common challenges, such as the sampling strategies and using only a single analysis for detecting adaptive signatures, still exist in landscape genomic studies although many reviews discuss the abovementioned topics (Balkenhol et al., 2019). Instead of discussing the abovementioned common challenges in this study, we focused on the challenge of landscape genomics studies on tree species in identifying adaptive variation and their spatial patterns facing the changing climate.
First, the levels of commonality in genes or SNPs associated with climates that arose in landscape genomics studies are quite low. Although the large majority of landscape genomics studies utilize integrative methods for detecting putatively adaptive loci to illuminate the GEAs, few loci are shared between these approaches. These inconsistent patterns by different methods were detected in many studies, for example, in Mexican oak Q. rugose (Martins et al., 2018: only one SNP associated with temperature seasonality was identical between LFMM and BAYESCAN test) or Norway spruce in three independent landscape genomic studies across the Italian Alps sharing similar sampling areas and climates (Scalfi et al., 2014; Ćalić, 2015; Di Pierro et al., 2016: no identical adaptive genes were detected in more than two studies).
The low commonality in adaptive signals might be the evidence for lacking parallel evolution of adaptive traits in forest trees (Geraldes et al., 2014) or just because of false-positive results (Ćalić et al., 2015). Additionally, we believed that the varied methods applied for detecting adaptive SNPs have different assumptions, advantages, and limitations, which responded to the low commonality. However, the commonality levels for projecting genomic vulnerability under alternative climate scenarios using GF or GDM are relatively high. Fitzpatrick and Keller (2015) found that using GDM and GF for the projections of genomic vulnerability, the genetic offset of the circadian clock gene GIGANTEA-5 (GI5) associated with plant circadian clock and light perception pathways in balsam poplar is similar, although slight differences existed in its marginal area, both methods predict the range core of balsam poplar likely suffered minimal disruption of the existing GEAs. In the future, efforts by a combination of simulations, genomic data, and common garden experiments might be applied to demonstrate the high effectivity and accuracy of genomic offset under alternative climates (Fitzpatrick et al., 2021).
Second, the current studies of landscape genomics for evaluating and uncovering the adaptive variation in tree species focus only on a single species rather than at the community level (Table 1). Analyzing multiple species within the same landscapes makes it possible to assess the commonality of their eco-evolutionary dynamics across species and landscapes and thereby depict a thorough picture of how local adaptation is originated in nature (Bragg et al., 2015; Hand et al., 2015; Balkenhol et al., 2019). However, eco-evolutionary models require new data and methods for assessing the adaptive potential of species, which have only been possible for a few model species so far (Waldvogel et al., 2020). In addition, the present challenge of illuminating ecological adaptation at the community level is how to simulate the patterns of local adaptation of species or populations and their adaptive potential under future climate changes, while a possible way to overcome these inconveniences is integrating the prediction methods including GDM, GF, or RONA into the analytical framework of landscape community genomics.
Finally, the investigators of landscape genomics must consider the genomic sequencing strategy employed and the genomic resources available for their focal species. Prevalent sequencing methods in the landscape genomics studies of non-model species currently take advantage of reduced-representation methods [e.g., genotype-by-sequencing (GBS) and restriction-site associated DNA sequencing (RADseq)] and RNA sequencing. However, the number of SNPs obtained and the ability to detect genes underlying local adaptation from the abovementioned methods may be influenced due to the differences in library preparation, SNP densities, and the bioinformatics parameters applied to SNP filtering (Hoban et al., 2016; Lowry et al., 2017; McKinney et al., 2017). As more and more forest tree genomes have been published (e.g., Table 1 in Ingvarsson et al., 2016) and sequencing costs fall, whole-genome resequencing is thriving and becoming an option for landscape genomics studies (Lin et al., 2018; Zhu et al., 2020), which can provide unprecedented marker density and determine other genetic variation such as structural variants and mutations in regulatory elements, increasing power for the detection of local adaptation and providing novel insights into the role of selection, recombination, and gene flow in promoting or impairing local adaptation to new habitats compared with reduced-representation methods (Fuentes-Pardo and Ruzzante, 2017; Bourgeois and Warren, 2021). In addition, the degrees of linkage disequilibrium (LD) in the studied species will also influence the power of detecting adaptive SNPs. Considering the low LD rates in tree species, using these methods such as reduced-representation methods will fail to detect loci that underlie most local adaptation and adaptive phenotypic variation (Bragg et al., 2015; Hoban et al., 2016). We advocated obtaining detailed LD information of focal species using whole-genome sequencing before the studies of landscape genomics in future because the resources of reference genome are critical to fully address the issues of local adaptation (Manel et al., 2016). Moreover, prior knowledge about LD decay from the reference genome of focal species can inform sampling strategies and sequencing selections to maximize opportunities to identify adaptive SNPs (Bragg et al., 2015).
Conclusion
Understanding the genetic mechanism of adaptation is the key issue for molecular ecology and evolutionary biology. We reviewed the existing theories and methods that belong to landscape genomics for detecting adaptive evolution in species and advocated utilizing an integrated analytical framework to illuminate the GEAs between genetic and environmental data. We particularly emphasized the effectivity and necessity of multiple methods for detecting signatures of local adaptation combined with models for predicting adaptation potential in tree conservation. With the low sequencing cost, ease availability of high-solution environmental data, and newly developed genomic tools in the near future, we believe that the conservation efforts and management interventions for forest trees will benefit from advancing studies of landscape genomics.
Author Contributions
LF and FD conceived the study and wrote the manuscript. Both authors designed the focus, structure and content of the review.
Funding
This study was financially supported by the 111 Project (No. B20050) of BJFU, the National Natural Science Foundation of China (42071060 and 31901075), and the China Postdoctoral Science Foundation (2018M633490).
Conflict of Interest
The authors declare that the research was conducted in the absence of any commercial or financial relationships that could be construed as a potential conflict of interest.
Publisher’s Note
All claims expressed in this article are solely those of the authors and do not necessarily represent those of their affiliated organizations, or those of the publisher, the editors and the reviewers. Any product that may be evaluated in this article, or claim that may be made by its manufacturer, is not guaranteed or endorsed by the publisher.
Acknowledgments
The authors thank three reviewers and Rong Wang in the East China Normal University for useful comments on the early draft, and Keke Liu and Min Qi for their contributions on Table 1.
References
Ahrens, C. W., Byrne, M., and Rymer, P. D. (2019). Standing genomic variation within coding and regulatory regions contributes to the adaptive capacity to climate in a foundation tree species. Mol. Ecol. 28, 2502–2516. doi: 10.1111/mec.15092
Aitken, S. N., and Bemmels, J. B. (2016). Time to get moving: assisted gene flow of forest trees. Evol. Appl. 9, 271–290. doi: 10.1111/eva.12293
Aitken, S. N., and Whitlock, M. C. (2013). Assisted gene flow to facilitate local adaptation to climate change. Annu. Rev. Ecol. Evol. Syst. 44, 367–388. doi: 10.1146/annurev-ecolsys-110512-135747
Aitken, S. N., Yeaman, S., Holliday, J. A., Wang, T., and Curtis-Mclane, S. (2008). Adaptation, migration or extirpation: climate change outcomes for tree populations. Evol. Appl. 1, 95–111. doi: 10.1111/j.1752-4571.2007.00013.x
Alberto, F. J., Aitken, S. N., Alía, R., González-Martínez, S. C., Hänninen, H., Kremer, A., et al. (2013). Potential for evolutionary responses to climate change - evidence from tree populations. Global Change Biol. 19, 1645–1661. doi: 10.1111/gcb.12181
Allendorf, F. W., Hohenlohe, P. A., and Luikart, G. (2010). Genomics and the future of conservation genetics. Nat. Rev. Genet. 11, 697–709. doi: 10.1038/nrg2844
Anderson, J. T., and Song, B.-H. (2020). Plant adaptation to climate change-Where are we? J. Syst. Evol. 58, 533–545. doi: 10.1111/jse.12649
Balkenhol, N., Dudaniec, R. Y., Krutovsky, K. V., Johnson, J. S., Cairns, D. M., Segelbacher, G., et al. (2019). “Landscape genomics: Understanding relationships between environmental heterogeneity and genomic characteristics of populations,” in Population Genomics: Concepts, Approaches and Applications, ed. O. P. Rajora (Cham: Springer International Publishing), 261–322. doi: 10.1186/s12868-016-0283-6
Barrett, R. D. H., and Schluter, D. (2008). Adaptation from standing genetic variation. Trends Ecol. Evol. 23, 38–44. doi: 10.1016/j.tree.2007.09.008
Bay, R. A., Harrigan, R. J., Underwood, V. L., Gibbs, H. L., Smith, T. B., and Ruegg, K. (2018). Genomic signals of selection predict climate-driven population declines in a migratory bird. Science 359, 83–85. doi: 10.1126/science.aan4380
Bell, D. A., Robinson, Z. L., Funk, W. C., Fitzpatrick, S. W., Allendorf, F. W., Tallmon, D. A., et al. (2019). The exciting potential and remaining uncertainties of genetic rescue. Trends Ecol. Evol. 34, 1070–1079. doi: 10.1016/j.tree.2019.06.006
Borrell, J. S., Zohren, J., Nichols, R. A., and Buggs, R. J. A. (2020). Genomic assessment of local adaptation in dwarf birch to inform assisted gene flow. Evol. Appl. 13, 161–175. doi: 10.1111/eva.12883
Bourgeois, Y. X. C., and Warren, B. H. (2021). An overview of current population genomics methods for the analysis of whole-genome resequencing data in eukaryotes. Mol. Ecol. 30, 6036–6071. doi: 10.1111/mec.15989
Bradley St Clair, J., and Howe, G. T. (2007). Genetic maladaptation of coastal Douglas-fir seedlings to future climates. Global Change Biol. 13, 1441–1454. doi: 10.1111/j.1365-2486.2007.01385.x
Bragg, J. G., Supple, M. A., Andrew, R. L., and Borevitz, J. O. (2015). Genomic variation across landscapes: insights and applications. New Phytol. 207, 953–967. doi: 10.1111/nph.13410
Breed, M. F., Harrison, P. A., Blyth, C., Byrne, M., Gaget, V., Gellie, N. J. C., et al. (2019). The potential of genomics for restoring ecosystems and biodiversity. Nat. Rev. Genet. 20, 615–628. doi: 10.1038/s41576-019-0152-0
Breed, M. F., Stead, M. G., Ottewell, K. M., Gardner, M. G., and Lowe, A. J. (2013). Which provenance and where? Seed sourcing strategies for revegetation in a changing environment. Conserv. Genet. 14, 1–10. doi: 10.1007/s10592-012-0425-z
Broadhurst, L. M., Lowe, A., Coates, D. J., Cunningham, S. A., Mcdonald, M., Vesk, P. A., et al. (2008). Seed supply for broadscale restoration: maximizing evolutionary potential. Evol. Appl. 1, 587–597. doi: 10.1111/j.1752-4571.2008.00045.x
Browne, L., Wright, J. W., Fitz-Gibbon, S., Gugger, P. F., and Sork, V. L. (2019). Adaptational lag to temperature in valley oak (Quercus lobata) can be mitigated by genome-informed assisted gene flow. Proc. Natl. Acad. Sci. U.S.A. 116, 25179–25185. doi: 10.1073/pnas.1908771116
ĆaliĆ, I. (2015). Estimation of Adaptive Genetic Variation in Norway spruce (Picea abies (L.) Karst) to Climate Change. PhD Doctoral dissertation. Florence: University of Florence-Italy.
ĆaliĆ, I., Bussotti, F., Martínez-García, P., and Neale, D. (2015). Recent landscape genomics studies in forest trees-what can we believe? Tree Genet. Genomes 12, 1–7. doi: 10.1007/s11295-015-0960-0
Cao, Y. N., Zhu, S. S., Chen, J., Comes, H. P., Wang, I. J., Chen, L. Y., et al. (2020). Genomic insights into historical population dynamics, local adaptation, and climate change vulnerability of the East Asian tertiary relict Euptelea (Eupteleaceae). Evol. Appl. 13, 2038–2055. doi: 10.1111/eva.12960
Capblancq, T., Fitzpatrick, M. C., Bay, R. A., Exposito-Alonso, M., and Keller, S. R. (2020). Genomic prediction of (mal)adaptation across current and future climatic landscapes. Annu. Rev. Ecol. Evol. Syst. 51, 245–269. doi: 10.1146/annurev-ecolsys-020720-042553
Capblancq, T., Luu, K., Blum, M. G. B., and Bazin, E. (2018). Evaluation of redundancy analysis to identify signatures of local adaptation. Mol. Ecol. Resour. 18, 1223–1233. doi: 10.1111/1755-0998.12906
Carvalho, C. S., Forester, B. R., Mitre, S. K., Alves, R., Imperatriz-Fonseca, V. L., Ramos, S. J., et al. (2021). Combining genotype, phenotype, and environmental data to delineate site-adjusted provenance strategies for ecological restoration. Mol. Ecol. Resour. 21, 44–58. doi: 10.1111/1755-0998.13191
Caye, K., Jumentier, B., Lepeule, J., and François, O. (2019). LFMM 2: fast and accurate inference of gene-environment associations in genome-wide studies. Mol. Biol. Evol. 36, 852–860. doi: 10.1093/molbev/msz008
Coop, G., Witonsky, D., Di Rienzo, A., and Pritchard, J. K. (2010). Using environmental correlations to identify loci underlying local adaptation. Genetics 185, 1411–1423. doi: 10.1534/genetics.110.114819
De Lafontaine, G., Napier, J. D., Petit, R. J., and Hu, F. S. (2018). Invoking adaptation to decipher the genetic legacy of past climate change. Ecology 99, 1530–1546. doi: 10.1002/ecy.2382
De Mita, S., Thuillet, A.-C., Gay, L., Ahmadi, N., Manel, S., Ronfort, J., et al. (2013). Detecting selection along environmental gradients: analysis of eight methods and their effectiveness for outbreeding and selfing populations. Mol. Ecol. 22, 1383–1399. doi: 10.1111/mec.12182
Di Pierro, E. A., Mosca, E., Rocchini, D., Binelli, G., Neale, D. B., and La Porta, N. (2016). Climate-related adaptive genetic variation and population structure in natural stands of Norway spruce in the South-Eastern Alps. Tree Genet. Genomes 12, 1–15. doi: 10.1007/s11295-016-0972-4
Du, F. K., Wang, T., Wang, Y., Ueno, S., and De Lafontaine, G. (2020). Contrasted patterns of local adaptation to climate change across the range of an evergreen oak, Quercus aquifolioides. Evol. Appl. 13, 2377–2391. doi: 10.1111/eva.13030
Duruz, S., Sevane, N., Selmoni, O., Vajana, E., Leempoel, K., Stucki, S., et al. (2019). Rapid identification and interpretation of gene-environment associations using the new R. SamBada landscape genomics pipeline. Mol. Ecol. Resour. 19, 1355–1365. doi: 10.1111/1755-0998.13044
Egan, S. P., Ragland, G. J., Assour, L., Powell, T. H., Hood, G. R., Emrich, S., et al. (2015). Experimental evidence of genome-wide impact of ecological selection during early stages of speciation-with-gene-flow. Ecol. Lett. 18, 817–825. doi: 10.1111/ele.12460
Elith, J., and Leathwick, J. R. (2009). Species distribution models: ecological explanation and prediction across space and time. Annu. Rev. Ecol. Evol. Syst. 40, 677–697. doi: 10.1146/annurev.ecolsys.110308.120159
Ellis, N., Smith, S. J., and Pitcher, C. R. (2012). Gradient forests: calculating importance gradients on physical predictors. Ecology 93, 156–168. doi: 10.1890/11-0252.1
Eveno, E., Collada, C., Guevara, M. A., Léger, V., Soto, A., Díaz, L., et al. (2008). Contrasting patterns of selection at Pinus pinaster Ait. drought stress candidate genes as revealed by genetic differentiation analyses. Mol. Biol. Evol. 25, 417–437. doi: 10.1093/molbev/msm272
Excoffier, L., Hofer, T., and Foll, M. (2009). Detecting loci under selection in a hierarchically structured population. Heredity 103, 285–298. doi: 10.1038/hdy.2009.74
Fenderson, L. E., Kovach, A. I., and Llamas, B. (2020). Spatiotemporal landscape genetics: investigating ecology and evolution through space and time. Mol. Ecol. 29, 218–246. doi: 10.1111/mec.15315
Feng, L., Ruhsam, M., Wang, Y. H., Li, Z. H., and Wang, X. M. (2020). Using demographic model selection to untangle allopatric divergence and diversification mechanisms in the Rheum palmatum complex in the Eastern Asiatic Region. Mol. Ecol. 29, 1791–1805. doi: 10.1111/mec.15448
Ferrier, S., Drielsma, M., Manion, G., and Watson, G. (2002). Extended statistical approaches to modelling spatial pattern in biodiversity in northeast New South Wales. II. community-level modelling. Biodivers. Conserv. 11, 2309–2338. doi: 10.1023/A:1021302930424
Ferrier, S., Manion, G., Elith, J., and Richardson, K. (2007). Using generalized dissimilarity modelling to analyse and predict patterns of beta diversity in regional biodiversity assessment. Divers. Distrib. 13, 252–264. doi: 10.1111/j.1472-4642.2007.00341.x
Fitzpatrick, M. C., and Keller, S. R. (2015). Ecological genomics meets community-level modelling of biodiversity: mapping the genomic landscape of current and future environmental adaptation. Ecol. Lett. 18, 1–16. doi: 10.1111/ele.12376
Fitzpatrick, M. C., Chhatre, V. E., Soolanayakanahally, R. Y., and Keller, S. R. (2021). Experimental support for genomic prediction of climate maladaptation using the machine learning approach Gradient Forests. Mol. Ecol. Resour. 21, 2749–2765. doi: 10.1111/1755-0998.13374
Fitzpatrick, S. W., and Funk, W. C. (2021). “Genomics for genetic rescue,” in Population Genomics: Wildlife, eds P. A. Hohenlohe and O. P. Rajora (Cham: Springer International Publishing), 437–471. doi: 10.1007/13836_2019_64
Forester, B. R., Jones, M. R., Joost, S., Landguth, E. L., and Lasky, J. R. (2016). Detecting spatial genetic signatures of local adaptation in heterogeneous landscapes. Mol. Ecol. 25, 104–120. doi: 10.1111/mec.13476
Forester, B. R., Lasky, J. R., Wagner, H. H., and Urban, D. L. (2018). Comparing methods for detecting multilocus adaptation with multivariate genotype-environment associations. Mol. Ecol. 27, 2215–2233. doi: 10.1111/mec.14584
Frichot, E., and Francois, O. (2015). LEA: an R package for landscape and ecological association studies. Methods Ecol. Evol. 6, 925–929. doi: 10.1111/2041-210x.12382
Frichot, E., Schoville, S. D., Bouchard, G., and François, O. (2013). Testing for associations between loci and environmental gradients using latent factor mixed models. Mol. Biol. Evol. 30, 1687–1699. doi: 10.1093/molbev/mst063
Fuentes-Pardo, A. P., and Ruzzante, D. E. (2017). Whole-genome sequencing approaches for conservation biology: advantages, limitations and practical recommendations. Mol. Ecol. 26, 5369–5406. doi: 10.1111/mec.14264
Gaitán-Espitia, J. D., and Hobday, A. J. (2021). Evolutionary principles and genetic considerations for guiding conservation interventions under climate change. Global Change Biol. 27, 475–488. doi: 10.1111/gcb.15359
Gautier, M. (2015). Genome-wide scan for adaptive divergence and association with population-specific covariates. Genetics 201, 1555–1579. doi: 10.1534/genetics.115.181453
Geraldes, A., Farzaneh, N., Grassa, C. J., McKnown, A. D., Guy, R. D., Mansfield, S. D., et al. (2014). Landscape genomics of Populus trichocarpa: the role of hybridization, limited gene flow, and natural selection in shaping patterns of population structure. Evolution 68, 3260–3280. doi: 10.1111/evo.12497
Gougherty, A. V., Keller, S. R., and Fitzpatrick, M. C. (2021). Maladaptation, migration and extirpation fuel climate change risk in a forest tree species. Nat. Clim. Change 11, 166–171. doi: 10.1038/s41558-020-00968-6
Gugger, P. F., Fitz-Gibbon, S. T., Albarrán-Lara, A., Wright, J. W., and Sork, V. L. (2021). Landscape genomics of Quercus lobata reveals genes involved in local climate adaptation at multiple spatial scales. Mol. Ecol. 30, 406–423. doi: 10.1111/mec.15731
Günther, T., and Coop, G. (2013). Robust identification of local adaptation from allele frequencies. Genetics 195, 205–220. doi: 10.1534/genetics.113.152462
Hamrick, J. L., Godt, M. J. W., and Sherman-Broyles, S. L. (1992). “Factors influencing levels of genetic diversity in woody plant species,” in Population Genetics of Forest Trees, eds W. T. Adams, S. H. Strauss, D. L. Copes, and A. R. Griffin (Dordrecht: Springer), 95–124.
Hand, B. K., Lowe, W. H., Kovach, R. P., Muhlfeld, C. C., and Luikart, G. (2015). Landscape community genomics: understanding eco-evolutionary processes in complex environments. Trends Ecol. Evol. 30, 161–168. doi: 10.1016/j.tree.2015.01.005
Hardy, O. J., and Pavoine, S. (2012). Assessing phylogenetic signal with measurement error: a comparison of mantel tests, blomberg et al.’s K, and phylogenetic distograms. Evolution 66, 2614–2621. doi: 10.1111/j.1558-5646.2012.01623.x
Harmon, L. J., and Glor, R. E. (2010). Poor statistical performance of the mantel test in phylogenetic comparative analyses. Evolution 64, 2173–2178. doi: 10.1111/j.1558-5646.2010.00973.x
Hoban, S., Kelley, J. L., Lotterhos, K. E., Antolin, M. F., Bradburd, G., Lowry, D. B., et al. (2016). Finding the genomic basis of local adaptation: pitfalls, practical solutions, and future directions. Am. Nat. 188, 379–397. doi: 10.1086/688018
Hoffmann, A. A., Sgrò, C. M., and Kristensen, T. N. (2017). Revisiting adaptive potential, population size, and conservation. Trends Ecol. Evol. 32, 506–517. doi: 10.1016/j.tree.2017.03.012
Hohenlohe, P. A., Funk, W. C., and Rajora, O. P. (2021). Population genomics for wildlife conservation and management. Mol. Ecol. 30, 62–82. doi: 10.1111/mec.15720
Hohenlohe, P. A., Phillips, P. C., and Cresko, W. A. (2010). Using population genomics to detect selection in natural populations: key concepts and methodological considerations. Int. J. Plant Sci. 171, 1059–1071. doi: 10.1086/656306
Holderegger, R., Kamm, U., and Gugerli, F. (2006). Adaptive vs. neutral genetic diversity: implications for landscape genetics. Landscape Ecol. 21, 797–807. doi: 10.1007/s10980-005-5245-9
Holliday, J. A., Aitken, S. N., Cooke, J. E., Fady, B., Gonzalez-Martinez, S. C., Heuertz, M., et al. (2017). Advances in ecological genomics in forest trees and applications to genetic resources conservation and breeding. Mol. Ecol. 26, 706–717. doi: 10.1111/mec.13963
Ingvarsson, P. K., Hvidsten, T. R., and Street, N. R. (2016). Towards integration of population and comparative genomics in forest trees. New Phytol. 212, 338–344. doi: 10.1111/nph.14153
Isabel, N., Holliday, J. A., and Aitken, S. N. (2020). Forest genomics: advancing climate adaptation, forest health, productivity, and conservation. Evol. Appl. 13, 3–10. doi: 10.1111/eva.12902
Jia, K. H., Zhao, W., Maier, P. A., Hu, X. G., Jin, Y., Zhou, S. S., et al. (2020). Landscape genomics predicts climate change-related genetic offset for the widespread Platycladus orientalis (Cupressaceae). Evol. Appl. 13, 665 676. doi: 10.1111/eva.12891
Jombart, T., Pontier, D., and Dufour, A. B. (2009). Genetic markers in the playground of multivariate analysis. Heredity 102, 330–341. doi: 10.1038/hdy.2008.130
Joost, S., Bonin, A., Bruford, M. W., Després, L., Conord, C., Erhardt, G., et al. (2007). A spatial analysis method (SAM) to detect candidate loci for selection: towards a landscape genomics approach to adaptation. Mol. Ecol. 16, 3955–3969. doi: 10.1111/j.1365-294X.2007.03442.x
Joost, S., Kalbermatten, M., and Bonin, A. (2008). Spatial analysis method (sam): a software tool combining molecular and environmental data to identify candidate loci for selection. Mol. Ecol. Resour. 8, 957–960. doi: 10.1111/j.1755-0998.2008.02162.x
Joost, S., Vuilleumier, S., Jensen, J. D., Schoville, S., Leempoel, K., Stucki, S., et al. (2013). Uncovering the genetic basis of adaptive change: on the intersection of landscape genomics and theoretical population genetics. Mol. Ecol. 22, 3659–3665. doi: 10.1111/mec.12352
Jordan, R., Hoffmann, A. A., Dillon, S. K., and Prober, S. M. (2017). Evidence of genomic adaptation to climate in Eucalyptus microcarpa: implications for adaptive potential to projected climate change. Mol. Ecol. 26, 6002–6020. doi: 10.1111/mec.14341
Jump, A. S. (2006). Genetic effects of chronic habitat fragmentation in a wind-pollinated tree. Proc. Natl. Acad. Sci. U.S.A. 103, 8096–8100. doi: 10.1073/pnas.0510127103
Jump, A. S., and Peñuelas, J. (2005). Running to stand still: adaptation and the response of plants to rapid climate change. Ecol. Lett. 8, 1010–1020. doi: 10.1111/j.1461-0248.2005.00796.x
Jump, A. S., Ruiz-Benito, P., Greenwood, S., Allen, C. D., Kitzberger, T., Fensham, F., et al. (2017). Structural overshoot of tree growth with climate variability and the global spectrum of drought-induced forest dieback. Global Change Biol. 23, 3742–3757. doi: 10.1111/gcb.13636
Lefèvre, F., Koskela, J., Hubert, J., Kraigher, H., Longauer, R., Olrik, D. C., et al. (2013). Dynamic conservation of forest genetic resources in 33 European countries. Conserv. Biol. 27, 373–384. doi: 10.1111/j.1523-1739.2012.01961.x
Legendre, P., and Anderson, M. J. (1999). Distance-based redundancy analysis: testing multispecies responses in multifactorial ecological experiments. Ecol. Monogr. 69, 1–24. doi: 10.2307/2657192
Legendre, P., Fortin, M.-J., Borcard, D., and Peres-Neto, P. (2015). Should the Mantel test be used in spatial analysis? Methods Ecol. Evol. 6, 1239–1247. doi: 10.1111/2041-210x.12425
Lin, Y. C., Wang, J., Delhomme, N., Schiffthaler, B., Sundström, G., Zuccolo, A., et al. (2018). Functional and evolutionary genomic inferences in Populus through genome and population sequencing of American and European aspen. Proc. Natl. Acad. Sci. U.S.A. 115, E10970–E10978. doi: 10.1073/pnas.1801437115
Lowry, D. B., Hoban, S., Kelley, J. L., Lotterhos, K. E., Reed, L. K., Antolin, M. F., et al. (2017). Breaking RAD: an evaluation of the utility of restriction site associated DNA sequencing for genome scans of adaptation. Mol. Ecol. Resour. 17, 142–152. doi: 10.1111/1755-0998.12635
Manel, S., Perrier, C., Pratlong, M., Abi-Rached, L., Paganini, J., Pontarotti, P., et al. (2016). Genomic resources and their influence on the detection of the signal of positive selection in genome scans. Mol. Ecol. 25, 170–184. doi: 10.1111/mec.13468
Manion, G., Lisk, M., Ferrier, S., Nieto-Lugilde, D., and Fitzpatrick, M. (2014). GDM: Functions for Generalized Dissimilarity Modeling. R Package Version 1.2.3. Available at online: http://CRAN.R-project.org/package=gdm (accessed 18 January 2017).
Manthey, J. D., and Moyle, R. G. (2015). Isolation by environment in white-breasted nuthatches (Sitta carolinensis) of the Madrean Archipelago sky islands: a landscape genomics approach. Mol. Ecol. 24, 3628–3638. doi: 10.1111/mec.13258
Martins, K., Gugger, P. F., Llanderal-Mendoza, J., González-Rodríguez, A., Fitz-Gibbon, S. T., Zhao, J.-L., et al. (2018). Landscape genomics provides evidence of climate-associated genetic variation in Mexican populations of Quercus rugosa. Evol. Appl. 11, 1842–1858. doi: 10.1111/eva.12684
McKinney, G. J., Larson, W. A., Seeb, L. W., and Seeb, J. E. (2017). RADseq provides unprecedented insights into molecular ecology and evolutionary genetics: comment on breaking RAD by Lowry et al. (2016). Mol. Ecol. Resour. 17, 356–361. doi: 10.1111/1755-0998.12649
Milesi, P., Berlin, M., Chen, J., Orsucci, M., Li, L. L., Jansson, G., et al. (2019). Assessing the potential for assisted gene flow using past introduction of Norway spruce in southern Sweden: local adaptation and genetic basis of quantitative traits in trees. Evol. Appl. 12, 1946–1959. doi: 10.1111/eva.12855
Nagamitsu, T., and Shuri, K. (2021). Seed transfer across geographic regions in different climates leads to reduced tree growth and genetic admixture in Quercus mongolica var. crispula. Forest Ecol. Manag. 482:118787. doi: 10.1016/j.foreco.2020.118787
Neale, D. B., and Kremer, A. (2011). Forest tree genomics: growing resources and applications. Nat. Rev. Genet. 12, 111–122. doi: 10.1038/nrg2931
Oksanen, J., Blanchet, F. G., Kindt, R., Legendre, P., Minchin, P. R., O’Hara, R., et al. (2013). Package ‘Vegan’. Community Ecology Package, Version 2. Available online at: http://CRAN.R-project.org/package=vegan (accessed September 23, 2021).
Olazcuaga, L., Loiseau, A., Parrinello, H., Paris, M., Fraimout, A., Guedot, C., et al. (2020). A whole-genome scan for association with invasion success in the fruit fly Drosophila suzukii using contrasts of allele frequencies corrected for population structure. Mol. Biol. Evol. 37, 2369–2385. doi: 10.1093/molbev/msaa098
Petit, R. J., and Hampe, A. (2006). Some evolutionary consequences of being a tree. Annu. Rev. Ecol. Evol. Syst. 37, 187–214. doi: 10.1146/annurev.ecolsys.37.091305.110215
Pina-Martins, F., Baptista, J., Pappas, G., and Paulo, O. S. (2019). New insights into adaptation and population structure of cork oak using genotyping by sequencing. Global Change Biol. 25, 337–350. doi: 10.1111/gcb.14497
Rellstab, C. (2021). Genomics helps to predict maladaptation to climate change. Nat. Clim. Change 11, 85–86. doi: 10.1038/s41558-020-00964-w
Rellstab, C., Gugerli, F., Eckert, A. J., Hancock, A. M., and Holderegger, R. (2015). A practical guide to environmental association analysis in landscape genomics. Mol. Ecol. 24, 4348–4370. doi: 10.1111/mec.13322
Rellstab, C., Zoller, S., Walthert, L., Lesur, I., Pluess, A. R., Graf, R., et al. (2016). Signatures of local adaptation in candidate genes of oaks (Quercus spp.) with respect to present and future climatic conditions. Mol. Ecol. 25, 5907–5924. doi: 10.1111/mec.13889
Rinnan, D. S., and Lawler, J. (2019). Climate-niche factor analysis: a spatial approach to quantifying species vulnerability to climate change. Ecography 42, 1494–1503. doi: 10.1111/ecog.03937
Savolainen, O., Lascoux, M., and Merila, J. (2013). Ecological genomics of local adaptation. Nat. Rev. Genet. 14, 807–820. doi: 10.1038/nrg3522
Savolainen, O., Pyhäjärvi, T., and Knürr, T. (2007). Gene flow and local adaptation in trees. Annu. Rev. Ecol. Evol. Syst. 38, 595–619. doi: 10.2307/30033873
Scalfi, M., Mosca, E., Di Pierro, E. A., Troggio, M., Vendramin, G. G., Sperisen, C., et al. (2014). Micro and macro-geographic scale effect on the molecular imprint of selection and adaptation in Norway spruce. PLoS One 9:e115499. doi: 10.1371/journal.pone.0115499
Schoville, S. D., Bonin, A., François, O., Lobreaux, S., Melodelima, C., and Manel, S. (2012). Adaptive genetic variation on the landscape: methods and cases. Annu. Rev. Ecol. Evol. Syst. 43, 23–43. doi: 10.1146/annurev-ecolsys-110411-160248
Sork, V. L. (2017). Genomic studies of local adaptation in natural plant populations. J. Hered. 109, 3–15. doi: 10.1093/jhered/esx091
Sork, V. L., Aitken, S. N., Dyer, R. J., Eckert, A. J., Legendre, P., and Neale, D. B. (2013). Putting the landscape into the genomics of trees: approaches for understanding local adaptation and population responses to changing climate. Tree Genet. Genomes 9, 901–911. doi: 10.1007/s11295-013-0596-x
Stucki, S., Orozco-Terwengel, P., Forester, B. R., Duruz, S., Colli, L., Masembe, C., et al. (2017). High performance computation of landscape genomic models including local indicators of spatial association. Mol. Ecol. Resour. 17, 1072–1089. doi: 10.1111/1755-0998.12629
Tallmon, D. A., Luikart, G., and Waples, R. S. (2004). The alluring simplicity and complex reality of genetic rescue. Trends Ecol. Evol. 19, 489–496. doi: 10.1016/j.tree.2004.07.003
Ter Braak, C. J. F. (1986). Canonical correspondence analysis: a new eigenvector technique for multivariate direct gradient analysis. Ecology 67, 1167–1179. doi: 10.2307/1938672
Van Den Wollenberg, A. L. (1977). Redundancy analysis an alternative for canonical correlation analysis. Psychometrika 42, 207–219. doi: 10.1007/BF02294050
Waldvogel, A.-M., Feldmeyer, B., Rolshausen, G., Exposito-Alonso, M., Rellstab, C., Kofler, R., et al. (2020). Evolutionary genomics can improve prediction of species’ responses to climate change. Evol. Lett. 4, 4–18. doi: 10.1002/evl3.154
Walters, S. J., Robinson, T. P., Byrne, M., Wardell-Johnson, G. W., and Nevill, P. (2020). Contrasting patterns of local adaptation along climatic gradients between a sympatric parasitic and autotrophic tree species. Mol. Ecol. 29, 3022–3037. doi: 10.1111/mec.15537
Wang, I. J., and Bradburd, G. S. (2014). Isolation by environment. Mol. Ecol. 23, 5649–5662. doi: 10.1111/mec.12938
Whiteley, A. R., Fitzpatrick, S. W., Funk, W. C., and Tallmon, D. A. (2015). Genetic rescue to the rescue. Trends Ecol. Evol. 30, 42–49. doi: 10.1016/j.tree.2014.10.009
Wright, S. I., and Gaut, B. S. (2004). Molecular population genetics and the search for adaptive evolution in plants. Mol. Biol. Evol. 22, 506–519. doi: 10.1093/molbev/msi035
XLSTAT (2012). Leading Data Analysis and Statistical Solution for Microsoft Excel. New York, NY: Addinsoft SRL.
Zhao, W., Sun, Y.-Q., Pan, J., Sullivan, A. R., Arnold, M. L., Mao, J.-F., et al. (2020). Effects of landscapes and range expansion on population structure and local adaptation. New Phytol. 228, 330–343. doi: 10.1111/nph.16619
Keywords: changing environment, genotype-environment associations (GEAs), landscape genomics, local adaptation, tree conservation
Citation: Feng L and Du FK (2022) Landscape Genomics in Tree Conservation Under a Changing Environment. Front. Plant Sci. 13:822217. doi: 10.3389/fpls.2022.822217
Received: 25 November 2021; Accepted: 10 January 2022;
Published: 24 February 2022.
Edited by:
Jue Ruan, Chinese Academy of Agricultural Sciences (CAAS), ChinaReviewed by:
Jun Chen, Zhejiang University, ChinaChengjun Zhang, Kunming Institute of Botany (CAS), China
Suhua Shi, Sun Yat-sen University, China
Copyright © 2022 Feng and Du. This is an open-access article distributed under the terms of the Creative Commons Attribution License (CC BY). The use, distribution or reproduction in other forums is permitted, provided the original author(s) and the copyright owner(s) are credited and that the original publication in this journal is cited, in accordance with accepted academic practice. No use, distribution or reproduction is permitted which does not comply with these terms.
*Correspondence: Fang K. Du, dufang325@bjfu.edu.cn
†ORCID: Li Feng, orcid.org/0000-0002-8252-9463; Fang K. Du, orcid.org/0000-0002-7377-5259