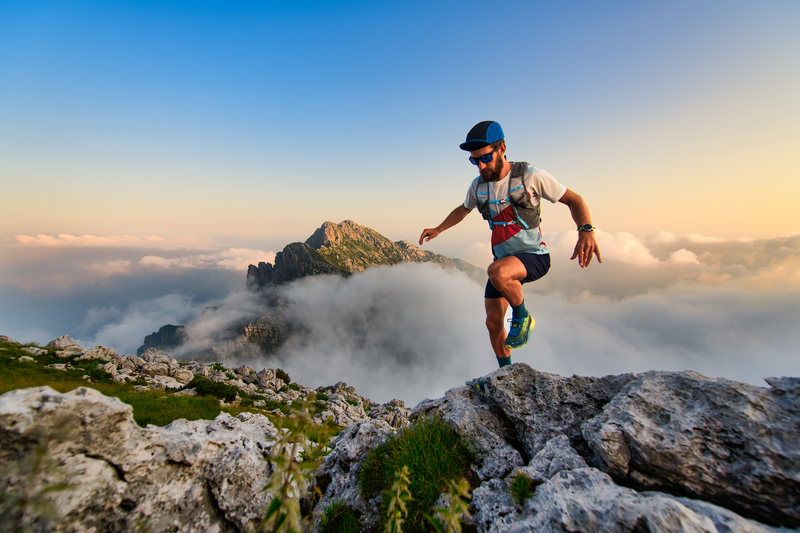
94% of researchers rate our articles as excellent or good
Learn more about the work of our research integrity team to safeguard the quality of each article we publish.
Find out more
ORIGINAL RESEARCH article
Front. Plant Sci. , 31 March 2022
Sec. Plant Bioinformatics
Volume 13 - 2022 | https://doi.org/10.3389/fpls.2022.761244
The timing of flowering is a key determinant for plant reproductive. It has been demonstrated that microRNAs (miRNAs) play an important role in transition from the vegetative to reproductive stage in cotton; however, knowledge remains limited about the regulatory role of miRNAs involved in flowering time regulation in cotton. To elucidate the molecular basis of miRNAs in response to flowering time in cotton, we performed high-throughput small RNA sequencing at the fifth true leaf stage. We identified 56 and 43 miRNAs that were significantly up- and downregulated in two elite early flowering cultivars (EFC) compared with two late flowering cultivars (LFC), respectively. The miRNA targets by RNA sequencing analysis showed that GhSPL4 in SBP transcription factor family targeted by GhmiR156 was significantly upregulated in EFCs. Co-expression regulatory network analysis (WGCNA) revealed that GhSOC1, GhAP1, GhFD, GhCOL3, and GhAGL16 act as node genes in the auxin- and gibberellin-mediated flowering time regulatory networks in cotton. Therefore, elucidation of miRNA-mediated flowering time regulatory network will contribute to our understanding of molecular mechanisms underlying flowering time in cotton.
MicroRNAs (miRNAs) are a class of endogenous small non-coding RNA molecules. In plants, miRNAs post-transcriptionally regulate gene expression by mediating the degradation of target mRNAs or by inhibiting the translation of target mRNAs (Fei et al., 2013; Iwakawa and Tomari, 2013; Ren et al., 2014; Song et al., 2019). For example, most miRNAs guide Argonaute (AGO) proteins cleave the target mRNAs on the basis of near-perfect sequence complementarity by forming an RNA-induced silencing complex (RISC; Eamens et al., 2009; Yu et al., 2017). It is now clear that miRNAs play an important role in plant development such as regulation of abiotic stress responses, meristem organization, and leaf morphology and size (Song et al., 2019).
Flowering time is a key trait that is strongly associated with crop yield (Itoh et al., 2010). Strict regulation of flowering time is critical for reproductive success, enabling the completion of plant generation under appropriate environmental conditions (Purugganan and Fuller, 2009). There are several miRNAs that have been shown to function in controlling flowering. For example, miR156 and miR172 regulate the vegetative to reproductive phase transition as part of the age-dependent flowering pathway (Wu et al., 2009). A decline in the miR156 expression level coincides with the upregulation of SQUAMOSA-PROMOTER BINDING PROTEIN-LIKE (SPL), which activates LEAFY (LFY), FRUITFULL (FUL), and APETALA1 (AP1; Yamaguchi et al., 2009). The miR172 represses the expression APETALA2 (AP2) by activation of SPLs, which further represses the floral inducer FT (Mathieu et al., 2009; Xie et al., 2020). In addition to miR156 and miR172, miR159, miR319, and miR399 also play important role in controlling flowering time. The miR159 represses the expression of miR156 by targeting MYB33 which binds to the LFY promoter (Gocal et al., 2001), and miR319 regulates the TEOSINTE BRANCHED/CYCLOIDEA/PCF (TCP) to promote flowering by binding to the CO promoter and activating transcription (Liu et al., 2017). MiRNA also plays an important role in flowering regulation of other crops. Monocot-specific miR528 promotes flowering under long-day conditions by targeting RED ANDFAR-RED INSENSITIVE 2 (OsRFI2) in rice (Yang et al., 2019). APETALA2-like gene targeted by miR172 promotes vegetative phase change in maize (Lauter et al., 2005). In Brachypodium distachyon, pooideae-specific miR5200 targets FT in flowering time regulation (Wu et al., 2013).
Gibberellic signaling pathway widely determines the flowering time in plants (Yamaguchi, 2008). Earlier studies showed that DELLA proteins (DELLAs) are negative regulator of gibberellin target genes such as REPRESSOR OF ga1-3 (RGA), GA-INSENSITIVE (GAI), RGA-LIKE 1 (RGL1), RGL2, and RGL3 (Achard et al., 2009). A recent study showed that DELLAs repress flowering in Arabidopsis through negative regulation in expression of CONSTANS (CO) which induce the expression of FLOWERING LOCUS T (FT; Wang et al., 2016; Xu et al., 2016). Furthermore, the interaction of CO with NF-YB2 is inhibited by the DELLA protein, thus suppressing flowering (Xu et al., 2016). Besides that, gibberellin signaling pathway regulates the DELLAs by crosstalk with auxin and cytokinin signaling pathway. For example, AUX/IAA proteins also repress the stability of DELLAs (Fu and Harberd, 2003). In addition, ARFs and IAAs directly regulate the GA metabolic enzymes such as GA20ox, GA3ox, and GA2ox (O'Neill and Ross, 2002; Frigerio et al., 2006).
Upland cotton (Gossypium hirsutum L.) is the most economically valuable textile crop in the world (Paterson et al., 2012). A total of 1,500 miRNAs have been identified in different cotton species,1 including 315 miRNAs in G. arboreum, 434 in G. barbadense, 465 in G. hirsutum, and 286 in G. raimondii. RNA sequencing (RNA-seq) is a powerful tool to study gene expression and gene regulatory relationships. Recent studies have shown that regions located to chromosome A05 and chromosome D03 are enriched in early maturity traits, and GhCAL regulates flowering time by controlling the transition from vegetative to reproductive growth (Ma et al., 2018; Cheng et al., 2020; Li et al., 2021). GhUCE and GhBRC1 regulate cotton flowering by integrating multiple hormone pathways (Ma et al., 2018; Sun et al., 2021). Recent study also demonstrated that auxin signaling-associated miR167 also directly affected the differentiation of floral in cotton (Arora and Chaudhary, 2021).
Although several studies on molecular mechanism on flowering time control have been carried out separately focusing on miRNAs and transcriptome sequencing, association analysis combining both omics methods has not been reported yet. Here, we performed an association analysis for mRNAs and miRNAs in four different varieties to gain a better understanding of gene expression and their regulation of this process.
The early-maturing upland cotton cultivars CZ-3 and 4-5-26 and the late-maturing cultivars S25 and 48xi were planted at the Biotechnology Research Institute of the Chinese Academy of Agricultural Sciences Experimental Field in Beijing City, and the plants were managed using general field management practices. The two early-flowering varieties CZ-3 and 4-5-26 belong to different background. However, S25 and 48xi belong to same background. Ten shoot apexes at the fifth true leaf stage were collected for one biological replicate, and two biological replicates were used for RNA extraction. These samples were immediately frozen in liquid nitrogen and stored at −80°C prior to use in the experiments.
miRNAs were isolated and purified using the RNAprep Pure kit (Tiangen, China) following the manufacturer’s procedure. Raw sequencing reads were processed using an in-house program, ACGT101-miR (LC Sciences, Houston, TX, United States) to remove adapter dimers, bad reads, low complexity reads, common RNA families (rRNA, tRNA, snRNA, and snoRNA), and repeats. After this filtering, the obtained over 7,800,000 valid reads per library were used for further analyses (Supplementary Figure S1). Subsequently, unique sequences with lengths of 18–25 nucleotides were mapped to specific species precursors in miRBase 22.0 by BLAST searches to identify known miRNAs and novel 3p- and 5p-derived miRNAs. The differentially expressed miRNAs were selected using |log2 (fold change)| > 1 and value of p < 0.05 thresholds. Target gene identification was performed with Psrobot.2 These sequence data have been submitted to the NCBI databases under accession number PRJNA785082.
RNA was extracted from two early flowering varieties, CZ-3 and 4-5-26, and two later flowering varieties, S25 and 48xi. Total RNA was isolated and purified using TRIzol reagent (Invitrogen, Carlsbad, CA, United States) following the manufacturer’s instructions. The RNA concentration and purity were determined using a NanoDrop ND-1000 spectrophotometer (NanoDrop, Wilmington, DE, United States). The RNA was sequenced (150-base paired-end reads) using an Illumina Novaseq™ 6000 instrument following the manufacturer’s recommended protocol. After removing the adaptor sequences, duplicated sequences, ambiguous reads, and low-quality reads, a total of 41,509,692 (CZ-3), 44,302,944 (4-5-26), 49,553,584 (S25), and 51,455,996 (48xi) valid reads were used in the analyses (Supplementary Figure S1). Gene expression levels were determined using the fragments per kilobase of transcript per million mapped reads (FPKM) method. The differentially expressed mRNAs were selected with fold change >2 or fold-change <0.5 and value of p < 0.05 using the R package edgeR.3 Functional annotation of the DEGs was conducted through the Cotton Functional Genomics website4 with a significance level of 0.05 and a minimum gene number for each analyzed term = 3. These sequence data have been submitted to the NCBI databases under accession number PRJNA785082.
The WGCNA package was used to analyze the co-expressed genes5 with all parameters set as defined: “soft_power = 26, minModuleSize = 30 and mergeCutHeight = 0.25.”
miRNA was reverse-transcribed using SuperScript™ II Reverse Transcriptase (Invitrogen, Carlsbad, United States) according to the manufacturer’s instructions. For quantitative real-time PCR (qRT-PCR), SYBR Green I was added to reaction mix and amplifications were performed on a CFX96 Real-Time PCR Detection System (Bio-Rad, Hercules, CA, United States). The ubiquitin gene GhUBQ7 (Ghir_A11G011460) was used as internal reference control, and the relative expression levels were calculated using the modified 2−ΔΔCT method. Values are means ± SD of three biological replicates. Student’s t-test was used for statistical analysis. Asterisks indicate statistically significant differences compared with the wild type (*p ≤ 0.05; **p ≤ 0.01).
For cotton transformation, the pCLCrV:VIGS constructs were constructed by a method modified from Gu et al. (2014). The basic constructs, pCLCrVA, pCLCrVB, and CLCrV-CHLI, were obtained from them. The fragment of pre-miR156 and a small tandem target mimic (STTM) sequence containing two imperfect binding sites separated by a 48 bp spacer, both were synthesized (Sangon) and inserted into the plant expression vector pCLCrVA by SpeI and PacI to get OE-miR156 and STTM-miRNA vectors. The above pCLCrVA vectors and pCLCrVB construct were introduced into the Agrobacterium tumefaciens strain EHA105. The Agrobacterium cultures were pelleted and resuspended. After 3 h incubation at room temperature, Agrobacterium strains harboring different pCLCrVAs were mixed with an equal volume of Agrobacterium harboring pCLCrVB separately. The mixed Agrobacterium solutions were infiltrated into the abaxial side of cotyledons of 2-week-aged cotton seedlings by needleless syringes through small wounds. Keep the plants at 24°C for 3 weeks till CLCrV-CHLI plants grew out white leaves; then move all plants to the environment at 28°C under long-day conditions (16 h light/8 h dark).
For Arabidoposis transformation, the fragment of pre-miR399e was synthesized (Sangon) and inserted into the plant expression vector pCAMBIA 2,300 using KpnI and PstI. The floral-dip method was used for transformation (Bent, 2006).
In this study, two elite early-flowering cultivars, CZ-3 and 4-5-26, and two elite later flowering cultivars, S25 and 48xi, were used at the fifth true leaf stage in cotton (Figures 1A–D). Compared with the S25 and 48xi, average node of 3.6–5.3 in first fruiting branch in EFC was lower than LFC. Flowering time was 15 days early in both CZ-3 and 4-5-26 (Figure 1E). Previous study showed that four flowering time genes such as GhFT, GhMADS22, GhCAL, and GhUCE promote the cotton flowering (Zhang et al., 2013; Guo et al., 2015; Ma et al., 2018; Cheng et al., 2020). Therefore, we conducted the expression level of those genes in early and late flowering cultivar at fifth leaves stage. Quantitative real-time PCR (qRT-PCR) analysis revealed that the expression level of GhFT, GhMADS22, GhCAL, and GhUCE was 6.75-, 10.2-, 67.07-, and 116.77-fold higher in CZ-3 than in 48xi, respectively (Figure 1F). These results suggest that there is a significant transition between early- and later-flowering cotton varieties at the fifth true leaf stage.
Figure 1. Different phenotypes in the fifth true leaf stage between two early- and two later-flowering cotton varieties. (A,B) Early-flowering CZ-3 and 4-5-26. (C,D) Later-flowering S25 and 48xi. The arrows point to the flower buds. Scale bars = 10 cm. (E) Node of first fruiting branch and flowering times in the four cotton varieties. (F) Expression levels of the flowering time regulatory genes GhCAL, GhMADS22, GhUCE, and GhFT in the cotton shoot apex. Values are means ± SD (n = 3).
To analyze the fluctuation of endogenous miRNA expression level in early- and late-flowering cotton varieties, we constructed four sRNA libraries from CZ-3, 4-5-26, S25, and 48xi and performed small RNA sequencing. After low-quality reads were removed from the raw data, the most abundant length of miRNA was 24 nt (Supplementary Table S1). This size accounted for over 68%, 69%, 70%, and 70% of the sRNAs in the CZ-3, 4-5-26, S25, and 48xi sequencing libraries, respectively (Supplementary Table S2). Novel miRNAs were identified using the MIREAP software based on their precursors, and the hairpin RNA structures containing sequences by using Mfold software. Minimal folding energy (dG in kcal/mol ≤ −17) was considered as novel miRNAs.
A total of 99 differentially-expressed miRNAs were obtained, including 79 known miRNAs and 20 novel miRNAs (log2 (fold change) >1 or <−1; thresholds of p < 0.05). Among these, 56 and 43 miRNAs were significantly up- and downregulated in the two EFCs, respectively (Figure 2A; Supplementary Table S3). Over 59.60% (59) of the mature miRNA sequences were 24 nt in length and 37 precursors were 101–150 length (Figures 2B,C). We also found that 63 miRNAs (including 15 novel miRNAs) map to the D-subgenome (Figure 2D). Besides known miRNA, the lengths of these novel miRNAs varied from 20 to 24 nt. The calculated minimal folding energy ranged from −30.90 to −121 with an average of −70.88 (Supplementary Figure S1). The length of precursor miRNA from 64 to 191 nt (Supplementary Table S3). The correlation assay of differentially expressed miRNA (DEmiRs) between two biological process was strong positive correlation (R2 > 0.9489) in four varieties (Supplementary Figure S2). To validate these miRNAs, qRT-PCR assays were performed for nine randomly selected miRNAs and consistent the results with miRNA-seq (Figure 3).
Figure 2. Overview of small RNA sequencing. (A) Relative changes in gene expression profiles of the DEmiRs between the early flowering varieties and the later flowering varieties. (B) The length distribution of 99 mature miRNAs. (C) The length distribution of 99 miRNA precursors. (D) Distribution of 99 mature miRNAs in the cotton A- and D-subgenomes.
Figure 3. Quantitative real-time PCR verification of seven significantly expressed miRNAs at the fifth true leaf stage in the cotton shoot apex. Values are means ± SD (n = 3).
Our results showed that seven miRNAs were uniquely expressed in the two EFCs including gra-MIR7506b, two gra-MIR8771b, two gra-MIR8780, and two novel miRNAs. While six miRNAs were only expressed in the two LFCs including tcc-miR398a, two gra-MIR8674b and three novel miRNAs (Supplementary Table S3). Fourteen members of miR6300 family and nine miR7489 family members were significantly upregulated in the two EFCs, whereas nine members of miR399 family were downregulated in the two LFCs. In addition, two conserved miRNAs, miR156 and miR319, which are known to function in flowering time regulation in plants, were significantly downregulated in two EFCs compared with the LFCs, while one miR164 and one miR166 showed the reverse expression pattern (Supplementary Table S3).
To better understand the molecular mechanism of miRNA regulation in cotton flowering time, we performed miRNA target gene prediction using the psRNATarget website.6 Combined with our RNA sequencing data, we retained 273 overlapping genes (Figure 4A). To better understand the functions of these overlapping genes, Gene Ontology (GO) analysis was performed for candidate target genes using the CottonFGD database, which provides annotation information for the “biological process,” “molecular function,” and “cellular component” GO categories (see footnote 4). As shown in Figure 4B, the “DNA binding” GO term was over-represented in the “molecular function” category. Consistently, genes in the GO term “nucleuses” were over-represented in the “cellular component” category. “DNA binding function” with 62 genes was predominant in the main “molecular function” category. The GO terms “response to hormone” and “sodium ion transport” represented major terms in the “biological process” category. This further suggests that miRNA target genes functioned in transcription and in response to hormones in cotton.
Figure 4. Overview of predicted target miRNA genes. (A) Venn diagram showing the overlapping genes between the total transcripts and the predicted target genes. (B) Histogram of gene ontology annotation in the three major gene ontology (GO) categories: black, “molecular function”; orange, “cellular component”; and blue, “biological process.” (C) Distribution of transcription factor (TF) families in the overlapping genes. (D) Distribution of up- and downregulated TF family genes.
Furthermore, how transcription factors (TFs) influence cotton flowering time, we performed functional analysis of predicted transcription factor genes. The results showed that 66 TF genes were differentially expressed, with 43 upregulated and 23 downregulated in the EFCs. Among (66) TF genes, bHLH (2), ERF (2), C3H (1), and MADS (1) TF genes were significantly enriched in the upregulated DEG group, while LSD (1) TF genes were enriched in the downregulated overlapping group. Except TF families mentioned above, genes encoding SBP-, MYB-, and ARF-type TFs showed both up- and downregulated expression profiles (Figures 4C,D). Interestingly, 30 (45%) of the genes encoding SPL family TFs were significantly upregulated in the EFCs, such as GH_D01G054600 (GhSPL4), GH_A03G089200 (GhSPL6), GH_D11G041400 (GhSPL13B), GH_A10G019300 (GhSBP1), and GH_A01G147900 (GhSPL17); the homologs of these gene in Arabidopsis are targeted by miR156 (Supplementary Table S4). To validate the expression levels in different cotton lines, qRT-PCR assays were performed for the six SPL TF genes which were upregulated in EFC compared with the LFCs, while miR156 expressed the downregulation in EFC (Figure 5A). To further characterize the miR156 and SPL4 expression level during cotton flowering, we analyzed the time-course expression from 14 to 42 days. As shown in Figures 5B,C, the relative expression level of miR156 in 28 days of CZ-3 was 1, while that of 48xi was 6-fold. At the same time, the expression level of SPL4 gene in SAM of CZ-3 was upregulated by 32.5 times from 35 to 42 days after sowing, while the expression of SPL4 in 48xi was only upregulated by 1 time during the same period.
Figure 5. Expression analysis of selected SPL and miR156. (A) Quantitative real-time PCR verification of miR156 target gene expression at the fifth true leaf stage in the cotton shoot apex. Values are the means ± SD (n = 3). (B,C) qRT-PCR analysis of miR156 and SPL4 in CZ-3 and 48xi.
To confirm the function of the miRNA target genes involved in cotton flowering regulation, we further conducted RNA sequencing (RNA-seq) analysis to identify the gene expression levels using apical meristems from the EFCs and LFCs. The correlation assay of DEGs between two biological process was strong positive correlation (R2 > 0.9945) in four varieties (Supplementary Figure S3). A total of 1,008 differentially expressed genes (DEGs; |log2FC| > 1 and value of p < 0.05) were identified, where 633 and 375 genes were significantly up- and downregulated in the ELCs, respectively (Figure 6A). Enrichment analysis of all the DEGs identified the significant GO function in “response to auxin” and “cytokinin metabolic process” (Figure 6B). Further analysis showed that the DEGs were also enriched in the GO terms “iron ion binding,” “ADP binding,” and “heme binding.” The 24 genes associated with “iron ion binding” included 15 cytochrome-related genes, 16 genes encoding ADP binding proteins, and 23 genes encoding heme binding proteins. These results exhibited that iron and heme binding activity play a pivotal role in the cotton flowering transition. Except for the above regulation, 46 and 27 genes were associated with oxidoreductase activity and protein dimerization activity, respectively. These results indicated that iron ion, heme, and ADP also function in the control of cotton flowering time.
Figure 6. Overview of significantly expressed genes in the fifth true leaf in cotton. (A) Changes in gene expression profile between early flowering varieties and later flowering varieties. DEGs, differentially expressed genes. (B) Histogram showing GO annotation of the DEGs. URGs, upregulated genes and DRGs, downregulated genes.
The phytohormones such as auxin, cytokinin, and gibberellin involve in control of flowering time. The expression of 12 genes was significantly upregulated in the EFCs, and six auxin pathway genes were significantly downregulated in the LFCs. These include seven small auxin upregulated RNA (SAUR) members, four auxin-responsive Gretchen Hagen 3 (GH3) members, three auxin/indole-3-acetic acid (Aux/IAA) protein family members, and three auxin-binding protein (ABP) members. Notably, four cytokinin dehydrogenase (CKX) family genes were significantly downregulated in the EFCs. In addition to auxin and cytokinin, gibberellin also functions in cotton flowering time control. Two gibberellin 2-beta-dioxygenase (GA2OX) family genes, two GhGAI and one gibberellin oxidase showed significant expression in the EFCs (Figure 7A). These results indicated that auxin, cytokinin, and gibberellin function in cotton flowering time control, and the functions of auxin and cytokinin might be diverse. We confirmed the expression level differences between EFCs and LFCs by qRT-PCR and consistent with the result of RNA-seq (Figure 7B). In further study of how flowering marker genes are differentially expressed in the fifth true leaf, 14 genes that participate in cotton flowering time control including GhGI, GhAGL5, GhAGL6, GhGAI, GhAP1, GhAGL16, GhSOC1, GhCOL3, and GhCAL. GhAGL5, GhAGL6, GhAP1, GhSOC1, GhCOL3, GhCAL, and GhMADS6 showed higher expression levels in the EFCs, which is consistent with previous research. Among these genes, GhSOC1 and GhCAL both showed two-fold increase in expression in the EFCs. Most strikingly, expression of GhMADS6, GhAP1, GhAGL5, and GhAGL6 increased six-, six-, 10-, and 12-fold in the EFCs, respectively, although GhGAI was downregulated by 21-fold compared with the LFCs. These results imply that GhAGL6, GhAP1, GhAGL16, GhSOC1, GhCOL3, and GhCAL may be potential key regulators of flowering time in cotton.
Figure 7. Expression analyses of hormone-related gene in DEG. (A) Expression heat map of hormone-related genes. (B) Quantitative real-time PCR verification of the expression of eight hormone-related genes in the four cotton varieties at the fifth true leaf stage in the shoot apex. Values are means ± SD (n = 3).
To confirm whether the differentially expressed miRNAs were involved in regulating flowering time, we used the virus-based miRNA silencing (VbMS) strategy to generate Gh-miR156 silenced and overexpressed plants. The OE-miR156 and STTM-miRNA vectors were constructed, containing the fragment of pre-miR156 and a small tandem target mimic (STTM) sequence including two imperfect binding sites separated by a 48 bp spacer, respectively. The positive control, G. hirsutum magnesium chelatase subunit I (CHLI) gene was well-silenced, resulting in a photobleaching phenotype in the third true leaf (Figure 8A). Compared with the control plants inoculated with the empty vector (CLCrV) in squaring stage, the silenced plants (STTM-miR156) begun to flower, while the overexpressed ones (OE-miR156) were still in vegetative stage at same time (Figure 8B). The squaring time of CLCrV, STTM-miR156, and OE-miR156 plants was 36, 30.5, and 40.3 DAG (Figure 8C), and the node positions of first fruit branch were 5, 4, and 6 (Figure 8D). These results suggested that Gh-miR156 participated regulating flowering time in cotton.
Figure 8. The GhmiR156 negatively regulated plant flowering. (A) Photobleaching phenotype in CHLI silenced plant. (B) Phenotype of GhmiR156 overexpressed and silenced cotton. (C) Time of squaring described by days after germination (DAG) in overexpressed and silenced plants. (D) Sketch map for node position of first fruit branch in overexpressed and silenced plants.
To determine whether miR399e mediated cotton flowering as well, we performed expression analyses in four cultivars by qRT-PCR. Compared with early flowering cultivars, the expression levels of miR399e of two later flowering cultivars were increased 18.6-fold and the target gene GhUBC24 reduced expression for 2.36-fold in average. We further introduced miR399e into Arabidopsis wild type (WT). The OE-miR399e plants showed the phenotype of delaying flowering compared to WT (Supplementary Figure S4). These results indicated miR399e negatively regulated flowering in cotton.
To further describe the miRNA-mediated flowering time regulatory network in cotton, we performed the weighted correlation network analysis (WGCNA) using whole-genome transcriptome data. We identified three modules (blue, turquoise, and brown) that consisted of 920 DEGs which are potentially regulated by SPL TFs (Supplementary Figure S5). The blue module contained 107 nodes and 968 edges, and GhCAL and GhSAUR36 were included in this module. GO analysis revealed that 4 (100%), 4 (80%), and 5 (83.3%) DEGs enriched in “heme binding,” “calcium ion binding,” and “iron ion binding” in the “molecular function” category, respectively, were significantly upregulated in the LFCs compared with the EFCs (Supplementary Figure S6). The brown module contained 61 nodes and 1,449 edges. Three, four, and eight of DEGs were enriched in the “flavin adenine dinucleotide binding,” “oxidoreductase activity,” and “catalytic activity” terms, respectively (Supplementary Figure S6). It is notable that the turquoise module contains 750 nodes and 75,160 edges (Supplementary Table S5). Enrichment analysis of all the DEGs identified 16 “molecular function” and seven “biological process” terms in the turquoise module. Noticeably, 45 of the DEGs were enriched in the “regulation of transcription” biological process term, and 12 DEGs were associated with flowering time pathways (Supplementary Figure S6). These include GhAP1C, GhAGL6, GhSOC1, GhMADS6, GhAP1, GhFD, GhAGL16, and GhCAL (Figure 9). In addition, four genes associated with auxin signal transduction were identified in the “biological process” category, including GhIAA14, GhIAA27, GhIAA29, and GhAUX22D. We also found that “response to auxin process” was enriched in the “biological process” category, and four (66.67%) small auxin upregulated RNA (SAUR) genes were upregulated in the EFCs, including SAUR24, SAUR32, SAUR50, and SAUR72. These results strongly suggest that auxin and conserved flowering marker genes regulated flowering time in cotton.
Figure 9. WGCNA analysis of the hub genes in the turquoise module. Red, flowering-related genes and purple, hormone-related genes.
MiRNAs, a class of small RNA molecules, have emerged as important regulators of various cellular processes through their interactions with their targets at the transcriptional and post-transcriptional levels (Song et al., 2019). However, the molecular mechanisms involved in the regulation of flowering time in cotton remain unclear. In this study, to better explore the roles of miRNA in flowering time control, we combined sRNA and transcriptome sequencing to identify the relationships between miRNAs and their target genes. In the upland cotton genome, genome-wide bioinformatics analysis predicted 315 miRNAs.1 In this study from sequencing analysis of sRNA libraries in four cotton varieties, a total of 186.8 million clean reads included 168 conserved miRNA families and 351 novel miRNAs. We identified 79 conserved miRNAs and 20 novel miRNAs that showed the significant differences in expression (Supplementary Table S3). The sRNA lengths ranged from 18 to 25 nt, and among these, the 24-nt sRNAs were the most abundant in the sequencing libraries, which was similar to previous observations in cotton miRNA sequencing (Li et al., 2012; Hu et al., 2020). It has been reported that many of the 24-nt sRNAs are heterochromatic siRNAs (hetsiRNAs) which mediate transcriptional gene silencing through DNA methylation (RdDM; Lu et al., 2005). Previous studies have shown that flowering-related SNPs are located on the cotton D subgenome; consistent with this notion, 61.62% of miRNAs that showed significant differential expression are located on the D-subgenome (Figure 2D; Ma et al., 2018). However, further studies will be required to verify the relationship between these miRNAs and flowering time.
Among identified miRNAs, more than 10 highly conserved miRNA were identified including miR156, miR159, miR319, miR399, miR7489, miR6300, miR7492, miR8674, miR8771, and miR8780, which is in line with previous study on the development of cotton seeds and fruiting branches (Wang et al., 2015; Sun et al., 2016). Moreover, miR156 was significantly expressed in sRNA sequencing data (Supplementary Table S3). Many studies showed that miR156 expression switches on in the vegetative stage in crops (Chen et al., 2014; An et al., 2015; Aung et al., 2015; Sun et al., 2015; Jia et al., 2017; Zhou et al., 2021). Other studies also stated that miR156 represses the expression of SPL that negatively mediate flowering time (Wang et al., 2009; Wu et al., 2009). It is notable that 9 of 111 miRNAs belong to the miR399 family (Supplementary Table S3), suggesting that there might be additional players in the regulatory pathways. It has been reported that miR399 acts to promote flowering by targeting PHO2 in response to ambient temperature changes (Kim et al., 2011). It also demonstrated that PHO2 and GI interact to mediate flowering time and phosphate homeostasis in rice (Li et al., 2017). Therefore, it is possible that miR399 may also regulate PHO2 to influence flowering time in cotton.
Identification of miRNA targets is important to understand miRNA-mediated processes. Bioinformatics analysis revealed 273 target genes including 30 SBP TF genes, of which 21 were upregulated and nine were downregulated in the early flowering varieties (Figures 4A–D). This result suggests that most of the SBP TFs positively regulated flowering progress in cotton. The qRT-PCR results also confirm that GhPSPL4, GhSBP1, GhSPL6, and GhSPL13B showed the reverse expression trends compared with miR156 (Figure 5). This result suggests that miR156 potentially repressed SPL gene expression in the timing of cotton flowering. A previous study showed that SPL functions as a core regulator of flowering time that positively mediates flowering in Arabidopsis through actions of the SPL-SOC1 module (Jung et al., 2012). Further analysis showed that SPL TFs directly bind to the promoters of FUL, LFY, and AP1, key genes that are active in regulating flowering (Xie et al., 2020). It is notable that except for the SBP-type TFs, MYB-, MIKC_MADS-, and ARF-type TF genes were also enriched in the 273 target genes identified (Figures 4C,D). Previous studies have shown that these types of TFs play an important role in floral organ specificity and the auxin response (Smaczniak et al., 2012; Korasick et al., 2014). This indicated that floral organs and auxin might influence cotton flowering time.
In addition, bioinformatics analysis also identified 920 DEGs with SPL binding sites (GTAC motifs) in their promoters including GhAP1, GhSOC1, GhAGL6, GhCOL3, and GhFD. WGCNA exhibited that GhSOC1, GhAGL16, GhCOL3, GhAGL6, GhAP1, GhCAL, and GhFD were enriched in the turquoise module (Supplementary Table S5). It has been reported that AGL6, SOC1, FD, and COL3 regulate flowering time in Arabidopsis thaliana (Moon et al., 2003; Abe et al., 2005; Li et al., 2008; Yoo et al., 2011; Tripathi et al., 2017; Cheng et al., 2020). The authors also stated the important role of GhCAL, GhAP1, and GhSOC1 in mediating cotton flowering (Zhang et al., 2016; Cheng et al., 2020). Hormone actions are intertwined in regulation of various plant growth and developmental processes, and auxin and gibberellin are the most well-studied phytohormones that are necessary for a variety of developmental activities, including flowering (Cheng et al., 2007). Previous studies showed that auxin directly regulates the expression of genes encoding GA metabolic enzymes (O'Neill and Ross, 2002; Frigerio et al., 2006). In addition, auxin affects the stability of DELLA proteins (Fu and Harberd, 2003). Thus, it is expected that the auxin and gibberellin pathways might be important role in regulating flowering time. Consistent with this opinion, GhSAUR, GhIAA, GhGH3, and GhGAI genes showed significant differential expression in our study (Figure 7B).
Elucidating the flowering time-associated molecular mechanism becomes the critical biological question that is not only for agricultural significance in shaping juvenile-to-adult transition, but also has significance due to increasing world population and extreme weather events (Xie et al., 2020). Experiments have shown that flowering time in cotton is related to the activities of auxin and gibberellins. Because of its significant influence on plant growth and development, premature flowering can decrease agricultural yield and biomass in major crops and an increase in the length of vegetative phase that could lead to reduce the seed set (Teotia and Tang, 2015). Understanding of molecular mechanism in controlling flowering pathway could have potential applications in many economically important crops.
The datasets presented in this study can be found in online repositories. The names of the repository/repositories and accession number(s) can be found at: http://www.ncbi.nlm.nih.gov/ PRJNA785082.
YZ, YWa, CL, and ZM designed the research. YZ and AM performed the research. YZ, SG, GS, and YWe analyzed the data. CL and RZ wrote the manuscript. All authors contributed to the article and approved the submitted version.
This research was funded by the National Natural Science Foundation of China (31771850).
The authors declare that the research was conducted in the absence of any commercial or financial relationships that could be construed as a potential conflict of interest.
All claims expressed in this article are solely those of the authors and do not necessarily represent those of their affiliated organizations, or those of the publisher, the editors and the reviewers. Any product that may be evaluated in this article, or claim that may be made by its manufacturer, is not guaranteed or endorsed by the publisher.
The Supplementary Material for this article can be found online at: https://www.frontiersin.org/articles/10.3389/fpls.2022.761244/full#supplementary-material
1. ^http://www.plantsrnas.org/
2. ^http://omicslab.genetics.ac.cn/psRobot/
3. ^http://www.bioconductor.org/packages/release/bioc/html/DESeq2.html
5. ^https://horvath.genetics.ucla.edu/html/CoexpressionNetwork/Rpackages/WGCNA/Tutorials/
Abe, M., Kobayashi, Y., Yamamoto, S., Daimon, Y., Yamaguchi, A., Ikeda, Y., et al. (2005). FD, a bZIP protein mediating signals from the floral pathway integrator FT at the shoot apex. Science 309, 1052–1056. doi: 10.1126/science.1115983
Achard, P., Gusti, A., Cheminant, S., Alioua, M., Dhondt, S., Coppens, F., et al. (2009). Gibberellin signaling controls cell proliferation rate in Arabidopsis. Curr. Biol. 19, 1188–1193. doi: 10.1016/j.cub.2009.05.059
An, Y., Guo, Y., Liu, C., and An, H. (2015). BdVIL4 regulates flowering time and branching through repressing miR156 in ambient temperature dependent way in Brachypodium distachyon. Plant Physiol. Biochem. 89, 92–99. doi: 10.1016/j.plaphy.2015.02.013
Arora, S., and Chaudhary, B. (2021). Global expression dynamics and miRNA evolution profile govern floral/fiber architecture in the modern cotton (Gossypium). Planta 254:62. doi: 10.1007/s00425-021-03711-3
Aung, B., Gruber, M. Y., Amyot, L., Omari, K., Bertrand, A., and Hannoufa, A. (2015). Ectopic expression of LjmiR156 delays flowering, enhances shoot branching, and improves forage quality in alfalfa. Plant Biotechnol. Rep. 9, 379–393. doi: 10.1007/s11816-015-0375-2
Bent, A. (2006). “Arabidopsis thaliana floral dip transformation method,” in Agrobacterium Protocols. ed. K. Wang (Totowa, NJ: Humana Press), 87–104.
Chen, Z., Li, F., Yang, S., Dong, Y., Yuan, Q., Wang, F., et al. (2014). Identification and functional analysis of flowering related microRNAs in common wild Rice (Oryza rufipogon Griff.). PLoS One 8:e82844. doi: 10.1371/journal.pone.0082844
Cheng, S., Chen, P., Su, Z., Ma, L., Hao, P., Zhang, J., et al. (2020). High-resolution temporal dynamic transcriptome landscape reveals a GhCAL-mediated flowering regulatory pathway in cotton (Gossypium hirsutum L.). Plant J. 19, 153–166. doi: 10.1111/pbi.13449
Cheng, Y., Dai, X., and Zhao, Y. (2007). Auxin synthesized by the YUCCA flavin monooxygenases is essential for embryogenesis and leaf formation in Arabidopsis. Plant Cell 19, 2430–2439. doi: 10.1105/tpc.107.053009
Eamens, A. L., Smith, N. A., Curtin, S. J., Wang, M.-B., and Waterhouse, P. M. (2009). The Arabidopsis thaliana double-stranded RNA binding protein DRB1 directs guide strand selection from microRNA duplexes. RNA 15, 2219–2235. doi: 10.1261/rna.1646909
Fei, Q., Xia, R., and Meyers, B. C. (2013). Phased, secondary, small interfering RNAs in posttranscriptional regulatory networks. Plant Cell 25, 2400–2415. doi: 10.1105/tpc.113.114652
Frigerio, M., Alabadí, D., Pérez-Gómez, J., García-Cárcel, L., Phillips, A. L., Hedden, P., et al. (2006). Transcriptional regulation of gibberellin metabolism genes by auxin signaling in Arabidopsis. Plant Physiol. 142, 553–563. doi: 10.1104/pp.106.084871
Fu, X., and Harberd, N. P. (2003). Auxin promotes Arabidopsis root growth by modulating gibberellin response. Nature 421, 740–743. doi: 10.1038/nature01387
Gocal, G. F. W., Sheldon, C. C., Gubler, F., Moritz, T., Bagnall, D. J., MacMillan, C. P., et al. (2001). GAMYB-like genes, flowering, and gibberellin signaling in Arabidopsis. Plant Physiol. 127, 1682–1693. doi: 10.1104/pp.010442
Gu, Z., Huang, C., Li, F., and Zhou, X. (2014). A versatile system for functional analysis of genes and microRNAs in cotton. Plant Biotechnol. J. 12, 638–649. doi: 10.1111/pbi.12169
Guo, D., Li, C., Dong, R., Li, X., Xiao, X., and Huang, X. (2015). Molecular cloning and functional analysis of the flowering locus T (FT) homolog GhFT1 from Gossypium hirsutum. J. Integr. Plant Biol. 57, 522–533. doi: 10.1111/jipb.12316
Hu, G., Lei, Y., Liu, J., Hao, M., Zhang, Z., Tang, Y., et al. (2020). The ghr-miR164 and GhNAC100 modulate cotton plant resistance against Verticillium dahlia. Plant Sci. 293:110438. doi: 10.1016/j.plantsci.2020.110438
Itoh, H., Nonoue, Y., Yano, M., and Izawa, T. (2010). A pair of floral regulators sets critical day length for Hd3a florigen expression in Rice. Nat. Genet. 42, 635–638. doi: 10.1038/ng.606
Iwakawa, H.-o., and Tomari, Y. (2013). Molecular insights into microRNA-mediated translational repression in plants. Mol. Cell 52, 591–601. doi: 10.1016/j.molcel.2013.10.033
Jia, X. L., Chen, Y. K., Xu, X. Z., Shen, F., Zheng, Q. B., Du, Z., et al. (2017). miR156 switches on vegetative phase change under the regulation of redox signals in apple seedlings. Sci. Rep. 7:14223. doi: 10.1038/s41598-017-14671-8
Jung, J.-H., Ju, Y., Seo, P. J., Lee, J.-H., and Park, C.-M. (2012). The SOC1-SPL module integrates photoperiod and gibberellic acid signals to control flowering time in Arabidopsis. Plant J. 69, 577–588. doi: 10.1111/j.1365-313X.2011.04813.x
Kim, W., Ahn, H. J., Chiou, T.-J., and Ahn, J. H. (2011). The role of the miR399-PHO2 module in the regulation of flowering time in response to different ambient temperatures in Arabidopsis thaliana. Mol. Cell 32, 83–88. doi: 10.1007/s10059-011-1043-1
Korasick, D. A., Westfall, C. S., Lee, S. G., Nanao, M. H., Dumas, R., Hagen, G., et al. (2014). Molecular basis for AUXIN RESPONSE FACTOR protein interaction and the control of auxin response repression. Proc. Natl. Acad. Sci. 111, 5427–5432. doi: 10.1073/pnas.1400074111
Lauter, N., Kampani, A., Carlson, S., Goebel, M., and Moose, S. P. (2005). microRNA172 down-regulates glossy15 to promote vegetative phase change in maize. Proc. Natl. Acad. Sci. U. S. A. 102, 9412–9417. doi: 10.1073/pnas.0503927102
Li, Q., Jin, X., and Zhu, Y.-X. (2012). Identification and analyses of miRNA genes in allotetraploid Gossypium hirsutum fiber cells based on the sequenced diploid G. raimondii genome. J. Genet. Genom. 39, 351–360. doi: 10.1016/j.jgg.2012.04.008
Li, D., Liu, C., Shen, L., Wu, Y., Chen, H., Robertson, M., et al. (2008). A repressor complex governs the integration of flowering signals in Arabidopsis. Dev. Cell 15, 110–120. doi: 10.1016/j.devcel.2008.05.002
Li, S., Ying, Y., Secco, D., Wang, C., Narsai, R., Whelan, J., et al. (2017). Molecular interaction between PHO2 and GIGANTEA reveals a new crosstalk between flowering time and phosphate homeostasis in Oryza sativa. Plant Cell Environ. 40, 1487–1499. doi: 10.1111/pce.12945
Li, L., Zhang, C., Huang, J., Liu, Q., Wei, H., Wang, H., et al. (2021). Genomic analyses reveal the genetic basis of early maturity and identification of loci and candidate genes in upland cotton (Gossypium hirsutum L.). Plant J. 19, 109–123. doi: 10.1111/pbi.13446
Liu, J., Cheng, X., Liu, P., Li, D., Chen, T., Gu, X., et al. (2017). MicroRNA319-regulated TCPs interact with FBHs and PFT1 to activate CO transcription and control flowering time in Arabidopsis. PLoS Genet. 13:e1006833. doi: 10.1371/journal.pgen.1006833
Lu, C., Tej, S. S., Luo, S., Haudenschild, C. D., Meyers, B. C., and Green, P. J. (2005). Elucidation of the small RNA component of the transcriptome. Science 309, 1567–1569. doi: 10.1126/science.1114112
Ma, Z., He, S., Wang, X., Sun, J., Zhang, Y., Zhang, G., et al. (2018). Resequencing a core collection of upland cotton identifies genomic variation and loci influencing fiber quality and yield. Nat. Genet. 50, 803–813. doi: 10.1038/s41588-018-0119-7
Mathieu, J., Yant, L. J., Mürdter, F., Küttner, F., and Schmid, M. (2009). Repression of flowering by the miR172 target SMZ. PLoS Biol. 7:e1000148. doi: 10.1371/journal.pbio.1000148
Moon, J., Suh, S.-S., Lee, H., Choi, K.-R., Hong, C. B., Paek, N.-C., et al. (2003). The SOC1 MADS-box gene integrates vernalization and gibberellin signals for flowering in Arabidopsis. Plant J. 35, 613–623. doi: 10.1046/j.1365-313X.2003.01833.x
O'Neill, D. P., and Ross, J. J. (2002). Auxin regulation of the gibberellin pathway in pea. Plant Physiol. 130, 1974–1982. doi: 10.1104/pp.010587
Paterson, A. H., Wendel, J. F., Gundlach, H., Guo, H., Jenkins, J., Jin, D., et al. (2012). Repeated polyploidization of Gossypium genomes and the evolution of spinnable cotton fibres. Nature 492, 423–427. doi: 10.1038/nature11798
Purugganan, M. D., and Fuller, D. Q. (2009). The nature of selection during plant domestication. Nature 457, 843–848. doi: 10.1038/nature07895
Ren, G., Xie, M., Zhang, S., Vinovskis, C., Chen, X., and Yu, B. (2014). Methylation protects microRNAs from an AGO1-associated activity that uridylates 5′ RNA fragments generated by AGO1 cleavage. Proc. Natl. Acad. Sci. 111, 6365–6370. doi: 10.1073/pnas.1405083111
Smaczniak, C., Immink, R. G. H., Muiño, J. M., Blanvillain, R., Busscher, M., Busscher-Lange, J., et al. (2012). Characterization of MADS-domain transcription factor complexes in Arabidopsis flower development. Proc. Natl. Acad. Sci. 109, 1560–1565. doi: 10.1073/pnas.1112871109
Song, X., Li, Y., Cao, X., and Qi, Y. (2019). MicroRNAs and their regulatory roles in plant–environment interactions. Annu. Rev. Plant Biol. 70, 489–525. doi: 10.1146/annurev-arplant-050718-100334
Sun, Q., Du, X., Cai, C., Long, L., Zhang, S., Qiao, P., et al. (2016). To be a flower or fruiting branch: insights revealed by mRNA and small RNA transcriptomes from different cotton developmental stages. Sci. Rep. 6:23212. doi: 10.1038/srep23212
Sun, R., Guo, T., Cobb, J., Wang, Q., and Zhang, B. (2015). Role of microRNAs during flower and storage root development in sweet potato. Plant Mol. Biol. Report. 33, 1731–1739. doi: 10.1007/s11105-015-0869-7
Sun, Q., Xie, Y., Li, H., Liu, J., Geng, R., Wang, P., et al. (2021). Cotton GhBRC1 regulates branching, flowering, and growth by integrating multiple hormone pathways. Crop J. 10, 75–87. doi: 10.1016/j.cj.2021.01.007
Teotia, S., and Tang, G. (2015). To bloom or not to bloom: role of microRNAs in plant flowering. Mol. Plant 8, 359–377. doi: 10.1016/j.molp.2014.12.018
Tripathi, P., Carvallo, M., Hamilton, E. E., Preuss, S., and Kay, S. A. (2017). Arabidopsis B-BOX32 interacts with CONSTANS-LIKE3 to regulate flowering. Proc. Natl. Acad. Sci. 114, 172–177. doi: 10.1073/pnas.1616459114
Wang, J.-W., Czech, B., and Weigel, D. (2009). miR156-regulated SPL transcription factors define an endogenous flowering pathway in Arabidopsis thaliana. Cell 138, 738–749. doi: 10.1016/j.cell.2009.06.014
Wang, Y., Ding, Y., Yu, D., Xue, W., and Liu, J. (2015). High-throughput sequencing-based genome-wide identification of microRNAs expressed in developing cotton seeds. Sci. China Life Sci. 58, 778–786. doi: 10.1007/s11427-015-4877-5
Wang, H., Pan, J., Li, Y., Lou, D., Hu, Y., and Yu, D. (2016). The DELLA-CONSTANS transcription factor cascade integrates gibberellic acid and photoperiod signaling to regulate flowering. Plant Physiol. 172, 479–488. doi: 10.1104/pp.16.00891
Wu, L., Liu, D., Wu, J., Zhang, R., Qin, Z., Liu, D., et al. (2013). Regulation of FLOWERING LOCUS T by a microRNA in Brachypodium distachyon. Plant Cell 25, 4363–4377. doi: 10.1105/tpc.113.118620
Wu, G., Park, M. Y., Conway, S. R., Wang, J.-W., Weigel, D., and Poethig, R. S. (2009). The sequential action of miR156 and miR172 regulates developmental timing in Arabidopsis. Cell 138, 750–759. doi: 10.1016/j.cell.2009.06.031
Xie, Y., Zhou, Q., Zhao, Y., Li, Q., Liu, Y., Ma, M., et al. (2020). FHY3 and FAR1 integrate light signal with the miR156-SPL module-mediated aging pathway to regulate Arabidopsis flowering. Mol. Plant 13, 483–498. doi: 10.1016/j.molp.2020.01.013
Xu, F., Li, T., Xu, P.-B., Li, L., Du, S.-S., Lian, H.-L., et al. (2016). DELLA proteins physically interact with CONSTANS to regulate flowering under long days in Arabidopsis. FEBS Lett. 590, 541–549. doi: 10.1002/1873-3468.12076
Yamaguchi, S. (2008). Gibberellin metabolism and its regulation. Annu. Rev. Plant Biol. 59, 225–251. doi: 10.1146/annurev.arplant.59.032607.092804
Yamaguchi, A., Wu, M.-F., Yang, L., Wu, G., Poethig, R. S., and Wagner, D. (2009). The microRNA-regulated SBP-box transcription factor SPL3 is a direct upstream activator of LEAFY, FRUITFULL, and APETALA1. Dev. Cell 17, 268–278. doi: 10.1016/j.devcel.2009.06.007
Yang, R., Li, P., Mei, H., Wang, D., Sun, J., Yang, C., et al. (2019). Fine-tuning of MiR528 accumulation modulates flowering time in rice. Mol. Plant 12, 1103–1113. doi: 10.1016/j.molp.2019.04.009
Yoo, S. K., Wu, X., Lee, J. S., and Ahn, J. H. (2011). AGAMOUS-LIKE 6 is a floral promoter that negatively regulates the FLC/MAF clade genes and positively regulates FT in Arabidopsis. Plant J. 65, 62–76. doi: 10.1111/j.1365-313X.2010.04402.x
Yu, Y., Jia, T., and Chen, X. (2017). The ‘how’ and ‘where’ of plant microRNAs. New Phytol. 216, 1002–1017. doi: 10.1111/nph.14834
Zhang, W., Fan, S., Pang, C., Wei, H., Ma, J., Song, M., et al. (2013). Molecular cloning and function analysis of two SQUAMOSA-Like MADS-Box genes from Gossypium hirsutum L. 55, 597–607. doi: 10.1111/jipb.12075,
Zhang, X., Wei, J., Fan, S., Song, M., Pang, C., Wei, H., et al. (2016). Functional characterization of GhSOC1 and GhMADS42 homologs from upland cotton (Gossypium hirsutum L.). Plant Sci. 242, 178–186. doi: 10.1016/j.plantsci.2015.05.001
Keywords: cotton, early flowering, transcriptome, miRNA, shoot apex
Citation: Zhou Y, Myat AA, Liang C, Meng Z, Guo S, Wei Y, Sun G, Wang Y and Zhang R (2022) Insights Into MicroRNA-Mediated Regulation of Flowering Time in Cotton Through Small RNA Sequencing. Front. Plant Sci. 13:761244. doi: 10.3389/fpls.2022.761244
Received: 19 August 2021; Accepted: 01 March 2022;
Published: 31 March 2022.
Edited by:
Ming Chen, Zhejiang University, ChinaReviewed by:
Maozhi Ren, Institute of Urban Agriculture (CAAS), ChinaCopyright © 2022 Zhou, Myat, Liang, Meng, Guo, Wei, Sun, Wang and Zhang. This is an open-access article distributed under the terms of the Creative Commons Attribution License (CC BY). The use, distribution or reproduction in other forums is permitted, provided the original author(s) and the copyright owner(s) are credited and that the original publication in this journal is cited, in accordance with accepted academic practice. No use, distribution or reproduction is permitted which does not comply with these terms.
*Correspondence: Yuan Wang, d2FuZ3l1YW4wN0BjYWFzLmNu; Rui Zhang, emhhbmdydWlAY2Fhcy5jbg==
†These authors have contributed equally to this work
Disclaimer: All claims expressed in this article are solely those of the authors and do not necessarily represent those of their affiliated organizations, or those of the publisher, the editors and the reviewers. Any product that may be evaluated in this article or claim that may be made by its manufacturer is not guaranteed or endorsed by the publisher.
Research integrity at Frontiers
Learn more about the work of our research integrity team to safeguard the quality of each article we publish.