- 1Fisher Delta Research, Extension, and Education Center, Division of Plant Science and Technology, University of Missouri, Portageville, MO, United States
- 2Agronomy Department, University of Florida, Gainesville, FL, United States
- 3Biological Systems Engineering, University of Wisconsin-Madison, Madison, WI, United States
- 4Division of Plant Science and Technology, University of Missouri, Columbia, MO, United States
- 5Department of Crop Sciences, University of Illinois, Urbana, IL, United States
- 6Department of Electrical Engineering and Computer Science, Bond Life Sciences Center, University of Missouri, Columbia, MO, United States
The widespread adoption of genetically modified (GM) dicamba-tolerant (DT) soybean was followed by numerous reports of off-target dicamba damage and yield losses across most soybean-producing states. In this study, a subset of the USDA Soybean Germplasm Collection consisting of 382 genetically diverse soybean accessions originating from 15 countries was used to identify genomic regions associated with soybean response to off-target dicamba exposure. Accessions were genotyped with the SoySNP50K BeadChip and visually screened for damage in environments with prolonged exposure to off-target dicamba. Two models were implemented to detect significant marker-trait associations: the Bayesian-information and Linkage-disequilibrium Iteratively Nested Keyway (BLINK) and a model that allows the inclusion of population structure in interaction with the environment (G×E) to account for variable patterns of genotype responses in different environments. Most accessions (84%) showed a moderate response, either moderately tolerant or moderately susceptible, with approximately 8% showing tolerance and susceptibility. No differences in off-target dicamba damage were observed across maturity groups and centers of origin. Both models identified significant associations in regions of chromosomes 10 and 19. The BLINK model identified additional significant marker-trait associations on chromosomes 11, 14, and 18, while the G×E model identified another significant marker-trait association on chromosome 15. The significant SNPs identified by both models are located within candidate genes possessing annotated functions involving different phases of herbicide detoxification in plants. These results entertain the possibility of developing non-GM soybean cultivars with improved tolerance to off-target dicamba exposure and potentially other synthetic auxin herbicides. Identification of genetic sources of tolerance and genomic regions conferring higher tolerance to off-target dicamba may sustain and improve the production of other non-DT herbicide soybean production systems, including the growing niche markets of organic and conventional soybean.
Introduction
Soybean [Glycine max (L.) Merr.] plays a multifaceted role in the global agricultural trade, economy, and food security due to its unique seed composition (Gale et al., 2019; Liu et al., 2020; Vieira and Chen, 2021). As a major source of protein and vegetable oil, soybean is widely used in the food, feed, and biofuel industries (Hartman et al., 2011; Vogel et al., 2021). In the United States, approximately 95% of the soybean acreage is grown with genetically-engineered herbicide-tolerant cultivars, of which nearly 55% are grown using the dicamba-tolerance trait (DT, 3,6-dichloro-2-methoxybenzoic acid) (Bayer CropScience LLC, 2021; USDA Economic Research Service, 2022). Dicamba is a synthetic auxin (Group 4 herbicide) that triggers rapid and uncontrolled growth of the stems, petioles, and leaves, often leading to plant death in sensitive dicots (Environmental Protection Agency, 2006; Grossmann, 2010). A distinguished characteristic of Group 4 herbicides is their high vapor pressure. Dicamba in specific has a vapor pressure of 2.0×10-5 mm HG at 25 C, which significantly increases the occurrence of off-target movement to adjacent fields (Behrens and Lueschen, 1979; Egan and Mortensen, 2012; Werle et al., 2018; Wechsler et al., 2019; Chism et al., 2020; Wagman et al., 2020). By comparison, glyphosate (N-(phosphonomethyl)glycine) has high water solubility and vapor pressure of 1.9×10−7 mm HG at 25°C (Battaglin et al., 2005).
The widespread adoption of DT cropping systems led to numerous cases of off-target damage to non-DT soybean as well as several other dicots plant species (Bradley, 2017; Bradley, 2018; Wechsler et al., 2019; Chism et al., 2020; Wagman et al., 2020). Between 2017 and 2021, the Environmental Protection Agency (EPA) received over 10,500 reports of dicamba-related injuries in various non-DT vegetations in 29 of the 34 states where the use of dicamba on DT crops is authorized (Echeverria, 2020; Tindall et al., 2021). Soybean is naturally sensitive to dicamba, and symptoms include crinkling and cupping of immature leaves, epinasty, plant height reduction, chlorosis, death of apical meristem, malformed pods, and ultimately yield reduction (Weidenhamer et al., 1989; Andersen et al., 2004; Grossmann, 2010; Kniss, 2018; Canella Vieira et al., 2022b). The severity of the symptoms and yield loss differ based on the timing of exposure (growth stage), dosage, frequency, and duration of exposure. It is well known that the expression of a phenotype is a function of the genotype (G), the environment (E), and the differential phenotypic response of genotypes to different environments (G×E) (de Leon et al., 2016). However, information is lacking regarding the effect of different genetic backgrounds and identification of genomic regions affecting the severity of symptoms and yield loss caused by off-target dicamba in soybean.
With the advances in the availability of high-dimensional genomic data (Song et al., 2013; Song et al., 2020) and comprehensive statistical models (Zhang et al., 2010; Liu et al., 2016; Huang et al., 2019), genome-wide association studies (GWAS) have been largely used as a common approach to help reveal the underlying genetic basis of a trait of interest during the past decade. With several thousand to millions of single nucleotide polymorphisms (SNPs), GWAS captures significant associations between the trait of interest and molecular markers using a broad range of linear or logistic regression models as well as machine and deep learning algorithms. In soybean, GWAS has unveiled the genetic architecture of multiple economic-important traits, including tolerance to biotic (Vuong et al., 2015; Chang et al., 2016; Canella Vieira et al., 2022c) and abiotic (Valliyodan et al., 2016; Zeng et al., 2017; Wu et al., 2020) stressors, seed composition (Hwang et al., 2014; Bandillo et al., 2015), agronomic (Sonah et al., 2015; Zhang et al., 2015), physiology-efficient (Lopez et al., 2022), as well as yield (Yoosefzadeh-Najafabadi et al., 2021) and domestication-related traits (Han et al., 2016; Wang et al., 2016b). To date, GWAS has not been conducted to identify genomic regions associated with soybean tolerance to dicamba or other herbicides. Therefore, the objective of this study was to identify significant marker-trait associations regulating the response of genetically diverse soybean accessions to off-target dicamba grown under prolonged exposure to dicamba under field conditions. Natural tolerance to off-target dicamba may sustain and improve the production of other non-DT herbicide soybean production systems, including the growing niche markets of organic and conventional soybean.
Materials and methods
Plant materials and data collection
A total of 382 genetically diverse soybean accessions with maturity groups (MG) ranging from MG 3 to 5 were used in this study. These comprise a subset of the USDA Soybean Germplasm Collection and originated from 15 countries, including Algeria (2), China (226), Costa Rica (1), Georgia (2), Indonesia (1), Japan (38), Nepal (1), North Korea (20), Russia (5), South Africa (1), South Korea (32), Taiwan (3), United States (40), and Vietnam (5). Five accessions have unknown origins. The USDA Soybean Germplasm Collection was genotyped with the SoySNP50K BeadChip (Song et al., 2013) and the data has been made available by the authors (Song et al., 2015) at SoyBase Genetics and Genomics Database (http://soybase.org/snps/download.php). SNPs were converted to numerical format (0, 1, and 2 for the homozygous minor allele, heterozygous, and homozygous major allele, respectively), and were excluded based on minor allele frequency (MAF)< 0.05 resulting in 31,957 SNPs. The across-genome SNP density on a chromosome basis was 1,598, ranging from 1,186 (Chr. 11) to 2,619 (Chr. 18).
Field trials were conducted in three environments for two years (2020-2021) using a two-replicate randomized complete block design at the Lee Farm in Portageville, MO (36°23’44.2”N lat; 89°36’52.3”W long) and Rhodes Farm in Clarkton, MO (36°29′14.8″N lat; 89°57′39.0″W long). Each plot consisted of a single 2.13 m long row spaced 0.76 m apart. The Lee Farm and Rhodes Farm have been exposed to prolonged and homogeneously distributed off-target dicamba damage since 2017, where significant yield losses due to off-target dicamba exposure have been reported between non-DT and DT soybean genotypes (Canella Vieira et al., 2022a; Chen et al., 2022; Canella Vieira et al, 2022b). Off-target dicamba exposure was a result of dicamba volatilization from nearby cropping systems consisting of DT soybean and cotton.
Each year, genotypes were visually assessed for off-target dicamba damage once in the early reproductive stage between R1 to R3 (approximately 100 to 130 DAP) (Fehr et al., 1971). Lines were rated on a 1 to 5 scale with 0.5 increments following the criteria described by Canella Vieira et al. (2022b). In summary, a rating of 1 showed none to minimal visual dicamba damage symptoms, including the typical crinkling and cupping of the newly-developing leaves, reduced canopy coverage, and plant stunting; a rating of 2 showed moderate tolerance with limited cupping of the newly-developing leaves and no visual impact on canopy coverage and vegetative growth; a 3 rating showed accentuated cupping of the newly-developing leaves and moderate reduction in canopy area and vegetative growth; a 4 rating showed severe cupping of the newly-developing leaves and pronounced reduction in canopy area and vegetative growth, and a rating of 5 showed extreme dicamba damage symptoms including severe cupping of the newly-developing leaves and intense reduction in canopy coverage and vegetative growth.
Adjusted means across environments were calculated using the function ‘ls_means’ of the R (R Core Team, 2022) package ‘lmerTest’ (Kuznetsova et al., 2017) based on a mixed-effects linear model conducted with the package ‘lme4’ (Bates et al., 2015). The model included the fixed effect of genotype, the random interaction between genotype and environment (G×E), the random effect of environment, and the nested random effect of replication within the environment. To measure the consistency and inter-relatedness of the damage ratings across environments, Cronbach’s alpha (α) score (Cronbach, 1951) was calculated each year using the R package ‘psych’ (Revelle, 2021) based on Eq. 1.
Where k represents the number of observations of off-target dicamba damage; is the average inter-item covariance of off-target dicamba damage scores between each pair of environments averaged for all pairs of environments; and is the average variance of off-target dicamba damage scores across all environments.
Genome-wide association study
Two models have been implemented to detect significant marker-trait associations. The Bayesian-information and Linkage-disequilibrium Iteratively Nested Keyway (BLINK) model (Huang et al., 2019) was conducted using the adjusted means across environments as phenotypic input in the R package ‘GAPIT’ (Lipka et al., 2012). It is an enhanced methodology based on the Fixed and Random Model Circulating Probability Unification (FarmCPU) (Liu et al., 2016). In summary, FarmCPU conducts two fixed-effect models iteratively and a filtering process to select a set of pseudo-SNPs that are not in linkage disequilibrium with each other as covariates. The first model tests one SNP at a time with multiple associated markers fitted as covariates to account for population stratification. The main goal is to control false positives and reduce false negatives, as well as calculate the p-values for all testing SNPs. The second model selects the covariate markers to directly control false associations instead of kinship. BLINK eliminates the requirement that genes underlying a trait are equally distributed across the genome, and also replaces the Restricted Maximum Likelihood (REML) with Bayesian Information Content (BIC) in a fixed-effect model to boost computing speed. The detailed methodology can be found in Huang et al. (2019).
To account for the variable patterns of genotype responses to off-target dicamba in different environments, a model that allows the inclusion of the population structure in interaction with environments was considered. The model was fitted with ASREML‐R (VSN‐International, England). Considering that yijk represents the kth (k=1,2) response of the ith (i=1,2,…,382) genotype in the jth (j=1,…,3) environment, the GWAS was conducted using the following linear mixed model in matrix form:
Where E corresponds to the main effect of the environments; R:E represents the effect of the replicates nested within environments; PC1:10 are the first 10 principal components (PC) derived from decomposing the G matrix using a principal component analysis (PCA) and are included in the model for correcting for population structure; the interactions between the first 10 components PC1:10 and environments were also included with the E×PCj term; the xk term corresponds to the kth molecular marker associated with βk (marker effect). All of the previous model terms were considered fixed terms. The random effect L is associated with the main effect of the genotypes, and e corresponds to the error term which captures the unexplained variability.
To control the comparison-wise error rate, the method proposed by Li and Ji (2015) was implemented. The effective number of independent tests (Meff) was derived by considering the eigenvalue decomposition of the matrix of correlations between markers. The resulting test was adjusted using the Meff with the following correction (Sidak, 1967):
Where αp is the comparison-wise error rate and αe corresponds to the experiment error-wise (αe=0.05) .
Results
Phenotypic distribution
Across the three testing environments, the frequency of off-target dicamba damage scores was consistent and normally distributed with over 45% of the observations between scores of 2 and 3 (moderately tolerant) and 39% between 3 and 3.5 (moderately susceptible) (Figure 1). Roughly 8% of the observations were either under the score of 2 (highly tolerant) or above the score of 4 (highly susceptible).
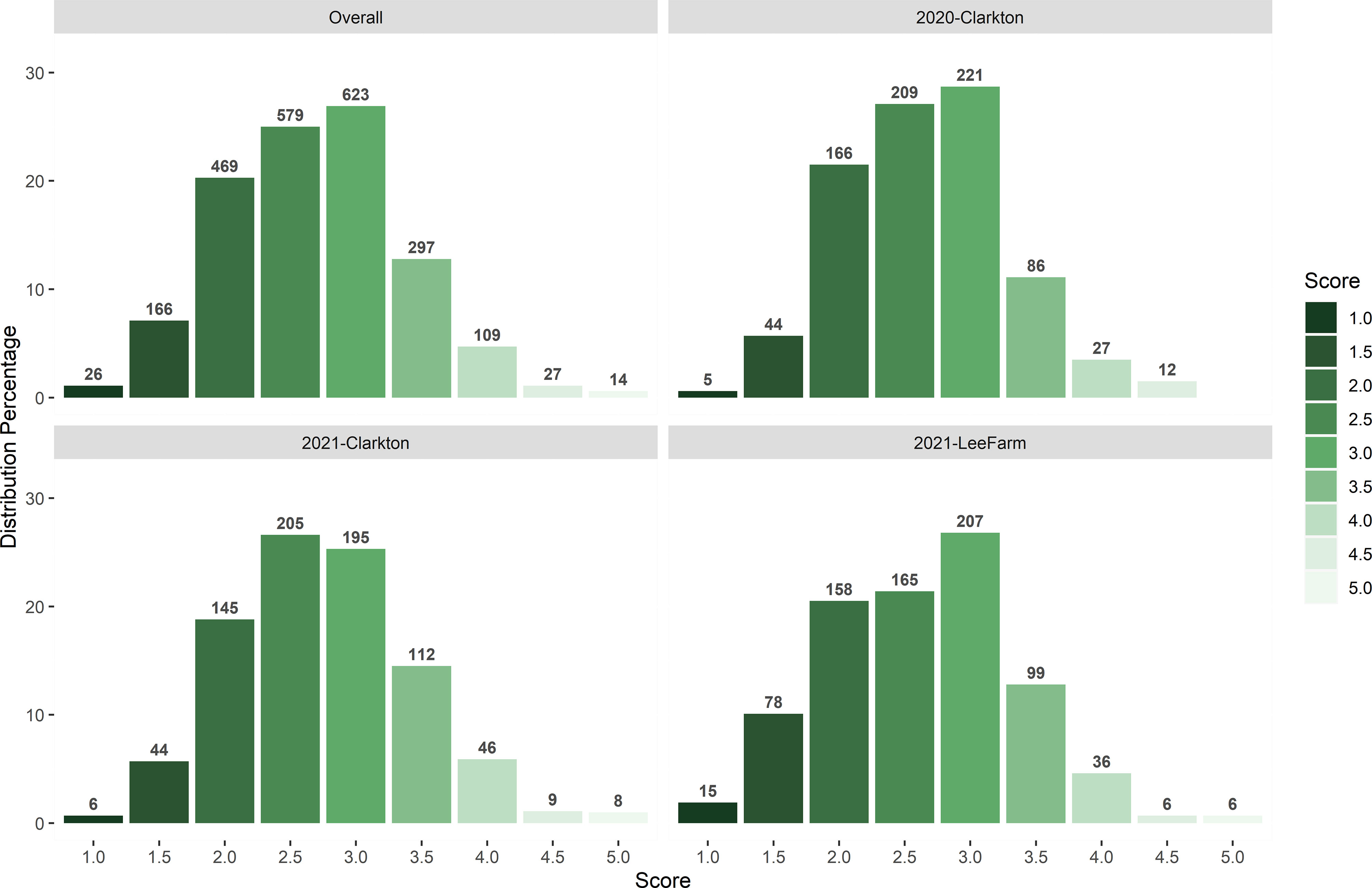
Figure 1 Distribution of off-target dicamba damage scores at each testing environment and across environments.
Across environments, scores were consistent with an overall Cronbach’s alpha (α) score of 0.89 (C.I 0.87 to 0.91). Within environments, scores ranged from 0.85 (2021-Clarkton) to 0.89 (2021-LeeFarm), indicating minor error variance and discrepancy across replications (Table 1). Cronbach’s α can be interpreted as the correlation of the test with itself, of which the error variance can be obtained by subtracting the squared α from 1.00 (Tavakol and Dennick, 2011). The error variance ranged from 0.21 to 0.26 which indicates consistency and inter-relatedness of the damage scores across and within environments. Scores above 0.70 (error variance of 0.51) are often considered acceptable (Bland and Altman, 1997; Bosma et al., 1997; McKinley et al., 1997; Kline, 1999; Amirrudin et al., 2020; Canella Vieira et al., 2022b). In plant breeding, reliability refers to multiple measurements across different environments that are independent of each other and may be explored as a new measurement of the influence of genetic versus nongenetic effects as opposed to heritability (Bernardo, 2020).
Significant marker-trait associations
The calculated effective number of independent tests (Meff) was 575, which returned a threshold of marker-trait association significance of logarithm of odds (LOD) of approximately 4.0. Using the proposed model that allows the inclusion of the population structure in interaction with environments (Eq. 2), three significant marker-trait associations were detected in chromosomes 10 (LG O), 15 (LG E), and 19 (LG L) (Figure 2). The SNP ss715622838 located at 5,457,236 bp of chromosome 15 (Genome assembly version Wm82.a2) had the highest LOD (4.5) with a favorable allele frequency of 13.9%. This SNP is located within the gene Glyma15g07710 which encodes a copper-containing oxidoreductase enzyme, tyrosinase. These copper containing enzymes can oxidize a wide range of aromatic compounds, including the oxidation of o-diphenols to their corresponding o-quinones (Bijelic et al., 2015; Sullivan, 2015; Min et al., 2019). Phase I of herbicide detoxification in plants involves oxidation by cytochrome P450s or hydrolysis by carboxylesterases (Kreuz et al., 1996; Barrett, 2000). Given the structural similarities between dicamba and o-diphenols, tyrosinase may also be involved in the hydroxylation of dicamba in soybean. The SNP ss715605561 located at 1,227,933 bp of chromosome 10 (Genome assembly version Wm82.a2) had the second-highest LOD (4.2) with a favorable allele frequency of 8.0%. It is located within the gene Glyma10g01700 which encodes a multidrug resistance protein (MRP). MRPs are essential in phase III of plant herbicide detoxification by facilitating the transport of glucose- or glutathione-herbicide conjugates into the vacuole (Riechers et al., 2010). Lastly, the SNP ss715635349 located at 44,580,800 bp of chromosome 19 (Genome assembly version Wm82.a2) had a LOD of 4.1 with a favorable allele frequency of 28.3% (Table 2). Interestingly, ss715635349 is located within the gene Glyma19g37108, a uridine diphosphate (UDP)-dependent glycosyltransferase gene. This genomic region contains additional five UDP-glycosyltransferase genes. The conjugation of Phase I-hydroxylated herbicides to endogenous sugar molecules such as glucose is catalyzed by UDP-dependent glycosyltransferases and represents an important phase II reaction of plant herbicide detoxification (Riechers et al., 2010).
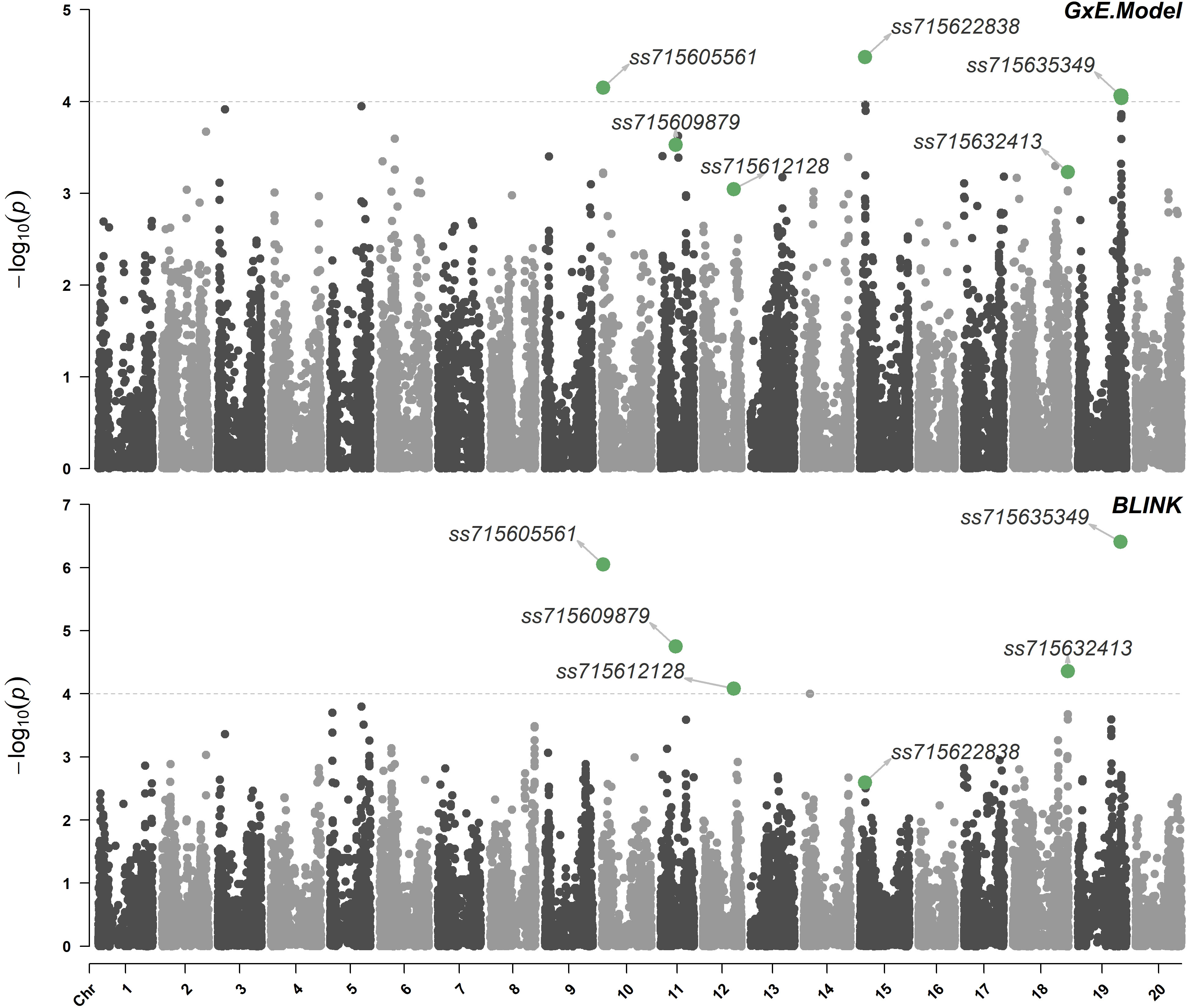
Figure 2 Manhattan plot highlighting significant marker-trait associations using the model that allows the inclusion of the population structure in interaction with environments (G×E) and BLINK. Threshold of marker-trait association significance of approximately 4.0.
Four significant marker-trait associations were detected in chromosomes 10 (LG O), 11 (LG B1), 18 (LG G), and 19 (LG L) using the BLINK model (Figure 2). The SNP ss715635349 located at 44,580,800 bp of chromosome 19 had the highest LOD (6.4) followed by the SNP ss715605561 located at 1,227,933 bp of chromosome 10 (LOD of 6.1). The SNP ss715609879 had a LOD of 4.8 with a favorable allele frequency of 24.0%. It is positioned at 15,740,804 bp of chromosome 11 (Genome assembly version Wm82.a2) and is located within Glyma11g29391, a lipid phosphate phosphatase gene. Lastly, the SNP ss715632413 located at 57,025,570 bp of chromosome 18 (Genome assembly version Wm82.a2) had a LOD of 4.4 with a favorable allele frequency of 19.4% (Table 2). Two genes with detoxification-related annotations (Glyma18g291800 and Glyma18g291700) are located within 50-kb of ss715632413 (Genome Browser, www.soybase.org).
Marker effect on observed phenotype
To assess the effect of significant SNPs on the observed phenotype, genotypes were classified according to the allelic combination of the significant SNPs ss715605561 (Chr. 10), ss715635349 (Chr. 19), and ss715632413 (Chr. 18). Favorable alleles were represented as 1 whereas unfavorable alleles were represented as 0. For instance, SNP: 0,0,0 represents the allelic combination where ss715605561, ss715635349, and ss715632413 are unfavorable, and SNP: 1,1,1 represents all favorable alleles. The mean score of dicamba damage in genotypes carrying all three favorable alleles was 1.58, whereas the mean score of genotypes carrying all three unfavorable alleles was 2.90 (Figure 3). The presence of the favorable allele of ss715605561 (SNP: 1,0,0; SNP: 1,1,0, and SNP: 1,1,1) and ss715635349 (SNP: 0,1,0; SNP: 0,1,1; SNP: 1,1,1) significantly reduced the overall damage from off-target dicamba as compared to all non-favorable alleles, while genotypes carrying only the favorable allele for ss715632413 (SNP: 0,0,1) did not show significant differences to the genotypes carrying only unfavorable alleles (Figure 3).
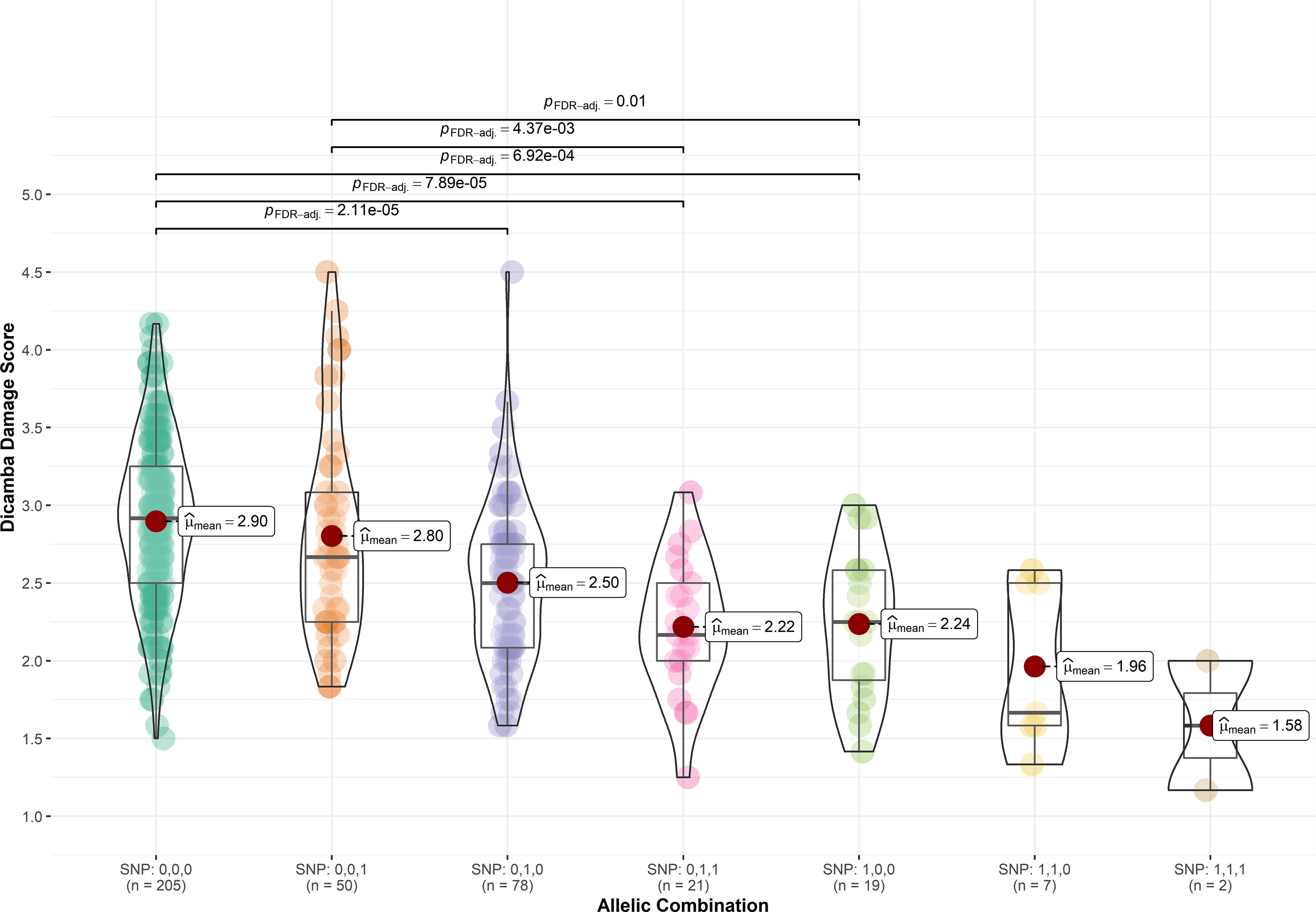
Figure 3 Distribution of off-target dicamba damage scores based on the allelic combination of SNPs ss715605561 (Chr. 10), ss715635349 (Chr. 19), and ss715632413 (Chr. 18). Favorable alleles were represented as 1 whereas unfavorable alleles were represented as 0.
In addition, to assess the potential of differentiating response classes using the significant SNPs, genotypes were classified as tolerant (score<= 2.5), moderate (2.5< score =< 3.5), and susceptible (score > 3.5). The classification distribution based on the allelic combination of these SNPs showed a substantial reduction in the susceptible class with the inclusion of the favorable alleles of ss715605561 and ss715635349 (Figure 4). On the other hand, the combination SNP: 0,0,0 had the highest concentration of susceptible (27%) and moderate (60%) genotypes and the lowest concentration of tolerant genotypes (12%) (Figure 4). Interestingly, no susceptible genotypes were observed in combinations SNP: 0,1,1, SNP: 1,0,0, SNP: 1,1,0, and SNP: 1,1,1, indicating that the selected SNPs can accurately select genotypes with higher tolerance response to off-target dicamba exposure.
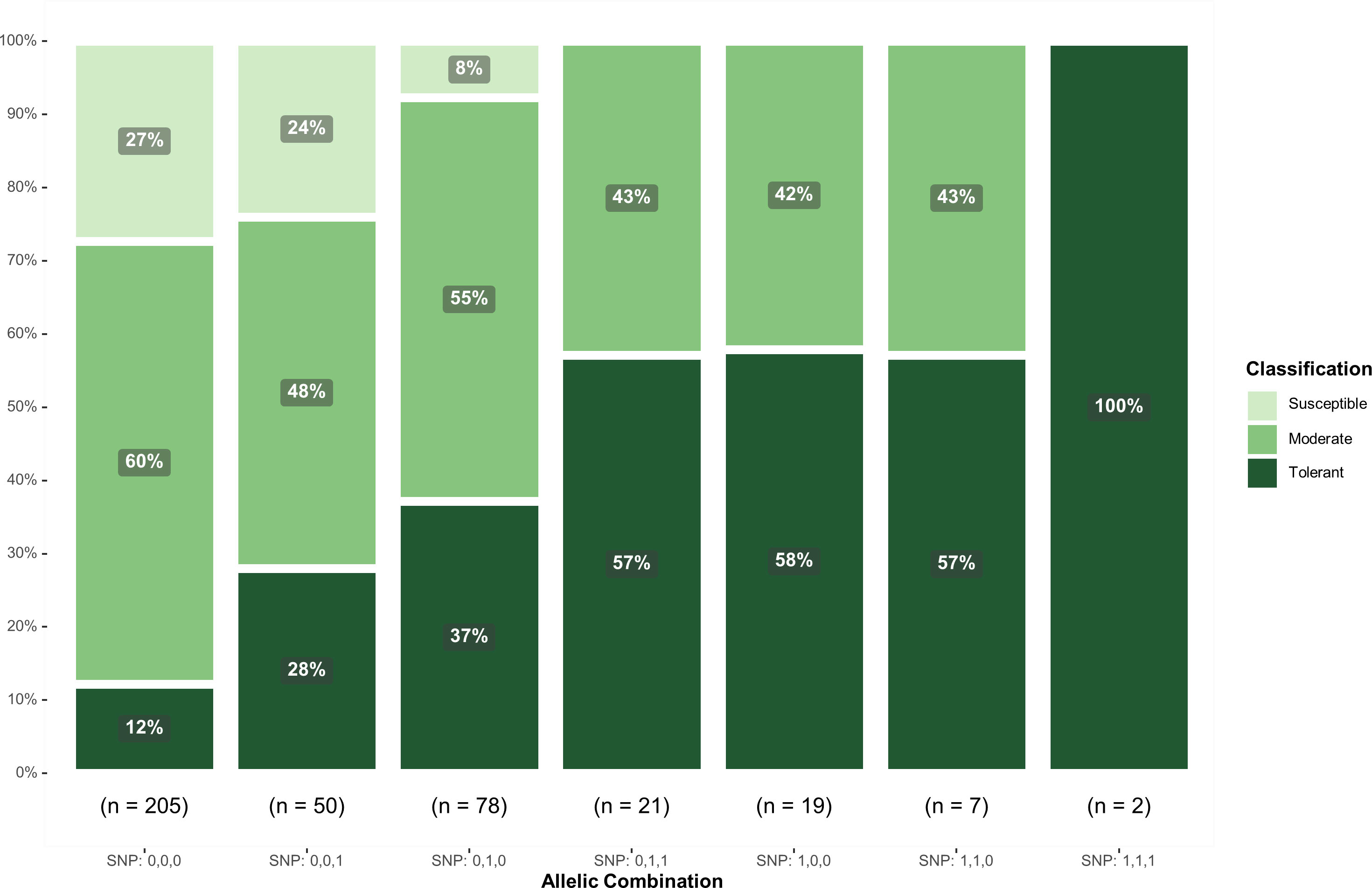
Figure 4 Classification of genotypes based on the allelic combination of SNPs ss715605561 (Chr. 10), ss715635349 (Chr. 19), and ss715632413 (Chr. 18). Favorable alleles were represented as 1 whereas unfavorable alleles were represented as 0.
Discussion
Soybean tolerant to postemergence applications of dicamba was developed under the premise of overcoming weeds resistant to glyphosate as well as allowing rotation and/or mixtures of herbicides to preserve biotechnology-based weed management strategies and maximize its efficacy (Behrens et al., 2007). The insertion of the bacterial gene dicamba monooxygenase (DMO) from Pseudomonas maltophilia (Strain DI-6) encoding the enzyme dicamba O-demethylase allows DT plants to metabolize dicamba to 3,6-dichlorosalicylic acid (DCSA), inactivating its herbicidal activity before it accumulates to toxic levels when expressed from either the nuclear genome or chloroplast genome of genetically engineered plants (Herman et al., 2005; Behrens et al., 2007; Wang et al., 2016a). In 2016, the first commercial dicamba-tolerant soybean cultivar was released in the United States and rapidly took over nearly 55% of the soybean acreage. As the incidents of off-target damage widely spread across soybean-growing states, many reports in the literature investigated the relationship between damage and potential yield losses. Soybean is two to six times more sensitive to dicamba when exposed at the early reproductive stage as compared to the vegetative stage (Kelley et al., 2005; Griffin et al., 2013; Robinson et al., 2013; Egan et al., 2014; Solomon and Bradley, 2014; Soltani et al., 2016; Kniss, 2018). Canella Vieira et al. (2022b) estimated yield losses caused by prolonged off-target dicamba exposure in 553 soybean breeding lines derived from 239 unique bi-parental populations. The study reported yield losses of 8.8% for every increment in damage score on a 1–4 scale with losses as high as 40%. Interestingly, certain genetic backgrounds consistently showed natural tolerance to off-target dicamba exposure with minimal symptoms and yield losses.
In this research, a total of 382 genetically diverse soybean accessions ranging from MG 3 to 5 were phenotypically screened based on the severity of damage across three environments subjected to prolonged off-target dicamba exposure. Although each field showed homogenous off-target dicamba distribution, it is practically impossible to assess the total dosage of dicamba received by each experimental plot in specific growth stages and throughout the season. Therefore, controlled dose-response experiments in the absence of off-target dicamba exposure should be conducted to precisely identify the threshold of which the identified genomic regions can sustain tolerance responses. Most accessions showed a moderate response, either moderately tolerant or moderately susceptible, with approximately 8% showing high tolerance (scores< 2) and susceptibility (scores > 4). No differences in off-target dicamba damage were observed across MG (Supplementary Table 1, MG 3: average damage of 2.8, MG 4: average damage of 2.7, MG 5: average damage of 2.5). Late-maturing soybean genotypes are associated with lower off-target dicamba damage due to a longer window to detoxify from low rates of dicamba between planting and flowering compared with early-maturing genotypes (Wax et al., 1969; Weidenhamer et al., 1989; McCown et al., 2018). Tolerant soybean accessions were identified across all MG, confirming that natural tolerance to off-target dicamba may be caused by physiological mechanisms other than the length of time for recovery (Canella Vieira et al., 2022b). In addition, no substantial geographical effects have been identified across continents, although accessions derived from Asia (average damage of 2.6) had on average lower off-target dicamba damage as compared to accessions derived from the Americas (average damage of 3.0). Specifically, South Korea (average damage of 2.5), Japan (average damage of 2.6), and China (average damage of 2.7) had on average lower off-target dicamba damage as compared to accessions derived from the United States (average damage of 2.3) and Costa Rica (average damage of 3.1), although the number of accessions was highly unbalanced across countries (Supplementary Table 1).
Plant introduction (PI) 424005 (average damage of 1.2, South Korea), PI 424038-B (average damage of 1.3, South Korea), PI 561701 (G88-20092, average damage of 1.4, United States), PI 603497 (average damage of 1.4, China), and PI 342434 (average damage of 1.5, Japan) were the five most tolerant accessions (Supplementary Table 1). On the other hand, PI 547862 (L83-570, average damage of 4.1, United States), PI 552538 (Dunbar, average damage of 4.2, United States), PI 598124 (Maverick, average damage of 4.3, United States), PI 603675 (average damage of 4.5, China), and PI 597387 (Pana, average damage of 4.5, United States) were the five most susceptible (Supplementary Table 1). Interestingly, four out of the five most susceptible accessions are genetically related to cultivars that widely contributed to the genetic basis of modern soybean cultivars in the United States (Gizlice et al., 1993; Gizlice et al., 1994; Carter et al., 2004; Hyten et al., 2006; Vieira and Chen, 2021). For instance, Maverick and Pana are derived from LN86-4668, which is a progeny of Fayette (PI 518674, direct progeny of PI 88788). PI 88788, which has been widely used as a genetic source of resistance to soybean cyst nematode (Heterodera glycine Ichinohe), is susceptible to off-target dicamba (average damage of 3.5). In general, soybean [Glycine max (L.) Merr.] shows a moderate response to off-target dicamba, and yield losses are expected when prolonged exposure occurs (Canella Vieira et al., 2022b). Furthermore, genetic variation conferring higher tolerance to off-target dicamba appears to be rare in landraces, highlighting the value of the USDA Soybean Germplasm Collection to restore economic-important alleles lost during domestication and intensive breeding (Gizlice et al., 1993; Gizlice et al., 1994; Carter et al., 2004; Hyten et al., 2006; Vieira and Chen, 2021). This has been the case in multiple economically important traits, including resistance to soybean cyst nematode (Anand et al., 1988; Young, 1990), root-knot nematodes (Meloidogyne spp.) (Luzzi et al., 1987), foliar feeding insects (van Duyn et al., 1971), and brow stem rot (Phialophora gregata) (Chamberlain and Bernard, 1968).
Two models were used to identify marker-trait associations regulating the response of soybean to off-target dicamba. BLINK minimizes false-positive associations and greatly improve computational efficiency in larger datasets (Huang et al., 2019). To account for possible underlying population structures affecting the observed response to off-target dicamba in different environments, a model including the first 10 principal components (PC) derived from decomposing the G matrix as well as the interaction between each PC and the environment was developed. In addition, this model allows the inclusion of all observed phenotypes (three environments × two replications per genotype) as opposed to only one adjusted mean per genotype, substantially reducing the ‘Curse of Dimensionality’ where the number of independent variables is far higher than the number of samples that are often seen in genomic studies (Nicholls et al., 2020). We observed that both models identified significant associations between ss715635349 (Chr. 19) and ss715605561 (Chr. 10) and the response to off-target dicamba. The BLINK model identified additional significant marker-trait associations on Chrs. 11 (ss715609879), 14 (ss715619759), and 18 (ss715632413), while the G×E Model identified an additional significant marker-trait association on Chr. 15 (ss715622838). The significant SNPs identified by both models are located within candidate genes with annotated functions involved with different phases of herbicide detoxification in herbicides.
Phase I typically involves oxidation by cytochrome P450s or hydrolysis by carboxylesterases. These reactions introduce a reactive functional group suitable for subsequent metabolism and detoxification since this initial oxidation step may not lead to complete detoxification (Kreuz et al., 1996; Barrett, 2000). Phase II detoxification reactions involve the conjugation of herbicides with reduced glutathione or glucose and are catalyzed by glutathione S-transferases or UDP-dependent glycosyltransferases (Riechers et al., 2010). The SNP ss715635349 is located within the gene Glyma19g37108, a uridine diphosphate (UDP)-dependent glycosyltransferase gene. Within a 30-kb window from ss715635349 (44,550,000 to 44,610,000 bp) there are another five UDP-dependent glycosyltransferases genes (Genome Browser, www.soybase.org ). Given the frequency of the favorable allele of ss715635349, we hypothesize that the ability to complete phase II detoxification of dicamba is relatively common (28.3%) and may explain the overall moderate response of soybean to off-target dicamba. Phase III of herbicide detoxification involves the active transport (ATP-dependent) of non-phytotoxic herbicide conjugates into the vacuole by proteins in the multidrug resistance-associated protein (MRP) family or by other transport mechanisms (Kreuz et al., 1996; Riechers et al., 2010). The SNP ss715605561 is located within the MRP gene Glyma10g01700. The low favorable allele frequency (8.0%) could explain the rare occurrence of highly dicamba-tolerant soybean phenotypes. Based on these results, we hypothesize that most soybean genotypes conduct phase I ring hydroxylation and phase II detoxification of dicamba with glucose but have a rate-limiting step in the final phase III transport of non-phytotoxic dicamba-glucose conjugates into the vacuole. However, further metabolite profiling and subcellular transport studies are warranted to test this hypothesis directly.
Although the response to off-target dicamba appears to be a highly complex trait regulated by multiple genes involved in several biochemical pathways, the combination of ss715605561 (Chr. 10), ss715635349 (Chr. 19), and ss715632413 (Chr. 18) can accurately distinguish among tolerant to susceptible genotypes. Accessions carrying the favorable alleles for these SNPs showed the lowest average off-target dicamba damage and the highest frequency of tolerant and moderate classes. Future plant breeding research will utilize and apply these alleles in marker-assisted selection programs targeting identification and development of genotypes with higher tolerance to off-target dicamba. Additionally, molecular physiology research is currently underway to investigate expression patterns and functional roles of the alleles and encoded proteins identified by our GWAS analysis.
Conclusions
Widespread adoption of DT production systems has frequently resulted in yield losses in non-DT soybean genotypes from off-target dicamba exposure. Identification of genetic sources of tolerance and genomic regions conferring higher tolerance to off-target dicamba in non-DT soybean genotypes may sustain and improve other non-DT soybean production systems, including the growing niche markets of organic and conventional soybean. Herein, we report several genetically diverse accessions that can be used as genetic sources for improved tolerance to off-target dicamba. Two genomic regions on Chrs. 10 and 19 were identified that may be directly associated with the ability of soybean to detoxify dicamba and/or transport non-phytotoxic dicamba metabolites into the vacuole. Three significant SNPs accurately distinguished between tolerant and susceptible genotypes. Intensive breeding that targeted single economically important traits may have caused selective sweeps where alleles conferring tolerance to off-target dicamba were lost after many breeding cycles. With the advancements in targeted gene-editing techniques, our results may facilitate identifying and developing conventional soybean cultivars with improved tolerance to off-target dicamba as well as other synthetic auxin herbicides. Further research investigating the physiological mechanisms underlying natural tolerance to dicamba in soybean and dose-response studies in controlled environments can help to determine the threshold at which tolerant genotypes maintain minimal symptomology following off-target dicamba exposure.
Data availability statement
The datasets presented in this study can be found in online repositories. The name of the repository and link to the data can be found as follows: Dryad; https://doi.org/10.5061/dryad.gmsbcc2s4.
Author contributions
CC, DJ, JFZ, BD, DR, DX, GS, PC, and HN contributed to conception and design of the study. CC, BD, DR, and PC contributed to funding resources of the study. CC, EdN, DL, and SS contributed to collection of data used in this study. CC, DJ, and JZ contributed to the statistical analysis of this study. CC wrote the first draft of the manuscript. All authors contributed to manuscript revision, read, and approved the submitted version.
Funding
United Soybean Board (2020-2022, project number 2120-172-0147), Screening and breeding soybean for natural tolerance to off-target dicamba damage Mid-south Soybean Board (2019-2023, project number 20-455-23), Screening and Selecting Non-Xtend Soybeans for Dicamba Tolerance.
Acknowledgments
The authors thank the University of Missouri-Fisher Delta Research, Extension, and Education Center soybean breeding team for their hard work and support in preparing and conducting the field trials. The authors also extend their gratitude to the funding agencies that supported this work including the Mid-South Soybean Board (MSSB), United Soybean Board (USB), and Missouri Soybean Merchandising Council (MSMC).
Conflict of interest
The authors declare that the research was conducted in the absence of any commercial or financial relationships that could be construed as a potential conflict of interest.
Publisher’s note
All claims expressed in this article are solely those of the authors and do not necessarily represent those of their affiliated organizations, or those of the publisher, the editors and the reviewers. Any product that may be evaluated in this article, or claim that may be made by its manufacturer, is not guaranteed or endorsed by the publisher.
Supplementary material
The Supplementary Material for this article can be found online at: https://www.frontiersin.org/articles/10.3389/fpls.2022.1090072/full#supplementary-material
References
Amirrudin, M., Nasution, K., Supahar, S. (2020). Effect of variability on cronbach alpha reliability in research practice. Jurnal Matematika Statistika dan Komputasi 17, 223–230. doi: 10.20956/jmsk.v17i2.11655
Anand, S. C., Gallo, K. M., Baker, I. A., Hartwig, E. E. (1988). Soybean plant introductions with resistance to races 4 or 5 of soybean cyst nematode. Crop Sci. 28, 563–564. doi: 10.2135/cropsci1988.0011183X002800030029x
Andersen, S. M., Clay, S. A., Wrage, L. J., Matthees, D. (2004). Soybean foliage residues of dicamba and 2,4-d and correlation to application rates and yield. Agron. J. 96, 750–760. doi: 10.2134/agronj2004.0750
Bandillo, N., Jarquin, D., Song, Q., Nelson, R., Cregan, P., Specht, J., et al. (2015). A population structure and genome-wide association analysis on the USDA soybean germplasm collection. Plant Genome 8, 1–13. doi: 10.3835/plantgenome2015.04.0024
Barrett, M. (2000). “The role of cytochrome P450 enzymes in herbicide metabolism,” in Herbicides and their mechanisms of action. Eds. Cobb, A. H., Kirkwood, R. C. (Boca Raton, FL: CRC Press), 25–37.
Bates, D., Mächler, M., Bolker, B. M., Walker, S. C. (2015). Fitting linear mixed-effects models using lme4. J. Stat. Softw 67, 1–48. doi: 10.18637/jss.v067.i01
Battaglin, W. A., Kolpin, D. W., Scribner, E. A., Kuivila, K. M., Sandstrom, M. W. (2005). Glyphosate, other herbicides, and transformation products in Midwestern streams 20021. J. Am. Water Resour. Assoc. 41, 323–332. doi: 10.1111/j.1752-1688.2005.tb03738.x
Bayer CropScience LLC (2021) Bayer Fuels leading market positions in crop science through delivery of unmatched innovation. Available at: https://media.bayer.com/baynews/baynews.nsf/id/9F6D3923DFF05C43C125877200331872?open&ref=irrefndcd.
Behrens, R., Lueschen, W. E. (1979). Dicamba volatility. Weed Sci. 27, 486–493. doi: 10.1017/S0043174500044453
Behrens, M. R., Mutlu, N., Chakraborty, S., Dumitru, R., Jiang, W. Z., LaVallee, B. J., et al. (2007). Dicamba resistance: Enlarging and preserving biotechnology-based weed management strategies. Sci. (1979) 316, 1185–1188. doi: 10.1126/science.1141596
Bernardo, R. (2020). Reinventing quantitative genetics for plant breeding: something old, something new, something borrowed, something BLUE. Heredity 125, 375–385. doi: 10.1038/s41437-020-0312-1
Bijelic, A., Pretzler, M., Molitor, C., Zekiri, F., Rompel, A. (2015). The structure of a plant tyrosinase from walnut leaves reveals the importance of “Substrate-guiding residues” for enzymatic specificity. Angewandte Chemie Int. Edition 54, 14677–14680. doi: 10.1002/anie.201506994
Bland, J. M., Altman, D. G. (1997). Statistics notes: Cronbach’s alpha. BMJ 314, 572–572. doi: 10.1136/bmj.314.7080.572
Bosma, H., Marmot, M. G., Hemingway, H., Nicholson, A. C., Brunner, E., Stansfeld, S. A. (1997). Low job control and risk of coronary heart disease in whitehall ii (prospective cohort) study. BMJ 314, 558–558. doi: 10.1136/bmj.314.7080.558
Bradley, K. (2017) A final report on dicamba-injured soybean acres. Available at: https://ipm.missouri.edu/IPCM/2017/10/final_report_dicamba_injured_soybean/.
Bradley, K. (2018) July 15 dicamba injury update. different year, same questions. Available at: https://ipm.missouri.edu/IPCM/2018/7/July-15-Dicamba-injury-update-different-year-same-questions/.
Canella Vieira, C., Sarkar, S., Tian, F., Zhou, J., Jarquin, D., Nguyen, H. T., et al. (2022a). Differentiate soybean response to off-target dicamba damage based on UAV imagery and machine learning. Remote Sense 14, 1618. doi: 10.3390/rs14071618
Canella Vieira, C., Zhou, J., Cross, C., Heiser, J. W., Diers, B., Riechers, D. E., et al. (2022b). Differential responses of soybean genotypes to off-target dicamba damage. Crop Sci. 62, 1472–1483. doi: 10.1002/csc2.20757
Canella Vieira, C., Zhou, J., Usovsky, M., Vuong, T., Howland, A. D., Lee, D., et al. (2022c). Exploring machine learning algorithms to unveil genomic regions associated with resistance to southern root-knot nematode in soybeans. Front. Plant Sci. 13. doi: 10.3389/fpls.2022.883280
Carter, T. E., Nelsom, R., Sneller, C. H., Cui, Z. (2004). Soybeans: Improvement, production, and uses. Eds. Shibles, R. M., Harper, J. E., Wilson, R. F., Shoemaker, R. C. (Madison, WI, USA: American Society of Agronomy, Crop Science Society of America, and Soil Science Society of America). doi: 10.2134/agronmonogr16.3ed
Chamberlain, D. W., Bernard, R. L. (1968). Resistance to brown stem rot in soybeans. Crop Sci. 8, 728–729. doi: 10.2135/cropsci1968.0011183X000800060026x
Chang, H.-X., Lipka, A. E., Domier, L. L., Hartman, G. L. (2016). Characterization of disease resistance loci in the USDA soybean germplasm collection using genome-wide association studies. Phytopathology 106, 1139–1151. doi: 10.1094/PHYTO-01-16-0042-FI
Chen, P., Shannon, J. G., Canella Vieira, C., Nascimento, E. F., Ali, M. L., Lee, D., et al. (2022). Registration of ‘S16-3747GT’: A high-yielding determinate maturity group V soybean cultivar with broad biotic and abiotic stressors tolerance. J. Plant Regist 16, 550–563. doi: 10.1002/plr2.20222
Chism, B., Tindall, K., Orlowski, J. (2020). Dicamba use on genetically modified dicamba-tolerant (DT) cotton and soybean: Incidents and impacts to users and non-users from proposed registrations (Washington, DC). Available at: https://www.regulations.gov/document/EPA-HQ-OPP-2020-0492-0003.
Cronbach, L. J. (1951). Coefficient alpha and the internal structure of tests. Psychometrika 16, 297–334. doi: 10.1007/BF02310555
de Leon, N., Jannink, J.-L., Edwards, J. W., Kaeppler, S. M. (2016). Introduction to a special issue on genotype by environment interaction. Crop Sci. 56, 2081–2089. doi: 10.2135/cropsci2016.07.0002in
Echeverria, M. (2020) Memorandum supporting decision to approve registration for the uses of dicamba on dicamba tolerant cotton and soybean (Washington, D. C). Available at: https://www.epa.gov/sites/default/files/2020-10/documents/dicamba-decision_10-27-2020.pdf (Accessed May 20, 2022).
Egan, J. F., Barlow, K. M., Mortensen, D. A. (2014). A meta-analysis on the effects of 2,4-d and dicamba drift on soybean and cotton. Weed Sci. 62, 193–206. doi: 10.1614/WS-D-13-00025.1
Egan, J. F., Mortensen, D. A. (2012). Quantifying vapor drift of dicamba herbicides applied to soybean. Environ. Toxicol. Chem. 31, 1023–1031. doi: 10.1002/etc.1778
Environmental Protection Agency (2006) Reregistration eligibility decision for dicamba and associated salts. Available at: https://archive.epa.gov/pesticides/reregistration/web/pdf/dicamba_red.pdf.
Fehr, W. R., Caviness, C. E., Burmood, D. T., S., P. J. (1971). Stage of development descriptions for soybeans, Glycine max (L.) Merrill. Crop Sci. 11, 929–931. doi: 10.2135/cropsci1971.0011183X001100060051x
Gale, F., Valdes, C., Ash, M. (2019). Interdependence of China, united states, and Brazil in soybean trade. Economic Res. Service - USDA, 1–48.
Gizlice, Z., Carter, T. E., Burton, J. W. (1993). Genetic diversity in north American soybean: I. multivariate analysis of founding stock and relation to coefficient of parentage. Crop Sci. 33, 614–620. doi: 10.2135/cropsci1993.0011183x003300030038x
Gizlice, Z., Carter Jnr, T. E., Burton, J. W. (1994). Genetic base for north American public soybean cultivars released between 1947 and 1988. Crop Sci. 34, 1143–1151. doi: 10.2135/cropsci1994.0011183X003400050001x
Griffin, J. L., Bauerle, M. J., Stephenson, D. O., Miller, D. K. (2013). And boudreaux, J Soybean response to dicamba applied at vegetative and reproductive growth stages. M. Weed Technol. 27, 696–703. doi: 10.1614/WT-D-13-00084.1
Grossmann, K. (2010). Auxin herbicides: current status of mechanism and mode of action. Pest Manag Sci. 66, 113–120. doi: 10.1002/ps.1860
Han, Y., Zhao, X., Liu, D., Li, Y., Lightfoot, D. A., Yang, Z., et al. (2016). Domestication footprints anchor genomic regions of agronomic importance in soybeans. New Phytol. 209, 871–884. doi: 10.1111/nph.13626
Hartman, G. L., West, E. D., Herman, T. K. (2011). Crops that feed the world 2. soybean–worldwide production, use, and constraints caused by pathogens and pests. Food Secur 3, 5–17. doi: 10.1007/s12571-010-0108-x
Herman, P. L., Behrens, M., Chakraborty, S., Chrastil, B. M., Barycki, J., Weeks, D. P. (2005) A three-component dicamba o-demethylase from pseudomonas maltophilia, strain di-6: Gene isolation, characterization, and heterologous expression J. Biol. Chem. 280, 24759–24767. doi: 10.1074/jbc.M500597200
Huang, M., Liu, X., Zhou, Y., Summers, R. M., Zhang, Z. (2019). BLINK: A package for the next level of genome-wide association studies with both individuals and markers in the millions. Gigascience 8, 1–12. doi: 10.1093/gigascience/giy154
Hwang, E.-Y., Song, Q., Jia, G., Specht, J. E., Hyten, D. L., Costa, J., et al. (2014). A genome-wide association study of seed protein and oil content in soybean. BMC Genomics 15, 1. doi: 10.1186/1471-2164-15-1
Hyten, D. L., Song, Q., Zhu, Y., Choi, I.-Y., Nelson, R. L., Costa, J. M., et al. (2006). Impacts of genetic bottlenecks on soybean genome diversity. Proc. Natl. Acad. Sci. 103, 16666–16671. doi: 10.1073/pnas.0604379103
Kelley, K. B., Wax, L. M., Hager, A. G., Riechers, D. E. (2005). Soybean response to plant growth regulator herbicides is affected by other postemergence herbicides. Weed Sci. 53, 101–112. doi: 10.1614/WS-04-078R
Kline, P. (1999). Handbook of psychological testing. 2nd edition (London, UK: Routledge). Available at: https://www.taylorfrancis.com/books/mono/10.4324/9781315812274/handbook-psychological-testing-paul-kline.
Kniss, A. R. (2018). Soybean response to dicamba: A meta-analysis. Weed Technol. 32, 507–512. doi: 10.1017/wet.2018.74
Kreuz, K., Tommasini, R., Martinoia, E. (1996). Old enzymes for a new job (Herbicide detoxification in plants). Plant Physiol. 111, 349–353. doi: 10.1104/pp.111.2.349
Kuznetsova, A., Brockhoff, P. B., Christensen, R. H. B. (2017). lmerTest package: Tests in linear mixed effects models. J. Stat. Softw 82, 1–26. doi: 10.18637/jss.v082.i13
Lipka, A. E., Tian, F., Wang, Q., Peiffer, J., Li, M., Bradbury, P. J., et al. (2012). GAPIT: Genome association and prediction integrated tool. Bioinformatics 28, 2397–2399. doi: 10.1093/bioinformatics/bts444
Liu, X., Huang, M., Fan, B., Buckler, E. S., Zhang, Z. (2016). Iterative usage of fixed and random effect models for powerful and efficient genome-wide association studies. PloS Genet. 12, 1–24. doi: 10.1371/journal.pgen.1005767
Liu, S., Zhang, M., Feng, F., Tian, Z. (2020). Toward a “Green revolution” for soybean. Mol. Plant 13, 688–697. doi: 10.1016/j.molp.2020.03.002
Lopez, M. A., Moreira, F. F., Hearst, A., Cherkauer, K., Rainey, K. M. (2022). Physiological breeding for yield improvement in soybean: solar radiation interception-conversion, and harvest index. Theor. Appl. Genet. 135, 1477–1491. doi: 10.1007/s00122-022-04048-5
Luzzi, B. M., Boerma, H. R., Hussey, R. S. (1987). Resistance to three species of root-knot nematode in soybean. Crop Sci. 27, 258–262. doi: 10.2135/cropsci1987.0011183X002700020027x
McCown, S., Barber, T., Norsworthy, J. K. (2018). Response of non–Dicamba-Resistant soybean to dicamba as influenced by growth stage and herbicide rate. Weed Technol. 32, 513–519. doi: 10.1017/wet.2018.64
McKinley, R. K., Manku-Scott, T., Hastings, A. M., French, D. P., Baker, R. (1997). Reliability and validity of a new measure of patient satisfaction with out of hours primary medical care in the united kingdom: development of a patient questionnaire. BMJ 314, 193–193. doi: 10.1136/bmj.314.7075.193
Min, K., Park, G. W., Yoo, Y. J., Lee, J.-S. (2019). A perspective on the biotechnological applications of the versatile tyrosinase. Bioresource technology. 289, 1–8. doi: 10.1016/j.biortech.2019.121730
Nicholls, H. L., John, C. R., Watson, D. S., Munroe, P. B., Barnes, M. R. (2020). And cabrera, c Reaching the end-game for GWAS: Machine learning approaches for the prioritization of complex disease loci. P. Front. Genet. 11, 1–15. doi: 10.3389/fgene.2020.00350
R Core Team (2022) R: A language and environment for statistical computing. Available at: http://www.r-project.org/.
Revelle, W. (2021). Psych: Procedures for psychological, psychometric, and personality research 1–457. Available at: https://www.scholars.northwestern.edu/en/publications/psych-procedures-for-personality-and-psychological-research.
Riechers, D. E., Kreuz, K., Zhang, Q. (2010). Detoxification without intoxication: Herbicide safeners activate plant defense gene expression. Plant Physiol. 153, 3–13. doi: 10.1104/pp.110.153601
Robinson, A. P., Simpson, D. M., Johnson, W. G. (2013). Response of glyphosate-tolerant soybean yield components to dicamba exposure. Weed Sci. 61, 526–536. doi: 10.1614/WS-D-12-00203.1
Sidak, Z. (1967). Rectangular confidence regions for the means of multivariate normal distributions. Journal of the American statistical association. 62, 626–633. doi: 10.2307/2283989
Solomon, C. B., Bradley, K. W. (2014). Influence of application timings and sublethal rates of synthetic auxin herbicides on soybean. Weed Technol. 28, 454–464. doi: 10.1614/WT-D-13-00145.1
Soltani, N., Nurse, R. E., Sikkema, P. H. (2016). Response of glyphosate-resistant soybean to dicamba spray tank contamination during vegetative and reproductive growth stages. Can. J. Plant Sci. 96, 160–164. doi: 10.1139/cjps-2015-0175
Sonah, H., O’Donoughue, L., Cober, E., Rajcan, I., Belzile, F. (2015). Identification of loci governing eight agronomic traits using a GBS-GWAS approach and validation by QTL mapping in soya bean. Plant Biotechnol. J. 13, 211–221. doi: 10.1111/pbi.12249
Song, Q., Hyten, D. L., Jia, G., Quigley, C., Fickus, E. W., Nelson, R. L., et al. (2013). Development and evaluation of SoySNP50K, a high-density genotyping array for soybean. PloS One 8, 1–12. doi: 10.1371/journal.pone.0054985
Song, Q., Hyten, D. L., Jia, G., Quigley, C., Fickus, E. W., Nelson, R. L., et al. (2015). Fingerprinting soybean germplasm and its utility in genomic research. G3 Genes|Genomes|Genetics 5, 1999–2006. doi: 10.1534/g3.115.019000
Song, Q., Yan, L., Quigley, C., Fickus, E., Wei, H., Chen, L., et al. (2020). Soybean BARCSoySNP6K: An assay for soybean genetics and breeding research. Plant J. 104, 800–811. doi: 10.1111/tpj.14960
Sullivan, M. L. (2015). Beyond brown: polyphenol oxidases as enzymes of plant specialized metabolism. Front. Plant Sci. 5 5, 1–7. doi: 10.3389/fpls.2014.00783
Tavakol, M., Dennick, R. (2011). Making sense of cronbach’s alpha. Int. J. Med. Educ. 2, 55. doi: 10.5116/ijme.4dfb.8dfd
Tindall, K., Becker, J., Orlowski, J., Hawkins, C., Kells, B. (2021) Status of over-the-Top dicamba: Summary of 2021 usage, incidents and consequences of off-target movement, and impacts of stakeholder-suggested mitigations (Washington, D.C). Available at: https://downloads.regulations.gov/EPA-HQ-OPP-2020-0492-0021/content.pdf (Accessed May 20, 2022).
USDA Economic Research Service (2022)Recent trends in GE adoption. In: Adoption of genetically engineered crops in the U.S. Available at: https://www.ers.usda.gov/data-products/adoption-of-genetically-engineered-crops-in-the-u-s/recent-trends-in-ge-adoption (Accessed October 11, 2022).
Valliyodan, B., Ye, H., Song, L., Murphy, M., Shannon, J. G., Nguyen, H. T. (2016). Genetic diversity and genomic strategies for improving drought and waterlogging tolerance in soybeans. J. Exp. Bot. 68, 1835–1849. doi: 10.1093/jxb/erw433
van Duyn, J. W., Turnipseed, S. G., Maxwell, J. D. (1971). Resistance in soybeans to the lvlexican bean beetle. Crop Sci. 11, 572–573. doi: 10.2135/cropsci1971.0011183X001100040035x
Vieira, C. C., Chen, P. (2021). The numbers game of soybean breeding in the united states. Crop Breed. Appl. Biotechnol. 21, 387521–387531. doi: 10.1590/1984
Vogel, J. T., Liu, W., Olhoft, P., Crafts-Brandner, S. J., Pennycooke, J. C., Christiansen, N. (2021). Soybean yield formation physiology – a foundation for precision breeding based improvement. Front. Plant Sci. 12. doi: 10.3389/fpls.2021.719706
Vuong, T. D., Sonah, H., Meinhardt, C. G., Deshmukh, R., Kadam, S., Nelson, R. L., et al. (2015). Genetic architecture of cyst nematode resistance revealed by genome-wide association study in soybean. BMC Genomics 16, 593. doi: 10.1186/s12864-015-1811-y
Wagman, M., Farruggia, F., Odenkirchen, E., Connolly, J. (2020). Dicamba DGA and BAPMA salts – 2020 ecological assessment of dicamba use on dicamba-tolerant (DT) cotton and soybean including effects determinations for federally listed threatened and endangered species (Washington, DC). Available at: https://www.regulations.gov/document/EPA-HQ-OPP-2020-0492-0002.
Wang, J., Chu, S., Zhang, H., Zhu, Y., Cheng, H., Yu, D. (2016b). Development and application of a novel genome-wide SNP array reveals domestication history in soybean. Sci. Rep. 6, 1–10. doi: 10.1038/srep20728
Wang, C., Glenn, K. C., Kessenich, C., Bell, E., Burzio, L. A., Koch, M. S., et al. (2016a). Safety assessment of dicamba mono-oxygenases that confer dicamba tolerance to various crops. Regul. Toxicol. Pharmacol. 81, 171–182. doi: 10.1016/j.yrtph.2016.08.014
Wax, L. M., Knuth, L. A., Slife, F. W. (1969). Response of soybeans to 2,4-d, dicamba, and picloram. Weed Sci. 17, 388–393. doi: 10.1017/S004317450005431X
Wechsler, S., Smith, D., McFadden, J., Dodson, L., Williamson, S. (2019) The use of genetically engineered dicamba-tolerant soybean seeds has increased quickly, benefiting adopters but damaging crops in some fields. Available at: https://www.ers.usda.gov/amber-waves/2019/october/the-use-of-genetically-engineered-dicamba-tolerant-soybean-seeds-has-increased-quickly-benefiting-adopters-but-damaging-crops-in-some-fields/.
Weidenhamer, J. D., Triplett, G. B., Sobotka, F. E. (1989). Dicamba injury to soybean. Agron. J. 81, 637–643. doi: 10.2134/agronj1989.00021962008100040017x
Werle, R., Oliveira, M. C., Jhala, A. J., Proctor, C. A., Rees, J., Klein, R. (2018). Survey of Nebraska farmers’ adoption of dicamba-resistant soybean technology and dicamba off-target movement. Weed Technol. 32, 754–761. doi: 10.1017/wet.2018.62
Wu, C., Mozzoni, L. A., Moseley, D., Hummer, W., Ye, H., Chen, P., et al. (2020). Genome-wide association mapping of flooding tolerance in soybean. Mol. Breed. 40, 4. doi: 10.1007/s11032-019-1086-0
Yoosefzadeh-Najafabadi, M., Torabi, S., Tulpan, D., Rajcan, I., Eskandari, M. (2021). Genome-wide association studies of soybean yield-related hyperspectral reflectance bands using machine learning-mediated data integration methods. Front. Plant Sci. 12. doi: 10.3389/fpls.2021.777028
Young, L. D. (1990). Soybean germplasm evaluated for resistance to races 3, 5, and 14 of soybean cyst nematode. Crop Sci. 30, 735–736. doi: 10.2135/cropsci1990.0011183X003000030052x
Zeng, A., Chen, P., Korth, K., Hancock, F., Pereira, A., Brye, K., et al. (2017). Genome-wide association study (GWAS) of salt tolerance in worldwide soybean germplasm lines. Mol. Breed. 37, 30. doi: 10.1007/s11032-017-0634-8
Zhang, Z., Ersoz, E., Lai, C., Todhunter, R. J., Tiwari, H. K., Gore, M. A., et al. (2010). Mixed linear model approach adapted for genome-wide association studies. Nat. Publishing Group 42, 355–360. doi: 10.1038/ng.546
Keywords: soybean, plant breeding, dicamba, synthetic auxin, genomics, GWAS, G×E
Citation: Canella Vieira C, Jarquin D, do Nascimento EF, Lee D, Zhou J, Smothers S, Zhou J, Diers B, Riechers DE, Xu D, Shannon G, Chen P and Nguyen HT (2022) Identification of genomic regions associated with soybean responses to off-target dicamba exposure. Front. Plant Sci. 13:1090072. doi: 10.3389/fpls.2022.1090072
Received: 04 November 2022; Accepted: 24 November 2022;
Published: 09 December 2022.
Edited by:
Ainong Shi, University of Arkansas, United StatesReviewed by:
Qijian Song, Agricultural Research Service, United States Department of Agriculture (USDA), United StatesMohsen Yoosefzadeh Najafabadi, University of Guelph, Canada
Copyright © 2022 Canella Vieira, Jarquin, do Nascimento, Lee, Zhou, Smothers, Zhou, Diers, Riechers, Xu, Shannon, Chen and Nguyen. This is an open-access article distributed under the terms of the Creative Commons Attribution License (CC BY). The use, distribution or reproduction in other forums is permitted, provided the original author(s) and the copyright owner(s) are credited and that the original publication in this journal is cited, in accordance with accepted academic practice. No use, distribution or reproduction is permitted which does not comply with these terms.
*Correspondence: Henry T. Nguyen, bmd1eWVuaGVucnlAbWlzc291cmkuZWR1