- 1Hubei Collaborative Innovation Centre for Grain Industry, College of Agriculture, Yangtze University, Jingzhou, China
- 2Institute of Plant Breeding, Genetics and Genomics University of Georgia, Athens, GA, United States
- 3Department of Animal Genetics, Breeding and Reproduction, College of Animal Science, South China Agricultural University, Guangzhou, Guangdong, China
- 4School of Agriculture, University of Cape Coast, Cape Coast, Ghana
Improving grain yield potential in rice is an important step toward addressing global food security challenges. The meta-QTL analysis offers stable and robust QTLs irrespective of the genetic background of mapping populations and phenotype environment and effectively narrows confidence intervals (CI) for candidate gene (CG) mining and marker-assisted selection improvement. To achieve these aims, a comprehensive bibliographic search for grain yield traits (spikelet fertility, number of grains per panicle, panicles number per plant, and 1000-grain weight) QTLs was conducted, and 462 QTLs were retrieved from 47 independent QTL research published between 2002 and 2022. QTL projection was performed using a reference map with a cumulative length of 2,945.67 cM, and MQTL analysis was conducted on 313 QTLs. Consequently, a total of 62 MQTLs were identified with reduced mean CI (up to 3.40 fold) compared to the mean CI of original QTLs. However, 10 of these MQTLs harbored at least six of the initial QTLs from diverse genetic backgrounds and environments and were considered the most stable and robust MQTLs. Also, MQTLs were compared with GWAS studies and resulted in the identification of 16 common significant loci modulating the evaluated traits. Gene annotation, gene ontology (GO) enrichment, and RNA-seq analyses of chromosome regions of the stable MQTLs detected 52 potential CGs including those that have been cloned in previous studies. These genes encode proteins known to be involved in regulating grain yield including cytochrome P450, zinc fingers, MADs-box, AP2/ERF domain, F-box, ubiquitin ligase domain protein, homeobox domain, DEAD-box ATP domain, and U-box domain. This study provides the framework for molecular dissection of grain yield in rice. Moreover, the MQTLs and CGs identified could be useful for fine mapping, gene cloning, and marker-assisted selection to improve rice productivity.
Introduction
Rice is an important cereal crop and provides over 21% of caloric intake for more than half of the global population (Zhang et al., 2014; Vishnukiran et al., 2020; Zhao et al., 2020). Rapid population growth remains a major threat to food security worldwide, thus there is a need to augment efforts to increase the yield potential of rice. Spikelet fertility, panicle number per plant, number of grains per panicle, and thousand-grain weight are key indicators of grain yield and continue to be major targets in breeding programs (Sakamoto and Matsuoka, 2008; Huang et al., 2013; Li et al., 2019). Grain yield is quantitatively inherited and controlled by multiple loci (genes) (Xing and Zhang, 2010; Bai et al., 2012); therefore, it is imperative to ascertain the genetic bases of grain yield to boost rice productivity. With the advancement of QTL mapping, breeders have been able to identify genomic regions influencing complex agronomic traits (Daware et al., 2017) and have expedited the process of improving quantitative traits through marker-assisted selection (Zhang et al., 2010). However, the application of QTLs by breeders in a broader spectrum has major setbacks due to the genetic background of the populations used in QTL mapping and phenotype environment (Arcade et al., 2004; Daware et al., 2017). Therefore, a comprehensive analysis of identified QTLs is required to make these QTLs applicable in rice breeding and to decipher the underlying genetic factors conferring grain yield. Essentially, QTL meta-analysis has been shown to be a reliable and effective instrument (Goffinet and Gerber, 2000).
An integrated meta-QTL analysis uses QTLs from independent trials regardless of their genetic backgrounds, cross types/parents, size of populations, years, and locations to identify consensus QTLs (Goffinet and Gerber, 2000; Arcade et al., 2004; Sosnowski et al., 2012). This approach has been evidenced to reliably refine QTLs location and narrow confidence intervals (CI) which enhance the precision of marker-assisted selection (MAS) and discovery of candidate genes (Martinez et al., 2016; Zhang et al., 2017). Furthermore, a meta-analysis of QTLs attempts to clarify if QTLs encompassing different loci from different studies are the same loci or if they represent the same position on a linkage map of species under study. To date, numerous MQTLs for important agronomic traits have been identified in different crops such as wheat (Acuña-Galindo et al., 2015; Darzi-Ramandi et al., 2017; Soriano and Alvaro, 2019; Liu et al., 2020; Saini et al., 2021; Amo and Soriano, 2022; Miao et al., 2022; Saini et al., 2022; Yang et al., 2021), soybean (Zhao-Ming et al., 2011; Hwang et al., 2016; Qin et al., 2018), maize (Semagn et al., 2013; Wang et al., 2013; Martinez et al., 2016; Chen et al., 2017; Zhao et al., 2018; Kaur et al., 2021), barley (Zhang et al., 2017; Khahani et al., 2019), peanut (Lu et al., 2018), and sorghum (Aquib and Nafis, 2021). However, reports on Meta-QTLs for grain yield in rice under field conditions are limited. More so, the understanding of the molecular basis of QTLs/genes controlling grain yield is insufficient. Sandhu et al. (2021) detected 76 MQTLs governing grain number per panicle, spikelet fertility, and panicle number per plant on 29 QTL studies published from 2000 to 2022. Also, Wu et al. (2016) reported ten MQTLs associated with spikelet fertility on 82 QTL studies from 1996 to 2013. In another study, Khahani et al. (2021) integrated 101 studies in rice and confined these QTLs to meta-QTLs with reduced confidence intervals for use in MAS and discovered candidate genes for future functional validation.
This study performed a comprehensive meta-QTL analysis examining QTLs for spikelet fertility (SF), panicle number per plant (PN), grain number per panicle (GNP), and 1000-grain weight retrieved from 47 QTL studies published between 2002 and 2022 in 101 biparental populations evaluated across diverse environments and under field condition. The main objective of this study was to conduct meta-QTL analysis and identify stable and robust MQTLs and candidate genes (CGs) to aid rice breeding through MAS and enhance molecular dissection of grain yield targeted at improving the yield potential of rice.
Materials and methods
To enhance understanding, a schematic flow chart has been drawn and presented in Figure 1, which illustrates the approach adopted in executing this study. Further description of these steps is as ensued:
Literature review and data collection on QTLs
A comprehensive bibliographic survey for QTLs associated with spikelet fertility (SF), grain number per panicle (GNP), number of panicles per plant (PN), and thousand-grain weight (TGW) published from 2002 to 2022 was conducted using Google Scholar (https://scholar.google.com/), PubMed (http://www.ncbi.nlm.nih.gov/pubmed), and Gramene QTL database platform (http://archive.gramene.org/qtl/) (Table 1 and Supplementary Table 1). The information gathered in each study during QTL compilation was as ensued: (i) Linked markers of individual QTLs, (ii) Position (cM) of QTLs on genetic map, (iii) size and type of mapping population, (iv) phenotypic variance explained (R2) value of each QTLs, and (v) LOD scores of individual QTLs. The original identities of all QTLs were maintained for ease of analysis.
Consensus map construction and QTL projection
The LPMerge in R package programming was used to build the reference map (Endelman and Plomion, 2014). The most comprehensive and high-density genetic map with different marker types developed by Wu et al. (2016) was selected. Further, some of the markers flanking the QTLs reported in individual studies were also incorporated into the reference map. The marker names were checked to ensure consistency and avoid duplication on the consensus map. The initial QTLs were projected using BioMercator v4.2.3 software (Sosnowski et al., 2012) (https://versailles.inra.fr/Tools/BioMercator-v4) based on the approach described in (Chardon et al., 2004). Before QTL projection, 95% confidence interval (CI) was determined using the following formulas:
for backcross (BC) and F2, double haploid (DH), and recombinant inbred line (RIL), where N represents the size of the mapping population and R2 is the phenotypic variance explained by individual QTLs (Darvasi and Soller, 1997; Guo et al., 2006). 3% and 10% were used for QTLs that lack LOD scores and R2 values. All QTLs with adequate information required by the software were projected onto the consensus/reference map.
Analysis of meta-quantitative trait loci (MQTLs)
Analysis of Meta-QTL was performed using BioMercator v4.2.3 (Sosnowski et al., 2012). For analysis, two approaches were used, considering the amount of QTLs in each chromosome. When the number of QTLs on each chromosome is ≤ 10, the method reported by Goffinet and Gerber (2000) was used; if the number of QTLs on each chromosome is greater than 10, the method proposed by Veyrieras et al. (2007) was utilized. Firstly, the lowest Akaike information criterion (AIC) value was selected as the best fit model. Secondly, the best fit model was considered significant in identifying MQTL from the following: AIC3, AIC, Bayesian information criterion (BIC), average weight of evidence (AWE), and corrected AIC. Based on the five criteria, the lowest value was selected to obtain MQTLs with their respective genetic positions (cM), confidence intervals (CI), and percent membership of initial QTLs to each MQTL. Using Rice SNP-seek database, all MQTLs identified in this study were verified with Genome-wide Association study results related to the studied traits (Mansueto et al., 2017). The naming of MQTL was based on their positions on the chromosome (i.e. MQTL1.1, MQTL1.2, and MQTL1.3).
Candidate Gene (CG) excavation within the most stable MQTLs interval
To explore functional genes modulating the evaluated traits, the flanking marker sequences were employed to determine the genomic positions of MQTLs in this study. Essentially, the MQTLs with at least 6 of the initial QTLs clusters were selected as the most robust and stable MQTLs. The Gramene database (https://archive.gramene.org/markers/) and available literature were used to retrieve flanking marker sequences of the robust MQTLs. Phytozome v13 was used to blast the sequences against the rice reference genome version 7.0 (Oryza sativa v7.0) to obtain the physical positions of stable MQTLs. All model genes were extracted from the physical intervals of the stable and robust MQTLs using the rice genome annotation project database (https://rice.uga.edu/cgi-bin/gbrowse/rice/) and annotated using (http://www.ricedata.cn, https://rice.uga.edu/cgi-bin/gbrowse/rice/, http://shigen.nig.ac/jp/rice/oryzabase/, and https://rapdb.dna.affrc.go.jp/).
Functional analysis of candidate genes
GENEDENOVO cloud platform (https://www.omicshare.com/tools/) was used to perform gene ontology (GO) enrichment analysis. The protein-protein interaction network was predicted using STRING (Szklarczyk et al., 2019). Based on the annotation information of significant CGs enriched by Gene ontology (GO) analysis, the CGs in each MQTLs with known proteins associated with grain yield were selected. In silico expression analysis was performed for these CGs using RNA-Seq gene expression data (Kawahara et al., 2013). A heatmap was constructed to visualize the expression of CGs using Morpheus online tool (https://software.broadinstitute.org/morpheus/).
Results
Major features of QTLs used in the meta-analysis
To unravel consensus chromosomal regions related to rice grain yield, we compiled QTL information on 462 QTLs obtained from 47 independent QTL studies (Table 1 and Supplementary Table 1). The population type consisted of RIL (26), BC (11), F2 (9), and DH (1) with the mapping population size ranging from 24 to 472 (Table 1). The 462 QTLs were unequally distributed on all chromosomes (Figure 2A). A total of 197 QTLs were found on chromosomes 1, 3, and 5, followed by 47 QTLs on chromosome 2 and 48 QTLs on chromosome 6. The least QTLs (17 QTLs) were concentrated on Chr 8. Among the studied traits reported, TGW had the greatest number of QTL (179 QTL) followed by SF (127 QTL), GNP (85), and PN (71) (Figure 2B). Notably, most of the traits were enclosed in Chr 3, Chr 5, and Chr 6 (Figure 2B). QTLs explained between 0.03% and 52.0% of the phenotypic variance (PVE or R2), with an average of 8.52%. In total, 67.53% of the initial QTLs showed PVE<10%, indicating that individual QTLs explained only a small proportion of phenotype variation (Figure 2C). This result indicates that grain yield appears to be mostly controlled by loci with minor genetic effects and complex genetic architecture. For each QTL, LOD score ranged from 0.00 to 210.50, with an average of 10.09. The distribution of LOD scores of individual QTLs is presented in Figure 2D.
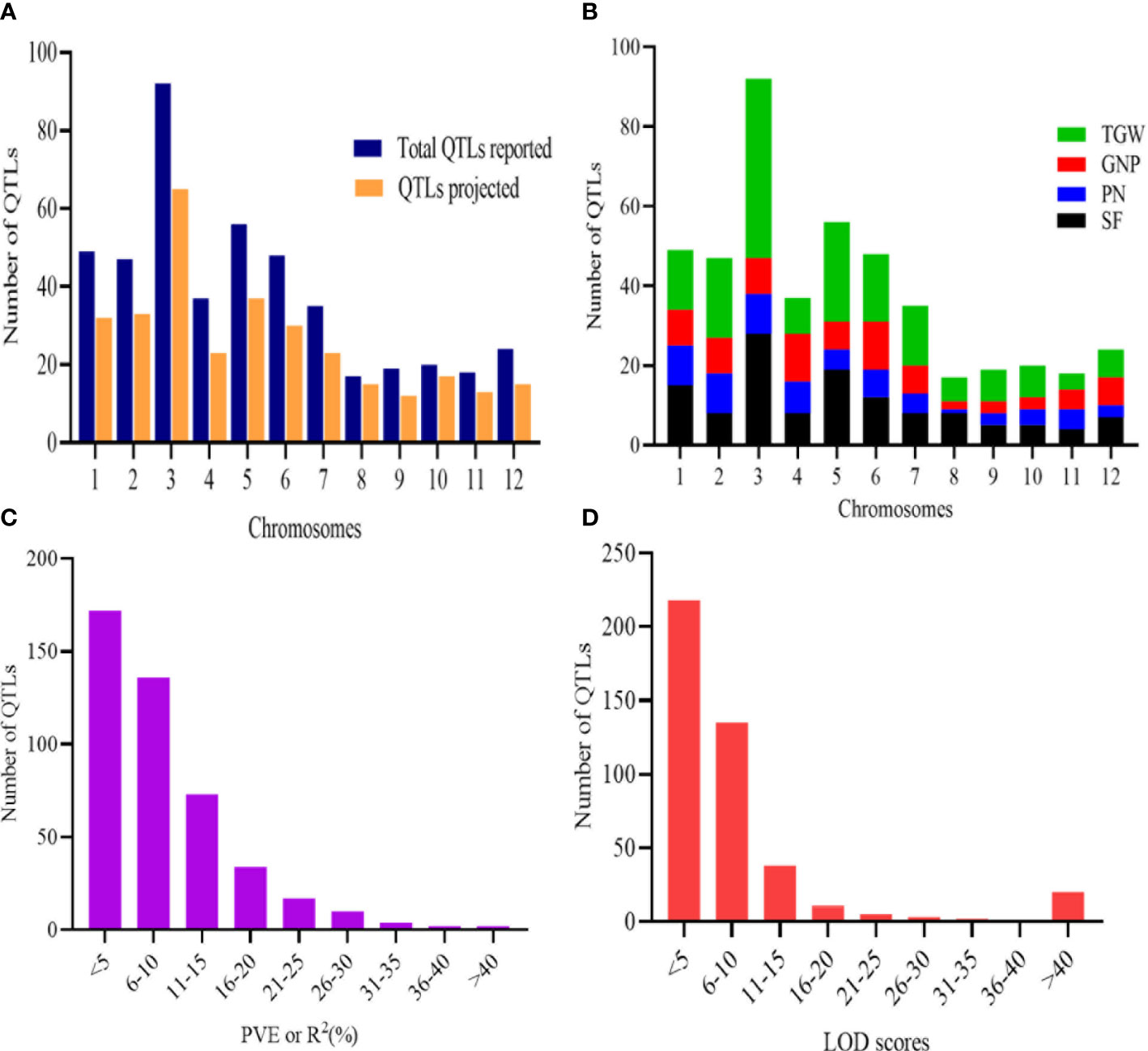
Figure 2 Basic features of the QTLs. (A) Distribution of initial QTLs and the QTLs projected. (B) Trait-wise distribution of QTLs on the 12 chromosomes of rice. (C) PVE or R2 (%) of initial QTLs. (D) LOD scores of initial QTL involved in MQTLs.
Consensus map of rice
The consensus map consisted of 7,050 markers (Figure 3) which comprised SNP, SSR, RFLPs, STS, RAPDs, ALFPs, MITEs, FNP, SLAF, SSLP, and InDels. Also, genes such as Grh, Prp2, Pox5, Pla1, Rpr2, W1, and cen1 were included in the reference map. The consensus/reference map had a cumulative map length of 2,945.67 cM with a genetic distance of individual chromosomes ranging from 144.00 cM to 383.80 cM (Supplementary Table 2). The mean marker densities per chromosome ranged from 7.25 cM to 21.49 cM and 13.10 cM for the whole genome. The highest number of markers were found on Chr 1 (972 markers) followed by Chr 3 (835 markers) and Chr 2 (733 markers) (Figure 3). However, Chr 10 harbored the lowest number of markers (328 markers) on the reference map.
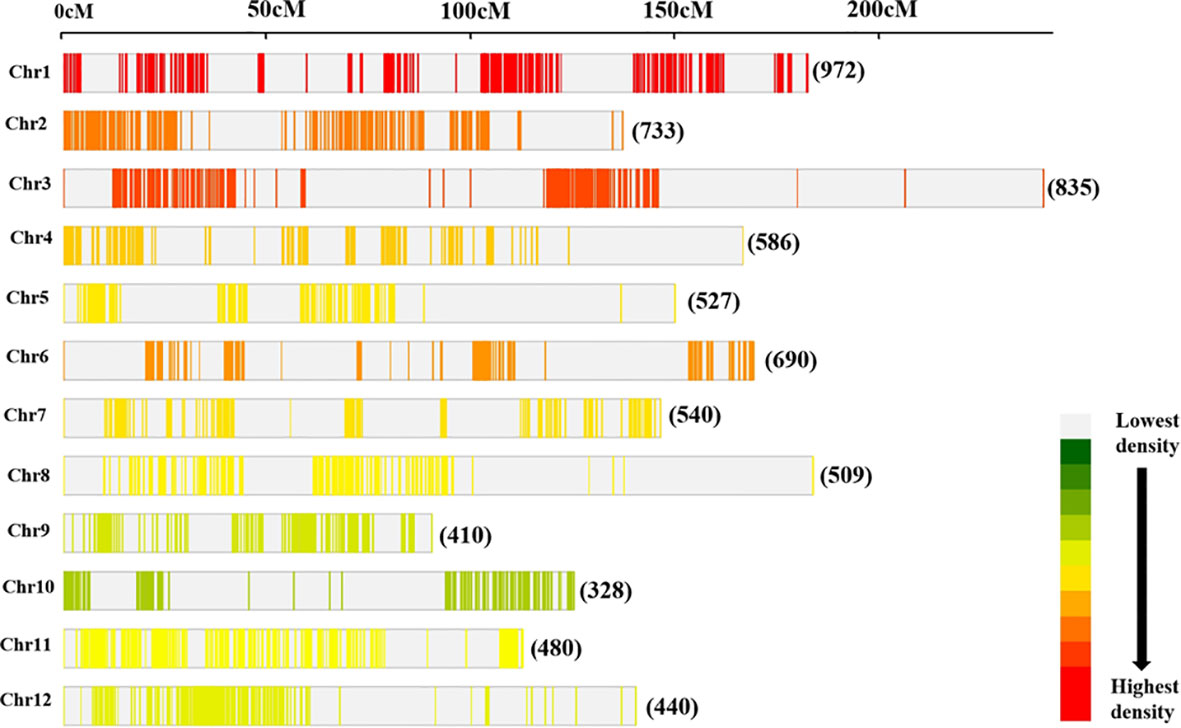
Figure 3 Distribution of markers on the consensus map utilized for meta-analysis of QTLs. The number of loci mapped on individual rice chromosome is shown.
QTL projection and analysis of meta-QTL
Of the total 462 QTLs available, only 313 QTLs could be successfully projected onto the reference map; the remaining 149 QTLs could not be projected due to inadequate information required for the QTL projection. The MQTL analysis refined the projected QTLs (313 QTLs) to 62 MQTLs (Figure 4 and Table 2). There was an uneven distribution of MQTLs on the rice chromosome. MQTLs on each chromosome ranged from two (Chr 8) to nine (Chr 3) with an average of 5.16 MQTLs per chromosome. The projection yielded the detection of 62 MQTLs, which involved 232 QTLs (Table 2 and Figure 4); the remaining 81 QTLs could not be allocated to any of the MQTLs partly due to lack of associated markers on the reference map and initial maps, low phenotypic variance explained by individual QTLs, relatively low LOD score values, and large confidence interval (CI), respectively.
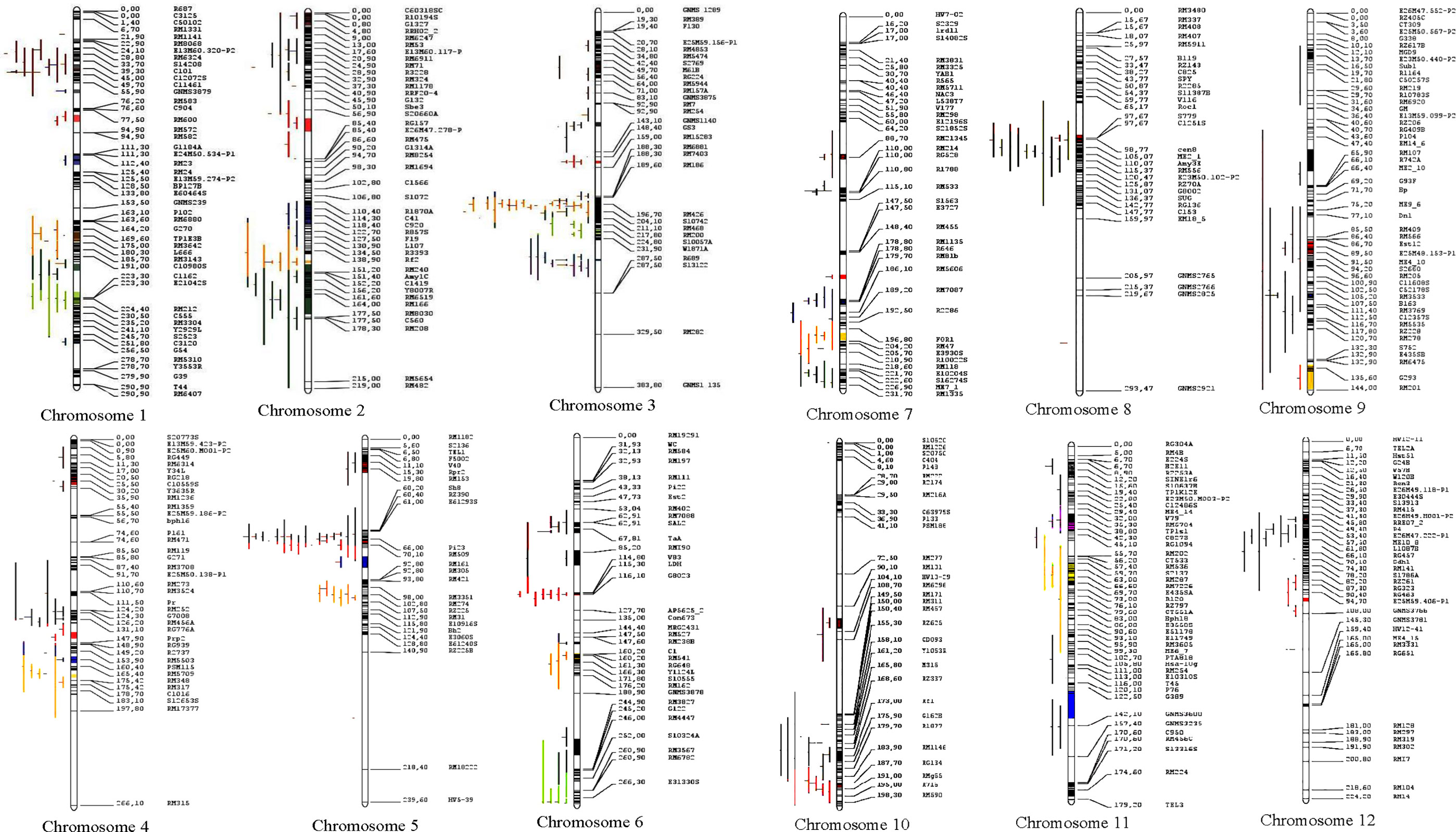
Figure 4 Distribution of MQTLs for grain yield traits on the 12 chromosomes of rice. Different colors on the left side of the maps indicate the initial QTLs involved in MQTLs.
The explained phenotypic variation by each MQTL identified ranged from 0.09% to 27.6% with a mean PVE (%) of 9.26% (Table 2). Individual MQTLs differed markedly for many traits modulating MQTL (Table 2). The MQTLs association with studied traits varied markedly from a solitary trait controlled by MQTL1.2 to 14 traits regulated by MQTL3.5 (Figure 5A). Out of 62 MQTLs, 50 MQTLs were related to at least two QTLs (controlling more than two different traits) from different mapping populations and genetic backgrounds. This result showed significant co-localization of chromosome regions regulating grain yield in rice. A total of 7 MQTLs (MQTL1.1, MQTL2.6, MQTL4.2, MQTL5.5, MQTL6.3, MQTL10.2, and MQTL12.2) were identified to contain more than six QTLs (Table 2). The 3 MQTLs (MQTL3.5, MQTL5.2, and MQTL8.2) were detected to contain more than 10 QTLs. However, 12 MQTLs (MQTL1.1, MQTL1.4, MQTL1.8, MQTL2.1, MQTL4.4, MQTL5.4, MQTL6.4, MQTL7.3, MQTL8.1, MQTL9.1, MQTL9.4, and MQTL12.1) were reported to harbor a single initial QTL governing spikelet fertility, thousand-grain weight, and grain number per panicle. Interestingly, none of the MQTLs showed overlapped regions in this study.
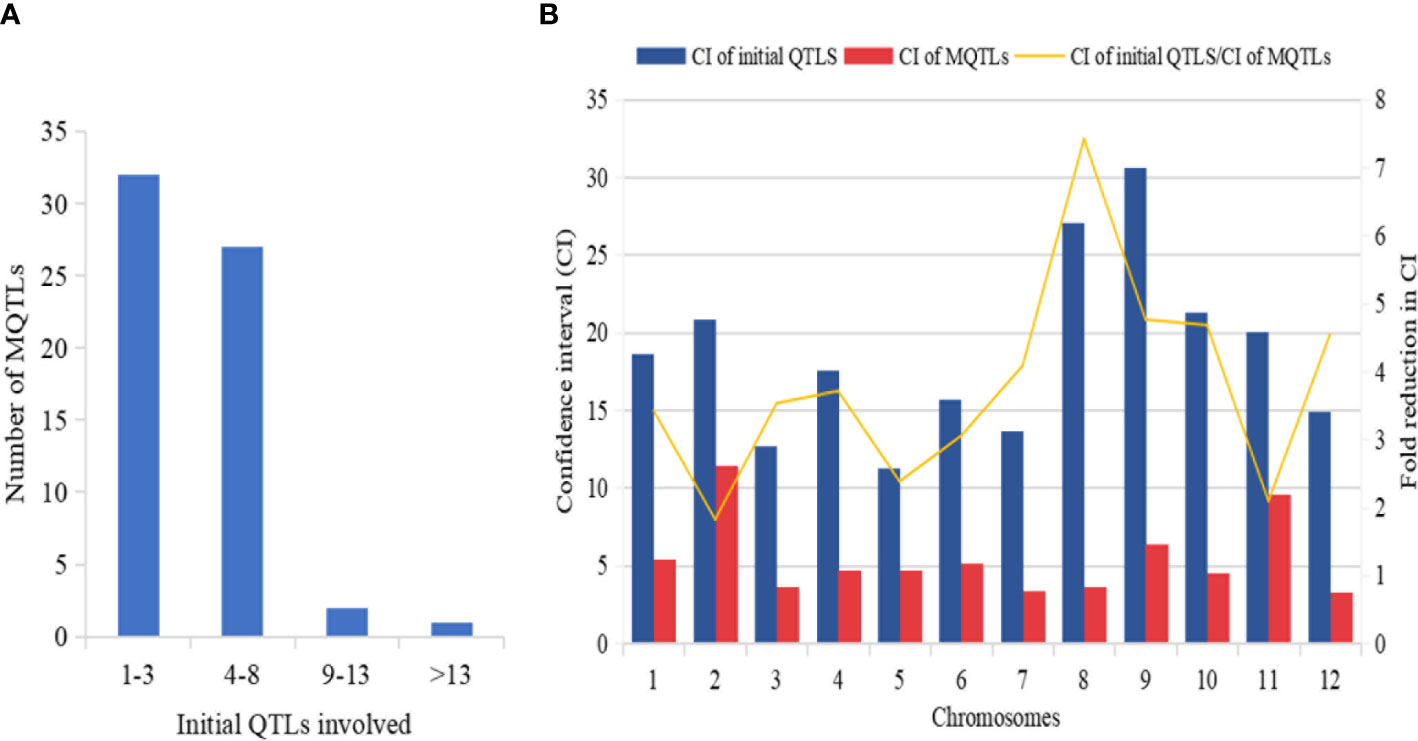
Figure 5 Basic information of MQTLs identified in this study. (A) Frequency of MQTLs and the number of initial QTLs involved. (B) Comparison of CI of initial QTLs and MQTLs, exhibiting fold reduction in CI of initial QTLs and MQTLs.
The mean confidence interval of the MQTLs showed a markedly significant reduction (3.40 fold) compared to the CI of the initial QTLs (Figure 5B). The CI of initial QTLs ranged from 0.60 cM to 112.29 cM with a mean CI of 18.03. The CI of the reported MQTLs ranged from 0.24 cM to 29.45 cM with a mean CI of 10.91 cM. The average CI of MQTLs per chromosome showed a narrow CI ranging from 3.29 cM to 9.58 cM compared to the CI of initial QTLs which showed a wider CI ranging from 11.32 cM to 30.59 cM (Figure 5B), respectively. The fold reduction (average) in the size of CI of MQTLs was highest for Chr 8 (7.42 fold) followed by Chr 9 (4.76 fold); Chr 2 (1.80 fold) recorded the lowest fold reduction in the size of CI of MQTLs (Figure 5B).
Validation of MQTLs with GWAS
To check the efficacy of identified MQTLs, the physical position of all MQTLs was compared with the GWAS results available on Rice SNP-seek database. Consequently, 16 MQTLs co-located with SNP peak loci in rice GWAS for 1000-grain weight and spikelet fertility traits (Supplementary Figure 1). Among the 16 MQTLs, only two MQTLs (MQTL3.2 and MQTL3.5) were co-located with GWAS signals for both 1000-grain weight and spikelet fertility. This indicates the coherence of the two methods in identifying common chromosome regions associated with the studied traits.
Candidate gene mining of the most stable and robust MQTLs
To identify potential CGs in the MQTLs regions, MQTLs that harbored at least six of the initial QTL clusters were selected as the most stable and robust MQTLs. Based on this, 10 of the 62 MQTLs were selected. However, CGs could not be extracted in the two MQTL intervals due to the unavailability of flanking marker sequences. A total of 2,030 putative CGs underlying 8 MQTLs regions were identified including 321 genes of unknown function (Supplementary Table 3). There were numerous genes and/or gene families with related functions in various MQTL intervals (Figure 6) which included: 69 F-box-like domain, 15 AP2/ERF domain, 2 U-box domain protein, 8 ABC transporter-like, 4 MADs-Box domain-containing protein, 31 DUF domain proteins, 49 zinc finger domain-containing protein, 18 MYB family protein, 1 sugar transporter, 17 cytochrome P450, 1 Lysm domain-containing protein, 20 pentatricopeptide repeat protein family, 4 protein phosphatase 2C family, 21 ubiquitin ligase domain protein, 22 auxin-response family protein, 5 DEAD-box-ATP families, 27 receptor-like kinase/OsWAK family, 1 PHD finger protein, 4 UDP-glucose pyrophosphorylase protein, and 8 homeobox domain-containing protein (Figure 6). These genes were chosen for more detailed study on the basis that they encoded proteins directly associated with grain yield. Clusters of genes with similar functions were also identified in some MQTL regions which included: (i) F-box protein, (ii) prolamin precursor, expressed, (iii) ubiquitin carboxyl-terminal hydrolase, family 1, (iv) retrotransposons, and (v) zinc fingers (Supplementary Table 3).
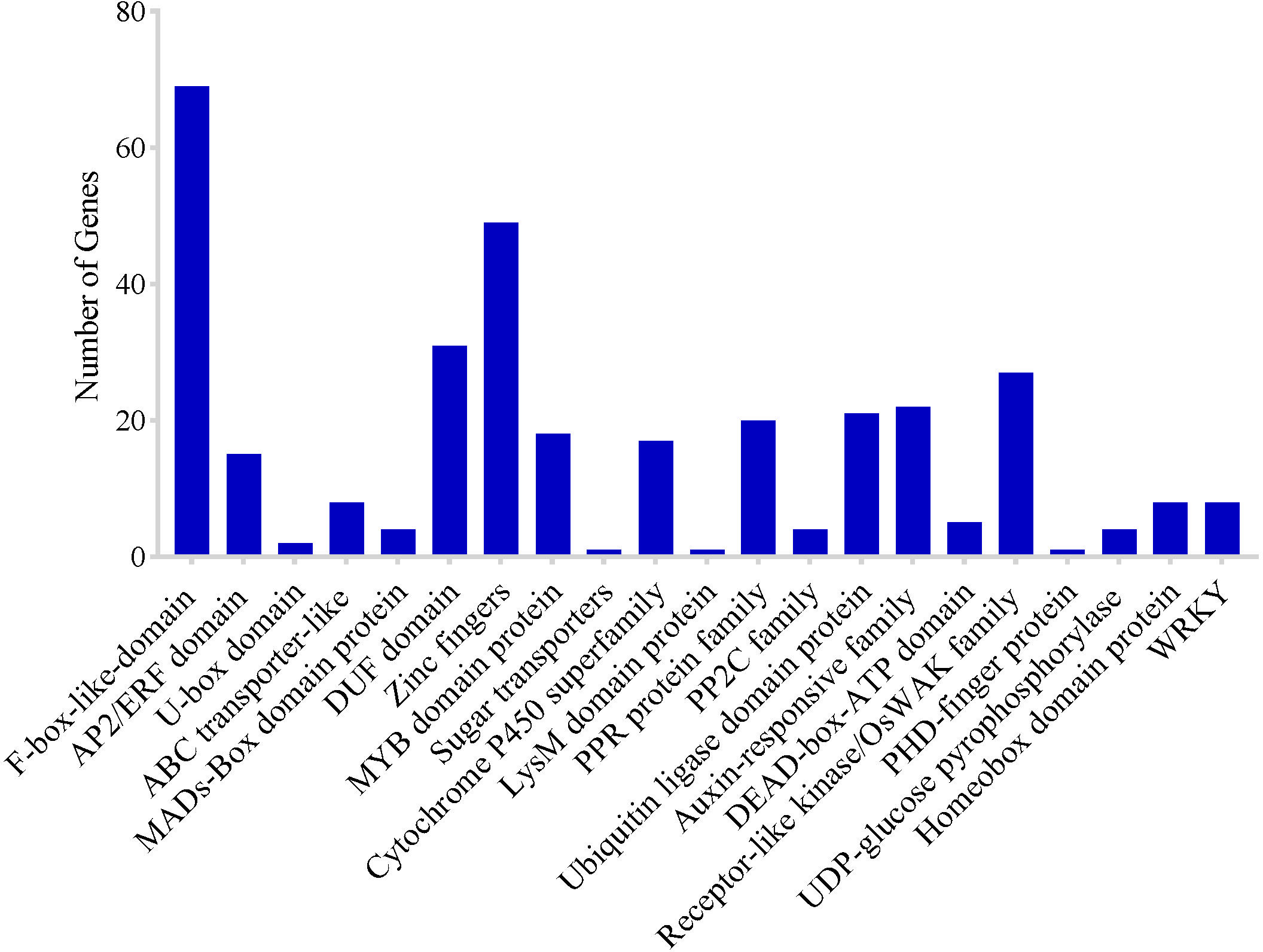
Figure 6 Frequency of candidate genes encoding known protein families related to grain yield of rice.
Gene Ontology (GO) and expression of CGs
Based on the GO enrichment analysis, 1,139 out of 2,030 CGs were annotated of which 34 GO terms were significantly enriched (Figure 7). Out of the 34 GO terms, 13, 10, and 11 GO terms were significantly enriched in biological process, cellular component, and molecular function, respectively. The most enriched GO terms in the biological process category were: metabolic process, cellular process, and single-organism process. For the cellular component, the most enriched GO terms were: cell, cell part, organelle, and membrane. High percentages of genes were enriched in the molecular process category which included binding and catalytic activity (Figure 7). These results indicate the significant association of these terms with grain yield in rice. The CGs significantly enriched during GO analysis were further subjected to protein-protein interaction to predict their relationships. Based on the analysis, six key interactions were determined, including an interaction between LOC_Os04g43410 and 109 genes, LOC_Os04g43380 and 101 genes, LOC_Os02g56130 and 43 genes, LOC_Os01g13520 and 33 genes, LOC_Os02g55410 and 29 genes, and LOC_Os12g26060 and 25 genes (Figure 8), respectively.
To discover potential CGs underlying the promising MQTLs genomic regions, we focused on CGs which encode proteins associated with grain yield in rice (Figure 6). A total of 327 CGs were identified to belong to the above proteins. Moreover, the RNA-seq data of genes that is publicly available on the rice genome annotation project database were employed to heatmap the expression of the CGs in pre-emergence inflorescence, post-emergence inflorescence, anther, pistil, seed 10DAP, embryo, seed 5DAP, endosperm, and shoot (Figure 9). Based on the heatmap, 52 of the 327 genes showed high fold change expression in anther, pistil, seed, embryo, and inflorescence (Figure 9 and Table 3); hence, they could be grain yield regulatory genes. The MQTL1.1 possessed the highest number of potential CGs followed by MQTL4.2, MQTL2.6, MQTL5.5, MQTL5.2, and MQTL12.2. However, MQTL3.5 and MQTL10.2 had the lowest number of genes with high expression in yield-related tissues (Table 3).
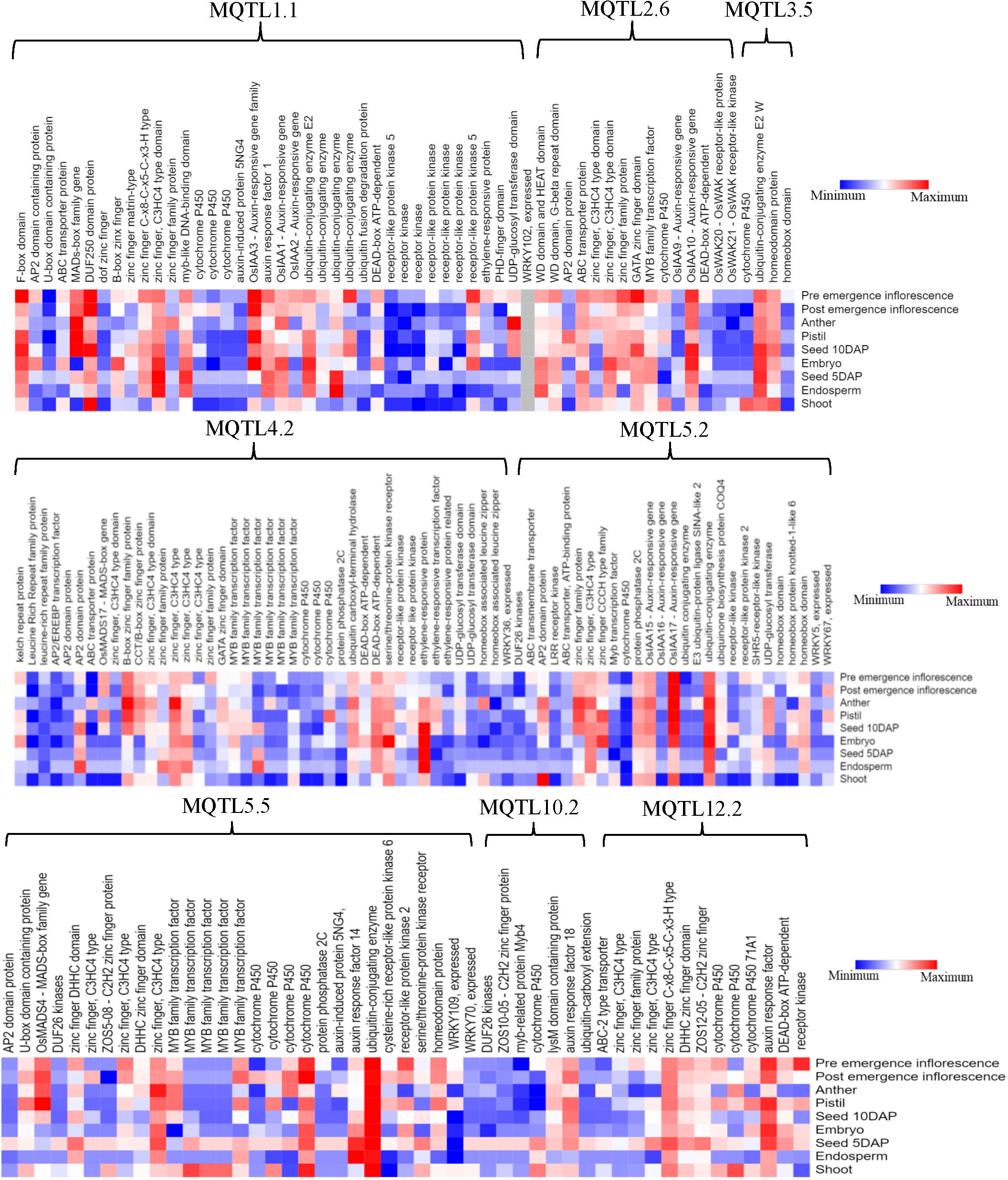
Figure 9 Expression profile of significant proteins encoded by 176 candidate genes underlying the following 8 MQTLs regions: MQTL1.1, MQTL2.6, MQTL3.5, MQTL4.2, MQTL5.2, MQTL5.5, MQTL10.2, and MQTL12.2.
Discussion
Increasing the productivity of rice is one of the foremost constraints rice breeders face to ensure adequate food supply to a rapidly growing global population. Most important agronomic traits such as spikelet fertility, number of grains per panicle, panicle number per plant, and thousand-grain weight are complex and modulated by numerous polygenic loci. Over the years, the success in the introgression of QTLs in breeding programs by marker-assisted selection has been slow due to inconsistency in QTL genetic backgrounds and phenotype environment. Integration of QTLs information from diverse independent studies and exploiting meta-analysis to identify MQTL regions has proven to be an ideal approach to accelerating QTLs fine mapping and gene cloning (Wang et al., 2013; Wang et al., 2015; Martinez et al., 2016; Wu et al., 2016; Chen et al., 2017).
To decipher the molecular mechanism underlying grain yield, MQTL analysis was conducted based on the QTLs conferring grain yield collected from previous independent studies. The initial step in the meta-analysis of QTL is the QTL projection onto the reference map. A characteristics of the reference map utilized in the current study showed that the highest marker saturation was reported on chromosome 1 followed by chromosomes 3 and 2 with the lowest marker density found on chromosome 10. Individual chromosomes had uneven marker distribution, and the density of markers at either end of the chromosome differed. This is primarily due to the use of independent genetic maps with different marker types and number of markers. Interestingly, similar cases have been reported in earlier studies on meta-analysis of QTLs (Venske et al., 2019; Liu et al., 2020; Saini et al., 2021; Yang et al., 2021; Saini et al., 2022). More so, the QTLs investigated were not evenly distributed across the entire rice chromosomes. Chromosomes 1, 2, 3, and 5 reported the highest number of QTLs which is consistent with the previous studies (Xing and Zhang, 2010; Swamy and Sarla, 2011; Swamy et al., 2011; Wu et al., 2016; Khahani et al., 2020). However, chromosomes 8, 9, and 10 reported the lowest number of QTLs. The possible reason for this phenomenon could be the low level of polymorphism associated with these chromosomes. Out of the total QTLs projected, only 32.47% constitute PVE >10%, indicating that the individual QTLs contributed very little to phenotypic variability. This shows that grain yield is primarily determined by minor effects loci, representing a complex genetic architecture.
The MQTL analysis identifies stable and robust QTLs irrespective of the genetic background of mapping population, phenotype environment, and marker density which are the main constraints of QTL mapping (Arcade et al., 2004; Zhang et al., 2010; Daware et al., 2017; Bilgrami et al., 2018; Khahani et al., 2019). Further, association mapping approach has also been shown as an alternative instrument with better accuracy compared to QTL mapping of complex agronomic traits (Daware et al., 2017). However, this method faces remarkable false positive outcomes because of the population structure employed in the analysis (Daware et al., 2017). Owing to this, analysis of MQTL is regarded as the most reliable approach for detection of stable loci modulating quantitative traits. In this study, a total of 313 QTLs were confined into 62 MQTLs across the 12 chromosomes for the evaluated traits. Chromosome 3 reported the highest number of MQTLs (9 MQTLs) and the least MQTL were located on chromosome 8 (2 MQTLs). Intriguingly, our findings corroborate with a previous study on MQTLs in rice (Khahani et al., 2021). More than 80% (50/62) of MQTLs harbored at least two of the initial QTLs. Furthermore, some of the MQTLs contained as high as 10 initial QTLs exhibiting MQTLs robustness. The reference map developed and utilized in this study is much more informative compared to those employed in the previous studies (Wu et al., 2016; Khahani et al., 2020; Khahani et al., 2021). We also found that six out of the total MQTLs identified had common genomic positions with previously reported MQTLs (Wu et al., 2016; Sandhu et al., 2021). This includes regions on chromosomes 2 (MQTL2.3), 4 (MQTL4.1, MQTL4.2), 7 (MQTL7.2), 9 (MQTL9.1), and 12 (MQTL12.1). It is worth noting that, the average confidence interval of MQTLs remarkably reduced up to 3.4 folds times compared to the confidence interval of original QTLs. Our study clearly shows that the meta-analysis is effective in locating consensus and exact QTLs. Previous studies on meta-analysis in wheat, rice, maize, soybean, and cotton, reported 10% to 21% reduction in the total QTLs with two to four times reduction in the average confidence interval of original QTLs (Guo et al., 2006; Rong et al., 2007; Ballini et al., 2008; Courtois et al., 2009; Lanaud et al., 2009; Löffler, 2009; Khahani et al., 2020). MQTLs identified were compared with GWAS studies via the Rice SNP-seek database which led to the identification of common significant regions. Consequently, 16 (25.81%) important GWAS peaks for spikelet fertility (SF) and 1000-grain weight (TGW) were co-located with our MQTLs. Our results demonstrate the coherence of the two approaches in pinpointing common loci associated with the studied traits. However, there are also reports which contradict the result in the present study with 63%, 61.3% and 38.66% MQTLs validated with GWAS (Yang et al., 2021; Amo and Soriano, 2022; Saini et al., 2022). These phenomena may be ascribed to the material utilized for initial QTL mapping (finally used for MQTL analysis) and GWAS.
Identification of underlying genes in genomic regions of major QTLs is of great interest in breeding programs. More so, MQTLs with narrow and precise physical regions are beneficial in candidate gene mining. According to Kaur et al. (2021), MQTLs with 5-8 initial QTL clusters with moderately high R2 values could be considered important for mining candidate genes. The genes present in the 8 MQTLs regions were analyzed using rice databases (http://www.ricedata.cn, http://shigen.nig.ac/jp/rice/oryzabase/, and http://rice.uga.edu.cgi-bin/gbrowse/rice). We identified 2,030 genes and as many as 327 genes were found to encode proteins (sugar transporters, PPR repeat-containing protein-like genes, cytochrome P450, zinc fingers, F-box genes, PP2C family, MADs-Box domain-containing protein, AP2 domain-containing protein, and cytokinase oxidase genes) associated with yield (Wu et al., 2016; Chen et al., 2020; Jiang et al., 2022).
Several genes of unknown functions were also found in stable MQTLs intervals; further study is necessary to explore the functional role of those genes in regulating grain yield. A bioinformatics pipeline implementing the genomic sequences of stable MQTLs was used to identify potential candidate genes. These pipelines involved three steps, thus retrieving candidate genes from rice genome project annotation database, visualizing the molecular function of candidate genes by GO enrichment analysis, and implying candidate genes in rice yield-related tissues based on their expression pattern. Consequently, 52 genes were considered potential candidate genes (Table 3). Most of the identified candidate genes are associated with the terms of binding, catalytic activity, cell, cell part, cellular, and metabolic process. These terms have functions related to yield (Mao et al., 2010; Li and Li, 2014; Elattar et al., 2021). In the stable MQTL regions, most of the candidate genes encode zinc finger family protein, ubiquitin ligase domain-containing protein, auxin-responsive gene family, AP2 domain-containing protein, cytochrome P450, receptor-like kinase protein, and MADs-box family protein. Furthermore, zinc fingers have been reported to play a vital role in plant growth, development, and response to abiotic stresses (Li et al., 2013). For instance, a zinc finger protein DST regulates the expression of OsCKX2 and increases cytokinin level in reproductive shoot apical meristem, which in turn elevates meristem activity, panicle branching, and increased grain number (Li et al., 2013). Similarly, DHHC-zinc finger protein gene has been reported to regulate plant architecture and grain yield (Zhou et al., 2017). AP2 domain-containing proteins have been reported to play essential roles in regulating plant growth and development. Overexpression of OsAP2-39 decreased biomass and grain yield in transgenic lines of rice (Yaish et al., 2010). Overexpression of OsIAA10 (auxin-responsive gene in MQTL2.6) was reported to increase rice susceptibility to rice dwarf virus (Jin et al., 2016; Qin et al., 2020). One reported gene, OsBIHD1 (encodes homeodomain protein, putative, expressed) was found in MQTL3.5. Overexpression of OsBIHD1 revealed an increased salt sensitivity and oxidative stress in rice (Luo et al., 2005). In addition, a clone gene Oshox22 (encodes homeobox associated leucine zipper, putative, expressed) was identified in MQTL4.2. Overexpression of Oshox22 increased ABA sensitivity and reduced salt drought and stress tolerance (Zhang et al., 2012).
In MQTL5.2 lies two reported genes, RSR1 and OsAPIP6. The overexpression of RSR1 resulted in the change of amylopectin and starch gelatinization (Fu and Xue, 2010). OsAPIP6 interacted with AvrPiz-t in vitro and reduced flg22-induced ROS generation, suppressed defense gene expression, and increased rice plant susceptibility to M. Oryza (Park et al., 2012). We also found two reported genes, OsPUB44 (encodes U-box domain-containing protein, expressed) and OsMADS4 (encodes MADS-box family gene with MIKCc type-box) in MQTL5.5. OsPUB44 positively control immune responses of rice plant to bacterial blight (Ishikawa et al., 2014). In another study, U-box domain family protein has been reported to play a vital role in rice pollen development (Chen et al., 2019). A mutant of OsPUB73 (U-box domain protein) showed low pollen fertility suggesting that OsPUB73 was responsible for pollen exine or tapetal development and led to pollen partial sterility (Chen et al., 2019). The genes, OsMADS4 interacted with rice AP3 orthologs SPW1 and facilitate stamen and lodicule development (Yao et al., 2008). In previous studies, MADs box genes have been implicated to modulate stamen development in rice (Yamaguchi et al., 2006; Hu et al., 2011; Yan et al., 2015; Wei et al., 2019), arabidopsis (Ito et al., 2004; Ito et al., 2007), and maize (Schreiber et al., 2004). Two genes, CYP94C2b (cytochrome P450, putative, expressed) and OsARF24 (auxin response factor, putative, expressed) underlie MQTL 12.2 have been reported to enhance salt tolerance in rice (Li et al., 2014) and control cell growth (Kurotani et al., 2015).
Conclusion
In the present study, we integrated QTLs conferring spikelet fertility, panicle number per plant, thousand-grain weight, and number of grains per panicle which led to the identification of 62 MQTLs. 16 of these MQTLs were successfully verified with GWAS studies on the studied traits. 10 MQTLs that harbored at least six of the initial QTLs were selected as the most stable and robust chromosome regions to mine CGs and positional cloning that could be useful for breeding programs. Based on the bioinformatics pipelines, 52 potential CGs were identified to be associated with grain yield. The high expression of these genes was evidenced in yield-related tissues and maybe grain yield regulatory genes. This study provides a useful framework for genetic dissection of grain yield under unstressed conditions via positional cloning, fine mapping, and CGs validation by gene editing technique. The remaining novel CGs detected in this study could be validated or functionally characterized via gene editing, overexpression, knockout techniques, and candidate gene-based association mapping to ascertain their roles in regulating grain yield in future studies.
Data availability statement
The original contributions presented in the study are included in the article/Supplementary Material. Further inquiries can be directed to the corresponding author.
Author contributions
XT, NEO, and KDA conceptualized and design the study. XT supervised the study. KDA, AA, and NEO curated data and perform the analysis. KDA wrote the manuscript. SFB, SA, and AA revised and edited the manuscript. All authors have read and approved the final version of the manuscript.
Funding
This study was supported by The Key R&D Project in Hubei Province, China (2020BBB060).
Conflict of interest
The authors declare that the research was conducted in the absence of any commercial or financial relationships that could be construed as a potential conflict of interest.
Publisher’s note
All claims expressed in this article are solely those of the authors and do not necessarily represent those of their affiliated organizations, or those of the publisher, the editors and the reviewers. Any product that may be evaluated in this article, or claim that may be made by its manufacturer, is not guaranteed or endorsed by the publisher.
Supplementary material
The Supplementary Material for this article can be found online at: https://www.frontiersin.org/articles/10.3389/fpls.2022.1035851/full#supplementary-material
References
Acuña-Galindo, M. A., Mason, R. E., Subramanian, N. K., Dirk, B. H. (2015). Meta-analysis of wheat QTL regions associated with adaptation to drought and heat stress. Crop Sci. 55, 477–492. doi: 10.2135/cropsci2013.11.0793
Ahmed, E. Y. M., Zhang, Y. P., Yu, J. P., Rehman, R. M. A., Zhang, Z. Y., Zhang, H. L., et al. (2016). Mapping of three QTLs for seed setting and analysis on the candidate gene for qSS-1 in rice (Oryza sativa l). J. Int. Agric. 15, 735–743. doi: 10.1016/S2095-3119(15)61299-0
Amo, A., Soriano, J. M. (2022). Unravelling consensus genomic regions conferring leaf rust resistance in wheat via meta-QTL analysis. Plant Genome. 15, e20185. doi: 10.1002/tpg2.20185
Aquib, A., Nafis, S. (2021). Identifying meta-QTLs for stay-green in sorghum. bioRxiv. 4–11, 1–39. doi: 10.1101/2021.08.06.455413
Arcade, A., Aymeric, L., Matthieu, F., Brigitte, M., Fabien, C., Alain, C., et al. (2004). BioMercator: Integrating genetic maps and QTL towards discovery of candidate genes. Bioinformatics. 20, 2324–2326. doi: 10.1093/bioinformatics/bth230
Bai, X. F., Luo, L. J., Yan, W., Kovi, M. R., Xing, Y. Z. (2011). Quantitative trait loci for rice yield-related traits using recombinant inbred lines derived from two diverse cultivars. J. Genet. 90, 209–215. doi: 10.1007/s12041-011-0057-y
Bai, X., Wu, B., Xing, Y. (2012). Yield-related QTLs and their applications in rice genetic improvement. J. Integr. Plant Biol. 54, 300–311. doi: 10.1111/j.1744-7909.2012.01117.x
Ballini, E., Morel, J. B., Droc, G., Price, A., Courtois, B., Notteghem, J., et al. (2008). A genome-wide meta-analysis of rice blast resistance genes and quantitative trait loci provides new insights into partial and complete resistance. Mol. Plant Microbe Interact. 21, 859–868. doi: 10.1094/MPMI-21-7-0859
Beerelli, K., Balakrishnan, D., Addanki, K. R., Surapaneni, M., Rao, V. Y., Neelamraju, S. (2022). Mapping of QTLs for yield traits using F2:3:4 populations derived from two alien introgression lines reveals qTGW8.1 as a consistent qtl for grain weight from Oryza nivara. Front. Plant Sci. 13. doi: 10.3389/fpls.2022.790221
Bilgrami, S. S., Fakheri, B. A., Shariati, J. V., Razavi, K., Mahdinezhad, N., Tavakol, E., et al. (2018). Evaluation of agro-morphological traits related to grain yield of iranian wheat genotypes in drought-stress and normal irrigation conditions. Aust. J. Crop Sci. 12, 738–748. doi: 10.21475/ajcs.18.12.05.PNE878
Cao, L. Y., Wu, J. L., Fan, Y. Y., Cheng, S. H., Huang, J. Y. Z. (2010). QTL analysis for heading date and yield traits using recombinant inbred lines of indica rice grown in different cropping seasons. Plant Breed. 129, 676–682. doi: 10.1111/j.1439-0523.2010.01772.x
Chardon, F., Virlon, B., Moreau, L., Falque, M., Joets, J., Decousset, L., et al. (2004). Genetic architecture of flowering time in maize as inferred from quantitative trait loci meta-analysis and synteny conservation with the rice genome. Genetics. 168, 2169–2185. doi: 10.1534/genetics.104.032375
Chen, L., An, Y., Li, Y. X., Li, C., Shi, Y., Song, Y. (2017). Candidate loci for yield-related traits in maize revealed by a combination of meta-QTL analysis and regional association mapping. Front. Plant Sci. 8. doi: 10.3389/fpls.2017.02190
Chen, L., Deng, R., Liu, G., Jin, J., Wu, J., Liu, X. (2019). Cytological and transcriptome analyses reveal OsPUB73 defect affects the gene expression associated with tapetum or pollen exine abnormality in rice. BMC Plant Biol. 19, 546. doi: 10.1186/s12870-019-2175-2
Chen, Z., Shen, Z., Xu, L., Zhao, D., Zou, Q. (2020). Regulator network analysis of rice and maize yield-related genes. Front. Cell Dev. Biol. 8. doi: 10.3389/fcell.2020.621464
Chin, J. H., Chu, S. H., Jiang, W., Cho, Y., Basyirin, R., Brar, D. S., et al. (2011). Identification of QTLs for hybrid fertility in inter-subspecific crosses of rice (Oryza sativa l). Genes Genom. 33, 39–48. doi: 10.1007/s13258-010-0100-z
Courtois, B., Ahmadi, N., Khowaja, F., Price, A. H., Rami, J. F., Frouin, J., et al. (2009). Rice root genetic architecture: Meta-analysis from a drought QTL database. Rice. 2, 115–128. doi: 10.1007/s12284-009-9028-9
Darvasi, A., Soller, M. (1997). A simple method to calculate resolving power and confidence interval of QTL map location. Behav.Genet. 27, 125–132. doi: 10.1023/A:1025685324830
Darzi-Ramandi, H., Vahid Shariati, J., Tavakol, E., Najafi-Zarini, H., Bilgrami, S. S., Razavi, K. (2017). Detection of consensus genomic regions associated with root architecture of bread wheat on groups 2 and 3 chromosomes using qtl meta-analysis. Aust. J. Crop Sci. 11, 777–785. doi: 10.21475/ajcs.17.11.07.pne312
Daware, A. V., Srivastava, R., Singh, A. K., Parida, S. K., Tyagi, A. K. (2017). Regional association analysis of metaqtls delineates candidate grain size genes in rice. Front. Plant Sci. 8. doi: 10.3389/fpls.2017.00807
Deshmukh, R., Singh, A., Jain, N., Anand, S., Gacche, R., Singh, A., et al. (2010). Identification of candidate genes for grain number in rice (Oryza sativa l). Funct. Integr. Genomics 10, 339–347. doi: 10.1007/s10142-010-0167-2
Elattar, M. A., Karikari, B., Li, S., Song, S., Cao, Y., Aslam, M., et al. (2021). Identification and validation of major qtls, epistatic interactions, and candidate genes for soybean seed shape and weight using two related RIL populations. Front. Genet. 12. doi: 10.3389/fgene.2021.666440
Endelman, J. B., Plomion, C. (2014). LPmerge: An r package for merging genetic maps by linear programming. Bioinformatics 30, 1623–1624. doi: 10.1093/bioinformatics/btu091
Feng, Y., Yuan, X., Wang, Y., Yang, Y., Zhang, M., Yu, H., et al. (2021). Validation of a QTL for grain size and weight using an introgression line from a cross between Oryza sativa and Oryza minuta. Rice 14, 43. doi: 10.1186/s12284-021-00472-1
Fu, F. F., Xue, H. W. (2010). Coexpression analysis identifies rice starch regulator1, a rice AP2/EREBP family transcription factor, as a novel rice starch biosynthesis regulator. Plant Physiol. 154, 927–938. doi: 10.1104/pp.110.159517
Fu, Q., Zhang, P., Tan, L., Zhu, Z., Ma, D., Fu, Y., et al. (2010). Analysis of QTLs for yield-related traits in yuanjiang common wild rice (Oryza rufipogon griff). J. Genet. Genomics 37, 147–157. doi: 10.1016/S1673-8527(09)60033-3
Gao, F. Y., Zeng, L. H., Qiu, L., Lu, X. J., Ren, J. S., Wu, X. T. (2016). QTL mapping of grain appearance quality traits and grain weight using a recombinant inbred population in rice (Oryza sativa l). J. Integr. Agric. 15, 1693–1702. doi: 10.1016/S2095-3119(15)61259-X
Goffinet, B., Gerber, S. (2000). Quantitative trait loci: A meta-analysis. Genetics. 155, 463–473. doi: 10.1093/genetics/155.1.463
Guo, B., Sleper, D. A., Lu, P., Shannon, J. G., Nguyen, H. T., Arelli, P. R. (2006). QTLs associated with resistance to soybean cyst nematode in soybean: Meta-analysis of QTL locations. Crop Sci. 46, 595–602. doi: 10.2135/cropsci2005.04-0036-2
Hittalmani, S., Huang, N., Courtois, B., Venuprasad, R., Shashidhar, H. E., Zhuang, J. Y., et al. (2003). Identification of QTL for growth- and grain yield-related traits in rice across nine locations of Asia. Theor. Appl. Genet. 107, 679–690. doi: 10.1007/s00122-003-1269-1
Huang, R., Jiang, L., Zheng, J., Wang, T., Wang, H., Huang, Y., et al. (2013). Genetic bases of rice grain shape: so many genes, so little known. Trends Plant Sci. 18, 218–226. doi: 10.1016/j.tplants.2012.11.001
Hu, Z., Cao, L., Sun, X., Zhu, Y., Zhang, T., Jiang, L., et al. (2018). Fine mapping of a major quantitative trait locus, qgnp7(t), controlling grain number per panicle in African rice (Oryza glaberrima s). Breed Sci. 68, 606–613. doi: 10.1270/jsbbs.18084
Hu, L., Liang, W., Yin, C., Cui, X., Zong, J., Wang, X., et al. (2011). Rice MADS3 regulates ROS homeostasis during late anther development. Plant Cell. 23, 515–533. doi: 10.1105/tpc.110.074369
Hwang, S., King, C. A., Chen, P., Ray, J. D., Cregan, B. P., Carter, E. T., et al. (2016). Meta-analysis to refine map position and reduce confidence intervals for delayed-canopy-wilting QTLs in soybean. Mol. Breeding. 36, 1–14. doi: 10.1007/s11032-016-0516-5
Ishikawa, K., Yamaguchi, K., Sakamoto, K., Yoshimura, S., Inoue, K., Tsuge, S., et al. (2014). Bacterial effector modulation of host E3 ligase activity suppresses PAMP-triggered immunity in rice. Nat. Commun. 5, 5430. doi: 10.1038/ncomms6430
Ito, T., Ng, K. H., Lim, T. S., Yu, H., Meyerowitz, M. E. (2007). The homeotic protein AGAMOUS controls late stamen development by regulating a jasmonate biosynthetic gene in arabidopsis. Plant Cell. 19, 3516–3529. doi: 10.1105/tpc.107.055467
Ito, T., Wellmer, F., Yu, H., Das, P., Ito, N., Alves-Ferreira, M., et al. (2004). The homeotic protein AGAMOUS controls microsporogenesis by regulation of sporocyteless. Nature. 430, 356–360. doi: 10.1038/nature02733
Jiang, H., Zhang, A., Liu, X., Chen, J. (2022). Grain size associated genes and the molecular regulatory mechanism in rice. Int. J. Mol. Sci. 23, 3169. doi: 10.3390/ijms23063169
Jia, B., Zhao, X., Qin, Y., Irfan, M., Kim, T. H., Wang, B., et al. (2019). Quantitative trait loci mapping of panicle traits in rice. Mol. Biol. Res. Commun. 8, 9–15. doi: 10.22099/mbrc.2019.31550.1366
Jin, L., Qin, Q., Wang, Y., Pu, Y., Liu, L., Wen, X., et al. (2016). Rice dwarf virus P2 protein hijacks auxin signaling by directly targeting the rice OsIAA10 protein, enhancing viral infection and disease development. PloS Pathog. 12, e1005847. doi: 10.1371/journal.ppat.1005847
Kaur, S., Rakshit, S., Choudhary, M., Das, A. K., Kumar, R. (2021). Meta-analysis of QTLs associated with popping traits in maize (Zea mays l). PloS One 16, e0256389. doi: 10.1371/journal.pone.0256389
Kawahara, Y., de la Bastide, M., Hamilton, J. P., Kanamori, H., Mccombie, W. R., Ouyang, S., et al. (2013). Improvement of the Oryza sativa nipponbare reference genome using next generation sequence and optical map data. Rice 6, 4. doi: 10.1186/1939-8433-6-4
Khahani, B., Tavakol, E., Shariati, V. (2019). Genome-wide meta-analysis on yield and yield-related QTLs in barley (Hordeum vulgare l). Mol. Breeding. 39, 56. doi: 10.1007/s11032-019-0962-y
Khahani, B., Tavakol, E., Shariati, V., Fornara, F. (2020). Genome wide screening and comparative genome analysis for meta-QTLs, ortho-MQTLs and candidate genes controlling yield and yield-related traits in rice. BMC Genomics 21, 294. doi: 10.1186/s12864-020-6702-1
Khahani, B., Tavakol, E., Shariati, V., Rossini, L. (2021). Meta-QTL and ortho-MQTL analyses identified genomic regions controlling rice yield, yield-related traits and root architecture under water deficit conditions. Sci. Rep. 11, 6942. doi: 10.1038/s41598-021-86259-2
Kim, C. K., Chu, S. H., Park, H. Y., Seo, J., Kim, B., Lee, G., et al. (2017). Identification of heterosis QTLs for yield and yield-related traits in indica-japonica recombinant inbred lines of rice (Oryza sativa l). Plant breed. Biotech. 5, 371–389. doi: 10.9787/PBB.2017.5.4.371
Kulkarni, S. R., Balachandran, S. M., Ulaganathan, K., Balakrishnan, D., M. Praveen, A., Prasad, S. H., et al. (2020). Molecular mapping of QTLs for yield related traits in recombinant inbred line (RIL) population derived from the popular rice hybrid KRH-2 and their validation through SNP genotyping. Sci. Rep. 10, 13695. doi: 10.1038/s41598-020-70637-3
Kurotani, K. I., Hayashi, K., Hatanaka, S., Toda, Y., Ogawa, D., Ichikawa, H., et al. (2015). Elevated levels of CYP94 family gene expression alleviate the jasmonate response and enhance salt tolerance in rice. Plant Cell Physio. 56, 779–789. doi: 10.1093/pcp/pcv006
Kwon, S. J., Cho, Y. C., Kwon, S. W., Oh, C. S., Suh, J. P., Hwang, H. G., et al. (2008). QTL mapping of agronomic traits using an RIL population derived from a cross between temperate japonica cultivars in rice (Oryza sativa l.). Breed. Sci. 58, 271–279. doi: 10.1270/jsbbs.58.271
Lanaud, C., Fouet, O., Clément, D., Boccara, M., Risterucci, A. M., Surujdeo-Maharaj, S., et al. (2009). A meta–QTL analysis of disease resistance traits of Theobroma cacao l. Mol. Breeding. 24, 361–374. doi: 10.1007/s11032-009-9297-4
Liang, Y., Zhan, X., Gao, Z., Lin, Z., Yang, Z., Zhang, Y., et al. (2012). Mapping of QTLs associated with important agronomic traits using three populations derived from super hybrid rice Xieyou9308. Euphytica. 184, 1–13. doi: 10.1007/s10681-011-0456-4
Li, G., Cheng, Y., Yin, M., Yang, J., Ying, J., Zhu, C. (2021). Detection of QTLs for panicle-related traits using an indica × japonica recombinant inbred line population in rice. PeerJ. 9, e12504. doi: 10.7717/peerj.12504
Li-jun, Z., Ling, J., Xi, L., Hong, C., Liang-ming, C., Shi-jia, L., et al. (2009). Mapping and interaction of QTLs for thousand-grain weight and percentage of grains with chalkiness in rice. Acta Agron. Sin. 35, 255–261. doi: 10.1016/S1875-2780(08)60061-9
Li, N., Li, Y. (2014). Ubiquitin-mediated control of seed size in plants. Front. Plant Sci. 5. doi: 10.3389/fpls.2014.00332
Li, G., Liang, W., Zhang, X., Ren, H., Hu, J., Bennett, M. J., et al. (2014). Rice actin-binding protein RMD is a key link in the auxin–actin regulatory loop that controls cell growth. Proc. Natl. Acad. Sci. U.S.A. 111, 10377–10382. doi: 10.1073/pnas.1401680111
Li, R., Li, M., Ashraf, U., Liu, S., Zhang, J. (2019). Exploring the relationships between yield and yield-related traits for rice varieties released in china from 1978 to 2017. Front. Plant Sci. 10. doi: 10.3389/fpls.2019.00543
Liu, T., Mao, D., Zhang, S., Xu, C., Xing, Y. (2009). Fine mapping SPP1, a QTL controlling the number of spikelets per panicle, to a BAC clone in rice (Oryza sativa). Theor. Appl. Genet. 118, 1509–1517. doi: 10.1007/s00122-009-0999-0
Liu, Y., Salsman, E., Wang, R., Galagedara, N., Zhang, Q., Fiedler, J. D., et al. (2020). Meta-QTL analysis of tan spot resistance in wheat. Theor. Appl. Genet. 133, 2363–2375. doi: 10.1007/s00122-020-03604-1
Li, X., Wei, Y., Li, J., Yang, F., Chen, Y., Chen, Y., et al. (2020). Identification of QTL TGW12 responsible for grain weight in rice based on recombinant inbred line population crossed by wild rice Oryza minuta introgression line K1561 and indica rice G1025. BMC Genet. 21, 10. doi: 10.1186/s12863-020-0817-x
Li, S., Zhao, B., Yuan, D., Duan, M., Qian, Q., Tang, L., et al. (2013). Rice zinc finger protein DST enhances grain production through controlling Gn1a/OsCKX2 expression. Proc. Natl. Acad. Sci. U.S.A. 110, 3167–3172. doi: 10.1073/pnas.1300359110
Löffler, M. (2009). Revealing the genetic architecture of FHB resistance in hexaploid wheat Triticum aestivum l.by QTL. Mol. Breeding. 23, 473–488. doi: 10.1007/s11032-008-9250-y
Lu, Q., Liu, H., Hong, Y., Li, H., Liu, H., Li, X., et al. (2018). Consensus map integration and QTL meta- analysis narrowed a locus for yield traits to 0.7 cM and refined a region for late leaf spot resistance traits to 0.38 cM on linkage group A05 in peanut (Arachis hypogaea l). BMC Genomics 19, 887. doi: 10.1186/s12864-018-5288-3
Luo, H., Song, F., Zheng, Z. (2005). Overexpression in transgenic tobacco reveals different roles for the rice homeodomain gene OsBIHD1 in biotic and abiotic stress responses. J. Exp. Bot. 56, 2673–2682. doi: 10.1093/jxb/eri260
Mansueto, L., Fuentes, R. R., Borja, F. N., Detras, J., Abrio-Santos, J. M., Chebotarov, D., et al. (2017). Rice SNP-seek database update: New SNPs, InDels, and queries. Nucleic Acids Res. 45, D1075–D1081. doi: 10.1093/nar/gkw1135
Mao, H., Sun, S., Yao, J., Wang, C., Yu, S., Xu, C., et al. (2010). Linking differential domain functions of the GS3 protein to natural variation of grain size in rice. Proc. Natl. Acad. Sci. U.S.A. 107, 19579–19584. doi: 10.1073/pnas.1014419107
Marathi, B., Guleria, S., Mohapatra, T., Parsad, R., Mariappan, N., Kurungara, V. K., et al. (2012). QTL analysis of novel genomic regions associated with yield and yield related traits in new plant type based recombinant inbred lines of rice (Oryza sativa l). BMC Plant Biol. 12, 137. doi: 10.1186/1471-2229-12-137
Marri, P. R., Sarla, N., Reddy, L. V., Siddiq, E. A. (2005). Identification and mapping of yield and yield related QTLs from an indian accession of Oryza rufipogon. BMC Genet. 6, 33. doi: 10.1186/1471-2156-6-33
Martinez, A. K., Soriano, J. M., Tuberosa, R., Koumproglou, R., Jahrmann, T., Salvi, S. (2016). Yield QTLome distribution correlates with gene density in maize. Plant Sci. 242, 300–309. doi: 10.1016/j.plantsci.2015.09.022
Miao, Y., Jing, F., Ma, J., Liu, Y., Zhang, P., Chen, T., et al. (2022). Major genomic regions for wheat grain weight as revealed by QTL linkage mapping and meta-analysis. Front. Plant Sci. 13. doi: 10.3389/fpls.2022.802310
Niu, X., Zhu, Y., Sun, Z., Yu, S., Zhuang, J., Fan, Y. (2020). Identification and validation of quantitative trait loci for grain number in rice (Oryza sativa l). Agronomy 10, 180. doi: 10.3390/agronomy10020180
Park, C. H., Chen, S., Shirsekar, G., Zhou, B., Khang, C. H., Songkumarn, P., et al. (2012). The Magnaporthe oryzae effector avrpiz-t targets the RING E3 ubiquitin ligase APIP6 to suppress pathogen-associated molecular pattern-triggered immunity in rice. Plant Cell. 24, 4748–4762. doi: 10.1105/tpc.112.105429
Qiao, Y., Jiang, W., Rahman, L., Chu, S. H., Piao, R., Han, L. (2008). Two reciprocal backcross populations of rice and comparison of molecular linkage maps and QTLs for morphological traits in two reciprocal backcross populations of rice. Mol. Cells 25, 417–427.
Qing-Quan, C., Si-Bin, Y., Chun-Hai, L., Tong-Min, M., Qing-Quan, C. (2007). QTL identification for seed setting rate of rice in various environments. Chin. J. Agric. Biotech. 4, 239–245. doi: 10.1017/S1479236207001970
Qin, Q., Li, G., Jin, L., Huang, Y., Wang, Y., Wei, C., et al. (2020). Auxin response factors (ARFs) differentially regulate rice antiviral immune response against rice dwarf virus. PloS Pathog. 16, e1009118. doi: 10.1371/journal.ppat.1009118
Qin, H., Liu, Z., Wang, Y., Xu, M., Mao, X., Qi, H., et al. (2018). Meta-analysis and overview analysis of quantitative trait locis associated with fatty acid content in soybean for candidate gene mining. Plant Breed. 137, 181–193. doi: 10.1111/pbr.12562
Rahman, L., Khanam, M. S., Koh, H. J. (2008). QTL analysis for yield related traits using populations derived from an indica-japonica hybrid in rice (Oryza sativa l). Czech J. Genet. Plant Breed. 44, 93–104. doi: 10.17221/16/2008-CJGPB
Rong, J., Feltus, F. A., Waghmare, V. N., Pierce, G. J., Chee, P. W., Draye, X., et al. (2007). Meta-analysis of polyploid cotton QTL shows unequal contributions of subgenomes to a complex network of genes and gene clusters implicated in lint fiber development. Genetics. 176, 2577–2588. doi: 10.1534/genetics.107.074518
Sabouri, H., Sabouri, A., Dadras, A. R. (2009). Genetic dissection of biomass production, harvest index and panicle characteristics in indica-indica crosses of iranian rice (Oryza sativa l). cultivars. Aust. J. Crop Sci. 3, 155–166.
Sabouri, A., Toorchi, M., Rabiei, B., Aharizad, S., Moumeni, A., Singh, R. K. (2010). Identification and mapping of qtls for agronomic traits in indica–indica cross of rice (Oryza sativa l). Cereal Res. Commun. 38, 317–326. doi: 10.1556/CRC.38.2010.3.2
Saini, D. K., Chahal, A., Pal, N., Srivastava, P., Gupta, P. K. (2022). Meta-analysis reveals consensus genomic regions associated with multiple disease resistance in wheat (Triticum aestivum l). Mol. Breeding. 42, 11. doi: 10.1007/s11032-022-01282-z
Saini, D. K., Chopra, Y., Pal, N., Chahal, A., Srivastava, P., Gupta, P. K., et al (2021). Meta-QTLs, ortho-MQTLs and candidate genes for nitrogen use efficiency and root system architecture in bread wheat (Triticum aestivum L.). Physiol Mol Biol Plants. 27, 2245–2267. doi: 10.1007/s12298-021-01085-0
Sakamoto, T., Matsuoka, M. (2008). Identifying and exploiting grain yield genes in rice. Curr. Opin. Plant Biol. 11, 209–214. doi: 10.1016/j.pbi.2008.01.009
Sandhu, N., Pruthi, G., Raigar, O. P., Singh, M. P., Phagna, K., Kumar, A., et al. (2021). Meta-QTL analysis in rice and cross-genome talk of the genomic regions controlling nitrogen use efficiency in cereal crops revealing phylogenetic relationship. Front. Genet. 12. doi: 10.3389/fgene.2021.807210
Schreiber, D. N., Bantin, J., Dresselhaus, T. (2004). The MADS box transcription factor ZmMADS2 is required for anther and pollen maturation in maize and accumulates in apoptotic bodies during anther dehiscence. Plant Physiol. 134, 1069–1079. doi: 10.1104/pp.103.030577
Sekhar, S., Kumar, J., Mohanty, S., Mohanty, N., Panda, R. S., Das, S., et al. (2021). Identification of novel QTLs for grain fertility and associated traits to decipher poor grain filling of basal spikelets in dense panicle rice. Sci. Rep. 11, 13617. doi: 10.1038/s41598-021-93134-7
Semagn, K., Beyene, Y., Warburton, M. L., Tarekegne, A., Mugo, S., Meisel, B., et al. (2013). Meta-analyses of QTL for grain yield and anthesis silking interval in 18 maize populations evaluated under water-stressed and well-watered environments. BMC Genomics 14, 313. doi: 10.1186/1471-2164-14-313
Seo, J., Reflinur, R., Kim, S., Woo, M. O., Koh, H. J. (2014). Identification of quantitative trait loci for agronomic traits in two rice populations derived from a cross with a wide compatibility line. Plant Breed. Biotech. 2, 231–246. doi: 10.9787/PBB.2014.2.3.231
Septiningsih, E. M., Prasetiyono, J., Lubis, E., Tai, T. H., Tjubaryat, T., Moeljopawiro, S., et al. (2003). Identification of quantitative trait loci for yield and yield components in an advanced backcross population derived from the Oryza sativa variety IR64 and the wild relative O. rufipogon. Theor. Appl. Genet. 107, 1419–1432. doi: 10.1007/s00122-003-1373-2
Soriano, J. M., Alvaro, F. (2019). Discovering consensus genomic regions in wheat for root-related traits by QTL meta-analysis. Sci. Rep. 9, 10537. doi: 10.1038/s41598-019-47038-2
Sosnowski, O., Charcosset, A., Joets, J. (2012). Biomercator v3: An upgrade of genetic map compilation and quantitative trait loci meta-analysis algorithms. Bioinformatics. 28, 2082–2083. doi: 10.1093/bioinformatics/bts313
Sun, Z., Yin, X., Ding, J., Yu, D., Hu, M., Sun, X., et al. (2017). QTL analysis and dissection of panicle components in rice using advanced backcross populations derived from Oryza sativa cultivars HR1128 and nipponbare. PloS One 12, e0175692. doi: 10.1371/journal.pone.0175692
Swamy, B. P. M., Kaladhar, K., Reddy, G. A., Viraktamath, B. C., Sarla, N. (2014). Mapping and introgression of qtl for yield and related traits in two backcross populations derived from Oryza sativa cv. swarna and two accessions of O. nivara. J. Genet. 93, 643–654. doi: 10.1007/s12041-014-0420-x
Swamy, B. P. M., Sarla, N. (2011). Meta-analysis of yield QTLs derived from inter-specific crosses of rice reveals consensus regions and candidate genes. Plant Mol. Biol. Rep. 12, 663–680. doi: 10.1007/s11105-010-0274-1
Swamy, B. P. M., Vikram, P., Dixit, S., Ahmed, H. U., Kumar, A. (2011). ). meta-analysis of grain yield qtl identified during agricultural drought in grasses showed consensus. BMC Genomics 12, 319. doi: 10.1186/1471-2164-12-319
Szklarczyk, D., Gable, A. L., Lyon, D., Junge, A., Wyder, S., Huerta-Cepas, J., et al. (2019). STRING v11: Protein-protein association networks with increased coverage, supporting functional discovery in genome-wide experimental datasets. Nucleic Acids Res. 47, D607–D613. doi: 10.1093/nar/gky1131
Takai, T., Fukuta, Y., Shiraiwa, T., Horie, T. (2005). Time-related mapping of quantitative trait loci controlling grain-filling in rice (Oryza sativa l). J. Exp. Bot. 56, 2107–2118. doi: 10.1093/jxb/eri209
Tang, S. Q., Shao, G. N., Wei, X. J., Chen, M. L., Sheng, Z. H., Luo, J., et al. (2013). QTL mapping of grain weight in rice and the validation of the QTL qTGW3.2. Gene. 527, 201–206. doi: 10.1016/j.gene.2013.05.063
Thomson, M. J., Tai, T. H., McClung, A. M., Lai, X. H., Hinga, M. E., Lobos, K. B., et al. (2003). Mapping quantitative trait loci for yield,yield components and morphological traits in an advanced backcross population between Oryza rufipogon and the Oryza sativa cultivar jefferson. Theor. Appl. Genet. 107, 479–493. doi: 10.1007/s00122-003-1270-8
Tian, Y., Zhang, H., Xu, P., Chen, X., Liao, Y., Han, B., et al. (2015). Genetic mapping of a qtl controlling leaf width and grain number in rice. Euphytica. 202, 1–11. doi: 10.1007/s10681-014-1263-5
Venske, E., Dos Santos, R. S., Farias, D., Rother, V., da Maia, L. C., Pegoraro, C., et al. (2019). Meta-analysis of the qtlome of fusarium head blight resistance in bread wheat : refining the current puzzle. Front. Plant Sci. 10. doi: 10.3389/fpls.2019.00727
Venu, R. C., Ma, J., Jia, Y., Liu, G., Jia, M. H., Nobuta, K., et al. (2014). Identification of candidate genes associated with positive and negative heterosis in rice. PloS One 9, e95178. doi: 10.1371/journal.pone.0095178
Veyrieras, J. B., Goffinet, B., Charcosset, A. (2007). MetaQTL: A package of new computational methods for the meta-analysis of QTL mapping experiments. BMC Bioinf. 8, 49. doi: 10.1186/1471-2105-8-49
Vishnukiran, T., Neeraja, C. N., Jaldhani, V., Vijayalakshmi, P., Rao, P. R., Subrahmanyam, D., et al. (2020). A major pleiotropic QTL identified for yield components and nitrogen content in rice (Oryza sativa l). under differential nitrogen field conditions. PloS One 15, 1–22. doi: 10.1371/journal.pone.0240854
Wang, Y., Huang, Z., Deng, D., Ding, H., Zhang, R., Wang, S., et al. (2013). Meta-analysis combined with syntenic metaqtl mining dissects candidate loci for maize yield. Mol. Breed. 31, 601–614. doi: 10.1007/s11032-012-9818-4
Wang, Y., Wang, J., Zhai, L., Liang, C., Chen, K., Xu, J. (2020). Identify QTLs and candidate genes underlying source-, sink-, and grain yield-related traits in rice by integrated analysis of bi-parental and natural populations. PloS One 15, e0237774. doi: 10.1371/journal.pone.0237774
Wang, Y., Xu, J., Deng, D., Ding, H., Bian, Y. (2015). A comprehensive meta-analysis of plant morphology,yield, stay-green, and virus disease resistance QTL in maize (Zea mays l). Planta. 243, 459–471. doi: 10.1007/s00425-015-2419-9
Wei, Z., Teo, N., Zhou, W., Shen, L. (2019). Dissecting the function of MADS-box transcription factors in orchid reproductive development. Front. Plant Sci. 10. doi: 10.3389/fpls.2019.01474
Wei, Z., Zhongquan, C., Yongliang, W., Qigui, J., Jun, W., Shurong, L., et al. (2016). Quantitative trait locus analysis for grain size related traits of rice. Mol. Plant Breeding. 7, 1–19. doi: 10.5376/mpb.2016.07.0012
Wu, Y., Huang, M., Tao, X., Guo, T., Chen, Z., Xiao, W. (2016). Quantitative trait loci identification and meta-analysis for rice panicle-related traits. Mol. Genet. Genom. 291, 1927–1940. doi: 10.1007/s00438-016-1227-7
Xing, Y., Zhang, Q. (2010). Genetic and molecular bases of rice yield. Annu. Rev. Plant Biol. 61, 421–442. doi: 10.1146/annurev-arplant-042809-112209
Xu, F., Sun, X., Chen, Y., Huang, Y., Tong, C., Bao, J. (2015). Rapid identification of major QTLs associated with rice grain weight and their utilization. PloS One 10, e0122206. doi: 10.1371/journal.pone.0122206
Yaish, M. W., El-Kereamy, A., Zhu, T., Beatty, P. H., Good, A. G., Bi, Y. M., et al. (2010). The APETALA-2-like transcription factor OsAP2-39 controls key interactions between abscisic acid and gibberellin in rice. PloS Genet. 6, e1001098. doi: 10.1371/journal.pgen.1001098
Yamaguchi, T., Lee, D. Y., Miyao, A., Hirochika, H., An, G., Hirano, H. Y. (2006). Functional diversification of the two c-class MADS box genes OSMADS3 and OSMADS58 in Oryza sativa. Plant Cell. 18, 15–28. doi: 10.1105/tpc.105.037200.1
Yang, Y., Amo, A., Wei, D., Chai, Y., Zheng, J., Qiao, P., et al. (2021). Large-Scale integration of meta-qtl and genome-wide association study discovers the genomic regions and candidate genes for yield and yield-related traits in bread wheat. Theor. Appl. Genet. 134, 3083–3109. doi: 10.1007/s00122-021-03881-4
Yan, D., Zhang, X., Zhang, L., Ye, S., Zeng, L., Liu, J., et al. (2015). Curved chimeric palea1 encoding an EMF1-like protein maintains epigenetic repression of OsMADS58 in rice palea development. Plant J. 82, 12–24. doi: 10.1111/tpj.12784
Yao, S. G., Ohmori, S., Kimizu, M., Yoshida, H. (2008). Unequal genetic redundancy of rice PISTILLATA orthologs, OsMADS2 and OsMADS4, in lodicule and stamen development. Plant Cell Physiol. 49, 853–857. doi: 10.1093/pcp/pcn050
You, A., Lu, X., Jin, H., Ren, X., Liu, K., Yang, G., et al. (2006). Identification of quantitative trait loci across recombinant inbred lines and testcross populations for traits of agronomic importance in rice. Genetics. 172, 1287–1300. doi: 10.1534/genetics.105.047209
Yue, F., Zha, R. R., Ze-chuan, L., Li-yong, C., Xing-hua, W., Shi-hua, C. H. (2015). Quantitative trait locus analysis for rice yield traits under two nitrogen levels. Rice Sci. 22, 108–115. doi: 10.1016/j.rsci.2015.05.014
Zhang, C., Bai, M. Y., Chong, K. (2014). Brassinosteroid-mediated regulation of agronomic traits in rice. Plant Cell Rep. 33, 683–696. doi: 10.1007/s00299-014-1578-7
Zhang, S., Haider, I., Kohlen, W., Jiang, L., Bouwmeester, H., Meijer, A. H., et al. (2012). Function of the HD-zip I gene Oshox22 in ABA-mediated drought and salt tolerances in rice. Plant Mol. Biol. 80, 571–585. doi: 10.1007/s11103-012-9967-1
Zhang, L. Y., Liu, D. C., Guo, X. L., Yang, W. L., Sun, J. Z., Wang, D. W., et al. (2010). Genomic distribution of quantitative trait loci for yield and yield-related traits in common wheat. J. Integ. Plant Biol. 52, 996–1007. doi: 10.1111/j.1744-7909.2010.00967.x
Zhang, X., Shabala, S., Koutoulis, A., Shabala, L., Zhou, M. (2017). Meta-analysis of major QTL for abiotic stress tolerance in barley and implications for barley breeding. Planta. 245, 283–295. doi: 10.1007/s00425-016-2605-4
Zhang, H., Zhu, Y. J., Zhu, A. D., Fan, Y. Y., Huang, T. X. (2020). Fine-mapping of qTGW2, a quantitative trait locus for grain weight in rice (Oryza sativa l). PeerJ. 8, e8679. doi: 10.7717/peerj.8679
Zhao, M., Lin, Y., Chen, H. (2020). Improving nutritional quality of rice for human health. Theor. Appl. Genet. 133, 1397–1413. doi: 10.1007/s00122-019-03530-x
Zhao-Ming, Q., Ya-Nan, S., Qiong, W., Chun-Yan, L., Guo-Hua, H., Qing-Shan, C. (2011). A meta-analysis of seed protein concentration QTL in soybean. Can. J. Plant Sci. 91, 221–230. doi: 10.4141/CJPS09193
Zhao, X., Peng, Y., Zhang, J., Fang, P., Wu, B. (2018). Identification of QTLs and meta-QTLs for seven agronomic traits in multiple maize populations. Crop Sci. 520, 507–520. doi: 10.2135/cropsci2016.12.0991
Zhou, B., Lin, J. Z., Peng, D., Yang, Y. Z., Guo, M., Tang, D. Y., et al. (2017). Plant architecture and grain yield are regulated by the novel DHHC-type zinc finger protein genes in rice (Oryza sativa l). Plant Sci. 254, 12–21. doi: 10.1016/j.plantsci.2016.08.015
Zhou, S., Zhu, M., Wang, F., Huang, J., Wang, G. (2013). Mapping of QTLs for yield and its components in a rice recombinant inbred line population. Pak. J. Bot. 45, 183–189.
Zhuang, J. Y., Rao, Z. M., Wu, J. L., Xia, Y. W., Zheng, K. L. (2002). Analysis on additive effects and additive-by-additive epistatic effects of QTLs for yield traits in a recombinant inbred line population of rice. Theor. Appl. Genet. 105, 1137–1145. doi: 10.1007/s00122-002-0974-5
Keywords: Meta-QTL analysis, rice, grain yield, Genome-Wide Association Studies, candidate genes, marker-assisted selection
Citation: Aloryi KD, Okpala NE, Amo A, Bello SF, Akaba S and Tian X (2022) A meta-quantitative trait loci analysis identified consensus genomic regions and candidate genes associated with grain yield in rice. Front. Plant Sci. 13:1035851. doi: 10.3389/fpls.2022.1035851
Received: 03 September 2022; Accepted: 19 October 2022;
Published: 16 November 2022.
Edited by:
Takahiko Kubo, Kyushu University, JapanReviewed by:
Xiaoqian Wang, Shandong Agricultural University, ChinaRitu Batra, Chaudhary Charan Singh University, India
Irfat Jan, Chaudhary Charan Singh University, India, in collaboration with reviewer RB
Copyright © 2022 Aloryi, Okpala, Amo, Bello, Akaba and Tian. This is an open-access article distributed under the terms of the Creative Commons Attribution License (CC BY). The use, distribution or reproduction in other forums is permitted, provided the original author(s) and the copyright owner(s) are credited and that the original publication in this journal is cited, in accordance with accepted academic practice. No use, distribution or reproduction is permitted which does not comply with these terms.
*Correspondence: Xiaohai Tian, eGlhb2hhaXRAc2luYS5jb20=
†These authors have contributed equally to this work