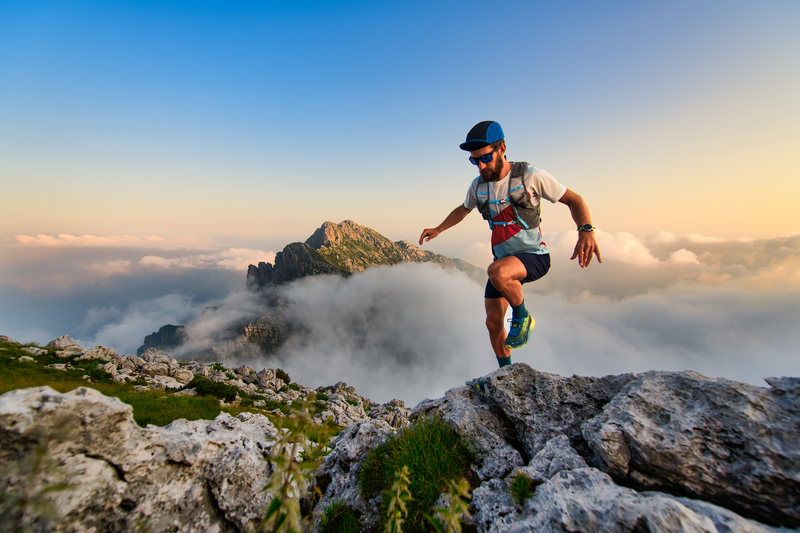
95% of researchers rate our articles as excellent or good
Learn more about the work of our research integrity team to safeguard the quality of each article we publish.
Find out more
ORIGINAL RESEARCH article
Front. Plant Sci. , 11 October 2022
Sec. Plant Abiotic Stress
Volume 13 - 2022 | https://doi.org/10.3389/fpls.2022.1011064
This article is part of the Research Topic Construction of Regulatory Networks in Plant Abiotic Stress Responses, Volume II View all 5 articles
Drought is one of the most severe abiotic stresses that influence wheat production across the globe. Understanding the molecular regulatory network of wheat in response to drought is of great importance in molecular breeding. Noncoding RNAs influence plant development and resistance to abiotic stresses by regulating gene expression. In this study, whole-transcriptome sequencing was performed on the seedlings of two wheat varieties with contrasting levels of drought tolerance under drought and control conditions to identify long noncoding RNAs (lncRNAs), micro RNAs (miRNAs), and mRNAs related to drought stress and explore the potential lncRNA-miRNA-mRNA regulatory modules in controlling wheat drought stress response. A total of 1515 differentially expressed lncRNAs (DELs), 209 differentially expressed miRNAs (DEMs), and 20462 differentially expressed genes (DEGs) were identified. Of the 20462 DEGs, 1025 were identified as potential wheat drought resistance-related DEGs. Based on the regulatory relationship and expression patterns of DELs, DEMs, and DEGs, 10 DEL-DEM-DEG regulatory modules related to wheat drought stress response were screened, and preliminary expression verification of two important candidate modules was performed. Our results revealed the possible roles of lncRNA-miRNA-mRNA modules in regulatory networks related to drought tolerance and provided useful information as valuable genomic resources in molecular breeding of wheat.
Drought stress is one of the major agricultural risks, and improving drought-tolerant crops is an urgent need in China and around the world (Araus et al., 2008). Wheat (Triticum aestivum L.) is one of the three major food crops in the world. Mining its candidate genes in response to drought stress and analyzing its genetic regulatory basis can provide new gene resources and a genetic basis for molecular design breeding of drought-tolerant wheat. Noncoding RNAs (ncRNAs) are a class of RNA molecules that do not encode proteins and have catalytic activity, and are widely present in various organisms (Mattick, 2009). Based on structure, ncRNAs can be divided into linear ncRNAs (linear-ncRNAs) and circular ncRNAs (circRNAs). Linear ncRNAs include microRNAs (miRNAs) and long noncoding RNAs (lncRNAs) (Mattick, 2005). In recent years, studies have found that ncRNAs, especially miRNAs and lncRNAs, can regulate gene expression at the gene transcriptional and protein translational levels and participate in almost all important life processes such as development, differentiation, and metabolism (Wang and Chang, 2011; Zou et al., 2020).
MiRNAs negatively regulate the expression of target genes at the post-transcriptional level, and their important roles in plant growth and development, stress response, and other physiological and biochemical reactions have been verified (Carrington and Ambros, 2003; Khraiwesh et al., 2012). In addition, lncRNAs can competitively bind with miRNAs to coordinately regulate the expression of target genes, and thereby life processes (Guttman and Rinn, 2012; Xu et al., 2020). Recent studies have identified a large number of lncRNAs and miRNAs related to growth and development and abiotic stress response in wheat. Lu et al. (2020b) found that 7435 lncRNAs were co-expressed with 7191 mRNAs, and 6 lncRNA-mRNA modules associated with cold stress response in wheat were obtained. Using two pairs of near-isogenic lines, Zhou et al. (2020) identified 5399 tillering-associated lncRNAs in wheat. Cao et al. (2021) performed genome-wide profiling of lncRNAs during wheat spike development in 6 different stages and identified a total of 8889 expressed lncRNAs, among which 2753 were differentially expressed in various developmental stages. Akdogan et al. (2016) investigated drought stress-responsive miRNAs in the root and leaf tissues of bread wheat. Their results showed that 285 and 244 miRNAs were differentially expressed in leaf and root tissues, respectively. A total of 79 miRNAs (46 known and 33 novel miRNAs) that showed significant differential expression during wheat grain development under both high- and low-nitrogen treatments were identified (Hou et al., 2020). Under cold stress, a total of 192 miRNAs from 105 families and nine novel miRNAs were identified. Among them, 34 conserved and five novel miRNAs were differentially expressed between cold-stressed samples and controls (Song et al., 2017).
At present, research on ncRNAs related to drought stress tolerance in wheat mostly focuses on the combined analyses of single ncRNAs and mRNAs (Lu et al., 2020a; Madhawan et al., 2020; Wu et al., 2021), with no reports available on the combined analyses of lncRNAs, miRNAs, and mRNAs. Therefore, this study aims to explore the role of lncRNAs, miRNAs, and mRNAs in wheat drought resistance, and identify differentially expressed lncRNAs, miRNAs, and genes for the same. Based on the expression patterns of ncRNAs and mRNAs, lncRNA-miRNA-mRNA regulatory modules related to wheat drought stress response were screened, and preliminary expression verification of important candidate modules was performed. The results of this study will provide a basis for the next step analysis of the regulatory functions among lncRNAs, miRNAs, and genes related to drought resistance in wheat.
Two wheat (Triticum aestivum L.) varieties, ZM13 and JM38, were used in this study. ZM13 is a drought-tolerant variety (marked as DT), and JM38 is a drought-sensitive variety (marked as DS). Wheat seeds were sterilized with 75% (v/v) ethanol for 2 min and 2% (v/v) H2O2 for 30 min, followed by washing thrice with sterile water (Quan et al., 2016). The seeds were then germinated in distilled water at room temperature. After 3 days, the germinated seeds were transplanted into 96-well plastic boxes (13cm×8.5cm×11cm) and cultivated in a light incubator at 22/20 ˚C with a 14h light/10h dark photoperiod (Sun et al., 2020). For the drought treatment of wheat seedlings, 7-day-old (one-leaf-one-heart period) wheat seedlings were cultured in Hoagland nutrient solution with or without 20% PEG 6000.
Relative water content (RWC) of leaf and physiological traits were measured after drought stress for four days. The last fully expanded leaves were sampled to measure RWC according to the Barrs and Weatherley method (Barrs and Weatherley, 1962). Malondialdehyde (MDA) content was measured as previously described (Cao et al., 2009). Peroxidase (POD) activity was determined as described previously (Chen et al., 2013). Proline content was measured according to the Qin et al. (2014) method. Plant height and root length were measured after drought stress for 7 days.
Total RNA was extracted from wheat root samples after four days of drought treatment using a TruSeq Stranded Total RNA Sample Preparation kit (Illumina, San Diego, USA). The RNA concentration and purity were assessed by a NanoDrop2000 (NanoDrop, Wilmington, DE, USA). The RNA integrity was assessed using both gel electrophoresis and the Agilent Bioanalyzer 2100 System (Agilent, Palo Alto, CA, USA). The ribosomal RNA (rRNA) was removed from total RNA with a Ribo-Zero™ rRNA Removal Kit (Illumina, San Diego, USA).
For lncRNA-seq, RNA was fragmented into small pieces and the first-strand cDNA synthesized with SuperScript II Reverse Transcription (Invitrogen, CA, USA). After purification, the second-strand cDNA library was synthesized following several rounds of PCR amplification. RNA library was sequenced on an Illumina NovaSeq 6000 platform at Biomarker Technologies Co., Ltd. (Beijing, China) with paired-end reads being generated.
For small RNA sequencing, total RNA was ligated to 5’ and 3’ RNA adaptors according to the manual of NEBNext Multiplex Small RNA Library Prep Set for Illumina (NEB, MA, USA). RNAs were reverse transcribed to cDNAs, following PCR amplification. PAGE gel was used to electrophoresis fragment screening purposes and rubber cutting recycling as the pieces get small RNA libraries. PCR products were then purified (AMPure XP system) and library quality assessed. The clustering of the index-coded samples was performed on a cBot Cluster Generation System using TruSeq PE Cluster Kit v4-cBot-HS (Illumia) according to the manufacturer’s instructions. After cluster generation, the libraries were sequenced by Biomarker Technologies Co., Ltd. (Beijing, China) on an Illumina NovaSeq 6000 platform with single-end reads being generated.
All raw read sequences were uploaded to the Sequence Read Archive (SRA) of NCBI under the accession numbers PRJNA838787 (lncRNA and mRNA) and PRJNA837867 (miRNA), respectively.
Clean data (clean reads) were obtained by first trimming adapters, and then removing reads containing ploy-N and low-quality reads from raw data with Cutadapt. Simultaneously, Q20, Q30, GC-content, and sequence duplication levels of the clean data were calculated (Ewing et al., 1998). The transcriptome was then assembled using the StringTie (1.3.1) based on the reads mapped to the reference genome using HISAT (2.0.4) (Pertea et al., 2016). The assembled transcripts were annotated using the GffCompare program. Unknown transcripts were used to screen for putative lncRNAs. Four computational approaches, including CPC (Coding Potential Calculator) (Kong et al., 2007), CNCI (Coding-Non-Coding Index) (Sun et al, 2013), Pfam (Protein family) (Finn et al., 2013), and CPAT (Coding Potential Assessment Tool) (Wang et al., 2013) were combined to screen RNA with no coding function from transcripts longer 200 nucleotides (nt) and with exon number greater than two (Kelley and Rinn, 2012). Different types of lncRNAs, including long intergenic non-coding RNA (lincRNA), intronic lncRNA, anti-sense lncRNA, and sense lncRNA were selected using Cuffcompare.
Based on the mode of action of lncRNAs and their target genes, two prediction methods were adopted. First, a Perl script was used to set the adjacent genes within 100 kb upstream and downstream of lncRNA as its cis-target genes. In addition, the trans-target genes of lncRNAs were predicted by the correlation analysis method between the expression levels of lncRNAs and mRNAs in the samples. Pearson correlation coefficient method was used to analyze the correlation between lncRNAs and mRNAs in the samples, and the gene with an absolute correlation value greater than 0.9 and p-value less than 0.01 was taken as the trans-target gene of lncRNA.
First, clean data (clean reads) were obtained by first trimming adapters, and then removing reads containing ploy-N and low-quality reads from raw data. Reads were trimmed and cleaned by removing sequences smaller than 18 nt or longer than 30 nt. Simultaneously, Q20, Q30, GC-content, and sequence duplication levels of the clean data were calculated. All downstream analyses were based on clean data with high quality. Use Bowtie tools soft, the clean reads respectively with Silva database, GtRNAdb database, Rfam database, and Repbase database sequence alignment, filter ribosomal RNA (rRNA), transfer RNA (tRNA), small nuclear RNA (snRNA), small nucleolar RNA (snoRNA), and other ncRNA and repeats, respectively (Langmead et al., 2009). The remaining reads were used to detect known miRNAs by comparing with them genome and known miRNAs from miRBase release 22 (http://www.mirbase.org). MiRDeep2 software was used to predict novel miRNAs (Friedlander et al., 2012). TargetFinder software was used based on sequence information to predict miRNA target genes (Allen et al., 2005).
StringTie (1.3.1) (Trapnell et al., 2010) was used to calculate FPKMs (Fragments Per Kilobase of transcript per Million fragments mapped) of lncRNAs and mRNAs in each sample. TPM algorithm was used to normalize the expression levels of miRNAs in each sample (Li et al., 2009). DESeq R package (1.10.1) was used to analyze the differential expression levels of two groups (Love et al., 2014). The criteria to identify differentially expressed genes (DEGs), differentially expressed lncRNAs (DELs) and differentially expressed miRNAs (DEMs) were adjusted to p-value < 0.05 and absolute value log2 (fold change) > 1.
Gene Ontology (GO) enrichment analysis of the DEGs was implemented by the topGO R package (Ashburner et al., 2000). KOBAS software was used to test the statistical enrichment of DEGs in KEGG (Kyoto Encyclopedia of Genes and Genomes) pathways (Kanehisa et al., 2004).
Quick RNA isolation Kit (Tiangen Biochemical Technology Co., Ltd., Beijing, China) was used to extract RNA according to the manufacturer’s instructions and DNase I treatment was used to remove DNA contamination. Synthesis of the first strand of cDNA was carried out according to the instructions of the kit (TaKaRa, Beijing, China). mRNA and lncRNA were detected by TB Green Premix Ex Taq II (TliRNaseH Plus) and ROX plus (TaKaRa, Beijing, China). The first-strand cDNA synthesis reagent of miRNA was performed simultaneously by A addition method and reverse transcription reaction, and NovoScript® miRNA First-Strand cDNA Synthesis and SYBR qPCR Kit (Suzhou Nearshore Protein Technology Co., Ltd., Suzhou, China) was used. qRT-PCR reactions were conducted using the following protocol: 95 ˚C for 2 min, followed by 40 cycles of 95 ˚C for 20 s and 60 ˚C for 20 s and 72 ˚C for 20 s. The wheat elongation factor (TaEF-1a) gene was used as an internal reference for mRNAs and lncRNAs expression analyses. miRNAs expression analyses using TaU6 gene as an internal reference gene. Threshold values (CT) were generated using the ABI PRISM 7500 system (Applied Biosystems, Foster City, CA, USA), and the transcript levels assessed using the comparative 2-ΔΔCT method. All primers used in this study are listed in supplementary Table S1.
As observed in Figure 1, drought stress inhibited plant height and root length after 7 days of treatment. However, the plant height and root length of DT were significantly higher compared to those of DS after 7 days of drought stress (Figures 1A–C). Physiological traits of the two wheat varieties were measured after four days of drought stress. The results showed that DT had higher leaf RWC, proline content, and POD activity but lower MDA content compared to DS under drought stress conditions (Figures 1D–G). The above results proved that DT had a stronger ability to resist drought stress than DS.
Figure 1 Phenotypes of the two wheat varieties under drought stress and control conditions. (A) Phenotypes of the two wheat varieties at the seedling stage under drought stress and control conditions for seven days. DS, drought-sensitive variety JM38, DT, drought-tolerant variety ZM13; Scale bars, 5 cm. (B) Height of plants subjected to drought stress for seven days. Values are means ± SD. **P < 0.01. (C) Root length in plants subjected to drought stress for seven days. (D) Relative water content of plant leaves subjected to drought stress for four days. (E) Peroxidase activity of plant leaves subjected to drought stress for four days. (F) Malindialdehyde content of plant leaves subjected to drought stress for four days. (G) Proline content of plant leaves subjected to drought stress for four days.
Roots of the two wheat varieties were collected as three biological replicates at the seedling stage under drought and control conditions. A total of 210.36 Gb clean data were obtained after filtering raw data obtained by the sequencing platform. For each sample, the clean data were higher than 14.50 Gb with Q30 ≥ 93.01% (Table 1). Clean reads of each sample were aligned with the wheat reference genome, and alignment efficiency ranged from 71.35 to 82.74% (Table 1). The coding potential of the transcript was screened by the most widely used coding potential analysis methods, including CPC analysis, CNCI analysis, CPAT analysis, and Pfam protein domain analysis, to determine whether the transcript was lncRNA or not. The noncoding transcripts identified by the four analysis methods were intersected, and 15307 lncRNAs were identified (Supplementary Figure S1), including 13360 (87.3%) lincRNAs, 935 (6.1%) intronic lncRNAs, 680 (4.4%) antisense lncRNAs, and 332 (2.2%) sense lncRNAs (Supplementary Figure S2). The identified lncRNAs were subjected to subsequent analyses.
To understand drought-responsive and tolerance-related miRNAs in wheat, small RNA libraries of 12 samples were sequenced. In total, 375.98 million raw short reads were obtained. A total of 261.42 million clean reads were acquired after filtering out reads with low-quality and substandard sequence lengths (fewer than 18 nt or greater than 30 nt). For each sample, the clean reads were higher than 20.20 million. Q30 scores of all samples ranged from 95.06 to 96.93%, indicating that the sequence data were of reliable quality (Table 1). rRNA, tRNA, snRNA, snoRNA, and repetitive sequences were filtered out from the clean reads to obtain unannotated reads. The unannotated reads were aligned with the wheat reference genome, and the percentage of mapped reads in each sample’s unannotated reads ranged from 18.02 to 34.34% (Table 1). It was found that 93 known miRNAs and 779 novel miRNAs were expressed (Supplementary Table S2). In addition, the length distribution of the expressed known miRNAs was highly enriched in 21 and 22 nt and for novel miRNAs in 24 and 21 nt. (Supplementary Figure S3).
To investigate the expression profiles of genes present in wheat roots in response to drought stress, expression levels were compared based on the FPKM values. In total, 15909 differentially expressed genes (DEGs) were identified in DS under drought conditions compared to control conditions (CK), including 6733 up-regulated and 9176 down-regulated genes, respectively. A total of 11233 DEGs were identified in DT following drought treatment compared to CK, including 5488 up-regulated and 5745 down-regulated genes, respectively. Venn diagram analysis showed that 6680 DEGs were commonly identified between DS and DT. In addition, 9229 and 4553 DEGs were specific to DS and DT, respectively (Figures 2A, B), and a total of 20462 DEGs were identified in both varieties.
Figure 2 Differentially expressed genes (DEGs), differentially expressed lncRNAs (DELs), and differentially expressed miRNAs (DEMs) at the seedling stage of the two wheat varieties under drought stress relative to control conditions. DS, drought-sensitive variety JM38, DT, drought-tolerant variety ZM13. (A, B) Venn diagram and volcano plots of DEGs of JM38 (DS) and ZM13 (DT). (C, D) Venn diagram and volcano plots of DELs of JM38 (DS) and ZM13 (DT). (E, F) Venn diagram and volcano plots of DEMs of JM38 (DS) and ZM13 (DT).
Of all DEGs, the following three types were screened as drought resistance-related DEGs for further analyses. The first type of DEGs were specifically up- or down-regulated in DT with an absolute value of log2(fold change in DT) > 2. The second type of DEGs were up- or down-regulated in both DT and DS with log2(fold change in DT)/log2(fold change in DS) > 3. The third type of DEGs showed opposite expression patterns in DT and DS. Finally, a total of 1025 drought resistance-related DEGs were screened from 20462 DEGs (Figure 3; Supplementary Table S3). To verify the accuracy of the RNA-seq data, 12 drought resistance-related DEGs were randomly selected for further qRT-PCR. The qRT-PCR and RNA-seq findings were highly consistent, indicating the reliability of the mRNA data (Supplementary Figure S4).
Figure 3 Heatmap of the expression of 1025 drought resistance-related DEGs. DS, drought-sensitive variety JM38, DT, drought-tolerant variety ZM13. (A) DEGs were specifically up-regulated in DT with log2 (fold change in DT) > 2. (B) DEGs were specifically down-regulated in DT with log2 (fold change in DT) < -2. (C) DEGs were up-regulated in both DT and DS with log2(fold change in DT)/log2(fold change in DS) > 3. (D) DEGs were down-regulated in both DT and DS with log2(fold change in DT)/log2(fold change in DS) > 3. (E) DEGs were down-regulated in DS and up-regulated in DT. (F) DEGs were up-regulated in DS and down-regulated in DT.
GO enrichment analysis was performed on the 1025 drought resistance-related DEGs. A total of 751 DEGs were assigned into three categories, namely biological processes (BP), cellular components (CC), and molecular functions (MF). GO terms metallic, cellular, and single organic processes were significantly enriched in BP. The significantly enriched GO terms in MF included catalytic activity, binding, and transcription factor activity, and those in CC included membrane, membrane part, and cell (Supplementary Figure S5). For all drought resistance-related DEGs, a total of 394 DEGs were mapped to different KEGG pathways. The results showed that pathways, such as nitrogen metabolism, alanine, aspartate, and glutamate metabolism, diterpenoid biosynthesis, phenylpropanoid biosynthesis, betalain biosynthesis, and glycerophospholipid metabolism, were significantly enriched (Supplementary Figure S6). In addition, pathways, including plant-pathogen interaction, phenylpropanoid biosynthesis, plant hormone signal transduction, starch and sucrose metabolism, and flavonoid biosynthesis, were enriched with more DEGs. (Supplementary Figure S6).
When plant cells are stimulated by the outside environments, they can transmit signals into the cell by activating the plant-pathogen interaction pathway, and then activate a series of other proteins (such as protein kinases) to transmit these signals (Li et al., 2020). In the present study, 48 drought resistance-related DEGs were enriched in the plant-pathogen interaction pathway, including ten disease resistance proteins RPM1, six serine/threonine-protein kinases FRK1, five EIX (ethylene-inducing xylanase) receptors, three brassinosteroid insensitive 1-associated receptor kinase 1 (BAK1), three calmodulins (CALM), three serine/threonine-protein kinases PBS1, and three pathogenesis-related protein 1 (PR1), etc. (Figure 4). The mitogen-activated protein kinases (MAPK) signaling pathway is one of the important pathways in the eukaryotic signaling network, which can coordinate cellular responses to achieve normal plant growth and development, immune responses, and responses to abiotic stress (Danquah et al., 2014). A total of 24 drought resistance-related DEGs, including two serine/threonine-protein kinases FLS2, four WRKY transcription factors, two transcription factor MYC2, and one abscisic acid receptor PYL were enriched in the MAPK signaling pathway (Figure 4). External environmental stress will stimulate the production of phytohormones and promote the plant adaptation to stressful environment (Yu et al., 2020). Here, 32 drought resistance-related DEGs were found to be enriched in the plant hormone signal transduction pathway, including four jasmonate ZIM domain-containing proteins (JAZ), two ABA-responsive element binding factors (ABF), one SAUR family protein, one ethylene-insensitive protein 2 (EIN2) and one transcription factor TGA (Figure 4). In addition, we found that many drought resistance-related DEGs were involved in multiple pathways, such as BAK1 and PR1 were simultaneously involved in the plant-pathogen interaction pathway, MAPK signaling pathway, and plant hormone signal transduction pathway. FLS2, WRKY transcription factor 33 (WRKY33), and FRK1 were also involved in the plant-pathogen interaction pathway and MAPK signaling pathway. PYL, EIN2, and MYC2 were involved in both MAPK signaling pathway and plant hormone signal transduction pathway (Figure 4). These genes might be promoted the production of phytohormones by participating in the transduction of stimulatory signals to improve the drought resistance of wheat.
Figure 4 Heatmap of the expression of drought-resistant DEGs involved in important pathways. DS, drought-sensitive variety JM38, DT, drought-tolerant variety ZM13, RPM1, disease resistance protein RPM1, FRK1, serine/threonine-protein kinase FRK1, EIX 1-2, ethylene-inducing xylanase receptor, BAK1, brassinosteroid insensitive 1-associated receptor kinase 1, CALM, calmodulins, PR1, pathogenesis-related protein 1, PBS1, serine/threonine-protein kinase PBS1, KCS, 3-ketoacyl-CoA synthase, FLS2, serine/threonine-protein kinase FLS2, EFR, serine/threonine-protein kinase EFR, PTI1, pto-interacting protein 1, WRKY52, WRKY transcription factor 52, WRKY33, WRKY transcription factor 33, WRKY2, WRKY transcription factor 2, CERK1, chitin elicitor receptor kinase 1, RIN4, RPM1-interacting protein 4, BRI1, brassinosteroid insensitive 1, JAZ, jasmonate ZIM domain-containing protein, ABF, ABA-responsive element binding factor, MYC2, transcription factor MYC2, SAUR, SAUR family protein, GID1, gibberellin receptor GID1, gibberellin receptor GID1, PIF3, phytochrome-interacting factor 3, PYL, abscisic acid receptor PYL, EIN2, ethylene-insensitive protein 2, TGA, transcription factor TGA, CHiB endochitinase B, ER, serine/threonine-protein kinase ERECTA, NR, nitrate reductase, NRT, nitrate transporter, CAH, carbonic anhydrase, GDH, glutamate dehydrogenase, GS, glutamine synthetase, GOGAT, glutamate synthase, AS, Asparagine synthase, AGXT2, alanine-glyoxylate transaminase.
In addition, we also identified 5 drought resistance-related DEGs that were enriched in the glutamate metabolism pathway and nitrogen metabolism pathway, including three glutamate dehydrogenases (GDH), one glutamine synthetase (GS), and one glutamate synthase (GOGAT) (Figure 4). In addition to these DEGs, 11 drought resistance-related DEGs including five nitrate reductases (NR), three nitrate transporters (NRT), and three carbonic anhydrases (CAH) were enriched in the nitrogen metabolism pathway, of which two NRT (TraesCS6B02G044400 and TraesCS6D02G035900) showed a down-regulated expression in DS, but an up-regulated expression in DT, and two NR (TraesCS6B02G024900 and TraesCS7D02G073700) specifically showed up-regulated expression in DT only (Figure 4).
A total of 831 differentially expressed lncRNAs (DELs) were identified in DS under drought conditions compared to CK, including 446 up-regulated and 385 down-regulated DELs, respectively (Supplementary Table S4). A total of 788 DELs were identified in DT following drought treatment compared to CK, including 523 up-regulated and 265 down-regulated DELs, respectively (Figures 2C, D; Supplementary Table S4). The results showed that 104 DELs were commonly identified between DS and DT. In addition, 727 and 684 DELs were specific to DS and DT, respectively (Figures 2C, D). A total of 1515 DELs were identified in both varieties. Eight DELs were randomly selected for further qRT-PCR, including 5 up-regulated and 3 down-regulated DELs. The qRT-PCR and RNA-seq findings were highly consistent, indicating the reliability of the lncRNA data (Supplementary Figure S7).
A total of 155 differentially expressed miRNAs (DEMs) were identified in DS under drought conditions compared to CK, including 121 up-regulated and 34 down-regulated DEMs, respectively (Supplementary Table S5). A total of 123 DEMs were identified in DT following drought treatment compared to CK, including 81 up-regulated and 42 down-regulated DEMs, respectively (Supplementary Table S5). Venn diagram analysis showed that 69 DEMs were commonly identified between DS and DT. In addition, 86 and 54 DEMs were specific to DS and DT, respectively. A total of 209 DEMs were identified in both varieties (Figures 2E, F). To verify the accuracy of small RNA-Seq data, 6 up-regulated and 2 down-regulated DEMs were randomly selected for further qRT-PCR. The qRT-PCR and small RNA-seq findings were highly consistent, indicating the reliability of the miRNA data (Supplementary Figure S7).
Recent studies have shown are interactions among RNA molecules, such as lncRNA and miRNA, miRNA and mRNA, lncRNA and mRNA, and that these interactions form a regulatory network of lncRNA-miRNA-mRNA (Guttman and Rinn, 2012; Xu et al., 2020). By combining with miRNAs, lncRNAs reduce the ‘silencing effect’ of miRNAs on target genes, thereby regulating the target genes of miRNAs (Guttman and Rinn, 2012; Xu et al., 2020). To explore the lncRNA-miRNA-mRNA regulatory modules related to drought stress in wheat, DEMs were studied, and DEGs and DELs that had a targeting relationship with the DEMs but with the opposite expression pattern were searched. On this basis, DELs and DEGs having a targeting relationship with each other were retained. For example, by centering on up-regulated DEMs, we looked for DEGs and DELs that had a targeting relationship with these DEMs and with down-regulated expression. Or by centering on down-regulated DEMs, we looked for DEGs and DELs that had a targeting relationship with these DEMs and with up-regulated expression. Then, these DELs and DEGs having a targeting relationship with each other were then retained. Finally, the following two types of regulatory modules were obtained, namely DEL (down-regulated)-DEM (up-regulated)-DEG (down-regulated) and DEL (up-regulated)-DEM (down-regulated)-DEG (up-regulated).
In the present study, 10 regulatory modules were identified in both varieties. All identified modules belonged to the first type, lncRNA (down-regulated)-miRNA(up-regulated)-mRNA (down-regulated) (Figure 5). A total of 8 DEL-DEM-DEG regulatory modules were identified in DS, including 9 up-regulated DEMs, 13 down-regulated DELs, and 17 down-regulated DEGs (Figure 5). Two DEL-DEM-DEG regulatory modules were identified in DT, including 2 up-regulated DEMs, 2 down-regulated DELs, and 4 down-regulated DEGs (Figure 5). DEMs novel-miR-417 and novel-miRNA-340 were commonly identified between DS and DT that targeted DELs and DEGs differently in the modules of the two wheat varieties. In DS, the targeted DEL of novel-miRNA-340 was MSTRG.56120.4 and the targeted DEGs were TraesCS5D02G032189, TraesCS1B02G061200, TraesCS1A02G003100, and TraesCS2B02G026700, respectively. But in DT, the targeted DEL and DEG of novel-miRNA-340 were MSTRG.188250.2 and TraesCS7B02G476700, respectively. Moreover, in DS, the targeted DEL and DEGs of novel-miRNA-417 were MSTRG.106880.1 and TraesCS3B02G606700, TraesCS2D02G260300, TraesCS1B02G009400, and Triticum-aestivum-newGene-103110, respectively. But in DT, the targeted DEL and DEGs of novel-miRNA-417 were MSTRG.148484.1 and TraesCS2B02G034900, TraesCS7B02G442300, and Triticum-aestivum-newGene3-31222, respectively (Figure 5). These results suggested that two wheat cultivars have different response mechanisms under drought stress.
Figure 5 LncRNA-miRNA-mRNA regulatory modules associated with drought stress response were screened in DS (A) and DT (B). Lines with arrows at both ends represent a targeting relationship between the two RNAs.
Gene annotation and KEGG pathways of 21 DEGs were analyzed in 10 modules. Among these genes, 16 were annotated to different functions, including six disease resistance proteins, one transcription factor, one potassium ion transporter, one peroxidase, one glycosyltransferase, one aquaporin, and one anthranilate O-methyltransferase. KEGG pathways involving these genes included plant-pathogen interaction pathway, plant hormone signal transduction pathway, phenylpropanoid biosynthesis pathway, starch and sucrose metabolism pathway, and aminoacyl-tRNA biosynthesis pathway (Figure 6).
Figure 6 Heatmap of the expression of DEGs in lncRNA-miRNA-mRNA regulatory modules and their functional annotations. DS, drought-sensitive variety JM38, DT, drought-tolerant variety ZM13.
Expression patterns of regulatory modules containing novel-miR-340 and novel-miR-417 were verified by qRT-PCR using the root cDNA of DS and DT as templates after four days of drought treatment. The results showed that the expression levels of MSTRG.148484.1 and MSTRG.188250.2 in DT were significantly decreased while the expression levels of MSTRG.106880.1 and MSTRG.56120.4 in DS were significantly decreased. Novel-miR-417 and novel-miR-340 were significantly upregulated in both cultivars after drought stress. TraesCS2B02G034900 and TraesCS7B02G442300 were significantly decreased only in DT after drought stress, while the expression levels of TraesCS3B02G606700, TraesCS1B02G009400, TraesCS5D02G032189, TraesCS1B02G061200, TraesCS1A02G003100 and TraesCS2B02G026700 in DS were significantly decreased. In addition, TraesCS2D02G260300 and TraesCS7B02G476700 were significantly downregulated after drought stress in both cultivars (Figure 7). These results indicated a competing expression pattern between the members of two candidate modules under drought stress conditions.
Figure 7 Validation of the expression patterns of lncRNA-miRNA-mRNA regulatory modules by qRT-PCR. The column represents the relative expression levels of qRT-PCR, and the broken line represents the log2(fold change) of RNA-seq or small RNA-seq. Values are means ± SD. *P < 0.05. **P < 0.01. ns, no significance.
A large number of drought resistance-related DEGs were found enriched in three interconnected pathways, namely the plant-pathogen interaction pathway, plant hormone signaling transduction pathway, and MAPK signaling pathway (Figure 8). Some drought resistance-related DEGs were enriched in plant-pathogen interaction pathways, including threonine-protein kinase FLS2, brassinosteroid insensitive 1-associated receptor kinase 1 (BAK1), WRKY33, serine/threonine-protein kinase (FRK1), and pathogenesis-related protein PR1 which were also involved in the MAPK signal pathway (Figure 8). In addition, the ABA receptor protein PYL, transcription factor MYC2, and EIN2 in the MAPK signaling pathway were also involved in the plant hormone signal transduction pathway (Figure 8). Drought resistance-related DEGs which were enriched in phytohormone signal transduction pathways also included the ABF, transcription factor TGA, and JAZ. The above-mentioned drought resistance-related DEGs were also found to regulate stomatal opening and root growth through their interaction with each other, thereby enabling plants to adapt to stressful environments. For example, FLS2 and BAK1 can inhibit the expression of FRK1 and PR1 by indirectly affecting WRKY33, thus initiating the stress response mechanism in plants (Figure 8). PYL can not only improve plant adaptation to stress by activating mitogen-activated protein kinase (MPK1) but also indirectly affect plant stomatal closure by activating ABF (Figure 8). In addition, the interaction between JAZ and MYC2 can indirectly affect the growth of plant roots and improve their adaptability to stress. EIN2 can generate a defense response to the external environment by activating the expression of basic endochitinase B (CHiB) (Figure 8).
Figure 8 Expression patterns of DEGs in important pathways. Different colors represent different pathways. Genes containing more than two colors indicate their involvement in multiple pathways simultaneously. DS, drought-sensitive variety JM38, DT, drought-tolerant variety ZM13.
In addition to the above important pathways, a few drought resistance-related DEGs were significantly enriched in nitrogen metabolism and amino acid metabolism pathways with a close relationship observed between the two. In addition to being one of the nutrient elements necessary for plant growth and development, nitrogen also participates in and regulates physiological and biochemical processes, such as adaptation, repair, and compensation of plants under drought stress (Masclaux-Daubresse et al., 2010; Xu et al., 2012). Nitrate in the soil is transported to plant root cells by specific NRT, where it is reduced to nitrite by NR in the cytoplasm (Forde, 2002; Parker and Newstead, 2014). Nitrite is transferred to the chloroplast or plastid and reduced to ammonium nitrogen by nitrite reductase (NiR) (Stif et al., 2009). Ammonium nitrogen enters the GS/GOGAT cycle and is assimilated into organic nitrogen, such as glutamate, which is involved in the biosynthesis of other amino acids and proteins (Redinbaugh and Campbell, 1998). Studies have confirmed that external stress can induce the accumulation of nitrate in the roots of plants to improve plant resistance to external stress. Chen et al. (2012) showed that PEG-simulated drought promotes the accumulation of NO3- in roots by altering the expression levels of NRT1.5 to improve drought tolerance in plants. NR is known to mediate nitric oxide (NO) in plants (Pan et al., 2019). NR-induced NO improves tolerance to various stresses in plants (Kaya et al., 2020b). Kaya (2020a) showed the requirement of NR for salicylic acid-induced water stress tolerance in pepper plants. In this study, nitrogen uptake-related enzymes such as NRT and NR were significantly up-regulated in DT but significantly down-regulated in DS (Figure 8), suggesting that the nitrogen uptake was enhanced in drought-resistant wheat varieties. In addition, a few studies demonstrated that plants mainly accumulate proline by enhancing the proline biosynthesis pathway with glutamate as a prerequisite to maintain normal growth under osmotic stress conditions (Rhodes et al., 1987). In plants, glutamate mainly assimilates inorganic nitrogen through the GS/GOGAT cycle (Guan et al., 2014). Asparagine synthase (AS) can enhance plant disease and stress resistance by regulating nitrogen metabolism (Olea et al., 2004; Horst et al., 2010). In the present study, GDH, GS, and GOGAT were significantly down-regulated in DT and significantly up-regulated in DS (Figure 8). Further, five AS were significantly down-regulated in DT but not observed differently in DS (Figure 8). The above-mentioned drought-resistance-related DEGs may regulate the resistance of wheat to drought stress by participating in nitrogen metabolism and amino acid metabolic pathways.
To explore the lncRNA-miRNA-mRNA regulatory modules related to drought stress response in wheat, DEMs were studied, and DEGs and DELs that had a targeting relationship with DEMs and had the opposite expression pattern were searched. Finally, a total of 10 DEL-DEM-DEG regulatory modules were identified in both varieties (Figure 5). DELs, DEMs, and DEGs in each module were targeted at each other. Further, we used the gene expression profiling data published on the WheatOmics (Ma et al., 2021) data website (http://wheatomics.sdau.edu.cn/) to determine whether the regulatory modules screened in this study are tissue-specific. The expression analysis of DEGs from 10 modules in wheat roots, stems, leaves, spikes, and grains suggested that these genes were mainly expressed in wheat roots (Supplementary Figure S8). Therefore, we speculated that the regulatory modules screened in this study were tissue-specific and mainly regulated the expression of genes in wheat roots under drought stress. Using the published miRNA data (Akdogan et al., 2016) of wheat after drought stress, we verified the expression of 4 known miRNAs in the regulatory modules screened in this study from which 2 of the miRNAs (tae-miR156 and tae-miR1120c-5p) has been reported an up-regulated expression pattern previously. In addition, we also used the degradome data on the miRNA target gene prediction platform WPMIAS (Whole-degradome-based Plant MicroRNA-Target Interaction Analysis Server) (Fei et al., 2019) to verify the prediction results of tae-miR156 target genes. The results showed that most of the predicted tae-miR156 target genes (85.71%) based on the degradome were also predicted in this study.
Among these modules, DEMs novel-miR-340 and novel-miR-417 were commonly identified between DS and DT which targeted DELs and DEGs differently in the modules of the two varieties (Figure 5). Combined with qRT-PCR results, it was speculated that in DS, MSTRG.56120.4 can inhibit TraesCS5D02G032189, TraesCS1B02G061200, TraesCS1A02G003100, and TraesCS2B02G026700 expression by activating novel-miRNA-340 (Figure 9). In DT, novel-miRNA-340 was activated by MSTRG.188250.2, resulting in the down regulation of TraesCS7B02G476700 (Figure 9). The annotation function of TraesCS7B02G476700 is aquaporin (AQP). Plant AQP can not only promote long-distance water transport from plant roots to leaves but also intracellular and extracellular transmembrane transport of water as a stress response mechanism (Maurel et al., 2009). By analyzing the published gene expression profiling data (http://wheatomics.sdau.edu.cn/), we found that the expression level of TraesCS7B02G476700 was also decreased when wheat subjected to low phosphorus (Oono et al., 2013) and salt stress (Zhang et al., 2016) (Supplementary Figure S8). In addition, the ortholog of TraesCS7B02G476700 gene in rice, rMip1, was also induced by water and salt stress (Liu et al., 1994). The qRT-PCR results also confirmed that novel-miR-417 and its target-related DELs and DEGs showed an expression trend consistent with the sequencing results of both varieties. Among them, DEG TraesCS2D02G260300 targeted by novel-miR-417 in DS is a peroxidase (POD) gene. POD can regulate the balance of reactive oxygen species in organisms by catalyzing the redox of H2O2, and play an important role in plant growth and development and abiotic stress response (Meloni et al., 2003). By analyzing the expression profile data of TraesCS2D02G260300 under different abiotic stresses, we found that the transcription of TraesCS2D02G260300 can be induced by a variety of abiotic stresses. For example, the expression level of TraesCS2D02G260300 was decreased under low phosphorus (Oono et al., 2013), heat (Kumar et al., 2015), and salt stress (Zhang et al., 2016), whereas increased under cold stress (Li et al., 2015) (Supplementary Figure S8). In summary, these two regulatory modules centered on novel-miR-340 and novel-miR-417 may play a role in wheat drought stress response, and regulate different genes in the two drought-resistant types of wheat, thus showing different drought resistance performance.
Figure 9 Prediction of the regulatory patterns of two candidate lncRNA-miRNA-mRNA modules in the two wheat cultivars.
The original contributions presented in the study are publicly available. This data can be found here: NCBI, PRJNA838787 and PRJNA837867.
NL: conceptualization, experimentation, writing of the original draft, reviewing, and editing. TL: conceptualization, experimentation, reviewing, and editing. FG: experimentation and data curation. JY: data curation. YS: experimentation. SW: data curation and editing. DS: conceptualization, writing, reviewing, and editing. All authors contributed to the article and approved the submitted version.
This work was funded by Fundamental Research Program of Shanxi Province (20210302124148), Scientific and Technologial Innovation Programs of Higher Education Institutions in Shanxi (2021L124) and the Scientific and Technological Innovation Programs of Shanxi Agricultural University (2020BQ30).
The authors declare that the research was conducted in the absence of any commercial or financial relationships that could be construed as a potential conflict of interest.
All claims expressed in this article are solely those of the authors and do not necessarily represent those of their affiliated organizations, or those of the publisher, the editors and the reviewers. Any product that may be evaluated in this article, or claim that may be made by its manufacturer, is not guaranteed or endorsed by the publisher.
The Supplementary Material for this article can be found online at: https://www.frontiersin.org/articles/10.3389/fpls.2022.1011064/full#supplementary-material
Supplementary Figure 1 | Number of predicted lncRNAs using the four analytical methods. CPC, coding potential calculator, CNCI, coding-non-coding index, Pfam, protein family, CPAT, coding potential assessment tool.
Supplementary Figure 2 | Statistical plot of lncRNAs.
Supplementary Figure 3 | Length distribution of miRNAs.
Supplementary Figure 4 | Validation of the expression patterns of randomly selected DEGs by qRT-PCR. The column represents the relative expression level of qRT-PCR, and the broken line represents the log2(fold change) of RNA-Seq. Positive values on the y axis represent up-regulation of genes, and negative values represent down-regulation of genes.
Supplementary Figure 5 | GO enrichment analysis of drought resistance-related DEGs.
Supplementary Figure 6 | KEGG enrichment analysis of drought resistance-related DEGs. (A) Scatter plot of KEGG pathway enrichment analysis. Each circle in the figure represents a KEGG pathway, the ordinate represents the name of the pathway, and the abscissa represents the enrichment factor. The larger the enrichment factor, the more significant the enrichment level of DEGs in the pathway. (B) Histogram of KEGG enrichment analysis. Only KEGG pathways with enriched DEGs greater than or equal to 4 are listed. The numbers in the figure represent the number and percentage of DEGs enriched in each KEGG pathway.
Supplementary Figure 7 | Validation of the expression patterns of randomly selected differentially expressed lncRNAs and differentially expressed miRNAs by qRT-PCR. The column represents the relative expression levels of qRT-PCR, and the broken line the log2(fold change) of RNA-seq or small RNA-seq.
Supplementary Figure 8 | Expression of important DEGs in the lncRNA-miRNA-mRNA regulatory module in different tissues of wheat and under various abiotic stresses. (A) Expression of DEGs in different tissues of wheat. (B) Expression of important DEGs under low phosphorus stress. (C) Expression of important DEGs under cold stress. (D) Expression of important DEGs under heat stress. (E) Expression of important DEGs under salt stress.
Akdogan, G., Tufekci, E. D., Uranbey, S., Unver, T. (2016). miRNA-based drought regulation in wheat. Funct. Integr. Genomics 16, 221–233. doi: 10.1007/s10142-015-0452-1
Allen, E., Xie, Z., Gustafson, A., Carrington, J. (2005). microRNA-directed phasing during trans-acting siRNA biogenesis in plants. Cell 121, 207–222. doi: 10.1016/j.cell.2005.04.004
Araus, J., Slafer, G., Royo, C., Serret, M. (2008). Breeding for yield potential and stress adaptation in cereals. Crit. Rev. Plant Sci. 27, 377–412. doi: 10.1080/07352680802467736
Ashburner, M., Ball, C., Blake, J., Botstein, D., Butler, H., Cherry, J., et al. (2000). Gene ontology: tool for the unification of biology. the gene ontology consortium. Nat. Genet. 1, 25–29. doi: 10.1038/75556
Barrs, H., Weatherley, P. (1962). A reexamination of the relative turgidity techniquefor estimating water deficit in leaves. Aust. J. Biol. Sci. 15, 413–428. doi: 10.1071/BI9620413
Cao, P., Fan, W., Li, P., Hu, Y. (2021). Genome-wide profiling of long noncoding RNAs involved in wheat spike development. BMC Genomics 22, 493. doi: 10.1186/s12864-021-07851-4
Cao, Y., Wei, Q., Liao, Y., Song, H., Li, X., Xiang, C., et al. (2009). Ectopic overexpression of AtHDG11 in tall fescue resulted in enhanced tolerance to drought and salt stress. Plant Cell Rep. 28, 579–588. doi: 10.1007/s00299-008-0659-x
Carrington, J., Ambros, V. (2003). Role of microRNAs in plant and animal development. Science 301, 336–338. doi: 10.1126/science.1085242
Chen, Y., Liu, Z., Feng, L., Zheng, Y., Li, D., Li, X., et al. (2013). Genome-wide functional analysis of cotton (Gossypium hirsutum) in response to drought. PloS One 8, e80879. doi: 10.1371/journal.pone.0080879
Chen, C., Lv, X., Li, J., Yi, H., Gong, J. (2012). Arabidopsis NRT1.5 is another essential component in the regulation of nitrate reallocation and stress tolerance. Plant Physiol. 159, 1582–1590. doi: 10.1104/pp.112.199257
Danquah, A., de Zelicourt, A., Colcombet, J., Hirt, H. (2014). The role of ABA and MAPK signaling pathways in plant abiotic stress responses. Biotechnol. Adv. 32, 40–52. doi: 10.1016/j.biotechadv.2013.09.006
Ewing, B., Hillier, L., Wendl, M. C., Green, P. (1998). Base-calling of automated sequencer traces using phred. i. accuracy assessment. Genome Res. 8, 175–185. doi: 10.1101/gr.8.3.175
Fei, Y., Mao, Y., Shen, C., Wang, R., Zhang, H., Huang, J. (2019). WPMIAS: Whole-degradome-based plant microRNA-target interaction analysis server. Bioinformatics 36, 1937–1939. doi: 10.1093/bioinformatics/btz820
Finn, R., Bateman, A., Clements, J., Coggill, P., Eberhardt, R., Eddy, S., et al. (2013). Pfam: the protein families database. Nucleic Acids Res. 42, D222–D230. doi: 10.1093/nar/gkt1223
Forde, B. (2002). The role of long-distance signalling in plant responses to nitrate and other nutrients. J. Exp. Bot. 366, 39–43. doi: 10.1093/jexbot/53.366.39
Friedlander, M., Mackowiak, S., Li, N., Chen, W., Rajewsky, N. (2012). miRDeep2 accurately identifies known and hundreds of novel microRNA genes in seven animal clades. Nucleic Acids Res. 40, 37–52. doi: 10.1093/nar/gkr688
Guan, M., Møller, I., Schjoerring, J. (2014). Two cytosolic glutamine synthetase isoforms play specific roles for seed germination and seed yield structure in arabidopsis. J. Exp. Bot. 66, 203–212. doi: 10.1093/jxb/eru411
Guttman, M., Rinn, J. (2012). Modular regulatory principles of large non-coding RNAs. Nature 482, 339–346. doi: 10.1038/nature10887
Horst, R., Doehlemann, G., Wahl, R., Hofmann, J., Schmiedl, A., Kahmann, R., et al. (2010). Ustilago maydis infection strongly alters organic nitrogen allocation in maize and stimulates productivity of systemic source leaves. Plant Physiol. 152, 293–308. doi: 10.1104/pp.109.147702
Hou, G., Du, C., Gao, H., Liu, S., Sun, W., Lu, H., et al. (2020). Identification of microRNAs in developing wheat grain that are potentially involved in regulating grain characteristics and the response to nitrogen levels. BMC Plant Biol. 20, 87. doi: 10.1186/s12870-020-2296-7
Kanehisa, M., Goto, S., Kawashima, S., Okuno, Y., Hattori, M. (2004). The KEGG resource for deciphering the genome. Nucleic Acids Res. 32, D277–D280. doi: 10.1093/nar/gkh063
Kaya, C. (2020a). Nitrate reductase is required for salicylic acid-induced water stress tolerance of pepper by upraising the AsA-GSH pathway and glyoxalase system. Physiol. Plantarum 172, 351–370. doi: 10.1111/ppl.13153
Kaya, C., Ashraf, M., Alyemeni, M., Ahmad, P. (2020b). The role of endogenous nitric oxide in salicylic acid-induced up-regulation of ascorbate-glutathione cycle involved in salinity tolerance of pepper (Capsicum annuum l.) plants. Plant Physiol. Biochem. 147, 10–20. doi: 10.1016/j.plaphy.2019.11.040
Kelley, D., Rinn, J. (2012). Transposable elements reveal a stem cell-specific class of long noncoding RNAs. Genome Biol. 13, R107. doi: 10.1186/gb-2012-13-11-r107
Khraiwesh, B., Zhu, J., Zhu, J. (2012). Role of miRNAs and siRNAs in biotic and abiotic stress responses of plants. BBA-Gene Regul. Mech. 1819, 137–148. doi: 10.1016/j.bbagrm.2011.05.001
Kong, L., Zhang, Y., Ye, Z., Liu, X., Zhao, S., Wei, L., et al. (2007). CPC: assess the protein-coding potential of transcripts using sequence features and support vector machine. Nucleic Acids Res. 35, W345–W349. doi: 10.1093/nar/gkm391
Kumar, R., Goswami, S., Sharma, S., Kala, Y., Rai, G., Mishra, D., et al. (2015). Harnessing next generation sequencing in climate change: RNA-seq analysis of heat stress-responsive genes in wheat (Triticum aestivum l.). OMICS 10, 632–647. doi: 10.1089/omi.2015.0097
Langmead, B., Trapnell, C., Pop, M., Salzberg, S. (2009). Ultrafast and memory-efficient alignment of short DNA sequences to the human genome. Genome Biol. 10, R25. doi: 10.1186/gb-2009-10-3-r25
Li, G., Chen, T., Zhang, Z., Li, B., Tian, S. (2020). Roles of aquaporins in plant-pathogen interaction. Plants-Basel 9, 1134. doi: 10.3390/plants9091134
Li, B., Ruotti, V., Stewart, R., Thomson, J., Dewey, C. (2009). RNA-Seq gene expression estimation with read mapping uncertainty. Bioinformatics 26, 493–500. doi: 10.1093/bioinformatics/btp692
Liu, Q., Umeda, M., Uchimiya, H. (1994). Isolation and expression analysis of two rice genes encoding the major intrinsic protein. Plant Mol. Biol. 26, 2003–2007. doi: 10.1007/BF00019511
Li, Q., Zheng, Q., Shen, W., Cram, D., Fowler, D., Wei, Y., et al. (2015). Understanding the biochemical basis of temperature-induced lipid pathway adjustments in plants. Plant Cell 27, 86–103. doi: 10.1105/tpc.114.134338
Love, M., Huber, W., Anders, S. (2014). Moderated estimation of fold change and dispersion for RNA-seq data with DESeq2. Genome Biol. 15, 550. doi: 10.1186/s13059-014-0550-8
Lu, Q., Guo, F., Xu, Q., Cang, J. (2020a). LncRNA improves cold resistance of winter wheat by interacting with miR398. Funct. Plant Biol. 47, 544–557. doi: 10.1071/FP19267
Lu, Q., Xu, Q., Guo, F., Lv, Y., Song, C., Feng, M., et al. (2020b). Identification and characterization of long non-coding RNAs as competing endogenous RNAs in the cold stress response of triticum aestivum. Plant Biol. 22, 635–645. doi: 10.1111/plb.13119
Madhawan, A., Sharma, A., Bhandawat, A., Rahim, M. S., Kumar, P., Mishra, A., et al. (2020). Identification and characterization of long non-coding RNAs regulating resistant starch biosynthesis in bread wheat (Triticum aestivum l.). Genomics 112, 3065–3074. doi: 10.1016/j.ygeno.2020.05.014
Masclaux-Daubresse, C., Reisdorf-Cren, M., Orsel, M. (2010). Leaf nitrogen remobilization for plant development and grain filling. Plant Biol. 10, 23–36. doi: 10.1111/j.1438-8677.2008.00097.x
Mattick, J. (2005). The functional genomics of noncoding RNA. Science 309, 1527–1528. doi: 10.1126/science.1117806
Mattick, J. (2009). The genetic signatures of noncoding RNAs. PloS Genet. 5, e1000459. doi: 10.1371/journal.pgen.1000459
Maurel, C., Santoni, V., Luu, D., Wudick, M., Verdoucq, L. (2009). The cellular dynamics of plant aquaporin expression and functions. Curr. Opin. Plant Biol. 12, 690–698. doi: 10.1016/j.pbi.2009.09.002
Ma, S., Wang, M., Wu, J., Guo, W., Chen, Y., Li, G., et al. (2021). WheatOmics: A platform combining multiple omics data to accelerate functional genomics studies in wheat. Mol. Plant 14, 1965–1968. doi: 10.1016/j.molp.2021.10.006
Meloni, D., Oliva, M., Martinez, C., Cambraia, J. (2003). Photosynthesis and activity of superoxide dismutase, peroxidase and glutathione reductase in cotton under salt stress. Environ. Exp. Bot. 49, 69–76. doi: 10.1016/S0098-8472(02)00058-8
Olea, F., Pérez-García, A., Francisco, R., Rivera, M., Cañas, R., Avila, C., et al. (2004). Up-regulation and localization of asparagine synthase in tomato leaves infexted by the bacterial pathogen pseudomonas syringae. Plant Cell Physiol. 45, 770–780. doi: 10.1093/pcp/pch092
Oono, Y., Kobayashi, F., Kawahara, Y., Yazawa, T., Handa, H., Itoh, T., et al. (2013). Characterisation of the wheat (Triticum aestivum l.) transcriptome by de novo assembly for the discovery of phosphate starvation-responsive genes: gene expression in pi-stressed wheat. BMC Genomics 14, 77. doi: 10.1186/1471-2164-14-77
Pan, Q., Geng, C., Li, D., Xu, S., Mao, D., Umbreen, S., et al. (2019). Nitrate reductase-mediated nitric oxide regulates the leaf shape in arabidopsis by mediating the homeostasis of reactive oxygen species. Int. J. Mol. Sci. 20, 2235 doi: 10.3390/ijms20092235
Parker, J., Newstead, S. (2014). Molecular basis of nitrate uptake by the plant nitrate transporter NRT1.1. Nature 507, 68–72. doi: 10.1038/nature13116
Pertea, M., Kim, D., Pertea, G., Leek, J., Salzberg, S. (2016). Transcript-level expression analysis of RNA-seq experiments with HISAT, StringTie and ballgown. Nat. Protoc. 11, 1650–1667. doi: 10.1038/nprot.2016.095
Qin, L., Li, Y., Li, D., Xu, W., Zheng, Y., Li, X. (2014). Arabidopsis drought-induced protein Di19-3 participates in plant response to drought and high salinity stresses. Plant Mol. Biol. 86, 609–625. doi: 10.1007/s11103-014-0251-4
Quan, X., Zeng, J., Ye, L., Chen, G., Han, Z., Shah, J., et al. (2016). Transcriptome profiling analysis for two Tibetan wild barley genotypes in responses to low nitrogen. BMC Plant Biol. 16, 30–45. doi: 10.1186/s12870-016-0721-8
Redinbaugh, M., Campbell, W. (1998). Nitrate regulation of the oxidative pentose phosphate pathway in maize (Zea mays l.) root plastids: induction of 6-phosphogluconate dehydrogenase activity, protein and transcript levels. Plant Sci. 134, 129–140. doi: 10.1016/S0168-9452(98)00048-X
Rhodes, D., Handa, S., Bressan, R. (1987). Metabolic changes associated with adaptation of plant cells to water stress. Plant Physiol. 82, 890–903. doi: 10.1104/pp.82.4.890
Song, G., Zhang, R., Zhang, S., Li, Y., Gao, J., Han, X., et al. (2017). Response of microRNAs to cold treatment in the young spikes of common wheat. BMC Genomics 18, 212. doi: 10.1186/s12864-017-3556-2
Stif, P., Hirasawa, M., Cassan, N., Lagoutte, B., Tripathy, J., Knaff, D. (2009). New insights into the catalytic cycle of plant nitrite reductase. electron transfer kinetics and charge storage. Biochemistry 48, 2828–2838. doi: 10.1021/bi802096f
Sun, L., Luo, H., Bu, D., Zhao, G., Yu, K., Zhang, C., et al. (2013). Utilizing sequence intrinsic composition to classify protein-coding and long non-coding transcripts. Nucleic Acids Res. 41, e166. doi: 10.1093/nar/gkt646
Sun, Y., Song, K., Sun, L., Qin, Q., Jiang, T., Jiang, Q., et al. (2020). Morpho-physiological and transcriptome analysis provide insights into the effects of zinc application on nitrogen accumulation and metabolism in wheat (Triticum aestivum l.). Plant Physiol. Biochem. 149, 111–120. doi: 10.1016/j.plaphy.2020.01.038
Trapnell, C., Williams, B., Pertea, G., Mortazavi, A., Kwan, G., Baren, M., et al. (2010). Transcript assembly and quantification by RNA seq reveals unannotated transcripts and isoform switching during cell differentiation. Nat. Biotechnol. 28, 511–515. doi: 10.1038/nbt.1621
Wang, K., Chang, H. (2011). Molecular mechanisms of long noncoding RNAs. Mol. Cell 43, 904–914. doi: 10.1016/j.molcel.2011.08.018
Wang, L., Park, H., Dasari, S., Wang, S., Kocher, J., Li, W. (2013). CPAT: Coding-potential assessment tool using an alignment-free logistic regression model. Nucleic Acids Res. 41, e74. doi: 10.1093/nar/gkt006
Wu, B., Jiang, S., Zhang, M., Guo, X., Wang, S., Xin, X. (2021). Exploration of wheat yellow mosaic virus-responsive miRNAs and their targets in wheat by miRNA and degradome sequencing. J. Biosci. 46, 83. doi: 10.1007/s12038-021-00207-w
Xu, H., Chen, W., Zheng, F., Tang, D., Liu, D., Wang, G., et al. (2020). Reconstruction and analysis of the aberrant lncRNA-miRNA-mRNA network in systemic lupus erythematosus. Lupus 29, 398–406. doi: 10.1177/0961203320908927
Xu, G., Fan, X., Miller, A. (2012). Plant nitrogen assimilation and use efficiency. Annu. Rev. Plant Biol. 63, 153–182. doi: 10.1146/annurev-arplant-042811-105532
Yu, Z., Duan, X., Luo, L., Dai, S., Ding, Z., Xia, G. (2020). How plant hormones mediate salt stress responses. Trends Plant Sci. 25, 1117–1130. doi: 10.1016/j.tplants.2020.06.008
Zhang, Y., Liu, Z., Khan, A. A., Lin, Q., Han, Y., Mu, P., et al. (2016). Expression partitioning of homeologs and tandem duplications contribute to salt tolerance in wheat (Triticum aestivum l.). Sci. Rep. 6, 21476. doi: 10.1038/srep21476
Zhou, W., Shi, H., Wang, Z., Zhao, Y., Gou, X., Li, C., et al. (2020). Identification of lncRNAs involved in wheat tillering development in two pairs of near-isogenic lines. Funct. Integr. Genomics 20, 669–679. doi: 10.1007/s10142-020-00742-z
Keywords: wheat, drought stress, long noncoding RNA, micro RNA, mRNA
Citation: Li N, Liu T, Guo F, Yang J, Shi Y, Wang S and Sun D (2022) Identification of long non-coding RNA-microRNA-mRNA regulatory modules and their potential roles in drought stress response in wheat (Triticum aestivum L.). Front. Plant Sci. 13:1011064. doi: 10.3389/fpls.2022.1011064
Received: 03 August 2022; Accepted: 23 September 2022;
Published: 11 October 2022.
Edited by:
Ji Huang, Nanjing Agricultural University, ChinaReviewed by:
Yuhan Fei, Tsinghua University, ChinaCopyright © 2022 Li, Liu, Guo, Yang, Shi, Wang and Sun. This is an open-access article distributed under the terms of the Creative Commons Attribution License (CC BY). The use, distribution or reproduction in other forums is permitted, provided the original author(s) and the copyright owner(s) are credited and that the original publication in this journal is cited, in accordance with accepted academic practice. No use, distribution or reproduction is permitted which does not comply with these terms.
*Correspondence: Daizhen Sun, c2R6NjRAMTI2LmNvbQ==
†These authors have contributed equally to this work
Disclaimer: All claims expressed in this article are solely those of the authors and do not necessarily represent those of their affiliated organizations, or those of the publisher, the editors and the reviewers. Any product that may be evaluated in this article or claim that may be made by its manufacturer is not guaranteed or endorsed by the publisher.
Research integrity at Frontiers
Learn more about the work of our research integrity team to safeguard the quality of each article we publish.