- Centre for Soybean Research of the State Key Laboratory of Agrobiotechnology and School of Life Sciences, The Chinese University of Hong Kong, Shatin, Hong Kong SAR, China
Arabidopsis thaliana has been used regularly as a model plant in gene expression studies on transcriptional reprogramming upon pathogen infection, such as that by Pseudomonas syringae pv. tomato DC3000 (Pst DC3000), or when subjected to stress hormone treatments including jasmonic acid (JA), salicylic acid (SA), and abscisic acid (ABA). Reverse transcription-quantitative polymerase chain reaction (RT-qPCR) has been extensively employed to quantitate these gene expression changes. However, the accuracy of the quantitation is largely dependent on the stability of the expressions of reference genes used for normalization. Recently, RNA sequencing (RNA-seq) has been widely used to mine stably expressed genes for use as references in RT-qPCR. However, the amplification step in RNA-seq creates an intrinsic bias against those genes with relatively low expression levels, and therefore does not provide an accurate quantification of all expressed genes. In this study, we employed mass spectrometry-based label-free quantification (LFQ) in proteomic analyses to identify those proteins with abundances unaffected by Pst DC3000 infection. We verified, using RT-qPCR, that the levels of their corresponding mRNAs were also unaffected by Pst DC3000 infection. Compared to commonly used reference genes for expression studies in A. thaliana upon Pst DC3000 infection, the candidate reference genes reported in this study generally have a higher expression stability. In addition, using RT-qPCR, we verified that the mRNAs of the candidate reference genes were stably expressed upon stress hormone treatments including JA, SA, and ABA. Results indicated that the candidate genes identified here had stable expressions upon these stresses and are suitable to be used as reference genes for RT-qPCR. Among the 18 candidate reference genes reported in this study, many of them had greater expression stability than the commonly used reference genes, such as ACT7, in previous studies. Here, besides proposing more appropriate reference genes for Arabidopsis expression studies, we also demonstrated the capacity of mass spectrometry-based LFQ to quantify protein abundance and the possibility to extend protein expression studies to the transcript level.
Introduction
Pathogen infection of plants has been a major cause of yield loss in agriculture (Gorshkov and Tsers, 2022). Upon pathogen infection, plants perceive the signal elicited by the secretions from the pathogens known as pathogen-associated molecular patterns (PAMPs) or microbe-associated molecular patterns (MAMPs; Medzhitov and Janeway, 1997), through pattern recognition receptors (PRRs). The signal perception triggers a series of defense responses including the generation of reactive oxygen species (ROS), fluctuations in the cellular calcium level, activation of proteins, such as mitogen-activated protein kinases (MAPKs), and GTP-binding proteins (G-proteins), and synthesis of stress hormones such as jasmonic acid (JA), salicylic acid (SA), and ethylene (Zhang and Zhou, 2010; Li et al., 2016b; Ku et al., 2020). These signaling events regulate the expressions of defense-related genes such as Pathogenesis-Related (PR) genes (Zhang and Zhou, 2010; Li et al., 2016b; Ku et al., 2020). PAMP-triggered immunity (PTI) describes the general pathogen resistance responses in plants (Zhang and Zhou, 2010; Li et al., 2016b; Ku et al., 2020). Some plants are known to have specific pathogen recognition mechanisms termed effector-triggered immunity (ETI; Dodds and Rathjen, 2010). ETI triggers hypersensitive response (HR) and usually leads to programmed cell death (PCD) at the area of infection to prevent the invading pathogens from spreading. In HR, ROS is produced to cause a series of cellular events including the disruption of cell membrane, thickening of the cell wall, and the production of stress hormones such as JA and SA. PTI and ETI lead to systemic acquired resistance (SAR) against a broad spectrum of pathogens (Dodds and Rathjen, 2010; Zhang and Zhou, 2010; Li et al., 2016b; Ku et al., 2020). The interactions among different signaling pathways, such as those of JA, SA, and abscisic acid (ABA), result in further complexity of the regulatory processes when under stress (Ku et al., 2018). These cellular events involve extensive transcriptional reprogramming (Li et al., 2016a). RNA sequencing (RNA-seq) has been used as the platform to study the global gene expression changes in plants upon pathogen infection (Zhu et al., 2013; Martin et al., 2016; Matic et al., 2016; Gupta and Senthil-Kumar, 2017; Poretti et al., 2021). Reverse transcription-quantitative polymerase chain reaction (RT-qPCR) is regarded as the gold standard for quantifying gene expressions due to its sensitivity, accuracy, and reproducibility (Gökmen-Polar, 2019). Although digital PCR (dPCR) has been shown to out-perform RT-qPCR in terms of sensitivity, accuracy, and reproducibility (Gökmen-Polar, 2019), RT-qPCR remains a more common approach for routine gene expression quantification due to the lower cost compared to dPCR. However, the accuracy of expression quantification by RT-qPCR is largely dependent on the expression stability of the reference gene used for normalization.
RNA-seq is commonly employed to mine reference genes for RT-qPCR (Yim et al., 2015; Kudo et al., 2016; Carmona et al., 2017; Zhou et al., 2017; Pombo et al., 2019). However, the accuracy of RNA-seq data has been a concern. PCR bias and GC content bias are hurdles for the accurate quantitative analysis of high-throughput sequencing data. In addition, the accuracy of quantitative gene expression analyses is highly influenced by the algorithms and pipelines for RNA-seq data analyses (Benjamini and Speed, 2012; Parekh et al., 2016; Corchete et al., 2020). In an attempt to assess the different RNA-seq data analysis pipelines, it was found that each of the 192 pipelines examined in the study had its advantages and disadvantages for quantitative gene expression analysis (Corchete et al., 2020).
Many previous researches have attempted to determine the correlation between mRNA and protein levels in plants. It was concluded that the correlation between mRNA levels and protein abundances is largely dependent on the plant species, tissue type, developmental stage, and stress condition of the plant (Nakaminami et al., 2014; Ponnala et al., 2014; Wang et al., 2017; Ding et al., 2020; Ren et al., 2022). Nevertheless, a significant correlation between mRNA and protein levels has been reported (Arefian et al., 2019; Ding et al., 2020; Shu et al., 2022; Zhu et al., 2022). Recent technologies have enabled high-throughput proteomic analyses through mass spectrometry, which does not involve amplification steps and thus may complement the limitations due to biased quantification in RNA-seq. In this study, we employed mass spectrometry-based label-free quantification (LFQ) to search for proteins that have stable abundances in Arabidopsis thaliana despite Pseudomonas syringae pv. tomato DC3000 (Pst DC3000) infection and tested the expression stability of their corresponding mRNAs by RT-qPCR. Compared to reference genes commonly used in previous studies, the mRNA levels of these proteins were generally more stable upon Pst DC3000 infection. We then extended the assessment of the stability of mRNA levels of these proteins to stress hormone treatments, including JA, SA and ABA. Altogether, 18 candidate reference genes were identified and tested. Using A. thaliana as the model, we revealed a set of more suitable reference genes for expression studies on Pst DC3000 infection and stress hormone treatments. We also demonstrated the advantage of using mass spectrometric analysis for mining genes which have stable protein and mRNA abundances upon various treatments.
Materials and methods
Plant materials and treatment conditions
For Pst DC3000 inoculation, A. thaliana plants (Col-0) were grown on Floragard potting soil in a growth chamber under these conditions: 22–24°C, light intensity 80–120 μE with a 16-h light:8-h dark cycle; relative humidity 70–80%. The rosette leaves of 5-week-old plants were inoculated with Pst DC3000 according to the protocol reported in previous studies (Cheung et al., 2013; Mi et al., 2013). At 0 day and 3 days post-inoculation (dpi), the aerial parts of the inoculated plants were collected, snap-frozen in liquid nitrogen, and stored at –80°C. The tissues of three individual plants were pooled as one biological replicate for total protein or total RNA extraction. A total of three biological replicates per treatment were collected for total protein extraction while two biological replicates per treatment were collected for total RNA extraction.
For JA treatment, the seeds of A. thaliana Col-0 were surface-sterilized by shaking in 100% household bleach for 3 min. After that, the bleach was removed, and the seeds were rinsed three times with sterilized water. The surface-sterilized seeds were then placed on Murashige & Skoog (MS) agar plates supplemented with 3% sucrose with or without 5 μM JA (Zhang et al., 2017). The seeded agar plates were then kept at 4°C in the dark for 2 days to break dormancy. After that, the plates were moved into a growth chamber under these conditions: 22°C–24°C, light intensity 80–120 μE with a 16-h light:8-h dark cycle, for 17 days. Then the seedlings were removed from the agar plates, frozen in liquid nitrogen, and stored at –80°C before total RNA extraction. Samples for two biological replicates per treatment were collected. Each biological replicate consisted of at least seven seedlings pooled together for total RNA extraction.
For SA treatment, the seeds of A. thaliana Col-0 were surface-sterilized as described above. The surface-sterilized seeds were then placed on MS agar plates without sucrose and with or without 50 μM SA (Sakurai et al., 2011; Sugano et al., 2016; Zhang et al., 2017). The seeded plates were then kept at 4°C in the dark for 2 days before being moved into a growth chamber under the same growth conditions as above for 17 days. After that, the seedlings were harvested from the agar plates, frozen in liquid nitrogen, and stored at –80°C before total RNA extraction. Two biological replicates per treatment were collected. Each biological replicate consisted of at least seven seedlings pooled together for total RNA extraction.
For ABA treatment, the seeds of A. thaliana Col-0 were sown on Floragard potting soil in a growth chamber under the same growth conditions as described above. Then the rosette leaves of 4-week-old plants were detached and floated on a perfusion solution (50 mM KCl, 10 mM MES, pH 7.0) under light for 2 h before being treated with ABA. ABA was first dissolved in 10% (v/v) methanol (MeOH) before being added to the perfusion solution to reach a final concentration of ABA at 10 μM and MeOH at 0.1% (v/v). The detached leaves were treated with ABA under light for 2 h. Then they were frozen in liquid nitrogen and stored at –80°C before total RNA extraction. Two biological replicates were sampled for each treatment, and each biological replicate consisted of the rosette leaves of at least three plants pooled together for total RNA extraction.
Protein extraction and protein profile analysis by liquid chromatography–tandem mass spectrometry (LC–MS/MS)
Total protein was extracted from the plant samples according to a previously described protocol (Marx et al., 2016; Cheng et al., 2022), with minor modifications. The plant samples were ground to a fine powder in liquid nitrogen using mortar and pestle. Around 100 mg of the powder was resuspended in five volumes of total protein extraction buffer [290 mM sucrose, 250 mM Tris (pH 7.6), 50 mM Na pyrophosphate, 25 mM EDTA (pH 8.0), 25 mM NaF, 10 mM KCl, 1 mM (NH4)6Mo7O24, 1 mM phenylmethylsulfonyl fluoride (PMSF), and 1X Halt™ Protease Inhibitor Cocktail (Cat#78430, Thermo Fisher Scientific, Waltham, MA, United States)]. The plant proteins in the extract were precipitated using the chloroform/methanol method (Wessel and Flügge, 1984). After that, the protein pellet was lyzed with five volumes of lysis buffer (w/v) [8 M urea, 50 mM Tris–HCl (pH 8.0), 30 mM NaCl, 20 mM sodium butyrate, 1 mM CaCl2, and 1X Halt™ Protease Inhibitor Cocktail (Cat#78430, Thermo Fisher Scientific, Waltham, MA, United States)]. The protein concentration was determined using the Pierce™ BCA Protein Assay Kit (Cat#23225, Thermo Fisher Scientific, Waltham, MA, United States). Then, 10 μg of each protein sample was reduced with 5 mM dithiothreitol (DTT) at 37°C for 30 min, alkylated with 20 mM iodoacetamide at room temperature for 30 min, and underwent a final reduction with 5 mM DTT at 37°C for 30 min. Then the protein mixture was incubated with trypsin in a ratio of 1/20 (w/w) of the protein amount at 37°C overnight.
The peptides resulting from trypsin digestion were desalted using Pierce™ C18 Spin Column (Cat#89873, Thermo Fisher Scientific, Waltham, MA, United States), and each sample was analyzed independently for protein identification using LC–MS/MS. Five hundred nanograms of desalted peptides from each sample were injected into the LC Ultimate 3000 RSLCnano system equipped with a C-18 μ-precolumn (300-μm i.d. × 5 mm) with an Acclaim Pepmap RSLC nanoViper C-18 column (75 μm × 25 cm) coupled to the Orbitrap Fusion Lumos Tribrid mass spectrometer (Thermo Fisher Scientific, Waltham, MA, United States). The peptide samples were separated against the gradient profile with a 50°C chamber at a flow rate of 0.3 μLmin−1, using a mixture of ultrapure water with 1.9% acetonitrile and 0.1% formic acid as mobile phase A and ultrapure water with 2% acetonitrile and 0.1% formic acid as mobile phase B, with the following gradient profile setting in the LC: 0% mobile phase B for the initial 5 min; at 5–8 min, 0–6% mobile phase B; 8–48 min, 6–18% mobile phase B; 48–58 min, 18–30% mobile phase B; 58–65 min, 30–80% mobile phase B; and then at 65–75 min, 0% mobile phase B for re-equilibration of the column. Each desalted peptide sample was analyzed twice (as technical replicates) to eliminate the instrumental variations.
The raw data files were generated using Xcalibur software (Thermo Fisher Scientific, Waltham, MA, United States) for MS/MS identification using Proteome Discoverer v2.4 (Thermo Fisher Scientific, Waltham, MA, United States) against the A. thaliana protein database (TAIR10) with the built-in SEQUEST HT program at the following settings: MS precursor mass tolerance of 10 ppm, fragment mass tolerance of 0.02 Da, a maximum of 2 missed trypsin cleavage, fixed N-terminal protein acetylation (+42.011 Da), dynamic cysteine carbamidomethylation (+57.021 Da) and methionine oxidation (+15.995 Da). Peptide validation using the built-in Percolator program was accepted at a false discovery rate (FDR) with a q-value <0.01. Samples were compared using the LFQ method according to the protocol in Proteome Discoverer v2.4 (Thermo Fisher Scientific, Waltham, MA, United States). The proteomic dataset was deposited to PRIDE (PRoteomics IDEntifications Database; Project accession: PXD035677). The grouping of the protein samples from different treatments was presented using PCA plot (Supplementary Figure S1). Proteins appearing in all biological replicates with an adjusted value of p > 0.05 for the difference in the abundance (using the Benjamini-Hochberg correction) between 0 and 3 dpi with Pst DC3000, i.e., no significant difference in abundance, were short-listed as possible reference gene candidates. Among the short-listed candidates, 18 proteins were highly ranked as stably expressed by Proteome Discoverer v2.4 (Thermo Fisher Scientific, Waltham, MA, United States) and were included in the final list of candidate reference genes (Supplementary Table S1).
Primer design
Primers for RT-qPCR were designed according to the mRNA sequences of the candidate reference genes. The primer specificity was determined by the Primer-BLAST function on the NCBI platform against the A. thaliana genome (taxid: 3702) and validated by melting curve analysis after RT-qPCR (Supplementary Figure S2). For references genes commonly used in previous studies, the primer sequences were adopted from the corresponding publications (Czechowski et al., 2005; Jeong et al., 2011; Huang et al., 2013; Chen et al., 2014; Cheong et al., 2014; Cuéllar Pérez et al., 2014; Kim and Hwang, 2014; Zhang et al., 2015; Huot et al., 2017; Jia et al., 2018; Wu et al., 2019; Cui et al., 2021; Romero-Pérez et al., 2021; Gao et al., 2022). The primer sequences are listed in Supplementary Table S1.
Total RNA extraction, cDNA synthesis, and RT-qPCR
The plant samples were ground in liquid nitrogen to a fine powder. After that, total RNA was extracted using Trizol™ Reagent (Cat#15596018, Thermo Fisher Scientific, Waltham, MA, United States) according to the manufacturer’s protocol. Then, the RNA was quantitated using the Qubit™ RNA Broad Range (BR) Assay Kit (Cat#Q10211, Thermo Fisher Scientific, Waltham, MA, United States) with the use of Qubit 2.0 Fluorometer (Cat#Q32866, Thermo Fisher Scientific, Waltham, MA, United States) according to the manufacturer’s protocol. For Pst DC3000 infection and ABA treatment, 320 ng of total RNA was used for cDNA synthesis. For JA and SA treatments, 640 ng of total RNA was used for cDNA synthesis. For cDNA synthesis, the RNA was first treated with DNase I according to the manufacturer’s protocol (Cat#18068015, Thermo Fisher Scientific, Waltham, MA, United States). The DNase I-treated RNA was then subjected to cDNA synthesis using the High-Capacity cDNA Reverse Transcription Kit with RNase Inhibitor (Cat#4374966, Thermo Fisher Scientific, Waltham, MA, United States) according to the manufacturer’s protocol, with the random primers being replaced by oligo(dT)20 to make up a final concentration of 20 μM oligo(dT)20. After that, the cDNA was diluted 30 folds before being used for qPCR. For qPCR, 3 μl diluted cDNA was added to a 20-μL qPCR reaction mix with 1X SsoAdvanced Universal SYBR Green Supermix (Cat#1725270, Bio-Rad, Hercules, CA, United States) and 0.15 μM each of forward and reverse primers. Quantitative PCR and melting curve analyses (from 95°C to 65°C) were performed using a CFX96 Touch Real-Time PCR system (Bio-Rad, Hercules, CA, United States).
Stability analysis of the candidate reference genes and reference genes commonly used in previous studies
In each treatment, three technical replicates of the qPCR were performed for each primer pair. The six Ct values from the six technical replicates in total of two biological replicates were used as the inputs for stability analyses using programs including geNorm via the R-based package ctrlGene (ver. 1.0.0; Vandesompele et al., 2002; Zhong, 2019), Normfinder via RefFinder (Andersen et al., 2004; Xie et al., 2012), BestKeeper (Pfaffl et al., 2004), the comparative ΔCt method (Silver et al., 2006), and RefFinder (Xie et al., 2012) with default parameters.
Results
Identification of stably expressed proteins unaffected by Pst DC3000 infection
Five-week-old A. thaliana plants (Col-0) were inoculated with Pst DC3000. Total protein was extracted from the inoculated plants at 0 and 3 dpi, and then subjected to mass spectrometry-based LFQ analyses. Eighteen proteins were found to have stable abundances between 0 and 3 dpi (Figure 1). The gene names and accession numbers corresponding to the proteins are listed in Supplementary Table S1. The abundance of the proteins corresponding to 12 reference genes commonly used in previous studies (Czechowski et al., 2005; Jeong et al., 2011; Huang et al., 2013; Chen et al., 2014; Cheong et al., 2014; Cuéllar Pérez et al., 2014; Kim and Hwang, 2014; Zhang et al., 2015; Huot et al., 2017; Jia et al., 2018; Wu et al., 2019; Cui et al., 2021; Romero-Pérez et al., 2021; Gao et al., 2022) were also investigated using the protein dataset. The gene names and accession numbers are listed in Supplementary Table S1. Among the 12 reference genes commonly used in previous studies, ACT1, ACT8, PP2AA3, UBQ5, UBC9, and TIP41 were found from the protein dataset. The abundances of ACT1, ACT8, PP2AA3, and UBQ5 did not show statistically significant difference between 0 dpi and 3 dpi, the abundance of UBC9 was higher at 0 dpi compared to 3 dpi, while the abundance of TIP41 was too low to be quantified (Supplementary Figure S3). The protein abundance could be found from the protein dataset deposited to PRIDE (PRoteomics IDEntifications Database; Project accession: PXD035677). The chromatograms of the LC/MS–MS analyses are shown in Supplementary Figures S4, S5.
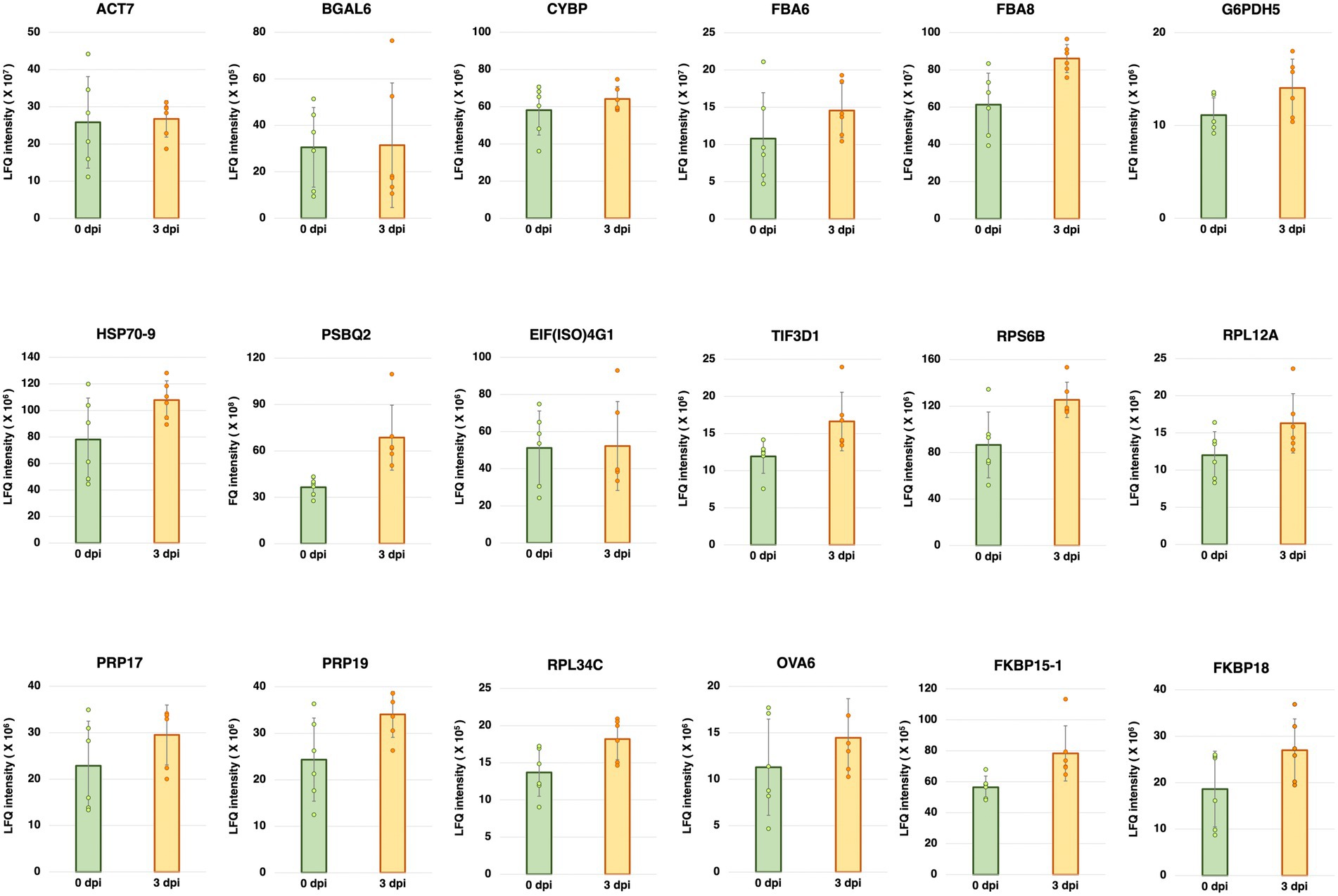
Figure 1. Label-free quantification (LFQ) intensities of the candidate reference genes having stable abundances between 0 and 3 days post-inoculation (dpi) with Pseudomonas syringae pv. tomato DC3000 (Pst DC3000) in 5-week-old A. thaliana ecotype Col-0. The protein abundance levels of these candidate reference genes, calculated from LFQ using Proteome Discoverer v2.4 (Thermo Fisher Scientific, Waltham, MA, United States), were not statistically different between 0 and 3 dpi (p > 0.05). Three individual plants were pooled as one biological replicate, each with two technical repeats of LFQ analysis. Error bar represents the standard deviation of a total of six technical repeats based on three biological replicates.
Amplification specificity and efficiency
To test the abundance of the mRNAs corresponding to the stably expressed proteins shown in Figure 1, specific primers were designed based on their mRNA sequences. The specificity of each primer pair was first determined using the Primer-BLAST function on the NCBI platform against the A. thaliana genome (taxid: 3702) and validated by melting curve analyses after RT-qPCR (Supplementary Figure S2).
Using RT-qPCR, we analyzed the expression levels of the 18 candidate reference genes and 12 reference genes commonly used in previous studies (Supplementary Table S1; Czechowski et al., 2005; Jeong et al., 2011; Huang et al., 2013; Chen et al., 2014; Cheong et al., 2014; Cuéllar Pérez et al., 2014; Kim and Hwang, 2014; Zhang et al., 2015; Huot et al., 2017; Jia et al., 2018; Wu et al., 2019; Cui et al., 2021; Romero-Pérez et al., 2021; Gao et al., 2022) at 0 and 3 dpi with Pst DC3000 inoculation. We also evaluated the expression stability of these candidate reference genes in stress hormone treatments, including JA, SA, and ABA. The distributions of the Ct values of the candidate reference genes were shown by boxplots (Figure 2). In Pst DC300 infection, the average expression values of the reference genes commonly used in previous studies ranged from the log2 values of 4.71 and 5.14 (Figure 2A), which are equivalent to Ct values of 26.26 to 35.38. Meanwhile, the average expression values of the candidate reference genes predicted in this study ranged from the log2 values of 4.41–5.05 (Figure 2A), which are equivalent to Ct values of 21.20-33.21. In Pst DC3000 infection, the expression levels of the reference genes commonly used in previous studies are generally lower than those of the candidate reference genes predicted in this study. Considering all the treatments, the average expression values of the candidate reference genes predicted in this study were in the range of log2 values of 4.40–5.10, equivalent to the Ct values of 21.12–34.22. Based on the Ct values obtained from RT-qPCR and the LFQ intensities obtained from the mass spectrometry-based proteomic analysis, the mRNA level was found to be positively correlated to the protein abundance (Supplementary Figure S6).
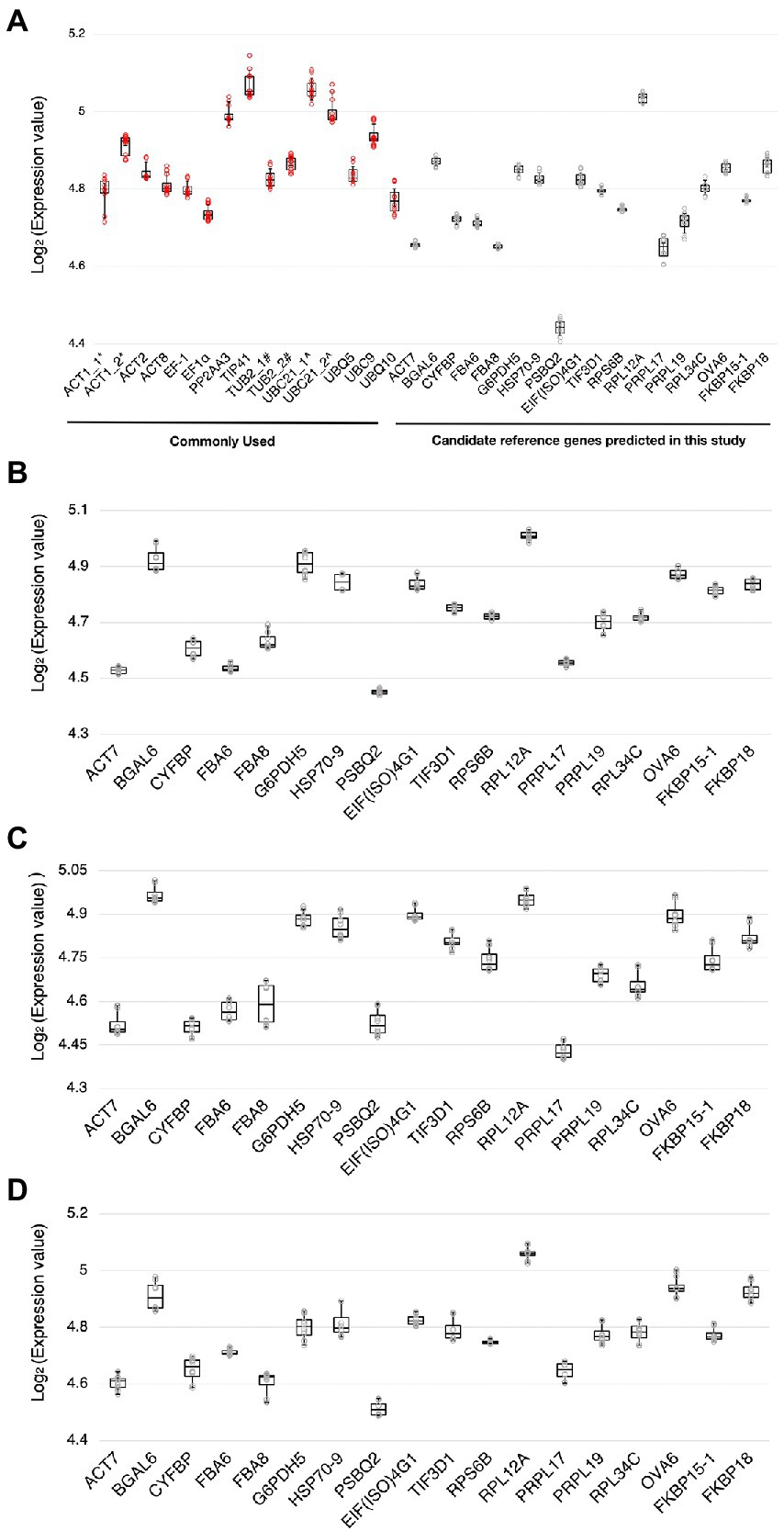
Figure 2. Ranges of expression levels of the candidate reference genes and reference genes commonly used in previous studies. (A) A boxplot showing the range of expression values [log2(Ct value)] of the candidate reference genes and the reference genes commonly used in previous studies obtained by RT-qPCR when subjected to Pst DC3000 at 0 dpi and 3 dpi. (B–D) Boxplots showing the range of expression values [log2(Ct value)] of the candidate reference genes when subjected to jasmonic acid (JA) (B), salicylic acid (SA) (C), and abscisic acid (ABA) (D) treatments and the corresponding mock control treatments. The solid line inside each box represents the median expression value and the lower and upper edges of the boxes denote the 25th and 75th percentiles, while the whiskers represent the maximum and the minimum values. Each dot represents the expression value calculated from each technical replicate of RT-qPCR. Three technical repeats were performed for each biological replicate, with two biological replicates in total.
Stability analysis of the mRNAs
Expression stability comparison between the candidate reference genes and reference genes commonly used in previous studies upon Pst DC3000 infection
To compare the expression stabilities of the 18 candidate reference genes predicted in this study and those of the 12 reference genes commonly used in previous studies, the expression stabilities were ranked using geNorm (Vandesompele et al., 2002) and RefFinder (Xie et al., 2012).
Stability analysis by geNorm
In geNorm analysis, the expression stability of the candidate reference genes was calculated using pairwise comparisons (Vandesompele et al., 2002), and presented in the form of the average expression stability value (M), with a lower M representing a higher stability (Vandesompele et al., 2002). Genes with M < 1.5 are commonly considered stably expressed (Vandesompele et al., 2002; Walker et al., 2009; Jin et al., 2019; Fu et al., 2022). All the candidate reference genes and the reference genes commonly used in previous studies showed M < 1.5 (Figure 3). However, the M values of the candidate reference genes reported in this study were generally lower than those of the reference genes commonly used in previous studies (Figure 3).
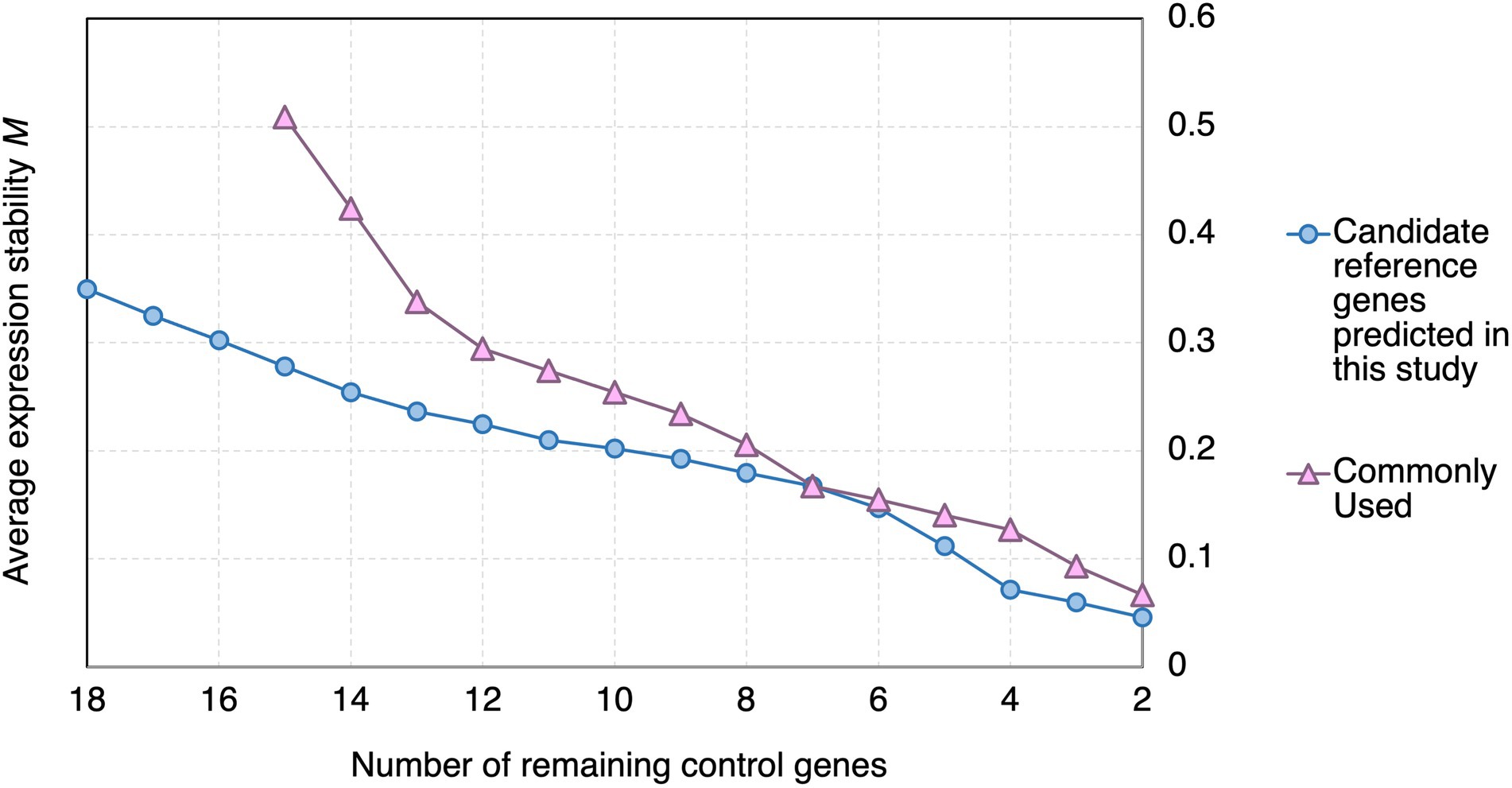
Figure 3. geNorm analysis of the average stability values of the candidate reference genes predicted in this study and reference genes commonly used in previous studies upon Pst DC3000 infection. Average expression stability values (M) of the remaining control genes during the step exclusion of the least stable control among the reference gene candidate upon Pst DC3000 infection using geNorm. The higher the average M, the lower rank of the expression stability of the reference gene candidate.
Using geNorm analysis, the optimal number of reference genes required for expression normalization in RT-qPCR was determined by the pairwise variation calculation (Vn/Vn + 1), in which n represents the number of reference genes required for expression normalization in RT-qPCR. All the candidate reference genes and the reference genes commonly used in previous studies showed Vn/Vn + 1 values smaller than 0.15, which is the cut-off value indicating the expression stability (Vandesompele et al., 2002; Figure 4). However, the candidate reference genes predicted in this study showed general smaller V values compared to the reference genes commonly used in previous studies (Figure 4).
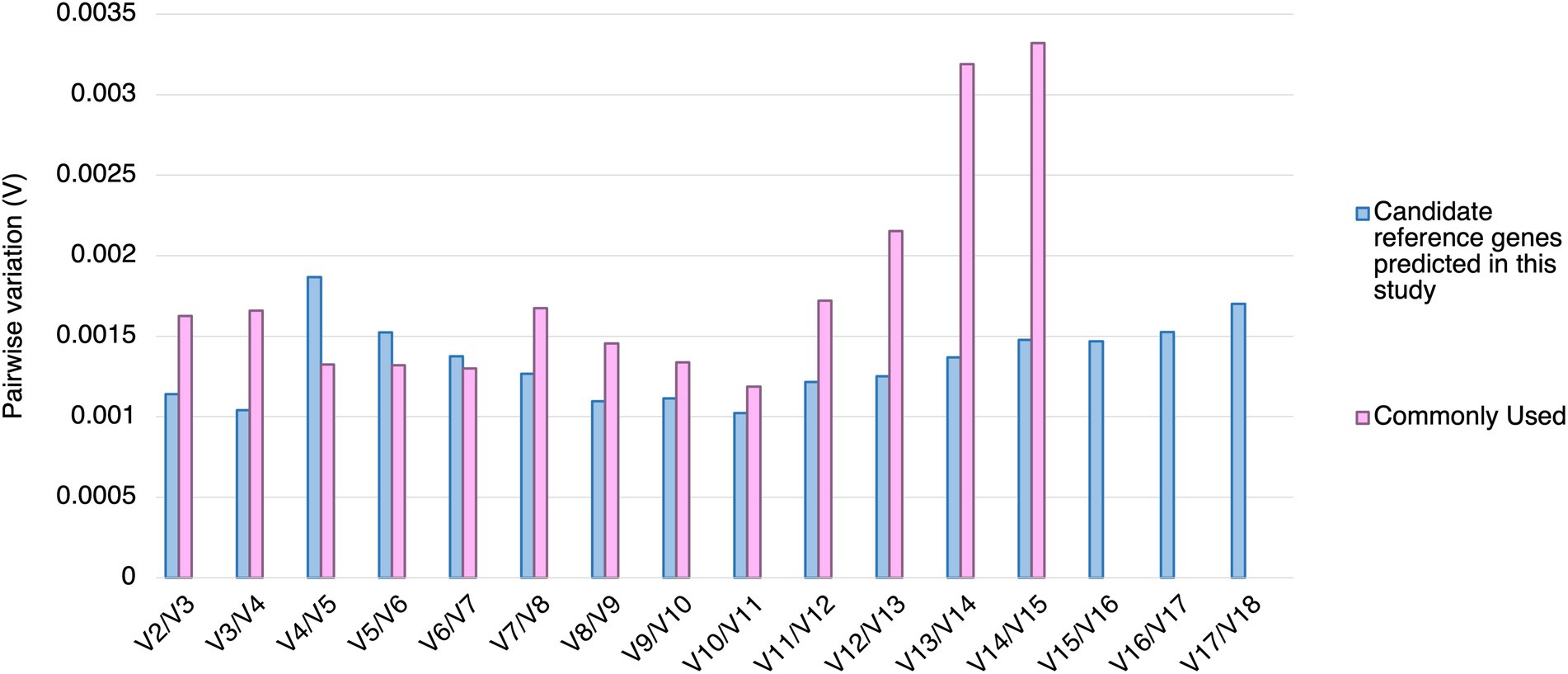
Figure 4. Pairwise variation (V) of the candidate reference genes predicted in this study and reference genes commonly used in previous studies calculated by geNorm. The pairwise variation representing (Vn/Vn + 1). The threshold value of V for accessing the optimal number of the reference genes for RT-qPCR normalization is 0.15. n represents the number of reference gene required for expression normalization in RT-qPCR.
Comprehensive stability analysis by RefFinder
The results of geNorm analysis suggest that the candidate reference genes predicted in this study are generally more stable than the reference genes commonly used in previous studies upon Pst DC3000 infection. The stabilities were further analyzed using RefFinder (Xie et al., 2012), which calculates the comprehensive gene stability by integrating the algorithms of geNorm, NormFinder, BestKeeper, and the comparative ΔCt method (Xie et al., 2012). The result from RefFinder also suggests that the candidate reference genes reported in this study are generally more stable than the reference genes commonly used in previous studies upon Pst DC3000 infection (Figure 5). Among all the genes, ACT7, TIF3D1, and RPS6B, which are candidate reference genes reported in this study, were the most stably expressed upon Pst DC3000 infection (Figure 5).
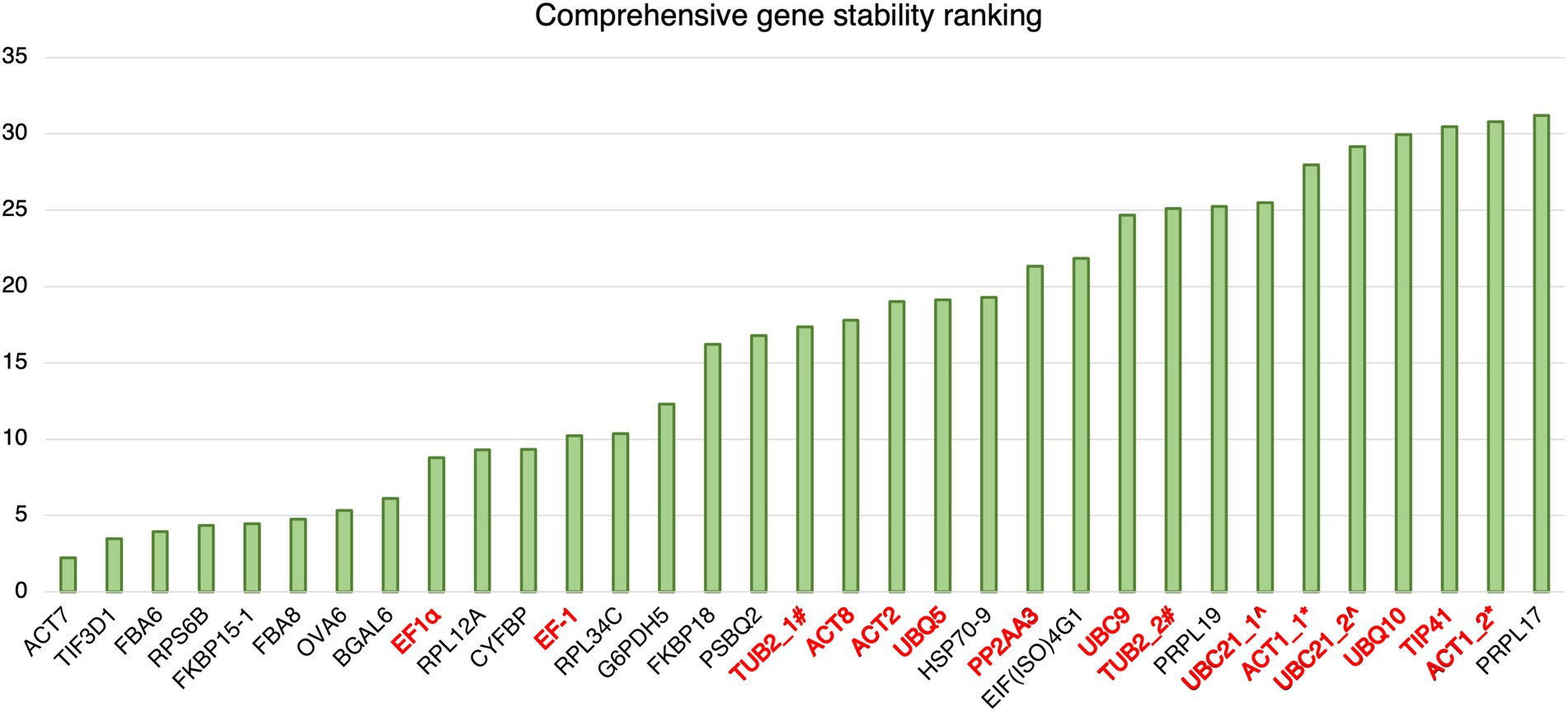
Figure 5. Comprehensive gene stability ranking of the predicted reference gene candidates and the commonly used reference gene for Pst DC3000 inoculation. The stability ranking was generated using the RefFinder. A lower stability ranking refers to a higher stability of the reference gene. Gene names in black: candidate reference genes reported in this study; gene names in red: reference genes commonly used in previous studies. #TUB2_1 and TUB2_2 refer to two different primer pairs for TUB2 (Wu et al., 2019; Gao et al., 2022). ^UBC21_1 and UBC21_2 refer to two different primer pairs for UBC21 (Cuéllar Pérez et al., 2014; Gao et al., 2022). *ACT1_1 and ACT1_2 refer to two different primer pairs for ACT1 (Kim and Hwang, 2014; Zhang et al., 2015).
Expression stability analyses of the candidate reference genes in various treatments
The above results suggest that the expressions of the candidate reference genes predicted in this study are generally more stable than those of the reference genes commonly used in previous studies. In addition to Pst DC3000 infection, we further analyzed the expression stabilities of the candidate reference genes in stress hormone treatments including JA, SA, and ABA. To rank the expression stability of the 18 candidate references genes under different treatments, programs including geNorm (Vandesompele et al., 2002), NormFinder (Andersen et al., 2004), BestKeeper (Pfaffl et al., 2004), and RefFinder (Xie et al., 2012) were used.
Stability analysis by geNorm
In geNorm analysis, all the 18 candidate reference genes had M values <1 in all the different treatments (Figure 6; Supplementary Table S2). The M values in Pst DC3000 infection are generally lower than those in other treatment (Supplementary Table S2). In Pst DC3000 infection, among the candidate genes, RPS6B and FKBP15-1 were the most stably expressed (M = 0.046) while PRPL17 was the least stably expressed (M = 0.35; Supplementary Table S2). For gene expression analyses using RT-qPCR, multiple reference genes are usually required for accurate expression normalization if the expression stability of the reference gene is low (Vandesompele et al., 2002). Among the 18 candidate reference genes, all the Vn/Vn + 1 values were much lower than the cut-off value of 0.15 (Figure 7). Such results suggest that using two reference genes would be good enough, eliminating the need of a third one, for expression normalization (Vandesompele et al., 2002).
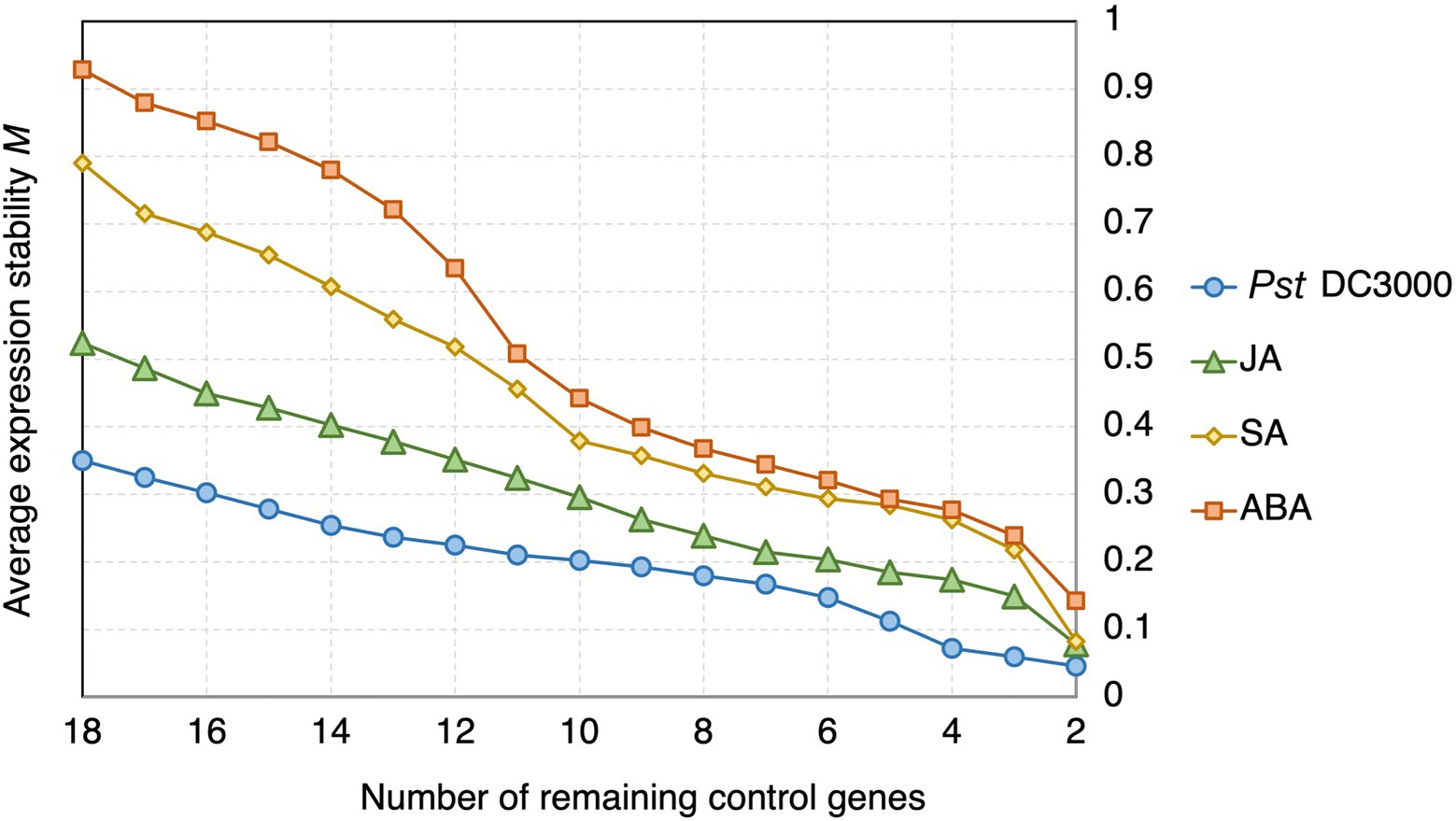
Figure 6. geNorm analysis of the average expression stability values of the candidate reference genes under Pst DC3000, JA, SA, and ABA treatments. Average expression stability values (M) of the remaining control genes were calculated by a stepwise exclusion of the least stable control gene among the reference gene candidates under each treatment using geNorm. The higher the M value, the lower is the ranking of the reference gene candidate in terms of expression stability.
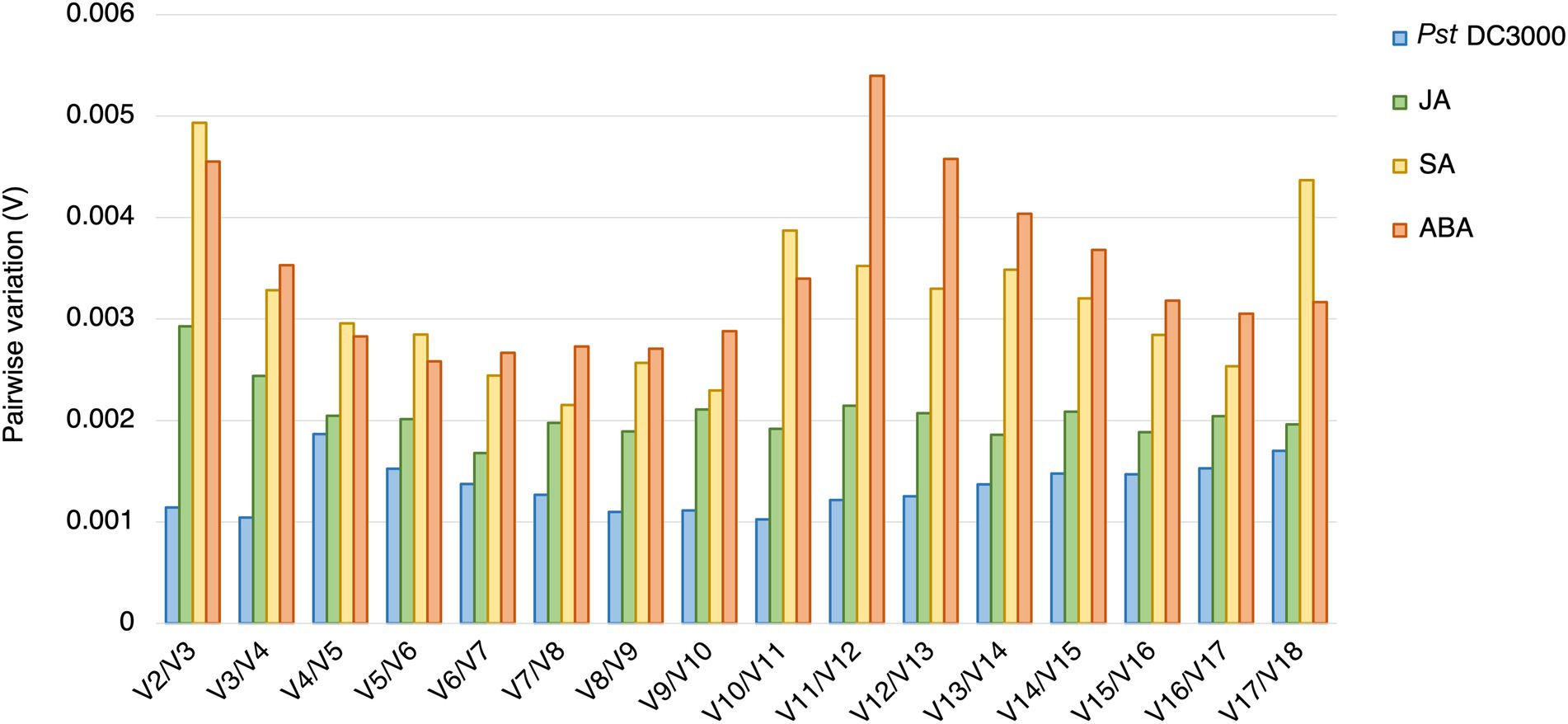
Figure 7. Pairwise variations (V) of the candidate reference genes. The pairwise variations (V) of the candidate reference genes calculated using geNorm, and V = Vn/Vn + 1, where n is the number of reference genes required for expression normalization in RT-qPCR. The threshold value of V for achieving the optimal number of reference genes for RT-qPCR normalization is 0.15. All the candidate genes had V values well below the threshold value under all the treatments, indicating a minimal number of reference genes from among these candidates is required for normalization in RT-qPCR.
Stability analysis by NormFinder
The expression stability values for each candidate reference gene was also analyzed using the linear mixed-effect model-based NormFinder, in which the variations between the input Ct values are considered in testing the gene expression stability (Andersen et al., 2004). Similar to geNorm, a higher stability value represents a lower expression stability of the reference gene candidate. The range of the stability values varied among treatments. It ranged between 0.041 and 0.528 under Pst DC3000 infection, between 0.088 and 0.765 with JA treatment, between 0.266 and 1.333 with SA treatment, and between 0.316 and 1.18 with ABA treatment (Supplementary Table S2). These results mean that the expression stability of the candidate reference genes varied under different treatments, but all had the highest stability upon Pst DC3000 infection.
Stability analysis by BestKeeper
The stability of the reference gene candidates was also tested using BestKeeper, in which the standard descriptive statistics for the genes are considered (Pfaffl et al., 2004). The BestKeeper algorithm suggests excluding those candidate reference genes with a standard deviation (SD) > 1.0 in Ct due to low expression stability (Pfaffl et al., 2004; Piehler et al., 2010). Among all the candidate reference genes for all the treatments, only FBA8 under SA treatment had an SD > 1 (SD = 1.125) in its Ct values while all the other genes had their SD < 1 for all the treatments (Supplementary Table S2). Similar to the results from NormFinder, results from BestKeeper suggest that the expression stability of the reference genes varies under different treatments, but with the highest stability upon Pst DC3000 infection in general. Nevertheless, the results strongly suggest the stability of all the candidate genes was acceptable as reference genes under all the treatment conditions tested.
Stability analysis by the comparative ΔCt method
We also used the comparative ΔCt method (Silver et al., 2006) to evaluate the relative stability of the candidate reference genes. The method compares the relative expression fluctuation of the two reference gene by measuring differences between their ΔCt values (Silver et al., 2006; Nagy et al., 2017). The average SD of the 18 candidate reference genes ranged from 0.26 to 0.55 for Pst DC3000 treatment, 0.39 to 0.82 for JA treatment, 0.62 to 1.39 for SA treatment and 0.73 to 1.33 for ABA treatment (Supplementary Table S2). Among all the candidates, TIF3D1 was the most stably expressed in both Pst DC3000 and SA treatments while RPS6B and FKBP15-1 were the most stable with JA and ABA treatments, respectively (Supplementary Table S2). Similar to the results from NormFinder and BestKeeper, the results in the comparative ΔCt method showed that the stability of the reference genes varied with treatments, but they all had the highest expression stability upon Pst DC3000 infection.
Stability analysis by RefFinder
To gain a comprehensive view of the stability ranking of the 18 candidate reference genes, the results from geNorm, NormFinder, BestKeeper, and the comparative ΔCt method were integrated using RefFinder (Xie et al., 2012; Supplementary Table S2). The results showed that the candidate reference genes had different degrees of expression stability under different treatments (Supplementary Table S2). Based on the comprehensive ranking, TIF3D1, RPS6B, and FKBP15-1 were the most stably expressed under Pst DC3000 treatment, RPS6B, PSBQ2, and FKBP15-1 most stably expressed with JA treatment, TIF3D1, CYFBP, and FBA6 most stably expressed under SA treatment, and PRPL17, PSBQ2, and FKBP15-1 most stably expressed with ABA treatment (Supplementary Table S2).
To see whether a single reference gene would be suitable for expression normalization for samples treated with different stresses, we estimated the geometric means of the rankings from RefFinder of each reference gene under different treatment combinations and suggested the most suitable candidate reference genes under these treatments (Table 1).
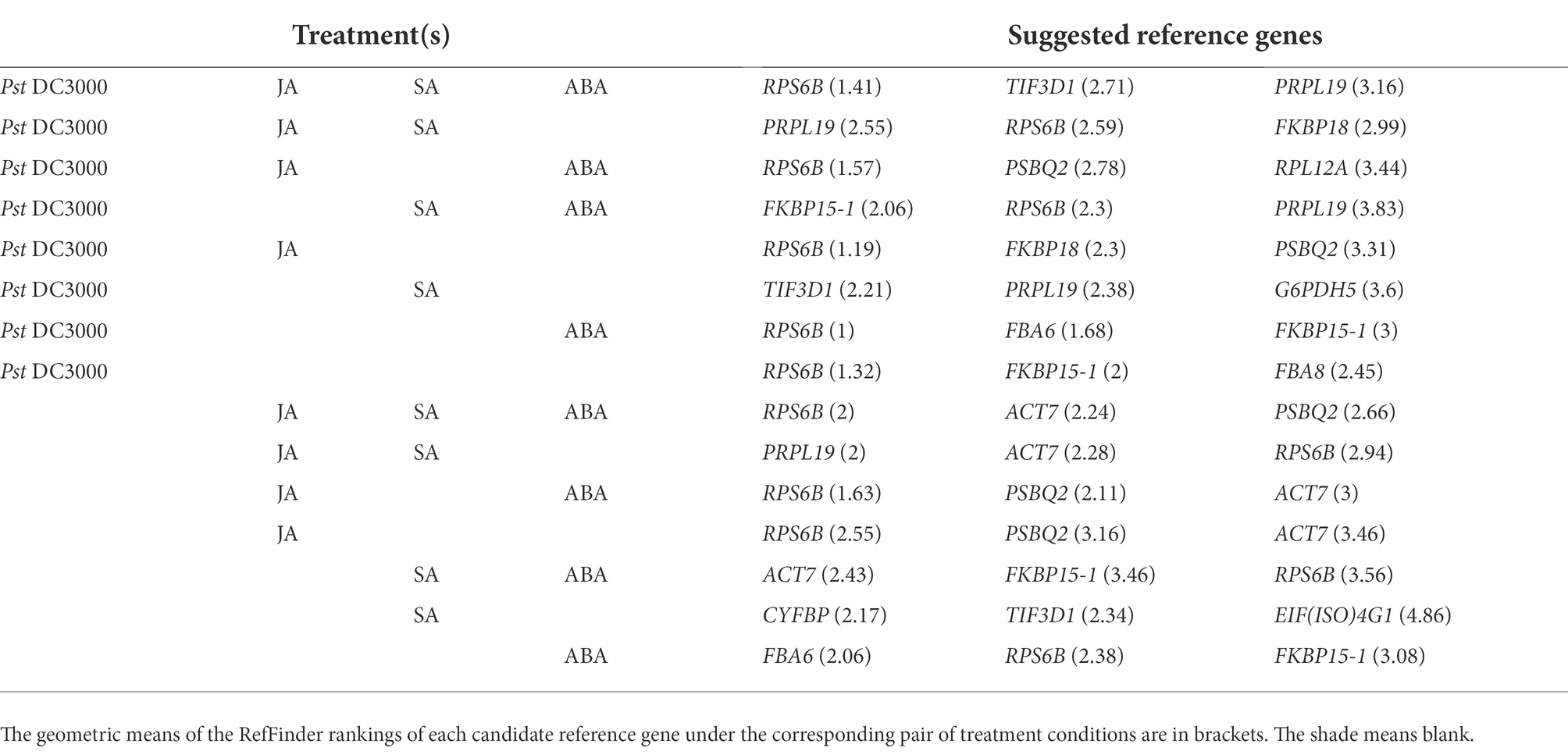
Table 1. Appropriate A. thaliana reference genes for expression normalization under different combinations of treatments, including Pseudomonaas syringae pv. tomato DC3000 (Pst DC3000), jasmonic acid (JA), salicylic acid (SA), and abscisic acid (ABA).
Discussion
In this study, we employed mass spectrometry-based LFQ and identified the A. thaliana proteins having stable abundances upon Pst DC3000 treatment. The candidate proteins fell into these categories: structural protein [ACT7 (Paez-Garcia et al., 2018)], basal metabolism-related proteins [BGAL6 (Dwevedi and Kayastha, 2010), CYFBP (Daie, 1993), FBA6 (Carrera et al., 2021), FBA8 (Lu et al., 2012), G6PDH5 (Sharkey and Weise, 2016), and PSBQ2 (Yi et al., 2006), protein-folding regulators HSP70-9 (Sung et al., 2001), FKBP15-1 and FKBP18 (Harrar et al., 2001)], and translation regulatory proteins [EIF(ISO)4G1 (Martínez-Silva et al., 2012), TIF3D1 (Raabe et al., 2019), RPS6B (Horiguchi et al., 2012), RPL12A, RPL17, RPL19, and RPL34C (Martinez-Seidel et al., 2020), and OVA6 (Berg et al., 2005)]. The results are consistent with the notion that genes involved in the maintenance of basal cellular functions tend to have stable expressions (Eisenberg and Levanon, 2013). It was therefore reasonable to expect the levels of the mRNAs encoding these proteins to also be relatively stable and that the study of expression stability upon pathogen infection could be applied to other treatments. The protein abundance and the mRNA level were found to be positively correlated (Supplementary Figure S6). In addition to the positive correlation, the slope of the line of best fit (Supplementary Figure S6) suggests that the mass spectrometry-based LFQ in proteomic analysis is more sensitive than RT-qPCR for expression quantitation. Such a high sensitivity of the mass spectrometry-based LFQ in proteomic analysis will enable accurate quantitation particularly if the experimental data fit the line of best fit well.
For the reference genes commonly used in previous studies, upon Pst DC3000 infection, the expression levels were generally lower than those of the candidate reference genes used in this study (Figure 2). The result is consistent with the lower abundance of the proteins compared to the proteins corresponding to the candidate reference genes reported in this study (Figure 1; Supplementary Figure S3). It is possible that proteins having higher abundances are more easily detectable by LC–MS/MS. In other words, the mass spectrometry-based proteomic analysis favors the detection of highly expressed proteins, which may imply the high levels of the corresponding mRNAs. The use of highly expressed genes for expression normalization in RT-qPCR facilitates the expression analysis when the input amount of cDNA or RNA is low.
The expression levels of the candidate reference genes were in a reasonably detectable range (Figure 2). The expression stability of the candidate reference genes was estimated using multiple programs including geNorm via the R-based package ctrlGene (ver. 1.0.0; Vandesompele et al., 2002; Zhong, 2019), Normfinder via RefFinder (Andersen et al., 2004; Xie et al., 2012), BestKeeper (Pfaffl et al., 2004) and the comparative ΔCt method (Silver et al., 2006). Since different programs employ different algorithms, they assigned different rankings to the same candidate reference genes (Supplementary Table S2). Similar observations were also reported in previous studies in which different reference genes were selected (Jia et al., 2019; Jin et al., 2019; Dudziak et al., 2020; Fu et al., 2022). To have a comprehensive view of expression stability, we employed RefFinder (Xie et al., 2012), in which an overall final ranking of the genes was generated based on the rankings in geNorm, Normfinder, BestKeeper, and the comparative ΔCt method.
The overall stability of the mRNA levels of the candidate reference genes was most closely reflected by the results from geNorm. The M values of the reference genes determined in previous studies using RNA-seq approaches ranged from 0.3 to 2.1 (Jin et al., 2019; Dudziak et al., 2020; Mao et al., 2021; Fu et al., 2022), compared to M values of 0.046–0.350 under Pst DC3000 infection, 0.078–0.524 with JA treatment, 0.082–0.790 with SA treatment, and 0.142–0.929 with ABA treatment in this study (Supplementary Table S2). As lower M values indicate higher expression stability, our results from this study showed that the candidate reference genes discovered here have a higher stability than the previously reported ones.
The results from NormFinder, BestKeeper, and the comparative ΔCt method all showed that these candidate reference genes had the highest expression stability upon Pst DC3000 infection compared to other treatments. This is to be expected, as these candidates were discovered based on the proteomic dataset upon Pst DC3000 infection. Although the stabilities of the candidate reference genes from other treatments were lower than those with Pst DC3000 infection, all the genes in all the treatments, except FBA8 with SA treatment, are regarded as suitable reference genes, according to the algorithm of BestKeeper.
Among the 18 candidate reference genes, only ACT7 (Czechowski et al., 2005) in A. thaliana and OVA6 in potato (Castro-Quezada et al., 2013) were previously reported to be suitable for being used as reference genes for RT-qPCR in multiple-treatment experiments. Based on the overall final ranking generated using RefFinder, ACT7 was out-performed by TIF3D1, RPS6B, FKBP15-1, and FBA8 in Pst DC3000 infection, by RPS6B, PSBQ2, and FKBP15-1 in JA treatment, by all candidate reference genes except RPS6B, OVA6, FKBP15-1, HSP70-9, and FBA8 in SA treatment, and by PRPL17, PSBQ2, and FKBP15-1 in ABA treatment (Supplementary Table S2). In previous studies on A. thaliana-Pst DC3000 interaction, depending on the stress responses, the plant samples may be harvested from 1 to 5 dpi (Mackey et al., 2003; Chow and Ng, 2017; Zhang et al., 2017; Cheng et al., 2022). Although the current study only addressed the expression stability of the candidate reference genes between 0 dpi and 3 dpi, the general expression stability of the genes upon various treatments may suggest the potential of these candidate reference genes for expression normalization at different time points after Pst DC3000 infection. For A. thaliana, Pst DC3000 has been commonly employed as the model bacterial pathogen (Xin and He, 2013). Besides being used as the model for studying plant-bacterium interaction, A. thaliana is also commonly used as the model for other plant-pathogen interactions. For example, A. thaliana-Hyaloperonospora arabidopsidis, A. thaliana-Alternaria brassicicola conidia, and A. thaliana-Cucumber mosaic virus have been employed as the models for plant-oomycete interaction, plant-fungus interaction, and plant-virus interactions, respectively, (Coates and Beynon, 2010; Pochon et al., 2012; Montes et al., 2019). Although the expression stability of the candidate reference genes was not tested upon the infection by the other pathogens, the expression stability of these candidate reference genes upon the treatment of various stress hormones was demonstrated. Since JA, SA, and ABA are major hormones regulating plant-pathogen interactions (Ku et al., 2018), the results may suggest the potential of these candidate reference genes for expression normalization upon the infection by other pathogens.
In conclusion, the results in this study suggest that mass spectrometry-based LFQ in proteomic analysis is an effective approach for mining proteins and their corresponding mRNAs with stable expression levels under different conditions. Compared to RNA-seq, mass spectrometry-based LFQ does not involve amplification steps which have been known to create biases that affect the accuracy of quantification. In addition, genes involved in the maintenance of basic cellular functions generally have stable expression levels. These factors enable the identification of elite reference genes from the proteomic dataset under different experimental treatments. The expression stability of the candidate reference genes in various treatments may suggest the potential of the genes to be employed as the reference genes in treatment conditions yet to be covered in the current study. The homologs of the candidate reference genes in other plant species may also be the potential candidates of reference genes for expression studies.
Data availability statement
The data presented in the study are deposited in PRIDE (PRoteomics IDEntifications Database, accession number PXD035677).
Author contributions
S-SC, Y-SK, and M-YC conducted the experiments and analyzed the data. S-SC and Y-SK drafted the manuscript. Y-SK and H-ML finalized the manuscript. H-ML acquired the funding. All authors contributed to the article and approved the submitted version.
Funding
This work was supported by the Hong Kong Research Grants Council: General Research Fund (14164617).
Acknowledgments
Jee-Yan Chu copy-edited this manuscript. Any opinions, findings, conclusions, or recommendations expressed in this publication do not reflect the views of the Government of the Hong Kong Special Administrative Region or the Innovation and Technology Commission.
Conflict of interest
The authors declare that the research was conducted in the absence of any commercial or financial relationships that could be construed as a potential conflict of interest.
Publisher’s note
All claims expressed in this article are solely those of the authors and do not necessarily represent those of their affiliated organizations, or those of the publisher, the editors and the reviewers. Any product that may be evaluated in this article, or claim that may be made by its manufacturer, is not guaranteed or endorsed by the publisher.
Supplementary material
The Supplementary material for this article can be found online at: https://www.frontiersin.org/articles/10.3389/fpls.2022.1001920/full#supplementary-material
References
Andersen, C. L., Jensen, J. L., and Ørntoft, T. F. (2004). Normalization of real-time quantitative reverse transcription-PCR data: a model-based variance estimation approach to identify genes suited for normalization, applied to bladder and colon cancer data sets. Cancer Res. 64, 5245–5250. doi: 10.1158/0008-5472.CAN-04-0496
Arefian, M., Vessal, S., Malekzadeh-Shafaroudi, S., Siddique, K. H. M., and Bagheri, A. (2019). Comparative proteomics and gene expression analyses revealed responsive proteins and mechanisms for salt tolerance in chickpea genotypes. BMC Plant Biol. 19:300. doi: 10.1186/s12870-019-1793-z
Benjamini, Y., and Speed, T. P. (2012). Summarizing and correcting the GC content bias in high-throughput sequencing. Nucleic Acids Res. 40:e72. doi: 10.1093/nar/gks001
Berg, M., Rogers, R., Muralla, R., and Meinke, D. (2005). Requirement of aminoacyl-tRNA synthetases for gametogenesis and embryo development in Arabidopsis. Plant J. 44, 866–878. doi: 10.1111/j.1365-313X.2005.02580.x
Carmona, R., Arroyo, M., Jiménez-Quesada, M. J., Seoane, P., Zafra, A., Larrosa, R., et al. (2017). Automated identification of reference genes based on RNA-seq data. Biomed. Eng. Online 16:65. doi: 10.1186/s12938-017-0356-5
Carrera, D. Á., George, G. M., Fischer-Stettler, M., Galbier, F., Eicke, S., Truernit, E., et al. (2021). Distinct plastid fructose bisphosphate aldolases function in photosynthetic and non-photosynthetic metabolism in Arabidopsis. J. Exp. Bot. 72, 3739–3755. doi: 10.1093/jxb/erab099
Castro-Quezada, P., Aarrouf, J., Claverie, M., Favery, B., Mugniéry, D., Lefebvre, V., et al. (2013). Identification of reference genes for normalizing RNA expression in potato roots infected with cyst nematodes. Plant Mol. Biol. Rep. 31, 936–945. doi: 10.1007/s11105-013-0566-3
Chen, C. C., Chien, W. F., Lin, N. C., and Yeh, K. C. (2014). Alternative functions of Arabidopsis YELLOW STRIPELIKE3: from metal translocation to pathogen defense. PLoS One 9:e98008. doi: 10.1371/journal.pone.0098008
Cheng, S.-S., Ku, Y.-S., Cheung, M.-Y., and Lam, H.-M. (2022). AtGAP1 promotes the resistance to pseudomonas syringae pv. Tomato DC3000 by regulating cell-wall thickness and stomatal aperture in Arabidopsis. Int. J. Mol. Sci. 23:7540. doi: 10.3390/ijms23147540
Cheong, M. S., Kirik, A., Kim, J. G., Frame, K., Kirik, V., and Mudgett, M. B. (2014). AvrBsT acetylates Arabidopsis ACIP1, a protein that associates with microtubules and is required for immunity. PLoS Pathog. 10:e1003952. doi: 10.1371/journal.ppat.1003952
Cheung, M. Y., Li, M. W., Yung, Y. L., Wen, C. Q., and Lam, H. M. (2013). The unconventional P-loop NTPase OsYchF1 and its regulator OsGAP1 play opposite roles in salinity stress tolerance. Plant Cell Environ. 36, 2008–2020. doi: 10.1111/pce.12108
Chow, H. T., and Ng, D. W.-K. (2017). Regulation of miR163 and its targets in defense against Pseudomonas syringae in Arabidopsis thaliana. Sci. Rep. 7:46433. doi: 10.1038/srep46433
Coates, M. E., and Beynon, J. L. (2010). Hyaloperonospora arabidopsidis as a pathogen model. Annu. Rev. Phytopathol. 48, 329–345. doi: 10.1146/annurev-phyto-080508-094422
Corchete, L. A., Rojas, E. A., Alonso-López, D., De Las Rivas, J., Gutiérrez, N. C., and Burguillo, F. J. (2020). Systematic comparison and assessment of RNA-seq procedures for gene expression quantitative analysis. Sci. Rep. 10:19737. doi: 10.1038/s41598-020-76881-x
Cuéllar Pérez, A., Nagels Durand, A., Vanden Bossche, R., De Clercq, R., Persiau, G., Van Wees, S. C. M., et al. (2014). The non-JAZ TIFY protein TIFY8 from Arabidopsis thaliana is a transcriptional repressor. PLoS One 9:e84891. doi: 10.1371/journal.pone.0084891
Cui, B., Xu, S., Li, Y., Umbreen, S., Frederickson, D., Yuan, B., et al. (2021). The Arabidopsis zinc finger proteins SRG2 and SRG3 are positive regulators of plant immunity and are differentially regulated by nitric oxide. New Phytol. 230, 259–274. doi: 10.1111/nph.16993
Czechowski, T., Stitt, M., Altmann, T., Udvardi, M. K., and Scheible, W.-R. (2005). Genome-wide identification and teseting of superior refernece genes for transcript normalization in Arabidopsis. Plant Physiol. 139, 5–17. doi: 10.1104/pp.105.063743
Daie, J. (1993). Cytosolic fructose-1,6-bisphosphatase: a key enzyme in the sucrose biosynthetic pathway. Photosynth. Res. 38, 5–14. doi: 10.1007/BF00015056
Ding, H., Mo, S., Qian, Y., Yuan, G., Wu, X., and Ge, C. (2020). Integrated proteome and transcriptome analyses revealed key factors involved in tomato (Solanum lycopersicum) under high temperature stress. Food Energy Secur. 9:e239. doi: 10.1002/fes3.239
Dodds, P. N., and Rathjen, J. P. (2010). Plant immunity: towards an integrated view of plant-pathogen interactions. Nat. Rev. Genet. 11, 539–548. doi: 10.1038/nrg2812
Dudziak, K., Sozoniuk, M., Szczerba, H., Kuzdraliński, A., Kowalczyk, K., Börner, A., et al. (2020). Identification of stable reference genes for qPCR studies in common wheat (Triticum aestivum L.) seedlings under short-term drought stress. Plant Methods 16:58. doi: 10.1186/s13007-020-00601-9
Dwevedi, A., and Kayastha, A. M. (2010). Plant β-galactosidases: physiological significance and recent advances in technological applications. J. Plant Biochem. Biotechnol. 19, 9–20. doi: 10.1007/bf03323431
Eisenberg, E., and Levanon, E. Y. (2013). Human housekeeping genes, revisited. Trends Genet. 29, 569–574. doi: 10.1016/j.tig.2013.05.010
Fu, N., Li, J., Wang, M., Ren, L., Zong, S., and Luo, Y. (2022). Identification and validation of reference genes for gene expression analysis in different development stages of Amylostereum areolatum. Front. Microbiol. 12, 1–9. doi: 10.3389/fmicb.2021.827241
Gao, Y., Li, Z., Yang, C., Li, G., Zeng, H., Li, Z., et al. (2022). Pseudomonas syringae activates ZAT18 to inhibit salicylic acid accumulation by repressing EDS1 transcription for bacterial infection. New Phytol. 233, 1274–1288. doi: 10.1111/nph.17870
Gökmen-Polar, Y. (2019). “Overview of PCR-based technologies and multiplexed gene analysis for biomarker studies,” in Predictive Biomarkers in Oncology. eds. S. Badve and G. L. Kumar (Cham: Springer Nature Switzerland AG), 63–73. doi: 10.1007/978-3-319-95228-4
Gorshkov, V., and Tsers, I. (2022). Plant susceptible responses: the underestimated side of plant–pathogen interactions. Biol. Rev. 97, 45–66. doi: 10.1111/brv.12789
Gupta, A., and Senthil-Kumar, M. (2017). Transcriptome changes in Arabidopsis thaliana infected with Pseudomonas syringae during drought recovery. Sci. Rep. 7:9124. doi: 10.1038/s41598-017-09135-y
Harrar, Y., Bellini, C., and Faure, J.-D. (2001). FKBPs: at the crossroads of folding and transduction. Trends Plant Sci. 6, 426–431. doi: 10.1016/s1360-1385(01)02044-1
Horiguchi, G., Van Lijsebettens, M., Candela, H., Micol, J. L., and Tsukaya, H. (2012). Ribosomes and translation in plant developmental control. Plant Sci. 191-192, 24–34. doi: 10.1016/j.plantsci.2012.04.008
Huang, T. Y., Desclos-Theveniau, M., Chien, C. T., and Zimmerli, L. (2013). Arabidopsis thaliana transgenics overexpressing IBR3 show enhanced susceptibility to the bacterium Pseudomonas syringae. Plant Biol. J. 15, 832–840. doi: 10.1111/j.1438-8677.2012.00685.x
Huot, B., Castroverde, C. D. M., Velásquez, A. C., Hubbard, E., Pulman, J. A., Yao, J., et al. (2017). Dual impact of elevated temperature on plant defence and bacterial virulence in Arabidopsis. Nat. Commun. 8, 1808–1811. doi: 10.1038/s41467-017-01674-2
Jeong, H. J., Kim, Y. J., Kim, S. H., Kim, Y. H., Lee, I. J., Kim, Y. K., et al. (2011). Nonsense-mediated mRNA decay factors, UPF1 and UPF3, contribute to plant defense. Plant Cell Physiol. 52, 2147–2156. doi: 10.1093/pcp/pcr144
Jia, D. H., Wang, B., Li, X. L., Tan, W., Gan, B. C., and Peng, W. H. (2019). Validation of reference genes for quantitative gene expression analysis in Auricularia cornea. J. Microbiol. Methods 163:105658. doi: 10.1016/j.mimet.2019.105658
Jia, X., Zeng, H., Wang, W., Zhang, F., and Yin, H. (2018). Chitosan oligosaccharide induces resistance to Pseudomonas syringae pv. tomato DC3000 in Arabidopsis thaliana by activating both salicylic acid–and jasmonic acid–mediated pathways. Mol. Plant Microbe Interact. 31, 1271–1279. doi: 10.1094/MPMI-03-18-0071-R
Jin, Y., Liu, F., Huang, W., Sun, Q., and Huang, X. (2019). Identification of reliable reference genes for qRT-PCR in the ephemeral plant Arabidopsis pumila based on full-length transcriptome data. Sci. Rep. 9, 8408–8411. doi: 10.1038/s41598-019-44849-1
Kim, D. S., and Hwang, B. K. (2014). An important role of the pepper phenylalanine ammonia-lyase gene (PAL1) in salicylic acid-dependent signalling of the defence response to microbial pathogens. J. Exp. Bot. 65, 2295–2306. doi: 10.1093/jxb/eru109
Ku, Y.-S., Cheng, S.-S., Gerhardt, A., Cheung, M.-Y., Contador, C. A., Poon, L.-Y. W., et al. (2020). Secretory peptides as bullets: effector peptides from pathogens against antimicrobial peptides from soybean. Int. J. Mol. Sci. 21, 1–26. doi: 10.3390/ijms21239294
Ku, Y.-S., Sintaha, M., Cheung, M.-Y., and Lam, H.-M. (2018). Plant hormone signaling crosstalks between biotic and abiotic stress responses. Int. J. Mol. Sci. 19:3206. doi: 10.3390/ijms19103206
Kudo, T., Sasaki, Y., Terashima, S., Matsuda-Imai, N., Takano, T., Saito, M., et al. (2016). Identification of reference genes for quantitative expression analysis using large-scale RNA-seq data of Arabidopsis thaliana and model crop plants. Genes Genet. Syst. 91, 111–125. doi: 10.1266/ggs.15-00065
Li, B., Meng, X., Shan, L., and He, P. (2016a). Transcriptional regulation of pattern-triggered immunity in plants. Cell Host Microbe 19, 641–650. doi: 10.1016/j.chom.2016.04.011
Li, L., Yu, Y., Zhou, Z., and Zhou, J. M. (2016b). Plant pattern-recognition receptors controlling innate immunity. Sci. China Life Sci. 59, 878–888. doi: 10.1007/s11427-016-0115-2
Lu, W., Tang, X., Huo, Y., Xu, R., Qi, S., Huang, J., et al. (2012). Identification and characterization of fructose 1,6-bisphosphate aldolase genes in Arabidopsis reveal a gene family with diverse responses to abiotic stresses. Gene 503, 65–74. doi: 10.1016/j.gene.2012.04.042
Mackey, D., Belkhadir, Y., Alonso, J. M., Ecker, J. R., and Dangl, J. L. (2003). Arabidopsis RIN4 is a target of the type III virulence effector AvrRpt2 and modulates RPS2-mediated resistance. Cell 112, 379–389. doi: 10.1016/S0092-8674(03)00040-0
Mao, M., Xue, Y., He, Y., Zhou, X., Hu, H., Liu, J., et al. (2021). Validation of reference genes for quantitative real-time PCR normalization in Ananas comosus var. bracteatus during chimeric leaf development and response to hormone stimuli. Front. Genet. 12:716137. doi: 10.3389/fgene.2021.716137
Martin, K., Singh, J., Hill, J. H., Whitham, S. A., and Cannon, S. B. (2016). Dynamic transcriptome profiling of bean common mosaic virus (BCMV) infection in common bean (Phaseolus vulgaris L.). BMC Genom. 17:613. doi: 10.1186/s12864-016-2976-8
Martinez-Seidel, F., Beine-Golovchuk, O., Hsieh, Y. C., and Kopka, J. (2020). Systematic review of plant ribosome heterogeneity and specialization. Front. Plant Sci. 11:948. doi: 10.3389/fpls.2020.00948
Martínez-Silva, A. V., Aguirre-Martínez, C., Flores-Tinoco, C. E., Alejandri-Ramírez, N. D., and Dinkova, T. D. (2012). Translation initiation factor AteiF(iso)4E is involved in selective mRNA translation in Arabidopsis thaliana seedlings. PLoS One 7:e31606. doi: 10.1371/journal.pone.0031606
Marx, H., Minogue, C. E., Jayaraman, D., Richards, A. L., Kwiecien, N. W., Siahpirani, A. F., et al. (2016). A proteomic atlas of the legume Medicago truncatula and its nitrogen-fixing endosymbiont Sinorhizobium meliloti. Nat. Biotechnol. 34, 1198–1205. doi: 10.1038/nbt.3681
Matic, S., Bagnaresi, P., Biselli, C., Orru, L., Carneiro, G. A., Siciliano, I., et al. (2016). Comparative transcriptome profiling of resistant and susceptible rice genotypes in response to the seedborne pathogen Fusarium fujikuroi. BMC Genom. 17:608. doi: 10.1186/s12864-016-2925-6
Medzhitov, R., and Janeway, C. A. (1997). Innate immunity: the virtues of a nonclonal system of recognition. Cell 91, 295–298. doi: 10.1016/S0092-8674(00)80412-2
Mi, H., Muruganujan, A., Casagrande, J. T., and Thomas, P. D. (2013). Large-scale gene function analysis with the panther classification system. Nat. Protoc. 8, 1551–1566. doi: 10.1038/nprot.2013.092
Montes, N., Alonso-Blanco, C., and García-Arenal, F. (2019). Cucumber mosaic virus infection as a potential selective pressure on Arabidopsis thaliana populations. PLoS Pathog. 15:e1007810. doi: 10.1371/journal.ppat.1007810
Nagy, N. A., Németh, Z., Juhász, E., Póliska, S., Rácz, R., Kosztolányi, A., et al. (2017). Evaluation of potential reference genes for real-time qPCR analysis in a biparental beetle, Lethrus apterus (Coleoptera: Geotrupidae). PeerJ. 5, 1–16. doi: 10.7717/peerj.4047
Nakaminami, K., Matsui, A., Nakagami, H., Minami, A., Nomura, Y., Tanaka, M., et al. (2014). Analysis of differential expression patterns of mRNA and protein during cold-acclimation and de-acclimation in Arabidopsis. Mol. Cell. Proteomics 13, 3602–3611. doi: 10.1074/mcp.M114.039081
Paez-Garcia, A., Sparks, J. A., de Bang, L., and Blancaflor, E. B. (2018). “Plant actin cytoskeleton: new functions from old scaffold,” in Concepts in Cell Biology - History and Evolution. eds. V. P. Sahi and F. Baluška (London, UK: Springer Nature), 103–137.
Parekh, S., Ziegenhain, C., Vieth, B., Enard, W., and Hellmann, I. (2016). The impact of amplification on differential expression analyses by RNA-seq. Sci. Rep. 6:25533. doi: 10.1038/srep25533
Pfaffl, M. W., Tichopad, A., Prgomet, C., and Neuvians, T. P. (2004). Determination of stable housekeeping genes, differentially regulated target genes and sample integrity: BestKeeper - excel-based tool using pair-wise correlations. Biotechnol. Lett. 26, 509–515. doi: 10.1023/B:BILE.0000019559.84305.47
Piehler, A. P., Grimholt, R. M., Øvstebø, R., and Berg, J. P. (2010). Gene expression results in lipopolysaccharide-stimulated monocytes depend significantly on the choice of reference genes. BMC Immunol. 11:21. doi: 10.1186/1471-2172-11-21
Pochon, S., Terrasson, E., Guillemette, T., Iacomi-Vasilescu, B., Georgeault, S., Juchaux, M., et al. (2012). The Arabidopsis thaliana-Alternaria brassicicola pathosystem: a model interaction for investigating seed transmission of necrotrophic fungi. Plant Methods 8:16. doi: 10.1186/1746-4811-8-16
Pombo, M. A., Ramos, R. N., Zheng, Y., Fei, Z., Martin, G. B., and Rosli, H. G. (2019). Transcriptome-based identification and validation of reference genes for plant-bacteria interaction studies using Nicotiana benthamiana. Sci. Rep. 9:1632. doi: 10.1038/s41598-018-38247-2
Ponnala, L., Wang, Y., Sun, Q., and van Wijk, K. J. (2014). Correlation of mRNA and protein abundance in the developing maize leaf. Plant J. 78, 424–440. doi: 10.1111/tpj.12482
Poretti, M., Sotiropoulos, A. G., Graf, J., Jung, E., Bourras, S., Krattinger, S. G., et al. (2021). Comparative transcriptome analysis of wheat lines in the field reveals multiple essential biochemical pathways suppressed by obligate pathogens. Front. Plant Sci. 12:720462. doi: 10.3389/fpls.2021.720462
Raabe, K., Honys, D., and Michailidis, C. (2019). The role of eukaryotic initiation factor 3 in plant translation regulation. Plant Physiol. Biochem. 145, 75–83. doi: 10.1016/j.plaphy.2019.10.015
Ren, H., Li, X., Guo, L., Wang, L., Hao, X., and Zeng, J. (2022). Integrative transcriptome and proteome analysis reveals the absorption and metabolism of selenium in tea alants [Camellia sinensis (L.) O. Kuntze]. Front. Plant Sci. 13:848349. doi: 10.3389/fpls.2022.848349
Romero-Pérez, A., Ameye, M., Audenaert, K., and Van Damme, E. J. M. (2021). Overexpression of F-box Nictaba promotes defense and anthocyanin accumulation in Arabidopsis thaliana after Pseudomonas syringae infection. Front. Plant Sci. 12:692606. doi: 10.3389/fpls.2021.692606
Sakurai, T., Kondou, Y., Akiyama, K., Kurotani, A., Higuchi, M., Ichikawa, T., et al. (2011). RiceFOX: a database of Arabidopsis mutant lines overexpressing rice full-length cDNA that contains a wide range of trait information to facilitate analysis of gene function. Plant Cell Physiol. 52, 265–273. doi: 10.1093/pcp/pcq190
Sharkey, T. D., and Weise, S. E. (2016). The glucose 6-phosphate shunt around the Calvin-Benson cycle. J. Exp. Bot. 67, 4067–4077. doi: 10.1093/jxb/erv484
Shu, J., Ma, X., Ma, H., Huang, Q., Zhang, Y., Guan, M., et al. (2022). Transcriptomic, proteomic, metabolomic, and functional genomic approaches of Brassica napus L. during salt stress. PLoS One 17:e0262587. doi: 10.1371/journal.pone.0262587
Silver, N., Best, S., Jiang, J., and Thein, S. L. (2006). Selection of housekeeping genes for gene expression studies in human reticulocytes using real-time PCR. BMC Mol. Biol. 7:33. doi: 10.1186/1471-2199-7-33
Sugano, S., Hayashi, N., Kawagoe, Y., Mochizuki, S., Inoue, H., Mori, M., et al. (2016). Rice OsVAMP714, a membrane-trafficking protein localized to the chloroplast and vacuolar membrane, is involved in resistance to rice blast disease. Plant Mol. Biol. 91, 81–95. doi: 10.1007/s11103-016-0444-0
Sung, D. Y., Kaplan, F., and Guy, C. L. (2001). Plant Hsp70 molecular chaperones: protein structure, gene family, expression and function. Physiol. Plant. 113, 443–451. doi: 10.1034/j.1399-3054.2001.1130402.x
Vandesompele, J., De Preter, K., Pattyn, F., Poppe, B., Van Roy, N., De Paepe, A., et al. (2002). Accurate normalization of real-time quantitative RT-PCR data by geometric averaging of multiple internal control genes. Genome Biol. 3:research0034. doi: 10.1186/gb-2002-3-7-research0034
Walker, C. G., Meier, S., Mitchell, M. D., Roche, J. R., and Littlejohn, M. (2009). Evaluation of real-time PCR endogenous control genes for analysis of gene expression in bovine endometrium. BMC Mol. Biol. 10:100. doi: 10.1186/1471-2199-10-100
Wang, J., Islam, F., Li, L., Long, M., Yang, C., Jin, X., et al. (2017). Complementary RNA-sequencing based transcriptomics and iTRAQ proteomics reveal the mechanism of the alleviation of quinclorac stress by salicylic acid in Oryza sativa ssp. japonica. Int. J. Mol. Sci. 18:1975. doi: 10.3390/ijms18091975
Wessel, D., and Flügge, U. I. (1984). A method for the quantitative recovery of protein in dilute solution in the presence of detergents and lipids. Anal. Biochem. 138, 141–143. doi: 10.1016/0003-2697(84)90782-6
Wu, Z., Han, S., Zhou, H., Tuang, Z. K., Wang, Y., Jin, Y., et al. (2019). Cold stress activates disease resistance in Arabidopsis thaliana through a salicylic acid dependent pathway. Plant Cell Environ. 42, 2645–2663. doi: 10.1111/pce.13579
Xie, F., Xiao, P., Chen, D., Xu, L., and Zhang, B. (2012). miRDeepFinder: a miRNA analysis tool for deep sequencing of plant small RNAs. Plant Mol. Biol. 80, 75–84. doi: 10.1007/s11103-012-9885-2
Xin, X. F., and He, S. Y. (2013). Pseudomonas syringae pv. tomato DC3000: a model pathogen for probing disease susceptibility and hormone signaling in plants. Annu. Rev. Phytopathol. 51, 473–498. doi: 10.1146/annurev-phyto-082712-102321
Yi, X., Hargett, S. R., Frankel, L. K., and Bricker, T. M. (2006). The PsbQ protein is required in Arabidopsis for photosystem II assembly/stability and photoautotrophy under low light conditions. J. Biol. Chem. 281, 26260–26267. doi: 10.1074/jbc.M603582200
Yim, A. K.-Y., Wong, J. W.-H., Ku, Y.-S., Qin, H., Chan, T.-F., and Lam, H.-M. (2015). Using RNA-seq data to evaluate reference genes suitable for gene expression studies in soybean. PLoS One 10:e0136343. doi: 10.1371/journal.pone.0136343
Zhang, H., Huang, L., Dai, Y., Liu, S., Hong, Y., Tian, L., et al. (2015). Arabidopsis AtERF15 positively regulates immunity against Pseudomonas syringae pv. tomato DC3000 and Botrytis cinerea. Front. Plant Sci. 6:686. doi: 10.3389/fpls.2015.00686
Zhang, R., Qi, H., Sun, Y., Xiao, S., and Lim, B. L. (2017). Transgenic Arabidopsis thaliana containing increased levels of ATP and sucrose is more susceptible to Pseudomonas syringae. PLoS One 12:e0171040. doi: 10.1371/journal.pone.0171040
Zhang, J., and Zhou, J. M. (2010). Plant immunity triggered by microbial molecular signatures. Mol. Plant 3, 783–793. doi: 10.1093/mp/ssq035
Zhong, S. (2019). ctrlGene: assess the stability of candidate housekeeping genes. Available at: https://cran.r-project.org/web/packages/ctrlGene/ (Accessed June 1, 2022).
Zhou, Z., Cong, P., Tian, Y., and Zhu, Y. (2017). Using RNA-seq data to select reference genes for normalizing gene expression in apple roots. PLoS One 12:e0185288. doi: 10.1371/journal.pone.0185288
Zhu, Y., Qiu, W., He, X., Wu, L., Bi, D., Deng, Z., et al. (2022). Integrative analysis of transcriptome and proteome provides insights into adaptation to cadmium stress in Sedum plumbizincicola. Ecotoxicol. Environ. Saf. 230:113149. doi: 10.1016/j.ecoenv.2021.113149
Keywords: pathogen infection, jasmonic acid, salicylic acid, abscisic acid, expression study, label-free quantification, RT-qPCR, reference gene
Citation: Cheng S-S, Ku Y-S, Cheung M-Y and Lam H-M (2022) Identification of stably expressed reference genes for expression studies in Arabidopsis thaliana using mass spectrometry-based label-free quantification. Front. Plant Sci. 13:1001920. doi: 10.3389/fpls.2022.1001920
Edited by:
Pramod Prasad, ICAR-Indian Institute of Wheat and Barley Research, Regional Station, IndiaReviewed by:
Rupam Kumar Bhunia, National Agri-Food Biotechnology Institute, IndiaJay Jayaraman, The New Zealand Institute for Plant and Food Research Ltd., New Zealand
Copyright © 2022 Cheng, Ku, Cheung and Lam. This is an open-access article distributed under the terms of the Creative Commons Attribution License (CC BY). The use, distribution or reproduction in other forums is permitted, provided the original author(s) and the copyright owner(s) are credited and that the original publication in this journal is cited, in accordance with accepted academic practice. No use, distribution or reproduction is permitted which does not comply with these terms.
*Correspondence: Yee-Shan Ku, ysamyku@cuhk.edu.hk; Hon-Ming Lam, honming@cuhk.edu.hk