- 1Department of Agronomy, Iowa State University, Ames, IA, United States
- 2Department of Mechanical Engineering, Iowa State University, Ames, IA, United States
- 3Department of Statistics, Iowa State University, Ames, IA, United States
- 4USDA—Agricultural Research Service, Corn Insects and Crop Genetics Research Unit, Ames, IA, United States
Mung bean [Vigna radiata (L.) Wilczek] is a drought-tolerant, short-duration crop, and a rich source of protein and other valuable minerals, vitamins, and antioxidants. The main objectives of this research were (1) to study the root traits related with the phenotypic and genetic diversity of 375 mung bean genotypes of the Iowa (IA) diversity panel and (2) to conduct genome-wide association studies of root-related traits using the Automated Root Image Analysis (ARIA) software. We collected over 9,000 digital images at three-time points (days 12, 15, and 18 after germination). A broad sense heritability for days 15 (0.22–0.73) and 18 (0.23–0.87) was higher than that for day 12 (0.24–0.51). We also reported root ideotype classification, i.e., PI425425 (India), PI425045 (Philippines), PI425551 (Korea), PI264686 (Philippines), and PI425085 (Sri Lanka) that emerged as the top five in the topsoil foraging category, while PI425594 (unknown origin), PI425599 (Thailand), PI425610 (Afghanistan), PI425485 (India), and AVMU0201 (Taiwan) were top five in the drought-tolerant and nutrient uptake “steep, cheap, and deep” ideotype. We identified promising genotypes that can help diversify the gene pool of mung bean breeding stocks and will be useful for further field testing. Using association studies, we identified markers showing significant associations with the lateral root angle (LRA) on chromosomes 2, 6, 7, and 11, length distribution (LED) on chromosome 8, and total root length-growth rate (TRL_GR), volume (VOL), and total dry weight (TDW) on chromosomes 3 and 5. We discussed genes that are potential candidates from these regions. We reported beta-galactosidase 3 associated with the LRA, which has previously been implicated in the adventitious root development via transcriptomic studies in mung bean. Results from this work on the phenotypic characterization, root-based ideotype categories, and significant molecular markers associated with important traits will be useful for the marker-assisted selection and mung bean improvement through breeding.
Introduction
There is an increasing demand, particularly in Western cultures, for plant-based protein sources, including analogs of meat, egg, and dairy (Wild et al., 2014; Joshi and Kumar, 2016; Niva et al., 2017; Aschemann-Witzel et al., 2020). Numerous factors influence this change, including social, political, environmental, ethical, health-focused, technological, and economical (Vinnari, 2008; Markiewicz, 2010). Pulses such as lentils (Lens culinaris), horse beans (Dolichos spp.), lupins (Lupinus albus L.), common beans (Phaseolus vulgaris), chickpea (Cicer arietinum), field peas (Pisum sativum), cowpeas (Vigna unguiculata), fava beans (Vicia faba), mung bean [Vigna radiata (L.) Wilczek], urd beans (Vigna mungo), and food-grade soybeans (Glycine max) have consistently been used as protein sources in the global south (Niva et al., 2017). The plant protein demand has been fueled by the sustainable production of pulses coupled with their health benefits and the production of meat analogs (Wild et al., 2014; Niva et al., 2017). Residents of sub-Saharan Africa, the Caribbeans, and South America consume more than 10 kg/capita/year of pulses, compared to 3 kg/capita/year among Western cultures (Akibode and Maredia, 2012).
Mung bean (V. radiata L. Wilczek), initially domesticated in India, is now cultivated in over 7 million hectares worldwide (Nair et al., 2019; Aski et al., 2021). Mung bean is a short-duration crop, usually between 60 and 90 days from planting to harvest (Sandhu and Singh, 2021). Mung beans, being relatively heat- and drought-tolerant, may be helpful in the agricultural adaptation to climate change (Pataczek et al., 2018; Wang et al., 2018). Mung bean is easily digestible, with seed composition of 22–28% protein, 1–1.5% fat, and 60–65% carbohydrates, as well as minerals, vitamins, and antioxidants (Jahan et al., 2020; Aski et al., 2021; Sandhu and Singh, 2021). Mung beans are consumed as whole grain, sprouted gram, split dhal, and mung bean flour in Indian dishes (Nair et al., 2019; Aski et al., 2021). The complementation of mung beans with cereals provides a balanced intake of required nutrients. In Western cultures, mung beans are consumed mostly as sprouts and, more recently, as processed products such as meat substitutes, egg substitutes, chips, no-nut butter, and pasta (Sandhu and Singh, 2021). The demand for plant-based protein in the United States has led to enhancing existing and establishment of breeding programs at United States institutions, including at Iowa State University (Sandhu and Singh, 2021). However, due to limited breeding efforts in North America, there is a knowledge gap in agronomic trait diversity including root traits that are emerging as important area of research and breeding efforts (Lynch, 2007; White et al., 2013; Burridge et al., 2017).
Root system architecture (RSA) can be defined as the morphology of the root system at many scales, both global (i.e., the entire root system) and local (i.e., primary and lateral root levels), as well as the spatial variability of the morphology (Hodge et al., 2009; Rogers and Benfey, 2015; Lobet et al., 2019; Aski et al., 2021). The morphometric traits include the number, length, volume, mass, shape, angle, depth, etc. The spatio-temporal variation seen in RSA of different plants reflects the phenotypic plasticity and the genotype × environment interaction (Rogers and Benfey, 2015; Lobet et al., 2019). Roots have a great impact on yield and plant fitness by providing plants with the structural stability, nutrient foraging, plant-microbe interactions, preventing soil erosion, aeration, and water extraction (Hodge et al., 2009; Rogers and Benfey, 2015).
The desired root phenotypes by plant breeders will be ones that enhances plant adaptation to the edaphic stress while maintaining or increasing yields, for example, deeper and proliferating roots are desired during water-deficient stresses in the changing climate (Gaur et al., 2008; Aski et al., 2021). Lynch and Brown (2001) coined the term “topsoil foraging ideotype,” which is characterized by proliferation of lateral roots, long root hairs, association with mycorrhizal fungi, and suited to uptake of the immobile phosphorus mineral from the topsoil stratum (White et al., 2013). The “steep, cheap, and deep” ideotype (Lynch, 2013) optimizes on the uptake of water and the soluble nitrogen in the soil minimizing leaching. The “steep, cheap, deep” is characterized by thick and long primary roots, high affinity for N by epidermal cells, and the high concentration of cortical aerenchyma cells (White et al., 2013). Falk et al. (2020b) used the term “informative root” (iRoot) category to capture the biological significance of the captured root traits as would simulate field conditions. They reported that the topsoil foraging had a faster total root length-growth rate (TRL_GR), wider (WID), and a large TRL upper root ratio (TRLUpper). The steep, cheap, and deep ideotype contained a deeper primary root length (PRL), faster TRL_GR, steep lateral root angles (LRA), and lower solidity traits (SOL2). These works have been possible due to the use of computer vision and machine learning in extraction of complex traits.
Advances in computer vision, machine learning, and high-throughput phenotyping (HTP) technologies, coupled with efficient statistical methods and collaborative research, have opened the way for more research to be carried out in plants as reviewed in Singh et al. (2016, 2018), Atkinson et al. (2019), Ghosal et al. (2019), Parmley et al. (2019), and Singh A. et al. (2021). The use of these technologies has been implemented in the collection of agronomic and yield estimation traits (Riera et al., 2021), detection of abiotic and biotic stress (Naik et al., 2017; Zhang et al., 2017; Nagasubramanian et al., 2018, 2019), and monitoring plant health (Ghosal et al., 2018). However, as shown in Falk et al. (2020a), computer vision and machine learning-based methods are essential to advance the root phenotyping and large-scale studies (Singh et al., 2016, 2018; Singh D. P. et al., 2021). Root phenotyping is classified depending on where it is carried out, i.e., in controlled environments or in the field, destructive or nondestructive, and whether the HTP uses 2-dimensional (2D) or 3-dimensional (3D) to capture the traits of interest (see reviews, Atkinson et al., 2019; Singh A. K. et al., 2021).
Previous methods developed for extracting roots in the field include destructive methods such as “shovelomics” (Trachsel et al., 2011), the use of soil cores (Wasson et al., 2016), and nondestructive methods such as electrical resistance tomography (Srayeddin and Doussan, 2009), electromagnetic inductance (Shanahan et al., 2015), and ground penetrating radar (Liu et al., 2018). Soil opacity is still a limiting factor to access roots in most field experiments. Controlled environmental methods include the use of rhizotrons, which utilize soil (Rellán-Álvarez et al., 2015), nonsoil methods such as hydroponics (Aski et al., 2021), transparent artificial growth media (Ma et al., 2019), and growth pouches (Tan and Nopamornbodi, 1979). The high-throughput nature of acquiring 2D root images from controlled environments necessitated the development of the image analysis software to extract the traits (Atkinson et al., 2019). Commercially available software includes WinRhizo (Regent Instruments, Quebec, Canada). The open-source software available for use includes SmartRoot (Lobet et al., 2011), RootNav (Pound et al., 2013), GiaRoots (Galkovskyi et al., 2012), DART (Le Bot et al., 2010), Ez-Rhizo (Armengaud, 2009), DIRT (Das et al., 2015), ARIA (Pace et al., 2014; Falk et al., 2020a), RhizoVision (Seethepalli et al., 2020), MyRoot (Betegón-Putze et al., 2019), and IJ_Rhizo (Pierret et al., 2013). A combination of the methods above has been used to study the roots of a variety of plants under various conditions. Species of plant roots studied include common bean (Bonser et al., 1996), maize (Hund et al., 2009; Zheng et al., 2020), wheat (Atkinson et al., 2015), pearl millet (Passot et al., 2016), soybean (Falk et al., 2020a), and canola (Gioia et al., 2016). In a recent study, Aski et al. (2021) utilized modified hydroponics to study the RSA phenotypic diversity of the mung bean mini-core collection at the World Vegetable Center (formerly known as Asian Vegetable Development and Research Center [AVRDC]) (Schafleitner et al., 2015). As this software is capable of generating the useful data on multiple phenotypic root traits, these also lend themselves to genetic studies.
Genome-wide association studies (GWAS) is a statistical tool that uses historical recombination events to uncover the significant genotypic variation associated with the phenotypic variation for the trait of interest (Huang and Han, 2014; Tibbs Cortes et al., 2021). GWAS has been extensively used to investigate important agronomic traits such as plant height, days to flower, yield, nutrient content, flood and drought tolerance, and insect and pest resistance in maize (Yang et al., 2014), soybean (Zhang et al., 2015; Fang et al., 2017), common bean (Kamfwa et al., 2015), mung bean (Sandhu and Singh, 2021), and rice (Huang et al., 2010) among others.
The current study was conducted with the objectives to (1) study the diversity of the RSA trait in the Iowa (IA) mung bean panel, (2) contextualize these RSA traits with root-based ideotypes, and (3) conduct GWAS on RSA traits and identify candidate genes for these associations.
Materials and Methods
Plant Materials
A total of 376 accessions were used in this study. A total of 372 Plant Introductions (PI) were filtered from the 482 IA mung bean panel (Sandhu and Singh, 2021) using the identity-by-state method in SNPRelate and genetic distance of Nei (Zheng et al., 2012). PIs that were common among the two methods were dropped. The 482 PIs were a part of the over 3,000 mung bean accessions obtained from the United States Department of Agriculture-Germplasm Resources Information Network (USDA-GRIN), in Griffin Georgia that were able to flower and form pods in IA conditions. Three Asian Vegetable mung bean (AVMU) lines, namely, AVMU001, AVMU0201, and AVMU9701, were included as checks, since they are improved cultivars from the WVC, formerly AVRDC (Fernandez and Shanmugasundaram, 1988).
Experimental Design and Germination Protocol
This study used a randomized incomplete block design, with each growth chamber serving as a replicate, for a total of eight replications for the experiment. Two growth chambers were used for an increased throughput. Each chamber had four blocks. Each block contained six complete and two incomplete sub-blocks. Each complete sub-block held twelve genotypes, while each incomplete sub-block held eleven genotypes. The genotypes were randomized within each block and sub-blocks. Randomization was generated using the R package blocksdesign (Edmondson and Edmondson, 2021). The procedures described in Falk et al. (2020a) were followed with little modification in the experimental design (Figure 1). First, ten seeds of each genotype were equally spaced near the top (∼1″) of a 9″ × 12″ germination paper. The paper was rolled into germination rolls. All the germination rolls for each sub-block were rubber banded and labeled with a tag. Once all the 376 were planted, water was filled halfway in the rectangular bucket, and the rolls transferred to a Conviron growth chamber (Controlled Environments Ltd., Winnipeg, Canada) set at 25°C for 16 h of light and 20°C for 8 h darkness. The lighting was set to 276–280 μmol/s/m2 and constantly monitored using the LI-250A photometer (Li-Cor Biosci-sciences, Lincoln, NE, United States). On the 5th day of germination, a representative sample for each genotype was picked and carefully placed onto the 12″ × 18″ blue germination paper (Anchor Paper, Minneapolis, MN, United States). Labeled bar-coded tags are stapled onto the 1″ folded top of the blue paper. A 12″ × 16″ brown blotting paper (Anchor Paper, Minneapolis, MN, United States) was carefully placed on top of the blue paper. Two full blue papers are clipped together using binder clips and placed in the rack on the plot number in the chamber. Chamber conditions were monitored daily.
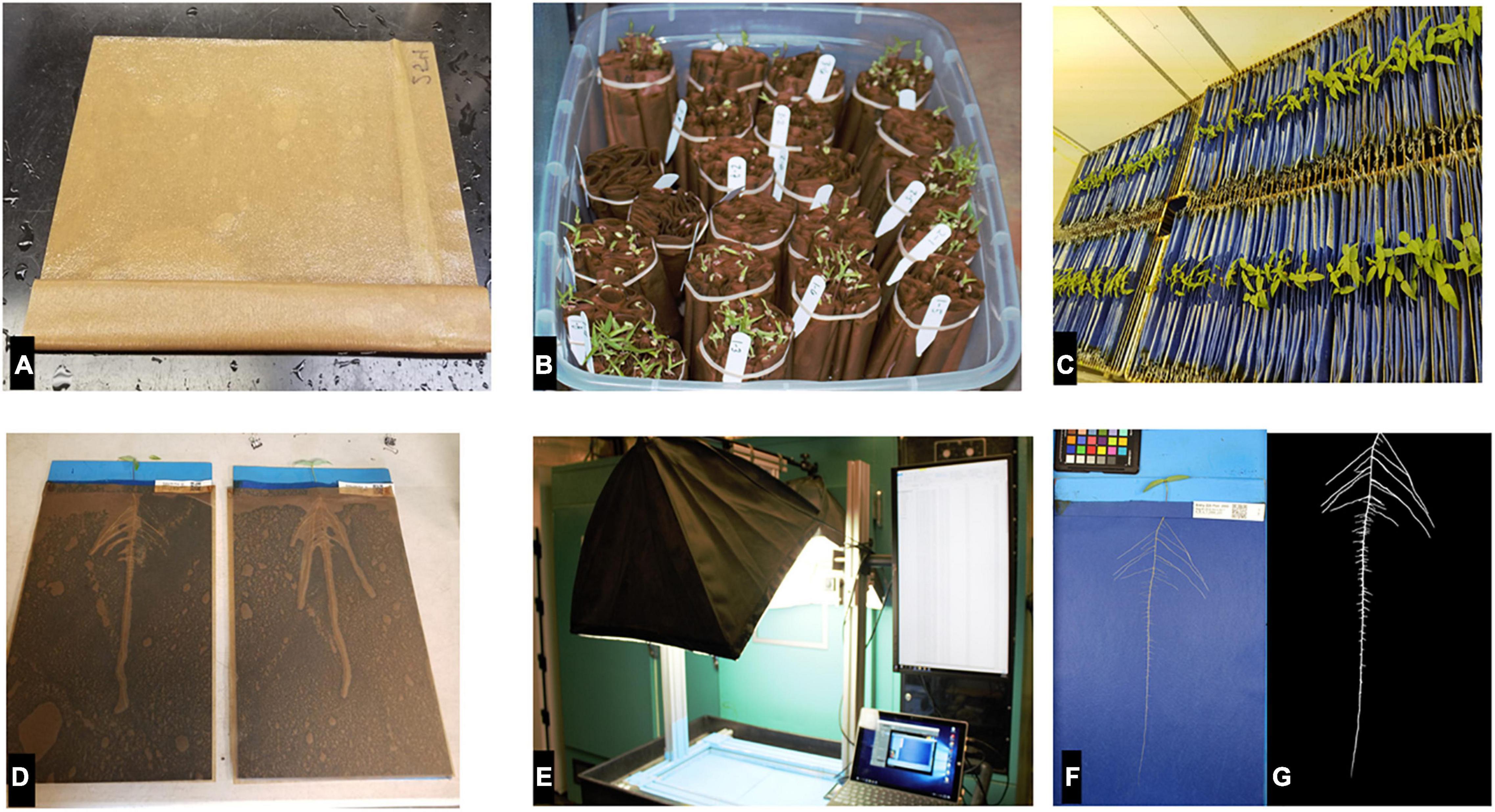
Figure 1. Workflow from seed to phenotyping roots, (A) germination roll, (B) germination rolls banded by sub-block, (C) seedlings in the four blocks inside the growth chamber, (D) genotypes pooled out for imaging, (E) imaging platform, (F) captured root, and (G) preprocessed root ready for trait extraction.
Imaging, Image Processing, and Trait Extraction
A high-throughput imaging station was set up similar to the one reported by Falk et al. (2020a). Images were captured using a Canon T5i digital SLR camera (lens: EF-S 18–55 mm f/3.5–5.6 IS II) (Canon USA, Inc., Melville, NY, United States). The setup allowed for the automated renaming of images captured using the SmartShooter software (Hart, 2021; Figure 1E). The seedlings were imaged on days 12, 15, and 18 without moving roots. Exceptions to moving were on day 12 where some secondary roots did not emerge from the fold and on day 18, when some of the roots of the genotypes were overgrowing the length of the blue paper. The days to image and the spacing were determined by a preliminary study. On the 18th day, the seedlings were cut at the junction between the shoot and camera-visible root section. The root and shoot of each genotype were placed in small brown bags. The wet-cut seedlings were dried in growth chambers set at 34°C/24 h for 2 days, with the light set to 276–280 μmol/s/m2 and stored for weighing. Each root and shoot of the genotype were measured using the Ohaus portable weighing balance (Ohaus Corporation, NJ, United States).
More than 9,000 (376 genotypes × 8 reps × 3 time points) images were collected from the whole experiment. The images were first rotated manually to portrait orientation to enable consistent preprocessing. Images with no germinated seed, herein referred to as blank, were excluded from processing. JPEGCrops (2021), an open-source software, was used to auto crop all the images in a batch by cutting off the top part with the labeled bar-coded tag. The images were then converted into black/white images by thresholding (heuristically using red, green, and blue, LAB, or Hue, Saturation, and Value color spaces). This was carried out using the image processing step and followed by the trait extraction step within the improved Automatic Root Image Analysis (ARIA) 2.0 tool (Falk et al., 2020a). Different color spaces were used due to the variations in the images caused by unequal lightning and water spots. The ARIA 2.0 tool runs on Matlab (2020a). Traits extracted in ARIA are shown in the Supplementary Materials (Supplementary Table 1).
Statistical Analysis
All the analyses were carried out using the R statistical software (R Core Team, 2021). A separate code was written for the extraction of median LRA. Outliers were filtered out using the Tukey’s box plot method (Hoaglin, 2003). A soybean genotype previously included was dropped due to clear visual differences with mung beans. The preprocessing steps above left 8,611 observations represent 375 genotypes for the analysis. Most of the reported analysis is from day 15 data with references and comparisons to days 12 and 18. Day 15 was chosen as a good representation of root growth between day 12 and day 18. A subset of ARIA traits was used for the analysis (Table 1). They were informed by traits used in a similar study by Aski et al. (2021) and traits important for the iRoot categories: topsoil foraging, and steep, cheap, and deep ideotypes described by Falk et al. (2020b). A mixed linear model (Eq. 1) was used to extract the best linear unbiased predictors (BLUPs) for each trait per genotype. All model variables were considered a random effect except chamber, which was a fixed effect. The model was run within the H2Cal function from the inti package (Lozano-Isla, 2021), which utilized the unbalanced data (Cullis et al., 2006; Piepho and Möhring, 2007; Schmidt et al., 2019). Broad sense heritability (H) was calculated using Eq. 2 (Cullis et al., 2006). Pearson correlations were used to draw correlations among the root traits.
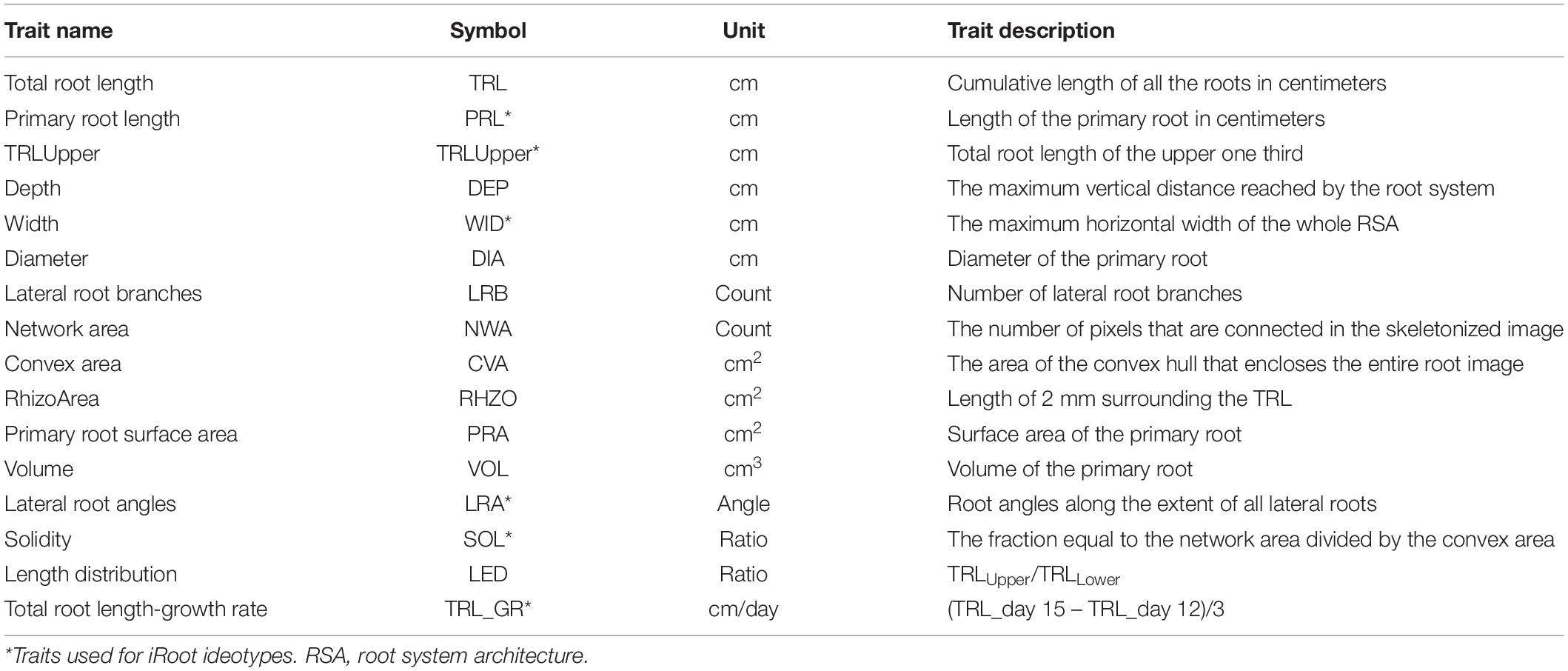
Table 1. Subset of traits of mung bean root architecture extracted from the Automated Root Image Analysis (ARIA) software and used for the analysis, clustering, and iRoot category.
Where μ is the overall population mean, Yijkl is the phenotypic trait, Chamberi is the fixed effect of the ith growth chamber (1| Chamber:Block)ij is the random interaction effect between the ith chamber and the jth block (1| Chamber:Block:Sub-block)ijk is the three way random interaction effect between the ith chamber, jth block and kth sub-block (1| Genotype)l is the random effect of the lth genotype, and eijkl is the random error term following the N(0,q2e).
Where is the genotypic variance and is the mean variance of the difference of two genotypic BLUPs for the genotypic effect (Schmidt et al., 2019).
Root Ideotypes, Phenotypic, and Genotypic Diversity
The iRoots were formed by first ranking the genotypes under each trait, getting the sum of the ranks and then ranking the sums for each category. For topsoil foraging, the genotypes were ranked individually under the TRL_GR, WID, and TRLUpper. The sum of the ranks was ranked, and this yielded to the final ranking of each genotype. A similar approach was used for the “steep, cheap, and deep” ideotype using the TRL_GR, steep LRA, and SOL2.
The principal component analysis (PCA) and hierarchical clustering were used in both the phenotypic and genotypic clustering of the genotypes using the Euclidean distance matrix. The base R function hclust with methods “complete” and “prcomp” was used. The package factoextra (Kassambara and Mundt, 2020) was used to determine the optimum number of clusters to be used by comparing 30 different indices. The clusters were related to the country of origin. Heat maps were developed according to the trait performance and iRoot category ranking using the Complex Heatmap package (Gu et al., 2016). Phenotypic and genotypic dendrograms were made using the dendextend (Galili, 2015) and circlize (Gu et al., 2014) packages. The pairwise fixation index (Fst) was calculated between the two genotypic clusters using the function genet.dist (method = “WC84”) within the ade4 package (Dray Stéphane, 2007). Fst is an indication of the amount of differentiation within subpopulations, with low Fst indicating high gene flow (low genetic diversity) (Wright, 1965).
Genome-Wide Association Analysis
In total, 26,550 SNPs (marker data) were obtained using genotype-by-sequencing and preprocessed earlier by Sandhu and Singh (2021). Sites with >15% missing data and minor allele frequency > 0.01 were filtered out. GWAS was carried out using BLUPs on all the trait data. Associations were conducted using the Trait Analysis by aSSociation, Evolution, and Linkage (TASSEL, Bradbury et al., 2007) software using a linear mixed model (Yu et al., 2006). Both the kinship matrix and PCA were generated in TASSEL controlling for population structure. Bonferroni correction with p-value = 0.05 was used to control for false positives and declare significant associations (Kuo, 2017). Manhattan plots for visualizing the associations were carried out in R using the CMplot library in the rMVP package (Yin et al., 2021). Authors also used a newly developed computational framework, selection of variables with embedded screening (SVEN), a Bayesian based model to run GWAS (Li et al., 2020). The identification of candidate genes was carried out by locating the significant SNP on the sequenced and annotated mung bean genome using the “genome data viewer” tool at the National Center for Biotechnology Information (NCBI; Kang et al., 2014).
Results
Descriptive Statistics, Correlation, and Heritabilities
We observed the significant phenotypic variability for root traits. The coefficient of variation (CV) ranged from 2 to 19% and standard deviation (SD) from 0.01 to 628.67 (different units of measurements for traits). Most of the traits had low SD, i.e., <10 except for TRL, VOL, TRLUpper, and CVA that had SD < 100, while RHZO had an SD > 500. TRL, TRLUpper, CVA, WID, NWA, RHZO, and TRL_GR had 10% < CV < 20%, while the rest of the traits had CV < 10% (Table 2). Day 12 CV ranged from 0 to 22% while for day 18 was 0–28%. Dry matter weight measurements, including shoot dry weight (SDW), root dry weight (RDW), and total dry weight (TDW) had CV 24, 28, and 26%, respectively, at day 18 (Supplementary Table 2).
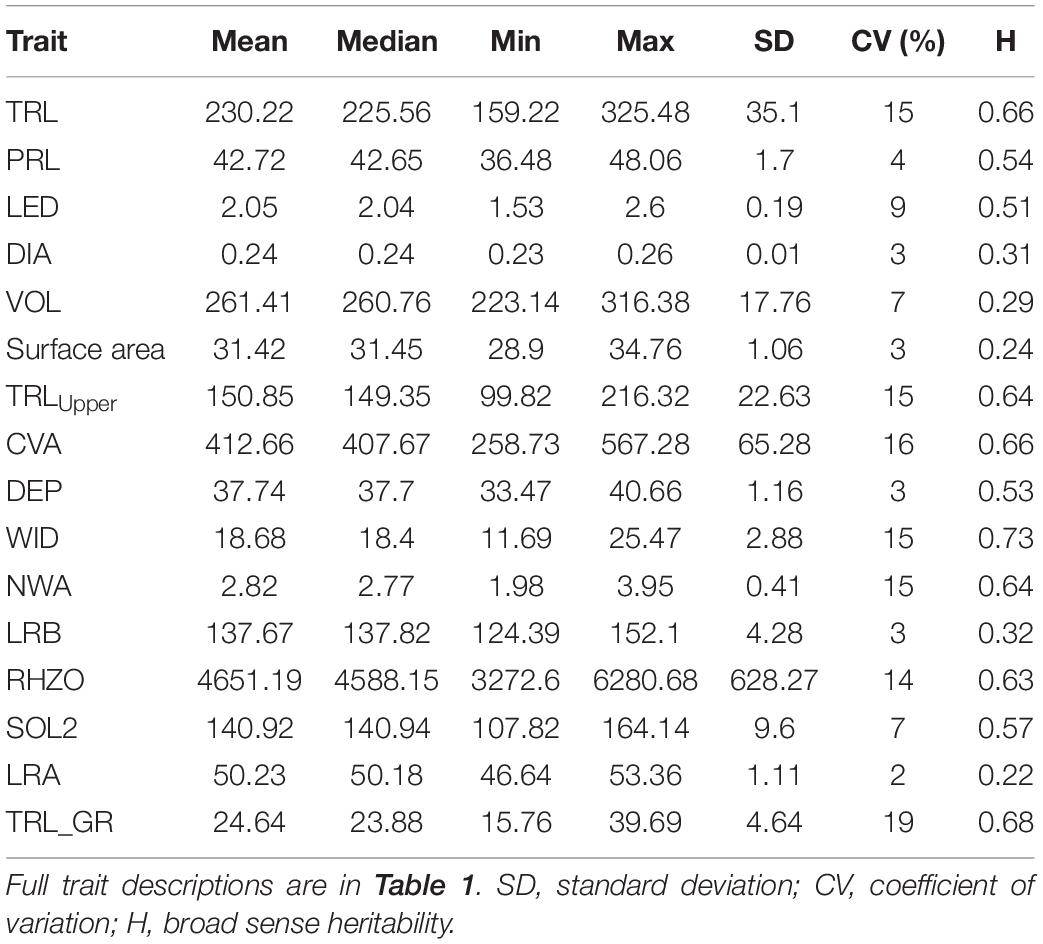
Table 2. Descriptive statistics and broad sense heritability of a subset of root traits from day 15 of the Iowa (IA) mung bean genotypes estimated from eight replications.
The correlation between the root traits varied. TRLUpper was highly correlated with WID, CVA, and TRL_GR. NWA was highly correlated with WID, CVA, TRL_GR, TRLUpper, RHIZO, and TRL. LRA had the lowest correlation with the other traits. There was no correlation between LRA and VOL, and DIA (Figure 2). Correlation on day 12 was high. Negative correlations were observed at day 18 with SOL2 being negatively correlated to most traits and LRA negatively correlated with root shoot ratio (RSR) (image not shown).
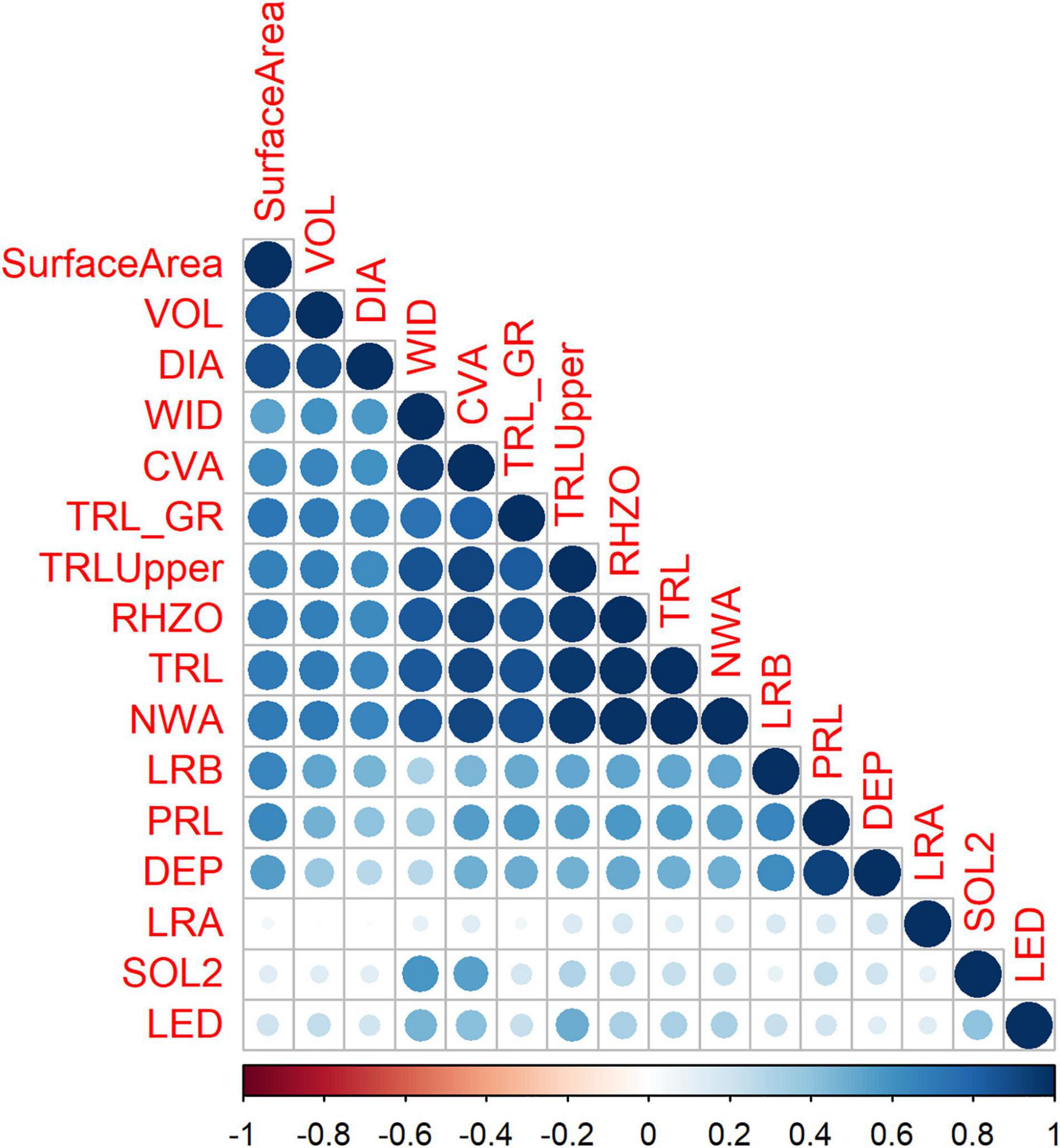
Figure 2. Correlations among the root traits at day 15 using the Iowa (IA) mung bean panel. Experiments were conducted in controlled environment chambers and included eight replications.
Broad sense H ranged from 0.22 to 0.73. LRA and WID had the lowest and highest H at 0.22 and 0.73, respectively. DIA, VOL, surface area, LRB, and LRA had H < 0.5, while TRL, PRL, LED, TRLUpper, CVA, DEP, WID, NWA, RHZO, SOL2, and TRL_GR had H > 0.5 (Table 2 and Figure 3). H was high at days 15 and 18 and low on days 12 for most of the traits. Day 12 H ranged from 0.24 to 0.51, with TRLUpper having the highest H. Day 18 H ranged from 0.23 to 0.87, with dry weight traits (i.e., SDW, RDW, and TDW) showing higher levels at 0.84, 0.87, and 087, respectively (Supplementary Table 2).
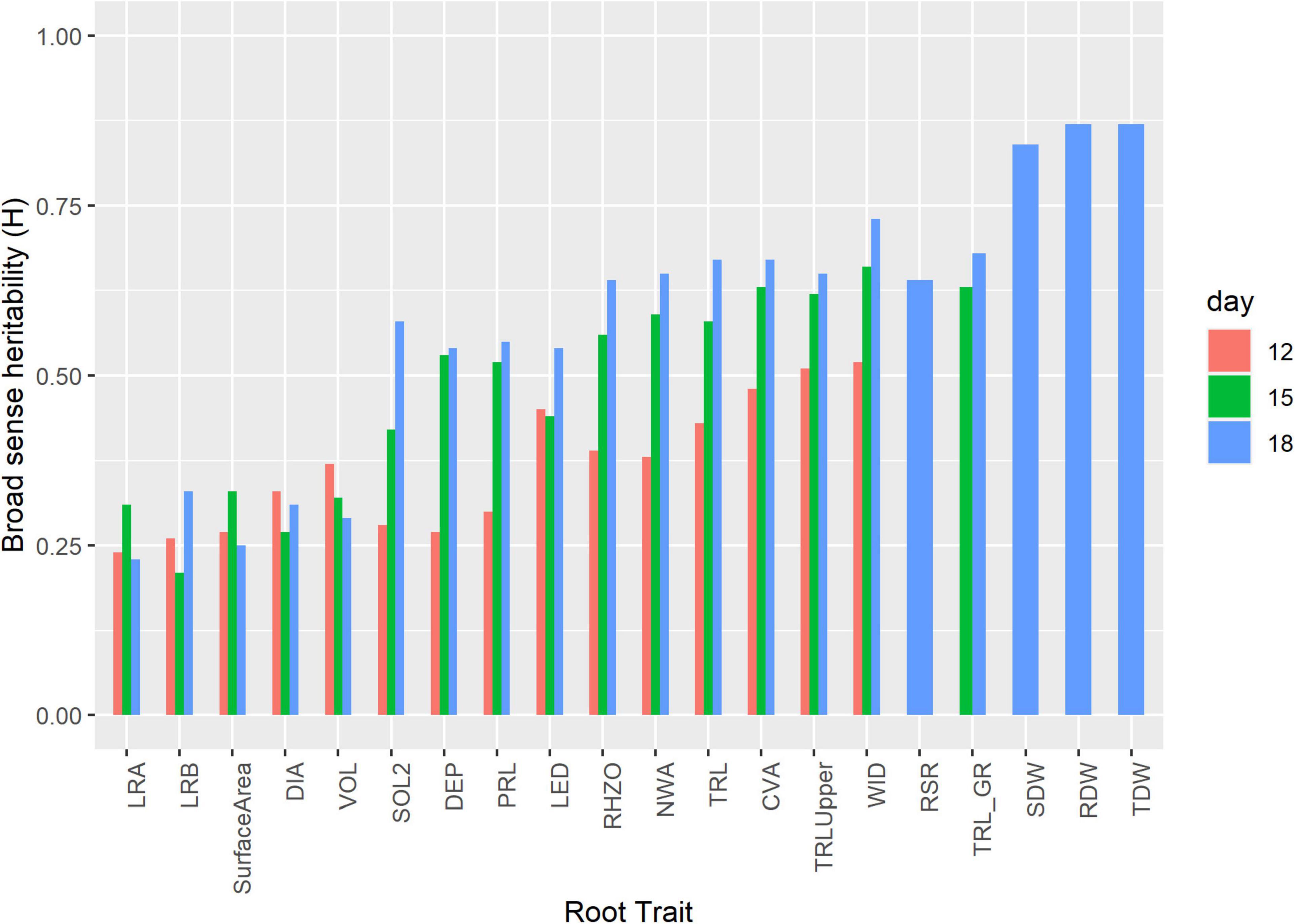
Figure 3. Broad sense heritability (H) of select root related traits at days 12, 15, and 18. Experiments were carried out with the IA mung bean panel with genotypes grown in growth chambers.
Root Ideotypes
We described two root ideotypes, namely, topsoil foraging and “steep, cheap, and deep.” PI425425 (India), PI425045 (Philippines), PI425551 (Korea), PI264686 (Philippines), and PI425085 (Sri Lanka) emerged as top five in the topsoil foraging category. PI425594 (unknown origin), PI425599 (Thailand), PI425610 (Afghanistan), PI425485 (India), and AVMU0201 (Taiwan) were top five in the “steep, cheap, and deep” ideotype (Table 3 and Figure 4). For day 18, the PI425551 (Korea), PI264686 (Philippines), PI426026 (Thailand), PI425085 (Sri Lanka), and PI426042 (Australia) were the top five in the topsoil foraging category. In the “steep, cheap, and deep” ideotype, PI264686 (Philippines), PI425551 (Korea), PI363514 (India), and PI425599 (Thailand) were the top four (Supplementary Table 3). No iRoot categories were created on day 12 since TRL_GR could not be calculated.
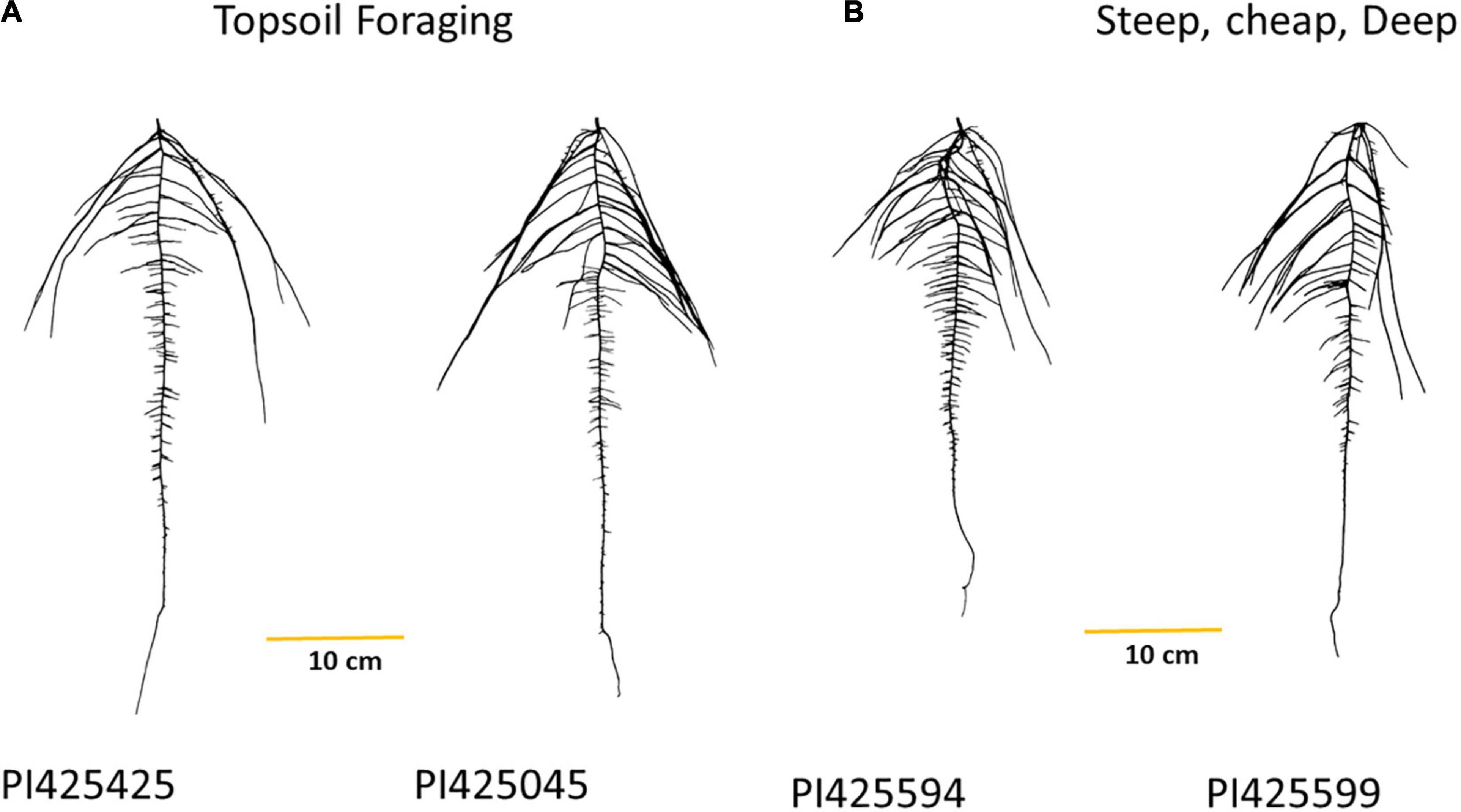
Figure 4. Mung bean iRoot ideotypes, (A) top two genotypes in the topsoil foraging and (B) top two genotypes in the steep, cheap, and deep after ranking the genotypes in the IA mung bean panel.
Phenotypic and Genotypic Clusters
Three distinct phenotypic clusters were observed using the root trait data, while two clusters were observed from the SNP data of the genotypes (Figure 5). Phenotypic clusters 1, 2, and 3 had 69, 163, and 135 genotypes, respectively. Genotypic clusters 1 and 2 had 48 and 319 genotypes, respectively. India had the highest number of genotypes in both genotypic clusters 1 (37) and 2 (197). The United Kingdom had 13 genotypes in genotypic cluster 2, while the rest of the countries had less than 10 genotypes in each cluster. The United Kingdom and United States had no genotypes in genotypic cluster 1 (Supplementary Figure 1). Similarly in the phenotypic clusters 1, 2, and 3, India led with 17, 94, and 123 genotypes. The rest of the countries had less than ten genotypes (Supplementary Table 3). On day 18, there were two phenotypic clusters and two genotypic clusters. Phenotypic clusters 1 and 2 had 250 and 117 genotypes, respectively. Genotypic clusters had similar composition as from day 15. In the phenotypic cluster, India had 132 and 102 genotypes in clusters 1 and 2. The rest had less than 10 genotypes (Supplementary Figure 2 and Supplementary Table 4).
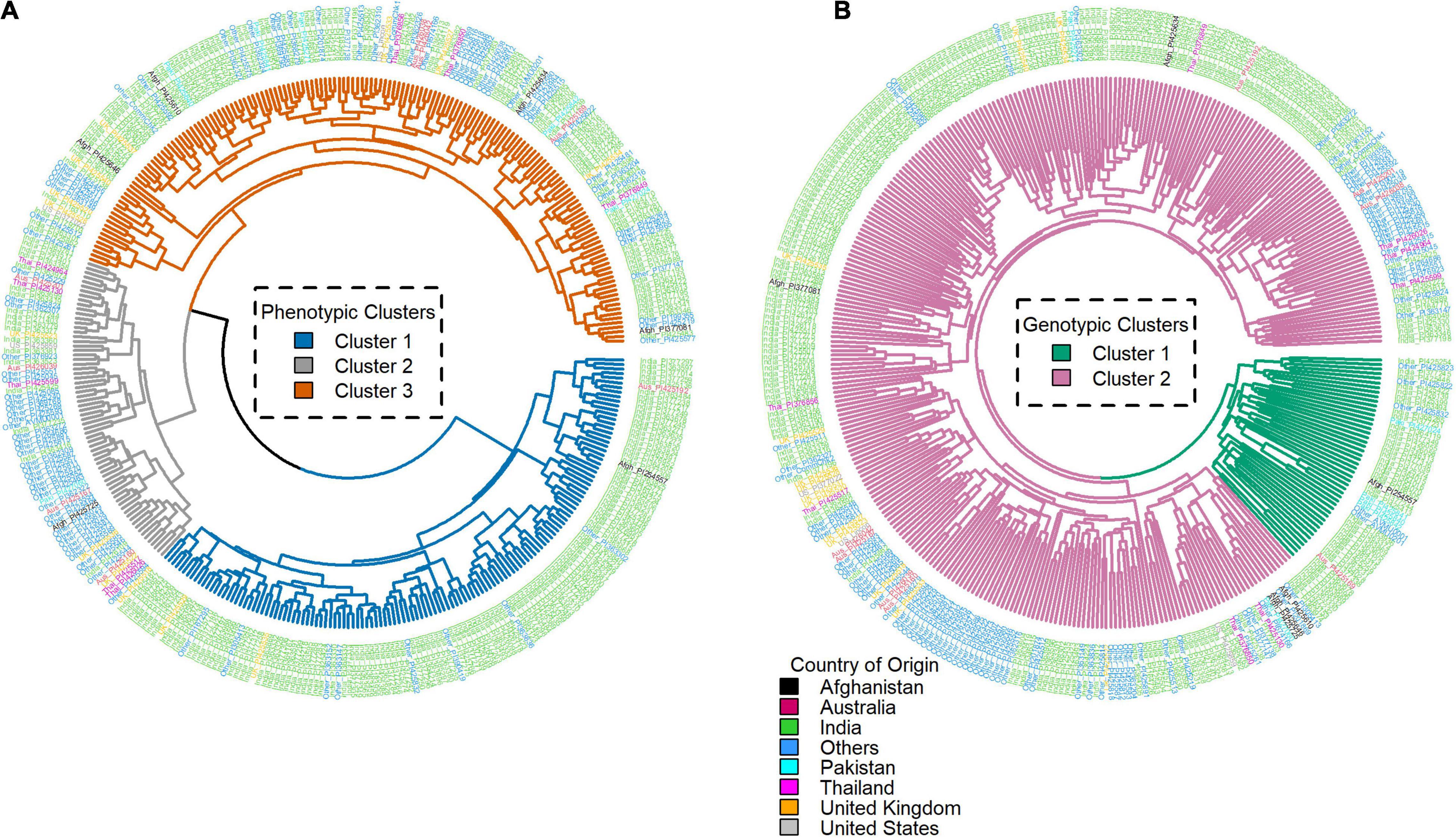
Figure 5. Day 15 phenotypic (A) and genotypic (B) clusters of the root traits and single-nucleotide polymorphism (SNP) data, respectively, generated using hierarchical clustering of the core traits for all the genotypes. The labels represent the Plant Introductions (PI) and country of origin.
PC1 and PC2 explained 7.6 and 3.9% of the total genotypic variation in the IA mung bean GWAS panel (Figure 6). The PCs were not able to discern any distinct subpopulations. Superimposition of iRoot ranking on the PCs showed that genotypes from India dominated both in the “steep, cheap, and deep” and topsoil foraging (Figures 6C,D). For day 18, the top genotypes in the “steep, cheap, and deep” category are mostly from India, while in the topsoil foraging, they are mostly from the other countries, Australia, the United Kingdom, and others with few from India (Supplementary Figure 3). The complex heat map showed the patterns and correlations among the genotypic clusters, iRoot type rank, and root trait performance used in clustering (Figure 7). Most of the traits in genotypic cluster 2 had a better ranking in the topsoil foraging, while cluster 1 contained mostly the worst ranked in the same category. Genotypes were evenly distributed in ranking among the genotypic clusters 1 and 2 in the “steep, cheap, and deep” iRoot category. Some of the best genotypes for the traits, including TRLUpper, RHZO, NWA, WID, and CVA, were in genotypic cluster 2, while cluster 1 was dominated by low values in the above traits. LRA, SOL2, LED, LRB, PRL, and DEP looked evenly distributed within genotypic clusters 1 and 2 (Figure 7). The pairwise Fst was 0.05.
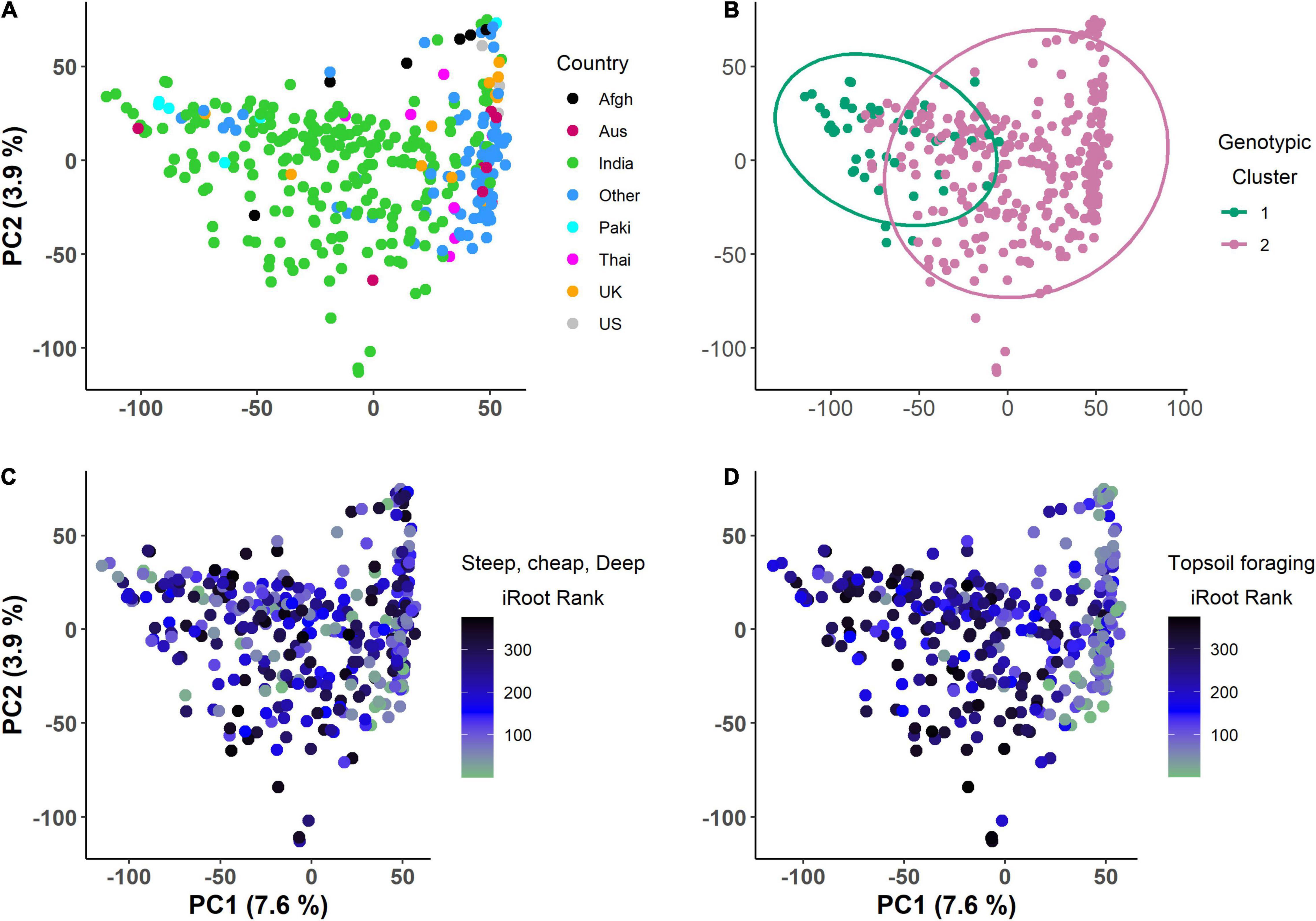
Figure 6. Day 15 principal component analysis of the genotypes for the IA mung bean panel, (A) colored by country of origin, (B) colored by genotypic clusters, (C) color gradient showing ranking in the steep, cheap, and deep iRoot category, and (D) color gradient showing ranking in the topsoil foraging iRoot category (the lower the rank, the better the genotype).
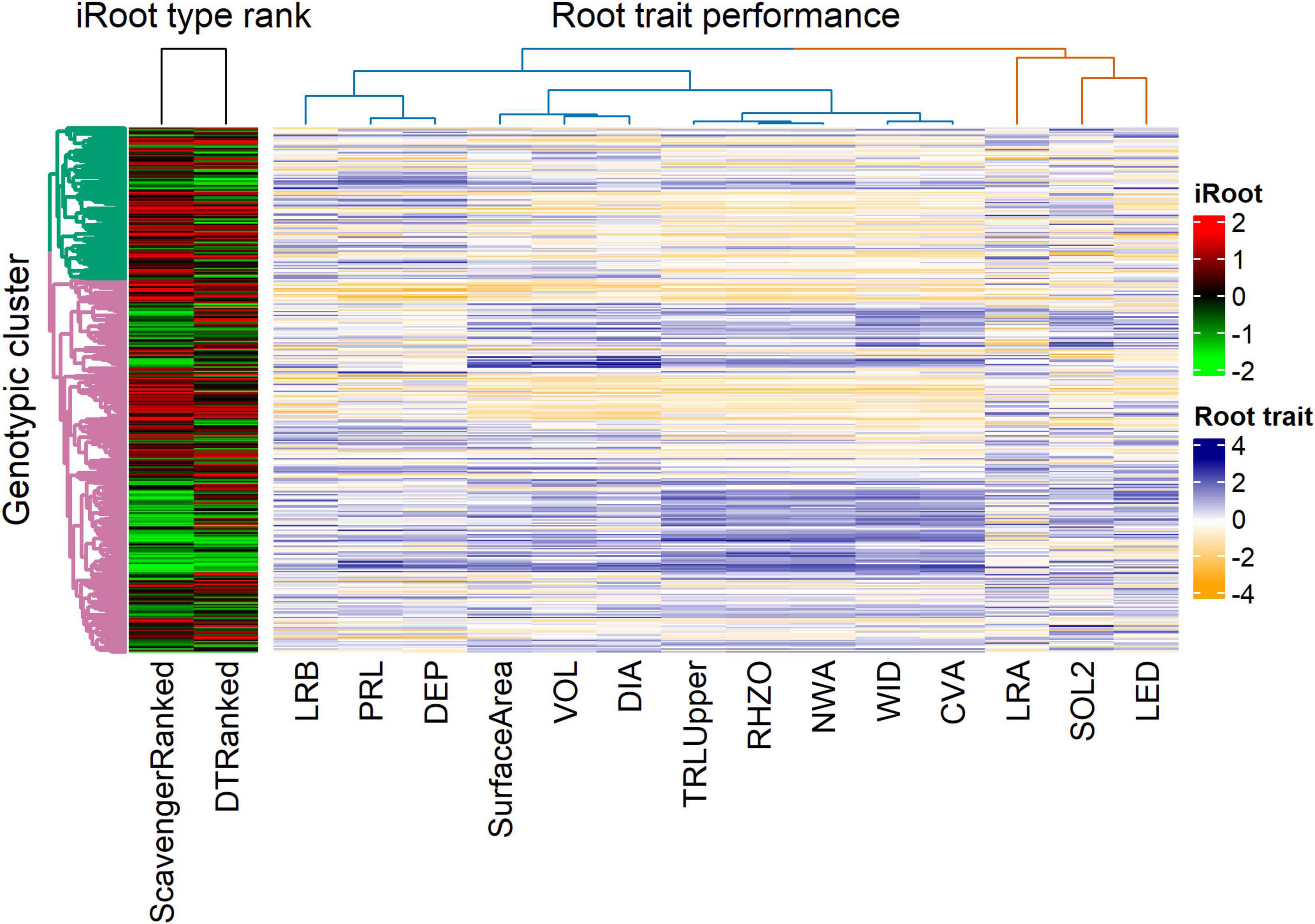
Figure 7. Heat map revealing patterns between the genotypic clusters, iRoot category, and root traits performance for the genotypes in the IA mung bean panel. The dendrogram on the y-axis represents the genotypic clusters (green = 1 and pink = 2). The dendrograms on the top represent the iRoot category and root trait performance.
Genome-Wide Association Studies and Candidate Genes
Association studies revealed significant SNPs for traits on different days. Day 12 LRA had seven significant SNPs. Day 15 LED had one significant SNP. On day 18, TRL_GR, TDW, and volume each had one significant SNP, while LED had two significant SNPs (Figure 8). Out of the seven SNPs for day 12 LRA, the first three had no mapping on the mung bean genome with no gene ID, genomic context, and gene description. On day 18, significant marker 8_10447903 for LED is an uncharacterized gene. Significant SNPs were found for the same trait LED, for days 15 and 18, marker 8_11481602 and marker 8_10447903, respectively. A summary of the significant SNP associations from the TASSEL software is presented in Table 4.
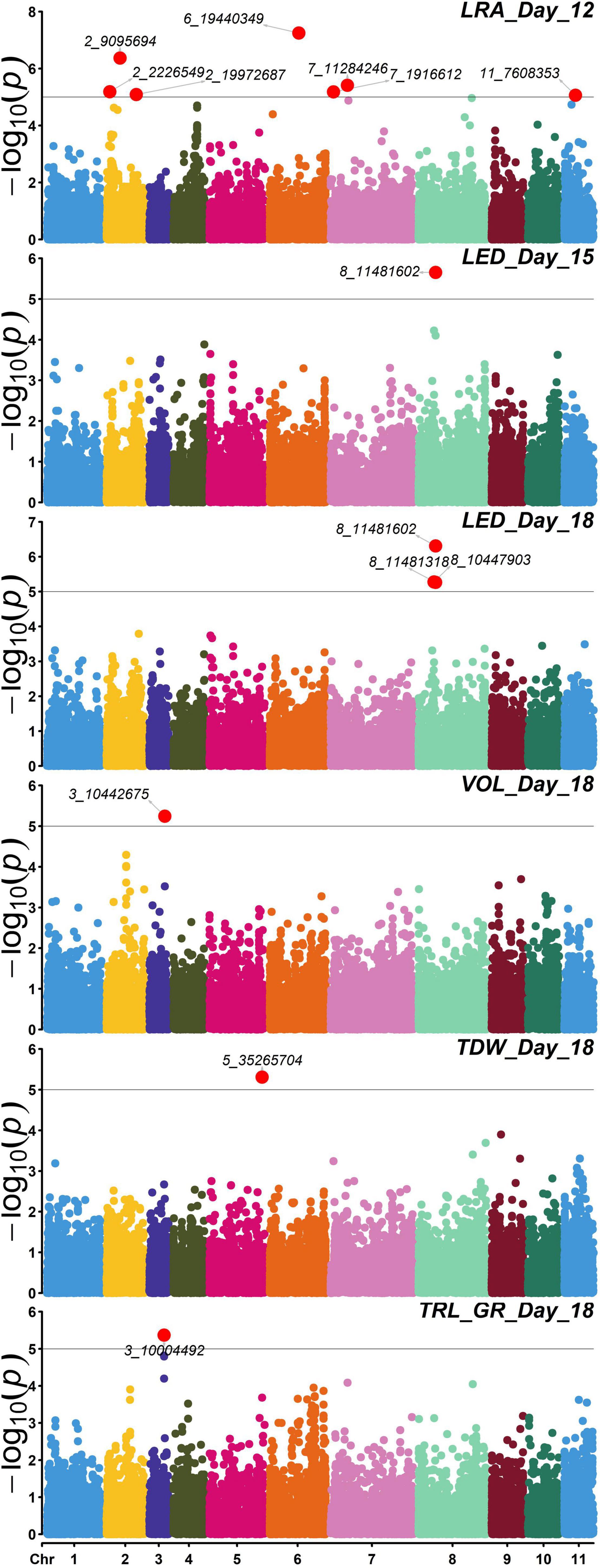
Figure 8. Manhattan plots of –log10(p) vs chromosomes of SNP markers associated with the mung bean traits lateral root angles (LRA) (day 12), length distribution (LED) (day 15), and volume (VOL), LED, total dry weight (TDW), total root length-growth rate (TRL_GR) for day 18. The threshold line is the Bonferroni correction at p = 0.05 [at –log10(p) = 5]. Significant SNPs are highlighted in red and annotated with the marker name. Trait—SNP associations performed using the Trait Analysis by aSSociation, Evolution, and Linkage (TASSEL) software.
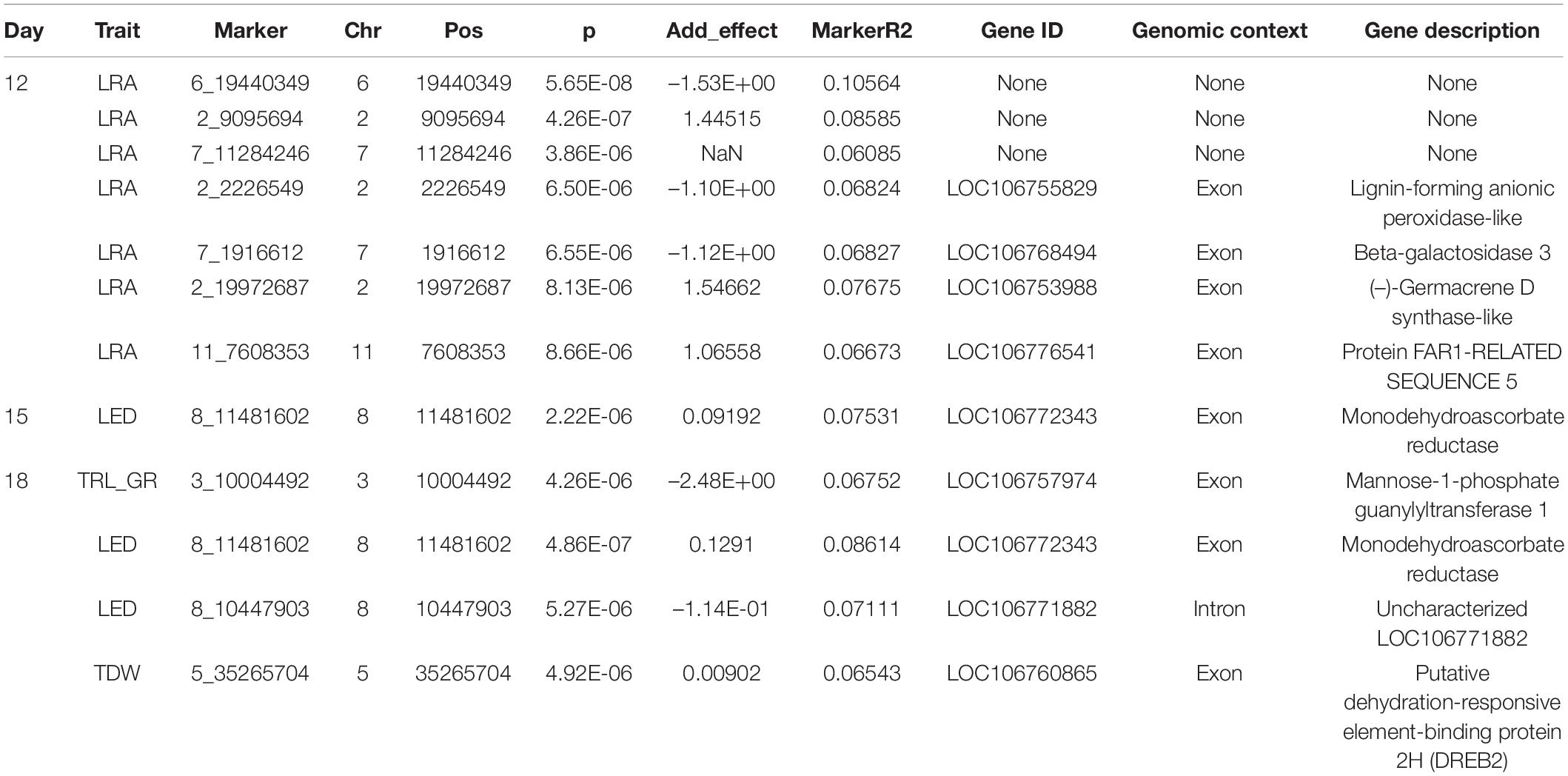
Table 4. Significant single-nucleotide polymorphisms (SNPs) for results of association studies for traits across days 12, 15, and 18 as run in the Trait Analysis by aSSociation, Evolution, and Linkage (TASSEL) software.
Day 12 SNP markers 2_2226549, 2_19972687, 7_19972687, and 11_7608353 were associated with LRA. Marker 2_2226549 is located within an exon for a gene described as lignin forming anionic peroxidase (LOC106755829). Marker 2_19972687 is located within an exon encoding a gene (-)-germacrene D synthase-like (LOC106753988). Marker 7_19972687 is located within an exon of the beta-galactosidase 3 gene (LOC106768494). Marker 11_7608353 associated with LRA also located within an exon for a gene described as protein FAR1-RELATED SEQUENCE 5 (LOC106776541). The same significant SNP marker 8_11481602 associated with LED from days 15 and 18 was found within the exon of a monodehydroascorbate reductase gene (LOC106772343). Day 18 SNP marker 8_10447903 was found within an intron for an uncharacterized gene but close to the LOC106772343 gene. Day 18 SNP marker 3_10004492 associated with TRL_GR is located within the exon for a gene coding for mannose-1-phosphate guanylyltransferase 1 (LOC106757974). Day 18 SNP maker 5_35265704 associated with TDW is located in the exon for a gene described as putative dehydration-responsive element-binding protein 2H (LOC106760865).
Selection of variables with embedded screening resulted in several significant markers for most of the traits across the 3 days (Figure 9, Supplementary Figures 4–6, and Supplementary Table 5). Two markers for LED (8_44518003) and TDW (5_35265704) from day 18 did overlap with TASSEL results. Marker 8_44518003 is an exon within the gene encoding monodehydroascorbate reductase (LOC106772343), while marker 5_35265704 was found within the gene encoding putative dehydration-responsive element-binding protein 2H (LOC106760865). Day 18 marker for TRLUpper (2_22583526) was found within an exon in the gene encoding coilin (LOC106756657), while marker for DEP (5_23119832) was found in an exon within the gene encoding expansin-A11 (LOC106761944).
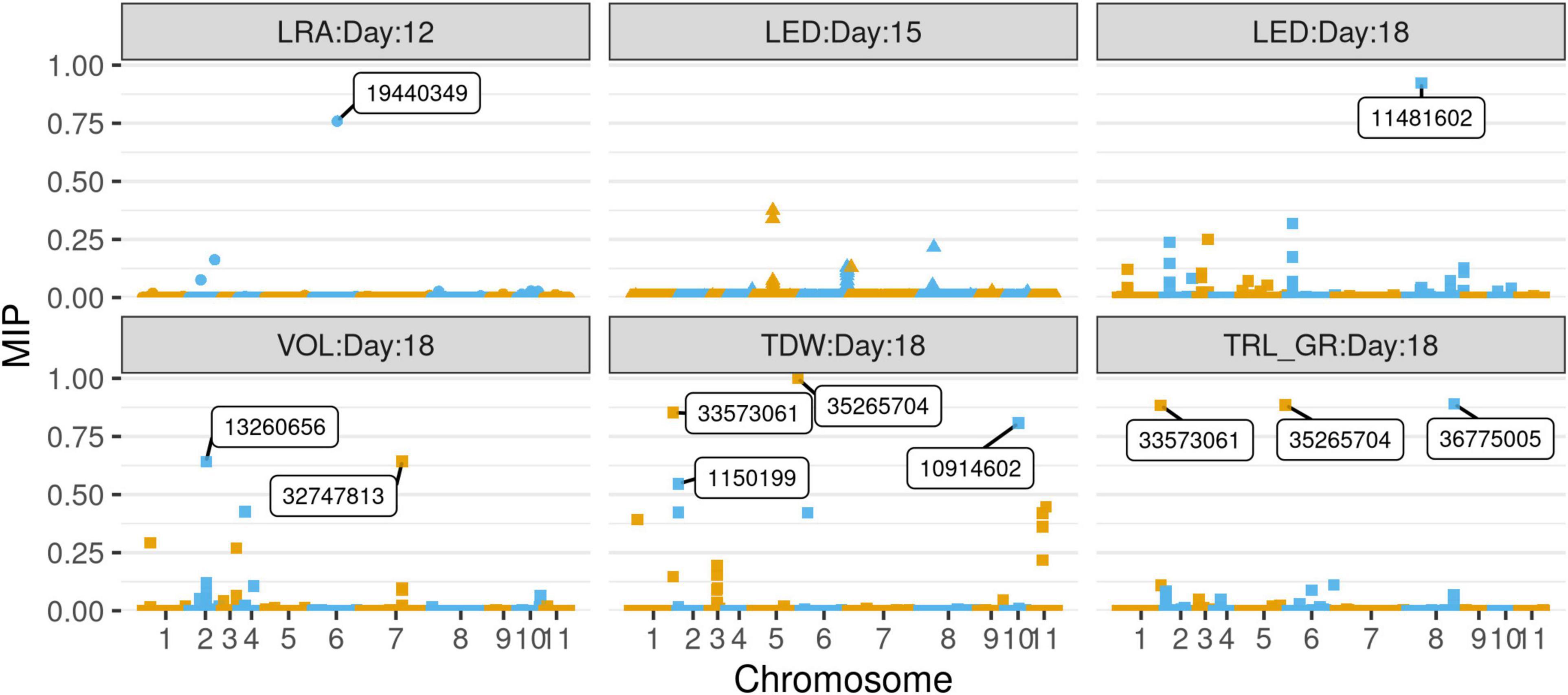
Figure 9. Selection of variables with embedded screening (SVEN) plots of marginal inclusion probability (MIP) vs chromosomes of SNP markers associated with the mung bean traits LRA (day 12), LED (day 15), and LED, VOL, TDW, TRL_GR for day 18. Significant SNPs are boxed with the marker name.
Discussion
Controlled environments have been successfully used to study organisms out of their in situ environments (Crop Science Controlled Environment Research Guidelines, 2021). Plants in controlled environments may be exposed to similar conditions as would be in the field to help better achieve the objectives under study (Tibbitts and Langhans, 1993). There have been successful results for measuring various above-ground phenotypes in controlled environments, but below-ground phenotypes pose additional challenges (White et al., 2013). While studies in controlled environments do not imitate what in situ root environments look like, they are helpful in a priori screening of genotypes to minimize the heavy below-ground phenotyping work required in the field (Lynch and Brown, 2012; Li R. et al., 2015; Ye et al., 2018).
Mung beans are mostly grown on residual moisture after primary crops in most of Southeast Asia (Poehlman and Milton, 1991; Aski et al., 2021). In the Western world, mung beans planted in the summers depend highly on the moisture residue, often following a wet, cold spring. In IA, mung beans are planted around the first week of June, capitalizing on the intense solar radiation for rapid growth (Sandhu and Singh, 2021). This would explain why mung beans, like other legume species, would be ideal with the “steep, cheap, and deep” root ideotypes, to chase the water and the soluble nitrogen before the establishment of root nodules for atmospheric nitrogen fixation. Schneider et al. (2021) showed that steep root angles improved nitrogen uptake in silico in maize. Using the OpenSimRoot model, an 11% increase in nitrogen uptake and a 4% increase in plant biomass were predicted at 40 days of growth (Schneider et al., 2021).
Lynch and Brown (2012) showed that common bean genotypes with wide basal root angles were superior in phosphorus (P) acquisition, while the ones with narrow basal root angles were superior in water acquisition during drought conditions. A recent study looked at the P efficiency of mung bean root morphology traits in low and optimum conditions (Reddy et al., 2020), a trait associated with topsoil foraging. They found Indian improved cultivars would be better with regards to P foraging. We identified the top genotypes, including PI425425 (India), PI425045 (Philippines), PI425551 (Korea), PI264686 (Philippines), and PI425085 (Sri Lanka), in the topsoil foraging (Table 3). Our hypothesis is that this represents the improved germplasm developed in India or after migration from India, while some of the lower ranks are still landraces or wild relatives, but this will need to be evaluated further in field conditions. For example, AVMU0201 is from Taiwan, the World Vegetable Center (Brassica, 2014) (formerly AVRDC), which has been breeding mung beans since the 1970s. Accessions PI425045 and PI264686 are from the Philippines, which also hosts a duplicate mung bean collection at the University of the Philippines, Los Banos (Poehlman and Milton, 1991).
We reported a wide variability of the root trait phenotype in the IA mung bean panel during the early stages of development (Table 2). Indian genotypes represented 24, 56, and 89% in the phenotypic clusters 1, 2, and 3 with an overall presence of 67% (Supplementary Figure 4). The high H for traits on days 15 and 18 could be due to better capture of the traits by ARIA unlike day 12 as it might be too early for trait development and differentiation. The high correlation could also be explained by the fact that young plants are utilizing all nutrients for the vegetative growth. These conclusions cannot be assumed to represent the rest of the developmental stages of mung bean plants, prompting the need for further studies. Similar observations were made in soybean (Falk et al., 2020b).
Genetic variability is one of the most important factors in a breeder’s toolbox (Cobb et al., 2019). Indian genotypes were 76 and 67% in the genotypic clusters 1 and 2 with an overall presence of 63% (Supplementary Figure 4). The lack of clear subpopulations as indicated by the PCs shows the homogeneity within the mungbean accessions (Figure 6). Previous studies have shown similar results using simple sequence repeat markers in the Indonesian germplasm (Lestari et al., 2014) and the USDA germplasm (Wang et al., 2018). In other studies, the STRUCTURE (Pritchard et al., 2000) analysis showed between 3 and 6 subpopulations although no clear pattern was seen according to their geographical origins (Lestari et al., 2014; Wang et al., 2018; Sandhu and Singh, 2021). A similar observation, attributed to population admixture, was shown in common bean (Burle et al., 2010). The low Fst of 0.05 shows a high gene flow or low differentiation between the two genotypic clusters. The low Fst in this study confirms similar earlier reports within the USDA collections (Wang et al., 2018) and within the Indonesian germplasm (Lestari et al., 2014). Overall, results indicate a narrow genetic diversity in mung bean (Poehlman and Milton, 1991; Singh D. P. et al., 2021). Early breeders had to opt for mutation breeding to increase the genetic diversity. The narrow genetic base can be explained by the self-pollinated nature, very low cross-pollination frequencies, and poor hybridization of mung bean with other Vigna species (Poehlman and Milton, 1991; Singh D. P. et al., 2021). The narrow genetic diversity within the IA panel seems to reflect the fact that most of the accessions were collected on the Indian subcontinent, where mung bean was domesticated (Fuller, 2007). Our results support the idea that, in pulses, the lack of genetic diversity is due in part to the continuous use of a few genotypes as parents in the population development (Kumar et al., 2011). This shows the urgency of breeding efforts to diversify the genetic basis.
Adaptive roots to biotic and abiotic stresses will play a key role in bridging the yield gap in crop plants in the changing climate. A solid understanding of the genetic and environmental factors impacting the RSA will be important to the breeding of stable cultivars (see review, Lynch, 2007; Koevoets et al., 2016). RSA traits associated with response to abiotic stresses, including nutrient deficiency, drought tolerance, salinity, flooding, and temperature, and the underlying candidate genes have previously been studied (see review, Koevoets et al., 2016). Narrow LRA, high LED, and increased LRB were highly correlated to high P accumulation in Arabidopsis (Gruber et al., 2013), maize (Zhu et al., 2005), and common bean (Bonser et al., 1996). Auxins and strigolactones are key regulators in root and shoot development. An auxin receptor TRANSPORT INHIBITOR RESPONSE1 (TIR1) was shown to be responsible for the change in LRB as a response to low P levels (Pérez-Torres et al., 2008). Reduced LRB and increased PRL are characteristics of the “steep, cheap, and deep” ideotype, where the plant increases resource allocation to chase water and the mobile N in the deeper soil as evidenced in Arabidopsis and maize (Lynch, 2013). The nitrate transporters NRT1.1 and NRT2.1 were identified for the reduced LRB and increased PRL (Linkohr et al., 2002). The extended root system in Arabidopsis (Yu et al., 2008), rice, cotton, and poplar (Yu et al., 2013) was attributed to HD-ZIP transcription factor (HDG11) which promotes cell elongation by up-regulating cell wall loosening proteins hence important for drought tolerance.
In the current study, several putative candidate genes were identified for root traits associated with genes involved in the plant growth and development and stress tolerance response (Table 4). Lagrimini et al. (1997) proposed that anionic peroxidases, associated with LOC106755829 (day 12 LRA), play a role in plant host defense using a transformed tobacco (Nicotiana tabacum L.) plant. They have also been identified as major enzymes in cell wall lignification and found in large quantities in the xylem tissue (Sasaki et al., 2007). (-)- Germacrene D synthase, associated with LOC106753988 (day 12 LRA), is a member of sesquiterpene synthases family of plant proteins that have the capability of converting a precursor molecule farnesyl diphosphate into many sesquiterpene isoforms (Picaud et al., 2006). (-)- Germacrene D synthase catalyzes the formation (-)- Germacrene D, which is known to have strong effects on insects. Beta-galactosidase 3 is associated with Loci LOC106768494 (day 12 LRA), which has been implicated in adventitious root development via transcriptomic studies in mung bean (Li S.-W. et al., 2015). In rice, beta-galactosidase 1 and 2 were found to be highly expressed in the root and shoot seedlings, with less expression in flowers and immature seeds. Beta-galactosidases are important in the breakdown of molecular complexes (carbohydrates, glycolipids, and glycoproteins) that contain galactose (Chantarangsee et al., 2007). Beta-galactosidases would be important in the supply of the required energy from storage reserves during the rapid growth phase. The Far-related sequence (FRS) family, associated with LOC106776541 (day 12 LRA), is conserved among plants. Genes in this family are involved in multiple cellular processes (Lin Ma-FAR1). For example, Arabidopsis (Arabidopsis thaliana) mutants of fhy3 were less sensitive to both osmotic and salinity stress while also reducing the ABA-dependent inhibition of seedling root elongation, seedling greening, and germination (Tang et al., 2013; Ma and Li, 2018).
Transcripts of the gene encoding monodehydroascorbate reductase associated with LOC106772343 (day 15 and 18 LED), an antioxidant enzyme, were significantly reduced in the root elongation zone when roots for tall fescue (Festuca arundinaceaSchreb.cv. “K-31”) when exposed to water stress (Xu et al., 2015). Water stress is associated with high concentration of reactive oxygen species. A mutation in the Arabidopsis CYT1 gene encoding mannose-1-phosphate guanylyltransferase 1 associated with LOC106757974 (day 18 TRL_GR) showed deficiency in the cell wall after depletion of GDP mannose. The mutants exhibited radial swelling and accumulation of callose at the root tip. The functional analysis revealed mannose-1-phosphate guanylyltransferase 1 is involved in N-glycosylation during the cellulose synthesis (Lukowitz et al., 2001). An orthologous gene (DREB1A/CBF3 and DREB2A) associated with LOC106760865 (day 18 TDW), in Arabidopsis, encodes transcription factors that are involved in activating downstream genes involved in drought and cold stress (Sakuma et al., 2006). In another study, DREB2A proteins were found to increase the stress tolerance by modulating root architecture traits like the lateral root number and root length (Shukla et al., 2006; Agarwal et al., 2010).
Selection of variables with embedded screening loci associated with LOC106756657 (day 18 TRLUpper) and LOC106761944 (day 18 DEP) were associated with the adventitious root development in mung bean like the TASSEL results (Li S.-W. et al., 2015). Coilin is important in the formation of Cajal bodies, which are mostly associated with RNA processes. Kanno et al. (2016) suggest that coilin may be acting in multiple levels fine tuning expression of some genes important for environmental adaptation. Expansins are proteins involved with cell wall loosening and modification, partly mediated by the pH expansion of the cell wall during plant growth (Lee et al., 2001). In rice, Zhiming et al. (2011) identified a gene encoding EXPA17 that was important for the root hair growth, which requires intensive cell wall modification.
The high H among the dry weight measurements can be used in the selection of parents with the root to shoot ratio (RSR) previously used as a measure of the photosynthetic materials allocations (Figure 3). During a low supply of water, nitrogen, and phosphorus in the soil, more resources are allocated to roots relative to shoots (Xu et al., 2015; Lynch et al., 2021). Within legumes QTLs for fibrous rooting/surface area (Abdel-Haleem et al., 2011), root length (Prince et al., 2015), lateral root number, and root thickness (Manavalan et al., 2015; Prince et al., 2019) in soybean have been mapped. In cowpea, QTLs for basal root angle, root diameter, median width, and width accumulation were reported (Burridge et al., 2017). In pea, root length QTL (Fondevilla et al., 2010) and in common bean basal root angle QTL have been identified. Root length density, root surface area, RDW ratio, and root depth in chickpea have been mapped (Jaganathan et al., 2015). In cereals for, maize and sorghum associations with area, convex hull area, median width, maximum width, width-profile angle, and adjusted depth were identified (Zheng et al., 2020), deep root mass, and the number of deep roots in rice (Courtois et al., 2013) and PRL, RDW in wheat (Sanguineti et al., 2007).
Our study has elucidated the phenotypic and genotypic variability for the root traits in the 375 genotypes in the IA mung bean panel. We identified candidate genotypes that can now be advanced to the greenhouse or field for further testing, especially for the root ideotypes. If their trait response and expression can be confirmed, these can be utilized as parents in the breeding program. Using GWAS, we identified significant markers associated with several RSA traits. Taken together, the ideotypes after field evaluation and significant markers can be utilized as tools for marker-assisted selection and crop improvement in mung bean breeding programs.
Data Availability Statement
A subset of the dataset and the R scripts used in the analysis can be found in the github account mungbeanpaper, https://github.com/yalek/mungbeanpaper.git. Raw data is provided in the Supplementary Material. Marker dataset can be found at Dryad Data, doi: 10.5061/dryad.wdbrv15mb. Images can be provided upon request.
Author Contributions
KC and AS conceptualized and designed the experiments. KC performed data collection with the assistance of student workers, did the image preprocessing, data analysis, and wrote the first draft manuscript with feedback from AS and SC on GWAS, and SD and AS on phenotypic analysis. TJ helped with image preprocessing. BG provided feedback on root imaging software. AS provided overall leadership on the project. All authors revised and approved the submitted manuscript.
Funding
This material is based upon work supported by the National Science Foundation under Predictive Plant Phenomics (P3) Grant No. DGE-1545453 (KC), the USDA National Institute of Food and Agriculture (NIFA) Food and Agriculture Cyberinformatics Tools (FACT) (award 2019-67021-29938) (AS and BG), RF Baker Funding (AS), USDA-NIFA Hatch project (IOW03717) (SD), USDA-CRIS (IOW04714) project (AS), USDA Agricultural Research Service, project 5030-21000-069-00D (SC) and AI Institute for Resilient Agriculture (AIIRA), supported by the NSF and USDA award #2021-67021-35329 to BG and AS.
Conflict of Interest
The authors declare that the research was conducted in the absence of any commercial or financial relationships that could be construed as a potential conflict of interest.
Publisher’s Note
All claims expressed in this article are solely those of the authors and do not necessarily represent those of their affiliated organizations, or those of the publisher, the editors and the reviewers. Any product that may be evaluated in this article, or claim that may be made by its manufacturer, is not guaranteed or endorsed by the publisher.
Acknowledgments
We are grateful to Asheesh K. Singh for his infrastructural support on the project. We are grateful to Ken Moore for initial ideas on the experimental designs. We would like to thank the undergraduate students Grace Heck, Megan Besch, Michael Cook, Erin Stichter, and Melinda Zubrod for their unwavering support of this project. We also thank all the graduate students Joscif Raigne, Clayton Carley, Liza Van der Laan, Matthew Carroll, Ashlyn Rairdin, and Sarah Jones for their immense help in data collection and discussions. We also express gratitude to Jennifer Hicks for helping with procuring materials for the project. We are grateful to Aaron Brand for making sure the growth chambers ran smoothly. The findings and conclusions in this publication are those of the author(s) and should not be construed to represent any official USDA or US Government determination or policy. Mention of trade names or commercial products in this publication is solely for the purpose of providing specific information and does not imply recommendation or endorsement by the US Department of Agriculture. USDA is an equal opportunity provider and employer.
Supplementary Material
The Supplementary Material for this article can be found online at: https://www.frontiersin.org/articles/10.3389/fpls.2021.808001/full#supplementary-material
References
Abdel-Haleem, H., Lee, G.-J., and Boerma, R. H. (2011). Identification of QTL for increased fibrous roots in soybean. Theor. Appl. Genet. 122, 935–946. doi: 10.1007/s00122-010-1500-9
Agarwal, P., Agarwal, P. K., Joshi, A. J., Sopory, S. K., and Reddy, M. K. (2010). Overexpression of PgDREB2A transcription factor enhances abiotic stress tolerance and activates downstream stress-responsive genes. Mol. Biol. Rep. 37, 1125–1135. doi: 10.1007/s11033-009-9885-8
Akibode, S., and Maredia, M. (2012). Global and Regional Trends in Production, Trade and Consumption of Food Legume Crops. Available online at: https://ageconsearch.umn.edu/record/136293 (accessed October 11, 2021).
Armengaud, P. (2009). EZ-Rhizo software: the gateway to root architecture analysis. Plant Signal. Behav. 4, 139–141. doi: 10.4161/psb.4.2.7763
Aschemann-Witzel, J., Gantriis, R. F., Fraga, P., and Perez-Cueto, F. J. A. (2020). Plant-based food and protein trend from a business perspective: markets, consumers, and the challenges and opportunities in the future. Crit. Rev. Food Sci. Nutr. 61, 3119–3128. doi: 10.1080/10408398.2020.1793730
Aski, M. S., Rai, N., Reddy, V. R. P., Gayacharan, Dikshit, H. K., Mishra, G. P., et al. (2021). Assessment of root phenotypes in mungbean mini-core collection (MMC) from the World Vegetable Center (AVRDC) Taiwan. PLoS One 16:e0247810. doi: 10.1371/journal.pone.0247810
Atkinson, J. A., Pound, M. P., Bennett, M. J., and Wells, D. M. (2019). Uncovering the hidden half of plants using new advances in root phenotyping. Curr. Opin. Biotechnol. 55, 1–8. doi: 10.1016/j.copbio.2018.06.002
Atkinson, J. A., Wingen, L. U., Griffiths, M., Pound, M. P., Gaju, O., Foulkes, M. J., et al. (2015). Phenotyping pipeline reveals major seedling root growth QTL in hexaploid wheat. J. Exp. Bot. 66, 2283–2292. doi: 10.1093/jxb/erv006
Betegón-Putze, I., González, A., Sevillano, X., Blasco-Escámez, D., and Caño-Delgado, A. I. (2019). MyROOT: a method and software for the semiautomatic measurement of primary root length in Arabidopsis seedlings. Plant J. 98, 1145–1156. doi: 10.1111/tpj.14297
Bonser, A. M., Lynch, J., and Snapp, S. (1996). Effect of phosphorus deficiency on growth angle of basal roots in Phaseolus vulgaris. New Phytol. 132, 281–288. doi: 10.1111/j.1469-8137.1996.tb01847.x
Bradbury, P. J., Zhang, Z., Kroon, D. E., Casstevens, T. M., Ramdoss, Y., and Buckler, E. S. (2007). TASSEL: software for association mapping of complex traits in diverse samples. Bioinformatics 23, 2633–2635. doi: 10.1093/bioinformatics/btm308
Brassica (2014). Home - World Vegetable Center. Available online at: https://avrdc.org/ (accessed October 09, 2021).
Burle, M. L., Fonseca, J. R., Kami, J. A., and Gepts, P. (2010). Microsatellite diversity and genetic structure among common bean (Phaseolus vulgaris L.) landraces in Brazil, a secondary center of diversity. Theor. Appl. Genet. 121, 801–813. doi: 10.1007/s00122-010-1350-5
Burridge, J. D., Schneider, H. M., Huynh, B.-L., Roberts, P. A., Bucksch, A., and Lynch, J. P. (2017). Genome-wide association mapping and agronomic impact of cowpea root architecture. Theor. Appl. Genet. 130, 419–431. doi: 10.1007/s00122-016-2823-y
Chantarangsee, M., Tanthanuch, W., Fujimura, T., and Fry, S. C. Ketudat Cairns, J. (2007). Molecular characterization of β-galactosidases from germinating rice (Oryza sativa). Plant Sci. 173, 118–134. doi: 10.1016/j.plantsci.2007.04.009
Cobb, J. N., Juma, R. U., Biswas, P. S., Arbelaez, J. D., Rutkoski, J., Atlin, G., et al. (2019). Enhancing the rate of genetic gain in public-sector plant breeding programs: lessons from the breeder’s equation. Theor. Appl. Genet. 132, 627–645. doi: 10.1007/s00122-019-03317-0
Courtois, B., Audebert, A., Dardou, A., Roques, S., Ghneim-Herrera, T., and Droc, G. et al. (2013). Genome-wide association mapping of root traits in a japonica rice panel. PLoS One 8:e78037. doi: 10.1371/journal.pone.0078037
Crop Science Controlled Environment Research Guidelines (2021). Available online at: https://www.crops.org/publications/journals/author-resources/cs-instructions/controlled-environment-research/ (accessed October 6, 2021).
Cullis, B. R., Smith, A. B., and Coombes, N. E. (2006). On the design of early generation variety trials with correlated data. J. Agric. Biol. Environ. Stat. 11, 381–393.
Das, A., Schneider, H., Burridge, J., Ascanio, A. K. M., Wojciechowski, T., Topp, C. N., et al. (2015). Digital imaging of root traits (DIRT): a high-throughput computing and collaboration platform for field-based root phenomics. Plant Methods 11:51. doi: 10.1186/s13007-015-0093-3
Dray Stéphane, D. A.-B. (2007). The ade4 Package: implementing the duality diagram for ecologists. J. Stat. Softw. 22, 1–20.
Edmondson, R. N., and Edmondson, M. R. (2021). Package ‘blocksdesign’. Available online at: https://cran.r-project.org (accessed October 27, 2021).
Falk, K. G., Jubery, T. Z., O’Rourke, J. A., Singh, A., Sarkar, S., Ganapathysubramanian, B., et al. (2020b). Soybean root system architecture trait study through genotypic, phenotypic, and shape-based clusters. Plant Phenomics 2020:1925495. doi: 10.34133/2020/1925495
Falk, K. G., Jubery, T. Z., Mirnezami, S. V., Parmley, K. A., Sarkar, S., Singh, A., et al. (2020a). Computer vision and machine learning enabled soybean root phenotyping pipeline. Plant Methods 16:5. doi: 10.1186/s13007-019-0550-5
Fang, C., Ma, Y., Wu, S., Liu, Z., Wang, Z., Yang, R., et al. (2017). Genome-wide association studies dissect the genetic networks underlying agronomical traits in soybean. Genome Biol. 18:161. doi: 10.1186/s13059-017-1289-9
Fernandez, G. C. J., and Shanmugasundaram, S. (1988). “The AVRDC mungbean improvement program: the past, present and future,” in Proceedings of the 1988 Second International Symposium held at Bangkok, Bangkok, 58–70.
Fondevilla, S., Fernández-Aparicio, M., Satovic, Z., Emeran, A. A., Torres, A. M., Moreno, M. T., et al. (2010). Identification of quantitative trait loci for specific mechanisms of resistance to Orobanche crenata Forsk. in pea (Pisum sativum L.). Mol. Breed. 25, 259–272. doi: 10.1007/s11032-009-9330-7
Fuller, D. Q. (2007). Contrasting patterns in crop domestication and domestication rates: recent archaeobotanical insights from the Old World. Ann. Bot. 100, 903–924. doi: 10.1093/aob/mcm048
Galili, T. (2015). dendextend: an R package for visualizing, adjusting and comparing trees of hierarchical clustering. Bioinformatics 31, 3718–3720. doi: 10.1093/bioinformatics/btv428
Galkovskyi, T., Mileyko, Y., Bucksch, A., Moore, B., Symonova, O., Price, C. A., et al. (2012). GiA Roots: software for the high throughput analysis of plant root system architecture. BMC Plant Biol. 12:116. doi: 10.1186/1471-2229-12-116
Gaur, P. M., Krishnamurthy, L., and Kashiwagi, J. (2008). Improving Drought-Avoidance Root Traits in Chickpea (Cicer arietinum L.) -Current Status of Research at ICRISAT. Plant Prod. Sci. 11, 3–11.
Ghosal, S., Blystone, D., Singh, A. K., Ganapathysubramanian, B., Singh, A., and Sarkar, S. (2018). An explainable deep machine vision framework for plant stress phenotyping. Proc. Natl. Acad. Sci. U.S.A. 115, 4613–4618. doi: 10.1073/pnas.1716999115
Ghosal, S., Zheng, B., Chapman, S. C., Potgieter, A. B., Jordan, D. R., Wang, X., et al. (2019). A weakly supervised deep learning framework for sorghum head detection and counting. Plant Phenomics 2019, 1525874. doi: 10.34133/2019/1525874
Gioia, T., Galinski, A., Lenz, H., Müller, C., Lentz, J., Heinz, K., et al. (2016). GrowScreen-PaGe, a non-invasive, high-throughput phenotyping system based on germination paper to quantify crop phenotypic diversity and plasticity of root traits under varying nutrient supply. Funct. Plant Biol. 44, 76–93. doi: 10.1071/FP16128
Gruber, B. D., Giehl, R. F. H., Friedel, S., and von Wirén, N. (2013). Plasticity of the Arabidopsis root system under nutrient deficiencies. Plant Physiol. 163, 161–179. doi: 10.1104/pp.113.218453
Gu, Z., Eils, R., and Schlesner, M. (2016). Complex heatmaps reveal patterns and correlations in multidimensional genomic data. Bioinformatics 32, 2847–2849. doi: 10.1093/bioinformatics/btw313
Gu, Z., Gu, L., Eils, R., Schlesner, M., and Brors, B. (2014). circlize Implements and enhances circular visualization in R. Bioinformatics 30, 2811–2812. doi: 10.1093/bioinformatics/btu393
Hart, F. (2021). Smart Shooter Photography Software. Available on: https://kuvacode.com/smartshooter3 (accessed September 13, 2021).
Hodge, A., Berta, G., Doussan, C., Merchan, F., and Crespi, M. (2009). Plant root growth, architecture and function. Plant Soil 321, 153–187.
Huang, X., and Han, B. (2014). Natural variations and genome-wide association studies in crop plants. Annu. Rev. Plant Biol. 65, 531–551. doi: 10.1146/annurev-arplant-050213-035715
Huang, X., Wei, X., Sang, T., Zhao, Q., Feng, Q., Zhao, Y., et al. (2010). Genome-wide association studies of 14 agronomic traits in rice landraces. Nat. Genet. 42, 961–967. doi: 10.1038/ng.695
Hund, A., Trachsel, S., and Stamp, P. (2009). Growth of axile and lateral roots of maize: I development of a phenotying platform. Plant Soil 325, 335–349. doi: 10.1007/s11104-009-9984-2
Jaganathan, D., Thudi, M., Kale, S., Azam, S., Roorkiwal, M., Gaur, P. M., et al. (2015). Genotyping-by-sequencing based intra-specific genetic map refines a “QTL-hotspot” region for drought tolerance in chickpea. Mol. Genet. Genomics 290, 559–571. doi: 10.1007/s00438-014-0932-3
Jahan, I., Rahman, M. M., Tuzzohora, M. F., Hossain, M. A., Begum, S. N., Burritt, D. J., et al. (2020). Phenotyping of mungbean (Vigna radiata L.) genotypes against salt stress and assessment of variability for yield and yield attributing traits. J. Plant Stress Physiol. 6, 7–17.
Joshi, V., and Kumar, S. (2016). Meat analogues: plant based alternatives to meat products- a review. Int. J. Food Ferment. Technol. 5, 107–119. doi: 10.5958/2277-9396.2016.00001.5
JPEGCrops (2021). Available online at: http://ekot.dk/programmer/JPEGCrops/ (accessed September 13, 2021).
Kamfwa, K., Cichy, K. A., and Kelly, J. D. (2015). Genome-wide association study of agronomic traits in common bean. Plant Genome 8:elantgenome2014. 09.0059.
Kanno, T., Lin, W. D., Fu, J. L., Wu, M. T., Yang, H. W., Lin, S. S., et al. (2016). Identification of coilin mutants in a screen for enhanced expression of an alternatively spliced GFP reporter gene in Arabidopsis thaliana. Genetics 203, 1709–1720. doi: 10.1534/genetics.116.190751
Kassambara, A., and Mundt, F. (2020). factoextra: Extract and Visualize the Results of Multivariate Data Analyses. Available online at: https://CRAN.R-project.org/package=factoextra (accessed September 27, 2021).
Kang, Y. J., Kim, S. K., Kim, M. Y., Lestari, P., Kim, K. H., Ha, B.-K., et al. (2014). Genome sequence of mungbean and insights into evolution within Vigna species. Nat. Commun. 5:5443. doi: 10.1038/ncomms6443
Koevoets, I. T., Venema, J. H., Elzenga, J. T. M., and Testerink, C. (2016). Roots withstanding their environment: exploiting root system architecture responses to abiotic stress to improve crop tolerance. Front. Plant Sci. 7:1335. doi: 10.3389/fpls.2016.01335
Kumar, J., Choudhary, A. K., Solanki, R. K., and Pratap, A. (2011). Towards marker-assisted selection in pulses: a review. Plant Breed. 130, 297–313. doi: 10.1007/s00299-017-2127-y
Kuo, K. H. M. (2017). Multiple testing in the context of gene discovery in sickle cell disease using genome-wide association studies. Genomics Insights 10:1178631017721178. doi: 10.1177/1178631017721178
Lagrimini, L. M., Gingas, V., Finger, F., Rothstein, S., and Liu, T. (1997). Characterization of antisense transformed plants deficient in the tobacco anionic peroxidase. Plant Physiol. 114, 1187–1196. doi: 10.1104/pp.114.4.1187
Le Bot, J., Serra, V., Fabre, J., Draye, X., Adamowicz, S., and Pagès, L. (2010). DART: a software to analyse root system architecture and development from captured images. Plant Soil 326, 261–273.
Lee, Y., Choi, D., and Kende, H. (2001). Expansins: ever-expanding numbers and functions. Curr. Opin. Plant Biol. 4, 527–532. doi: 10.1016/s1369-5266(00)00211-9
Lestari, P., Kim, S. K., Reflinur, Kang, Y. J., Dewi, N., and Lee, S.-H. (2014). Genetic diversity of mungbean (Vigna radiata L.) germplasm in Indonesia. Plant Genet. Resour. .12, S91–S94.
Li, D., Dutta, S., and Roy, V. (2020). Model Based Screening Embedded Bayesian Variable Selection for Ultra-high Dimensional Settings. arXiv [Preprint]. Available online at: http://arxiv.org/abs/2006.07561 (accessed September 27, 2021).
Li, R., Zeng, Y., Xu, J., Wang, Q., Wu, F., Cao, M., et al. (2015). Genetic variation for maize root architecture in response to drought stress at the seedling stage. Breed. Sci. 65, 298–307. doi: 10.1270/jsbbs.65.298
Li, S.-W., Shi, R.-F., and Leng, Y. (2015). De novo characterization of the mung bean transcriptome and transcriptomic analysis of adventitious rooting in seedlings using RNA-Seq. PLoS One 10:e0132969. doi: 10.1371/journal.pone.0132969
Linkohr, B. I., Williamson, L. C., Fitter, A. H., and Leyser, H. M. O. (2002). Nitrate and phosphate availability and distribution have different effects on root system architecture of Arabidopsis. Plant J. 29, 751–760. doi: 10.1046/j.1365-313x.2002.01251.x
Liu, X., Dong, X., Xue, Q., Leskovar, D. I., Jifon, J., Butnor, J. R., et al. (2018). Ground penetrating radar (GPR) detects fine roots of agricultural crops in the field. Plant Soil 423, 517–531. doi: 10.1007/s11104-017-3531-3
Lobet, G., Paez-Garcia, A., Schneider, H., Junker, A., Atkinson, J. A., and Tracy, S. (2019). Demystifying roots: a need for clarification and extended concepts in root phenotyping. Plant Sci. 282, 11–13. doi: 10.1016/j.plantsci.2018.09.015
Lobet, G., Pagès, L., and Draye, X. (2011). A novel image-analysis toolbox enabling quantitative analysis of root system architecture. Plant Physiol. 157, 29–39. doi: 10.1104/pp.111.179895
Lozano-Isla, F. (2021). inti: Tools and Statistical Procedures in Plant Science. Available online at: https://cran.r-project.org/web/packages/inti/citation.html (accessed September 13, 2021).
Lukowitz, W., Nickle, T. C., Meinke, D. W., Last, R. L., Conklin, P. L., and Somerville, C. R. (2001). Arabidopsis cyt1 mutants are deficient in a mannose-1-phosphate guanylyltransferase and point to a requirement of N-linked glycosylation for cellulose biosynthesis. Proc. Natl. Acad. Sci. U.S.A. 98, 2262–2267. doi: 10.1073/pnas.051625798
Lynch, J. P. (2013). Steep, cheap and deep: an ideotype to optimize water and N acquisition by maize root systems. Ann. Bot. 112, 347–357. doi: 10.1093/aob/mcs293
Lynch, J. P., and Brown, K. M. (2001). Topsoil foraging – an architectural adaptation of plants to low phosphorus availability. Plant Soil 237, 225–237.
Lynch, J. P., and Brown, K. M. (2012). New roots for agriculture: exploiting the root phenome. Philos. Trans. R. Soc. Lond. B Biol. Sci. 367, 1598–1604. doi: 10.1098/rstb.2011.0243
Lynch, J. P., Strock, C. F., Schneider, H. M., Sidhu, J. S., Ajmera, I., Galindo-Castañeda, T., et al. (2021). Root anatomy and soil resource capture. Plant Soil 466, 21–63.
Ma, L., and Li, G. (2018). FAR1-RELATED SEQUENCE (FRS) and FRS-RELATED FACTOR (FRF) family proteins in Arabidopsis growth and development. Front. Plant Sci. 9:692. doi: 10.3389/fpls.2018.00692
Ma, L., Shi, Y., Siemianowski, O., Yuan, B., Egner, T. K., Mirnezami, S. V., et al. (2019). Hydrogel-based transparent soils for root phenotyping in vivo. Proc. Natl. Acad. Sci. U.S.A. 116, 11063–11068. doi: 10.1073/pnas.1820334116
Manavalan, L. P., Prince, S. J., Musket, T. A., Chaky, J., Deshmukh, R., Vuong, T. D., et al. (2015). Identification of novel QTL governing root architectural traits in an interspecific soybean population. PLoS One 10:e0120490. doi: 10.1371/journal.pone.0120490
Markiewicz, K. (2010). The Economics of Meeting Future Protein Demand. Wageningen: Wageningen University.
Nagasubramanian, K., Jones, S., Sarkar, S., Singh, A. K., Singh, A., and Ganapathysubramanian, B. (2018). Hyperspectral band selection using genetic algorithm and support vector machines for early identification of charcoal rot disease in soybean stems. Plant Methods 14:86. doi: 10.1186/s13007-018-0349-9
Nagasubramanian, K., Jones, S., Singh, A. K., Sarkar, S., Singh, A., and Ganapathysubramanian, B. (2019). Plant disease identification using explainable 3D deep learning on hyperspectral images. Plant Methods 15:98. doi: 10.1186/s13007-019-0479-8
Naik, H. S., Zhang, J., Lofquist, A., Assefa, T., Sarkar, S., Ackerman, D., et al. (2017). A real-time phenotyping framework using machine learning for plant stress severity rating in soybean. Plant Methods 13:23. doi: 10.1186/s13007-017-0173-7
Nair, R. M., Pandey, A. K., War, A. R., Hanumantharao, B., Shwe, T., Alam, A., et al. (2019). Biotic and abiotic constraints in mungbean production-progress in genetic improvement. Front. Plant Sci. 10:1340. doi: 10.3389/fpls.2019.01340
Niva, M., Vainio, A., and Jallinoja, P. (2017). “10 - Barriers to increasing plant protein consumption in western populations,” in Vegetarian and Plant-Based Diets in Health and Disease Prevention (bll 157–171), ed. F. Mariotti (Cambridge, MA: Academic Press).
Pace, J., Lee, N., Naik, H. S., Ganapathysubramanian, B., and Lübberstedt, T. (2014). Analysis of maize (Zea mays L.) seedling roots with the high-throughput image analysis tool ARIA (Automatic Root Image Analysis). PLoS One 9:e108255. doi: 10.1371/journal.pone.0108255
Parmley, K. A., Higgins, R. H., Ganapathysubramanian, B., Sarkar, S., and Singh, A. K. (2019). Machine Learning Approach for Prescriptive Plant Breeding. Sci. Rep. 9:17132. doi: 10.1038/s41598-019-53451-4
Passot, S., Gnacko, F., Moukouanga, D., Lucas, M., Guyomarc’h, S., Ortega, B. M., et al. (2016). Characterization of pearl millet root architecture and anatomy reveals three types of lateral roots. Front. Plant Sci. 7:829. doi: 10.3389/fpls.2016.00829
Pataczek, L., Zahir, Z. A., Ahmad, M., Rani, S., Nair, R., Schafleitner, R., et al. (2018). Beans with Benefits—The Role of Mungbean (Vigna radiate) in a Changing Environment. Am. J. Plant Sci. 09, 1577–1600. doi: 10.4236/ajps.2018.97115
Pérez-Torres, C.-A., López-Bucio, J., Cruz-Ramírez, A., Ibarra-Laclette, E., Dharmasiri, S., Estelle, M., et al. (2008). Phosphate availability alters lateral root development in Arabidopsis by modulating auxin sensitivity via a mechanism involving the TIR1 auxin receptor. Plant Cell 20, 3258–3272. doi: 10.1105/tpc.108.058719
Picaud, S., Olsson, M. E., Brodelius, M., and Brodelius, P. E. (2006). Cloning, expression, purification and characterization of recombinant (+)-germacrene D synthase from Zingiber officinale. Arch. Biochem. Biophys. 452, 17–28. doi: 10.1016/j.abb.2006.06.007
Piepho, H.-P., and Möhring, J. (2007). Computing heritability and selection response from unbalanced plant breeding trials. Genetics 177, 1881–1888. doi: 10.1534/genetics.107.074229
Pierret, A., Gonkhamdee, S., Jourdan, C., and Maeght, J.-L. (2013). IJ_Rhizo: an open-source software to measure scanned images of root samples. Plant Soil 373, 531–539. doi: 10.1093/aobpla/plab056
Pound, M. P., French, A. P., Atkinson, J. A., Wells, D. M., Bennett, M. J., and Pridmore, T. (2013). RootNav: navigating images of complex root architectures. Plant Physiol. 162, 1802–1814. doi: 10.1104/pp.113.221531
Prince, S. J., Song, L., Qiu, D., Maldonado Dos, Santos, J. V., Chai, C., et al. (2015). Genetic variants in root architecture-related genes in a Glycine soja accession, a potential resource to improve cultivated soybean. BMC Genomics 16:132. doi: 10.1186/s12864-015-1334-6
Prince, S. J., Valliyodan, B., Ye, H., Yang, M., Tai, S., Hu, W., et al. (2019). Understanding genetic control of root system architecture in soybean: insights into the genetic basis of lateral root number. Plant Cell Environ. 42, 212–229. doi: 10.1111/pce.13333
Pritchard, J. K., Stephens, M., and Donnelly, P. (2000). Inference of population structure using multilocus genotype data. Genetics 155, 945–959. doi: 10.1093/genetics/155.2.945
R Core Team (2021). R: A Language and Environment for Statistical Computing. Vienna: R Foundation for Statistical Computing.
Reddy, V. R. P., Aski, M. S., Mishra, G. P., Dikshit, H. K., Singh, A., Pandey, R., et al. (2020). Genetic variation for root architectural traits in response to phosphorus deficiency in mungbean at the seedling stage. PLoS One 15:e0221008. doi: 10.1371/journal.pone.0221008
Rellán-Álvarez, R., Lobet, G., Lindner, H., Pradier, P.-L., Sebastian, J., Yee, M.-C., et al. (2015). GLO-Roots: an imaging platform enabling multidimensional characterization of soil-grown root systems. eLife 4:e07597. doi: 10.7554/eLife.07597
Riera, L. G., Carroll, M. E., Zhang, Z., Shook, J. M., Ghosal, S., Gao, T., et al. (2021). Deep multiview image fusion for soybean yield estimation in breeding applications. Plant Phenomics 2021:9846470. doi: 10.34133/2021/9846470
Rogers, E. D., and Benfey, P. N. (2015). Regulation of plant root system architecture: implications for crop advancement. Curr. Opin. Biotechnol. 32, 93–98. doi: 10.1016/j.copbio.2014.11.015
Sakuma, Y., Maruyama, K., Osakabe, Y., Qin, F., Seki, M., Shinozaki, K., et al. (2006). Functional analysis of an Arabidopsis transcription factor, DREB2A, involved in drought-responsive gene expression. Plant Cell 18, 1292–1309. doi: 10.1105/tpc.105.035881
Sandhu, K., and Singh, A. (2021). Strategies for the utilization of the USDA mung bean germplasm collection for breeding outcomes. Crop Sci. 61, 422–442. doi: 10.1002/csc2.20322
Sanguineti, M. C., Li, S., Maccaferri, M., Corneti, S., Rotondo, F., Chiari, T., et al. (2007). Genetic dissection of seminal root architecture in elite durum wheat germplasm. Ann. Appl. Biol. 151, 291–305. doi: 10.1111/j.1744-7348.2007.00198.x
Sasaki, S., Shimizu, M., Wariishi, H., Tsutsumi, Y., and Kondo, R. (2007). Transcriptional and translational analyses of poplar anionic peroxidase isoenzymes. J. Wood Sci. 53, 427–435. doi: 10.1007/s10086-007-0888-6
Schafleitner, R., Nair, R. M., Rathore, A., Wang, Y.-W., Lin, C.-Y., Chu, S.-H., et al. (2015). The AVRDC - The World Vegetable Center mungbean (Vigna radiata) core and mini core collections. BMC Genomics 16:344. doi: 10.1186/s12864-015-1556-7
Schmidt, P., Hartung, J., Rath, J., and Piepho, H.-P. (2019). Estimating broad-sense heritability with unbalanced data from agricultural cultivar trials. Crop Sci. 59, 525–536.
Schneider, H. M., Lor, V. S. N., Hanlon, M. T., Perkins, A., Kaeppler, S. M., Borkar, A. N., et al. (2021). Root angle in maize influences nitrogen capture and is regulated by calcineurin B-like protein (CBL)-interacting serine/threonine-protein kinase 15 (ZmCIPK15). Plant Cell Environ. doi: 10.1111/pce.14135 [Epub ahead of print].
Seethepalli, A., Guo, H., Liu, X., Griffiths, M., Almtarfi, H., Li, Z., et al. (2020). RhizoVision crown: an integrated hardware and software platform for root crown Phenotyping. Plant Phenomics 2020:3074916. doi: 10.34133/2020/3074916
Shanahan, P. W., Binley, A., Whalley, W. R., and Watts, C. W. (2015). The use of electromagnetic induction to monitor changes in soil moisture profiles beneath different wheat genotypes. Soil Sci. Soc. Am. J. 79, 459–466. doi: 10.2136/sssaj2014.09.0360
Shukla, R. K., Raha, S., Tripathi, V., and Chattopadhyay, D. (2006). Expression of CAP2, an APETALA2-family transcription factor from chickpea, enhances growth and tolerance to dehydration and salt stress in transgenic tobacco. Plant Physiol. 142, 113–123. doi: 10.1104/pp.106.081752
Singh, A., Ganapathysubramanian, B., Singh, A. K., and Sarkar, S. (2016). Machine learning for high-throughput stress phenotyping in plants. Trends Plant Sci. 21, 110–124. doi: 10.1016/j.tplants.2015.10.015
Singh, A., Jones, S., Ganapathysubramanian, B., Sarkar, S., Mueller, D., Sandhu, K., et al. (2021). Challenges and opportunities in machine-augmented plant stress phenotyping. Trends Plant Sci. 26, 53–69. doi: 10.1016/j.tplants.2020.07.010
Singh, A. K., Ganapathysubramanian, B., Sarkar, S., and Singh, A. (2018). Deep learning for plant stress phenotyping: trends and future perspectives. Trends Plant Sci. 23, 883–898. doi: 10.1016/j.tplants.2018.07.004
Singh, A. K., Singh, A., Sarkar, S., Ganapathysubramanian, B., Schapaugh, W., Miguez, F. E., et al. (2021). “High-Throughput Phenotyping in Soybean,” in High-Throughput Crop Phenotyping (bll 129–163), eds J. Zhou and H. T. Nguyen (Cham: Springer International Publishing).
Singh, D. P., Singh, A. K., and Singh, A. (2021). “Chapter 25 - Breeding of crop ideotypes,” in Plant Breeding and Cultivar Development (bll 497–516), eds D. P. Singh, A. K. Singh, and A. Singh (Cambridge, MA: Academic Press).
Srayeddin, I., and Doussan, C. (2009). Estimation of the spatial variability of root water uptake of maize and sorghum at the field scale by electrical resistivity tomography. Plant Soil 319, 185–207.
Tan, K. H., and Nopamornbodi, V. (1979). Effect of different levels of humic acids on nutrient content and growth of corn (Zea mays L.). Plant Soil 51, 283–287.
Tang, W., Ji, Q., Huang, Y., Jiang, Z., Bao, M., Wang, H., et al. (2013). FAR-RED ELONGATED HYPOCOTYL3 and FAR-RED IMPAIRED RESPONSE1 transcription factors integrate light and abscisic acid signaling in Arabidopsis. Plant Physiol. 163, 857–866. doi: 10.1104/pp.113.224386
Tibbitts, T. W., and Langhans, R. W. (1993). “Controlled-environment studies,” in Photosynthesis and Production in a Changing Environment: A Field and Laboratory Manual (bll 65–78), eds D. O. Hall, J. M. O. Scurlock, H. R. Bolhàr-Nordenkampf, R. C. Leegood, and S. P. Long (Dordrecht: Springer).
Tibbs Cortes, L., Zhang, Z., and Yu, J. (2021). Status and prospects of genome-wide association studies in plants. Plant Genome 14:e20077. doi: 10.1002/tpg2.20077
Trachsel, S., Kaeppler, S. M., Brown, K. M., and Lynch, J. P. (2011). Shovelomics: high throughput phenotyping of maize (Zea mays L.) root architecture in the field. Plant Soil 341, 75–87.
Vinnari, M. (2008). The future of meat consumption — Expert views from Finland. Technol. Forecast. Soc. Change 75, 893–904.
Wang, L., Bai, P., Yuan, X., Chen, H., Wang, S., Chen, X., et al. (2018). Genetic diversity assessment of a set of introduced mung bean accessions (Vigna radiata L.). Crop J. 6, 207–213. doi: 10.1016/j.cj.2017.08.004
Wasson, A., Bischof, L., Zwart, A., and Watt, M. (2016). A portable fluorescence spectroscopy imaging system for automated root phenotyping in soil cores in the field. J. Exp. Bot. 67, 1033–1043. doi: 10.1093/jxb/erv570
White, P. J., George, T. S., Gregory, P. J., Bengough, A. G., Hallett, P. D., and McKenzie, B. M. (2013). Matching roots to their environment. Ann. Bot. 112, 207–222. doi: 10.1093/aob/mct123
Wild, F., Czerny, M., Janssen, A. M., Kole, A. P. W., and Domig, K. J. (2014). The evolution of a plant-based alternative to meat: from niche markets to widely accepted meat alternatives. Agro Food Ind. Hi Tech 25, 45–49.
Wright, S. (1965). The interpretation of population structure by f-statistics with special regard to systems of mating. Evolution 19, 395–420.
Xu, W., Cui, K., Xu, A., Nie, L., Huang, J., and Peng, S. (2015). Drought stress condition increases root to shoot ratio via alteration of carbohydrate partitioning and enzymatic activity in rice seedlings. Acta Physiol. Plant. 37:9.
Yang, N., Lu, Y., Yang, X., Huang, J., Zhou, Y., Ali, F., et al. (2014). Genome wide association studies using a new nonparametric model reveal the genetic architecture of 17 agronomic traits in an enlarged maize association panel. PLoS Genet. 10:e1004573. doi: 10.1371/journal.pgen.1004573
Ye, H., Roorkiwal, M., Valliyodan, B., Zhou, L., Chen, P., Varshney, R. K., et al. (2018). Genetic diversity of root system architecture in response to drought stress in grain legumes. J. Exp. Bot. 69, 3267–3277. doi: 10.1093/jxb/ery082
Yin, L., Zhang, H., Tang, Z., Xu, J., Yin, D., Zhang, Z., et al. (2021). rMVP: a memory-efficient, visualization-enhanced, and parallel-accelerated tool for genome-wide association study. Genomics Proteomics Bioinformatics. doi: 10.1016/j.gpb.2020.10.007 [Epub ahead of print].
Yu, H., Chen, X., Hong, Y.-Y., Wang, Y., Xu, P., Ke, S.-D., et al. (2008). Activated expression of an Arabidopsis HD-START protein confers drought tolerance with improved root system and reduced stomatal density. Plant Cell 20, 1134–1151. doi: 10.1105/tpc.108.058263
Yu, J., Pressoir, G., Briggs, W. H., Vroh Bi, I., Yamasaki, M., Doebley, J. F., et al. (2006). A unified mixed-model method for association mapping that accounts for multiple levels of relatedness. Nat. Genet. 38, 203–208. doi: 10.1038/ng1702
Yu, L., Chen, X., Wang, Z., Wang, S., Wang, Y., Zhu, Q., et al. (2013). Arabidopsis enhanced drought tolerance1/HOMEODOMAIN GLABROUS11 confers drought tolerance in transgenic rice without yield penalty. Plant Physiol. 162, 1378–1391. doi: 10.1104/pp.113.217596
Zhang, J., Naik, H. S., Assefa, T., Sarkar, S., Reddy, R. V. C., Singh, A., et al. (2017). Computer vision and machine learning for robust phenotyping in genome-wide studies. Sci. Rep. 7:44048. doi: 10.1038/srep44048
Zhang, J., Song, Q., Cregan, P. B., Nelson, R. L., Wang, X., Wu, J., et al. (2015). Genome-wide association study for flowering time, maturity dates and plant height in early maturing soybean (Glycine max) germplasm. BMC Genomics 16:217. doi: 10.1186/s12864-015-1441-4
Zheng, X., Levine, D., Shen, J., Gogarten, S. M., Laurie, C., and Weir, B. S. (2012). A high-performance computing toolset for relatedness and principal component analysis of SNP data. Bioinformatics 28, 3326–3328. doi: 10.1093/bioinformatics/bts606
Zheng, Z., Hey, S., Jubery, T., Liu, H., Yang, Y., Coffey, L., et al. (2020). Shared Genetic Control of Root System Architecture between Zea mays and Sorghum bicolor. Plant Physiol. 182, 977–991. doi: 10.1104/pp.19.00752
Zhiming, Y., Bo, K., Xiaowei, H., Shaolei, L., Youhuang, B., Wona, D., et al. (2011). Root hair-specific expansins modulate root hair elongation in rice. Plant J. 66, 725–734. doi: 10.1111/j.1365-313X.2011.04533.x
Keywords: root system architecture, GWAS, high throughput phenotyping, phenomics, pulses
Citation: Chiteri KO, Jubery TZ, Dutta S, Ganapathysubramanian B, Cannon S and Singh A (2022) Dissecting the Root Phenotypic and Genotypic Variability of the Iowa Mung Bean Diversity Panel. Front. Plant Sci. 12:808001. doi: 10.3389/fpls.2021.808001
Received: 02 November 2021; Accepted: 06 December 2021;
Published: 27 January 2022.
Edited by:
Rodomiro Ortiz, Swedish University of Agricultural Sciences, SwedenReviewed by:
Santosh Kumar Gupta, National Institute of Plant Genome Research (NIPGR), IndiaKarl Peter Pauls, University of Guelph, Canada
Copyright © 2022 Chiteri, Jubery, Dutta, Ganapathysubramanian, Cannon and Singh. This is an open-access article distributed under the terms of the Creative Commons Attribution License (CC BY). The use, distribution or reproduction in other forums is permitted, provided the original author(s) and the copyright owner(s) are credited and that the original publication in this journal is cited, in accordance with accepted academic practice. No use, distribution or reproduction is permitted which does not comply with these terms.
*Correspondence: Arti Singh, YXJ0aUBpYXN0YXRlLmVkdQ==