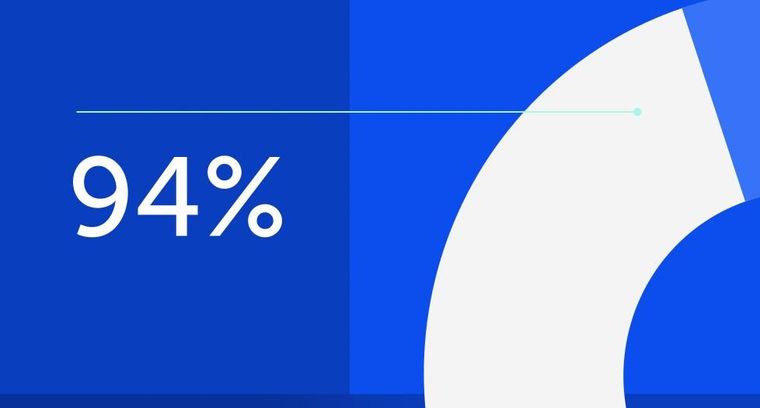
94% of researchers rate our articles as excellent or good
Learn more about the work of our research integrity team to safeguard the quality of each article we publish.
Find out more
ORIGINAL RESEARCH article
Front. Plant Sci., 16 December 2021
Sec. Plant Breeding
Volume 12 - 2021 | https://doi.org/10.3389/fpls.2021.753755
This article is part of the Research TopicTrends in Cotton Breeding: Meeting the Challenges of the 21st CenturyView all 22 articles
Upland cotton (Gossypium hirsutum) is widely planted around the world for its natural fiber, and producing high-quality fiber is essential for the textile industry. CCRI70 is a hybrid cotton plant harboring superior yield and fiber quality, whose recombinant inbred line (RIL) population was developed from two upland cotton varieties (sGK156 and 901-001) and were used here to investigate the source of high-quality related alleles. Based on the material of the whole population, a high-density genetic map was constructed using specific locus-amplified fragment sequencing (SLAF-seq). It contained 24,425 single nucleotide polymorphism (SNP) markers, spanning a distance of 4,850.47 centimorgans (cM) over 26 chromosomes with an average marker interval of 0.20 cM. In evaluating three fiber quality traits in nine environments to detect multiple environments stable quantitative trait loci (QTLs), we found 289 QTLs, of which 36 of them were stable QTLs and 18 were novel. Based on the transcriptome analysis for two parents and two RILs, 24,941 unique differentially expressed genes (DEGs) were identified, 473 of which were promising genes. For the fiber strength (FS) QTLs, 320 DEGs were identified, suggesting that pectin synthesis, phenylpropanoid biosynthesis, and plant hormone signaling pathways could influence FS, and several transcription factors may regulate fiber development, such as GAE6, C4H, OMT1, AFR18, EIN3, bZIP44, and GAI. Notably, the marker D13_56413025 in qFS-chr18-4 provides a potential basis for enhancing fiber quality of upland cotton via marker-assisted breeding and gene cloning of important fiber quality traits.
Upland cotton (Gossypium hirsutum L., 2n = 52) is a widely planted cash crop providing natural fiber. Due to its excellent environmental adaptability and yield, among the four cultivated tetraploid species, it is G. hirsutum that contributes almost 95% of the harvested cotton production (Yoo and Wendel, 2014). Given the increasing demand for high-quality fiber from the textile industry and the role of multigenes’ contribution and environmental factors, it is the goal of cotton breeders worldwide to develop new upland cotton varieties simultaneously featuring superior fiber quality and high yield.
Genome sequencing studies of G. raimondii (Paterson et al., 2012; Wang K. et al., 2012), G. arboreum (Li et al., 2014; Du et al., 2018; Huang et al., 2020), G. barbadense (Liu X. et al., 2015; Yuan et al., 2015; Hu et al., 2019), and G. hirsutum (Li et al., 2015; Zhang T. et al., 2015; Hu et al., 2019; Wang M. et al., 2019; Huang et al., 2020) using next-generation and third generation sequencing technology have provided a solid basis for constructing the genetic map of cotton and understanding further its functional genomics. Benefiting from rapid progress in sequencing and DNA marker technologies, marker-assisted breeding has become one of the most efficient tools to help breeders globally improve agronomic traits and shorten the breeding cycle in multiple key crops. Recently, specific locus-amplified fragment sequencing (SLAF-seq) and genotyping-by-sequencing have merged as efficient tools largely applied to upland cotton for exploring its genotypic variants. SLAF-seq has several distinguishing advantages: (i) deep sequencing to ensure genotyping accuracy; (ii) reduced representation strategy to reduce sequencing costs; and (iii) a double barcode system for large populations (Sun et al., 2013). Many cotton genetic linkage maps have been constructed and quantitative trait loci (QTL) identified using different kinds of populations and DNA markers (Ulloa et al., 2002, 2005; Rong et al., 2004; Chen et al., 2009; Sun et al., 2012; Zhang et al., 2012, 2016; Zhou et al., 2017; Zhang et al., 2020; Hulse-Kemp et al., 2015; Shi et al., 2015; Wang H. et al., 2015; Wang Y. et al., 2015; Yuan et al., 2015; Zhang Z. et al., 2015; Li et al., 2016; Liu et al., 2017; Qi et al., 2017; Chandnani et al., 2018; Diouf et al., 2018; Zou et al., 2018; Wang et al., 2021). Since the fiber quality is a quantitative trait and one controlled by multiple genes, QTLs might cumulatively contribute to its phenotypic variation, which provides a reasonable way to improve fiber quality via marker-assisted selection (MAS) (Paterson et al., 2003; Rong et al., 2004; Zhang et al., 2012; Wang H. et al., 2015; Wang Y. et al., 2015).
Based on an assessment of previous research, fiber development could be classified into four stages: initiation [−3 to 3 days postanthesis (DPA)], elongation (3–23 DPA), secondary wall biosynthesis (20–40 DPA), and maturity (40–50 DPA) (Basra and Malik, 1984; Kim and Triplett, 2001; Lee et al., 2006, 2007; Haigler et al., 2012; Mathangadeera et al., 2020; Patel et al., 2020). Initiation, elongation, and secondary wall biosynthesis stages will determine fiber quality traits, namely fiber length (FL), fiber strength (FS), and fiber micronaire (FM), respectively. Transcriptome sequencing, better known as RNA-Seq, which takes full advantage of gene expression and transcriptional regulation, has proven itself a robust and suitable procedure for analyzing the transcriptome profile during various stages of fiber development (Applequist et al., 2001; Gilbert et al., 2014; Yoo and Wendel, 2014; Islam et al., 2016; Li P.-t. et al., 2017; Li X. et al., 2017; Lu et al., 2017; Zou et al., 2019; Jiang et al., 2021).
The hybrid variety “CCRI70”, a nationally approved variety in China released in 2008, is capable of good yield and has high fiber quality, whose recombinant inbred line (RIL) population consisting of 250 individuals was developed to investigate the source of high-quality related alleles for further upland cotton breeding (Zou et al., 2018; Deng et al., 2019). In this study, the CCRI70 F8:9 RIL population was used to evaluate fiber quality performance in nine environments and to construct a genetic linkage map. The whole-genome-based high-density genetic map contained 24,425 single nucleotide polymorphism (SNP) markers spanning a distance of 4,850.47 cM. Meanwhile, 289 QTLs for the three fiber quality traits and seven QTL clusters were identified. Accompanying the RNA-Seq analysis done for the whole process of fiber development, differentially expressed genes (DEGs) in those obtained QTLs and clusters were also identified, which provides new and timely insights into the genetic basis of fiber development and fiber quality traits.
The 250 individual F8:9 RILs were developed from the hybrid cotton variety CCRI70 whose parents are “sGK156” (P1) and “901-001” (P2); this population was developed from 2011 onward at the experimental farm of the Institute of Cotton Research, Chinese Academy of Agricultural Sciences (CAAS), in Anyang, Henan Province. The CCRI70 (F1) was obtained and planted in Sanya, where the F2 seeds were harvested. Shuttle breeding was applied to further develop the population during 2012–2016 between Anyang and Sanya. Full details of the procedure used to generate the RIL populations were reported already (Zou et al., 2018).
In 2017, the RIL population was planted in double-row plots 5-m long, 80 cm apart, and with a 25-cm spacing between adjacent plants. “Lumianyan28” was also planted, as a control, for every 19 RILs at the Anyang experimental station of Institute of Cotton Research of CAAS, in Henan Province. Leaf samples of the RIL population were collected for sequencing. The P1and P2, and the two RILs, MBZ70-053 (L1) and MBZ70-236 (L2), known to differ in their fiber quality performance were designated for RNA sequencing. Concerning the above four types of plant materials, P2 and L1 performed high-fiber quality, and P1 and L2 showed low-fiber quality in terms of their FL and FS. The day of anthesis was marked as 0 DPA, and flowers were marked by hanging labels with the flowering date recorded. Cotton bolls were collected in the morning at 5, 10, 15, 20, 25, and 30 DPA with three biological replicates, with at least five bolls collected in each replicate. The fiber samples were collected using sterilized medical scalpel and tweezers. Then all the samples were frozen in liquid nitrogen and stored at −80°C for RNA-Seq analysis.
The 250 CCRI70 RILs and the two parents were planted in at least two replications in nine environments across 2 years and six locations, including the F5:7 and F5:8 families, reported previously (Zou et al., 2018). In 2015, the RIL population was planted in Anyang of Henan Province (15AY, 36°10′N, 114°35′E), Linqing of Shandong Province (15LQ, 36°68′N, 115°72′E), and Alaer of the Xinjiang Autonomous Region (15ALE, 40°22′N, 80°30′E). In 2016, the population was planted in Anyang (16AY), Linqing (16LQ), Alaer (16ALE), Changde of Hunan Province (16CD, 29°2′N, 111°41′E), Shihezi (16SHZ, 44°27′N, 85°94′E), and Kuerle (16KEL, 41°68′N, 86°06′E) of the Xinjiang Autonomous Region.
Thirty mature, fully opened bolls from every plot were harvested to test their fiber quality (i.e., FL, FS, and FM traits) by using an HVI1000 (Uster Technologies, Switzerland) with the HVICC Calibration at the Cotton Quality Supervision, Inspection, and Testing Center, Ministry of Agriculture, Anyang, China (Zhang Z. et al., 2015). The descriptive statistics of these fiber quality traits were calculated using SPSS 20.0 and Microsoft Excel 2010 software. IciMapping 4.1 was used to analyze the traits’ heritability (Li et al., 2007; Meng et al., 2015).
Leaf samples of the RIL population were used to extract genomic DNAs; this is done using the TaKaRa MiniBEST Plant Genomic DNA Extraction kit (TaKaRa, Dalian). According to the pilot experiment and the preexperiment in silico simulation, SLAF libraries for 250 RILs were constructed by using two endonucleases in combination, HaeIII and SspI (New England Biolabs, NEB, United States), to digest the genomic DNA (Zhang et al., 2017). The details and procedures of the SLAF-seq strategy are described in Zhang J. et al. (2015). Paired-end (PE) sequencing libraries of the parents with insert sizes ranging from 200 to 500 bp were generated according to the manufacturer’s instructions (Illumina, San Diego, CA, United States). Likewise, following the manufacturer’s recommendations, all PE sequencing (each 125 bp fragment of the 250 RILs and each 150 bp fragment of parents) were conducted on the Illumina Hi-Seq 2500 system (Illumina, San Diego, CA, United States).
The identification and genotyping of SNP markers were implemented using the procedures of Sun et al. (2013) and Zhang J. et al. (2015). After removing the adapters and filtering out any low quality reads (i.e., quality score < 20e), the reads belonging to each RIL were recognized by their unique duplex barcode sequences (Zhang Z. et al., 2015). The clean data set of the population was then mapped onto the G. hirsutum reference genome (Hu et al., 2019) using BWA software (Li and Durbin, 2009). Reads mapping onto the same position with a more than 95% shared identity were recorded as a single locus. GATK (McKenna et al., 2010) was used to process the bam files, and these were then filtered according to GATK’s recommended parameters. The SNP markers were genotyped under the sequencing depth of parents with more than 10-fold and individuals above 1-fold. Before constructing the genetic linkage map, SNP markers underwent further filtering by removing those markers with no position or at least two positions; those which showed no polymorphism between parents; those that were heterozygous in either parent; and those with a missing rate above 50%, or with segregation distortion having a p-value < 0.001 for the chi-square test (Zhang Z. et al., 2015).
After genotyping and filtering the SNP marker, the genetic linkage map was built using MSTmap software and referring to the reference genome (Hu et al., 2019). To be considered for linkage map construction, markers had to have a LOD (log of odds) threshold between 4 and 20 (Wu et al., 2008). Next, SNP markers in the linkage group from the same chromosome were merged together, and these SNP markers per chromosome were then regulated again by MSTmap.
The QTLs for the FS, FL, and FM traits in the nine environments were identified using WinQTLCart 2.5 software (Wang et al., 2007) and applying the composite interval mapping (CIM) method (Zeng, 1994). LOD thresholds were determined with 1,000 permutations at a 1-cM walk (p = 0.05) (Churchill and Doerge, 1994), and QTLs designated so that their LOD scores were above the threshold. The additive QTL was named as “q” with the trait name, followed by the corresponding chromosome number and QTL number according to the genetic position. The QTLs that could be detected in no less than three environments were recognized as being stable QTLs (Sun et al., 2012). Places on the genetic map where confidence intervals overlapped stable QTLs for different traits were considered to be QTL clusters (Zhang et al., 2020), in which genome fragments affect more than one trait. Based on the physical position of markers for stable QTLs and QTL clusters, according to their annotation (Hu et al., 2019), those genes located internally were deemed “promising genes” (except the QTLs longer than 10 MB). QTL clusters were visualized using MapChart 2.2.
To compare our stable QTLs with those found in previous studies, the CottonQTLdb database1 was searched for using the physical positions of the border markers of QTLs (Rong et al., 2007; Lacape et al., 2010; Said et al., 2013, 2015; Fang et al., 2014). As we used the newly published reference genome, we mapped our QTL results on the former (Zhang T. et al., 2015) using Bowtie software (Langmead et al., 2009). Locating the physical confidence intervals of previous QTLs based on simple sequence repeat (SSR) markers using CottonFGD database,2 via comparison with the CottonQTLdb database, the former study of CCRI70, the SNP loci of genome-wide association studies (GWASs) (Fang et al., 2017a,b,c; Huang et al., 2017; Ma et al., 2018), and the QTLs with overlapping confidence intervals on the physical map were considered as the same QTL.
Total RNA from each sample was extracted following the manufacturer’s protocol with the RNAprep Pure Plant Kit (Polysaccharides & Polyphenolics-rich, Tiangen, Beijing, China). The quantification and purity of the RNA were respectively assessed by a NanoDrop 2000 spectrophotometer (Thermo Fisher Scientific, Waltham, MA, United States) and a NanoPhotometer spectrophotometer (IMPLEN, CA, United States). The RNA integrity was confirmed using the RNA Nano 6000 Assay Kit of the Bioanalyzer 2100 system (Agilent Technologies, CA, United States). Next, according to the manufacturer’s recommendations, 2 μg RNA per sample was used for the transcriptome library construction, implemented with the Illumina TruSeq™ RNA Sample Preparation Kit (Illumina, San Diego, CA, United States). In total, 72 libraries were separately sequenced using Illumina Novaseq 6000 (BerryGenomics Co., Ltd., Beijing, China) for the two RILs and the two parents at six developing stages (5, 10, 15, 20, 25, and 30 DPA) with three biological replicates (4 × 6 × 3 = 72).
Trimmomatic software was then utilized to process all the generated raw data in the Fastq format (Bolger et al., 2014). After removing those reads having the adapter, poly-N (N ≥ 10%), and low-quality reads (more than half of the bases with a Phred quality ≤ 3), the clean data set was finally generated. Meanwhile, the GC percentage and Q30 were calculated to evaluate the quality of the clean data. HISAT2 v2.1.0 (Pertea et al., 2016) under its default parameters was used to carry out the sequence alignments. The fragments per kilobase of exon per million reads (FPKM) values of genes were quantified by StringTie v1.3.5 (Pertea et al., 2015) and these were then subjected to a Pearson correlation analysis to reveal their correlation coefficients. Those samples with a correlation coefficient <0.8 between the biological replicates were removed from the dataset. Bcftools 1.8 and snpEff 5.0, each set to its recommended parameters, were used to respectively identify and annotate the SNPs in the RNA-Seq data (Cingolani et al., 2012).
Based on the gene count number of each sample, the DESeq2 package for R was run to identify the DEGs (Love et al., 2014), for which the dual screening criteria were an FDR value < 0.05, and | log2FoldChange| > 1 between each pairwise comparison. The DEGs were obtained from vertical comparisons in the superior trait group (i.e., high fiber quality group, L1 and P2) vis-à-vis the inferior trait group (i.e., low fiber quality group, L2 and P1) at six stages, and also from horizontal comparisons within each group between the different developmental stages.
To predict the gene function and related pathways of the promising genes and DEGs detected during the course of fiber development, the BLASTX program, GO databases, and KAAS3 based on Kyoto Encyclopedia of Genes and Genomes (KEGG) were all employed (Altschul et al., 1990; Wu et al., 2006; Moriya et al., 2007; Xie et al., 2011). To identify the transcription factors of the promising genes, the PlantTFDB4 resource was queried (Jin et al., 2014, 2015, 2016; Tian et al., 2020).
A weighted gene co-expression network analysis (WGCNA) was performed and WGCNA R package was used to identify modules and hub (or highly correlated) genes that were strongly associated with the fiber development stages (Langfelder and Horvath, 2008). Those DEGs, with a coefficient (>0.6) within each sample, were subsequently clustered into different modules (Pei et al., 2017). The hub genes were selected according to the module membership (KME) values.
The phenotypic data for the CCRI70 RIL population were selected and evaluated in nine environments during 2015 and 2016 (Figures 1A–C). The range in the difference between the two parents in nine environments for the FS, FL, and FM traits was 0.55–5.25 cN/Tex, 0.05–2.65 mm, and 0–0.8 units, respectively (Supplementary Table 1), with significant differences found for FS and FL. In addition, all three traits for fiber quality had approximately normal distributions whose absolute skewness values did not exceed 0.5, and they were characterized by transgressive segregation with respect to parental performance in all nine environments (Figures 1D–F). L1 and L2 respectively had excellent and poor performance in terms of fiber quality in all nine testing environments (Supplementary Table 1). When calculated and compared across the nine environments, the 15LQ site had the highest heritability (no less than 75% for FS, FL, and FM), whereas 16ALE had the lowest (no more than 60% for FS, FL, and FM), a disparity perhaps arising from environmental factors. Apart from 16ALE, in the eight environments, the heritability for FS, FL, and FM traits were 60.8–80.1%, 65.29–77.6%, and 66.0–78.2%, respectively (Supplementary Table 1). The two-way analysis of variance (ANOVA) revealed highly significant effects of genotype (G), the environment (E), and the interaction between genotype and the environment (G × E) on all three traits. Importantly, genotype explained the most variance while the G × E interaction was responsible for more than 25% of the variation in each of the three traits (Table 1).
Figure 1. The phenotypic data analysis of the parents and two RILs in nine environments. The phenotypic data analysis of the parents and two RILs for (A) fiber strength, (B) fiber length, and (C) micronaire. Normal distribution analysis of (D) fiber strength, (E) fiber length, and (F) micronaire.
After library construction, sequencing, and filtering, 1.1 TB of clean data was obtained, for which the Q30 bases were 94.76% and its mapping ratio was 99.51%. Specifically, 82.01 GB per each parent and 3.72 GB per each RIL were obtained; comparing both to the upland cotton reference genome (2.14 GB), indicating that the sequencing depth of our study was 35.41-fold for each parent and 1.61-fold per progeny, respectively (Supplementary Table 2).
A total of 1,943,288 SNPs were detected in sGK-156 whereas 2,073,924 SNPs were identified in 901-001, whose homozygosity SNP markers amounted to 8,11,619 and 8,82,936, respectively. After filtering these markers, 24,425 SNP markers were retained for the genetic map’s construction.
A genetic map of 24,425 SNP markers and 4850.47 cM total distance over 26 chromosomes was built, having an average marker interval of 0.20 cM, wherein the A subgenome (At) contained 14,220 SNP markers and 2564.93 cM and the D subgenome (Dt) contained 10,205 SNP markers and 2285.54 cM (Figures 2A,B). The longest chromosome was chr06, which harbored 313 SNP markers with a length of 227.05 cM; conversely, the shortest was chr23 with 642 markers and a length of 151.39 cM. Using the Spearman coefficient for estimating the correlation with the physical mapping, except for chr08, the absolute Spearman values were all greater than 0.9 for all other chromosomes (Table 2).
Figure 2. Detailed information about the genetic map. (A) The distribution of SLAF-SNP markers in the genetic map. (B) Collinearity analysis of markers between the physical map and genetic map.
Based on the high-density linkage map and phenotype data for the nine environments, 289 QTLs for fiber quality traits were detected on the whole genome. Specifically, 36 of them were stable QTLs, of which 15 were located on At and another 21 were detected on Dt. The stable QTLs were mainly distributed on chromosomes 6, 10, 14, 16, 18, 24, and 25 (Supplementary Tables 3–5). Concerning the sign of QTL’s additive effect, a positive additive effect meant the high-quality related alleles originated from sGK-156, whereas negative meant the alleles were from 901-001.
There were 108 QTLs detected for FS, 17 of them (At was five and Dt was 12) were stable QTLs and mainly distributed on chromosomes 6, 14, 16, 18, and 24 (Figures 3A,D). Twelve of the 17 QTLs had negative additive effects, where the sGK156 alleles reduced the FS. In at least five environments, qFS-chr18-1, qFS-chr16-4, and qFS-chr10-6 could be detected with negative additive effects.
Figure 3. Detailed information about the QTLs. Total QTLs and stable QTLs of (A) fiber strength, (B) fiber length, and (C) micronaire distribution on the 26 chromosomes. (D) Fiber strength stable QTLs distribution on the 11 chromosomes. In (E) chr10 and (F) chr24, the FS and FL stable QTLs distribution in present and previous works.
Ninety-six QTLs were detected for FL, of which 11 (At had seven and Dt had four) were stable QTLs and mainly distributed on chromosome 5, 6, 10, 14, 24, and 25 (Figure 3B). Eight of the 11 QTLs had negative effects, where sGK156 alleles decreased the FL. qFL-chr05-2, qFL-chr10-6, and qFL-chr24-1 could be detected in at least four environments with positive additive effects. Likewise, qFL-chr05-3, qFL-chr10-5, and qFL-chr25-2 were detectable in four environments, albeit with negative additive effects.
In addition, 85 QTLs were identified for FM, among which eight (At had three and Dt had five) were stable QTLs (Figure 3C) and distributed on chromosome 2, 3, 8, 17, 18, 21, 22, and 25. Six of the eight QTLs had positive additive effects, whereby sGK156 increased the FM. In six environments, qFM-chr03-2 could be detected with negative additive effects, whereas qFM-chr08-3 and qFM-chr22-1 were detectable in four environments with positive additive effects.
According to the overlapping of confidence intervals of stable QTLs for the different traits, seven QTL clusters (qCl-chr07-1, qCl-chr10-1, qCl-chr14-1, qCl-chr16-1, qCl-chr17-1, qCl-chr24-1, qCl-chr25-1) were identified on seven chromosomes (7, 10, 14, 16, 17, 24 and 25) (Supplementary Table 6), of which two were positioned on At and another five on Dt. In five QTL clusters (qCl-chr07-1, qCl-chr10-1, qCl-chr14-1, qCl-chr16-1, qCl-chr24-1), the QTLs for FS and FL traits shared the same direction of additive effects. Besides, additive effects from qCl-chr17-1 were in opposing directions for FS and FM, and likewise for qCl-chr25-1 with respect to the FL and FM traits. These results suggested that the clusters could improve FS and FL simultaneously yet decrease the FM.
Comparing these stable QTLs with earlier published work for CCRI70 (Zou et al., 2018) and other research, 18 stable QTLs in the current study were novel and another 18 were either the same or shared overlapping physical confidence intervals with some in previous studies. Of these, 11 QTLs were matched those in the cotton QTL database, one was the same as found in the other CCRI70 study, two more partially overlapped with those reported in Zhang et al. (2020), and four more shared some overlap with previous GWAS results (Supplementary Table 7 and Figures 3E,F).
To reveal the patterns of gene expression during fiber developmental stages, transcriptome sequencing was conducted using fibers sampled at 5, 10, 15, 20, 25, and 30 DPA (Supplementary Table 8). As a result, 2,760.21 million clean reads were obtained. The raw data have been submitted to the National Genomics Data Center (accession numbers CRA002982 and CRA004731). The average number of clean reads, GC content, and Q30 value were, respectively, 38.34 million, 44.92%, and 94.93% for each sample, implying that the quality of the RNA-Seq data was reliable. After filtering out four low-correlation samples, the FPKM values were calculated for the biological replicates, and their percentages in the categories of 0.5 ≤ FPKM < 5, 5 ≤ FPKM ≤ 100, and FPKM > 100, respectively were 23.50, 12.24, and 0.78% on average (Figure 4A). Overall, 3,44,844 genetic variants were obtained on the 26 chromosomes referring to TM-1 when using the SNPEff program. As a result, 1,32,691; 1,85,414; 92,620; and 63,865 variants were detected in the 5’ UTR, 3’ UTR, exon and intron regions, respectively, where 52,097 were missense variants (Supplementary Table 9). Furthermore, via vertical comparisons, 238/110, 118/217, 469/638, 185/535, 677/2474, and 98/203 up-/downregulated DEGs relative to the inferior group for fiber traits were identified (Figure 4B). Meanwhile, in the horizontal comparisons, 24,266 unique DEGs were distinguished between different developmental stages, with 24,941 unique DEGs obtained overall. At the six developmental time points, four genes (GH_A01G0307, GH_D02G0703, GH_D03G0833, and GH_D05G1628) were significantly differentially expressed between two groups among all six stages. Meanwhile, 13 and 16 genes were expressed differently in five and four stages, respectively (Figure 4C). Detailed information for the FPKM of DEGs at different developmental stages, including their log2FoldChangeand FDR values, whether they were up- or downregulated, and also their respective annotations can be found in Supplementary Table 10.
Figure 4. RNA-seq analysis statistics. (A) Statistics for transcript levels of each sample at each development stage, the numbers of expressed genes were divided by 0.5 < FPKM < 5, 5 < FPKM < 100, and FPKM > 100; (B) vertical and horizontal comparisons between different groups (elite group relative to the poor group) and stages; (C) the upset diagram showed the same and different DEGs identified at six developmental stages.
Weighted gene co-expression network analysis was performed using 5,378 DEGs identified via the vertical comparisons. The topology overlap matrix was built with the hierarchical clustering method, and the dynamic tree cut modules, in which the modules shared high correlation (Supplementary Figure 1A). After merging the analogous expression patterns, 11 modules were finally identified (Supplementary Figure 1B). Among these, two modules (blue and brown) were specifically associated with high-quality groups at 5 and 25 DPA, when the fiber was respectively in the elongation and secondary wall biosynthesis stages. The pink module was specifically associated with low-quality groups at 30 DPA.
In the WGCNA, KME is a value that describes the eigengene connectivity. In this study, 22 hub genes were obtained with the KME values >0.94 (Supplementary Table 11). In the brown module, which was strongly associated with the high-quality group during fiber elongation, the hub genes were annotated as a disproportionating enzyme, CP12 domain-containing protein 3, cell elongation protein/DWARF1/DIMINUTO (DIM), 2-cysteine peroxiredoxin B, glyoxalase 2-4, nuclear-encoded CLP protease P7, and glucose-6-phosphate/phosphate translocator-related. GH_D13G0256 was annotated as DRF1 in Arabidopsis thaliana. It is involved in brassinosteroid biosynthesis, converting 24-methylenecholesterol to campesterol (Du and Poovaiah, 2005). Accordingly, DRF1 loss-of-function mutants showed typical BR-deficient phenotypes, whereas DWF1 overexpression enhanced growth and development in A. thaliana (Youn et al., 2018). In upland cotton, brassinosteroid was reported to play a significant role in fiber elongation as well (Yang et al., 2014; Tian and Zhang, 2021). In the blue module, the hub genes strongly associated with the high-quality group during secondary wall biosynthesis were annotated as B12D protein, sucrose-6F-phosphate phosphohydrolase family protein, calmodulin-domain protein kinase 9, RNA polymerase Rpb6, shaggy-like protein kinase 41, FK506-binding-like protein, staurosporin, and temperature-sensitive 3-like b and small ubiquitin-like modifier 1. Both GH_A07G0466 and GH_D07G0468 were identified as B12D proteins and separately distributed on At and Dt subgenomes. Similarly, GH_A12G0434 and GH_D12G0446 were annotated as shaggy-like protein kinase 41 and distributed on chromosomes A12 and D12, respectively.
From the 36 stable QTLs, 1,476 promising genes were distinguished from 29 QTLs, whereas no genes were identified from the three QTLs (i.e., qFS-chr07-2, qFL-chr05-3, and qFM-chr25-1), with the narrow confidence intervals, and another four QTLs (qFS-chr14-3, qFL-chr07-3, qFL-chr25-2, and qFM-chr08-3) more than 20 MB in size on the physical map. Among those 1,476 unique promising genes, 1,005 of them were for the FS trait, 412 were for the FL trait, and 241 were for the FM trait (Supplementary Table 12). Among the stable QTLs, qFS-chr17-2 harbored the most promising genes, 237, and six QTLs (qFM-chr02-1, qFM-chr22-1, qFS-chr25-10, qFS-chr16-6, qFS-chr06-3, and qFL-chr24-1) contained no more than 20 such genes. The qFS-chr18-1 and qFL-chr24-1, which could be detected in most environments, contained 77 and 20 promising genes, respectively. Among the seven QTL clusters where 182 promising genes were identified, qCl-chr10-1 had the most genes, with 54, whereas two clusters (qCl-chr17-1 and qCl-chr24-1) had less than 20 each (Supplementary Figure 2).
The 1,476 promising genes were annotated with 4,109 GO terms. To the categories of biological process, cellular component, and molecular function belonged 2,602; 525; and 982 GO terms, respectively. In the three categories, 157, 82, and 87 genes were respectively enriched in terms of “regulation of transcription, “DNA-templated”, “cell wall”, and “sequence-specific DNA binding” (Supplementary Table 13).
Of the 1,476 promising genes, 834 were expressed (FPKM > 0.5), of which 246 were highly expressed (i.e., FPKM > 10). Comparing these promising genes with DEGs found revealed that 473 were expressed differentially during the process of fiber development, consisting of 320, 120, and 83 DEGs for FS, FL, and FM, respectively (Supplementary Table 9). Applying the KAAS for their pathway enrichment analysis, 473 differentially expressed promising genes were enriched in 130 pathways, these mainly for glycolysis/gluconeogenesis, protein processing in endoplasmic reticulum, amino sugar and nucleotide sugar metabolism, endocytosis, cysteine and methionine metabolism, pyruvate metabolism, phenylpropanoid biosynthesis, and plant-pathogen interaction pathway (Supplementary Table 13).
When deriving a genetic map, it has often been constructed with restriction fragment length polymorphism (RFLP) markers, SSR markers, and SNP markers with gene chip and sequencing technologies (Ulloa et al., 2002, 2005; Rong et al., 2004; Chen et al., 2009; Sun et al., 2012; Wang P. et al., 2012; Zhang et al., 2012, 2016; Zhou et al., 2017; Zhang et al., 2020; Hulse-Kemp et al., 2015; Shi et al., 2015; Wang H. et al., 2015; Wang Y. et al., 2015; Yuan et al., 2015; Zhang Z. et al., 2015; Li et al., 2016; Liu et al., 2017; Qi et al., 2017; Chandnani et al., 2018; Diouf et al., 2018; Tan et al., 2018; Zou et al., 2018; Shi et al., 2020; Wang et al., 2021). The SNP marker development from next generation sequencing technology and genotyping with a reference genome provides a high-density molecular marker and unprecedented accuracy. In this study, a high-density genetic map containing 24,425 SNP markers and spanning a genetic distance of 4850.47 cM was constructed, wherein the high-density markers almost covered the entire genome of upland cotton. There were 75 just gaps (>5 cM) on the 26 chromosomes, and 38 of them were on chr02, chr07, chr21, and chr22, these being the chromosomes with the four fewest markers. In future work, we will consider increasing the marker density of these four chromosomes. Nonetheless, this genetic map provides a valuable new tool for studying QTLs and potential candidate gene identification and also informing cotton molecular breeding programs of upland cotton.
Fiber quality traits are quantitative plant traits that are sensitive to the environment and other factors; so detecting QTLs in multiple environments was necessary to be done here, especially as it could improve accuracy. Some QTLs could be detected only in specific environments, whereas others that may be detected in multiple environments and across generations are considered as stable QTLs. In our study, the planting locations’ selection in experimental design took into account both geographic and temporal replicates. For example, Anyang and Linqing are located in the Yellow River basin while Alaer, Kuerle, and Shihezi are in the Xinjiang Autonomous Region. Meanwhile, Alaer, Anyang, and Linqing had been arranged for 2 years. It was our goal to detect the stable QTLs of upland cotton across multiple environments. Notably, of the36 stable QTLs, qFL-chr11-2 and qFM-chr02-1 were specifically detected in the Yellow River basin, whereas qFM-chr17-2 was only identified in the Xinjiang Autonomous Region, and hence it might be environment-specific QTLs. Meanwhile, the fact that qFS-chr18-1, qFL-chr24-1, and qFM-chr03-2 were detected in at least six environments is strong evidence suggesting that genetic factors may play a dominant role in fiber quality traits.
Among the 36 stable QTLs found in this study, 18 had been identified before (eight for FS, six for FL, and four for FM), but the other 18 were novel (nine for FS, five for FL, and four for FM). Together, they provide a sound basis for further functional characterization and upland cotton marker-assisted breeding programs. Our work differs from previous research, in that the CCRI70 RIL population is a breeding population developed from two upland cotton cultivars, which themselves are breeding material rather than germplasm material. Moreover, CCRI70 (F1) was the first nationally approved hybrid cotton capable of a high yield of superior fiber quality. The goal of this study was to detect QTLs and identify promising genes related to key fiber quality traits, which could be used to reveal the parental source of fiber quality related alleles. Compared with previous QTL studies of fiber quality, few fiber quality related QTLs, especially FS-related QTLs, were identified on chr18 (D13) (Diouf et al., 2018; Gu et al., 2020; Wang et al., 2020; Zhang et al., 2020). However, we detected two stable QTLs related with FS and one related with FM on that chromosome, and the qFS-chr18-1 was detected in eight of the nine testing environments. Next, focusing on the novel QTLs, we combined DNA sequencing and RNA-Seq data to explore the molecular mechanisms of fiber quality traits and genetic variants’ distribution in the QTLs among the high- and low-fiber quality group. In qFS-chr18-4 (Figure 5A), 901-001 increased the FS, for which 19 SNPs were identified from DNA sequencing data and 10 were detected from RNA-Seq data, which performed differently between the two groups. The 10 SNPs were located in the mRNA of five promising genes (Figure 5B); four led to missense variants and six were detectable on the 5’/3’ UTR. Besides, the high- and low-fiber quality groups we studied had some differences and also same genotypes with TM-1 (Hu et al., 2019), which suggests the variants could increase FS. By scanning the SNPs in the natural population (Zhang et al., 2020) and reanalyzing the genotypic data using the newest reference genome (Hu et al., 2019) for marker D13_56413025, the phenotypes of the materials having the same and different allele with 901-001 showed significant differences at 16ALE and 17KEL environments, with p-values < 0.05. In 16ALE, the FS phenotypes of materials with T spanned 25.60-31.70 cN/tex with an average of 29.48 cN/tex, while the phenotypes with C were 22.70–32.80 cN/tex with an average of 26.08 cN/tex. Meanwhile, in 17KEL, the range of the holding T allele was 27.20–37.00 cN/tex, averaging 31.28 cN/tex, whereas the range of C allele was 24.50–33.60 cN/tex with an average of 27.86 cN/tex. The FS increased from 3.40 to 3.41 cN/tex (Figure 5C). The marker D13_56413025 also led to missense variant in the highly expressed promising gene GH_D13G1887 (FPKM > 10), making it worthy of further study and providing information to better understand the underlying genetic mechanisms, as well as a reference for improving the upland cotton fiber quality.
Figure 5. Detailed genetic variants distribution in qFS-18-4. (A) The distribution of qFS-18-4 in chr18. (B) Ten different SNPs between high- and low-group distributed in the QTL. The SNP leading to missense variants were marked as red triangle, whereas the variants located on UTR were marked as a blue triangle. Marker GH_D13G1855 was marked in red. (C) In marker D13_56413025, the phenotypes of the materials having T and C showed significant differences (p < 0.05) in 16ALE and 17KEL.
The promising genes identification was confirmed by the CI of stable QTLs, whose dynamics of expression are of significance for functional characterization. We identified 24,941 unique DEGs through the comparative transcriptome analysis (Supplementary Table 8) and 1,476 promising genes were detected in stable QTLs (Supplementary Table 9). A total of 473 of them expressed differentially during fiber development and were enriched in 130 pathways according to their KEGG analysis (Supplementary Table 12). These promising genes were all highly expressed DEGs (FPKM > 10) and enriched in critical pathways, as expected of significant promising genes. It is the primary and secondary cell wall deposition that determines the final FS trait (Pang et al., 2010). In our study, 10 significant promising genes enriched in the biological processes of pectin synthesis, phenylpropanoid biosynthesis, plant hormone signaling pathways, and transcription factors had an impact on FS and might regulate the process of fiber development in upland cotton (Supplementary Figure 3).
Three promising genes (GH_A06G0729, GH_D02G0231, GH_D11G1746) were enriched in the amino sugar and nucleotide sugar metabolism pathway. Among them, GH_D02G0231 was located in qFS-chr14-1 and identified as UDP-D-glucuronate 4-epimerase 6 (GAE6). UDP-d-galacturonate is necessary for pectin’s synthesis, in that the latter is synthesized from UDP-d-glucuronate by GAE6 (Usadel et al., 2004). Furthermore, GAE6 was found involved in both cotton fiber and Arabidopsis root hair growth (Pang et al., 2010). Another five promising genes (GH_A06G0665, GH_D10G2039, GH_D13G2625, GH_D13G1855, and GH_D13G2599) were enriched in the phenylpropanoid biosynthesis pathway and annotated as aldehyde dehydrogenase 2C4, CINNAMATE 4-HYDROXYLASE (C4H), O-METHYLTRANSFERASE 1 (OMT1), and AMP-dependent synthetase and ligase family protein. Interestingly, GH_D10G2039, GH_D13G2625, and GH_D13G1855 each exhibited high expression levels during fiber developmental stages and were identified in qFS-chr20-1, qFS-chr18-1, and qFS-chr18-4, respectively. All currently known building blocks of the lignin polymer are produced by, or derived from the general phenylpropanoid pathway (Barros et al., 2016; Zhou et al., 2017; Vanholme et al., 2019), which suggests that OMT1 and C4H play key functions in the pathway and influence FS (Guo et al., 2001; Schilmiller et al., 2009; Sadeghi et al., 2013; Vanholme et al., 2013; Sundin et al., 2014; Ho-Yue-Kuang et al., 2016). In addition, one missense variant and one upstream gene variant were detected here in GH_D13G2625 and GH_D13G1855 between the high- and low-quality groups. Six promising genes (GH_A06G0641, GH_D07G1483, GH_D07G1512, GH_D08G0287, GH_D13G2571, GH_D13G2572) were enriched in the plant hormone signal transduction pathway. GH_D07G1483 was enriched into auxin-signaling pathway as AUXIN RESPONSE FACTOR 18 (ARF18) and located in qCl-chr16-1, a genome region that influences both the FS and FL of upland cotton. Through phylogenetic analysis, ARF18 was classified into the class containing ARF1, ARF2, ARF9, and ARF11 (Okushima et al., 2005), all of which function as transcription repressors (Ulmasov et al., 1997). In Brassica napus L, ARF18 regulates cell growth in the silique wall and determines the seed weight (Liu J. et al., 2015). GH_D13G2571 was identified in qFS-chr18-1 and annotated as a transcription factor (TF) ETHYLENE INSENSITIVE 3 (EIN3), which is considered the key transcriptional regulator of the ethylene response in plants (Dolgikh et al., 2019) and accordingly has the most prominent role in ethylene signaling (Alonso et al., 2003; Binder et al., 2007; Binder, 2020). Meanwhile, GH_D07G1512, located in both qFL-chr16-3 and qFS-chr16-4, was annotated as JASMONATE RESISTANT1 (JAR1), which could activate JA signaling (Meesters et al., 2014). GH_D13G2571 and GH_D07G1512 were enriched in the ethylene signaling and jasmonic acid (JA) signaling pathways, respectively. EIN3 could regulate ETHYLENE RESPONSE FACTOR 1 (ERF1), and GbERF1-like reportedly activates the JA/ET signaling pathway and lignin synthesis (Guo et al., 2016). In addition, they reached their peak expression levels at 25 DPA, when the fiber cell was in the secondary wall biosynthesis stage, thus suggesting that they regulate the ethylene and jasmonic acid pathways and thus affect FS. Further, there were three missense variants and two synonymous variants identified in GH_D13G2571 between the high- and low-quality groups, a finding that merits further investigation. Besides, another three transcription factors were identified, which might regulate fiber cell development and affect FS. GH_D06G0054 was annotated here as transcription factor bZIP44. It has been reported that AtbZIP44 TF could positively regulate AtMAN7 expression and influence not only the loosening of the cell wall in the micropylar endosperm upon germination but could also be involved in the elongation of radicle cells (Iglesias-Fernández et al., 2013). GH_A06G0641 was annotated as DELLA family member GIBBERELLIC ACID INSENSITIVE (GAI), whose mutants were shown to reduce GA responses. GAI is involved in regulating stem elongation via the GA signaling pathway (Peng et al., 1997; Tan et al., 2019), which also plays a crucial role in trichome development (Wang Z. et al., 2019). GH_D01G2072 was identified as TEOSINTE BRANCHED1, CYCLOIDEA, PCF8 (TCP8) belonging to the TCP family class I group (Cubas et al., 2001), for which gene redundancy is a common feature. The TCP family class I group was shown to be involved in regulating leaf development (Aguilar Martinez and Sinha, 2013). Both TCP8 and TCP15, other members of this member of the family class I group, could regulate filament elongation (Gastaldi et al., 2020). Moreover, GhTCP14, a class I member in upland cotton, was also identified as producing higher transcripts during fiber initiation and elongation in G. hirsutum, which enhanced the abundance of trichomes on the stem, inflorescence, and root parts (Wang et al., 2013; Wang Z. et al., 2019). To sum up, within the stable QTLs, it was the 10 significant promising genes related to pectin synthesis, phenylpropanoid biosynthesis, plant hormone signaling pathways as well as some transcription factors that played vital roles in determining the FS trait of upland cotton.
To investigate the parental source of high-quality related alleles, we constructed a genetic map of the hybrid CCRI70 RIL population by using SLAF-seq, this containing 24,425 SNP markers spanning a distance of 4850.47 cM. To detect stable QTL across multiple environments, we evaluated three key fiber quality traits in nine environments at six locations in 2015 and 2016. This revealed 289 QTLs, 36 of these were stable QTLs (17 for FS, 11 for FL, and eight for FM), of which 15 were located on At and another 21 detected on Dt. Comparing the stable QTLs with those in previous studies, 18 were already known (eight for FS, six for FL, and four for FM) yet the other 18 were novel (nine for FS, five for FL, and four for FM); they provide a robust basis for understanding the distribution and the sources of high fiber quality-related alleles in cotton plants. Notably, qFS-chr18-4 and marker D13_56413025 could serve as important references for upland cotton maker-assisted selection breeding. Transcriptome sequencing for 72 libraries of the two parents and two RILs during fiber development with three biological repeats was performed, this aiming to understand the potential candidate genes expression patterns and DEGs (differentially expressed genes) in superior and inferior fiber quality groups during the process of fiber development. Based on this transcriptome analysis, 24,941 unique DEGs were identified via vertical and horizontal comparisons, from which 473 of the 1,476 promising genes were identified as DEGs during fiber development. Among 320 differentially expressed promising genes for FS, 10 significant promising genes with high expression levels were enriched in the pathways of pectin synthesis, phenylpropanoid biosynthesis, and plant hormone signaling; these, as well as several others, annotated as transcription factors may jointly impact FS and regulate fiber development. Altogether, our results provide a fresh basis for improving cotton fiber quality via marker-assisted breeding that could benefit the textile industry.
The datasets presented in this study can be found in online repositories. The names of the repository/repositories and accession number(s) can be found below: https://ngdc.cncb.ac.cn/, (CRA002982 and CRA00473).
XJ: conceptualization, formal analysis, validation, software, and writing—original draft. JG: resources. JZ, HC, ZK and JP: investigation. ZZ: software and methodology. YS: methodology. JL, QG, and SZ: visualization. AL and WG: data curation. XD and SF: project administration. JC: formal analysis. TJ and RW: software. QC: investigation and writing—review and editing. SW: writing—review and editing. HS and YY: conceptualization, writing—review and editing, supervision, and funding acquisition. All authors contributed to the article and approved the submitted version.
This work was supported by grants from the National Natural Science Foundation of China (31621005, 31371668, and 32070560), the China Agriculture Research System of MOF and MARA, Central Public-interest Scientific Institution Basal Research Fund (No. Y2017PT51), the National Agricultural Science and Technology Innovation project for CAAS (CAAS-ASTIP-2016-ICR), and the Agro-Industry Research and Development Special Fund of China (CN) (1610162019010101).
The authors declare that the research was conducted in the absence of any commercial or financial relationships that could be construed as a potential conflict of interest.
All claims expressed in this article are solely those of the authors and do not necessarily represent those of their affiliated organizations, or those of the publisher, the editors and the reviewers. Any product that may be evaluated in this article, or claim that may be made by its manufacturer, is not guaranteed or endorsed by the publisher.
The Supplementary Material for this article can be found online at: https://www.frontiersin.org/articles/10.3389/fpls.2021.753755/full#supplementary-material
Supplementary Figure 1 | Weighted gene co-expression network analysis (WGCNA) of DEGs. (A) Hierarchical dendrogram showing co-expression modules identified by WGCNA. Each leaf in the tree represents one gene. The major tree was divided into 11 modules in total. (B) Module–sample relationships. With the correlation coefficient and the e-value shown in each square, each column represents a sample tissue and replication.
Supplementary Figure 2 | Heatmap of expression in QTL clusters. (A) qCl-chr10-1, (B) qCl-chr14-1, (C) qCl-chr16-1, (D) qCl-chr17-1, and (E) qCl-chr24-1 distribution and heatmaps of potential candidate genes expression [log2(FPKM + 1)].
Supplementary Figure 3 | Dynamics of 10 significant promising genes’ expression. Expression dynamics of GH_D02G0231 (A), GH_D10G2039 (B), GH_D13G2625 (C), GH_D13G1855 (D), GH_D07G1483 (E), GH_D13G2571 (F), GH_D07G1512 (G), GH_D06G0054 (H), GH_A06G0641 (I), and GH_D01G2072 (J) in fiber developmental stages.
Aguilar Martinez, J., and Sinha, N. (2013). Analysis of the role of Arabidopsis class I TCP genes AtTCP7, AtTCP8, AtTCP22, and AtTCP23 in leaf development. Front. Plant Sci. 4:406. doi: 10.3389/fpls.2013.00406
Alonso, J. M., Stepanova, A. N., Solano, R., Wisman, E., Ferrari, S., Ausubel, F. M., et al. (2003). Five components of the ethylene-response pathway identified in a screen for weak ethylene-insensitive mutants in Arabidopsis. Proc. Natl. Acad. Sci. U.S.A. 100, 2992–2997.
Altschul, S. F., Gish, W., Miller, W., Myers, E. W., and Lipman, D. J. (1990). Basic local alignment search tool. J. Mol. Biol. 215, 403–410.
Applequist, W. L., Cronn, R., and Wendel, J. F. (2001). Comparative development of fiber in wild and cultivated cotton. Evol. Dev. 3, 3–17.
Barros, J., Serrani-Yarce, J. C., Chen, F., Baxter, D., Venables, B. J., and Dixon, R. A. (2016). Role of bifunctional ammonia-lyase in grass cell wall biosynthesis. Nat. Plants 2, 1–9.
Binder, B. M., Walker, J. M., Gagne, J. M., Emborg, T. J., Hemmann, G., Bleecker, A. B., et al. (2007). The Arabidopsis EIN3 binding F-Box proteins EBF1 and EBF2 have distinct but overlapping roles in ethylene signaling. Plant Cell 19, 509–523.
Bolger, A. M., Lohse, M., and Usadel, B. (2014). Trimmomatic: a flexible trimmer for Illumina sequence data. Bioinformatics 30, 2114–2120.
Chandnani, R., Kim, C., Guo, H., Shehzad, T., Wallace, J. G., He, D., et al. (2018). Genetic analysis of gossypium fiber quality traits in reciprocal advanced backcross populations. Plant Genome 11:57. doi: 10.3835/plantgenome2017.06.0057
Chen, H., Qian, N., Guo, W., Song, Q., Li, B., Deng, F., et al. (2009). Using three overlapped RILs to dissect genetically clustered QTL for fiber strength on Chro. D8 in Upland cotton. Theoret. Appl. Genet. 119, 605–612.
Churchill, G. A., and Doerge, R. W. (1994). Empirical threshold values for quantitative trait mapping. Genetics 138, 963–971. doi: 10.1093/genetics/138.3.963
Cingolani, P., Platts, A., Wang, L. L., Coon, M., Nguyen, T., Wang, L., et al. (2012). A program for annotating and predicting the effects of single nucleotide polymorphisms. SnpEff. Fly 6, 80–92. doi: 10.4161/fly.19695
Cubas, P., Coen, E., and Zapater, J. M. (2001). Ancient asymmetries in the evolution of flowers. Curr. Biol. 11, 1050–1052.
Deng, X., Gong, J., Liu, A., Shi, Y., Gong, W., Ge, Q., et al. (2019). QTL mapping for fiber quality and yield-related traits across multiple generations in segregating population of CCRI 70. J. Cotton Res. 2:13. doi: 10.1186/s42397-019-0029-y
Diouf, L., Magwanga, R. O., Gong, W., He, S., Pan, Z., Jia, Y. H., et al. (2018). QTL mapping of fiber quality and yield-related traits in an intra-specific upland cotton using genotype by sequencing (GBS). Int. J. Mol. Sci. 19:441.
Dolgikh, V. A., Pukhovaya, E. M., and Zemlyanskaya, E. V. (2019). Shaping ethylene response: the role of EIN3/EIL1 transcription factors. Front. Plant Sci. 10:1030.
Du, L., and Poovaiah, B. W. (2005). Ca2+/calmodulin is critical for brassinosteroid biosynthesis and plant growth. Nature 437, 741–745. doi: 10.1038/nature03973
Du, X., Huang, G., He, S., Yang, Z., Sun, G., Ma, X., et al. (2018). Resequencing of 243 diploid cotton accessions based on an updated A genome identifies the genetic basis of key agronomic traits. Nat. Genet. 50, 796–802.
Fang, D. D., Jenkins, J. N., Deng, D. D., McCarty, J. C., Li, P., and Wu, J. (2014). Quantitative trait loci analysis of fiber quality traits using a random-mated recombinant inbred population in Upland cotton (Gossypium hirsutum L.). BMC Genom. 15:397.
Fang, L., Gong, H., Hu, Y., Liu, C., Zhou, B., Huang, T., et al. (2017a). Genomic insights into divergence and dual domestication of cultivated allotetraploid cottons. Genome Biol. 18:33.
Fang, L., Wang, Q., Hu, Y., Jia, Y., Chen, J., Liu, B., et al. (2017b). Genomic analyses in cotton identify signatures of selection and loci associated with fiber quality and yield traits. Nat. Genet. 49:1089.
Fang, X., Liu, X., Wang, X., Wang, W., Liu, D., Zhang, J., et al. (2017c). Fine-mapping qFS07. 1 controlling fiber strength in upland cotton (Gossypium hirsutum L.). Theoret. Appl. Genet. 130, 795–806.
Gastaldi, V., Lucero, L. E., Ferrero, L. V., Ariel, F. D., and Gonzalez, D. H. (2020). Class-I TCP transcription factors activate the SAUR63 gene subfamily in gibberellin-dependent stamen filament elongation. Plant Physiol. 182, 2096–2110.
Gilbert, M. K., Kim, H. J., Tang, Y., Naoumkina, M., and Fang, D. D. (2014). Comparative transcriptome analysis of short fiber mutants Ligon-lintless 1 and 2 reveals common mechanisms pertinent to fiber elongation in cotton (Gossypium hirsutum L.). PLoS One 9:e95554.
Gu, Q., Ke, H., Liu, Z., Lv, X., Sun, Z., Zhang, M., et al. (2020). A high-density genetic map and multiple environmental tests reveal novel quantitative trait loci and candidate genes for fibre quality and yield in cotton. Theoret. Appl. Genet. 133, 3395–3408. doi: 10.1007/s00122-020-03676-z
Guo, D., Chen, F., Inoue, K., Blount, J. W., and Dixon, R. A. (2001). Downregulation of caffeic acid 3-O-methyltransferase and caffeoyl CoA 3-O-methyltransferase in transgenic alfalfa: impacts on lignin structure and implications for the biosynthesis of G and S lignin. Plant Cell 13, 73–88.
Guo, W., Jin, L., Miao, Y., He, X., Hu, Q., Guo, K., et al. (2016). An ethylene response-related factor, GbERF1-like, from Gossypium barbadense improves resistance to Verticillium dahliae via activating lignin synthesis. Plant Mol. Biol. 91, 305–318.
Haigler, C. H., Betancur, L., Stiff, M. R., and Tuttle, J. R. (2012). Cotton fiber: a powerful single-cell model for cell wall and cellulose research. Front. Plant Sci. 3:104. doi: 10.3389/fpls.2012.00104
Ho-Yue-Kuang, S., Alvarado, C., Antelme, S., Bouchet, B., Cézard, L., Le Bris, P., et al. (2016). Mutation in Brachypodium caffeic acid O-methyltransferase 6 alters stem and grain lignins and improves straw saccharification without deteriorating grain quality. J. Exp. Bot. 67, 227–237.
Hu, Y., Chen, J., Fang, L., Zhang, Z., Ma, W., Niu, Y., et al. (2019). Gossypium barbadense and Gossypium hirsutum genomes provide insights into the origin and evolution of allotetraploid cotton. Nat. Genet. 51, 739–748. doi: 10.1038/s41588-019-0371-5
Huang, C., Nie, X., Shen, C., You, C., Li, W., Zhao, W., et al. (2017). Population structure and genetic basis of the agronomic traits of upland cotton in China revealed by a genome-wide association study using high-density SNP s. Plant Biotechnol. J. 15, 1374–1386.
Huang, G., Wu, Z., Percy, R. G., Bai, M., Li, Y., Frelichowski, J. E., et al. (2020). Genome sequence of Gossypium herbaceum and genome updates of Gossypium arboreum and Gossypium hirsutum provide insights into cotton A-genome evolution. Nat. Genet. 52, 516–524.
Hulse-Kemp, A. M., Lemm, J., Plieske, J., Ashrafi, H., Buyyarapu, R., Fang, D. D., et al. (2015). Development of a 63K SNP array for cotton and high-density mapping of intraspecific and interspecific populations of Gossypium spp. G3 Genes Genomes Genet. 5, 1187–1209.
Iglesias-Fernández, R., Barrero-Sicilia, C., Carrillo-Barral, N., Oñate-Sánchez, L., and Carbonero, P. (2013). Arabidopsis thaliana bZIP 44: a transcription factor affecting seed germination and expression of the mannanase-encoding gene AtMAN7. Plant J. 74, 767–780.
Islam, M. S., Fang, D. D., Thyssen, G. N., Delhom, C. D., Liu, Y., and Kim, H. J. (2016). Comparative fiber property and transcriptome analyses reveal key genes potentially related to high fiber strength in cotton (Gossypium hirsutum L.) line MD52ne. BMC Plant Biol. 16:36.
Jiang, X., Fan, L., Li, P., Zou, X., Zhang, Z., Fan, S., et al. (2021). Co-expression network and comparative transcriptome analysis for fiber initiation and elongation reveal genetic differences in two lines from upland cotton CCRI70 RIL population. PeerJ 9:e11812. doi: 10.7717/peerj.11812
Jin, J., He, K., Tang, X., Li, Z., Lv, L., Zhao, Y., et al. (2015). An Arabidopsis transcriptional regulatory map reveals distinct functional and evolutionary features of novel transcription factors. Mol. Biol. Evol. 32, 1767–1773. doi: 10.1093/molbev/msv058
Jin, J., Tian, F., Yang, D.-C., Meng, Y.-Q., Kong, L., Luo, J., et al. (2016). PlantTFDB 4.0: toward a central hub for transcription factors and regulatory interactions in plants. Nucleic Acids Res. 45, D1040–D1045. doi: 10.1093/nar/gkw982
Jin, J., Zhang, H., Kong, L., Gao, G., and Luo, J. J. (2014). PlantTFDB 3.0: a portal for the functional and evolutionary study of plant transcription factors. Nucleic Acids Res. 42, D1182–D1187. doi: 10.1093/nar/gkt1016
Kim, H. J., and Triplett, B. A. (2001). Cotton fiber growth in planta and in vitro. Models for plant cell elongation and cell wall biogenesis. Plant Physiol. 127, 1361–1366.
Lacape, J.-M., Llewellyn, D., Jacobs, J., Arioli, T., Becker, D., Calhoun, S., et al. (2010). Meta-analysis of cotton fiber quality QTLs across diverse environments in a Gossypium hirsutum x G. barbadense RIL population. BMC Plant Biol. 10:132. doi: 10.1186/1471-2229-10-132
Langfelder, P., and Horvath, S. (2008). WGCNA: an R package for weighted correlation network analysis. BMC Bioinform. 9:559. doi: 10.1186/1471-2105-9-559
Langmead, B., Trapnell, C., Pop, M., and Salzberg, S. L. (2009). Ultrafast and memory-efficient alignment of short DNA sequences to the human genome. Genome Biol. 10:R25. doi: 10.1186/gb-2009-10-3-r25
Lee, J. J., Hassan, O. S., Gao, W., Wei, N. E., Kohel, R. J., Chen, X.-Y., et al. (2006). Developmental and gene expression analyses of a cotton naked seed mutant. Planta 223, 418–432. doi: 10.1007/s00425-005-0098-7
Lee, J. J., Woodward, A. W., and Chen, Z. J. (2007). Gene expression changes and early events in cotton fibre development. Ann. Bot. 100, 1391–1401. doi: 10.1093/aob/mcm232
Li, C., Dong, Y., Zhao, T., Li, L., Li, C., Yu, E., et al. (2016). Genome-wide SNP linkage mapping and QTL analysis for fiber quality and yield traits in the upland cotton recombinant inbred lines population. Front. Plant Sci. 7:1356. doi: 10.3389/fpls.2016.01356
Li, F., Fan, G., Lu, C., Xiao, G., Zou, C., Kohel, R. J., et al. (2015). Genome sequence of cultivated Upland cotton (Gossypium hirsutum TM-1) provides insights into genome evolution. Nat. Biotechnol. 33:524. doi: 10.1038/nbt.3208
Li, F., Fan, G., Wang, K., Sun, F., Yuan, Y., Song, G., et al. (2014). Genome sequence of the cultivated cotton Gossypium arboreum. Nat. Genet. 46, 567–572. doi: 10.1038/ng.2987
Li, H., and Durbin, R. (2009). Fast and accurate short read alignment with Burrows–Wheeler transform. Bioinformatics 25, 1754–1760. doi: 10.1093/bioinformatics/btp324
Li, H., Ye, G., and Wang, J. (2007). A modified algorithm for the improvement of composite interval mapping. Genetics 175, 361–374. doi: 10.1534/genetics.106.066811
Li, P.-t., Wang, M., Lu, Q.-w., Ge, Q., Liu, A.-y., Gong, J.-w., et al. (2017). Comparative transcriptome analysis of cotton fiber development of Upland cotton (Gossypium hirsutum) and chromosome segment substitution lines from G. hirsutum× G. barbadense. BMC Genomics 18:705. doi: 10.1186/s12864-017-4077-8
Li, X., Wu, M., Liu, G., Pei, W., Zhai, H., Yu, J., et al. (2017). Identification of candidate genes for fiber length quantitative trait loci through RNA-Seq and linkage and physical mapping in cotton. BMC Genomics 18:427. doi: 10.1186/s12864-017-3812-5
Liu, J., Hua, W., Hu, Z., Yang, H., Zhang, L., Li, R., et al. (2015). Natural variation in ARF18 gene simultaneously affects seed weight and silique length in polyploid rapeseed. Proc. Natl. Acad. Sci. U.S.A. 112, E5123–E5132. doi: 10.1073/pnas.1502160112
Liu, X., Teng, Z., Wang, J., Wu, T., Zhang, Z., Deng, X., et al. (2017). Enriching an intraspecific genetic map and identifying QTL for fiber quality and yield component traits across multiple environments in Upland cotton (Gossypium hirsutum L.). Mol. Genet. Genom. 292, 1281–1306. doi: 10.1007/s00438-017-1347-8
Liu, X., Zhao, B., Zheng, H.-J., Hu, Y., Lu, G., Yang, C.-Q., et al. (2015). Gossypium barbadense genome sequence provides insight into the evolution of extra-long staple fiber and specialized metabolites. Sci. Rep. 5:14139. doi: 10.1038/srep14139
Love, M. I., Huber, W., and Anders, S. (2014). Moderated estimation of fold change and dispersion for RNA-seq data with DESeq2. Genome Biol. 15:550. doi: 10.1186/s13059-014-0550-8
Lu, Q., Shi, Y., Xiao, X., Li, P., Gong, J., Gong, W., et al. (2017). Transcriptome analysis suggests that chromosome introgression fragments from sea island cotton (Gossypium barbadense) increase fiber strength in upland cotton (Gossypium hirsutum). G3 Genes Genomes Genet. 7, 3469–3479. doi: 10.1534/g3.117.300108
Ma, Z., He, S., Wang, X., Sun, J., Zhang, Y., Zhang, G., et al. (2018). Resequencing a core collection of upland cotton identifies genomic variation and loci influencing fiber quality and yield. Nat. Genet. 50, 803–813. doi: 10.1038/s41588-018-0119-7
Mathangadeera, R. W., Hequet, E. F., Kelly, B., Dever, J. K., and Kelly, C. M. (2020). Importance of cotton fiber elongation in fiber processing. Industr. Crops Product. 147:112217. doi: 10.1016/j.indcrop.2020.112217
McKenna, A., Hanna, M., Banks, E., Sivachenko, A., Cibulskis, K., Kernytsky, A., et al. (2010). The genome analysis toolkit: a MapReduce framework for analyzing next-generation DNA sequencing data. Genome Res. 20, 1297–1303. doi: 10.1101/gr.107524.110
Meesters, C., Mönig, T., Oeljeklaus, J., Krahn, D., Westfall, C. S., Hause, B., et al. (2014). A chemical inhibitor of jasmonate signaling targets JAR1 in Arabidopsis thaliana. Nat. Chem. Biol. 10, 830–836. doi: 10.1038/nchembio.1591
Meng, L., Li, H., Zhang, L., and Wang, J. (2015). QTL IciMapping: Integrated software for genetic linkage map construction and quantitative trait locus mapping in biparental populations. Crop. J. 3, 269–283. doi: 10.1016/j.cj.2015.01.001
Moriya, Y., Itoh, M., Okuda, S., Yoshizawa, A. C., and Kanehisa, M. (2007). KAAS: an automatic genome annotation and pathway reconstruction server. Nucleic Acids Res. 35, W182–W185. doi: 10.1093/nar/gkm321
Okushima, Y., Overvoorde, P. J., Arima, K., Alonso, J. M., Chan, A., Chang, C., et al. (2005). Functional genomic analysis of the AUXIN RESPONSE FACTOR gene family members in Arabidopsis thaliana: unique and overlapping functions of ARF7 and ARF19. Plant Cell 17, 444–463. doi: 10.1105/tpc.104.028316
Pang, C.-Y., Wang, H., Pang, Y., Xu, C., Jiao, Y., Qin, Y.-M., et al. (2010). Comparative proteomics indicates that biosynthesis of pectic precursors is important for cotton fiber and Arabidopsis root hair elongation. Mol. Cell. Proteom. 9, 2019–2033. doi: 10.1074/mcp.M110.000349
Patel, J. D., Huang, X., Lin, L., Das, S., Chandnani, R., Khanal, S., et al. (2020). The Ligon lintless-2 short fiber mutation is located within a terminal deletion of chromosome 18 in cotton. Plant Physiol. 183, 277–288. doi: 10.1104/pp.19.01531
Paterson, A. H., Wendel, J. F., Gundlach, H., Guo, H., Jenkins, J., Jin, D., et al. (2012). Repeated polyploidization of Gossypium genomes and the evolution of spinnable cotton fibres. Nature 492, 423–427. doi: 10.1038/nature11798
Paterson, A., Saranga, Y., Menz, M., Jiang, C.-X., and Wright, R. (2003). QTL analysis of genotype× environment interactions affecting cotton fiber quality. Theoret. Appl. Genet. 106, 384–396. doi: 10.1007/s00122-002-1025-y
Pei, G., Chen, L., and Zhang, W. (2017). WGCNA application to proteomic and metabolomic data analysis. Methods Enzymol. 585, 135–158. doi: 10.1016/bs.mie.2016.09.016
Peng, J., Carol, P., Richards, D. E., King, K. E., Cowling, R. J., Murphy, G. P., et al. (1997). The Arabidopsis GAI gene defines a signaling pathway that negatively regulates gibberellin responses. Genes Dev. 11, 3194–3205. doi: 10.1101/gad.11.23.3194
Pertea, M., Kim, D., Pertea, G. M., Leek, J. T., and Salzberg, S. L. (2016). Transcript-level expression analysis of RNA-seq experiments with HISAT, StringTie and Ballgown. Nat. Protoc. 11, 1650–1667. doi: 10.1038/nprot.2016.095
Pertea, M., Pertea, G. M., Antonescu, C. M., Chang, T.-C., Mendell, J. T., and Salzberg, S. L. (2015). StringTie enables improved reconstruction of a transcriptome from RNA-seq reads. Nat. Biotechnol. 33, 290–295. doi: 10.1038/nbt.3122
Qi, H., Wang, N., Qiao, W., Xu, Q., Zhou, H., Shi, J., et al. (2017). Construction of a high-density genetic map using genotyping by sequencing (GBS) for quantitative trait loci (QTL) analysis of three plant morphological traits in upland cotton (Gossypium hirsutum L.). Euphytica 213:83. doi: 10.1007/s10681-017-1867-7
Rong, J., Abbey, C., Bowers, J. E., Brubaker, C. L., Chang, C., Chee, P. W., et al. (2004). A 3347-locus genetic recombination map of sequence-tagged sites reveals features of genome organization, transmission and evolution of cotton (Gossypium). Genetics 166, 389–417. doi: 10.1534/genetics.166.1.389
Rong, J., Feltus, F. A., Waghmare, V. N., Pierce, G. J., Chee, P. W., Draye, X., et al. (2007). Meta-analysis of polyploid cotton QTL shows unequal contributions of subgenomes to a complex network of genes and gene clusters implicated in lint fiber development. Genetics 176, 2577–2588. doi: 10.1534/genetics.107.074518
Sadeghi, M., Dehghan, S., Fischer, R., Wenzel, U., Vilcinskas, A., Kavousi, H. R., et al. (2013). Isolation and characterization of isochorismate synthase and cinnamate 4-hydroxylase during salinity stress, wounding, and salicylic acid treatment in Carthamus tinctorius. Plant Signal. Behav. 8:e27335. doi: 10.4161/psb.27335
Said, J. I., Knapka, J. A., Song, M., and Zhang, J. (2015). Cotton QTLdb: a cotton QTL database for QTL analysis, visualization, and comparison between Gossypium hirsutum and G. hirsutum× G. barbadense populations. Mol. Genet. Genom. 290, 1615–1625. doi: 10.1007/s00438-015-1021-y
Said, J. I., Lin, Z., Zhang, X., Song, M., and Zhang, J. (2013). A comprehensive meta QTL analysis for fiber quality, yield, yield related and morphological traits, drought tolerance, and disease resistance in tetraploid cotton. BMC Genom. 14:776. doi: 10.1186/1471-2164-14-776
Schilmiller, A. L., Stout, J., Weng, J. K., Humphreys, J., Ruegger, M. O., and Chapple, C. (2009). Mutations in the cinnamate 4-hydroxylase gene impact metabolism, growth and development in Arabidopsis. Plant J. 60, 771–782. doi: 10.1111/j.1365-313X.2009.03996.x
Shi, Y., Li, W., Li, A., Ge, R., Zhang, B., Li, J., et al. (2015). Constructing a high-density linkage map for Gossypium hirsutum× Gossypium barbadense and identifying QTLs for lint percentage. J. Integrat. Plant Biol. 57, 450–467. doi: 10.1111/jipb.12288
Shi, Y., Liu, A., Li, J., Zhang, J., Li, S., Zhang, J., et al. (2020). Examining two sets of introgression lines across multiple environments reveals background-independent and stably expressed quantitative trait loci of fiber quality in cotton. Theoret. Appl. Genet. 133, 2075–2093. doi: 10.1007/s00122-020-03578-0
Sun, F.-D., Zhang, J.-H., Wang, S.-F., Gong, W.-K., Shi, Y.-Z., Liu, A.-Y., et al. (2012). QTL mapping for fiber quality traits across multiple generations and environments in upland cotton. Mol. Breed. 30, 569–582.
Sun, X., Liu, D., Zhang, X., Li, W., Liu, H., Hong, W., et al. (2013). SLAF-seq: an efficient method of large-scale de novo SNP discovery and genotyping using high-throughput sequencing. PLoS One 8:e58700. doi: 10.1371/journal.pone.0058700
Sundin, L., Vanholme, R., Geerinck, J., Goeminne, G., Höfer, R., Kim, H., et al. (2014). Mutation of the inducible Arabidopsis thaliana cytochrome P450 reductase2 alters lignin composition and improves saccharification. Plant Physiol. 166, 1956–1971.
Tan, H., Man, C., Xie, Y., Yan, J., Chu, J., and Huang, J. J. (2019). A crucial role of GA-regulated flavonol biosynthesis in root growth of Arabidopsis. Mol. Plant 12, 521–537. doi: 10.1016/j.molp.2018.12.021
Tan, Z., Zhang, Z., Sun, X., Li, Q., Sun, Y., Yang, P., et al. (2018). Genetic map construction and fiber quality QTL mapping using the CottonSNP80K array in upland cotton. Front. Plant Sci. 9:225. doi: 10.3389/fpls.2018.00225
Tian, F., Yang, D.-C., Meng, Y.-Q., Jin, J., and Gao, G. (2020). PlantRegMap: charting functional regulatory maps in plants. Nucleic Acids Res. 48, D1104–D1113. doi: 10.1093/nar/gkz1020
Tian, Y., and Zhang, T. (2021). MIXTAs and phytohormones orchestrate cotton fiber development. Curr. Opin. Plant Biol. 59:101975. doi: 10.1016/j.pbi.2020.10.007
Ulloa, M., Meredith, W. Jr., Shappley, Z., and Kahler, A. (2002). RFLP genetic linkage maps from four F 2.3 populations and a joinmap of Gossypium hirsutum L. Theoret. Appl. Genet. 104, 200–208. doi: 10.1007/s001220100739
Ulloa, M., Saha, S., Jenkins, J., Meredith, W. Jr., McCarty, J. Jr., and Stelly, D. (2005). Chromosomal assignment of RFLP linkage groups harboring important QTLs on an intraspecific cotton (Gossypium hirsutum L.) joinmap. J. Hered. 96, 132–144. doi: 10.1093/jhered/esi020
Ulmasov, T., Murfett, J., Hagen, G., and Guilfoyle, T. J. (1997). Aux/IAA proteins repress expression of reporter genes containing natural and highly active synthetic auxin response elements. Plant Cell 9, 1963–1971. doi: 10.1105/tpc.9.11.1963
Usadel, B., Schlüter, U., Mølhøj, M., Gipmans, M., Verma, R., Kossmann, J., et al. (2004). Identification and characterization of a UDP-d-glucuronate 4-epimerase in Arabidopsis. FEBS Lett. 569, 327–331. doi: 10.1016/j.febslet.2004.06.005
Vanholme, R., Cesarino, I., Rataj, K., Xiao, Y., Sundin, L., Goeminne, G., et al. (2013). Caffeoyl shikimate esterase (CSE) is an enzyme in the lignin biosynthetic pathway in Arabidopsis. Science 341, 1103–1106. doi: 10.1126/science.1241602
Vanholme, R., De Meester, B., Ralph, J., and Boerjan, W. (2019). Lignin biosynthesis and its integration into metabolism. Curr. Opin. Biotechnol. 56, 230–239. doi: 10.1016/j.copbio.2019.02.018
Wang, F., Zhang, J., Chen, Y., Zhang, C., Gong, J., Song, Z., et al. (2020). Identification of candidate genes for key fibre-related QTLs and derivation of favourable alleles in Gossypium hirsutum recombinant inbred lines with G. barbadense introgressions. Plant Biotechnol. J. 18, 707–720. doi: 10.1111/pbi.13237
Wang, H., Jin, X., Zhang, B., Shen, C., and Lin, Z. (2015). Enrichment of an intraspecific genetic map of upland cotton by developing markers using parental RAD sequencing. DNA Res. 22, 147–160. doi: 10.1093/dnares/dsu047
Wang, K., Wang, Z., Li, F., Ye, W., Wang, J., Song, G., et al. (2012). The draft genome of a diploid cotton Gossypium raimondii. Nat. Genet. 44, 1098–1103. doi: 10.1038/ng.2371
Wang, L., He, S., Dia, S., Sun, G., Liu, X., Wang, X., et al. (2021). Alien genomic introgressions enhanced fiber strength in upland cotton (Gossypium hirsutum L.). Industr. Crops Product. 159:113028. doi: 10.1016/j.indcrop.2020.113028
Wang, M., Tu, L., Yuan, D., Zhu, D., Shen, C., Li, J., et al. (2019). Reference genome sequences of two cultivated allotetraploid cottons, Gossypium hirsutum and Gossypium barbadense. Nat. Genet. 51:224. doi: 10.1038/s41588-018-0282-x
Wang, M.-Y., Zhao, P.-M., Cheng, H.-Q., Han, L.-B., Wu, X.-M., Gao, P., et al. (2013). The cotton transcription factor TCP14 functions in auxin-mediated epidermal cell differentiation and elongation. Plant Physiol. 162, 1669–1680. doi: 10.1104/pp.113.215673
Wang, P., Zhu, Y., Song, X., Cao, Z., Ding, Y., Liu, B., et al. (2012). Inheritance of long staple fiber quality traits of Gossypium barbadense in G. hirsutum background using CSILs. Theoret. Appl. Genet. 124, 1415–1428. doi: 10.1007/s00122-012-1797-7
Wang, S., Basten, C., and Zeng, Z. (2007). Windows QTL Cartographer 2.5. Department of Statistics. Raleigh, NC: North Carolina State University.
Wang, Y., Ning, Z., Hu, Y., Chen, J., Zhao, R., Chen, H., et al. (2015). Molecular mapping of restriction-site associated DNA markers in allotetraploid upland cotton. PLoS One 10:e0124781. doi: 10.1371/journal.pone.0124781
Wang, Z., Yang, Z., and Li, F. (2019). Updates on molecular mechanisms in the development of branched trichome in Arabidopsis and nonbranched in cotton. Plant Biotechnol. J. 17, 1706–1722. doi: 10.1111/pbi.13167
Wu, J., Mao, X., Cai, T., Luo, J., and Wei, L. (2006). KOBAS server: a web-based platform for automated annotation and pathway identification. Nucleic Acids Res. 34, W720–W724. doi: 10.1093/nar/gkl167
Wu, Y., Bhat, P. R., Close, T. J., and Lonardi, S. (2008). Efficient and accurate construction of genetic linkage maps from the minimum spanning tree of a graph. PLoS Genet. 4:e1000212. doi: 10.1371/journal.pgen.1000212
Xie, C., Mao, X., Huang, J., Ding, Y., Wu, J., Dong, S., et al. (2011). KOBAS 2.0: a web server for annotation and identification of enriched pathways and diseases. Nucleic Acids Res. 39, W316–W322. doi: 10.1093/nar/gkr483
Yang, Z., Zhang, C., Yang, X., Liu, K., Wu, Z., Zhang, X., et al. (2014). PAG1, a cotton brassinosteroid catabolism gene, modulates fiber elongation. New Phytol. 203, 437–448. doi: 10.1111/nph.12824
Yoo, M.-J., and Wendel, J. F. (2014). Comparative evolutionary and developmental dynamics of the cotton (Gossypium hirsutum) fiber transcriptome. PLoS Genet. 10:e1004073.
Youn, J. H., Kim, T. W., Joo, S. H., Son, S. H., Roh, J., Kim, S., et al. (2018). Function and molecular regulation of DWARF1 as a C-24 reductase in brassinosteroid biosynthesis in Arabidopsis. J. Exp. Bot. 69, 1873–1886. doi: 10.1093/jxb/ery038
Yuan, D., Tang, Z., Wang, M., Gao, W., Tu, L., Jin, X., et al. (2015). The genome sequence of Sea-Island cotton (Gossypium barbadense) provides insights into the allopolyploidization and development of superior spinnable fibres. Sci. Rep. 5:17662. doi: 10.1038/srep17662
Zhang, J., Zhang, Q., Cheng, T., Yang, W., Pan, H., Zhong, J., et al. (2015). High-density genetic map construction and identification of a locus controlling weeping trait in an ornamental woody plant (Prunus mume Sieb. et Zucc). DNA Res. 22, 183–191. doi: 10.1093/dnares/dsv003
Zhang, K., Zhang, J., Ma, J., Tang, S., Liu, D., Teng, Z., et al. (2012). Genetic mapping and quantitative trait locus analysis of fiber quality traits using a three-parent composite population in upland cotton (Gossypium hirsutum L.). Mol. Breed. 29, 335–348.
Zhang, T., Hu, Y., Jiang, W., Fang, L., Guan, X., Chen, J., et al. (2015). Sequencing of allotetraploid cotton (Gossypium hirsutum L. acc. TM-1) provides a resource for fiber improvement. Nat. Biotechnol. 33, 531–537. doi: 10.1038/nbt.3207
Zhang, Z., Ge, Q., Liu, A., Li, J., Gong, J., Shang, H., et al. (2017). Construction of a high-density genetic map and its application to QTL identification for fiber strength in Upland cotton. Crop. Sci. 57, 774–788.
Zhang, Z., Li, J., Jamshed, M., Shi, Y., Liu, A., Gong, J., et al. (2020). Genome-wide quantitative trait loci reveal the genetic basis of cotton fibre quality and yield-related traits in a Gossypium hirsutum recombinant inbred line population. Plant Biotechnol. J. 18, 239–253.
Zhang, Z., Li, J., Muhammad, J., Cai, J., Jia, F., Shi, Y., et al. (2015). High resolution consensus mapping of quantitative trait loci for fiber strength, length and micronaire on chromosome 25 of the upland cotton (Gossypium hirsutum L.). PLoS One 10:e0135430. doi: 10.1371/journal.pone.0135430
Zhang, Z., Shang, H., Shi, Y., Huang, L., Li, J., Ge, Q., et al. (2016). Construction of a high-density genetic map by specific locus amplified fragment sequencing (SLAF-seq) and its application to Quantitative Trait Loci (QTL) analysis for boll weight in upland cotton (Gossypium hirsutum). BMC Plant Biol. 16:79.
Zhou, M., Zhang, K., Sun, Z., Yan, M., Chen, C., Zhang, X., et al. (2017). LNK1 and LNK2 corepressors interact with the MYB3 transcription factor in phenylpropanoid biosynthesis. Plant Physiol. 174, 1348–1358.
Zou, X., Gong, J., Duan, L., Jiang, X., Zhen, Z., Fan, S., et al. (2018). High-density genetic map construction and QTL mapping for fiber strength on Chr24 across multiple environments in a CCRI70 recombinant inbred lines population. Euphytica 214:102. doi: 10.1007/s10681-018-2177-4
Keywords: Gossypium hirsutum, RIL, fiber quality, quantitative trait loci, RNA-seq
Citation: Jiang X, Gong J, Zhang J, Zhang Z, Shi Y, Li J, Liu A, Gong W, Ge Q, Deng X, Fan S, Chen H, Kuang Z, Pan J, Che J, Zhang S, Jia T, Wei R, Chen Q, Wei S, Shang H and Yuan Y (2021) Quantitative Trait Loci and Transcriptome Analysis Reveal Genetic Basis of Fiber Quality Traits in CCRI70 RIL Population of Gossypium hirsutum. Front. Plant Sci. 12:753755. doi: 10.3389/fpls.2021.753755
Received: 05 August 2021; Accepted: 11 November 2021;
Published: 16 December 2021.
Edited by:
Linghe Zeng, United States Department of Agriculture (USDA), United StatesReviewed by:
Jinfa Zhang, New Mexico State University, United StatesCopyright © 2021 Jiang, Gong, Zhang, Zhang, Shi, Li, Liu, Gong, Ge, Deng, Fan, Chen, Kuang, Pan, Che, Zhang, Jia, Wei, Chen, Wei, Shang and Yuan. This is an open-access article distributed under the terms of the Creative Commons Attribution License (CC BY). The use, distribution or reproduction in other forums is permitted, provided the original author(s) and the copyright owner(s) are credited and that the original publication in this journal is cited, in accordance with accepted academic practice. No use, distribution or reproduction is permitted which does not comply with these terms.
*Correspondence: Youlu Yuan, eXVhbnlvdWx1QGNhYXMuY24=; Haihong Shang, c2hhbmdoYWlob25nQGNhYXMuY24=; Shoujun Wei, d2Vpc2hvdWp1bkBjYWFzLmNu; Quanjia Chen, Y2hxamlhQDEyNi5jb20=
†These authors have contributed equally to this work
Disclaimer: All claims expressed in this article are solely those of the authors and do not necessarily represent those of their affiliated organizations, or those of the publisher, the editors and the reviewers. Any product that may be evaluated in this article or claim that may be made by its manufacturer is not guaranteed or endorsed by the publisher.
Research integrity at Frontiers
Learn more about the work of our research integrity team to safeguard the quality of each article we publish.