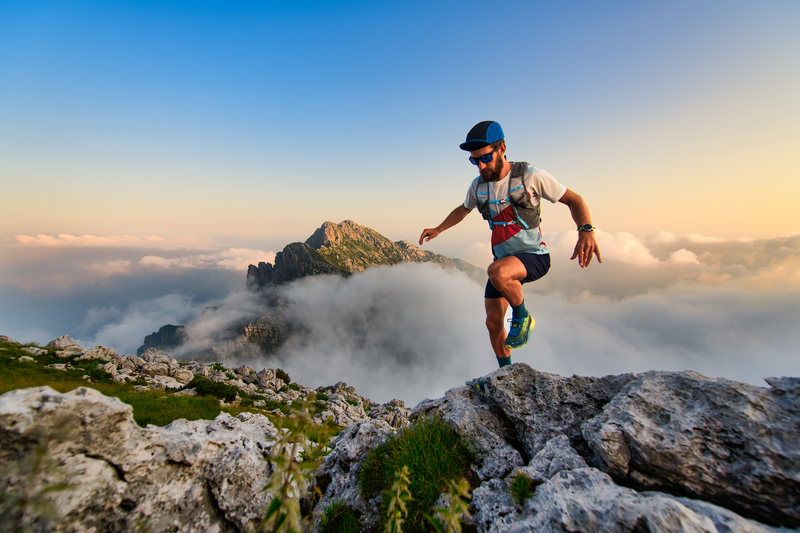
95% of researchers rate our articles as excellent or good
Learn more about the work of our research integrity team to safeguard the quality of each article we publish.
Find out more
REVIEW article
Front. Plant Sci. , 23 December 2021
Sec. Plant Biophysics and Modeling
Volume 12 - 2021 | https://doi.org/10.3389/fpls.2021.747142
This article is part of the Research Topic Virtual plants: Modeling plant architecture in changing environments, Volume II View all 7 articles
Functional–structural plant models (FSPMs) have been evolving for over 2 decades and their future development, to some extent, depends on the value of potential applications in crop science. To date, stabilizing crop production by identifying valuable traits for novel cultivars adapted to adverse environments is topical in crop science. Thus, this study will examine how FSPMs are able to address new challenges in crop science for sustainable crop production. FSPMs developed to simulate organogenesis, morphogenesis, and physiological activities under various environments and are amenable to downscale to the tissue, cellular, and molecular level or upscale to the whole plant and ecological level. In a modeling framework with independent and interactive modules, advanced algorithms provide morphophysiological details at various scales. FSPMs are shown to be able to: (i) provide crop ideotypes efficiently for optimizing the resource distribution and use for greater productivity and less disease risk, (ii) guide molecular design breeding via linking molecular basis to plant phenotypes as well as enrich crop models with an additional architectural dimension to assist breeding, and (iii) interact with plant phenotyping for molecular breeding in embracing three-dimensional (3D) architectural traits. This study illustrates that FSPMs have great prospects in speeding up precision breeding for specific environments due to the capacity for guiding and integrating ideotypes, phenotyping, molecular design, and linking molecular basis to target phenotypes. Consequently, the promising great applications of FSPMs in crop science will, in turn, accelerate their evolution and vice versa.
Global human population is growing rapidly and has been estimated to reach nearly 10 billion by 2050. However, overall crop production at current rate is insufficient for such great population (Ray et al., 2013). Undoubtfully, the growing population requires extra food supply as well as high food quality, which is in a conflict with shrinking availability of farmland due to industrialization and urbanization (Karki et al., 2013). Concomitantly, climate change is alarming and causing more droughts, heat shocks, and floods, which may further compromise crop productivity and grain quality (Altieri and Nicholls, 2017; Webber et al., 2018; Ababaei and Chenu, 2020). Accordingly, both the growing population and climate change constitute a roadblock in ensuring food security, which urges to increase crop productivity under likely harsher environments in a sustainable way.
To address such challenges, efforts in breeding have been attempted to develop novel high-yielding varieties under unfavorable environments along with improved agronomic managements (Henry and Nevo, 2014). However, the efforts have been hindered by the complex traits in controlling high yielding and quality under abiotic stresses (Hammer et al., 2005). Therefore, the adoption of new techniques and tools, e.g., plant/crop growth models in dissecting complex traits into secondary traits that can be related to specific morphophysiological pathways and genes, is important in tackling such challenges in crop production systems (Hammer et al., 2006; Tardieu and Tuberosa, 2010; Rebolledo et al., 2015). Such models based on concepts rooted in robust system biology and open frameworks that allow integrating knowledge of plant behaviors and research hypothesis (Yin and Struik, 2010; Hammer et al., 2016) and will be particularly useful for studying the interaction of genotypes and environments (G × E) precisely and decoding complex traits (Messina et al., 2015). For instance, crop/plant models are shown with a great capacity in realizing such aims (Hammer et al., 2006, 2010; Letort et al., 2008). Nevertheless, plant architectural or related traits, e.g., leaf/root three-dimensional (3D) characteristics are not often taken into account in crop models though leaf area and leaf area index that are key determinants in such models.
On the other hand, as for the architectural trait, it is one of key drives in functional–structural plant models (FSPMs). The concept and definition of FSPMs have been clearly described in many places (Vos et al., 2010; DeJong et al., 2011; Sievänen et al., 2014). Hence, in this study, we describe the model in brief only. FSPMs are dedicated in the simulation of both the plant architectural development and physiological activities at a resolution of individual organs under specific environments (Table 1) (Yan et al., 2004; Allen et al., 2005), originally derived from plant architectural models (De Reffye et al., 1988; Prusinkiewicz et al., 1988; Prusinkiewicz, 1998). For example, GreenLab (Hu et al., 2003), a typical FSPM, was initially tested for the key algorithms in biomass allocation (Song et al., 2003a) and morphological construction (Song et al., 2003b) for maize and was further developed with a systematic integration of interactive modules, i.e., developmental, biomass growth and partitioning, and architectural development and visualization (Yan et al., 2004; Guo et al., 2006); subsequently, the model was further generalized (Kang et al., 2008a) and widely applied to other crops (Dong et al., 2007; Kang et al., 2008b, 2012; Jullien et al., 2011). Simultaneously, many other FSPMs, model platforms, and tools have emerged worldwide (Fournier and Andrieu, 1999; Prusinkiewicz et al., 2000; Allen et al., 2005; Evers et al., 2006; Kniemeyer and Kurth, 2008; Pradal et al., 2008) with a particular focus on plant architectural development for diverse crops. In essence, FSPM has a robust physiological × architectural interaction at organ level in response to various environments (Hanan, 1997; Yan et al., 2004; Vos et al., 2010; El-Sharkawy, 2011; Henke et al., 2016; Postma et al., 2017; Schnepf et al., 2018; Zhou et al., 2020).
Table 1. The list of functional–structural plant models/platforms, brief description of characteristics, and basic functions.
It is noteworthy that FSPM framework is built on the multipurpose and multidisciplinary knowledge of structural and functional interactions on an organ level (Figure 1) and has been successfully applied for many plants under various environmental conditions, assisting in dealing with sustainable food production (Tardieu, 2003; Hanan and Prusinkiewicz, 2008; Evers et al., 2010; Vos et al., 2010; Guo et al., 2011). As a consequence, they have great potentials to attract more attention from scientists in various disciplines and can be the center of interest of debates in overcoming challenges arisen from the practice of crop production (Evers et al., 2018). Thus, in this study, we highlight the robust concepts of FSPMs with ecophysiological functions of a structural phytomer, flexible in allowing integration of disciplines for down- or upscaling. We then further illustrate unique potential roles of FSPMs in overcoming great challenges in sustaining crop productivity under environmental stresses. The following sections will demonstrate the role of FSPMs in: (i) assisting to design crop ideotypes with optimal use of resources, (ii) enhancing crop modeling ability by assisting to link phenotypes to genotypes, (iii) improving the efficiency and accuracy of molecular breeding, and (iv) guiding plant phenotyping for efficient breeding. Integration of favorable ideotype identification, traits discovery, and the reduction of the gap between phenotypes and genotypes collectively contribute to developing new cultivars for stable and sustainable production under adverse environments.
Figure 1. The schematic diagram of plant architecture and functional activities on the basis of individual organs for FSPMs downscaled to the cellular function or upscaled to the ecological function via leaf photosynthesis. Leaf photosynthesis can be decoded as a collection of cellular chloroplast photosynthesis per unit that can adopt leaf C3/C4 biochemical models (Farquhar et al., 1980) regulated by molecular network (Wu et al., 2016); while for field level, the estimation of grain yield from leaf photosynthesis is the intercepted light by the canopy as a function of LAI multiplying with LUE and HI. Leaf photosynthesis acts as a nexus in connecting cellular and molecular level to field level modeling. The curve shows net photosynthetic rate as a function of incident irradiance, CO2, H2O, and temperature. From left to right, the upscale from molecular to ecological level or vice versa for downscale from right to left. FSPMs, functional–structural plant models; LAI, leaf area index; LUE, light use efficiency; HI, harvest index.
A crop ideotype, originally defined by Donald (1968), is the combination of collective elite traits that may control crop growth and development, grain yield, and stress tolerance in specific environments (Qi et al., 2010; Andrivon et al., 2012; Rötter et al., 2015). Conventional breeding efforts depend on experienced breeders to combine the alleles in tedious and time-consuming field trials. However, the FSPM can provide in silico plants that aid to conduct virtual trials in achieving theoretical ideotypes by adjusting any combination of traits (Tardieu, 2003; Song et al., 2013; Picheny et al., 2017) and testifying them by rigorous field trials.
Functional–structural plant model requires a supply of resources including irradiance, nutrients, and H2O as a fuel and building materials for organ kinetics and morphophysiological activities as well as comprehending how to manipulate such resources in 3D development and ecophysiology of each phytomer precisely for optimal plant architecture (Ourry et al., 2001). Further, resource distribution within the plant confines many aspects of crop growth and development and grain yield formation as well as the risk of disease infection. Consequently, the crop performance is regulated considerably by the way crop interacts with the environments involved in the processes that lead to final product formation and quality.
One of prime features in FSPM is a plant composed of a network of structural units such as axes, internodes, leaf tips, and axillary buds (Vos et al., 2010; Sievänen et al., 2014). This offers the possibility to study and model the development and functioning of each metamer/organ and the interaction of each metamer via resource competition. Being a prerequisite factor for plant photosynthetic process, light absorption/interception is one of the important factors in determination of crop yield. The introduction of modeling paradigm that focuses on the spatial design of plant architectural traits and their development gives the opportunity to explore light absorption and photosynthesis for each structural element, biomass partitioning, and grain yield (Chelle and Andrieu, 1998; Cournède et al., 2008; Sarlikioti et al., 2011b; Da Silva et al., 2014; Sievänen et al., 2014; Christensen et al., 2018). In this context, Sarlikioti et al. (2011a) performed simulations with FSPM of tomato crop to define plant ideotype for optimal light distribution, absorption, and canopy photosynthesis. This study defined two ideotypes scenarios that exhibited an increase in light absorption, resultantly higher canopy photosynthesis, which, in turn, may potentially lead to an enhanced yield. Interestingly, they identified that internode length and leaf shape are the most essential architectural traits to be manipulated in optimizing light absorption. This demonstrates that plant architectural information may have significant importance in modern breeding to design genotypes with respect to efficient light absorption and canopy photosynthesis (Sarlikioti et al., 2011b).
Furthermore, the importance and implication of plant architecture in the identification of plant ideotypes with respect to light partitioning capacity in a crop mixture is highlighted (Barillot et al., 2014). In fact, in this study, the authors developed a deterministic model of pea (L-pea) with modules for vegetative topological development and organ dynamics, linked it with Architectural Model of Development Based on L-systems (ADEL)-Wheat (Fournier et al., 2003) in a common L-system platform, and applied the model to a cropping system of pea and wheat to assess light partitioning. Results illustrated that quantitative variation of architectural traits is a determining factor for light partitioning (Barillot et al., 2019) and that in intercropping systems (such as wheat and pea), light capture is principally linked to architectural characteristics. Such findings can facilitate the design of crop genotypes adapted to intercropping by capturing morphological traits that can be incorporated into modern breeding programs (Louarn et al., 2020). These studies demonstrated how far beyond can FSPMs provide a finer insight of light absorption and partitioning within plant canopy and also deliver tools that help to establish a fine set of architectural traits for maximizing canopy photosynthesis, allocation of assimilates to growing organs, and ultimately crop yield (Sarlikioti et al., 2011b; Teichmann and Muhr, 2015). Apart from abiotic environments, pea architectural details are reported to affect spatiotemporal epidemic development for Ascochyta blight (Le et al., 2009) and an ideotype with the combinations of optimal architectural traits is shown to minimize the epidemic development of pests and diseases in crops (Andrivon et al., 2012).
In addition to shoot ideotypes, root ideotypes have been explored in maximizing the uptake of resources in the soil. A “steep, cheap, and deep” ideotype with the ability of optimizing the acquisition of water and nitrogen was proposed by Lynch (2013). An ideotype of root system for efficient nitrogen acquisition in intensive cropping system was proposed by Mi et al. (2010) and further updated with more detailed root architecture including root branching, angle, and distribution (Mi et al., 2016). A novel irrigated ideotype with high resource use efficiency was proposed by Schmidt and Gaudin (2017). The functional–structural plant modeling has been applied to identify the ideotype of root system drought resistance for breeding (Ndour et al., 2017).
The modern molecular breeding (Moose and Mumm, 2008) with the guidance of crop design by employing the knowledge and tools arisen from contemporary functional genomics is fairly effective for breeding new cultivars (Hammer et al., 2016). A considerable literature has been dedicated to understanding how crop/plant modeling could help to decode complex traits for guiding molecular breeding (Hammer et al., 2005; Yin et al., 2005; Chapman, 2008). It is an extended form of the standard breeding approach by the prediction of genotypic breeding. As such, it allows the breeding procedure to be simulated and optimized prior to being tested in the field, thus increasing breeding efficiency and predictability (Hammer et al., 2006, 2016; Wan, 2006). Designing superior crop cultivars would be affordable for breeders due to genetic basis of agronomically important traits and allelic variations at those loci made available (Wan, 2006; Wang et al., 2011).
The framework and concept of FSPMs to represent the plant as a network of elementary units, i.e., phytomers and their structural–functional feedback, provide great opportunities to comprehend plant biological organization from molecular level to whole plant (Figure 1) (Hanan and Prusinkiewicz, 2008). There are possibilities to connect the whole plant trait to fundamental biology via FSPMs in accordance with the behavior of the entire plant systems biology (Letort et al., 2008; Xu and Buck-Sorlin, 2016). Molecular design has been attracting great interest in plant breeding programs (Wang et al., 2011). The link up of a given model measurable trait and tangible quantitative trait loci (QTL) is the key fact that makes crop models or FSPMs an integral tool for crop molecular genetics research and breeding (Tardieu et al., 2005; Quilot et al., 2006; Letort et al., 2008; Semenov and Halford, 2009). Accordingly, Xu et al. (2011) developed a model system of rice that represents plant structural kinetics in combination with ecophysiological processes using FSPMs and interactive modeling platform Growth Grammar-related Interactive Modelling Platform (GroIMP) (Kniemeyer and Kurth, 2008) along with the graph-based relational growth grammar formalism (Kurth et al., 2004), which is an extended L-system formalism. This prototype constitutes the first effort of a model system of rice FSPMs that will prominently integrate information on QTLs, environments, and their interactions in a network. This could help further for designing molecular specific traits in crop systems biology or in breeding. Plant under water stress has different responses underlined by various physiological processes that could account for emergent behavior. Associating alleles with particular responses will help to identify alleles for maintaining growth under stress (Tardieu et al., 2005). Leaf elongation rate depends on environmental variables, e.g., temperature, evaporative demand, and soil water status, so QTLs for these variables were established, enabling to predict the responses to different climatic conditions. The identification of QTLs in this study offers opportunities for improving drought adoption mechanisms via molecular breeding to design and assess traits that were elusive in previous selection study.
Overall, as a mechanistic and comprehensive tool, the FSPM can be used in molecular breeding work to assist in the design of new plant prototype. They will be for sure embrace the system design in addition to the synthesis of data and prediction of quantitative behavior, as proposed by Yin and Struik (2008) for future modeling of crop systems biology.
Crop models are usually capable in predicting crop phenology, biomass, and grain yield under various soil and climate conditions including abiotic stresses (Jones and Kiniry, 1986; Sinclair and Seligman, 1996; Wang et al., 2019). Initially, such models have been employed to assist crop management in a farming system with a simplification of plant architectural details. The model design based on robust physiological principles is in accordance with the systems biology (Hammer et al., 2005; Yin and Struik, 2009), e.g., Genotype-by-Environment Interaction on Crop Growth Simulator (GECROS) (Yin and van Laar, 2005). Crop models are shown to be promising to connect with molecular level mechanisms in assisting plant breeding for complex traits, e.g., drought tolerance (Hammer et al., 2005; Chapman, 2008). For example, a linkage between crop models and leaf biochemistry models has been proposed to reflect the adjustment of biochemical reaction in grain yield for crop improvement (Wu et al., 2016; Yin et al., 2018). Often, leaf area index is required in driving crop photosynthesis and productivity in modern crop models such as Decision Support System for Agrotechnology Transfer (DSSAT) (Jones et al., 2003) and Agricultural Production Systems Simulator (APSIM) (Hammer et al., 2010). Detailed root architecture is essential in investigating water or nutrient absorption under abiotic stresses (Fang et al., 2009; Hammer et al., 2009). Thus, the resolution of representing plants at organ level is helpful to enhance the capacity of crop modeling for the precise description of a plant.
Functional–structural plant models have been developed with a particular focus on a delicate description of plant structure, initially known as plant architectural models or virtual plants (De Reffye et al., 1988; Barthelemy and Caraglio, 2007). FSPMs add a structural dimension to conventional crop models (Vos et al., 2007). Upon the advent of FSPMs, supply fine details of plant architecture is reinforced for likely use in crop models (Wernecke et al., 2000; Vos et al., 2007; Fourcaud et al., 2008; Feng et al., 2014). For example, the GreenLab model (Hu et al., 2003; Yan et al., 2004; Kang et al., 2008a) takes fundamental ecophysiology in calculating biomass production and partitioning and links physiology with architectural models (Slavíková, 1980) for more precise prediction of crop production (De Reffye et al., 2009). Further, this model has been calibrated for many plants and has been shown to be able to accurately reproduce plant growth and architecture with phenotypic plasticity (Dingkuhn et al., 2005).
In earlier days even when crop models reached maturity (Sinclair and Seligman, 1996), high resolution of canopy architecture is not necessary and time-consuming in farming-scale simulations. Given fundamental biological functions closely associated with plant architecture, the description of shoot and root architecture may be valuable in crop models. Thus, it deserves more attention to improve the resolution of canopy architectural details, allowing matching the heterogeneity of environmental resources required for precision computation of crop productivity and design in molecular breeding with crop models (Dai et al., 2004; Evers et al., 2010; Yin et al., 2018).
Assessment of qualitative and quantitative traits rapidly, known as plant phenotyping (Granier and Devis, 2014), helps to explore functional diversities or performances of different plants in given environmental conditions. Plant phenotyping calls for strategies that include collecting data from the experiment and submitting those data to a crop/plant model that will provide predicted crop traits (Messina et al., 2015). The models should be, therefore, able to simulate G × E interactions and the resulting phenotypic plasticity (Dingkuhn et al., 2005). Within the context, Luquet et al. (2006) developed EcoMeristem, a FSPM explicitly conceived to simulate rice crop phenotypic plasticity on the basis of meristem behavior and associated adjustment processes (Dingkuhn et al., 2005). Luquet et al. (2012a) illustrated the practicability of the model while using it to explore the phenotypic and genetic diversity of early vigor and drought regulation in rice. In their study, according to optimized parameters, the model accurately simulated plant leaf area, plant height, and shoot dry weight under both the well-watered and drought conditions. This shows the capacity of the model to reproduce the behavior of morphophysiological traits. Model parameters can provide phenotypic data less noised by “genotype by environment interaction,” as the parameters showed less replicate effect compared to corresponding measurements (Rebolledo et al., 2012). In this context, a theoretical study based on linking parameter values of GreenLab (Yan et al., 2004; Guo et al., 2006) to hypothetical genes was done by Letort et al. (2008). This study simulated the virtual phenotypes resulting from hybridization of homozygous parents, showing that this virtual phenotype resulted from population cross could be used in QTL identification for further breeding use. Therefore, for building new ideotype concepts of phenotyping, FSPM approaches are needed because they help to integrate knowledge of physiology, explicit organ 3D characteristics, and genetics via such a bridge between plant science and functional genomics.
Functional–structural plant models are equipped with interactive modules built for precisely exploring plant morphogenesis, development, and growth in the context of environmental cues. They are specifically valuable in synthesizing research understanding and integrating discipline knowledge to generate tools with descriptive and mechanistic potentials (DeJong et al., 2011; Sievänen et al., 2014; Louarn and Song, 2020). Owing to their multidisciplinary characteristics, FSPMs are based on concepts, tools, and frameworks that emanate from various disciplines, thus the development of FSPMs involves scientists with a wide range of backgrounds including crop physiologists, plant biologists, plant ecologists, computer scientists, and agronomists, etc (Figure 1).
A modeling study with FSPMs may generate massive data at different scales, thus managing these data constitutes a new challenge for modelers (DeJong et al., 2011). Therefore, the integration of data acquisition techniques involving laser scanning, confocal laser imaging, and X-rays, underlined by remote sensing approach, led to the design of “3D” FSPMs that work at various spatial-temporal scales. Based on laser scanning technology, Boudon et al. (2014) developed algorithms for automatic identification of plant elementary units, further used to parameterize FSPMs and evaluate them, according to accurate and real generated data. Hakala et al. (2012) showed that the feasibility of analyzing spectral characteristics of the Light Detection and Ranging (LiDAR) 3D point clouds generates future prospect in FSPMs for identification of plant parts and their physiological conditions. The development of FSPMs provides platforms for computational modeling that depends on appropriate software and programming languages (Sievänen et al., 2014). For example, as established concepts are frequently adjusted to provide new approaches in modeling studies, L-system was an open tool and concept (Prusinkiewicz and Lindenmayer, 1990) with a multi-modules system for integrating previous modeled aspects of carbon dynamics (Allen et al., 2005), apical dominance (Prusinkiewicz et al., 2009), and biomechanics (Taylor-Hell, 2005; Prusinkiewicz et al., 2007) into a well-structured FSPM (Cieslak et al., 2011). This is illustrated in the study of Ong et al. (2014), where the programming language XL and GroIMP platform have been used to explore models of plant growth that allow appropriately the use of several structural scales in plant description, highlighting the multi-scalar potentials of FSPMs. The result pointed out 3 contrasting models that show the way for combining information from various scales in the models. These are top-down, bottom-up, and within a range of scales from microscopic cell-level process to macroscopic level of plants. The integration of discipline knowledge, techniques, and concepts for the development of FSPMs and explicit tools for usage beyond the individual discipline could produce user-oriented multifaceted models for application in studying complex systems (Boote et al., 1996; Sievänen et al., 2014).
Functional–structural plant models have been deployed to comprehend morphological, physiological, and biological processes that drive development, growth, and yield formation of crops in various environmental conditions and to simulate the consequences of crops × environments including the effect of biotic and abiotic stresses (Hanan and Prusinkiewicz, 2008; Sievänen et al., 2014). FSPMs offer considerable potentials for tackling current challenges including food security for greater human population and sustainability in the context of biotic/abiotic stress due to climate change (Chapman, 2008; Wang et al., 2019). Inherently, one of the greatest bottlenecks in crop production is managing biotic and abiotic factors that significantly reduce crop production (Maiti and Pratik, 2014). The usefulness of FSPMs at tackling these issues has been demonstrated (Garin et al., 2014; Gigot et al., 2014). For example, the drought stress occurring at crop establishment stage has been deleterious to rice crops (Courtois et al., 2000). The only way to alleviate that is for the plant to acquire sufficient resources and avoid soil evaporation and weed rivalry (Zhao et al., 2006). This is termed as “early vigor,” which confers drought avoidance ability in rice crops (Zhang et al., 2005). As the FSPM allows formalizing integratively, the genetic (G) × environment (E) bases of elemental process-based traits and their linkages, it was able to simulate genetic diversity of rice early vigor and its drought regulation (Luquet et al., 2012b). FSPM concepts were applied in the EcoMeristem (Luquet et al., 2006) to investigate the existence of negative linkages between the capacity of proper plant establishment and its drought tolerance (Luquet et al., 2012b). Indeed, those identified negative linkages could be attributed to the variation of resources per se and also the reaction of sink activities to available resource. The result of this study would eventually help rice breeders to better co-select early vigor and drought tolerance traits (Luquet et al., 2012a).
In a former study, a modeling framework was produced to simulate foliar fungal epidemics based on the OpenAlea platform (Pradal et al., 2008). This study is designed by implementation of two different pathosystems and yielded the simulation of the effect of canopy structural traits on fungal dissipation. This paves the way for modeling the complex dynamics of crop pathosystems for a good understanding of interactions that will probably make better protective strategies (Garin et al., 2014). The study by Gigot et al. (2014) proposed as a strategy for managing splash-dispersed fungal pathogen in wheat to define cultivar (whether sensible or tolerant) proportion as a function of host resistance capability. FSPM technique used in this study referred to a virtual 3D plant model, integrated to a module that predicts splash droplet dispersion of the fungal pathogen and the host resistance in wheat. This highlights how FSPMs, through its spatial-temporal characters, can make itself useful for understanding issues related to the dissipation of disease within plants.
After more than 2 decades of evolution of FSPM (Vos et al., 2010; Louarn and Song, 2020; De Reffye et al., 2021), the model has become widely known due to the continuous efforts from the pioneers in both the plant architectural modeling and functional–structural plant modeling community (Prusinkiewicz et al., 1988; Hanan, 1997; Hu et al., 2003; Godin and Sinoquet, 2005). The models have achieved great success in algorithms and prototypes for different plants or crops under various environments, receiving more attention nowadays and in future (Louarn and Song, 2020). The above paragraphs also demonstrated the great capacity for FSPMs in addressing the challenges in crop science. Despite this, we have identified constraints in both the modelization and practical aspects that may limit the potentials of extensive applications for FSPMs.
First of all, it is known that the major strength in FSPMs is the fine simulation of explicit plant morphogenesis, 3D architecture, and architectural development; nevertheless, the key functional parts in many FSPMs are, to some extent, based on or adopted from the physiological processes used in conventional crop models, in particular, in the beginning when to illustrate the role of FSPMs by integrating both the plant architecture and physiological functions (De Reffye et al., 2009), which is still widely used. For example, the estimation of canopy photosynthesis in many FSPMs is based on empirical light extinction within the canopy as a function of leaf area index (Vos et al., 2010; Pao et al., 2021) rather than mechanistic interaction of irradiance with individual leaves, though there are many studies available in investigating such interaction (Buck-Sorlin et al., 2011; Sarlikioti et al., 2011b). In addition, modeling transfer of incident light energy to the chemical energy in the form of carbohydrate in leaves can be realized by mechanistically biochemical model of leaf photosynthesis (Farquhar et al., 1980; Wu et al., 2016). Hence, the mechanistic process of carbohydrate should be introduced in the novel model stage. Taken another example, there is attempt in mathematically simulating biomass allocation among individual growing organs (Kang et al., 2008b; Reyes et al., 2019). However, the biomass flow into the sink governed by fundamental cellular activities is rarely studied. As FSPMs are maturing, it is time and necessary to mimic the mechanistic, physiological process rooted from an organ activity, which will be desirable and boosted in future development. It needs to dismantle the integrative sink strength into fundamental cellular activities driven by sucrose unloading and following sucrose degraded into glucose and fructose, which is regulated by a series of enzymes and genes (Ruan, 2014). Consequently, the participation from crop/plant scientists and a closer collaboration between model developers and those field scientists should be more encouraged for model development and practice, though the models have been initially developed by joint efforts from mathematicians, modelers, and computer scientists as well as with the participation of agroforestry scientists.
Practically, as the model considers both the fundamental biological processes and plant architecture, even visualization, it will require substantial computation time. We got to admit that the computation power has made great progress over the last decades. Nonetheless, it is still a major concern for the models applied to the complicated system in practice by integrating details including soil and atmosphere environments and crops. To address this, for instance, the visualization is made in separate rendering program depending on if it is required, e.g., GreenLab (Kang et al., 2008a,b). In addition, to facilitate the application, it is essential to have a user-friendly interface and practice the software or tools without knowing much about underlying model algorithms. At the current stage, the use of models is not easy for users in crop science who are not with fairly good backgrounds of FSPMs. It takes a while to train new users about how to use tools and software.
Crop science is confronted with the challenge for substantial improvement of crop productivity under climate change for increased human population. This requires elite cultivars tolerant to adverse environments to be bred. The ideotypes, traits, phenotypes, and molecular design breeding were integrated in a system via FSPMs for more efficient breeding. FSPM, by tracing organ kinetics, microenvironments, and their interactions, enables to understand and explore how the complex crop system work, which allows the model to be downscaled to the molecular level or upscaled to the plant community in a faithful way to the systems biology. Also, FSPMs may be envisaged to generate more substantial details arisen from the analysis of genotypic and environmental interaction at different scales. FSPMs provide algorithms, platforms, and tools in advancing the frontiers in crop science from molecular design to phenotypic-guided breeding, by which, sustainable crop production under adverse environments may be achieved. On the other hand, the existing and promising applications in advancing crop science will result in the evolution of FSPMs. Though the attempt for FSPMs applied to advancing the frontiers in crop science has been demonstrated, there is still much more study to be done in fulfilling the potentials. This includes bringing together scientists in different disciplines to work closer than ever in guiding molecular design for precise breeding via FSPMs.
SS drafted the manuscript. ZW and WS helped in manuscript draft. PR advised the manuscript. BC improved the writing. GL and YS conceived the idea and finalized the manuscript. All authors contributed to the article and approved the submitted version.
The study was financially supported by the National Key R&D Program of China (Grant nos. 2017YFD0301307 and 2017YFD0300204-3).
The authors declare that the research was conducted in the absence of any commercial or financial relationships that could be construed as a potential conflict of interest.
All claims expressed in this article are solely those of the authors and do not necessarily represent those of their affiliated organizations, or those of the publisher, the editors and the reviewers. Any product that may be evaluated in this article, or claim that may be made by its manufacturer, is not guaranteed or endorsed by the publisher.
We are much appreciated for the two reviewers for their constructive comments.
Ababaei, B., and Chenu, K. (2020). Heat shocks increasingly impede grain filling but have little effect on grain setting across the Australian wheat belt. Agric. For. Meteorol. 284:107889. doi: 10.1016/j.agrformet.2019.107889
Allen, M. T., Prusinkiewicz, P., and DeJong, T. M. (2005). Using L-systems for modeling source-sink interactions, architecture and physiology of growing trees: the L-PEACH model. New Phytol. 166, 869–880. doi: 10.1111/j.1469-8137.2005.01348.x
Altieri, M. A., and Nicholls, C. I. (2017). The adaptation and mitigation potential of traditional agriculture in a changing climate. Clim. Change 140, 33–45. doi: 10.1007/s10584-013-0909-y
Andrivon, D., Giorgetti, C., Baranger, A., Calonnec, A., and Sache, I. (2012). Defining and designing plant architectural ideotypes to control epidemics? Eur. J. Plant Pathol. 135, 611–617. doi: 10.1007/s10658-012-0126-y
Barillot, R., Chambon, C., and Andrieu, B. (2016). CN-Wheat, a functional–structural model of carbon and nitrogen metabolism in wheat culms after anthesis. I. Model description. Ann. Bot. 118, 997–1013. doi: 10.1093/aob/mcw143
Barillot, R., Chambon, C., Fournier, C., Combes, D., Pradal, C., and Andrieu, B. (2019). Investigation of complex canopies with a functional- structural plant model as exemplified by leaf inclination effect on the functioning of pure and mixed stands of wheat during grain filling. Ann. Bot. 123, 727–742. doi: 10.1093/aob/mcy208
Barillot, R., Escobar-Gutiérrez, A. J., Fournier, C., Huynh, P., and Combes, D. (2014). Assessing the effects of architectural variations on light partitioning within virtual wheat–pea mixtures. Ann. Bot. 114, 725–737. doi: 10.1093/aob/mcu099
Barthelemy, D., and Caraglio, Y. (2007). Plant architecture: a dynamic, multilevel and comprehensive approach to plant form, structure and ontogeny. Ann. Bot. 99, 375–407. doi: 10.1093/aob/mcl260
Bertheloot, J., Wu, Q., Cournède, P. H., and Andrieu, B. (2011). NEMA, a functional-structural model of nitrogen economy within wheat culms after flowering. II. Evaluation and sensitivity analysis. Ann. Bot. 108, 1097–1109. doi: 10.1093/aob/mcr125
Boote, K. J., Jones, J. W., and Pickering, N. B. (1996). Potential uses and limitations of crop models. Agron. J. 88:704. doi: 10.2134/agronj1996.00021962008800050005x
Boudon, F., Preuksakarn, C., Ferraro, P., Diener, J., Nacry, P., Nikinmaa, E., et al. (2014). Quantitative assessment of automatic reconstructions of branching systems obtained from laser scanning. Ann. Bot. 114, 853–862. doi: 10.1093/aob/mcu062
Buck-Sorlin, G., de Visser, P. H, Henke, M., Sarlikioti, V., van der Heijden, G. W., Marcelis, L. F., et al. (2011). Towards a functional–structural plant model of cut-rose: simulation of light environment, light absorption, photosynthesis and interference with the plant structure. Ann. Bot. 108, 1121–1134. doi: 10.1093/aob/mcr190
Chapman, S.. (2008). Use of crop models to understand genotype by environment interactions for drought in real-world and simulated plant breeding trials. Euphytica 161, 195–208. doi: 10.1007/s10681-007-9623-z
Chelle, M., and Andrieu, B. (1998). The nested radiosity model for the distribution of light within plant canopies. Ecol. Modell. 111, 75–91. doi: 10.1016/S0304-3800(98)00100-8
Christensen, A. J., Srinivasan, V., Hart, J. C., and Marshall-Colon, A. (2018). Use of computational modeling combined with advanced visualization to develop strategies for the design of crop ideotypes to address food security. Nutr. Rev. 76, 332–347. doi: 10.1093/nutrit/nux076
Cieslak, M., Seleznyova, A. N., Prusinkiewicz, P., and Hanan, J. (2011). Towards aspect-oriented functional-structural plant modelling. Ann. Bot. 108, 1025–1041. doi: 10.1093/aob/mcr121
Cournède, P. H., Mathieu, A., Houllier, F., Barthélémy, D., and de Reffye, P. (2008). Computing competition for light in the GREENLAB model of plant growth: a contribution to the study of the effects of density on resource acquisition and architectural development. Ann. Bot. 101, 1207–1219. doi: 10.1093/aob/mcm272
Courtois, B., McLaren, G., Sinha, P. K., Prasad, K., Yadav, R., and Shen, L. (2000). Mapping QTLs associated with drought avoidance in upland rice. Mol. Breed. 6, 55–66. doi: 10.1023/A:1009652326121
Da Silva, D., Qin, L., De Buse, C., and DeJong, T. M. (2014). Measuring and modelling seasonal patterns of carbohydrate storage and mobilization in the trunks and root crowns of peach trees. Ann. Bot. 114, 643–652. doi: 10.1093/aob/mcu033
Dai, Y., Dickinson, R. E., and Wang, Y. P. (2004). A two-big-leaf model for canopy temperature, photosynthesis, and stomatal conductance. J. Clim. 17, 2281–2299. doi: 10.1175/1520-0442(2004)017andlt;2281:ATMFCTandgt;2.0.CO;2
De Reffye, P., Heuvelink, E., Guo, Y., Hu, B. G., and Zhang, B. G. (2009). “Coupling process-based models and plant architectural models: a key issue for simulating crop production,” in Crop Modeling and Decision Support (Berlin, Heidelberg: Springer), 130–147. doi: 10.1007/978-3-642-01132-0_15
De Reffye, P., Hu, B., Kang, M., Véronique, L., and Marc, J. (2021). Two decades of research with the Greenlab model in agronomy. Ann. Bot. 127, 281–295. doi: 10.1093/aob/mcaa172
De Reffye, P, Edelin, C., Francon, J., Jaeger, M., and Puech, C. (1988). Plant models faithful to botanical structure and development. Comput. Graphics 22, 151–158. doi: 10.1145/378456.378505
DeJong, T. M., Da Silva, D., Vos, J., and Escobar-Gutierrez, A. (2011). Using functional-structural plant models to study, understand and integrate plant development and ecophysiology. Ann. Bot. 108, 987–989. doi: 10.1093/aob/mcr257
Dingkuhn, M., Luquet, D., Quilot, B., and De Reffye, P. (2005). Environmental and genetic control of morphogenesis in crops: towards models simulating phenotypic plasticity. Aust. J. Agric. Res. 56, 1–14. doi: 10.1071/AR05063
Donald, C. M.. (1968). The breeding of crop ideotypes. Euphytica 17, 385–403. doi: 10.1007/BF00056241
Dong, Q., Louarn, G., Wang, Y., Barczi, J. F., and De Reffye, P. (2007). Does the structure-function model greenlab deal with crop phenotypic plasticity induced by plant spacing? A case study on tomato. Ann. Bot. 101, 1195–1206. doi: 10.1093/aob/mcm317
Drouet, J. L., and Pages, L. (2003). GRAAL: a model of GRowth, Architecture and carbon ALlocation during the vegetative phase of the whole maize plant. Ecol. Modell. 165, 147–173. doi: 10.1016/S0304-3800(03)00072-3
Drouet, J. L., and Pagès, L. (2007). GRAAL-CN: a model of growth, architecture and allocation for carbon and nitrogen dynamics within whole plants formalised at the organ level. Ecol. Modell. 206, 231–249. doi: 10.1016/j.ecolmodel.2007.03.036
El-Sharkawy, M. A.. (2011). Overview: early history of crop growth and photosynthesis modeling. Biosystem 103, 205–211. doi: 10.1016/j.biosystems.2010.08.004
Evers, J. B., Letort, V., Renton, M., and Kang, M. (2018). Computational botany: advancing plant science through functional-structural plant modelling. Ann. Bot. 121, 767–772. doi: 10.1093/aob/mcy050
Evers, J. B., Vos, J., Andrieu, B., and Struik, P. C. (2006). Cessation of tillering in spring wheat in relation to light interception and red: far-red ratio. Ann. Bot. 97, 649–658. doi: 10.1093/aob/mcl020
Evers, J. B., Vos, J., Yin, X., Romero, P., van der Putten, P. E. L., and Struik, P. C. (2010). Simulation of wheat growth and development based on organ-level photosynthesis and assimilate allocation. J. Exp. Bot. 61, 2203–2216. doi: 10.1093/jxb/erq025
Fang, S., Yan, X., and Liao, H. (2009). 3D reconstruction and dynamic modeling of root architecture in situ and its application to crop phosphorus research. Plant J. 60, 1096–1108. doi: 10.1111/j.1365-313X.2009.04009.x
Farquhar, G. D., von Caemmerer, S., and Berry, J. A. (1980). A biochemical model of photosynthetic CO2 assimilation in leaves of C3 species. Planta 149, 78–90. doi: 10.1007/BF00386231
Feng, L., Mailhol, J. C., Rey, H., Griffon, S., Auclair, D., and de Reffye, P. (2014). Comparing an empirical crop model with a functional structural plant model to account for individual variability. Eur. J. Agron. 53, 16–27. doi: 10.1016/j.eja.2013.11.005
Fourcaud, T., Zhang, X. P., Stokes, A., Lambers, H., and Korner, C. (2008). Plant growth modeling and applications: the increasing importance of plant architecture in growth models. Ann. Bot. 101, 1053–1063. doi: 10.1093/aob/mcn050
Fournier, C., and Andrieu, B. (1999). ADEL-maize: an L-system based model for the integration of growth processes from the organ to the canopy. Application to regulation of morphogenesis by light availability. Agron. 19, 313–327. doi: 10.1051/agro:19990311
Fournier, C., Andrieu, B., Ljutovac, S., and Saint-Jean, S. (2003). “ADEL-wheat: a 3D architectural model of wheat development [Conference presentation],” in International Symposium on Plant Growth Modeling, Simulation, Visualization and their Applications (PMA03) (Beijing, China). Available online at: https://www.researchgate.net/publication/230758120.
Garin, G., Fournier, C., Andrieu, B., Houlès, V., Robert, C., and Pradal, C. (2014). A modelling framework to simulate foliar fungal epidemics using functional-structural plant models. Ann. Bot. 114, 795–812. doi: 10.1093/aob/mcu101
Gigot, C., De Vallavieille-Pope, C., Huber, L., and Saint-Jean, S. (2014). Using virtual 3-D plant architecture to assess fungal pathogen splash dispersal in heterogeneous canopies: a case study with cultivar mixtures and a non-specialized disease causal agent. Ann. Bot. 114:863–875. doi: 10.1093/aob/mcu098
Godin, C., and Sinoquet, H. (2005). Functional-structural plant modelling. New Phytol. 166, 705–708. doi: 10.1111/j.1469-8137.2005.01445.x
Granier, C., and Devis, V. (2014). Phenotyping and beyond: modelling the relationships between traits. Curr. Opin. Plant Biol. 18, 96–102. doi: 10.1016/j.pbi.2014.02.009
Guo, Y., Fourcaud, T., Jaeger, M., Zhang, X. P., and Li, B. G. (2011). Plant growth and architectural modelling and its applications. Ann. Bot. 107, 723–727. doi: 10.1093/aob/mcr073
Guo, Y., Ma, Y., Zhan, Z., Li, B., Dingkuhn, M., Luquet, D., et al. (2006). Parameter optimization and field validation of the functional-structural model GREENLAB for maize. Ann. Bot. 97, 217–230. doi: 10.1093/aob/mcj033
Hakala, T., Suomalainen, J., Kaasalainen, S., and Chen, Y. (2012). Full waveform hyperspectral LiDAR for terrestrial laser scanning. Opt. Express 20, 7119–7127. doi: 10.1364/OE.20.007119
Hammer, G., Cooper, M., Tardieu, F., Welch, S., Walsh, B., van Eeuwijk, F., et al. (2006). Models for navigating biological complexity in breeding improved crop plants. Trends Plant Sci. 11, 587–593. doi: 10.1016/j.tplants.2006.10.006
Hammer, G., Messina, C., Oosterom, E. V., Chapman, S., and Cooper, M. (2016). “Molecular breeding for complex adaptive traits: how integrating crop ecophysiology and modelling can enhance efficiency”. in Crop Systems Biology: Narrowing the Gaps Between Crop Modelling And Genetics, eds X. Yin and P. C. Struik (Cham: Springer International Publishing), 147–162. doi: 10.1007/978-3-319-20562-5_7
Hammer, G. L., Chapman, S. C., Oosterom, E. J. V., and Podlich, D. W. (2005). Trait physiology and crop modelling as a framework to link phenotypic complexity to underlying genetic systems. Crop Pasture Sci. 56, 947–960. doi: 10.1071/AR05157
Hammer, G. L., Dong, Z., McLean, G., Doherty, A., Messina, C., Schussler, J., et al (2009). Can changes in canopy and/or root system architecture explain historical maize yield trends in the U.S. corn belt? Crop. Sci. 49, 299–312. doi: 10.2135/cropsci2008.03.0152
Hammer, G. L., van Oosterom, E., Mclean, G., Chapman, S. C., Broad, I., Harland, P., et al. (2010). Adapting apsim to model the physiology and genetics of complex adaptive traits in field crops. J. Exp. Bot. 61, 2185–2202. doi: 10.1093/jxb/erq095
Hanan, J.. (1997). Virtual plants-integrating architectural and physiological models. Environ. Model. Software 121, 35–42. doi: 10.1016/S1364-8152(96)00040-0
Hanan, J., and Prusinkiewicz, P. (2008). Foreword: studying plants with functional-structural models. Funct. Plant Biol. 35, 6–8. doi: 10.1071/FPv35n10_FO
Henke, M., Kurth, W., and Buck-Sorlin, G. H. (2016). FSPM-P: towards a general functional-structural plant model for robust and comprehensive model development. Front. Comput. Sci. 10, 1103–1117. doi: 10.1007/s11704-015-4472-8
Henry, R. J., and Nevo, E. (2014). Exploring natural selection to guide breeding for agriculture. Plant Biotechnol. J. 12, 655–662. doi: 10.1111/pbi.12215
Hu, B. G., de Reffye, P. D., Zhao, X., Yan, H-P., and Kang, M-Z. (2003). “GreenLab: A new methodology towards plant functional-structural model - structural part. [Conference presentation]”, in International Symposium on Plant Growth Modeling, Simulation, Visualization and their Applications (PMA03) (Beijing: TsingHua University Press and Springer). Available online at: https://hal.inria.fr/inria-00121235.
Jones, C. A., and Kiniry, J. R. (1986). CERES-Maize: a simulation model of maize growth and development. Agric. For. Meteorol. 41, 339–339. doi: 10.1016/0168-1923(87)90089-X
Jones, J. W., Hoogenboom, G., Porter, C. H., Boote, K. J., Batchelor, W. D., Hunt, L. A., et al. (2003). The DSSAT cropping system model. Eur. J. Agron. 18, 235–265. doi: 10.1016/S1161-0301(02)00107-7
Jullien, A., Mathieu, A., Allirand, J. M., Pinet, A., Reffye, P. D., Cournède, P. H., et al. (2011). Characterisation of the interactions between architecture and source-sink relationships in winter oilseed rape (Brassica Napus L.) using the GreenLab model. Ann. Bot. 107, 765–779. doi: 10.1093/aob/mcq205
Kang, M. Z., Cournède, P. H., de Reffye, P. D., Auclair, D., and Hu, B. G. (2008a). Analytical study of a stochastic plant growth model: application to the greenlab model. Math. Comput. Simul. 78, 57–75. doi: 10.1016/j.matcom.2007.06.003
Kang, M. Z., Evers, J. B., Vos, J., and de Reffye, P. (2008b). The derivation of sink functions of wheat organs using the GreenLab model. Ann. Bot. 101, 1099–1108. doi: 10.1093/aob/mcm212
Kang, M. Z., Heuvelink, E., Carvalho, S. M. P., and Reffye, P. D. (2012). A virtual plant that responds to the environment like a real one: the case for chrysanthemum. New Phytol. 195, 384–395. doi: 10.1111/j.1469-8137.2012.04177.x
Karki, S., Rizal, G., and Quick, W. (2013). Improvement of photosynthesis in rice (oryza satival.) by inserting the C4 pathway. Rice 6:28. doi: 10.1186/1939-8433-6-28
Karwowski, R., and Prusinkiewicz, P. (2004). “The L–system–based plant–modeling environment L–studio 4.0 [Conference presentation],” in 4th International Workshop on Functional–Structural Plant Models (Montpellier, France). Available online at: http://amap.cirad.fr/workshop/FSPM04/index.html
Kniemeyer, O., and Kurth, W. (2008). “The modelling platform GroIMP and the programming language XL”, in Applications of Graph Transformations with Industrial Relevance, eds A. Schürr, M. Nagl, and A. Zündorf (Berlin, Heidelberg: Springer), 570–572. doi: 10.1007/978-3-540-89020-1_39
Kurth, W., Kniemeyer, O., and Buck-Sorlin, G. (2004). “Relational Growth grammars – a graph rewriting approach to dynamical systems with a dynamical structure [Conference presentation],” in Proceedings of the 2004 International Conference on Unconventional Programming Paradigms (Heidelberg; Berlin: Springer). doi: 10.1007/11527800_5
Le, M. C., Ney, B., Lemarchand, E., Schoeny, A., and Tivoli, B. (2009). Effect of pea plant architecture on the spatio-temporal epidemic development of ascochyta blight (Mycosphaerella pinodes) in the field. Plant Pathol. 58, 332–343. doi: 10.1111/j.1365-3059.2008.01947.x
Letort, V., Mahe, P., Cournede, P. H., De Reffye, P., and Courtois, B. (2008). Quantitative genetics and functional-structural plant growth models: simulation of quantitative trait loci detection for model parameters and application to potential yield optimization. Ann. Bot. 101, 1243–1254. doi: 10.1093/aob/mcm197
Louarn, G., Barillot, R., Combes, D., and Escobar-Gutiérrez, A. (2020). Towards intercrop ideotypes: non-random trait assembly can promote overyielding and stability of species proportion in simulated legume-based mixtures. Ann. Bot. 126, 671–685. doi: 10.1093/aob/mcaa014
Louarn, G., and Song, Y. (2020). Two decades of functional-structural plant modelling: now addressing fundamental questions in systems biology and predictive ecology. Ann. Bot. 126, 501–509. doi: 10.1093/aob/mcaa143
Luquet, D., Dingkuhn, M., Kim, H., Tambour, L., and Clement-Vidal, A. (2006). EcoMeristem, a model of morphogenesis and competition among sinks in rice. I. Concept, validation and sensitivity analysis. Funct. Plant Biol. 33, 309–323. doi: 10.1071/FP05266
Luquet, D., Rebodello, M. C., and Soulié, J. C. (2012a). “Functional-structural plant modeling to support complex trait phenotyping: Case of rice early vigour and drought tolerance using ecomeristem model [Conference presentation],” IEEE 4th International Symposium on Plant Growth Modeling, Simulation, Visualization and Applications (Shanghai: IEEE). doi: 10.1109/PMA.2012.6524845
Luquet, D., Soulié, J. C., Rebodello, M. C., Rouan, L., Clement-Vidal, A., and Dingkuhn, M. (2012b). Developmental dynamics and early growth vigour in rice 2. modelling genetic diversity using ecomeristem. J. Agron. Crop Sci. 198, 385–398. doi: 10.1111/j.1439-037X.2012.00527.x
Lynch, J. P.. (2013). Steep, cheap and deep: an ideotype to optimize water and N acquisition by maize root systems. Ann. Bot. 112, 347–357. doi: 10.1093/aob/mcs293
Maiti, R. K., and Pratik, S. (2014). Research advances in major cereal crops for adaptation to abiotic stresses. GM Crops and Food 5, 259–279. doi: 10.4161/21645698.2014.947861
Messina, C. D., Sinclair, T. R., Hammer, G. L., Curan, D., Thompson, J., Oler, Z., et al. (2015). Limited-transpiration trait may increase maize drought tolerance in the US corn belt. Agron. J. 107, 1978–1986. doi: 10.2134/agronj15.0016
Mi, G., Chen, F., Wu, Q., Lai, N., Yuan, L., and Zhang, F. (2010). Ideotype root architecture for efficient nitrogen acquisition by maize in intensive cropping systems. Sci. China: Life Sci. 53, 1369–1373. doi: 10.1007/s11427-010-4097-y
Mi, G., Chen, F., Yuan, L., and Zhang, F. (2016). Ideotype root system architecture for maize to achieve high yield and resource use efficiency in intensive cropping systems. Adv. Agron. 139, 73–97. doi: 10.1016/bs.agron.2016.05.002
Moose, S. P., and Mumm, R. H. (2008). Molecular plant breeding as the foundation for 21st century crop improvement. Plant Physiol. 147, 969–977. doi: 10.1104/pp.108.118232
Ndour, A., Vadez, V., Pradal, C., and Lucas, M. (2017). Virtual plants need water too: functional-structural root system models in the context of drought tolerance breeding. Front. Plant Sci. 8:1577. doi: 10.3389/fpls.2017.01577
Ong, Y., Streit, K., Henke, M., and Kurth, W. (2014). An approach to multiscale modelling with graph grammars. Ann. Bot. 114, 813–827. doi: 10.1093/aob/mcu155
Ourry, A., Mcduff, J. H., Volenec, J. J., and Gaudillere, J. P. (2001). “Nitrogen traffic during plant growth and development,” in Plant Nitrogen, eds P. J. Lea and J. F. Morot-Gaudry (Berlin, Heidelberg: Springer), 255–273. doi: 10.1007/978-3-662-04064-5_10
Pao, Y. C., Kahlen, K., Chen, T., Wiechers, D., and Stützel, H. (2021). How does structure matter? Comparison of canopy photosynthesis using one- and three-dimensional light models: a case study using greenhouse cucumber canopies. In Silico Plants 3:diab031. doi: 10.1093/insilicoplants/diab031
Picheny, V., Casadebaig, P., Trépos, R., Faivre, R., Da Silva, D., Vincourt, P., et al. (2017). Using numerical plant models and phenotypic correlation space to design achievable ideotypes. Plant, Cell Environ. 40, 1926–1939. doi: 10.1111/pce.13001
Postma, J. A., Kuppe, C., Owen, M. R., Mellor, N., Watt, M., Griffiths, M., et al. (2017). OPENSIMROOT: widening the scope and application of root architectural models. New Phytol. 215, 1274–1286. doi: 10.1111/nph.14641
Pradal, C., Dufour-Kowalski, S., Boudon, F., Fournier, C., and Godin, C. (2008). OpenAlea: a visual programming and component-based software platform for plant modelling. Funct. Plant Biol. 35, 751–760. doi: 10.1071/FP08084
Prusinkiewicz, P.. (1998). Modeling of spatial structure and development of plants: a review. Sci. Hortic. 74, 113–149. doi: 10.1016/S0304-4238(98)00084-3
Prusinkiewicz, P., Allen, M. T., Escobargutierrez, A. J., and Dejong, T. M. (2007). “Numerical methods for transport-resistance source-sink allocation models”, in Functional-Structural Plant Modelling in Crop Production, eds J. Vos, L. F. M. Marcelis, P. H. B. de Visser, P. C. Struik, and J. B. Evers (Dordrecht: Springer), 123–137. doi: 10.1007/1-4020-6034-3_11
Prusinkiewicz, P., Crawford, S., Smith, R. S., Ljung, K., Bennett, T., Ongaro, V., et al. (2009). Control of bud activation by an auxin transport switch. Proc. Natl. Acad. Sci. U. S. A. 106, 17431–17436. doi: 10.1073/pnas.0906696106
Prusinkiewicz, P., Karwowski, R., Mech, R., and Hanan, J. (2000). “L-studio/cpfg: a software system for modeling plants,” in Applications of Graph Transformations with Industrial Relevance, eds M. Nagl, A. Schürr, and M. Münch (Berlin, Heidelberg: Springer), 457–464. doi: 10.1007/3-540-45104-8_38
Prusinkiewicz, P., and Lindenmayer, A. (1990). “Graphical modeling using L-systems,” in The Algorithmic Beauty of Plants, The Virtual Laboratory (New York, NY: Springer), 1–50. doi: 10.1007/978-1-4613-8476-2_1
Prusinkiewicz, P., Lindenmayer, A., and Hanan, J. (1988). Developmental models of herbaceous plants for computer imagery purposes. Comput. Graphics 22, 141–150. doi: 10.1145/378456.378503
Qi, R., Ma, Y., Hu, B., Reffye, P. D., and Paul-Henry, C. (2010). Optimization of source-sink dynamics in plant growth for ideotype breeding: a case study on maize. Comput. Electronics in Agriculture 71, 96–105. doi: 10.1016/j.compag.2009.12.008
Quilot, B., Kervella, J., Genard, M., and Lescourret, F. (2006). Analysing the genetic control of peach fruit quality through an eco-physiological model combined with a QTL approach. J. Exp. Bot. 56, 3083–3092. doi: 10.1093/jxb/eri305
Ray, D. K., Mueller, N. D., West, P. C., and Foley, J. A. (2013). Yield trends are insufficient to double global crop production by 2050. PLoS ONE 8:e66428. doi: 10.1371/journal.pone.0066428
Rebolledo, M. C., Dingkuhn, M., Clement-Vidal, A., and Rouan, L. (2012). Phenomics of rice early vigour and drought response: are sugar related and morphogenetic traits relevant? Rice 5:22. doiI: 10.1186/1939-8433-5-22 doi: 10.1186/1939-8433-5-22
Rebolledo, M. C., Dingkuhn, M., Courtois, B., Gibon, Y., Clément-Vidal, A., Cruz, D. F., et al. (2015). Phenotypic and genetic dissection of component traits for early vigour in rice using plant growth modelling, sugar content analyses and association mapping. J. Exp. Bot. 66, 5555–5566. doi: 10.1093/jxb/erv258
Reyes, F., Pallas, B., Pradal, C., Vaggi, F., Zanotelli, D., Tagliavini, M., et al. (2019). Musca: a multi-scale source-sink carbon allocation model to explore carbon allocation in plants. an application on static apple-tree. Ann. Bot. 126, 571–585. doi: 10.1093/aob/mcz122
Rötter, R. P., Tao, F., Hohn, J. G., and Palosuo, T. (2015). Use of crop simulation modelling to aid ideotype design of future cereal cultivars. J. Exp. Bot. 66, 3463–3476. doi: 10.1093/jxb/erv098
Ruan, Y. L.. (2014). Sucrose metabolism: gateway to diverse carbon use and sugar signaling. Annu. Rev. Plant Biol. 65, 33–67. doi: 10.1146/annurev-arplant-050213-040251
Sarlikioti, V., De Visser, P. H. B., Buck-Sorlin, G. H., and Marcelis, L. F. M. (2011a). How plant architecture affects light absorption and photosynthesis in tomato: towards an ideotype for plant architecture using a functional-structural plant model. Ann. Bot. 108, 1065–1073. doi: 10.1093/aob/mcr221
Sarlikioti, V., De Visser, P. H. B., and Marcelis, L. F. M. (2011b). Exploring the spatial distribution of light interception and photosynthesis of canopies by means of a functional-structural plant model. Ann. Bot. 107, 875–883. doi: 10.1093/aob/mcr006
Schmidt, J. E., and Gaudin, A. C. M. (2017). Toward an integrated root ideotype for irrigated systems. Trends Plant Sci. 22, 433–443. doi: 10.1016/j.tplants.2017.02.001
Schnepf, A., Leitner, D., Landl, M., Lobet, G., Mai, T. H., Morandage, S., et al. (2018). CRootBox: a structural–functional modelling framework for root systems. Ann. Bot. 121, 1033–1053. doi: 10.1093/aob/mcx221
Semenov, M. A., and Halford, N. G. (2009). Identifying target traits and molecular mechanisms for wheat breeding under a changing climate. J. Exp. Bot. 60, 2791–2804. doi: 10.1093/jxb/erp164
Sievänen, R., Godin, C., DeJong, T. M., and Nikinmaa, E. (2014). Functional-structural plant models: a growing paradigm for plant studies. Ann. Bot. 114, 599–603. doi: 10.1093/aob/mcu175
Sinclair, T. R., and Seligman, N. G. (1996). Crop modeling: from infancy to maturity. Agron. J. 88, 698–704. doi: 10.2134/agronj1996.00021962008800050004x
Slavíková, J.. (1980). Tropical trees and forests. An architectural analysis. Biol Plant 22:230. doi: 10.1007/BF02892746
Song, Q., Zhang, G., and Zhu, X. G. (2013). Optimal crop canopy architecture to maximise canopy photosynthetic CO2 uptake under elevated CO2- a theoretical study using a mechanistic model of canopy photosynthesis. Funct. Plant Biol. 40, 108–124. doi: 10.1071/FP12056
Song, Y., Guo, Y., Li, B. G., and de Reffye, P. (2003a). Virtual maize model I. biomass partitioning based on plant topological structure. Acta Ecol. Sinica 23, 2333–2341. doi: 10.3321/j.issn:1000-0933.2003.11.017
Song, Y, Guo, Y., Li, B. G., and de Reffye, P. (2003b). Virtual maize model. plant geometric architecture constructing based on organ biomass accumulation. Ecol. J. 23(12), 2579–2586. doi: 10.3321/j.issn:1000-0933.2003.12.011
Tardieu, F.. (2003). Virtual plants: modelling as a tool for the genomics of tolerance to water deficit. Trends Plant Sci. 8, 9–14. doi: 10.1016/S1360-1385(02)00008-0
Tardieu, F., Reymond, M., Muller, B., Granier, C., Simonneau, T., Sadok, W., et al. (2005). Linking physiological and genetic analyses of the control of leaf growth under changing environmental conditions. Aust. J. Agric. Res. 56, 937–946. doi: 10.1071/AR05156
Tardieu, F., and Tuberosa, R. (2010). Dissection and modelling of abiotic stress tolerance in plants. Curr. Opin. Plant Biol. 13, 206–212. doi: 10.1016/j.pbi.2009.12.012
Taylor-Hell, J.. (2005). “Incorporating biomechanics into architectural tree models [Conference presentation],” in XVIII Brazilian Symposium on Computer Graphics and Image Processing (Natal: IEEE). doi: 10.1109/SIBGRAPI.2005.32
Teichmann, T., and Muhr, M. (2015). Shaping plant architecture. Front. Plant Sci. 6:233. doi: 10.3389/fpls.2015.00233
Vos, J., Evers, J. B., Buck-Sorlin, G. H., Andrieu, B., Chelle, M., and de Visser, P. H. (2010). Functional-structural plant modelling: a new versatile tool in crop science. J. Exp. Bot. 61, 2101–2115. doi: 10.1093/jxb/erp345
Vos, J., Marcelis, L. F. M., and Evers, J. B. (2007). “Functional-structural plant modelling in crop production: adding a dimension,” in Functional-Structural Plant Modelling in Crop Production, eds J. Vos, L. F. M. Marcelis, P. H. B. de Visser, P. C. Struik, and J. B. Evers (Dordrecht: Springer), 1–12. doi: 10.1007/1-4020-6034-3_1
Wan, J.. (2006). Perspectives of molecular design breeding in crops. Acta Agron. Sinica 32, 455–462. doi: 10.3321/j.issn:0496-3490.2006.03.023
Wang, E., Brown, H. E., Rebetzke, G. J., Zhao, Z., Zheng, B., and Chapman, S. C. (2019). Improving process-based crop models to better capture genotype × environment × management interactions. J. Exp. Bot. 70, 2389–2401. doi: 10.1093/jxb/erz092
Wang, J., Li, H., Zhang, X., Yin, C., Li, Y., Ma, Y., et al. (2011). Molecular design breeding in crops in China. Acta Agron. Sinica 37, 191–201. doi: 10.3724/SP.J.1006.2011.00191
Webber, H., Olesen, J. E., Müller, C., Fronzek, S., Ruane, A., Bourgault, M., et al. (2018). Diverging importance of drought stress for maize and winter wheat in Europe. Nat. Commun. 9, 4249. doi: 10.1038/s41467-018-06525-2
Wernecke, P., Buck-Sorlin, G. H., and Diepenbrock, W. (2000). Combining process- with architectural models: the simulation tool VICA. Syst. Anal. Modell. Simul. 39, 235–277. doi: 10.5555/363182.363207
Wu, A., Song, Y., van Oosterom, E. J., and Hammer, G. L. (2016). Connecting biochemical photosynthesis models with crop models to support crop improvement. Front. Plant Sci. 7:1518. doi: 10.3389/fpls.2016.01518
Xu, L., and Buck-Sorlin, G. (2016). “Simulating genotype-phenotype interaction using extended functional-structural plant models: approaches, applications and potential pitfalls,” in Crop Systems Biology, eds X. Yin and P. Struik (Cham: Springer), 33–53. doi: 10.1007/978-3-319-20562-5_2
Xu, L., Henke, M., Zhu, J., Kurth, W., and Buck-Sorlin, G. (2011). A functional-structural model of rice linking quantitative genetic information with morphological development and physiological processes. Ann. Bot. 107, 817–828. doi: 10.1093/aob/mcq264
Yan, H., Kang, M., De Reffye, P., and Dingkuhn, M. (2004). A dynamic, architectural plant model simulating resource-dependent growth. Ann. Bot. 93, 591–602. doi: 10.1093/aob/mch078
Yin, X., and Struik, P. (2008). Applying modelling experiences from the past to shape crop systems biology: the need to converge crop physiology and functional genomics. New Phytol. 179, 629–642. doi: 10.1111/j.1469-8137.2008.02424.x
Yin, X., and Struik, P. C. (2009). C3 and C4 photosynthesis models: an overview from the perspective of crop modelling. NJAS Wageningen J. Life Sci. 57, 27–38. doi: 10.1016/j.njas.2009.07.001
Yin, X., and Struik, P. C. (2010). Modelling the crop: from system dynamics to systems biology. J. Exp. Bot. 61, 2171–2183. doi: 10.1093/jxb/erp375
Yin, X., Struik, P. C., Tang, J., Qi, C., and Liu, T. (2005). Model analysis of flowering phenology in recombinant inbred lines of barley. J. Exp. Bot. 56, 959–965. doi: 10.1093/jxb/eri089
Yin, X., van der Linden, C. G., and Struik, P. C. (2018). Bringing genetics and biochemistry to crop modelling, and vice versa. Eur. J. Agron. 100, 132–140. doi: 10.1016/j.eja.2018.02.005
Yin, X., and van Laar, H. H. (2005). Crop Systems Dynamics: An Ecophysiological Simulation Model of Genotype-by-Environment Interactions. Wageningen: Wageningen Academic Publishers. doi: 10.3920/978-90-8686-539-0
Zhang, Z., Qu, X., Wan, S., Chen, L., and Zhu, Y. (2005). Comparison of QTL controlling seedling vigour under different temperature conditions using recombinant inbred lines in rice. Ann. Bot. 95, 423–429. doi: 10.1093/aob/mci039
Zhao, D. L., Atlin, G. N., Bastiaans, L., and Spiertz, J. H. J. (2006). Cultivar weed-competitiveness in aerobic rice: heritability, correlated traits, and the potential for indirect selection in weed-free environments. Crop Sci. 46, 372–380. doi: 10.2135/cropsci2005.0192
Keywords: functional-structural plant modeling, plant architecture, plant phenotyping, genotype to phenotype, assisted molecular breeding
Citation: Soualiou S, Wang Z, Sun W, de Reffye P, Collins B, Louarn G and Song Y (2021) Functional–Structural Plant Models Mission in Advancing Crop Science: Opportunities and Prospects. Front. Plant Sci. 12:747142. doi: 10.3389/fpls.2021.747142
Received: 25 July 2021; Accepted: 22 November 2021;
Published: 23 December 2021.
Edited by:
Zhong-Hua Chen, Western Sydney University, AustraliaReviewed by:
Mingkai Jiang, Western Sydney University, AustraliaCopyright © 2021 Soualiou, Wang, Sun, de Reffye, Collins, Louarn and Song. This is an open-access article distributed under the terms of the Creative Commons Attribution License (CC BY). The use, distribution or reproduction in other forums is permitted, provided the original author(s) and the copyright owner(s) are credited and that the original publication in this journal is cited, in accordance with accepted academic practice. No use, distribution or reproduction is permitted which does not comply with these terms.
*Correspondence: Gaëtan Louarn, Z2FldGFuLmxvdWFybkBpbnJhLmZy; Youhong Song, dXF5c29uZ0AxNjMuY29t
Disclaimer: All claims expressed in this article are solely those of the authors and do not necessarily represent those of their affiliated organizations, or those of the publisher, the editors and the reviewers. Any product that may be evaluated in this article or claim that may be made by its manufacturer is not guaranteed or endorsed by the publisher.
Research integrity at Frontiers
Learn more about the work of our research integrity team to safeguard the quality of each article we publish.