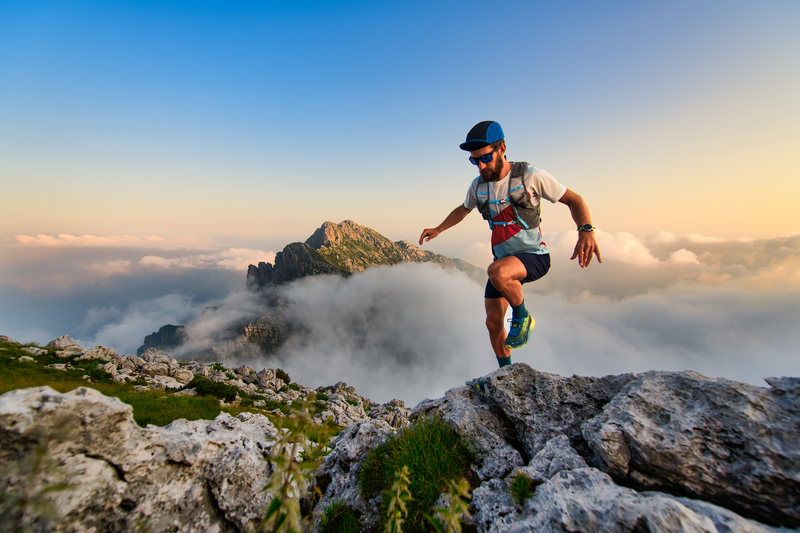
95% of researchers rate our articles as excellent or good
Learn more about the work of our research integrity team to safeguard the quality of each article we publish.
Find out more
ORIGINAL RESEARCH article
Front. Plant Sci. , 27 October 2021
Sec. Plant Abiotic Stress
Volume 12 - 2021 | https://doi.org/10.3389/fpls.2021.725436
This article is part of the Research Topic Salinity Tolerance: From Model or Wild Plants to Adapted Crops View all 27 articles
Screening and breeding more salt-tolerant varieties is an effective way to deal with the global reduction in rice (Oryza sativa L.) yield caused by salt stress. However, the molecular mechanism underlying differences in salt tolerance between varieties, especially between the subspecies, is still unclear. We herein performed a comparative transcriptomic analysis under salt stress in contrasting two rice genotypes, namely RPY geng (japonica, tolerant variety) and Luohui 9 (named as Chao 2R in this study, indica, susceptible variety). 7208 and 3874 differentially expressed genes (DEGs) were identified under salt stress in Chao 2R and RPY geng, separately. Of them, 2714 DEGs were co-expressed in both genotypes, while 4494 and 1190 DEGs were specifically up/down-regulated in Chao 2R and RPY geng, respectively. Gene ontology (GO) analysis results provided a more reasonable explanation for the salt tolerance difference between the two genotypes. The expression of normal life process genes in Chao 2R were severely affected under salt stress, but RPY geng regulated the expression of multiple stress-related genes to adapt to the same intensity of salt stress, such as secondary metabolic process (GO:0019748), oxidation-reduction process (GO:0009067), etc. Furthermore, we highlighted important pathways and transcription factors (TFs) related to salt tolerance in RPY geng specific DEGs sets based on MapMan annotation and TF identification. Through Meta-QTLs mapping and homologous analysis, we screened out 18 salt stress-related candidate genes (RPY geng specific DEGs) in 15 Meta-QTLs. Our findings not only offer new insights into the difference in salt stress tolerance between the rice subspecies but also provide critical target genes to facilitate gene editing to enhance salt stress tolerance in rice.
Rice (Oryza sativa L.) is one of the main food crops in the world and feeds nearly half of the world’s population (Parihar et al., 2015; Kong et al., 2019b). Soil salinity affects the growth and yield of rice in approximately one-third of the rice-growing land in the world (Hakim et al., 2014; Wang et al., 2018). Therefore, improving the salt tolerance of rice is essential to ensure food security for billions of people around the world. Plant salt stress tolerance is a very complex mechanism involving ion-homeostasis, ion-compartmentalization, osmoprotectant biosynthesis, and antioxidative defense (Ganie et al., 2019, 2021). So far, intensive efforts have been devoted to the dissection of genetic regulation of rice salt tolerance through reverse and forward genetic approaches (Ganie et al., 2016).
The salt stress tolerance of rice is related to the genotype, and different genotypes show discrepant salt stress tolerance (Ganie et al., 2016; Mansuri et al., 2019). Hundreds of salt stress-related QTLs have been identified among different rice populations to date (Kong et al., 2019b; Mansuri et al., 2020). However, only four salt stress-related genes have been cloned and experimentally verified in rice, namely, SKC1 (Lin et al., 2004; Hauser and Horie, 2010), HST1 (Takagi et al., 2015), OsHAK21 (He et al., 2019), and OsSTLK (Lin et al., 2020). Candidate genes in most QTLs reported so far are unknown due to the too large mapping interval. Meta-QTLs analysis can effectively narrow the mapping interval of QTLs to achieve reliable prediction of candidate genes by integrating the QTLs results from a large number of studies (Kong et al., 2020). 11 salt stress-related Meta-QTLs were reported via integrating QTL information from 12 studies (Islam et al., 2019). Recently, Mansuri et al. (2020) identified 46 Meta-QTLs of salt stress-related traits, including salinity tolerance score, shoot potassium concentration, shoot sodium concentration, chlorophyll content, shoot dry weight trait, etc., (Mansuri et al., 2020).
On the other hand, RNA-seq was utilized to detect genome-wide gene expression changes under salt stress and predict potential molecular networks in rice (Kong et al., 2019b; Mansuri et al., 2019, 2020). Many differentially expressed genes (DEGs) have been identified among the contrasting samples through RNA-sequencing analysis. For example, 995 and 1052 DEGs were identified in the shoot and root of Nipponbare (japonica) (Mizuno et al., 2010). Wang et al. (2018) conducted comparative transcriptome analysis between salt-tolerant and salt-sensitive genotypes of indica rice and reported 5,273 salt-responsive DEGs (286 DEGs only in the tolerant genotype) at the seedling stage (Wang et al., 2018). Another study compared transcriptome profiles of FL478 and its sensitive parent (IR29) and revealed 1063 DEGs were co-expressed in both FL478 and IR29 (Mansuri et al., 2019). It is well known that there are obvious differences in important agronomic traits and biotic/abiotic stresses between indica and japonica rice, and their hybrid progeny and derived lines often show obvious better-parent advantages in terms of yield and stress resistance (Yuan, 1994; Bian et al., 2010). Indica and japonica rice also have significant differences in abiotic stress resistance, including cold stress and salt stress (Yang et al., 2015). However, the molecular mechanism, key genes, and regulatory network of the difference in salt tolerance between indica and japonica rice are still unclear. In this study, we explored the genome-wide expression differences between RPY geng (japonica) and Chao 2R (indica) using RNA-seq technology. The results of integrating DEGs and Meta-QTLs highlighted the key salt stress-related genes and possible regulatory networks. Our finding not only provided a better insight into the difference in salt tolerance between indica and japonica rice but also contributed to potential gene targets for breeding better salt-tolerant varieties of indica-japonica hybrids.
Rice seedlings were grown in 96-well PCR plates with Yoshida solution (Coolaber, Beijing, China) replaced every 2 days, 26°C, and a 16/8 h light/dark photoperiod, 60% relative humidity in plant growth incubators (ZSX1500GS, Jingshen Instrument, Shanghai, China) for 14 days (Kong et al., 2020). Fourteen-day-old seedlings of RPY geng and Chao 2R were changed into 100 mM NaCl Yoshida solution for salt stress treatment (Supplementary Figure 1). Then, we sampled root tissues at 0-day (0d), 3-day (3d), and 7-day (7d), respectively. Three independent biological replicates were prepared for each treatment/control group, and at least 30 seedlings with uniform growth were sampled for each biological replicate. After all samples were collected and immediately stored in liquid nitrogen for the next step of RNA extraction.
To evaluate salt stress tolerance of RPY geng and Chao 2R, 14-day-old seedlings were treated with 125 mM NaCl Yoshida solution for 7 days to simulate salt stress. After salt stress treatment, the seedlings were grown in Yoshida solution for 7 days and the survival rate of the seedlings were calculated. 30–45 seedlings were used for each treatment and all treatments were placed randomly with three replicates. The final survival rate of rice seedlings came from the average of the survival rates of three replicates.
RNA extraction and cDNA library construction of all tested samples were conducted by Biomarker Technologies (Beijing, China) according to the standard procedures. All cDNA libraries were sequenced on an Illumina sequencing platform (HiSeq 2500) by paired-end (2 bp × 150 bp) method. After the raw reads were filtered by Trimmomatic (Bolger et al., 2014), a total of 116.45 Gb clean reads were generated, and the percentage of Q30 bases in each sample was not less than 92.38% (Supplementary Table 1). Clean reads were mapped to RPY geng genome (assembled by our team, unpublished data) using Hisat2 software and quantified the mapped reads using StringTie software (Pertea et al., 2016). Gene expression levels were estimated by fragments per kilobase of transcript per million fragments mapped reads (FPKM) method. DESeq2 was used to identify DEGs with these limits: False Discovery Rate (FDR) < 0.01 and Fold Change ≥2.
Gene ontology (GO) enrichment of DEGs was performed with GOseq R package, and GO terms with KS < 0.01 were considered to be significantly enriched (Young et al., 2010). KEGG pathway enrichment of DEGs was performed by KOBAS (Mao et al., 2005), and the pathway with P-value < 0.05 was considered to be significantly enriched.
MapMan annotation of RPY geng specific DEGs was conducted in the online annotation website1 with default parameters (Kong et al., 2020). iTAK software (Jin et al., 2017) was used2 to identify transcription factors (TFs) with default parameters.
Meta-QTLs were obtained from Mansuri et al. (2020) study. The physical locations of Meta-QTLs in IRGSP-1.0 genome were obtained by blasting SSR markers in the online blast tool3 of RAP-DB website (markers with a 100% match rate and the unique location in RAP-DB genome). IDs in RPY geng genome of DEGs were converted to IRGSP-1.0′ IDs through blastn with parameters: -max_target_seqs 1 -evalue 1e-6 -perc_identity 95. To ensure the accuracy of results, DEGs were further filtered based on the chromosome position and DEGs that were not on the same chromosome between RPY geng ID and IRGSP-1.0 ID were filtered out.
In this study, five randomly selected candidate genes for DEGs were used for RT-PCR analysis. The specific primers for these five genes and actin gene (an internal control) were designed by Primer 5.0 (Supplementary Table 2). The qRT-PCR reaction (10 μL) was formulated using the 2 X SYBR Green qPCR Master Mix (US Everbright®Inc., Suzhou, China). All qRT-PCRs were carried out on a CFX96 TouchTM Real-Time PCR Detection System (Bio-Rad, Hercules, CA, United States). Three biological replicates (from three independent RNA samples) were used for qRT-PCRs. For each biological replicate, three technical replicates were also conducted. The average threshold cycle (Ct) from three biological replicates was employed to calculate the gene expression fold change by the 2–ΔΔCT method (Kong et al., 2019a,b).
To characterize the difference in salt stress resistance between these two subspecies, we calculated the final survival rate of Chao 2R and RPY geng after salt treatment. We found that RPY geng had a higher survival rate than Chao 2R (81% vs. 31%) (Figure 1).
Figure 1. The final survival rate of rice seedlings (Chao 2R and RPY geng) after 7 days of salt stress treatment and 7 days of recovery from three independent replicates. *** represented P value < 0.001. The bar value was generated from three independent biological replicates. Survival rate = number of surviving seedlings/total number of seedlings in each biological replicate.
RNA-sequencing was performed on 18 samples (Supplementary Figure 1), and sequencing data for each sample exceeded 6G with high mapping rates (Supplementary Table 1). The overall gene expression levels of the three biological replicates of each treatment were similar (Figure 2A) and the three biological replicates were clustered together with the Pearson correlation coefficient approaching 1 (Figure 2B).
Figure 2. Sequencing data and differentially expressed genes (DEGs) results. (A) FPKM box diagram of all tested samples. (B) Heatmap of Pearson correlation coefficient for all tested samples. (C) Statistics results of DEGs in all comparison combinations. (D,E) The venn analysis of DEGs between Chao 2R and RPY geng in 3 or 7 days after stress treatment.
The results of DEGs analysis in RPY geng showed that there were 496 up-regulated and 644 down-regulated genes in 0d vs. 3d, 1352 up-regulated genes and 1489 down-regulated genes in 0d vs. 7d, and 969 up-regulated genes and 1085 down-regulated genes in 3d vs. 7d, respectively. However, Chao 2R had more DEGs than RPY geng in every comparison (Figure 2C). At 0d vs. 3d, RPY geng and Chao 2R shared 595 DEGs, at 0d vs. 7d, RPY geng and Chao 2R co-expressed 1744 DEGs (Figures 2D,E). Both RPY geng and Chao 2R had more DEGs in 0d vs. 7d than 0d vs. 3d, which indicated that more genes changed significantly the transcription levels with the extension of the salt stress time. The total number of DEGs in Chao 2R (7208) was significantly greater than that of RPY geng (3874), suggesting that the transcriptome of Chao 2R was more affected to salt stress, which along with the survival data indicated that Chao 2R was more sensitive to salt stress.
In total there were 2714 DEGs (G2) shared by Chao 2R and RPY geng, 4494 DEGs (G1) unique to Chao 2R and 1160 DEGs (G3) unique to RPY geng (Figure 3A). The top 20 GO terms of G1, G2, G3 were compared in Figures 3B–D. G2 were significantly enriched in well-known GO terms related to abiotic stresses, such as plant-type cell wall organization (GO:0042744), hydrogen peroxide metabolic process (GO:0042743), response to hydrogen peroxide (GO:0042542), and so on (Figure 3C). As expected, G1 and G3 were enriched in completely different GO terms. The GO terms of G3 were related to various stress-related processes (Figure 3D), while GO terms of G1 involved the necessary life activity pathways, namely, cell division (GO: 0051301), cell cycle process (GO:0022402), and etc., (Figure 3B). The above results indicated that under the same intensity of salt stress, the normal life process of Chao 2R were severely affected, and there were many DEGs in RPY geng that played important roles in salt stress tolerance. Therefore, we then focused on analyzing DEGs in G2 and G3. In G2, 24 DEGs showed the opposite gene expression profiles in Chao 2R and RPY geng (Figure 3E). We speculated that these DEGs may be related to the difference in salt stress tolerance between RPY geng and Chao 2R (Supplementary Table 3).
Figure 3. Go annotation and heatmap of DEGs. (A) Venn analysis of all DEGs between Chao 2R and RPY geng. (B) Top 20 Gene ontology (GO) terms of Chao-2R specific DEGs. (C) Top 20 GO terms of common DEGs in Chao 2R and RPY geng. (D) Top 20 GO terms of RPY geng specific DEGs. (E) Heatmap of DEGs with the opposite expressed profiles in two subspecies. RPY means RPY geng, C2R means Chao 2R, 0d, 3d, and 7d means control, 3rd day after treatment, and 7th day after treatment in panel (B).
Next, DEGs (G2 + G3) were further analyzed to explore the regulatory network of salt tolerance in RPY geng. The MapMan results showed that, RPY geng specific DEGs were mainly enriched in secondary metabolism, stress, signaling, transport, hormone metabolism, and other pathways in addition to essential cellular component pathways (protein, RNA, etc.) (Figure 4A). These DEGs were involved in many aspects of plant response to biotic stress, including signaling, mitogen activated protein kinases (MAPK) pathway, secondary metabolites, plant hormones (such as auxins, brassinosteroids, ABA, ethylene, SA, JA), and various functional proteins (Figure 4B). In addition, 26 types of TFs might also played an important role in salt stress tolerance, including AP2/ERF-ERF, bHLH, NAC, bZIP, MYB, and other TFs (Figure 4C). Under salt stress, the gene expression profiles of these TF genes were obviously differentiated with different direction, even within the same gene family, i.e., within AP2/ERF-ERF subfamily, EVM0018561 was up-regulated while EVM0000387 and EVM0019838 were down-regulated, within RPY geng, which suggested that the salt stress tolerance of RPY geng was a very complex process involving many different types of pathways and TFs (Figure 4D).
Figure 4. MapMan annotations and gene expression analysis of RPY geng specific DEGs (G2 + G3). (A) Functional categories of DEGs based on MapMan annotations. (B) The MapMan biotic stress overview of RPY geng specific DEGs. (C) The transcription factors (TFs) of RPY geng specific DEGs. (D) Gene expression profiles of TF genes. Blue boxes represented genes were differentially expressed on day 3 or/and on day 7 after salt stress treatment in panel (B). The percentage of different TFs in the total TFs was represented by pie chart in panel (C). The high-resolution of this figure was provided in Supplementary Figure 2.
In order to obtain salt-stress-related candidate genes, we thus collected 43 salt-stress-related Meta-QTLs (Figure 5A and Supplementary Table 4) for Meta-QTLs mapping and 330 salt-stress-related genes for homologous genes identification (Figure 5B and Supplementary Table 5). Here, 42 Meta-QTLs contained varying amounts of DEGs (from G2 + G3) ranging 3 to 71, and 9 Meta-QTLs contained different number of known genes from 1 to 5 (Figure 5A and Supplementary Table 6). Notably, G2 + G3 covered 70 known salt stress-related genes (Figure 5B and Supplementary Table 7), suggesting that the previously reported salt-stress-related genes also played important roles in RPY geng stress tolerance.
Figure 5. Homologous genes of known genes in Meta-QTLs. (A) Statistics of known genes (red) and new genes (blue) of DEGs in each Meta-QTLs. (B) The venn analysis result of G2 + G3 and known genes. (C) Known genes (red) and homologous genes of known genes (black) in Meta-QTLs. (D) RT-PCR and RNA-seq results of five randomly selected candidate genes. G2 + G3 represented RPY geng specific DEGs in panel (B); Red, black, and blue represented known genes, homologous genes of known genes, and Meta-QTLs, respectively; Blue represented gene expression changes relative to the control of RNA-seq in panel (D), details were provided in Supplementary Table 8. ***means that the difference is significant relative to the control (p < 0.001), and ns means that the difference is not significant compared to the control.
We noticed that there were still overfull DEGs among Meta-QTLs after Meta-QTLs mapping, which made it difficult to predict candidate genes. Due to homologous genes often have similar biological functions, we thus searched the homologous genes of 330 known genes in all Meta-QTLs using blastn (Evalue 1E-20). Finally, 18 homologous genes of known genes were obtained in 15 Meta-QTLs, which effectively narrowed the range of candidate genes (Figure 5C and Table 1). We randomly selected five genes for RT-PCR verification. The results of RT-PCR and RNA-seq consistently supported that these genes were salt stress-responsive genes (Figure 5D and Supplementary Table 8).
In view of the catastrophic effects of salt stress on rice, several studies have so far explored the transcriptional changes of rice under salt stress. These earlier studies focused on only a variety or one subspecies (Mizuno et al., 2010; Wang et al., 2018; Mansuri et al., 2019). For example, Nipponbare (janopinca) was used in Mizuno et al. (2010) study, Xian156 (indica) and IR28 (indica) were used in Wang et al. (2018) study, as well as FL478 (indica) and IR29 (indica) were used in Mansuri et al. (2019) study. However, the difference in salt tolerance between indica and japonica has not been fully explained, which may be related to the differences in genome and transcription between them. In order to take advantage of the difference between indica and japonica rice to cultivate new rice varieties with higher salt tolerance by inter-subspecies hybrids, it is necessary to explore the transcriptional differences in the tolerance of indica and japonica and the underlying molecular mechanisms. Our GO analysis implied that RPY geng had salt stress-related DEGs which conferred higher salt stress tolerance. The DEGs annotation results of these two varieties are completely consistent with the comparative analysis result of the family genes of Chao 2R and RPY geng genomes wherein the RPY geng-specific gene families are closely related to abiotic stress, while the Chao 2R-specific gene families are enriched in essential life activities (unpublished data). Therefore, the results of transcription and genomic data both suggest that the difference in salt stress tolerance between Chao 2R and RPY geng is due to differences in genetic data sets. In the current study, the integrated survival rate and transcription annotation results concluded that RPY geng (japonica) has stronger salt stress tolerance than Chao 2R (indica). Differently, we reported earlier that TNG67 (indica) is more tolerant to salt stress than TCN1 (japonica) (Kong et al., 2019b). Lee et al. (2003) also reported the salt tolerance level of indica was higher than that of japonica. Chen et al. (2018) reported that 9311 (indica) has higher salt stress than Nipponbare (japonica) and found that the abundance variation of OsHAK1 transcript underlies the differential salinity tolerance of 9311 and Nipponbare. These results indicated that the difference in salt tolerance between indica and japonica rice is not absolute, and the difference in salt tolerance may be related to important QTLs, such as the above-mentioned OsHAK1. Major QTLs related to salt stress difference between RPY geng and Chao 2R are expected to be fine mapped by combining QTL mapping in the derived populations from a cross of RPY geng and Chao 2R and RNA-seq analysis in immediate future.
Salt stress tolerance is a complicated quantitative trait controlled by multiple genes (Yang et al., 2015; Kong et al., 2019b). In this study, RPY geng (salt-tolerant rice) specific DEGs involved multiple biological pathways closely related to abiotic stress. Previous studies have shown that Ca2+-mediated signaling pathways, MAPK, ROS, and ABA signaling pathways played an important role in plant response to salt stress (Tuteja, 2007; Golldack et al., 2014). These signal genes were also significantly enriched in RPY geng specific DEGs, which was in line with the original studies (Tuteja, 2007; Golldack et al., 2014). A large number of novel TFs were identified in this study, suggesting that TFs also played crucial roles in response to salt stress and differential tolerance between indica and japonica rice. For example, previous studies have shown that WRKY TFs were important regulators of plant salt stress tolerance (Jiang et al., 2017). Another study reported that rice OsMYB2 TF was involved in the salt tolerance, cold tolerance and dehydration tolerance (An et al., 2012). Many phytohormones are important secondary signal molecules that can regulate a variety of external environmental stimuli (Duan et al., 2013). For example, several genes in ABA synthesis and signal transduction pathways can respond to salt stress and increase the ABA content in plants under salt stress (Wang et al., 2014; Shu et al., 2017). In addition, salt stress will reduce the activity level of GA, cause the accumulation of DELLA protein, and then inhibit plant growth to adapt to the stress (Achard et al., 2006). As shown in Figure 4B, these important DEGs involving multiple biological processes may endow RPY geng with higher stress tolerance than Chao 2R and provide potential gene targets for future gene editing verification.
Previous rice salt stress tolerance transcriptome analysis mostly described the GO or KEGG pathway involved in DEGs, but important DEGs closely related to stress tolerance were not focused (Mizuno et al., 2010; Wang et al., 2018; Mansuri et al., 2019). The continuous release of salt tolerance QTL results from different populations has made it possible for us to integrate the transcriptome results to highlight key candidate genes. Here, we innovatively integrated the results of multiple omics including our DEGs, the published Meta-QTLs, as well as known salt stress genes and finally obtained 18 candidate genes, which provided reliable targets for subsequent breeding. Previous studies reported that OsNAC2 encodes a NAC TF (Mao et al., 2020) and transgenic lines of over-expressing OsNAC2 gene are more sensitive to high salt stress (Shen et al., 2017). We also found a homologous gene (EVM0041760) of OsNAC2 in MQTL-2.3, and speculated that this gene may also play an important role in rice salt stress tolerance through a molecular regulatory mechanism similar to OsNAC2. Additionally, EVM0022292 in MQTL-5.3 is a homolog of four several known genes belonging to NAC domain transcription factor gene family [OsNAC5 (Takasaki et al., 2010); OsNAC9 (Liu et al., 2014); OsNAP (Chen et al., 2014); and OsNAC6 (Hu et al., 2008)], which emphasize the indispensable role of the NAC family in salt stress tolerance. Besides, OsHsfB2b, a heat shock factor was considered a core regulator in the defense response to heat stress and negatively regulates the tolerance of rice to drought stress. In this study, EVM0024006 and EVM0035261 were identified as homologous genes of OsHsfB2b suggesting that heat shock factors may also play an important role in salt stress, and there may be links between different stress regulatory networks. In addition, EVM0000377 and EVM0006395 were homologous genes of Osmyb4 and OsMYB91. Overexpression of the rice Osmyb4 gene enhanced chilling and freezing tolerance in Arabidopsis (Vannini et al., 2004) and OsMYB91 had a function in coordinating plant growth and salt stress tolerance in rice (Zhu et al., 2015). EVM0022714, EVM0025666, and EVM0013858 were homologous genes of RCc3 and RCc3 has been proven to improve root system architecture and enhance salt tolerance in rice (Li et al., 2018). OsVP1, the homologous gene of EVM0012484, participated in drought tolerance, salt tolerance, and cold tolerance in rice (Liu et al., 2010; Zhang et al., 2011). OsKAT1, the homologous gene of EVM0006884, was involved in salt tolerance of rice in cooperation with other K+ channels by participating in maintenance of cytosolic cation homeostasis during salt stress, and thus protected cells from Na+ (Obata et al., 2007). RSOsPR10, the homologous gene of EVM0024798, was a novel rice PR10 protein, which was rapidly induced in roots by salt, drought stresses and blast fungus infection possibly through activation of the jasmonic acid signaling pathway (Hashimoto et al., 2004). Another study reported that the expression of OsMGD (the homologous gene of EVM0009491) was induced by ethephon, gibberellin, drought, and salt treatment, suggesting that OsMGD plays a key role in abiotic stress response (Qi et al., 2004). Co-overexpression of OsGS1;1 (the homologous gene of EVM0009491) and OsGS2 in transgenic rice plants enhanced its tolerance to osmotic and salinity stress at the seedling stage (James et al., 2018). OsPM19L1, the homologous gene of EVM0011147, encoding Oryza sativa AWPM-19-like protein 1 was proven to be dramatically induced by 20% PEG stress, exogenous abscisic acid, salt, and cold stress and associated with stress tolerance through ABA-dependent pathway in rice (Chen et al., 2015). OsBIERF4 and OsEREBP2 were homologous genes of EVM0019096 and have been experimentally proven to be significantly induced by salt stress (Cao et al., 2006; Serra et al., 2013). Overall, our multi-omics analysis results highlighted the important regulatory genes of salt stress in Meta-QTLs. The candidate genes highlighted in this study provide reliable targets for gene editing to produce rice varieties with higher salt tolerance.
The comparative genomic results of Chao 2R and RPY geng under salt stress indicated that the difference in salt stress tolerance between indica and japonica rice is most likely due to the difference in the DEGs data set related to salt stress. Here, we further pointed out these genes involved in multiple regulatory pathways in the RPY geng-specific data set can be applied to future gene editing and breeding through multiple annotation methods and multi-omics joint analysis.
The original contributions presented in the study are publicly available. This data can be found here: National Center for Biotechnology Information (NCBI) BioProject database under accession number PRJNA732136.
WK performed all of the experiments, analyzed the data, prepared the figures and tables, and wrote the manuscript. WK and YL conceived and designed the experiments. TS and CZ performed parts of the experiments, figures, and tables. XD provided comments during the writing. All authors read and approved the final version of the manuscript.
This study was funded by the National Special Key Project for Transgenic Breeding (Grant No. 2016ZX08001001), the National Key Research and Development Program of China (2016YFD0100400).
The authors declare that the research was conducted in the absence of any commercial or financial relationships that could be construed as a potential conflict of interest.
All claims expressed in this article are solely those of the authors and do not necessarily represent those of their affiliated organizations, or those of the publisher, the editors and the reviewers. Any product that may be evaluated in this article, or claim that may be made by its manufacturer, is not guaranteed or endorsed by the publisher.
The authors thank the editor and reviewers whose constructive comments were helpful in improving the quality of this manuscript.
The Supplementary Material for this article can be found online at: https://www.frontiersin.org/articles/10.3389/fpls.2021.725436/full#supplementary-material
Supplementary Figure 1 | Schematic diagram of salt stress treatment of samples in this study.
Supplementary Figure 2 | The high-resolution figure of Figure 4.
Supplementary Table 1 | Sequencing data and sample mapping rates.
Supplementary Table 2 | Primers used in RT-PCR.
Supplementary Table 3 | Differentially expressed genes (DEGs) in G2 with the opposite expressed profiles in two subspecies.
Supplementary Table 4 | Meta-QTLs collected in this study.
Supplementary Table 5 | Salt stress-related genes collected in this study.
Supplementary Table 6 | RPY geng specific DEGs in Meta-QTLs.
Supplementary Table 7 | Seventy known salt stress-related genes in G2 and G3.
Supplementary Table 8 | RNA-seq results of five randomly selected DEGs.
Achard, P., Cheng, H., De Grauwe, L., Decat, J., Schoutteten, H., Moritz, T., et al. (2006). Integration of plant responses to environmentally activated phytohormonal signals. Science 311, 91–94. doi: 10.1126/science.1118642
An, Y., Dai, X., and Zhang, W. H. (2012). A R2R3-type MYB gene, OsMYB2, is involved in salt, cold, and dehydration tolerance in rice. J. Exp. Bot. 63, 2541–2556. doi: 10.1093/jxb/err431
Bian, J., Jiang, L., Liu, L., Xiao, Y., Wang, Z., Zhao, Z., et al. (2010). Identification of japonica chromosome segments associated with heterosis for yield in indica × japonica rice hybrids. Crop Sci. 50, 2328–2337. doi: 10.2135/cropsci2010.05.0242
Bolger, A. M., Lohse, M., and Usadel, B. (2014). Trimmomatic: a flexible trimmer for Illumina sequence data. Bioinformatics 30, 2114–2120. doi: 10.1093/bioinformatics/btu170
Cao, Y. F., Song, F. M., Goodman, R. M., and Zheng, Z. (2006). Molecular characterization of four rice genes encoding ethylene-responsive transcriptional factors and their expressions in response to biotic and abiotic stress. J. Plant Physiol. 163, 1167–1178. doi: 10.1016/j.jplph.2005.11.004
Chen, G., Liu, C. L., Gao, Z. Y., Zhang, Y., Zhang, A. P., Zhu, L., et al. (2018). Variation in the abundance of OsHAK1 transcript underlies the differential salinity tolerance of an indica and a japonica rice cultivar. Front. Plant Sci. 8:2216. doi: 10.3389/fpls.2017.02216
Chen, H., Lan, H., Huang, P., Zhang, Y., Yuan, X., Huang, X., et al. (2015). Characterization of OsPM19L1 encoding an AWPM-19-like family protein that is dramatically induced by osmotic stress in rice. Genet. Mol. Res. 14, 11994–12005. doi: 10.4238/2015.october.5.12
Chen, X., Wang, Y., Lv, B., Li, J., Luo, L., Lu, S., et al. (2014). The NAC family transcription factor OsNAP confers abiotic stress response through the ABA pathway. Plant Cell Physiol. 55, 604–619. doi: 10.1093/pcp/pct204
Duan, L., Dietrich, D., Ng, C. H., Chan, P. M. Y., Bhalerao, R., Bennett, M. J., et al. (2013). Endodermal ABA signaling promotes lateral root quiescence during salt stress in Arabidopsis seedlings. Plant Cell 25, 324–341. doi: 10.1105/tpc.112.107227
Ganie, S. A., Borgohain, M. J., Kritika, K., Talukdar, A., Pani, D. R., and Mondal, T. K. (2016). Assessment of genetic diversity of Saltol QTL among the rice (Oryza sativa L.) genotypes. Physiol. Mol. Biol. Plants 22, 107–114.
Ganie, S. A., Molla, K. A., Henry, R. J., Bhat, K. V., and Mondal, T. K. (2019). Advances in understanding salt tolerance in rice. Theor. Appl. Genet. 132, 851–870. doi: 10.1007/s00122-019-03301-8
Ganie, S. A., Wani, S. H., Henry, R., and Hensel, G. (2021). Improving rice salt tolerance by precision breeding in a new era. Curr. Opin. Plant Biol. 60:101996. doi: 10.1016/j.pbi.2020.101996
Golldack, D., Li, C., Mohan, H., and Probst, N. (2014). Tolerance to drought and salt stress in plants: unraveling the signaling networks. Front. Plant Sci. 5:151. doi: 10.3389/fpls.2014.00151
Hakim, M., Juraimi, A. S., Hanafi, M., Ali, E., Ismail, M. R., Selamat, A., et al. (2014). Effect of salt stress on morpho-physiology, vegetative growth and yield of rice. J. Environ. Biol. 35:317.
Hashimoto, M., Kisseleva, L., Sawa, S., Furukawa, T., Komatsu, S., and Koshiba, T. (2004). A novel rice PR10 protein, RSOsPR10, specifically induced in roots by biotic and abiotic stresses, possibly via the jasmonic acid signaling pathway. Plant Cell Physiol. 45, 550–559. doi: 10.1093/pcp/pch063
Hauser, F., and Horie, T. (2010). A conserved primary salt tolerance mechanism mediated by HKT transporters: a mechanism for sodium exclusion and maintenance of high K+/Na+ ratio in leaves during salinity stress. Plant Cell Environ. 33, 552–565. doi: 10.1111/j.1365-3040.2009.02056.x
He, Y., Yang, B., He, Y., Zhan, C., Cheng, Y., Zhang, J., et al. (2019). A quantitative trait locus, qSE3, promotes seed germination and seedling establishment under salinity stress in rice. Plant J. 97, 1089–1104. doi: 10.1111/tpj.14181
Hu, H., You, J., Fang, Y., Zhu, X., Qi, Z., and Xiong, L. (2008). Characterization of transcription factor gene SNAC2 conferring cold and salt tolerance in rice. Plant Mol. Biol. 67, 169–181. doi: 10.1007/s11103-008-9309-5
Islam, M., Ontoy, J., and Subudhi, P. K. (2019). Meta-analysis of quantitative trait loci associated with seedling-stage salt tolerance in rice (Oryza sativa L.). Plants 8:33. doi: 10.3390/plants8020033
James, D., Borphukan, B., Fartyal, D., Ram, B., Singho, J., Manna, M., et al. (2018). Concurrent overexpression of OsGS1;1 and OsGS2 genes in transgenic rice (Oryza sativa L.): impact on tolerance to abiotic stresses. Front. Plant Sci. 9:786. doi: 10.3389/fpls.2018.00786
Jiang, J., Ma, S., Ye, N., Ming, J., and Zhang, J. (2017). WRKY transcription factors in plant responses to stresses. J. Integr. Plant Biol. 59:86. doi: 10.1111/jipb.12513
Jin, J., Feng, T., De-Chang, Y., Yu-Qi, M., Lei, K., Luo, J., et al. (2017). PlantTFDB 4.0: toward a central hub for transcription factors and regulatory interactions in plants. Nucleic Acids Res. 45, D1040–D1045.
Kong, W., Zhong, H., Gong, Z., Fang, X., Sun, T., Deng, X., et al. (2019b). Meta-analysis of salt stress transcriptome responses in different rice genotypes at the seedling stage. Plants 8:64. doi: 10.3390/plants8030064
Kong, W., An, B., Zhang, Y., Yang, J., Li, S., Sun, T., et al. (2019a). Sugar transporter proteins (STPs) in gramineae crops: comparative analysis, phylogeny, evolution, and expression profiling. Cells 8:560. doi: 10.3390/cells8060560
Kong, W., Zhang, C., Qiang, Y., Zhong, H., Zhao, G., and Li, Y. (2020). Integrated RNA-seq analysis and Meta-QTLs mapping provide insights into cold stress response in rice seedling roots. Int. J. Mol. Sci. 21:4615. doi: 10.3390/ijms21134615
Lee, K. S., Choi, W. Y., Ko, J. C., Kim, T. S., and Gregorio, G. B. (2003). Salinity tolerance of japonica and indica rice (Oryza sativa L.) at the seedling stage. Planta 216, 1043–1046.
Li, X. X., Chen, R. R., Chu, Y. L., Huang, J. Y., Jin, L., Wang, G. X., et al. (2018). Overexpression of RCc3 improves root system architecture and enhances salt tolerance in rice. Plant Physiol. Biochem. 130, 566–576. doi: 10.1016/j.plaphy.2018.08.008
Lin, F., Li, S., Wang, K., Tian, H., Gao, J., Zhao, Q., et al. (2020). A leucine-rich repeat receptor-like kinase, OsSTLK, modulates salt tolerance in rice. Plant Sci. 296:110465. doi: 10.1016/j.plantsci.2020.110465
Lin, H., Zhu, M., Yano, M., Gao, J., Liang, Z., Su, W., et al. (2004). QTLs for Na+ and K+ uptake of the shoots and roots controlling rice salt tolerance. Theor. Appl. Genet. 108, 253–260. doi: 10.1007/s00122-003-1421-y
Liu, G., Li, X., Jin, S., Liu, X., Zhu, L., Nie, Y., et al. (2014). Overexpression of rice NAC gene SNAC1 improves drought and salt tolerance by enhancing root development and reducing transpiration rate in transgenic cotton. PLoS One 9:e86895. doi: 10.1371/journal.pone.0086895
Liu, S. P., Zheng, L. Q., Xue, Y. H., Zhang, Q. A., Wang, L., and Shou, H. X. (2010). Overexpression of OsVP1 and OsNHX1 increases tolerance to drought and salinity in rice. J. Plant Biol. 53, 444–452. doi: 10.1007/s12374-010-9135-6
Mansuri, R. M., Shobbar, Z.-S., Jelodar, N. B., Ghaffari, M., Mohammadi, S. M., and Daryani, P. (2020). Salt tolerance involved candidate genes in rice: an integrative meta-analysis approach. BMC Plant Biol. 20:452. doi: 10.1186/s12870-020-02679-8
Mansuri, R. M., Shobbar, Z.-S., Jelodar, N. B., Ghaffari, M. R., Nematzadeh, G.-A., and Asari, S. (2019). Dissecting molecular mechanisms underlying salt tolerance in rice: a comparative transcriptional profiling of the contrasting genotypes. Rice 12, 1–13.
Mao, C., He, J., Liu, L., Deng, Q., Yao, X., Liu, C., et al. (2020). OsNAC2 integrates auxin and cytokinin pathways to modulate rice root development. Plant Biotechnol. J. 18, 429–442. doi: 10.1111/pbi.13209
Mao, X., Cai, T., Olyarchuk, J. G., and Wei, L. (2005). Automated genome annotation and pathway identification using the KEGG Orthology (KO) as a controlled vocabulary. Bioinformatics 21, 3787–3793. doi: 10.1093/bioinformatics/bti430
Mizuno, H., Kawahara, Y., Sakai, H., Kanamori, H., Wakimoto, H., Yamagata, H., et al. (2010). Massive parallel sequencing of mRNA in identification of unannotated salinity stress-inducible transcripts in rice (Oryza sativa L.). BMC Genomics 11:683. doi: 10.1186/1471-2164-11-683
Obata, T., Kitamoto, H. K., Nakamura, A., Fukuda, A., and Tanaka, Y. (2007). Rice Shaker potassium channel OsKAT1 confers tolerance to salinity stress on yeast and rice cells. Plant Physiol. 144, 1978–1985. doi: 10.1104/pp.107.101154
Parihar, P., Singh, S., Singh, R., Singh, V. P., and Prasad, S. M. (2015). Effect of salinity stress on plants and its tolerance strategies: a review. Environ. Sci. Pollut. Res. 22, 4056–4075. doi: 10.1007/s11356-014-3739-1
Pertea, M., Kim, D., Pertea, G. M., Leek, J. T., and Salzberg, S. L. (2016). Transcript-level expression analysis of RNA-seq experiments with HISAT, StringTie and Ballgown. Nat. Protoc. 11:1650. doi: 10.1038/nprot.2016.095
Qi, Y. H., Yamauchi, Y., Ling, J. Q., Kawano, N., Li, D. B., and Tanaka, K. (2004). Cloning of a putative monogalactosyldiacylglycerol synthase gene from rice (Oryza sativa L.) plants and its expression in response to submergence and other stresses. Planta 219, 450–458.
Serra, T. S., Figueiredo, D. D., Cordeiro, A. M., Almeida, D. M., Lourenco, T., Abreu, I. A., et al. (2013). OsRMC, a negative regulator of salt stress response in rice, is regulated by two AP2/ERF transcription factors. Plant Mol. Biol. 82, 439–455. doi: 10.1007/s11103-013-0073-9
Shen, J., Lv, B., Luo, L., He, J., Mao, C., Xi, D., et al. (2017). The NAC-type transcription factor OsNAC2 regulates ABA-dependent genes and abiotic stress tolerance in rice. Sci. Rep. 7, 1–16. doi: 10.1002/9781118764374.ch1
Shu, K., Qi, Y., Chen, F., Meng, Y., Luo, X., Shuai, H., et al. (2017). Salt stress represses soybean seed germination by negatively regulating GA biosynthesis while positively mediating ABA biosynthesis. Front. Plant Sci. 8:1372. doi: 10.3389/fpls.2017.01372
Takagi, H., Tamiru, M., Abe, A., Yoshida, K., Uemura, A., Yaegashi, H., et al. (2015). MutMap accelerates breeding of a salt-tolerant rice cultivar. Nat. Biotechnol. 33, 445–449. doi: 10.1038/nbt.3188
Takasaki, H., Maruyama, K., Kidokoro, S., Ito, Y., Fujita, Y., Shinozaki, K., et al. (2010). The abiotic stress-responsive NAC-type transcription factor OsNAC5 regulates stress-inducible genes and stress tolerance in rice. Mol. Genet. Genomics 284, 173–183.
Tuteja, N. (2007). Abscisic acid and abiotic stress signaling. Plant Signal. Behav. 2, 135–138. doi: 10.4161/psb.2.3.4156
Vannini, C., Locatelli, F., Bracale, M., Magnani, E., Marsoni, M., Osnato, M., et al. (2004). Overexpression of the rice Osmyb4 gene increases chilling and freezing tolerance of Arabidopsis thaliana plants. Plant J. 37, 115–127.
Wang, J., Zhu, J., Zhang, Y., Fan, F., Li, W., Wang, F., et al. (2018). Comparative transcriptome analysis reveals molecular response to salinity stress of salt-tolerant and sensitive genotypes of indica rice at seedling stage. Sci. Rep. 8:2085.
Wang, Y., Wang, Y., Kai, W., Zhao, B., Chen, P., Sun, L., et al. (2014). Transcriptional regulation of abscisic acid signal core components during cucumber seed germination and under Cu2+, Zn2+, NaCl and simulated acid rain stresses. Plant Physiol. Biochem. 76, 67–76. doi: 10.1016/j.plaphy.2014.01.003
Yang, Y., Chen, H., Jen, W., Liu, L., and Chang, M. (2015). Comparative transcriptome analysis of shoots and roots of TNG67 and TCN1 rice seedlings under cold stress and following subsequent recovery: insights into metabolic pathways, phytohormones, and transcription factors. PLoS One 10:e0131391. doi: 10.1371/journal.pone.0131391
Young, M. D., Wakefield, M. J., Smyth, G. K., and Oshlack, A. (2010). Gene ontology analysis for RNA-seq: accounting for selection bias. Genome Biol. 11, 1–12.
Yuan, L. (1994). “Increasing yield potential in rice by exploitation of heterosis,” in Hybrid Rice Technology: New Developments and Future Prospects, ed. S. S. Virmani (Manila: International Rice Research Institute), 1–6.
Zhang, J. A., Li, J. Q., Wang, X. C., and Chen, J. (2011). OVP1, a Vacuolar H -translocating inorganic pyrophosphatase (V-PPase), overexpression improved rice cold tolerance. Plant Physiol. Biochem. 49, 33–38. doi: 10.1016/j.plaphy.2010.09.014
Keywords: salt stress, comparative transcriptome, Meta-QTLs mapping, rice (Oryza sativa L.), seedling stage
Citation: Kong W, Sun T, Zhang C, Deng X and Li Y (2021) Comparative Transcriptome Analysis Reveals the Mechanisms Underlying Differences in Salt Tolerance Between indica and japonica Rice at Seedling Stage. Front. Plant Sci. 12:725436. doi: 10.3389/fpls.2021.725436
Received: 15 June 2021; Accepted: 07 October 2021;
Published: 27 October 2021.
Edited by:
Crystal Sweetman, Flinders University, AustraliaReviewed by:
Showkat Ganie, Royal Holloway, University of London, United KingdomCopyright © 2021 Kong, Sun, Zhang, Deng and Li. This is an open-access article distributed under the terms of the Creative Commons Attribution License (CC BY). The use, distribution or reproduction in other forums is permitted, provided the original author(s) and the copyright owner(s) are credited and that the original publication in this journal is cited, in accordance with accepted academic practice. No use, distribution or reproduction is permitted which does not comply with these terms.
*Correspondence: Yangsheng Li, bHlzaDIwMDFAd2h1LmVkdS5jbg==
Disclaimer: All claims expressed in this article are solely those of the authors and do not necessarily represent those of their affiliated organizations, or those of the publisher, the editors and the reviewers. Any product that may be evaluated in this article or claim that may be made by its manufacturer is not guaranteed or endorsed by the publisher.
Research integrity at Frontiers
Learn more about the work of our research integrity team to safeguard the quality of each article we publish.