- 1Department of Agronomy and Plant Breeding, Faculty of Agriculture, University of Zanjan, Zanjan, Iran
- 2Agrobiotech for Health, Department of Plant Biology and Soil Science, Faculty of Biology, University of Vigo, Vigo, Spain
- 3Department of Plant Breeding and Biotechnology, University of Tabriz, Tabriz, Iran
- 4Department of Pharmacology, Pharmacy and Pharmaceutical Technology Department, Grupo I+D Farma (GI-1645), Faculty of Pharmacy, University of Santiago, Santiago de Compostela, Spain
The aim of this study was to better understand the response of ex vitro acclimatized plants grown to a set of mineral nutrient combinations based on Hoagland solution. To reach that, two computer-based tools were used: the design of experiments (DOE) and a hybrid artificial intelligence technology that combines artificial neural networks with fuzzy logic. DOE was employed to create a five-dimensional IV-design space by categorizing all macroelements and one microelement (copper) of Hoagland mineral solution, reducing the experimental design space from 243 (35) to 19 treatments. Typical growth parameters included hardening efficiency (Hard), newly formed shoot length (SL), total leaf number (TLN), leaf chlorophyll content (LCC), and leaf area (LA). Moreover, three physiological disorders, namely, leaf necrosis (LN), leaf spot (LS), and curled leaf (CL), were evaluated for each treatment (mineral formulation). All the growth parameters plus LN were successfully modeled using neuro-fuzzy logic with a high train set R2 between experimental and predicted values (72.67 < R2 < 98.79). The model deciphered new insights using different sets of “IF–THEN” rules, pinpointing the positive role of Mg2+ and Ca2+ to improve Hard, SL, TLN, and LA and alleviate LN but with opposite influences on LCC. On the contrary, TLN and LCC were negatively affected by the addition of NO3– into the media, while NH4+ in complex interaction with Cu2+ or Mg2+ positively enhanced SL, TLN, LCC, and LA. In our opinion, the approach and results achieved in this work are extremely fruitful to understand the effect of Hoagland mineral nutrients on the healthy growth of ex vitro acclimatized plants, through identifying key factors, which favor growth and limit physiological abnormalities.
Introduction
The first studies on the development of mineral nutrient solutions for the cultivation of healthy plants date back to the mid-1800s. They are mainly based on physiologically balanced formulations, i.e., Knop formulation (Loew and Aso, 1907). This approach was substituted by testing serial concentrations of elements of Knop. In this case, a similar osmotic concentration for all the media is tested, but each formulation differs from the others in the proportions of nutrient salts (Tottingham, 1914; Shive, 1915; Jones and Shive, 1921). Subsequently, the variation in the pH range of the formulated mineral solution was introduced (Arnon and Johnson, 1942).
Hoagland and colleagues soon reported outstanding improvements in mineral formulations for healthy commercial plant growth (Hoagland, 1920, 1937; Hoagland and Broyer, 1936; Arnon, 1937; Arnon and Hoagland, 1939), but also some associated physiological problems with those formulations (Hoagland and Arnon, 1941, 1948). In 1950, they established the most widely cited and used plant mineral nutrition formulation: Hoagland solution (Hoagland and Arnon, 1950). The new formulation was based on the quantification of the nutrients absorbed by the plant roots followed mainly by the substation of several mineral nutrients until satisfactory results were obtained (Hoagland, 1920; Arnon, 1938). This procedure made the task time-consuming, intensive, and laborious (Hoagland and Arnon, 1950 and references therein).
Although the Hoagland solution has been commonly used for several crops with good results, ex vitro culture of plants is still one of the most important challenges for researchers. For each case, different strengths of Hoagland solution need to be applied or its elements must be readjusted. For instance, a different strength of Hoagland solution (0.125-2×) was tested by Kang and Iersel (2004) for growing Salvia splendens, pinpointing 1–2 × as optimum levels for healthy plant growth. The half strength of Hoagland solution was determined as the best level for the healthy growth of Citrus sp. (Zhou et al., 2020). Also, the serial concentrations of Ca2+ and BO3– were tested based on the half strength of Hoagland to improve the healthy growth of Actinidia deliciosa L. (Sotiropoulos et al., 1999). However, finding an optimized solution of mineral nutrients remains a time-consuming, costly, and tedious task (Nezami-Alanagh et al., 2014).
Recently, computer-based tools for the design of experiments (DOE) made it possible to drastically reduce the number of combinations to be studied compared with traditional factorial designs (Niedz and Evens, 2016). Although DOE has been widely used to improve in vitro plant tissue culture (PTC) practice (Niedz and Evens, 2007; Poothong and Reed, 2015; Ayuso et al., 2017; Nezami-Alanagh et al., 2018, 2019; Chu et al., 2019; Hameg et al., 2020; Pence et al., 2020), as far as we know, there is no report regarding the application of the DOE to ensure adequate sampling of the design space in plant mineral solution formulations.
The next challenge of mineral nutrient studies is understanding ion-specific effects in n-dimensional design spaces. In this situation, the use of artificial intelligence (AI) tools has been recommended as a suitable alternative computational methodology to extract information from complex databases (Gago et al., 2010a; Gallego et al., 2011). Neuro-fuzzy logic is one of the powerful multiscale analysis systems of AI technology with the ability to model non-linear complex systems by setting simple “IF–THEN” rules together with the identification of the key factors to improve a specific response (Landin et al., 2009). In recent years, several studies have proved the efficacy of neuro-fuzzy logic in in vitro culture media improvements (Nezami-Alanagh et al., 2014, 2017; Ayuso et al., 2017), but despite these advances, the development of an optimal mineral formulation for plant growth continues to be a challenge.
Here, the commercially important kiwiberry or hardy kiwi (Atkinson and Macrae, 2007) plants were selected to establish the response of micropropagated plants to a set of mineral formulations during the ex vitro acclimatization (hardening) process. To that end, we implemented DOE to generate a multifactor design space to simultaneously study the influence of Hoagland mineral nutrients on a set of physiological responses, followed by applying neuro-fuzzy logic to model and unveil the key mineral nutrients to decipher hidden relationships between mineral nutrients and the studied parameters.
Materials and Methods
Plant Material and in vitro Culture Conditions
Micropropagated plants of Actinidia arguta (Sieb. and Zucc.) Planch. ex Miq. cv. “Issai” were obtained from the Department of Plant Biology and Soil Sciences (University of Vigo) as described in detail elsewhere (Hameg, 2019; Hameg et al., 2020). Briefly, micro-shoots were proliferated in Cheng medium (Cheng, 1975) containing 1 mg/L N6-benzyladenine (BAP), 1 mg/L gibberellic acid (GA3), 30 g/L sucrose, and 8 g/L agar. Medium pH was adjusted to 5.7 prior to autoclaving (121°C, 1 kg/cm2/s for 20 min). The cultures were kept under 16-h photoperiod (white fluorescent tubes; irradiance of 40 μmol/m2/s) and day/night temperature of 25 ± 1°C and cultured for 50 days (Hameg et al., 2018).
Direct ex vitro Simultaneous Rooting and Acclimatization Culture Conditions
Micro-shoots (∼3 cm in height) obtained from in vitro proliferation medium, after dipping the basal cut end of the micro-shoots in 250 ppm indole-3-butyric acid (IBA) solution for 10 min, were carefully planted into mini-pots (5 × 5 cm2) containing perlite, covered with glass vessels. The mini-pots were transferred into an automated growth room (Sanyo SGC066.CFX.F) under 16-h photoperiod (white fluorescent tubes; irradiance of 200 μmol/m2/s) and 18 ± 3°C. The initial value of relative humidity was set at 100% and decreased gradually during 21 days until 60% was reached (Gago et al., 2010b).
Thereafter, rooted plantlets were watered for 3 months with a set of mineral formulations (Table 1), based on the Hoagland solution (Hoagland and Arnon, 1950). Each replicate consisted of two transplant plastic containers each containing 10 plants. The experiments were carried out in duplicate.
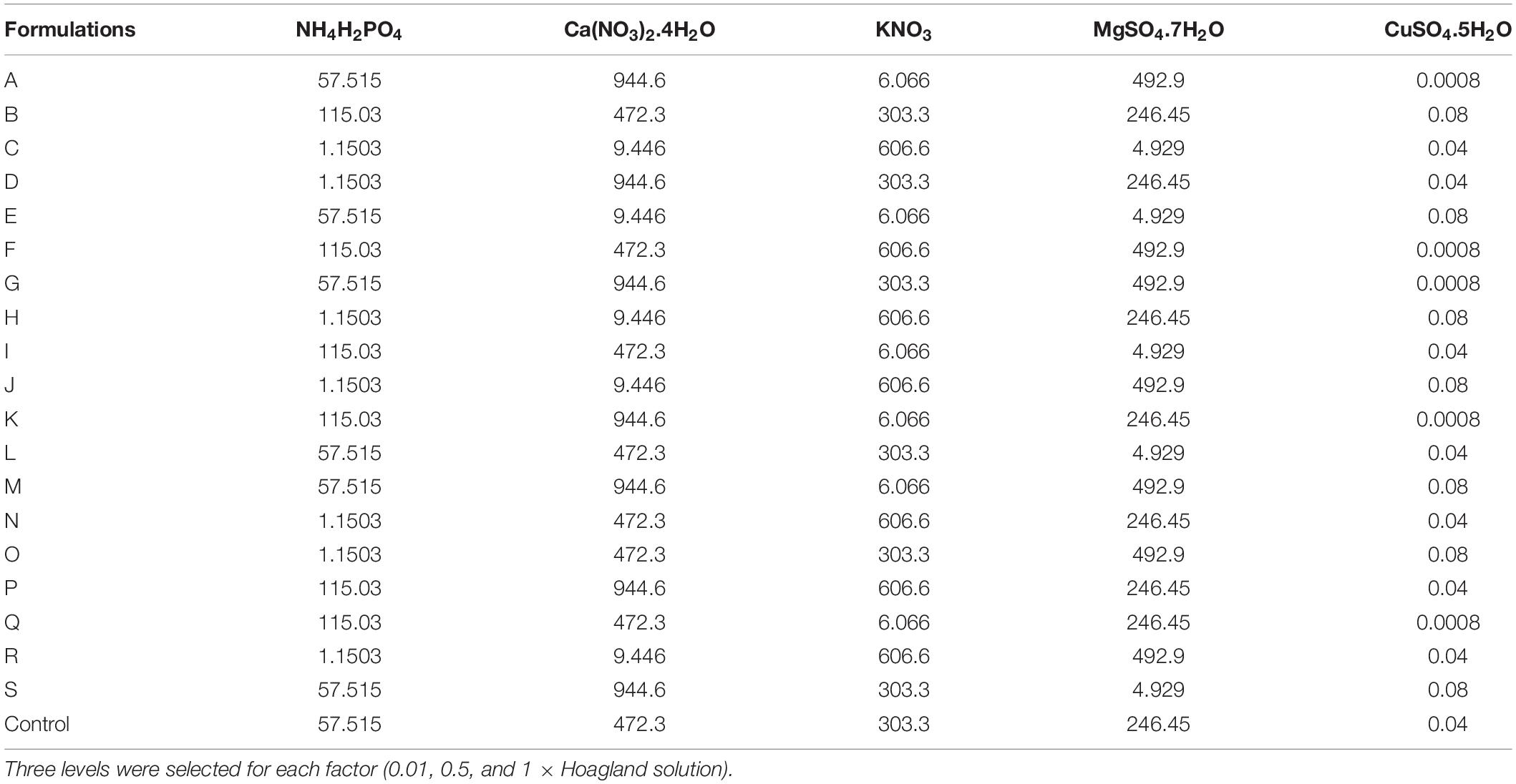
Table 1. Composition of the mineral formulations established by the five-factor design (19 treatments) based on Hoagland mineral nutrients plus half strength of Hoagland solution (as control) in mg/L.
Experimental Design and Data Acquisition
An experimental design was established to study the effects of the combination of six macronutrients (i.e., N, P, Ca, K, S, and Mg) and one micronutrient (Cu) of the Hoagland solution (Hoagland and Arnon, 1950) on the growth and development of acclimatized plants. To this end, the Hoagland salts, namely, (i) NH4H2PO4, (ii) KNO3, (iii) Ca(NO3)2.4H2O, (iv) MgSO4.7H2O, and (v) CuSO4.5H2O, were considered as five independent factors at three levels, expressed as × half-strength Hoagland solution concentrations (Table 1) for ensuring optimal sampling of the design space. In other words, here we select the space delimited by three levels of Hoagland solution varied between 0.01, 0.5, and 1 (×level). This means that various formulations of Hoagland solution were tested: some at a very low concentration of all ions (0.01×); others at middle concentrations (0.5 × level) similar to the control (½ strength), and the rest at full-strength Hoagland solution (1 × level).
The five-factor experimental design was a 19-point using IV-optimal response surface and the software application Design-Expert®8 (Design-Expert, 2010), and another point with half-strength Hoagland salt concentration as control (Table 1). The other salts in the Hoagland solution, including micronutrients and iron, were fixed based on the control medium (Supplementary Table 1).
Samples of plants were irrigated with each mineral formulation. After 3 months, five growth responses and three physiological disorders were evaluated:
1. Hardening efficiency (Hard): percentage of successfully acclimatized plants.
2. Shoot length (SL): length of shoots in cm.
3. Total leaf number (TLN): number of leaves.
4. Leaf chlorophyll content (LCC): measured by SPAD chlorophyll meter (Opti-Sciences CCM-200, United States), expressed as Chlorophyll Content Index (CCI).
5. Leaf area (LA): measured by CI-202 Laser Leaf Area Meter in cm2.
6. Leaf necrosis (LN): number of necrotic leaves per total leaf numbers in %.
7. Curled leaf (CL): number of curled leaves per total leaf numbers in %.
8. Leaf spot (LS): number of spotted leaves per total leaf numbers in %.
Artificial Neural Networks Modeling Tool
FormRules® v4.03 (Intelligensys Ltd., United Kingdom), a neuro-fuzzy logic software that combines artificial neural networks with fuzzy logic, was used to model the results and analyze how the solution components modulate its physiological effects through the simple “IF–THEN” rules that generate with a membership degree, as described in detail previously (Colbourn and Rowe, 2005; Gallego et al., 2011; Landin and Rowe, 2013; Nezami-Alanagh et al., 2018).
Different statistical criteria of software fitting, namely, Cross validation (CV), Leave One Out Cross Validation (LOOCV), Minimum Description Length (MDL), Bayesian Information Criterion (BIC), and the Structural Risk Minimization (SRM), were tested to build the model. Among them, SRM was selected, because it generated the most predictable models along with the minimum generalization error and the simplest and more intelligible rule sets avoiding overtraining (Vapnik, 1992; Shao et al., 2006). All data were used for training since SRM is a statistical significance method; the number of subsets ranged from 1 to 3, and a maximum of 4 inputs per submodel and 15 maximum nodes per input were selected (Table 2) as described elsewhere (García-Pérez et al., 2020; Hameg et al., 2020).
The model quality was assessed using the coefficient of determination of the training set (train set R2), expressed in percentage (for model predictability), and the analysis of variance (ANOVA) parameters (for model accuracy). Train set R2 values are calculated by the following equation (Shao et al., 2006):
where yi is the experimental value in the database, yi′ is the predicted value generated by the model, and yi″ is the mean of the dependent variable.
Train set R2 values between 70 and 99.9% are indicative of acceptable predictabilities, while values higher than 99.9% have been rejected due to model over fitting (Colbourn and Rowe, 2005; Landin et al., 2009). To test model accuracy, the software uses one-way ANOVA to evaluate statistical differences between predicted and experimental data. Models, which computed ratio f-value in the ANOVA greater than critical f-value for the corresponding degrees of freedom (α = 0.01), show good accuracy and no statistical differences among experimental and predicted values (Colbourn and Rowe, 2009).
Modeling was built according to the methodology described previously by Nezami-Alanagh et al. (2017) using the training parameters shown in Table 2.
To avoid the ion confounding effect (Niedz and Evens, 2007), the mineral composition of every formulation was expressed as ion concentrations instead of salt concentrations and introduced as inputs (NH4+, Ca2+, K+, Mg2+, NO3–, PO42–, SO42–, and Cu2+), while physiological parameters (Hard, SL, TLN, LCC, LA, LN, LS, and CL) were selected as outputs.
Results
The reduced experimental design allowed establishing just 19 treatments (mineral formulations) using different proportions of the mineral nutrients of Hoagland solution in a well-sampled design space. As it can be observed (Table 3), while some formulations promoted the highest plant survival (100% Hard) such as A and B, others such as C, F, H, J, and R were completely unviable (0% Hard), all plants showing 100% of leaf necrosis (C, H, J, and R) or 100% of spots (F) and dying during 3 months of acclimatization. Moreover, some other formulations (e.g., B, G, I, L, P, and S) and the control (½ Hoagland strength) also promoted rates of physiological disorders such as leaf spots, curling, and/or necrosis (Table 3). Thus, it is clear that the mineral composition of each formulation assayed plays an essential role in plant survival during the acclimatization procedure. However, these data are not very informative about which component of each formulation caused those positive or negative effects on plant growth and health, thus not much valuable knowledge can be drawn from these results.
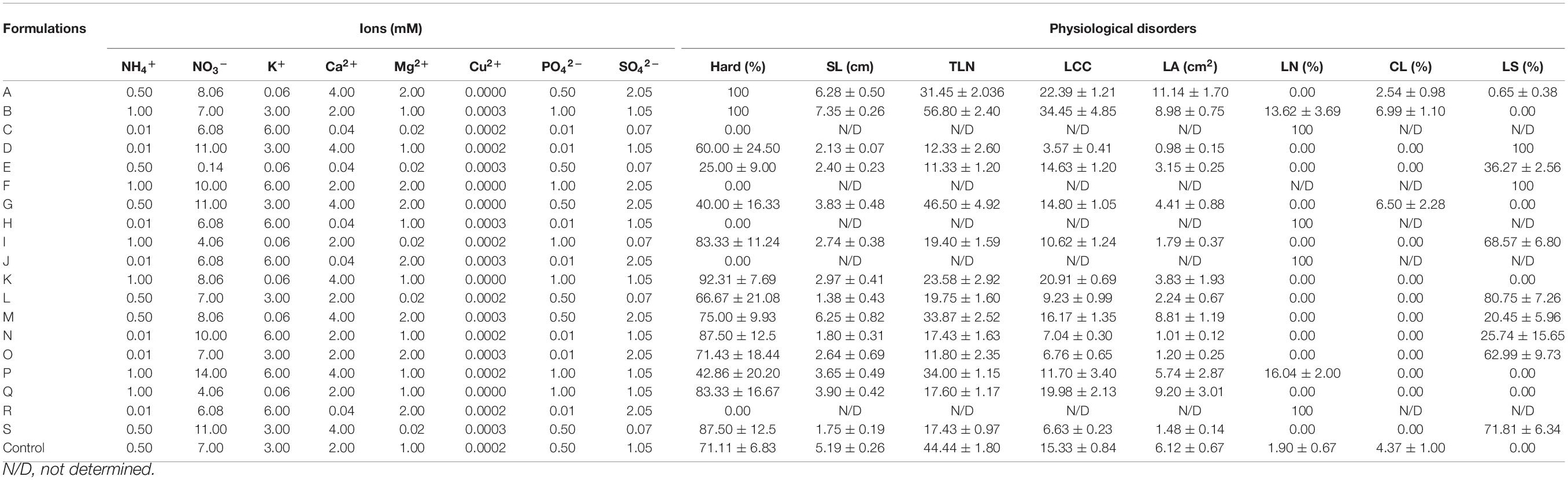
Table 3. Dataset used to build the neuro-fuzzy model, including the mineral ion composition as inputs and the experimental data obtained for each growth response and physiological disorder parameters (expressed as the mean ± standard error) as outputs.
The use of neuro-fuzzy logic models permitted the successful modeling of six out of eight parameters (outputs): Hard, SL, TLN, LCC, LA, and LN with high predictability (72.67 ≤ R2 ≤ 92.84%) and accuracy (f ratio > f critical). However, the reduced number of degrees of freedom did not allow confirmation of the accuracy of CL and LS models, despite their excellent predictability R2 > 93.71% (Figure 1 and Table 4).
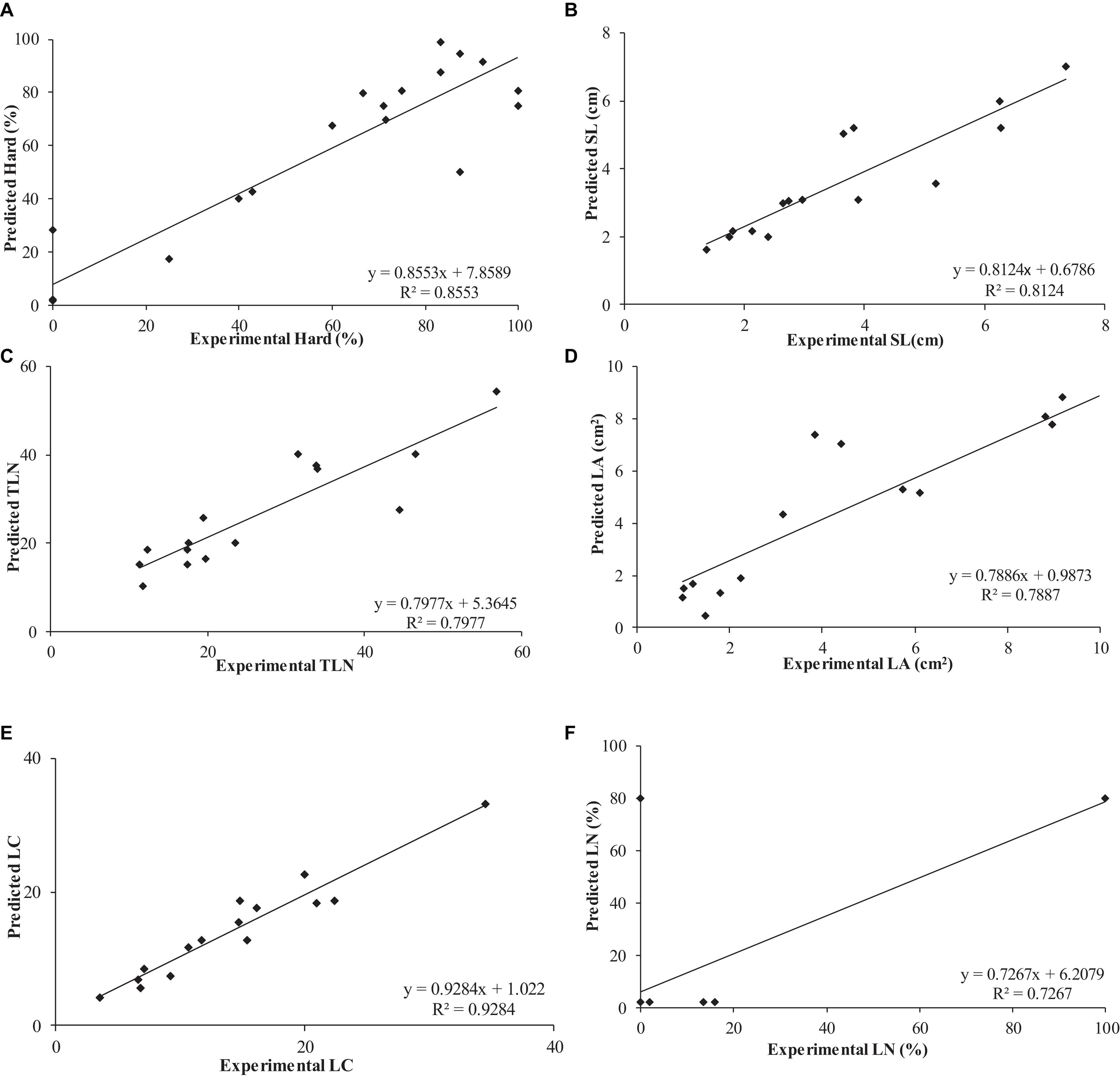
Figure 1. Determination of coefficient (R2) of experimental vs. predicted values obtained by neuro-fuzzy logic models for the different parameters or outputs studied: (A) Hard, (B) SL, (C) TLN, (D) LA, (E) LCC, and (F) LN.
Neuro-fuzzy logic generated 1–3 submodels for each parameter. Two submodels explain the variability of plant survival (Hard): the interaction of NO3– and Mg2+, highlighted as the inputs with the strongest effect, and the independent effect of Ca2+. The variance found for SL (81.24) TLN (79.77), and LCC (92.84) was mainly due to the interaction of NH4+ and Cu2+ (stronger effect) and the independent influence of Mg2+. For LCC, the independent influence of Ca2+ also played an essential role. For LA, the strongest effect was caused by the interaction of NH4+ and Mg2+ and the independent influence of NO3–. Finally, LN variations are explained by variations in Ca2+ ion concentrations (Table 4).
Neuro-fuzzy logic provides a set of useful “IF–THEN” rules to explain those effects and facilitate their understanding using words (linguistic tags), as well as to help researchers in decision-making. Table 5 summarizes all the rules with their membership degree. The rules showing the ion combination with the strongest effect and highest membership (1.00) for each parameter are in bold.
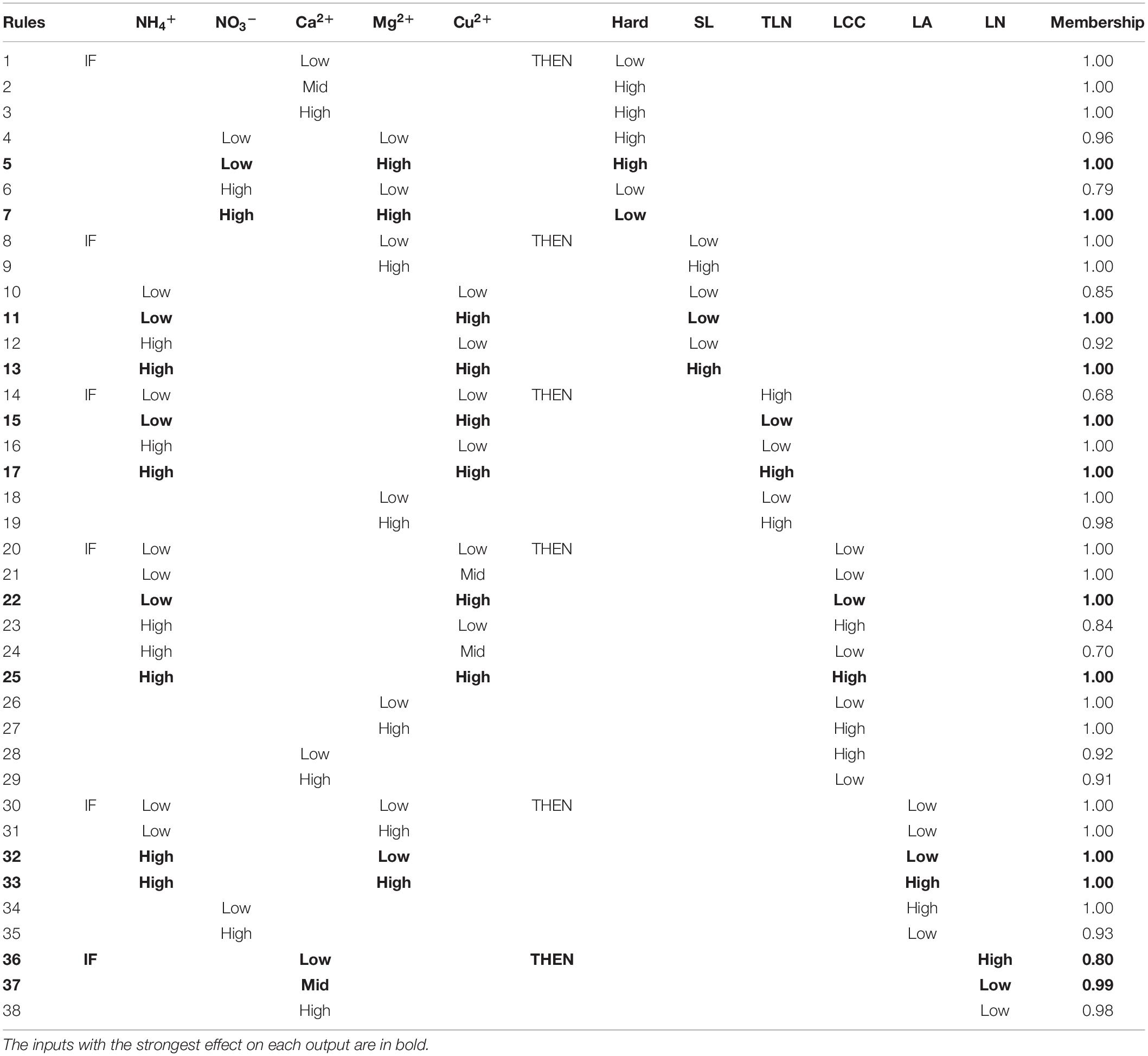
Table 5. Rules generated by neuro-fuzzy logic showing the best combination of inputs to obtain the highest and lowest physiological responses with their membership degree for each output.
The “IF–THEN” rules for Hard indicate that the highest survival of the acclimatized plants was obtained if a low amount of NO3– was supplied into the mineral formulation, particularly if combined with high Mg2+ content (rules 4–5; Table 5). Also, the model recommends supplementing the formulation with mid to high amounts of Ca2+ (rules 2–3; Table 5). The meaning of low, mid, or high concentrations for the different inputs can be found elsewhere (García-Pérez et al., 2020; Hameg et al., 2020).
The analysis of “IF–THEN” rules for SL, TLN, and LCC revealed that only Cu2+ and NH4+ supplied at high concentration lead to the longest shoots, the highest leaf number, and high chlorophyll content (rules 13, 17, and 25, respectively; Table 5). As for Hard, only mineral solutions supplemented with a high concentration of Mg2+ (rules 9, 19, and 27; Table 5) promoted high values for SL, TLN, and LCC. According to these rules, the hardy kiwi plants grown on mineral formulation B containing high concentrations of NH4+, Cu2+, and Mg2+, corresponded to the longest SL (7.35 cm), the highest TLN (56.8 cm), and the second highest (almost nine CCI) LCC (Table 2).
Leaf area was essentially predicted by the interaction of NH4+ and Mg2+: if high NH4+ was combined with high Mg2+, then high LA values (rule 33; Table 5) were achieved, but if high NH4+ was combined with low Mg2+, then low LA values were obtained (rule 32; Table 5). Also, the model pinpointed the negative independent influence of NO3–, only promoting the highest LA when low amount of NO3– was supplied into the mineral formulation (rule 34; Table 5; membership 1.00).
Physiological Disorders
In this study, different types of physiological disorders such as LN, CL, and LS during plant acclimatization were observed (Table 3 and Figure 2). However, only LN could be successfully modeled with the neuro-fuzzy logic, due to insufficient predictability of their models (f ratio < f critical, Table 4).
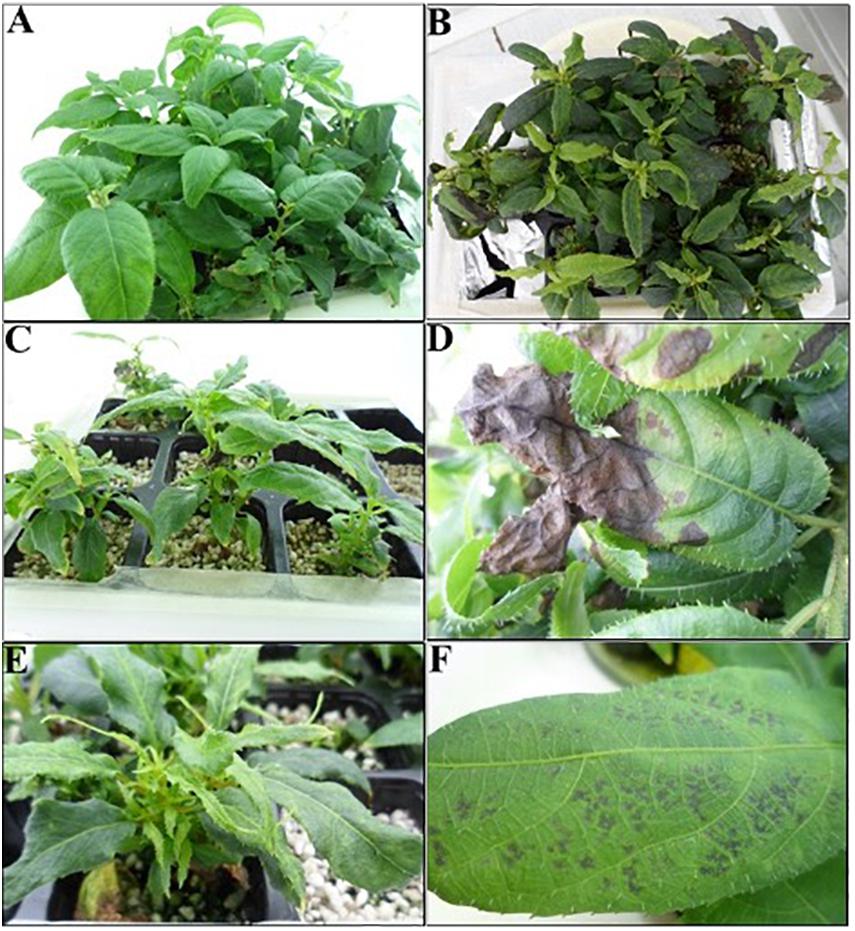
Figure 2. Growth quality of A. arguta plants watered with some mineral formulations in addition to physiological disorders observed in suboptimal media. (A–C) plants watered with (A,B), and control formulations, respectively. Physiological disorders detected during plant acclimatization: (D) leaf necrosis, (E) curling leaf, and (F) leaf spot symptoms.
Leaf necrosis variability is explained by Ca2+ concentration. The mid-high concentration of this ion (>1.03 mM) avoids leaf necrosis (rules 37–38; Table 5).
Discussion
Although Hoagland solution (Hoagland and Arnon, 1950) has basically been set up using asparagus, lettuce, tomato, or wheat (Hoagland, 1923; Arnon, 1937; Arnon and Stout, 1939; Hoagland and Arnon, 1948), subsequently, it has been widely used to irrigate almost all genotypes, including several Actinidia spp. (Sotiropoulos et al., 2005; Liang et al., 2018; Liu et al., 2019; Purohit et al., 2020). In this study, we have used an IV-optimal design space using DOE software through dividing all macroelements plus copper of Hoagland solution into five independent factors at three levels (Table 1) to get a better understanding of the responses of acclimatized plants. This approach allowed us to (i) establish a well-sampled design space and (ii) reduce the number of mineral formulations based on Hoagland levels used in the study from 243 to just 19 combinations.
Among the available machine learning algorithms used in plant nutrition studies (García-Pérez et al., 2020; Hameg et al., 2020; Niazian and Niedbała, 2020), here, the commercial neuro-fuzzy logic (FormRules®) that combines artificial neural networks with fuzzy logic was used to build the mathematical models.
Neuro-fuzzy logic has shown a sounding potential for data mining and generates knowledge from complex datasets of plant tissue culture studies (Gago et al., 2011; Nezami-Alanagh et al., 2019). In this work, the efficiency of this tool can be briefly summarized as (i) generating statistical mathematical models with high predictability (train set R2 > 70%) and accuracy (f ratio > f critical), which explain six out of the eight outputs with the related significant inputs (Table 4) as described by Shao et al. (2006) and (ii) constructing a set of “IF–THEN” rules to elucidate the complex non-linear relationships between inputs and outputs expressed in words (Table 5). As an example, all the plants irrigated with C, H, J, and R formulations did not survive and died within the first 3 weeks of the acclimatization process mainly due to the low Ca2+ content (Table 3), all of them showing the highest (100%) leaf necrosis ratios (Table 5: rules 1 and 36).
Nitrogen (N) is considered one of the essential mineral nutrients for plant growth and development (Raven, 2003; Wang et al., 2012; Liu et al., 2014). Among the N sources, NH4+ and NO3– ions are considered the most important, but plant species have different adaptabilities to uptake and utilize both (Crawford, 1995; Boudsocq et al., 2012; Gonçalves Fernandes, 2019; Asim et al., 2020). All the growth parameters studied were critically affected by one of the N sources or both, independently or in complex interactions with other ions such as Cu2+ or Mg2+ (Table 4). NO3– should be supplied at a low concentration within the design space (6 mM) to improve both Hard and LA, whereas NH4+ should be supplied at high concentrations (0.5 < × < 1.0 mM) in combination with high Cu2+ or Mg2+ to improve SL, TLN, LCC, and LA (Table 5). Thus, the results also suggest that the concentrations of NH4+, Cu2+, and Mg2+ in the Hoagland solution are optimal to irrigate Actinidia spp., particularly kiwiberry (A. arguta). However, the concentrations of salts containing NO3– should be reduced. In agreement with our findings, Clark et al. (2003) observed the preference of cranberry plants to absorb NH4+ compared with NO3– when testing different ratios of NO3–:NH4+ based on Hoagland solution. In their study, the plants receiving NO3– exhibited poorer growth and greater foliar chlorosis compared with plants grown with NH4+. In a recent study (Gonçalves Fernandes, 2019), in which Actinidia sp. plants were irrigated with two levels of these ions (0 and 3 mM) while keeping other mineral nutrients of solution at a fixed concentration, an increase in the lengths of shoots and roots was observed when solutions supplemented with 3 mM NO3– were used, while the plants irrigated with 3 mM NH4+ exhibited higher chlorophyll and protein contents. These controversial results may have their origin in the experimental design, which in our case allows us to detect interactions between nitrogen suppliers and other ions, which undoubtedly influence the preference of plants in the absorption of certain nutrients.
Neuro-fuzzy logic also identified Mg2+ as an ion, which positively affected five out of the six parameters (Hard, SL, TLN, LCC, and LA) independently or in complex interaction with other ions (Table 4). Mg2+ was widely described as an essential divalent cation for plant growth and development, being considered as a mobile element (Tang et al., 2012; Blasco et al., 2015; Rehman et al., 2018; Hauer-Jákli and Tränkner, 2019). It is a structural constituent of chlorophyll molecules and the subsequent transport of photo-assimilates, and it is involved in many biochemical and physiological plant processes. It is also required for the activity of many enzymes of respiration and nucleic acid biosynthesis. The absence of magnesium results in interveinal chlorosis and in premature leaf abscission (Bhatla and Lal, 2018).
Recently, it has been shown that Mg2+ uptake by Actinidia sp. is significantly affected by N-sources available in the solutions, thus plants, whose source of N is exclusively NH4+, present lower Mg values in shoots and roots compared with plants irrigated with NO3– (Gonçalves Fernandes, 2019). The antagonistic effect between Mg2+ and NH4+ may have its origin in uptake competition of these cations through the mechanism of charge balance in ion uptake, since N is a dominant macronutrient and its ionic form controls cation and anion uptake (Borgognone et al., 2013; Gonçalves Fernandes, 2019).
Ca2+ significantly affected three out of the six parameters. Despite the inclusion of a mid-high amount of this cation improving Hard and alleviating LN, the LCC was negatively influenced by high Ca2+ concentrations (Table 5). The dual function of calcium in plants, as the divalent cation, can be summarized in (i) contributing to the cell wall structure and strength and (ii) being a second messenger in many physiological and developmental processes (Thor, 2019). On the contrary, the visual symptoms of Ca2+ deficiency vary among species (de Freitas et al., 2016). In grapevine, it has been related to the appearance of necrosis at the margin of young leaves and the development of necrotic dots, rolling up, leaden, and yellow color in adult leaves (Bavaresco et al., 2010). We have observed leaf tip burning along with the development of necrosis in whole leaves when low amounts of Ca2+ were included in the formulations (Figure 2D). Recently, Teixeira da Silva et al. (2020) reviewed the effect of different ions on shoot tip necrosis (STN) in terms of morphological, biochemical, and molecular aspects, revealing that of all the ions, supply of sufficient Ca2+ in vitro cultures can prevent STN by inhibiting the accumulation of phenolic compounds and thus programmed cell death. Furthermore, the excessive synthesis and transport of auxin and ethylene in undesirable conditions were shown to decrease the mobility of Ca2+within a plant, resulting in Ca2+ deficiency and STN. They finally concluded that STN of in vitro shoots and/or plantlets can be hindered or reversed by altering the basal medium, mainly the concentration of Ca2+, adjusting the levels of auxins or cytokinins, or modifying culture conditions.
The copper ion (Cu2+) is considered an essential micronutrient that governs several important physiological roles during plant growth and development, mainly through its catalytic role in photosynthesis, respiration, and formation of lignin in the cell wall (Cuypers et al., 2000; Borghi et al., 2008; Printz et al., 2016). Our results pinpointed that this ion played an essential role in three out of the eight parameters (SL, TLN, and LCC) in interaction with NH4+. Both ions supplied at high concentration always (membership = 1) lead to long shoots, many leaves, and high chlorophyll contents. However, high Cu2+ concentrations combined with low NH4+ contents promote the opposite effect: short shoots, low leaf number, and chlorophyll content. These results suggest that the effect described on those growth and physiological parameters was more related to the level of NH4+ than the effect per se of the Cu2+.
In conclusion, the use of a reduced experimental design together with artificial intelligence tools has allowed us to study the simple or combined effect of nutrients in complex mineral formulations. Moreover, it has allowed us to establish the suitability of the full-strength Hoagland solution or propose its adjustment for better growth of the A. arguta plant during its acclimatization.
The nitrogen ions (NH4+ and NO3–) are essential to maintain plant growth and development. While the mathematical model obtained recommends maintaining the level of NH4+, Ca2+, Mg2+, and Cu2+ established in the full-strength Hoagland solution for irrigating kiwiberry plants, the NO3– concentration should be reduced for improving plant hardening (e.g., at half the KNO3– salt content of Hoagland solution).
Data Availability Statement
The original contributions presented in the study are included in the article/Supplementary Material, further inquiries can be directed to the corresponding author/s.
Author Contributions
SM performed the experiments. SM, BMZ, BK, and PG conceived and designed the experiments. SM, ML, and PG performed the DOE and machine learning models. SM and PG wrote the first draft of the manuscript. All authors contributed to manuscript revision, and read and approved the submitted version.
Funding
This study was partially financed by Grant No: 960610 of the Biotechnology Development Council of the Islamic Republic of Iran.
Conflict of Interest
The authors declare that the research was conducted in the absence of any commercial or financial relationships that could be construed as a potential conflict of interest.
Publisher’s Note
All claims expressed in this article are solely those of the authors and do not necessarily represent those of their affiliated organizations, or those of the publisher, the editors and the reviewers. Any product that may be evaluated in this article, or claim that may be made by its manufacturer, is not guaranteed or endorsed by the publisher.
Acknowledgments
The experimental part of this study was conducted at the University of Vigo by SM during her research stay at PG’s lab. We would like to thank the Department of Agronomy and Plant Breeding, Faculty of Agriculture, University of Zanjan and Department of Plant Biology and Soil Science, Faculty of Biology, Vigo University for the use of their equipment and facilities. We would also like to thank Sophie Eis for English language editing and review.
Supplementary Material
The Supplementary Material for this article can be found online at: https://www.frontiersin.org/articles/10.3389/fpls.2021.723992/full#supplementary-material
References
Arnon, D. I. (1937). Ammonium and nitrate nitrogen nutrition of barley at different seasons in relation to hydrogen-ion concentration, manganese, copper and hydrogen supply. Soil Sci. 44, 91–122.
Arnon, D. I. (1938). Microelements in culture-solution experiments with higher plants. Am. J. Bot. 25, 322–325. doi: 10.1002/j.1537-2197.1938.tb09223.x
Arnon, D. I., and Hoagland, D. R. (1939). A comparison of water culture and soil as media for crop production. Science 89, 512–514. doi: 10.1126/science.89.2318.512
Arnon, D. I., and Johnson, C. M. (1942). Influence of hydrogen ion concentration on the growth of higher plants under controlled conditions. Plant Physiol. 17, 525–539. doi: 10.1104/pp.17.4.525
Arnon, D. I., and Stout, P. R. (1939). Molybdenum as an essential element for higher plants. Plant Physiol. 14, 599–602. doi: 10.1104/pp.14.3.599
Asim, M., Ullah, Z., Xu, F., An, L., Aluko, O. O., Wang, Q., et al. (2020). Nitrate signaling, functions, and regulation of root system architecture: insights from Arabidopsis thaliana. Genes 11:633. doi: 10.3390/genes11060633
Atkinson, R. G., and Macrae, E. A. (2007). “Kiwifruit” in Transgenic Crop. eds E. C. Pua and M. R. Davey (Berlin: Springer).
Ayuso, M., Ramil-Rego, P., Landin, M., Gallego, P. P., and Barreal, M. E. (2017). Computer-assisted recovery of threatened plants: keys for breaking seed dormancy of Eryngium viviparum. Front. Plant Sci. 8:2092. doi: 10.3389/fpls.2017.02092
Bavaresco, L., Gatti, M., and Fregoni, M. (2010). “Nutritional deficiencies,” in Methodologies and Results in Grapevine Research. Eds S. Delrot, H. Medrano, E. Or, L. Bavaresco, and S. Grando. (Dordrecht: Springer). 165–191. doi: 10.1007/978-90-481-9283-0_12
Bhatla, S. C., and Lal, A. M. (2018). Plant Physiology, Development and Metabolism. Germany: Springer. doi: 10.1007/978-981-13-2023-1
Blasco, B., Graham, N. S., and Broadley, M. R. (2015). Antioxidant response and carboxylate metabolism in Brassica rapa exposed to different external Zn, Ca, and Mg supply. J. Plant Physiol. 176, 16–24. doi: 10.1016/j.jplph.2014.07.029
Borghi, M., Tognetti, R., Monteforti, G., and Sebastiani, L. (2008). Responses of two poplar species (Populus alba and Populus x canadensis) to high copper concentrations. Environ. Exp. Bot. 62, 290–299. doi: 10.1016/j.envexpbot.2007.10.001
Borgognone, D., Colla, G., Rouphael, Y., Cardarelli, M., Rea, E., and Schwarz, D. (2013). Effect of nitrogen form and nutrient solution pH on growth and mineral composition of self-grafted and grafted tomatoes. Sci. Hortic. 149, 61–69. doi: 10.1016/j.scienta.2012.02.012
Boudsocq, S., Niboyet, A., Lata, J. C., Raynaud, X., Loeuille, N., Mathieu, J., et al. (2012). Plant preference for ammonium versus nitrate: a neglected determinant of ecosystem functioning? Am. Nat. 180, 60–69. doi: 10.1086/665997
Cheng, T.-Y. (1975). Adventitious bud formation in cultire of Douglas fir (Pseudotsuga menziesii (Mirb.) Franco). Plant Sci. Lett. 5, 97–102.
Chu, U. C., Adelberg, J., Lowe, K., and Jones, T. J. (2019). Use of DoE methodology to optimize the regeneration of high-quality, single-copy transgenic Zea mays L. (maize) plants. Vitr. Cell. Dev. Biol. Plant 55, 678–694. doi: 10.1007/s11627-019-10002-w
Clark, M. B., Mills, H. A., Robacker, C. D., and Latimer, J. G. (2003). Influence of nitrate: ammonium ratios on growth and elemental concentration in two azalea cultivars. J. Plant Nutr. 26, 2503–2520. doi: 10.1081/PLN-120025475
Colbourn, E. A., and Rowe, R. C. (2005). “Neural computing and pharmaceutical formulation” in Encyclopaedia of Pharmaceutical Technology. eds J. Swarbrick and J. Boylan (New York: Marcel Dekker). 145–157.
Colbourn, E. A., and Rowe, R. C. (2009). Novel approaches to neural and evolutionary computing in pharmaceutical formulation: challenges and new possibilities. Future Med. Chem. 1, 713–726. doi: 10.4155/fmc.09.57
Crawford, N. M. (1995). Nitrate: nutrient and signal for plant growth. Plant Cell 7, 859–868. doi: 10.1105/tpc.7.7.859
Cuypers, A., Vangronsveld, J., and Clijsters, H. (2000). Biphasic effect of copper on the ascorbate-glutathione pathway in primary leaves of Phaseolus vulgaris seedlings during the early stages of metal assimilation. Physiol. Plant. 110, 512–517. doi: 10.1111/j.1399-3054.2000.1100413.x
de Freitas, S. T., do Amarante, C. V. T., and Mitcham, E. J. (2016). “Calcium deficiency disorders in plants” in Postharvest Ripening Physiology of Crops. ed. S. Pareek (Florida: CRC Press). 477–512.
Gago, J., Landín, M., and Gallego, P. P. (2010a). Artificial neural networks modeling the in vitro rhizogenesis and acclimatization of Vitis vinifera L. J. Plant Physiol. 167, 1226–1231. doi: 10.1016/j.jplph.2010.04.008
Gago, J., Martínez-Núñez, L., Landín, M., and Gallego, P. P. (2010b). Artificial neural networks as an alternative to the traditional statistical methodology in plant research. J. Plant Physiol. 167, 23–27. doi: 10.1016/j.jplph.2009.07.007
Gago, J., Pérez-Tornero, O., Landín, M., Burgos, L., and Gallego, P. P. (2011). Improving knowledge of plant tissue culture and media formulation by neurofuzzy logic: a practical case of data mining using apricot databases. J. Plant Physiol. 168, 1858–1865. doi: 10.1016/j.jplph.2011.04.008
Gallego, P. P., Gago, J., and Landin, M. (2011). “Artificial Neural Networks Technology to Model and Predict Plant Biology Process” in Artificial Neural Networks - Methodological Advances and Biomedical Applications. (Rijeka: InTech). 197–216. doi: 10.5772/14945
García-Pérez, P., Lozano-Milo, E., Landín, M., and Gallego, P. P. (2020). Combining medicinal plant in vitro culture with machine learning technologies for maximizing the production of phenolic compounds. Antioxidants 9:210. doi: 10.3390/antiox9030210
Gonçalves Fernandes, A. P. (2019). Effect of Different Forms of Nitrogen Nutrition on Kiwifruit Development and Resistance to Pseudomonas Syringae pv. actinidiae. Portugal: University of Porto. 1–85.
Hameg, R. (2019). Computer-based Tools for Designing Plant Tissue Culture Media. Spain: University of Vigo. 1–215.
Hameg, R., Arteta, T., Gallego, P. P., and Barreal, M. E. (2018). Selecting an efficient proliferation medium for Actinidia arguta ‘Issai’ explants. Acta Hortic. 1218, 565–572. doi: 10.17660/ActaHortic.2018.1218.77
Hameg, R., Arteta, T. A., Landin, M., Gallego, P. P., and Barreal, M. E. (2020). Modeling and optimizing culture medium mineral composition for in vitro propagation of Actinidia arguta. Front. Plant Sci. 11:554905. doi: 10.3389/fpls.2020.554905
Hauer-Jákli, M., and Tränkner, M. (2019). Critical leaf magnesium thresholds and the impact of magnesium on plant growth and photo-oxidative defense: a systematic review and meta-analysis from 70 years of research. Front. Plant Sci. 10:766. doi: 10.3389/fpls.2019.00766
Hoagland, D. R. (1937). Some aspects of the salt nutrition of higher plants. Bot. Rev. 3, 307–334. doi: 10.1007/BF02872346
Hoagland, D. R., and Arnon, D. I. (1941). Pysiological aspects of nutrient plant growth. Soil Sci. 51, 431–444.
Hoagland, D. R., and Arnon, D. I. (1948). Some problems with plant nutrition. Sci. Mon. 67, 201–209.
Hoagland, D. R., and Arnon, D. S. (1950). The water culture method for growing plants without soil. Calif. Agric. Exp. Stn. Circ. 347:32.
Hoagland, D. R., and Broyer, T. C. (1936). General nature of the process of salt accumulation by roots with description of experimental methods. Plant Physiol. 11, 471–507. doi: 10.1104/pp.11.3.471
Jones, L. H., and Shive, J. W. (1921). Effect of Ammonium Sulphate Upon Plants in Nutrient Solutions Supplied with Ferric Phosphate and Ferrous Sulphate as Sources of Iron. United States: United States Department of Agriculture. 701–728.
Kang, J., and Iersel, M. W. V. (2004). Affects shoot: root ratio, leaf area ratio, and growth of subirrigated salvia (Salvia splendens). Shoot 39, 49–54.
Landin, M., and Rowe, R. C. (2013). Artificial Neural Networks Technology to Model, Understand, and Optimize Drug Formulations. United Kingdom: Woodhead Publishing Limited. doi: 10.1533/9781908818508.7
Landin, M., Rowe, R. C., and York, P. (2009). Advantages of neurofuzzy logic against conventional experimental design and statistical analysis in studying and developing direct compression formulations. Eur. J. Pharm. Sci. 38, 325–331.
Liang, D., Gao, F., Ni, Z., Lin, L., Deng, Q., Tang, Y., et al. (2018). Melatonin improves heat tolerance in kiwifruit seedlings through promoting antioxidant enzymatic activity and glutathione S-transferase transcription. Molecules 23, 1–11. doi: 10.3390/molecules23030584
Liu, X., Xia, H., Yuan, X., Lv, X., and Liang, D. (2019). Effects of exogenous melatonin on the physiological characteristics of kiwifruit Hayward under salt stress. IOP Conf. Ser. Earth Environ. Sci. 237, 8–13. doi: 10.1088/1755-1315/237/5/052076
Liu, X. Y., Koba, K., Makabe, A., and Liu, C. Q. (2014). Nitrate dynamics in natural plants: insights based on the concentration and natural isotope abundances of tissue nitrate. Front. Plant Sci. 5:1–14. doi: 10.3389/fpls.2014.00355
Loew, O., and Aso, K. (1907). On physiologically balanced solutions. Shokubutsugaku Zasshi. 21, 68–83. doi: 10.15281/jplantres1887.21.243_68
Nezami-Alanagh, E., Garoosi, G.-A., Haddad, R., Maleki, S., Landin, M., and Gallego, P. P. (2014). Design of tissue culture media for efficient Prunus rootstock micropropagation using artificial intelligence models. Plant Cell. Tissue Organ. Cult. 117, 349–359. doi: 10.1007/s11240-014-0444-1
Nezami-Alanagh, E., Garoosi, G.-A., Landín, M., and Gallego, P. P. (2018). Combining DOE with neurofuzzy logic for healthy mineral nutrition of pistachio rootstocks in vitro culture. Front. Plant Sci. 9:1474. doi: 10.3389/fpls.2018.01474
Nezami-Alanagh, E., Garoosi, G. A., Landín, M., and Gallego, P. P. (2019). Computer-based tools provide new insight into the key factors that cause physiological disorders of pistachio rootstocks cultured in vitro. Sci. Rep. 9, 1–15. doi: 10.1038/s41598-019-46155-2
Nezami-Alanagh, E., Garoosi, G.-A., Maleki, S., Landín, M., and Gallego, P. P. (2017). Predicting optimal in vitro culture medium for Pistacia vera micropropagation using neural networks models. Plant Cell. Tissue Organ Cult. 129, 19–33. doi: 10.1007/s11240-016-1152-9
Niazian, M., and Niedbała, G. (2020). Machine learning for plant breeding and biotechnology. Agriculture 10:436. doi: 10.3390/agriculture10100436
Niedz, R. P., and Evens, T. J. (2007). Regulating plant tissue growth by mineral nutrition. Vitr. Cell. Dev. Biol. Plant 43, 370–381. doi: 10.1007/s11627-007-9062-5
Niedz, R. P., and Evens, T. J. (2016). Design of experiments (DOE)—history, concepts, and relevance to in vitro culture. Vitr. Cell. Dev. Biol. Plant 52, 547–562. doi: 10.1007/s11627-016-9786-1
Pence, V. C., Finke, L. R., and Niedz, R. P. (2020). Evaluating a DOE screen to reduce hyperhydricity in the threatened plant, Cycladenia humilis var. jonesii. Vitr. Cell. Dev. Biol. Plant 56, 215–229. doi: 10.1007/s11627-019-10038-y
Poothong, S., and Reed, B. M. (2015). Increased CaCl2, MgSO4, and KH2PO4 improve the growth of micropropagated red raspberries. Vitr. Cell. Dev. Biol. Plant 51, 648–658. doi: 10.1007/s11627-015-9720-y
Printz, B., Lutts, S., Hausman, J.-F., and Sergeant, K. (2016). Copper trafficking in plants and its implication on cell wall dynamics. Front. Plant Sci. 7:601. doi: 10.3389/fpls.2016.00601
Purohit, S., Rawat, J. M., Pathak, V. K., Singh, D. K., and Rawat, B. (2020). A hydroponic-based efficient hardening protocol for in vitro raised commercial kiwifruit (Actinidia deliciosa). Vitr. Cell. Dev. Biol. Plant. doi: 10.1007/s11627-020-10127-3
Raven, J. (2003). Can plants rely on nitrate? Trends Plant Sci. 8, 314–315. doi: 10.1016/S1360-1385(03)00125-0
Rehman, H. U., Alharby, H. F., Alzahrani, Y., and Rady, M. M. (2018). Magnesium and organic biostimulant integrative application induce physiological and biochemical changes in sunflower plants and its harvested progeny on sandy soil. Plant Physiol. Biochem. 126, 97–105. doi: 10.1016/j.plaphy.2018.02.031
Shao, Q., Rowe, R. C., and York, P. (2006). Comparison of neurofuzzy logic and neural networks in modelling experimental data of an immediate release tablet formulation. Eur. J. Pharm. Sci. 28, 394–404.
Sotiropoulos, T. E., Therios, I. N., and Dimassi, K. N. (1999). Calcium application as a means to improve tolerance of kiwifruit (Actinidia deliciosa L.) to boron toxicity. Sci. Hortic. 81, 443–449. doi: 10.1016/S0304-4238(99)00028-X
Sotiropoulos, T. E., Therios, I. N., and Dimassi, K. N. (2005). Uptake of boron by kiwifruit plants under various levels of shading and salinity. J. Plant Nutr. 27, 1979–1989. doi: 10.1081/pln-200030091
Tang, N., Li, Y., and Chen, L.-S. (2012). Magnesium deficiency-induced impairment of photosynthesis in leaves of fruiting Citrus reticulata trees accompanied by up-regulation of antioxidant metabolism to avoid photo-oxidative damage. J. Plant Nutr. Soil Sci. 175, 784–793. doi: 10.1002/jpln.201100329
Teixeira da Silva, J. A., Nezami-Alanagh, E., Barreal, M. E., Kher, M. M., Wicaksono, A., Gulyás, A., et al. (2020). Shoot tip Necrosis of in Vitro Plant Cultures: a Reappraisal of Possible Causes and Solutions. Berlin: Springer. doi: 10.1007/s00425-020-03449-4
Thor, K. (2019). Calcium—nutrient and messenger. Front. Plant Sci. 10:440. doi: 10.3389/fpls.2019.00440
Tottingham, W. E. (1914). A quantitative chemical and physiological study of nutrient solutions for plant cultures. J. Ecol. 2, 182–185.
Vapnik, V. (1992). Principles of Risk Minimization for Learning Theory. USA: AT &T Bell Laboratories. 831–838.
Wang, Y. Y., Hsu, P. K., and Tsay, Y. F. (2012). Uptake, allocation and signaling of nitrate. Trends Plant Sci. 17, 458–467. doi: 10.1016/j.tplants.2012.04.006
Keywords: Actinidia arguta, artificial intelligence, ex vitro acclimatization, DOE, kiwiberry, machine learning, healthy plants, physiological disorders
Citation: Maleki S, Maleki Zanjani B, Kohnehrouz BB, Landin M and Gallego PP (2021) Computer-Based Tools Unmask Critical Mineral Nutrient Interactions in Hoagland Solution for Healthy Kiwiberry Plant Acclimatization. Front. Plant Sci. 12:723992. doi: 10.3389/fpls.2021.723992
Received: 11 June 2021; Accepted: 29 September 2021;
Published: 28 October 2021.
Edited by:
Pierre Bonnet, CIRAD, UMR AMAP, FranceReviewed by:
Ali Parsaeimehr, Delaware State University, United StatesMaria Carmen Gomez-Jimenez, University of Extremadura, Spain
Copyright © 2021 Maleki, Maleki Zanjani, Kohnehrouz, Landin and Gallego. This is an open-access article distributed under the terms of the Creative Commons Attribution License (CC BY). The use, distribution or reproduction in other forums is permitted, provided the original author(s) and the copyright owner(s) are credited and that the original publication in this journal is cited, in accordance with accepted academic practice. No use, distribution or reproduction is permitted which does not comply with these terms.
*Correspondence: Bahram Maleki-Zanjani, Ym1hbGVraXpAem51LmFjLmly; Pedro Pablo Gallego, cGdhbGxlZ29AdXZpZ28uZXM=