- 1College of Agronomy and Biotechnology, China Agricultural University, Beijing, China
- 2College of Resources and Environmental Sciences, China Agricultural University, Beijing, China
- 3Engineering Technology Research Center for Agriculture in Low Plain Areas, Cangzhou, China
Nitrogen (N) remobilization is a critical process that provides substantial N to winter wheat grains for improving yield productivity. Here, the remobilization of N from anthesis to maturity in two wheat cultivars under three irrigation regimes was measured and its relationship to organ N concentration was examined. Based on spectral data of organ powder samples, partial least squares regression (PLSR) models were calibrated to estimate N concentration (Nmass) and validated against laboratory-based measurements. Although spectral reflectance could accurately estimate Nmass, the PLSR-based Nmass-spectra predictive model was found to be organ-specific, organs at the top canopy (chaff and top three leaves) received the best predictions (R2 > 0.88). In addition, N remobilization efficiency (NRE) in the top two leaves and top third internode was highly correlated with its corresponding N concentration change (ΔNmass) with an R2 of 0.90. ΔNmass of the top first internode (TIN1) explained 78% variation of the whole-plant NRE. This study provides a proof of concept for estimating N concentration and assessing N remobilization using hyperspectral data of individual organs, which offers a non-chemical and low-cost approach to screen germplasms for an optimal NRE in drought-resistance breeding.
Introduction
Wheat is one of the three major cereal crops providing over 700 million tons of grain worldwide per annual (FAO, 2019). The high yield potential and grain quality in wheat are dependent on the uptake and utilization of nutrients, from which nitrogen (N) is a key composition of chlorophyll maintaining photosynthesis assimilates that determines the grain yield ultimately. N also composes the gluten protein which is used for improving the viscoelastic properties of food products (Shewry, 2009). Previous studies have demonstrated that around 60–90% of N in grains at maturity (Barbottin et al., 2005; White et al., 2016) is remobilized from vegetative organs in cereal crops. In wheat, this proportion could be as high as 95% (Kichey et al., 2007). Post-anthesis N remobilization efficiency (NRE) is taken as an essential criterion for evaluating N recycling (Have et al., 2017). In addition to the genetic variation (Gaju et al., 2014), NRE varies between wheat organs, where leaves have higher NRE than stems and chaff (Pask et al., 2012). Besides, water deficit has been found to affect the N remobilization (Xu et al., 2006), and can result in an improved NRE in wheat (Bahrani et al., 2011). It has also been reported in rice that moderate soil drying is beneficial to improve NRE without sacrifice yield potential (Wang et al., 2016), while in most cases, water deficit or less irrigation is found to lead to lower productivity (Sun et al., 2006). Hence, balancing grain yield loss and N remobilization improvement is an eternal topic of sustainable agriculture.
N content varies significantly in different growth stages, especially after anthesis when N remobilizes from vegetative organs (including leaves, stems, and sheaths) to grains during the process of plant senescence and grain development in cereal crops (Bidinger et al., 1977; Yang and Zhang, 2006). Thus, optimizing NRE is critical to improve grain yield and N use efficiency. Typically, NRE is determined by N remobilization amount (NRA) in vegetative organs at anthesis and maturity (Gaju et al., 2014), while the estimation of NRE involves two key steps, dry mass (DM) and N concentration determination. The Kjedahl method is a traditional wet-chemical approach for measuring N concentration in plant tissues (Bertheloot et al., 2008). Alternatively, the combustion-based approach such as the Dumas method can also measure N concentration accurately (Simonne et al., 1994). However, these methods are usually labor-intensive and may cause environmental contamination (Galvez-Sola et al., 2015). Among others, the complex procedures of these laboratory-based N concentration determination methods are the major limiting factors of determining NRE in a large number of samples. Therefore, efficient evaluation of NRE requires a rapid and environmental-friendly method.
Near-infrared spectroscopy (NIRS) is considered as a “green” analytical tool for determining N concentration (Galvez-Sola et al., 2015; Gredilla et al., 2016). In addition to NIRS, the spectrum at the visible (VIS) region associated with chlorophylls absorption can also reflect nitrogen variations (Asner and Martin, 2008; Meacham-Hensold et al., 2020). Recently, spectral reflectance acquired by hyperspectral sensor (VIS and NIRS) instruments has been increasingly used for predicting N concentration in leaves (Ely et al., 2019; Meacham-Hensold et al., 2020), shoots (Nguyen et al., 2019), grains (Caporaso et al., 2018a), and the entire plants (Li et al., 2010; He J. et al., 2020). By analyzing the full-spectrum data with chemometric modeling techniques such as partial least square regression (PLSR), nutrient elements (e.g., N and micronutrients) could be estimated from hyperspectral reflectance (Vigneau et al., 2011; Serbin et al., 2012). The capability of estimating N concentration from spectroscopy has been verified to be robust in many previous studies, such as the NIRS (e.g., 830–2600 nm) for citrus leaf N (Galvez-Sola et al., 2015), the VIS-near-infrared (VIS-NIR, 400–1000 nm) spectroscopic analysis for cacao tree leaf N (Malmir et al., 2019), and the hyperspectral (VIS-NIR-SWIR, 350–2500 nm) determination for leaf N in various crop species. Despite its success in evaluating N status at leaf or canopy levels (Yu et al., 2013; Ely et al., 2019; Hasituya et al., 2020), the capability of using plant spectroscopy to characterize the N variations simultaneously in morphologically distinct organs and the feasibility of such an approach in evaluating the reallocation of N between organ tissues are rarely investigated (Vilmus et al., 2014).
As an alternative to NIRS, hyperspectral imaging (HSI) has several advantages to obtain spectral reflectance and analyze chemical properties (Caporaso et al., 2018a; Meacham-Hensold et al., 2020). HSI holds three-dimensional data that involves not only spectral data but also spatial information of the samples from the captured images (Gao and Smith, 2015; Fu et al., 2020), which makes it possible to investigate the variability of samples with texture differences. Hyperspectral images could be rapidly acquired with an HSI system under the controlled light condition as well as outdoor platforms with sunlight calibration in the field (Caporaso et al., 2018b; Malmir et al., 2019; Fu et al., 2020; Meacham-Hensold et al., 2020). Thanks to the flexibility of imaging a variety of samples, HSI enables rapid and repeatable measurements of N traits on the individual organs of the same plants, which brings new opportunities to study the variations and dynamics of plant-organ N traits and gain insights into the response of plant N reallocation to drought stress (Bertheloot et al., 2008; Hawkesford, 2017).
Therefore, this study intended to investigate the feasibility of using HSI-based spectroscopy to predict N variations in organs and to further evaluate the post-anthesis N remobilization in wheat organs under different irrigation regimes. For this aim, our main objectives were (1) to compare NRE between organs under different irrigation regimes, (2) to develop organ-specific N concentration prediction models using spectral reflectance, and (3) to evaluate N remobilization through N concentration change.
Materials and Methods
Field Treatments and Experimental Design
Two winter wheat (Triticum aestivum L.) cultivars of Jimai22 (JM22, high-yielding and cold-resistant cultivar) and Nongda399 (ND399, fast-growing and drought-resistant cultivar) were planted at Wuqiao Experimental Station of China Agricultural University, Cangzhou (37°41′N, 116°36′E), Hebei Province, China, in 2018–2019 winter wheat growing season. Soil fertility (0–20 cm) and characteristics of climate in the experimental filed are shown in Supplementary Table 1 and Supplementary Figure 1. During wheat growing season in this study, the experimental site received a total 66.6 mm precipitation, 3843 MJ/m2 solar radiation, 2021 sunshine hours, a daily average air temperature of 10.2°C, and a cumulative temperature above 0°C of 2977 degree-days (°C⋅d). Fertilizers were applied before sowing with a total of 240 kg N ha–1, 140 kg P2O5 ha–1, and 120 kg K2O ha–1, which were broadcast incorporated into the 20 cm surface layer of soil just before rotary tillage. Soil water content of 0–200 cm was determined and irrigated to 85% field capacity before sowing (Sun et al., 2019). After sowing, irrigations were applied at two critical crop developmental stages: upstanding (Z30) and anthesis (Z61), which are determined by using the Zadoks scale (Zadoks et al., 1974). Three irrigation regimes were as follows: W0, no irrigation after sowing; W1, irrigation (75 mm) at upstanding; and W2 (75 mm × 2), irrigation at stages of upstanding and anthesis. Each treatment includes three replicates, with nine experimental plots in total. The plot size was 10 m × 5 m, allowing for growing 30 rows of winter wheat at a row spacing of 0.15 m, with 30,000 seeds sown per plot.
Samples Collection
The above-ground part of wheat plants from two 1 m inner rows was sampled every 5 days from flowering time (anthesis, Z31) until maturity. After field sampling, the plants were then separated into leaves, internodes (including sheaths), and chaff (spike without grain). After separated into nine parts, these samples were dried at 105°C for half an hour and then at 70°C until constant weight, and the DM was determined. As Figure 1A shows, leaf organs include TL1 (top first leaf or flag leaf laminae), TL2 (top second leaf), TL3 (top third leaf), and RLs (remaining leaves), and internode organs include TIN1 (top first internode or peduncle, including leaf sheath), TIN2 (top second internode), TIN3 (top third internode), RINs (remaining internodes), and chaff (including glume, palea, lemma, rachis, and awn) (Barraclough et al., 2014).
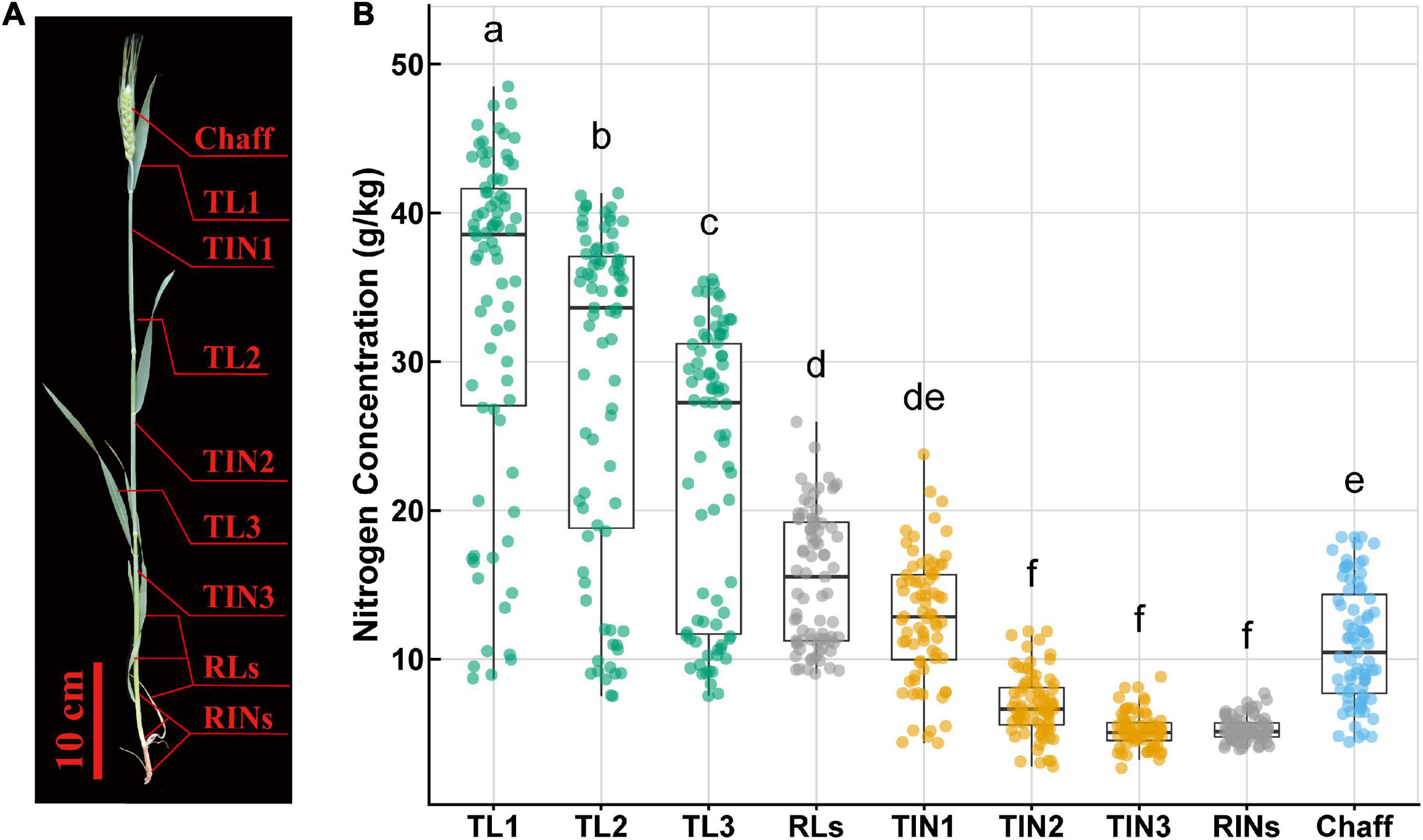
Figure 1. Schematic of wheat plant organs (A) and nitrogen concentration (Nmass) variation (B) within and among these organs. The different lowercase letters above the boxplot show significant different mean value (p < 0.05). TL1, top first leaf; TL2, top second leaf; TL3, top third leaf; RLs, remaining leaves; TIN1, top first internode; TIN2, top second internode; TIN3, top third internode; RINs, remaining internodes.
Measurements of N-Related Traits
The N concentration (Nmass), denoted as N mass per unit DM, was determined by using an automatic azotometer (Kjeltec 8400; Foss, Denmark) according to the Kjeldahl method (Horneck and Miller, 1998) following the manufacturer’s instructions. Post-anthesis NRE was calculated as the proportion of N in the crop component at anthesis which is not present in the vegetative parts at maturity (Gaju et al., 2014):
Where NRE is the N remobilization efficiency and NRA is the amount of N remobilized (kg N ha–1). NAAAnthesis is the amount of N (kg N ha–1) in vegetative organs at anthesis, and NAAMaturity is the amount of N reserved in the plant at maturity. In Eq. 1, Nmass represents N mass per unit DM (g N kg–1), and DM represents dry mass (kg⋅ha–1), which was determined by the dry weight of each sample.
The N concentration change (ΔNmass) between anthesis and maturity was calculated as follows:
Where NAnthesis and NMaturity represent Nmass of samples at anthesis and maturity, respectively.
The ΔNmass, N accumulation, and corresponding NRE of each organ were calculated separately. While the whole plant N accumulation is calculated as the sum of all separated organs’ accumulated N, the whole-plant NRE was then determined.
Spectral Reflectance Acquisition
A pipeline was developed to acquire spectral reflectance from a hyperspectral imager (Supplementary Figure 2). After collecting plants from the field, separated wheat organ samples were dried and grounded to fine powders. Then these powder samples (around 3–5 g, ∼1 cm depth for each) were placed on a plate and photographed in a hyperspectral image acquisition system (Pan et al., 2019). The images were collected by an SCO710-VP hyperspectral imager (SOC, San Diego, CA, United States) covering the VIS and NIR spectra between 375 and 1050 nm at 5 nm increments for a total of 128 bands. Raw images were calibrated by the gray reference panel with known reflectance. Spectral reflectance of each sample was acquired following the procedures described in our previous work (Hu et al., 2020), by using Spectral Radiance Analysis Software (Surface Optics Corporation, United States).
PLSR Predictive Model to Estimate Nmass From Spectra
Partial least squares regression (PLSR), which was proved to be an effective technique for building predictive models with spectral data (Serbin et al., 2012; Ely et al., 2019), was applied to develop Nmass spectra relationships. The predictive Nmass spectra model was built based on all the raw wavelength spectra for individual organs and across organs and cultivars. PLSR model was employed to predict Nmass from reflectance data with the “pls” package (Mevik et al., 2011) under the R software environment. During the procedure of model training and parameter fitting, 10 times repeated five-fold cross-validation method (Ali et al., 2017; Malmir et al., 2019) was conducted in the “caret” package (Kuhn, 2015; Heckmann et al., 2017). The optimal number of latent variables (also called model components) was determined based on the minimum predicted residual error sum of square (PRESS) statistic of the training model. Models were built on 75% of randomly selected experimental data for calibration and were used to predict the remaining (validation dataset of) 25%. A 10 times fivefold cross-validation was used to train the model. The accuracy of each model was evaluated based on the coefficients of determination (R2) and root mean square of error (RMSE) for predicted versus measured N in calibration and validation dataset. Bias was determined by the difference between the observed mean values and the predicted mean values for the validation dataset samples. Regression bias was calculated from the regression intercept. Variable importance of projection (VIP) values (Wold, 1995) of each PLSR model was evaluated to assess the relative contributions of different wavelengths over the full spectrum (Yendrek et al., 2017). VIP scores were used to identify the relative significant reflectance spectrum for each organ Nmass-spectra model. In addition, wavelengths of high VIP values (>0.8) were selected to recalibrate the Nmass-spectra model and compare to the all-wavelength model.
Statistical Analysis
The actual Nmass of different time points was pooled together for all statistical analysis. Tukey HSD test was performed to compare differences between multiple groups. Linear regression analysis was conducted to study the relationship between whole-plant NRE and organ NRE. The relationships between NRE (whole plant or separate organ) and ΔNmass were also investigated.
Results
N Concentration and Spectral Reflectance of the Nine Wheat Organs Powder
Chemical analysis in the laboratory showed that N concentration (Nmass) varied within and among different organs across all sampling time points (Figure 1B). Nmass ranged from 2.68 to 48.5 g/kg, showing a maximum difference of 45.82 g/kg (18-fold difference). As for different organs, the flag leaf or top first leaf (TL1) showed a larger range (8.72–48.5 g/kg) and a significantly higher Nmass than the other organs. Nmass was observed with significant difference between leaf organs, while within internodes only TIN1 was significantly higher than the other internodes. Leaves showed a higher average value and wider range than internodes and chaff. Besides, in leaves or internodes, Nmass showed a vertical distribution pattern, where the top ones had a higher average value and wider range than the basal ones.
The spectral reflectance varied substantially within and among these organs’ powder (Figure 2A and Supplementary Figure 3). Across all wavelengths, an obvious peak was observed in the VIS region, while a large continuous variation in reflectance from 780 to 950 nm was detected in the NIR. Among organs, the highest reflectance was found for the chaff and top second internodes (TIN2), respectively, in the VIS and NIR regions. In contrast, the lowest was found for the flag leaves (TL1) in the VIS, as well as for the RLs in the NIR. The RINs had higher reflectance in the red (620–650 nm) and red-edge regions than other organs. It is worth noting that, in the green (505–570 nm) region, the young or green organs showed an obvious peak, while the aging organs (RLs and RINs) did not (Supplementary Figure 3). In order to understand the diversity and general properties of all samples’ spectral reflectance, principal component analysis (PCA) was applied and results showed that the first three principal components explained 98% of the variance in this set of raw spectra. PCA plots from spectral reflectance showed that organs could be divided into two subgroups, leaf and non-leaf organs, as indicated by the vertical dashed line in the figure (Figure 2B). This is consistent with the spectra variation in the VIS region, where the four leaf organs had lower reflectance than the non-leaf organs.
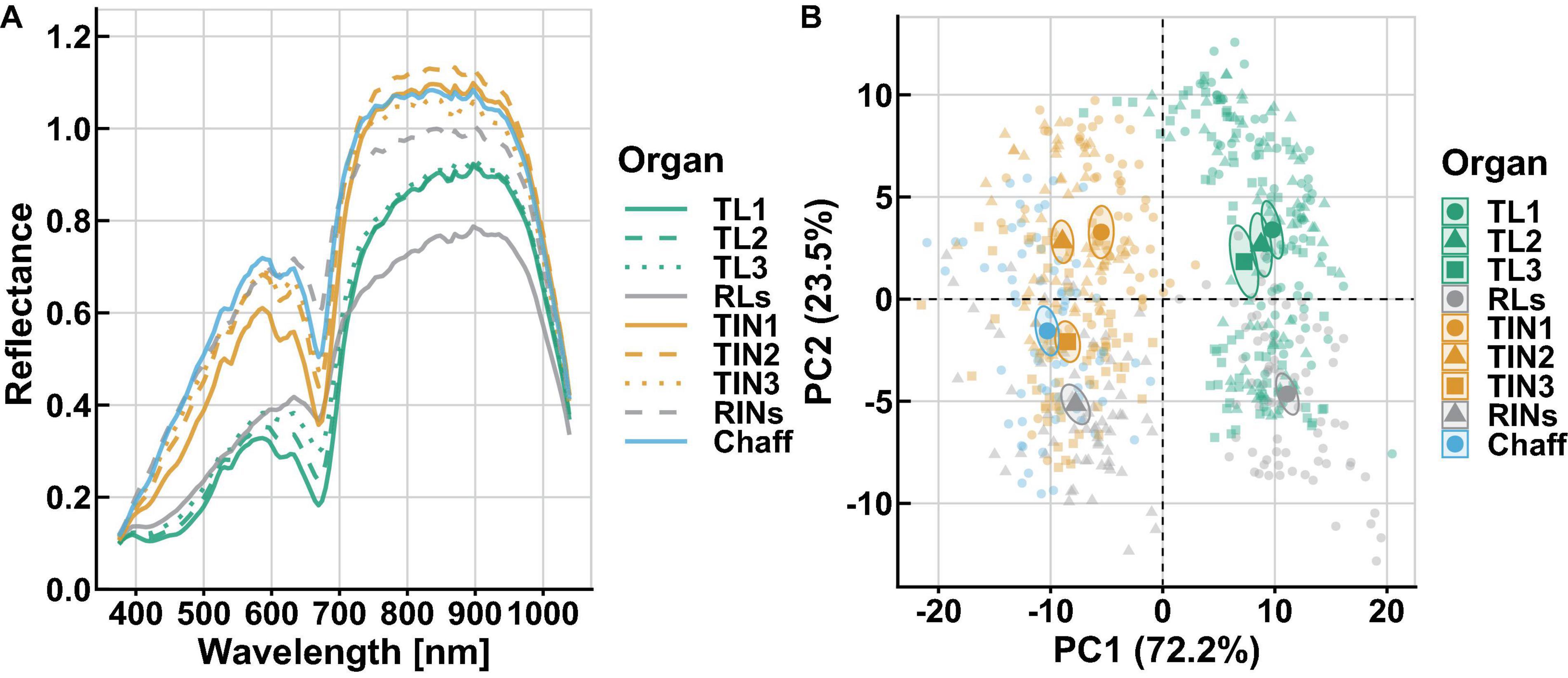
Figure 2. Spectra diversity within and among wheat organs. (A) Mean spectral reflectance for each organ is shown. (B) PCA plots from spectral reflectance categorized by organ group. In PCA plots, the first two PC which explained 95.7% variation, different colors and shapes correspond to each organ category, data points of individual are represented by transparent color points, and confidence ellipse and mean point of each group are shown by solid colors.
N Concentration Prediction From Spectral Reflectance at Organ Level
The Nmass prediction model was built with full range wavelength spectra for individual organs and across organs (mixed organs together). Except for the RINs, all organ-specific models were able to predict Nmass (Figure 3 and Table 1). For the calibration, R2 values ranged from 0.47 to 0.97 with the calibration RMSEs varying from 0.67 to 2.80 g/kg. Surprisingly, the validation accuracy was found to be comparable to the calibration with R2 values ranged from 0.26 to 0.95 g/kg. The best organ-specific models were found for TL1, TL2, TL3, chaff, and across organs (R2 > 0.88, Table 1). The moderate prediction models were found for RLs (R2 = 0.69, RMSE = 3.00 g/kg), TIN1 (R2 = 0.77, RMSE = 2.13 g/kg), TIN2 (R2 = 0.76, RMSE = 0.97 g/kg), and TIN3 (R2 = 0.69, RMSE = 0.71 g/kg) (Table 1). In contrast, the model failed to predict Nmass in RINs (R2 = 0.26, RMSE = 0.66 g/kg). When using the selected wavelengths to model the calibration, it did not perform better than all wavelengths model (Supplementary Table 4).
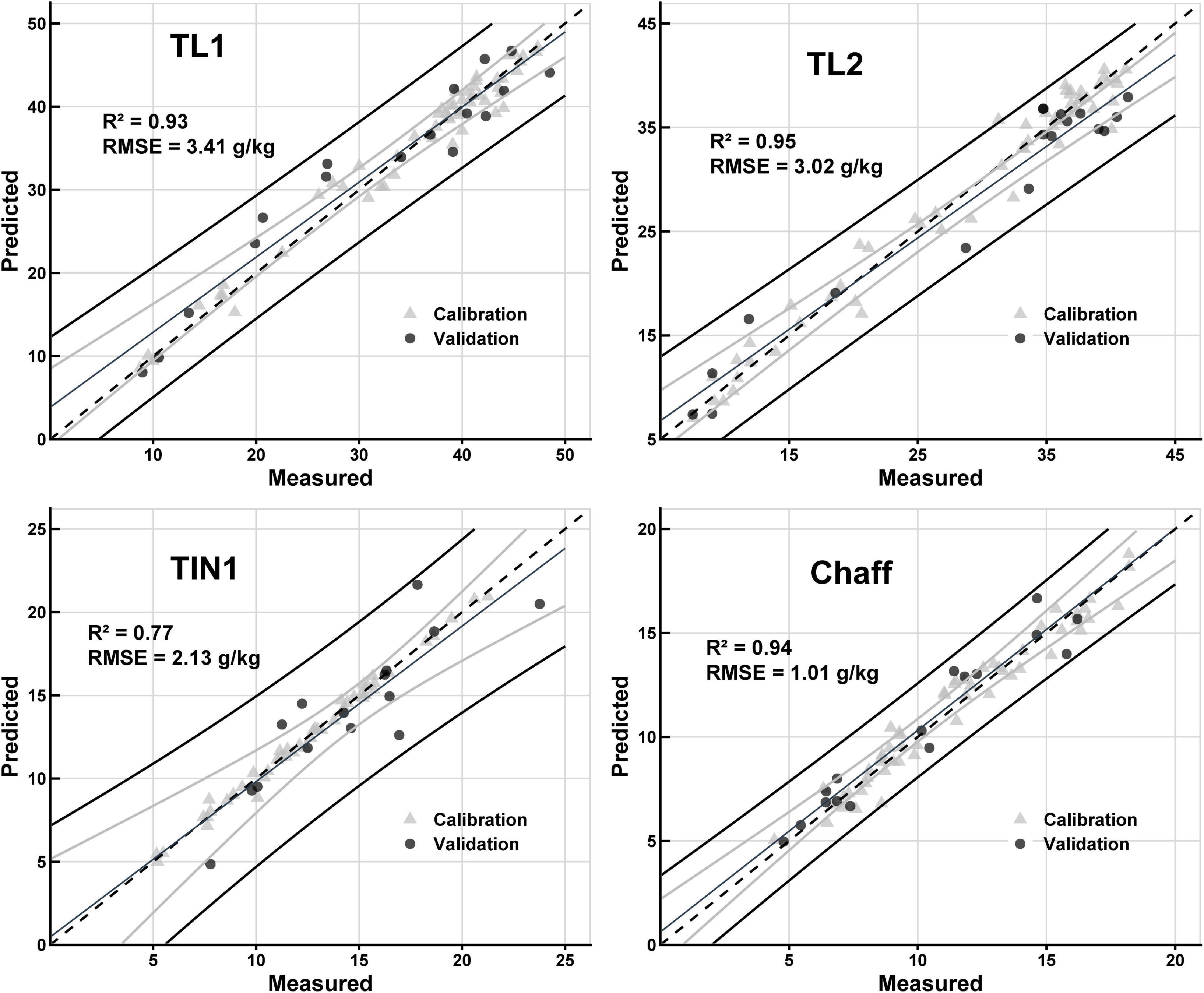
Figure 3. Results of PLSR predictive models for nitrogen concentration (Nmass). Comparison between measured Nmass and predicted Nmass for organs of TL1, TL2, TIN1, and Chaff. In the figures, calibration (gray triangle) and validation (solid circle) data points are illustrated. The dashed line shows 1:1 line, the fine black line shows regression line, the black lines show 95% prediction interval, and gray lines show 95% confidence interval of the validation dataset. Statistic parameters (R2 and RMSE) for the validation datasets are shown in the plots.
N Remobilization Varies Between Irrigation Regimes
During the anthesis and early grain developmental stages of winter wheat growing season, wheat experienced a non-precipitation period of 38 continuous days (Supplementary Figure 1b). Only a few rainfall days were recorded in the vegetative growth stages. The applied irrigation at these two critical growth stages resulted in a large difference between treatments. NRE of the two cultivars, JM22 and ND399, showed a small variation and shared a similar trend. Results showed that a low amount of irrigation (W0/W1) did not reduce NRE, compared to the higher irrigation (Figure 4A). NRA did not differ between W1 and W2, while they were much higher than W0, with a nearly doubled NRA value of W0 (Figure 4B). Figure 4C shows the contribution of nine organs to total NRA under three irrigation treatments. It is worth noting that NRA contribution from the main leaves (e.g., TL1 and TL2) decreased under W0 treatment when compared with W1 and W2 (Figure 4C). On the contrary, NRA contribution in RLs, RINs, and chaff showed increases under W0 treatment. In other organs, however, irrigation did not yield changes in NRA. Among these organs, the first two internodes, the first two leaves, and chaff are the major contribution organs, which contribute up to 80% to the total NRA (Figure 4C).
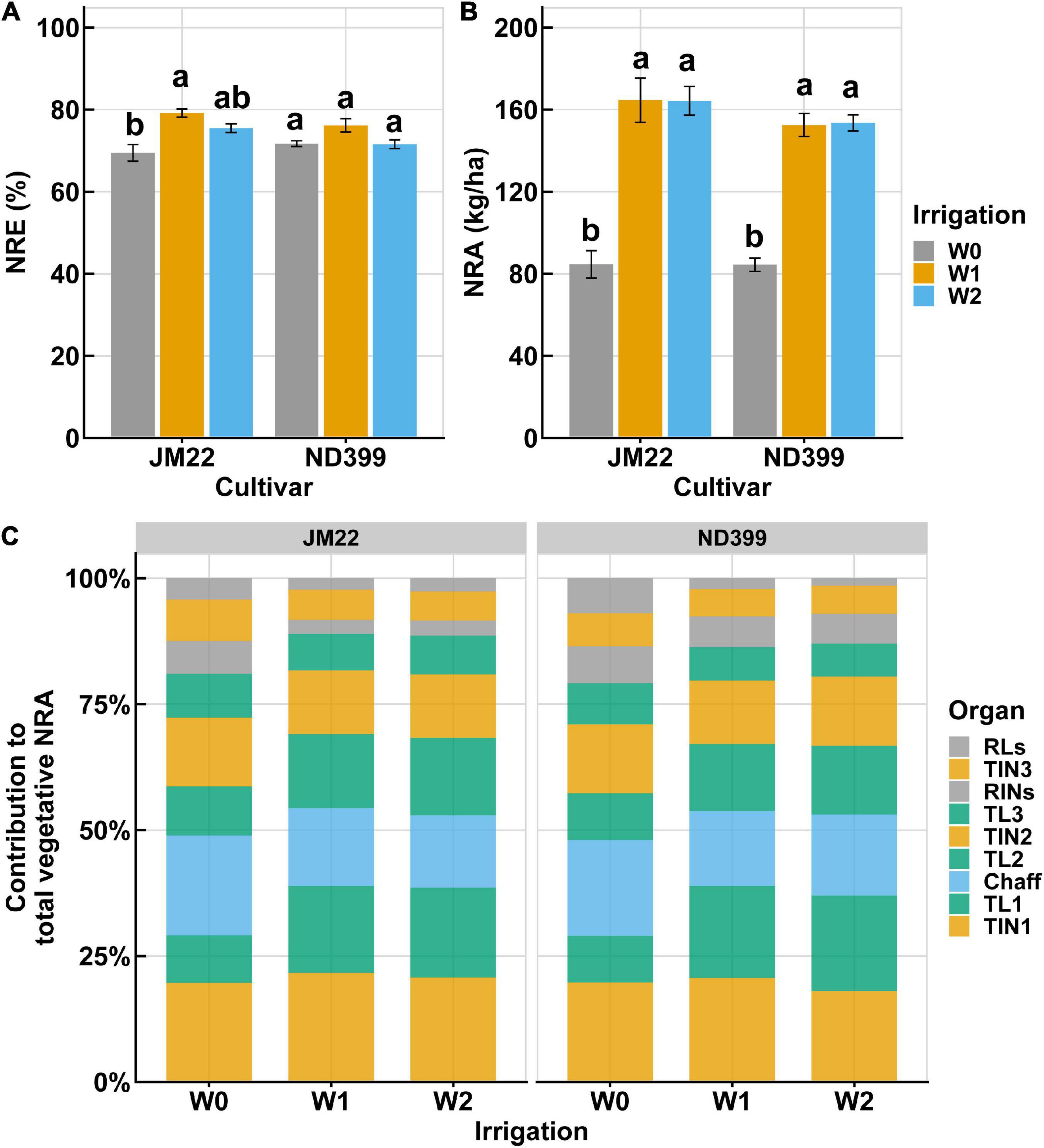
Figure 4. Nitrogen remobilization varies between three irrigation treatments. (A,B) Nitrogen remobilization efficiency (NRE) and nitrogen remobilization amount (NRA) of vegetative organs in winter wheat cultivar JM22 and ND399. Irrigation treatments are shown with three different colors. (C) Contribution of separated organs to total NRA.
Relationships Between N Remobilization-Related Traits
The organ-specific NRE was all significantly correlated with its corresponding post-anthesis ΔNmass, for all organs except chaff (Table 2). For organs of TL1, TL2, and TIN3, the organ-specific NRE shared high collinearity with ΔNmass, and the best correlation was observed for TIN3, with an R2 of 0.97. Furthermore, organ-specific NRE of TL1 and TIN1 could explain 70 and 93% variation of the whole-plant NRE at significant level (P < 0.05) (Figure 5), while organ-specific NRE of RLs and RINs could hardly explain any variation of whole-plant NRE. In addition, the observed ΔNmass of TIN1 could explain 78% variation of whole-plant NRE. Based on these results, it was decided to use organ-specific N concentration (e.g., TIN1) at anthesis and maturity to evaluate post-anthesis N remobilization.
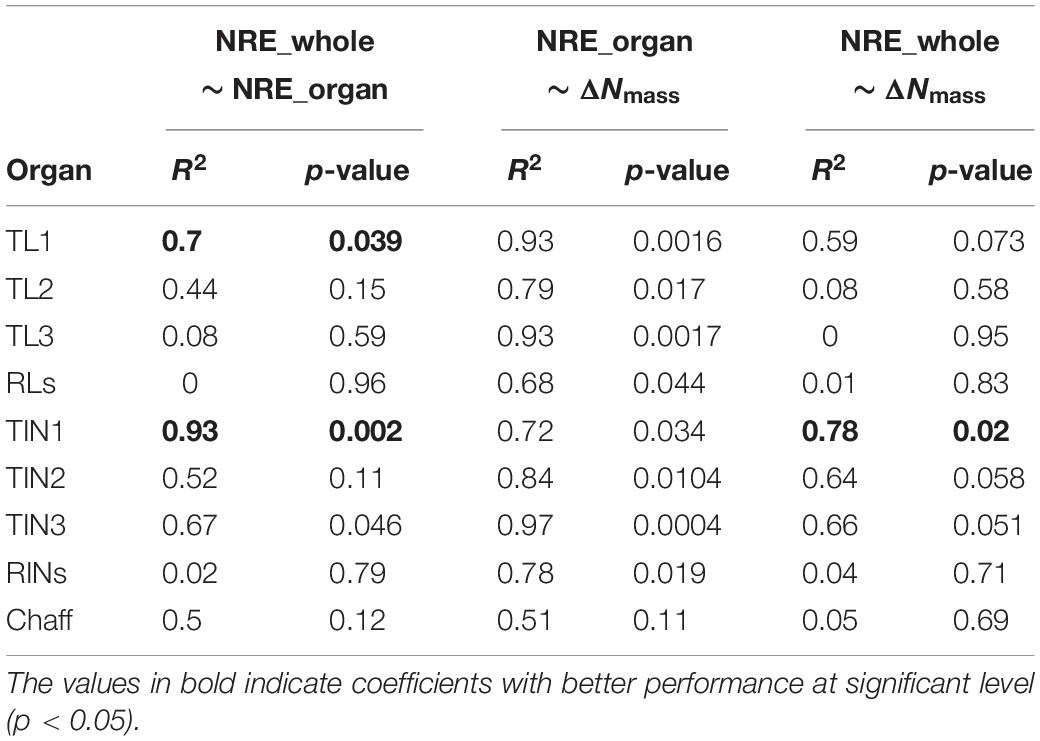
Table 2. The coefficients of determination (R2) between whole plant and organ NRE, between organ NRE and organ ΔNmass, and between whole-plant NRE and organ ΔNmass among wheat organs.
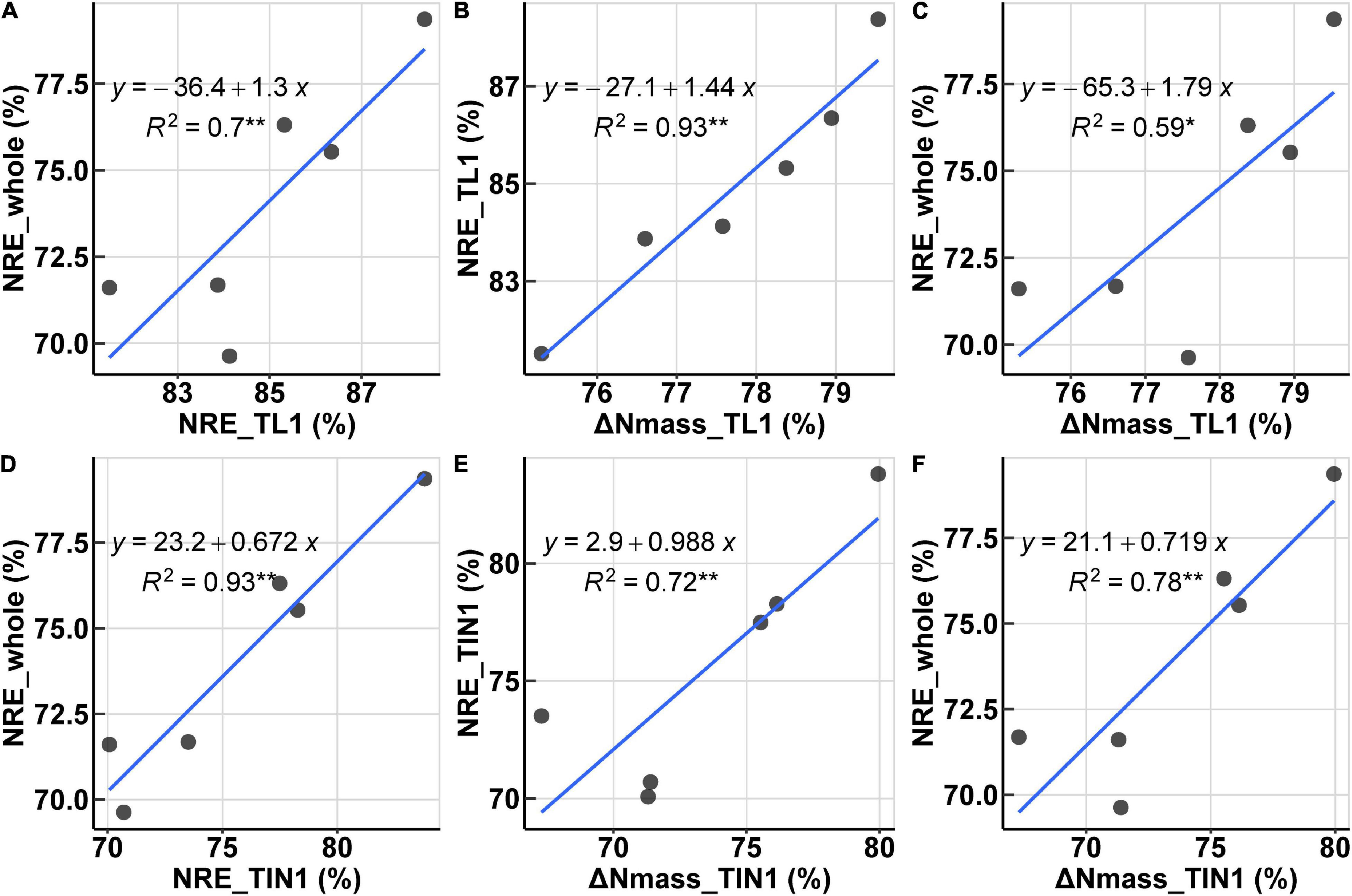
Figure 5. Relationships of N remobilization-related traits. (A,D) Relationships between NRE of separated organs and the whole plant. (B,E) Relationships between nitrogen concentration change (ΔNmass) and organ NRE. (C,F) Relationships between organ ΔNmass and whole-plant NRE. (A–C) are for TL1 and (D–F) are for TIN1.
Discussion
NRE Assessment Through Spectral Reflectance Estimated Nmass
Winter wheat production has been improved with high-input cropping systems over the past decades, so does the adaptive capacity of cultivars to less optimal water availability conditions (Voss-Fels et al., 2019). However, our understanding on the NRE adaptivity to drought is still limited due to the lack of efficient, repeatable approaches for evaluating the NRE. In this study, NRE variations under contrast irrigation regimes were evaluated by using hyperspectral-based N measures. Results revealed that under low precipitation, mild/moderate irrigation (W1) improved NRE a bit compared to the higher irrigation (W2), while whether W0 leads to a low NRE was dependent on the cultivar (Figure 4A). These results suggest that limited irrigation, which is beneficial to save water resources (Yang and Zhang, 2006; Li et al., 2019), is a cost-efficient approach for improving N use efficiency. On the other hand, grain yield was generally found to be decreased when mild irrigation was applied (Zaveri and Lobell, 2019), which is consist with our results that low yield was received from low irrigations (Supplementary Table 2). Balancing the grain yield and NRE improvement when applying water-saving management is always a trade-off. Although several studies reported that drying condition could benefit grain yield and nutrient use efficiency simultaneously (Wang et al., 2016; He G. et al., 2020), it is still challenging to determine the extent to which the irrigation should be optimized (Nguyen and Kant, 2018; Prey and Schmidhalter, 2019).
Our results not only confirmed the power of hyperspectral sensors in precisely estimating N concentration (Table 1) but also demonstrated the potential of assessing NRE variations through spectral reflectance (Table 2 and Figure 5). By predicting N concentration, N concentration change (ΔNmass) between the anthesis and post-anthesis (Barbottin et al., 2005; Kong et al., 2016) was able to be further estimated. The observed correlation between the actually measured NRE and ΔNmass (Table 2) suggested the possibility of evaluating N remobilization directly through spectral measurements. Our results have added to the growing evidence that plant spectroscopy could facilitate the study of N remobilization in response to irrigation (or fertilization) management that affects N remobilization, especially in terms of the timing and duration at organ level (Nehe et al., 2020). Notably, NRE measurement is a time-consuming and costly process which involves measurement of Nmass and dry matter simultaneously at the two critical stages (anthesis and maturity). Additionally, the whole-plant NRE could be estimated by acquiring spectral data of TIN1 powder samples at anthesis and maturity, suggesting a promising application in selecting N-efficient cultivars (Nguyen and Kant, 2018). For NRE correlations among organs, considering post NRE was determined by the contribution of organs. However, cultivars and environmental variations may have an influence on the relationships between NRE and ΔNmass, and also affect the use of specific organ data in assessing the whole-plant NRE. Further investigations are needed to improve the understanding of correlations between NRE and ΔNmass. Our study only provides a proof of concept for evaluating N remobilization under controlled light conditions following simple sample preparations (spectra acquired from organ powders). Nevertheless, it is still challenging to perform organ-specific HSI measurements in the field. Future improvement of the proposed method should be investigated by measuring individual plants and organs directly in the field.
Organ-Level Predictive Models
Predictive models calibrated across organs did not always succeed in predicting individual organs (data not shown). Similar results were also reported in models using leaf spectral reflectance in diverse species (Heckmann et al., 2017; Yendrek et al., 2017). This difference was validated by performing PCA for the spectral reflectance, in which leaf and non-leaf organs are statistically different as tested by a permutational multivariate analysis of variance (Anderson, 2017). These differences between leaf and non-leaf destructive organs might be attributed to their divergent biochemical composition. PCA results suggest that, even within the same type of organ (e.g., leaf and internode groups), large variations exist. Here, N concentrations of different vegetative organs were investigated and results suggest considerable variations distributed vertically among these organs (Figure 1B). These findings are similar to previous N partitioning research in wheat (Barraclough et al., 2014; Gaju et al., 2014). Collectively, our results confirmed the usefulness of considering organ-specific models.
In this study, similarly high predictive power (R2 ∼ 0.90) (Table 1) was achieved for leaf organs compared to previous Nmass estimations at leaf level (Serbin et al., 2012; Yendrek et al., 2017; Coast et al., 2019; Ely et al., 2019). Surprisingly, prediction for the chaff organ was comparable (R2 = 0.94) to the top leaf organs, which might be related to the specific role of chaff in maintaining a high N accumulation by acting as a temporary sink and source for N (Kong et al., 2016). Also, senescent leaves and internodes achieved relatively low predictive power (Table 1), which was similar to vertical canopy N predictions in rice (He J. et al., 2020). Small variations in spectra and N concentration may account for the low prediction power in these senescence organs. The relatively low N concentration might reduce the possibility of being detected by spectra, which is confirmed by a low coefficient of variation (CV) observed in the lower prediction models (Supplementary Table 5). In this situation, VIP values at some dominating wavelength were decreased (Supplementary Figure 4), resulting in a low contribution to the prediction (Yendrek et al., 2017). Regarding to the effect of organ on wavelength sore, one possible reason might be attributed from the biochemical composition between organs which more chlorophylls content is reserved in leaf or young organs dominating the reflection of N variations (Meacham-Hensold et al., 2020). For a robust PLSR model for predicting N in these organs having small variations, it might be possible by collecting a diverse range of Nmass data across more growth conditions and developmental stages or diverse genetic resources.
Hyperspectral Imaging as a Rapid and Cost-Effective Approach to Acquire Spectral Reflectance
This study used HSI to acquire reflectance data for estimating N concentration and assessing NRE variations of dried samples. As a spectroscopic technology, the biggest advantage is that the method is cost-efficient and environmentally friendly (Gredilla et al., 2016), and thus is suitable for repeated use. Due to its flexibility and convenience, spectral reflectance for a large number of samples was able to be acquired. For example, in our experiment, 18 samples were photographed (Supplementary Figure 2) in one image, making it possible for us to acquire more than 1000 samples within 10 h. To reduce the spatial complexity of analyzing the spectra while still taking the advantage of image data, regions of interest are typically selected to acquire average spectra (Malmir et al., 2019). Despite the benefits of HSI, this study only focused on the uses of organ powder samples and the average spectra of each sample, without discriminating spatial variations in each organ. The simplified approach for evaluating the whole-plant NRE through organ part (TIN1) spectra will facilitate balancing the irrigation and N management and screening high NRE cultivars. In our study, NRE of JM22 is superior to that in ND399 under sufficient water treatments (Supplementary Figure 5, W1 and W2). Hence, it is possible to screen high NRE cultivars under specific irrigation conditions. This study provides a proof of concept for evaluating N remobilization of wheat organs and whole plant through hyperspectral reflectance data. It is anticipated that HSI will be a promising tool for analyzing NRE spatial variation and its dynamics to uncover the underlying mechanism of N remobilization.
Conclusion
In this study, the N remobilization under three irrigation regimes was investigated using HSI. Results showed that NRE varied among irrigation regimes after sowing, where the mild irrigation (W1) achieved the best NRE, but the NRE reduced if no irrigation was applied. NRE in individual organs was correlated highly with the whole-plant NRE. Spectra-based models successfully predicted the N concentration in each organ. The N concentration change in a single organ (e.g., TIN1) between anthesis and maturity could explain 78% of the variation in the whole plant NRE. This study demonstrates the use of HSI in estimating N concentration and to aid the assessment of NRE variations from the organ to whole-plant levels, which holds great promise for guiding precision irrigation and N management for optimized NRE.
Data Availability Statement
The original contributions presented in the study are included in the article/Supplementary Material. Further inquiries can be directed to the corresponding author/s.
Author Contributions
WL, XZ, ZW, and YZ conceived and designed the experiments. WL, XZ, ZZ, YaL, and NH performed the experiments. WL, XZ, YiL, CY, and XY analyzed the data. WL, XZ, KY, and YZ wrote the manuscript. All authors read and approved the final manuscript.
Funding
This work was supported by the National Key Research and Development Program of China (Grant Nos. 2016YFD0300401, 2016YFD0300105, and 2017YFD0201705), the National Natural Science Foundation of China (31871563), the Modern Agro-Industry Technology Research System (CARS-3), and the CAU Basic Scientific Research Fund (2020RC037).
Conflict of Interest
The authors declare that the research was conducted in the absence of any commercial or financial relationships that could be construed as a potential conflict of interest.
Acknowledgments
We appreciate the generous help from Li Yang and Tianliang Zhang for providing the hyperspectral imager and training to us. We thank the staff of the Wuqiao Experimental Station of CAU for their help in field management.
Supplementary Material
The Supplementary Material for this article can be found online at: https://www.frontiersin.org/articles/10.3389/fpls.2021.657578/full#supplementary-material
References
Ali, M. M., Al-Ani, A., Eamus, D., and Tan, D. K. Y. (2017). Leaf nitrogen determination using non-destructive techniques–a review. J. Plant Nutr. 40, 928–953. doi: 10.1080/01904167.2016.1143954
Anderson, M. J. (2017). “Permutational multivariate analysis of variance (PERMANOVA),” in Wiley StatsRef: Statistics Reference Online, eds N. Balakrishnan, T. Colton, B. Everitt, W. Piegorsch, F. Ruggeri, and J. L. Teugels (Hoboken, NJ: John Wiley & Sons), 1–15.
Asner, G., and Martin, R. (2008). Spectral and chemical analysis of tropical forests: scaling from leaf to canopy levels. Remote Sens. Environ. 112, 3958–3970. doi: 10.1016/j.rse.2008.07.003
Bahrani, A., Abad, H. H. S., and Aynehband, A. (2011). Nitrogen remobilization in wheat as influenced by nitrogen application and post-anthesis water deficit during grain filling. [Article]. Afr. J. Biotechnol. 10, 10585–10594. doi: 10.5897/ajb11.013
Barbottin, A., Lecomte, C., Bouchard, C., and Jeuffroy, M.-H. (2005). Nitrogen remobilization during grain filling in wheat: genotypic and environmental effects. Crop Sci. 45, 1141–1150. doi: 10.2135/cropsci2003.0361
Barraclough, P. B., Lopez-Bellido, R., and Hawkesford, M. J. (2014). Genotypic variation in the uptake, partitioning and remobilisation of nitrogen during grain-filling in wheat. Field Crops Res. 156, 242–248. doi: 10.1016/j.fcr.2013.10.004
Bertheloot, J., Martre, P., and Andrieu, B. (2008). Dynamics of light and nitrogen distribution during grain filling within wheat canopy. Plant Physiol. 148, 1707–1720. doi: 10.1104/pp.108.124156
Bidinger, F., Musgrave, R., and Fischer, R. (1977). Contribution of stored pre-anthesis assimilate to grain yield in wheat and barley. Nature 270, 431–433.
Caporaso, N., Whitworth, M. B., and Fisk, I. D. (2018a). Near-infrared spectroscopy and hyperspectral imaging for non-destructive quality assessment of cereal grains. Appl. Spectr. Rev. 53, 667–687. doi: 10.1080/05704928.2018.1425214
Caporaso, N., Whitworth, M. B., and Fisk, I. D. (2018b). Protein content prediction in single wheat kernels using hyperspectral imaging. Food Chem. 240, 32–42. doi: 10.1016/j.foodchem.2017.07.048
Coast, O., Shah, S., Ivakov, A., Gaju, O., Wilson, P. B., Posch, B. C., et al. (2019). Predicting dark respiration rates of wheat leaves from hyperspectral reflectance. Plant Cell Environ. 42, 2133–2150. doi: 10.1111/pce.13544
Ely, K. S., Burnett, A. C., Lieberman-Cribbin, W., Serbin, S. P., and Rogers, A. (2019). Spectroscopy can predict key leaf traits associated with source-sink balance and carbon-nitrogen status. J. Exp. Bot. 70, 1789–1799. doi: 10.1093/jxb/erz061
FAO (2019). Crop Prospects and Food Situation [Online]. Available online at: http://www.fao.org/giews/reports/crop-prospects/en/
Fu, P., Meacham-Hensold, K., Guan, K., Wu, J., and Bernacchi, C. (2020). Estimating photosynthetic traits from reflectance spectra: a synthesis of spectral indices, numerical inversion, and partial least square regression. Plant Cell Environ. 43, 1241–1258. doi: 10.1111/pce.13718
Gaju, O., Allard, V., Martre, P., Le Gouis, J., Moreau, D., Bogard, M., et al. (2014). Nitrogen partitioning and remobilization in relation to leaf senescence, grain yield and grain nitrogen concentration in wheat cultivars. Field Crops Res. 155, 213–223. doi: 10.1016/j.fcr.2013.09.003
Galvez-Sola, L., Garcia-Sanchez, F., Perez-Perez, J. G., Gimeno, V., Navarro, J. M., Moral, R., et al. (2015). Rapid estimation of nutritional elements on citrus leaves by near infrared reflectance spectroscopy. Front. Plant Sci. 6:571. doi: 10.3389/fpls.2015.00571
Gao, L., and Smith, R. T. (2015). Optical hyperspectral imaging in microscopy and spectroscopy - a review of data acquisition. J. Biophotonics 8, 441–456. doi: 10.1002/jbio.201400051
Gredilla, A., Fdez-Ortiz de Vallejuelo, S., Elejoste, N., de Diego, A., and Madariaga, J. M. (2016). Non-destructive spectroscopy combined with chemometrics as a tool for green chemical analysis of environmental samples: a review. TRAC Trends Analyt. Chem. 76, 30–39. doi: 10.1016/j.trac.2015.11.011
Hasituya, Li, F., Elsayed, S., Hu, Y., and Schmidhalter, U. (2020). Passive reflectance sensing using optimized two- and three-band spectral indices for quantifying the total nitrogen yield of maize. Comput. Electron. Agric. 173:105403. doi: 10.1016/j.compag.2020.105403
Have, M., Marmagne, A., Chardon, F., and Masclaux-Daubresse, C. (2017). Nitrogen remobilization during leaf senescence: lessons from Arabidopsis to crops. J. Exp. Bot. 68, 2513–2529. doi: 10.1093/jxb/erw365
Hawkesford, M. J. (2017). Genetic variation in traits for nitrogen use efficiency in wheat. J. Exp. Bot. 68, 2627–2632. doi: 10.1093/jxb/erx079
He, G., Wang, Z., and Cui, Z. (2020). Managing irrigation water for sustainable rice production in China. J. Clean. Prod. 245:118928. doi: 10.1016/j.jclepro.2019.118928
He, J., Zhang, X., Guo, W., Pan, Y., Yao, X., Cheng, T., et al. (2020). Estimation of vertical leaf nitrogen distribution within a rice canopy based on hyperspectral data. [Original Research]. Front. Plant Sci. 10:1802. doi: 10.3389/fpls.2019.01802
Heckmann, D., Schluter, U., and Weber, A. P. M. (2017). Machine learning techniques for predicting crop photosynthetic capacity from leaf reflectance spectra. Mol. Plant 10, 878–890. doi: 10.1016/j.molp.2017.04.009
Horneck, D. A., and Miller, R. O. (1998). “Determination of total nitrogen in plant tissue,” in Handbook of Reference Methods for Plant Analysis, Vol. 2, ed. Y. Kalra (Boca Raton, FL: CRC Press), 75–83.
Hu, N., Li, W., Du, C., Zhang, Z., Gao, Y., Sun, Z., et al. (2020). Predicting micronutrients of wheat using hyperspectral imaging. Food Chem. 343:128473. doi: 10.1016/j.foodchem.2020.128473
Kichey, T., Hirel, B., Heumez, E., Dubois, F., and Le Gouis, J. (2007). In winter wheat (Triticum aestivum L.), post-anthesis nitrogen uptake and remobilisation to the grain correlates with agronomic traits and nitrogen physiological markers. Field Crops Res. 102, 22–32. doi: 10.1016/j.fcr.2007.01.002
Kong, L., Xie, Y., Hu, L., Feng, B., and Li, S. (2016). Remobilization of vegetative nitrogen to developing grain in wheat (Triticum aestivum L.). Field Crops Res. 196, 134–144. doi: 10.1016/j.fcr.2016.06.015
Li, F., Miao, Y., Hennig, S. D., Gnyp, M. L., Chen, X., Jia, L., et al. (2010). Evaluating hyperspectral vegetation indices for estimating nitrogen concentration of winter wheat at different growth stages. Precis. Agric. 11, 335–357.
Li, J., Wang, Y., Zhang, M., Liu, Y., Xu, X., Lin, G., et al. (2019). Optimized micro-sprinkling irrigation scheduling improves grain yield by increasing the uptake and utilization of water and nitrogen during grain filling in winter wheat. Agric. Water Manag. 211, 59–69. doi: 10.1016/j.agwat.2018.09.047
Malmir, M., Tahmasbian, I., Xu, Z., Farrar, M. B., and Bai, S. H. (2019). Prediction of macronutrients in plant leaves using chemometric analysis and wavelength selection. J. Soils Sediments 20, 249–259. doi: 10.1007/s11368-019-02418-z
Meacham-Hensold, K., Fu, P., Wu, J., Serbin, S., Montes, C. M., Ainsworth, E., et al. (2020). Plot-level rapid screening for photosynthetic parameters using proximal hyperspectral imaging. J. Exp. Bot. 71, 2312–2328. doi: 10.1093/jxb/eraa068
Mevik, B.-H., Wehrens, R., and Liland, K. H. (2011). Pls: Partial Least Squares and Principal Component Regression. R package version, 2.
Nehe, A. S., Misra, S., Murchie, E. H., Chinnathambi, K., Singh Tyagi, B., and Foulkes, M. J. (2020). Nitrogen partitioning and remobilization in relation to leaf senescence, grain yield and protein concentration in Indian wheat cultivars. Field Crops Res. 251:107778. doi: 10.1016/j.fcr.2020.107778
Nguyen, G. N., and Kant, S. (2018). Improving nitrogen use efficiency in plants: effective phenotyping in conjunction with agronomic and genetic approaches. Funct. Plant Biol. 45, 606–619. doi: 10.1071/FP17266
Nguyen, G. N., Maharjan, P., Maphosa, L., Vakani, J., Thoday-Kennedy, E., and Kant, S. (2019). A robust automated image-based phenotyping method for rapid vegetative screening of wheat germplasm for nitrogen use efficiency. [Original Research]. Front. Plant Sci. 10:1372. doi: 10.3389/fpls.2019.01372
Pan, X., Sun, L., Li, Y., Che, W., Ji, Y., Li, J., et al. (2019). Non-destructive classification of apple bruising time based on visible and near-infrared hyperspectral imaging. J. Sci. Food Agric. 99, 1709–1718. doi: 10.1002/jsfa.9360
Pask, A. J. D., Sylvester-Bradley, R., Jamieson, P. D., and Foulkes, M. J. (2012). Quantifying how winter wheat crops accumulate and use nitrogen reserves during growth. Field Crops Res. 126, 104–118. doi: 10.1016/j.fcr.2011.09.021
Prey, L., and Schmidhalter, U. (2019). Temporal and spectral optimization of vegetation indices for estimating grain nitrogen uptake and late-seasonal nitrogen traits in wheat. Sensors 19:4640. doi: 10.3390/s19214640
Serbin, S. P., Dillaway, D. N., Kruger, E. L., and Townsend, P. A. (2012). Leaf optical properties reflect variation in photosynthetic metabolism and its sensitivity to temperature. J. Exp. Bot. 63, 489–502. doi: 10.1093/jxb/err294
Simonne, E. H., Mills, H. A., Jones, J. B., Smittle, D. A., and Hussey, C. G. (1994). A comparison of analytical methods for nitrogen analysis in plant tissues. Commun. Soil Sci. Plant Anal. 25, 943–954.
Sun, H.-Y., Liu, C.-M., Zhang, X.-Y., Shen, Y.-J., and Zhang, Y.-Q. (2006). Effects of irrigation on water balance, yield and WUE of winter wheat in the North China Plain. Agric. Water Manag. 85, 211–218.
Sun, Z., Zhang, Y., Zhang, Z., Gao, Y., Yang, Y., Han, M., et al. (2019). Significance of disposable presowing irrigation in wheat in increasing water use efficiency and maintaining high yield under winter wheat-summer maize rotation in the North China Plain. Agric. Water Manag. 225:105766. doi: 10.1016/j.agwat.2019.105766
Vigneau, N., Ecarnot, M., Rabatel, G., and Roumet, P. (2011). Potential of field hyperspectral imaging as a non destructive method to assess leaf nitrogen content in Wheat. Field Crops Res. 122, 25–31. doi: 10.1016/j.fcr.2011.02.003
Vilmus, I., Ecarnot, M., Verzelen, N., and Roumet, P. (2014). Monitoring nitrogen leaf resorption kinetics by near-infrared spectroscopy during grain filling in durum wheat in different nitrogen availability conditions. Crop Sci. 54, 284–296. doi: 10.2135/cropsci2013.02.0099
Voss-Fels, K. P., Stahl, A., Wittkop, B., Lichthardt, C., Nagler, S., Rose, T., et al. (2019). Breeding improves wheat productivity under contrasting agrochemical input levels. Nat. Plants 5, 706–714. doi: 10.1038/s41477-019-0445-5
Wang, Z., Zhang, W., Beebout, S. S., Zhang, H., Liu, L., Yang, J., et al. (2016). Grain yield, water and nitrogen use efficiencies of rice as influenced by irrigation regimes and their interaction with nitrogen rates. Field Crops Res. 193, 54–69. doi: 10.1016/j.fcr.2016.03.006
White, A. C., Rogers, A., Rees, M., and Osborne, C. P. (2016). How can we make plants grow faster? A source-sink perspective on growth rate. J. Exp. Bot. 67, 31–45. doi: 10.1093/jxb/erv447
Wold, S. (1995). PLS for multivariate linear modeling. Chemometric methods in molecular design 195–218.
Xu, Z.-Z., Yu, Z.-W., and Wang, D. (2006). Nitrogen translocation in wheat plants under soil water deficit. Plant Soil 280, 291–303. doi: 10.1007/s11104-005-3276-2
Yang, J., and Zhang, J. (2006). Grain filling of cereals under soil drying. New phytol. 169, 223–236.
Yendrek, C. R., Tomaz, T., Montes, C. M., Cao, Y., Morse, A. M., Brown, P. J., et al. (2017). High-throughput phenotyping of maize leaf physiological and biochemical traits using hyperspectral reflectance. Plant Physiol. 173, 614–626. doi: 10.1104/pp.16.01447
Yu, K., Li, F., Gnyp, M. L., Miao, Y., Bareth, G., and Chen, X. (2013). Remotely detecting canopy nitrogen concentration and uptake of paddy rice in the Northeast China Plain. ISPRS J. Photogramm. Remote Sens. 78, 102–115. doi: 10.1016/j.isprsjprs.2013.01.008
Zadoks, J. C., Chang, T. T., and Konzak, C. F. (1974). A decimal code for the growth stages of cereals. Weed Res. 14, 415–421.
Keywords: hyperspectral, N concentration, PLSR, plant organ reflectance, winter wheat, N remobilization efficiency
Citation: Li W, Zhou X, Yu K, Zhang Z, Liu Y, Hu N, Liu Y, Yao C, Yang X, Wang Z and Zhang Y (2021) Spectroscopic Estimation of N Concentration in Wheat Organs for Assessing N Remobilization Under Different Irrigation Regimes. Front. Plant Sci. 12:657578. doi: 10.3389/fpls.2021.657578
Received: 23 January 2021; Accepted: 15 March 2021;
Published: 09 April 2021.
Edited by:
Shawn Carlisle Kefauver, University of Barcelona, SpainfReviewed by:
Salah Elsayed Mohamed Elsayed, University of Sadat City, EgyptAnne Marmagne, Institut National de Recherche pour l’Agriculture, l’Alimentation et l’Environnement (INRAE), France
Omar Vergara-Diaz, University of Barcelona, Spain
Copyright © 2021 Li, Zhou, Yu, Zhang, Liu, Hu, Liu, Yao, Yang, Wang and Zhang. This is an open-access article distributed under the terms of the Creative Commons Attribution License (CC BY). The use, distribution or reproduction in other forums is permitted, provided the original author(s) and the copyright owner(s) are credited and that the original publication in this journal is cited, in accordance with accepted academic practice. No use, distribution or reproduction is permitted which does not comply with these terms.
*Correspondence: Kang Yu, kangyu@cau.edu.cn; Zhimin Wang, zmwangcau@126.com; Yinghua Zhang, yhzhang@cau.edu.cn
†These authors have contributed equally to this work
‡Present address: Kang Yu, School of Life Sciences, Technical University of Munich, Freising, Germany