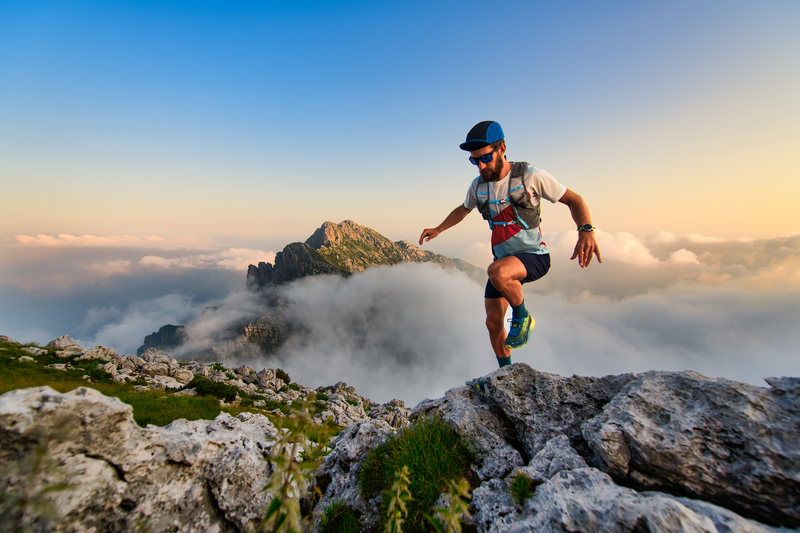
94% of researchers rate our articles as excellent or good
Learn more about the work of our research integrity team to safeguard the quality of each article we publish.
Find out more
OPINION article
Front. Plant Sci. , 16 April 2021
Sec. Plant Breeding
Volume 12 - 2021 | https://doi.org/10.3389/fpls.2021.651480
This article is part of the Research Topic Genomic Selection: Lessons Learned and Perspectives View all 22 articles
Continued increases in genetic gain demonstrate the success of established public and private plant breeding programs. Nevertheless, in the last two decades, a growing body of modern technologies has been developed and now awaits efficient integration into traditional breeding pipelines. This integration offers attractive benefits, yet comes with the challenges of making modifications in established and operational systems, a recent example of which is rice breeding (Collard et al., 2019). Newly available technologies, genomics rapid cycling (Crossa et al., 2017), high throughput phenotyping (HTP, phenomics) (Montesinos-López et al., 2017) and historical descriptions of environmental relatedness (enviromics) (Costa-Neto et al., 2020a,b; Resende et al., 2020; Rogers et al., 2021) are crucial to improving conventional breeding schemes and increasing genetic gain. Integrating these new technologies into routine breeding pipelines will support the delivery of cultivars with robust yields in the face of the expected unfavorable future environmental conditions caused by climate change and the consequently increased occurrence of biotic and abiotic stresses. Here, we briefly describe the use of these technologies and their implementation to provide cost-effective and time-saving approaches to plant breeding. We also give an overview of the interconnections between these techniques. Finally, we envision future perspectives to implement a more interconnected breeding approach that takes advantage of the so-called modern plant breeding triangle: integrating genomics, phenomics, and enviromics.
One of the most popular uses of genomics in breeding is the prediction of breeding values. Genomic selection (GS) reduces cycle time, increases the accuracy of estimated breeding values and improves selection accuracy. For instance, in maize, the effectiveness of GS has been proven for the case of bi-parental populations (Massman et al., 2013; Beyene et al., 2015; Vivek et al., 2017), as well as in multi-parental populations (Zhang et al., 2017). Its use has also been documented in species with long generation times such as trees (Grattapaglia et al., 2018) and dairy cattle breeding, where the reduction of the breeding cycle has increased the response to selection in comparison with the progeny testing system (García-Ruiz et al., 2016).
Genomic selection has been implemented in many crops, including wheat, chickpea, cassava and rice (Roorkiwal et al., 2016; Crossa et al., 2017; Wolfe et al., 2017; Huang et al., 2019), and the number of programs that are moving from “conventional” to GS is growing. Results in wheat show that genomic predictions used early in the breeding cycle led to a substantial increase in performance in later generations (Bonnett et al., 2021 this issue).
In genomic selection, the optimization of the training set composition is an important topic because training and testing sets should be genetically related in such a way that the genetic diversity present in the testing set could be covered and captured by the diversity in the training set. Breeding programs must start forming initial foundational core parents (training populations) that represent the genetic diversity found in the current progeny and conform to the testing population(s) to the greatest extent possible (Hickey et al., 2012). These foundational parents should be extensively phenotyped in different target populations of environments and genotyped with high-density marker systems. These training sets of foundation parents will be able to produce a model with a high accuracy for current highly selected progenies (Zhang et al., 2017).
The most important limitation to determining accurate phenotypes has been the time and cost required to measure traits in the field. Field phenomics aims to study all plant phenotypes under a range of environmental conditions. Modern phenomics methods are able to use hyperspectral/multispectral cameras to provide hundreds of reflectance data points at discrete narrow bands in many environments and at many stages of crop development. Phenotyping technology can now be used to quickly and accurately obtain data on agronomic traits based on advancements in plant phenotyping technologies (Atkinson et al., 2018). Therefore, the main goal of a high-throughput phenotype (HTP) is to reduce the cost of data per plot and to increase the prediction accuracy early in the crop-growing season with the use of highly heritable secondary phenotypes, closely related to the selection phenotypes. The cost of processing HTP data can be minimized by using open-source software, such as FieldImageR (Matias et al., 2020).
There is evidence that multi-trait analyses improve prediction accuracies when the genetic and residual correlations are considered in the modeling process. New genomic models that take the multiple traits and the multiple environments into consideration, along with trait × environment, trait × genotype, and trait × genotype × environment interactions, offer a huge potential for the exploitation of correlations between different variables and for the differentiation between effects. Integrating current GBLUP multi-trait models with models that consider the environmental information with the two- and three-way interaction terms provides a powerful, unified, whole genome prediction model.
The Bayesian multi-trait and multi-environment model (BMTME) (Montesinos-López et al., 2016, 2019a) allows for general covariance matrices for traits and environments that capture the correlations among traits and environments better. This unified model could be implemented to select genotypes with traits measured in one environment and to predict in other, untested environments. It could also be applied to predict traits that are costly or difficult to measure in all environments.
It is crucial to obtain large and inter-operable phenomics datasets from field phenotyping. This should be used to characterize the foundational core parents in the different environments and incorporate them into the visual data collected in the different environments. These data, along with pedigree and genomic information, can be used to fit Bayesian linear mixed models to compute BLUPs of the genetic values of the material in the training set. Breeding programs should collect multi-trait data on the multi-environment used for foundational core parents and exploit possible correlations among traits that will eventually increase prediction accuracy. The genomics and phenomics of the multi-trait foundation core parents are essential for use alongside enviromics data.
The phenotypic variation observed across diverse environments is a product of genetic and environmental variation. Thus, enviromics acts as a central bottleneck for the application of modern genomics-assisted prediction tools, especially for use across multiple environments. Novel approaches have integrated field trial data with DNA sequences using different sources of enviromics, such as linear and nonlinear reaction-norm models (e.g., Jarquín et al., 2014; Morais-Júnior et al., 2018; Millet et al., 2019; Monteverde et al., 2019; Costa-Neto et al., 2020a), crop growth model (CGM) outputs (Heslot et al., 2014; Rincent et al., 2017, 2019), CGM integrated with GS (Cooper et al., 2016; Messina et al., 2018; Robert et al., 2020) and historical weather records to predict cultivars in years to come (de los Campos et al., 2020).
For example, the strategy proposed by de los Campos et al. (2020) assesses genomic × environment (G × E) patterns learned from field trials and predicts the expected performance of a cultivar in an environment but also evaluates the expected distribution of a cultivar performance over other possible weather conditions, while accounting for uncertainty in model parameters. This is a new method for the analysis of multi-environment trials and can speed up the assessment of grain yield adaptability and stability.
Another recent example is the approach that can increase the resolution in multi-environment prediction for stability by taking advantage of large-scale enviromics with different kernel methods (Costa-Neto et al., 2020a). The environmental relatedness among field trials can be shaped using linear covariances (as proposed by Jarquín et al., 2014) and non-linear methods (Gaussian kernel, deep learning, and deep kernel) (Cuevas et al., 2016, 2017, 2018, 2019; Montesinos-López et al., 2018a,b, 2019b,c). The use of non-linear kernels has led to higher accuracy gains in the prediction of novel genotypes under known conditions, but mostly in the prediction of novel environment conditions (untested environments). This approach was expanded to take account of several environmental structures across different crop development stages (Costa-Neto et al., 2020b). For the latter, the authors observed an increased ability to explain G × E in terms of genotype-specific reaction norms for key environmental factors or key development stages. This increased ability to explain G × E was important to achieve higher accuracy gains in comparison with models without enviromic information.
In a recent research article, Rogers et al. (2021) emphasized the importance of incorporating high throughput environmental data into genomic prediction models in order to carry out predictions in new environments characterized with the same environmental characteristics. The author concluded that, among other factors, G × E interactions and environmental covariates should be incorporated into prediction models to improve prediction accuracy.
Progress toward the modernization of the statistical and quantitative genetic models for the analysis of plant breeding in multi-environment trials has become clearer as the availability of genomics, phenomics, and environments information has increased (see, among others, Vargas et al., 1998; Crossa et al., 2010; Burgueño et al., 2012; Heslot et al., 2014; Jarquín et al., 2014; Montesinos-López et al., 2017; Millet et al., 2019; Costa-Neto et al., 2020a; de los Campos et al., 2020; Robert et al., 2020). Thus, we see that all the elements described above offer a clear potential for the acceleration of genetic gains in plant breeding. However, an efficient data-based integration is required to achieve greater opportunity, particularly in terms of increasing prediction accuracy. Some of the major links between genomics, phenomics, and enviromics are outlined below, and their potential impacts are summarized in Figure 1.
Figure 1. The modern plant-breeding triangle incorporates genomics, phenomics, and enviromics. Connections between each of these elements can be beneficial for the acceleration of genetic gains.
Linking massive data sets from genomics and phenomics has complexities that require statistical models to deal with a very large number of correlated predictors. Montesinos-López et al. (2017) proposed linking genomics and phenomics with Bayesian functional regression models that consider all available reflectance bands (250 bands or wavelength), genomic or pedigree information, the main effects of lines and environments, as well as the effects of interaction. They observed that the models with wavelength × environment interaction terms were the most accurate for the prediction of performance in three different environments and at various crop development time points. The functional regression models are parsimonious and computationally efficient because the mathematical basis functions allow the selection of only 21 beta coefficients (rather than using all 250). Recently, Lopez-Cruz et al. (2020) proposed a method to predict the genetic merit of cultivars from high-dimensional HTP data by integrating high-dimensional regressions into the standard selection index methodology.
Multi-trait multi-environment data (MTME) take advantage of large-scale correlations among different traits evaluated across diverse environments to train accurate GS models. Because of this, the use of GS in MTME data is a promising approach to reduce field phenotyping efforts. For example, Ibba et al. (2020) evaluated the prediction performance of 13 quality traits in wheat using two multi-trait models and five data sets based on field evaluations over two consecutive years. In the second year (testing), lines were predicted using the quality information obtained in the first year (training). For most of the quality traits, they found moderate to high prediction accuracies, suggesting that the use of GS at earlier stages could be recommendable. Overall, the results indicate that the Bayesian MTME model helps capture the correlation among traits and the correlation among years, thus increasing prediction accuracy. Finally, we envision perspectives of modeling MTME-based reaction norms involving other omics, such as phenomics and enviromics. The latter can enhance the MTME analysis in terms of creating more biological models of crop growth, development, and yield components (e.g., Robert et al., 2020).
Since the 1960s, several researchers have suggested the use of environmental information to explain the differences in cultivars due to G × E interactions (e.g., Perkins and Jinks, 1968; Freeman and Perkins, 1971; Wood, 1976; Vargas et al., 1998; Crossa et al., 1999). The use of genomics with enviromics is the basis for the prediction of cultivars across diverse growing conditions (e.g., Jarquín et al., 2014; Messina et al., 2018; Millet et al., 2019), which is useful for the prediction of global warming.
However, the efforts to implement environmental covariates into genomic selection models usually focus on a few environmental covariates such as temperature, precipitation, and sun radiation defined over specific developmental stages of the crop. With the use of large-scale envirotyping data, it is possible to design a global-scale envirotyping network of field trials to train GS models and perform “enviromic assembly” to predict a wider number of growing conditions from historical climate and soil data (R package EnvRtype, Costa-Neto et al., 2020b). In addition, research is underway for the study of model Enviromic + Genomic prediction (E-GP) to link genotype-phenotype variations, as well as to explain phenotypic variations across environments. As a predictive breeding tool, E-GP can contribute to the study of G × E structures, in which, as an exploratory tool, E-GP can contribute to the optimization of experimental networks of field trials and lead to more efficient training sets for GS (e.g., Rincent et al., 2017). In addition, for the early stages of selection, genomics and enviromics can be used to design optimized phenotyping trials and predict the breeding values of the selection candidate (Morais-Júnior et al., 2018) or single cross-hybrid prediction (Costa-Neto et al., 2020a).
Through enviromic assembly, it is possible to establish relatedness among field trials and thus use only the most representative set of experiments for training GS models. Another perspective of E-GP is the use of large-scale environmental data in training models involving genotype-specific reaction norms (e.g., Ly et al., 2018; Millet et al., 2019) and phenotypic landscapes implemented by genomics with crop growth models (CGM) (e.g., Messina et al., 2018; Bustos-Korts et al., 2019; Robert et al., 2020). The possible use of image-based responses related to main environmental stresses, such as heat and drought-stress, can also boost the implementation of genomic-assisted platforms for predictive purposes and are capable of better representing the plant-environment interplay.
In order to meet the well-documented challenges of food and nutrition security, there is a pressing need to use new technologies to accelerate the progress of plant breeding. These methods can be incorporated into conventional phenotypic breeding programs or help redesign established phenotypic breeding pipelines to enable a gradual shift toward a more data-driven perspective. The benefits of phenomics and enviromics together in benchmark genomic pipelines offer the potential to deliver larger increases in accuracy and efficiency of breeding pipelines when we select better-adapted genotypes in a cost-effective manner, as well as in a reduced timeframe. Genomics, phenomics, multi-trait, and enviromics analyses are interconnected, and their use can be optimized based on resources and program structure. Together, they offer a pathway for conventional phenotypic breeding to envision a diverse set of opportunities to accelerate genetic gains.
JC prepared the first drafts of the opinion, RF-N, OM-L, GC-N, SD, and AM-L read and corrected the first version. AB produced several reviews of the documents and worked with JC to finalize the definitive version. All authors contributed to the article and approved the submitted version.
Open Access fees are received from the Bill and Melinda Gates Foundation. We acknowledge the financial support provided by the Bill and Melinda Gates Foundation [INV-003439 BMGF/FCDO Accelerating Genetic Gains in Maize and Wheat for Improved Livelihoods (AG2MW)] as well as USAID projects [Amend. No. 9 MTO 069033, USAID-CIMMYT Wheat/AGGMW, AGG-Maize Supplementary Project, AGG (Stress Tolerant Maize for Africa)] that generated the CIMMYT data analyzed in this study. We are also thankful for the financial support provided by the Foundations for Research Levy on Agricultural Products (F.F.J.) and the Agricultural Agreement Research Fund (J.A.) in Norway through NFR grant 267806 as well as the CIMMYT CRP (maize and wheat).
The authors declare that the research was conducted in the absence of any commercial or financial relationships that could be construed as a potential conflict of interest.
Atkinson, J. A., Jackson, R. J., Bentley, A. R., Ober, E., and Wells, D. M. (2018). Field phenotyping for the future. Annu. Plant Rev. Online. doi: 10.1002/9781119312994.apr0651
Beyene, Y., Semagn, K., Mugo, S., Tarekegne, A., Babu, R., Meisel, B., et al. (2015). Genetic gains in grain yield through genomic selection in eight bi-parental maize populations under drought stress. Crop Sci. 55, 154–163. doi: 10.2135/cropsci2014.07.0460
Burgueño, J., de los Campos, G., Weigel, K., and Crossa, J. (2012). Genomic prediction of breeding values when modeling genotype X environment interaction using pedigree and dense molecular markers. Crop Sci. 52, 707–719. doi: 10.2135/cropsci2011.06.0299
Bustos-Korts, D., Malosetti, M., Chenu, K., Chapman, S., Boer, M. P., Zheng, B., et al. (2019). From QTLs to adaptation landscapes: using genotype-to-phenotype models to characterize G × E over time. Front. Plant Sci. 10, 1–23. doi: 10.3389/fpls.2019.01540
Collard, B. C. Y., Gregorio, G. B., Thomson, M. J., Islam, M. R., Vergara, G. V., Laborte, A. G., et al. (2019). Transforming rice breeding: re-designing the irrigated breeding pipeline at the International Rice Research Institute (IRRI). Crop Breed Genet. Genome 1:e190008. doi: 10.20900/cbgg20190008
Cooper, M., Technow, F., Messina, C., Gho, C., and Radu Totir, L. (2016). Use of crop growth models with whole-genome prediction: application to a maize multienvironment trial. Crop Sci. 56, 2141–2156. doi: 10.2135/cropsci2015.08.0512
Costa-Neto, G., Fritsche-Neto, R., and Crossa, J. (2020a). Nonlinear kernels, dominance, and envirotyping data increase the accuracy of genome-based prediction in multi-environment trials. Heredity (Edinb). 126, 92–106. doi: 10.1038/s41437-020-00353-1
Costa-Neto, G., Galli, G., Fanelli, H., Crossa, J., and Fritsche-Neto, R. (2020b). EnvRtype: a software to interplay enviromics and quantitative genomics in agriculture. bioRxiv[preprint]. doi: 10.1101/2020.10.14.339705
Crossa, J., Pérez-Rodríguez, P., Cuevas, J., Montesinos-López, O. A., Jarquín, D., de Los Campos, G., et al. (2017). Genomic selection in plant breeding: methods, models, and perspectives. Trends Plant Sci. 22, 961–975. doi: 10.1016/j.tplants.2017.08.011
Crossa, J., Vargas, M., and Joshi, A. K. (2010). Linear, bilinear, and linear-bilinear fixed and mixed models for analyzing genotype x environment interaction in plant breeding and agronomy. Can. J. Plant Sci. 90, 561–574. doi: 10.4141/CJPS10003
Crossa, J., Vargas, M., Van Eeuwijk, F. A., Jiang, C., Edmeades, G. O., and Hoisington, D. (1999). Interpreting genotype x environment interaction in tropical maize using linked molecular markers and environmental covariables. Theor. Appl. Genet. 99, 611–625. doi: 10.1007/s001220051276
Cuevas, J., Crossa, J., Montesinos-López, O. A., Burgueño, J., Pérez-Rodríguez, P., and de los Campos, G. (2017). Bayesian genomic prediction with genotype x environment kernel models. G3: Genes|Genomes|Genetics 7, 41–53. doi: 10.1534/g3.116.035584
Cuevas, J., Crossa, J., Soberanis, V., Pérez-Elizalde, S., Pérez-Rodríguez, P., de los Campos, G., et al. (2016). Genomic prediction of genotype x environment interaction kernel regression models. Plant Genome 9, 1–20. doi: 10.3835/plantgenome2016.03.0024
Cuevas, J., Granato, I., Fritsche-Neto, R., Montesinos-Lopez, O. A., Burgueño, J., Bandeira e Sousa, M., et al. (2018). Genomic-enabled prediction kernel models with random intercepts for multi-environment trials. G3: Genes|Genomes|Genetics 8, 1347–1365. doi: 10.1534/g3.117.300454
Cuevas, J., Montesinos-López, O. A., Juliana, P., Guzmán, C., Pérez-Rodríguez, P., González-Bucio, J., et al. (2019). Deep kernel for genomic and near infrared prediction in multi-environments breeding trials. G3: Genes|Genomes|Genetics 9, 2913–2924. doi: 10.1534/g3.119.400493
de los Campos, G., Pérez-Rodríguez, P., Bogard, M., Gouache, D., and Crossa, J. (2020). A data-driven simulation platform to predict cultivars' performances under uncertain weather conditions. Nat. Commun. 11:4876. doi: 10.1038/s41467-020-18480-y
Freeman, G. H., and Perkins, J. M. (1971). Environmental and genotype-environmental components of variability: Viii Relations between genotypes grown in different environments and measures of these environments. Heredity (Edinb). 27, 15–23. doi: 10.1038/hdy.1971.67
García-Ruiz, A., Cole, J. B., VanRaden, P. M., Wiggans, G. R., Ruiz-lópez, F. J., and Van Tassell, C. P. (2016). Changes in genetic selection differentials and generation intervals in US Holstein dairy cattle as a result of genomic selection. Proc. Natl. Acad. Sci. U.S.A. 113, E3395–E4004. doi: 10.1073/pnas.1519061113
Grattapaglia, D., Silva-Junior, O. B., Resende, R. T., Cappa, E. P., Müller, B. S. F., and Tan, B. (2018) Quantitative genetics genomics converge to accelerate forest tree breeding. Front. Plant Sci. 871, 1–10. doi: 10.3389/fpls.2018.01693.
Heslot, N., Akdemir, D., Sorrels, M. E., and Jannink, J.-L. (2014). Integrating environmental covariates and crop modeling into the genomic selection framework to predict genotype by environment interactions. Theor. Appl. Genet. 127, 463–480. doi: 10.1007/s00122-013-2231-5
Hickey, J. M., Crossa, J., Babu, R., and de los Campos, G. (2012). Factors affecting the accuracy of genotype imputation in populations from several maize breeding programs. Crop Sci. 52, 654–663. doi: 10.2135/cropsci2011.07.0358
Huang, M., Balimponya, E. G., Mgonja, E. M., McHale, L. K., Luzi-Kihupi, A., Guo-Liang Wang, G.-L., et al. (2019). Use of genomic selection in breeding rice (Oryza sativa L.) for resistance to rice blast (Magnaporthe oryzae). Mol. Breed. 39:114. doi: 10.1007/s11032-019-1023-2
Ibba, M. I., Crossa, J., Montesinos-López, O. A., Montesinos-López, A., Juliana, P., Guzman, C., et al. (2020). Genome-based prediction of multiple wheat quality traits in multiple years. Plant Genome 1:14. doi: 10.1002/tpg2.20034
Jarquín, D., Crossa, J., Lacaze, X., Du Cheyron, P., Daucour, J., Lorgeou, J., et al. (2014). A reaction norm model for genomic selection using high-dimensional genomic and environmental data. Theor. Appl. Genet. 127, 595–607. doi: 10.1007/s00122-013-2243-1
Lopez-Cruz, M., Olson, E., Rovere, G., Crossa, J., Dreisigacker, S., Mondal, S, et al. (2020). Regularized selection indices for breeding value prediction using hyper-spectral image data. Sci. Rep. 10. doi: 10.1038/s41598-020-65011-2
Ly, D., Huet, S., Gauffreteau, A., Rincent, R., Touzy, G., Mini, A., et al. (2018). Whole-genome prediction of reaction norms to environmental stress in bread wheat (Triticum aestivum L.) by genomic random regression. F. Crop. Res. 216, 32–41. doi: 10.1016/j.fcr.2017.08.020
Massman, J. M., Jung, H.-J. G., and Bernardo, R. (2013). Genomewide selection versus marker-assisted recurrent selection to improve grain yield and stover-quality traits for cellulosic ethanol in maize. Crop Sci. 53, 58–66. doi: 10.2135/cropsci2012.02.0112
Matias, F. I., Caraza-Harter, M. V., and Endelman, J. B. (2020). FIELDimageR: an R package to analyze orthomosaic images from agricultural field trials. Plant Phenome J. 3, 1–6. doi: 10.1002/ppj2.20005
Messina, C. D., Technow, F., Tang, T., Totir, R., Gho, C., and Cooper, M. (2018). Leveraging biological insight and environmental variation to improve phenotypic prediction: integrating crop growth models (CGM) with whole genome prediction (WGP). Eur. J. Agron. 100, 151–162. doi: 10.1016/j.eja.2018.01.007
Millet, E. J., Kruijer, W., Coupel-Ledru, A., Alvarez Prado, S., Cabrera-Bosquet, L., Lacube, S., et al. (2019). Genomic prediction of maize yield across European environmental conditions. Nat. Genet. 51, 952–956. doi: 10.1038/s41588-019-0414-y
Montesinos-López, A., Montesinos-López, O. A., Cuevas, J., Mata-López, W. A., Burgueño, J., Mondal, S., et al. (2017). Genomic Bayesian functional regression models with interactions for predicting wheat grain yield using hyper-spectral image data. Plant Methods 13:62. doi: 10.1186/s13007-017-0212-4
Montesinos-López, A., Montesinos-López, O. A., Gianola, D., Crossa, J., and Hernández-Suárez, C. M. (2018a). Multi-environment genomic prediction of plant traits using deep learners with a dense architecture. G3: Genes|Genomes|Genetics 8, 3813–3828. doi: 10.1534/g3.118.200740
Montesinos-López, M. A., Montesinos-López, A., Luna-Vázquez, J. F., Toledo, F. H., Paulino Pérez-Rodríguez, P., Lillemo, M., et al. (2019a). An R package for bayesian analysis of multi-environment and multi-trait multi-environment data for genome-based prediction. G3. Genes|Genomes|Genetics 9, 1355–1369. doi: 10.1534/g3.119.400126
Montesinos-López, O. A., Martin-Vallejo, J., Crossa, J., Gianola, D., Hernández-Suárez, C., Montesinos-López, A., et al. (2019b). A benchmarking between deep learning, support vector machine and Bayesian threshold best linear unbiased prediction for predicting ordinal traits in plant breeding. G3: Genes|Genomes|Genetics 9, 601–618. doi: 10.1534/g3.118.200998
Montesinos-López, O. A., Martín-Vallejo, J., Crossa, J., Gianola, D., Hernández-Suárez, C. M., Montesinos-López, A., et al. (2019c). New deep learning genomic-based prediction model for multiple traits with binary, ordinal, and continuous phenotypes. G3: Genes|Genomes| 9, 1545–1556. doi: 10.1534/g3.119.300585
Montesinos-López, O. A., Montesinos-López, A., Crossa, J., Toledo, F. H., Pérez-Hernández, O., Eskridge, K. M., et al. (2016). A genomic Bayesian multi-trait and multi-environment model. G3 Genes|Genomes|Genetics 6, 2725–2744. doi: 10.1534/g3.116.032359
Montesinos-López, O. A., Montesinos-López, A., Gianola, D., Crossa, J., and Hernández-Suárez, C. M. (2018b). Multi-trait, multi-environment deep learning modeling for genomic-enabled prediction of plant. G3: Genes|Genomes|Genetics 8, 3829–3840. doi: 10.1534/g3.118.200728
Monteverde, E., Gutierrez, L., Blanco, P., Pérez de Vida, F., Rosas, J. E, Bonnecarrère, V., et al. (2019). Integrating molecular markers and environmental covariates to interpret genotype by environment interaction in rice (Oryza sativa L.) Grown in Subtropical Areas. G3 Genes|Genomes|Genetics 9, 1519–1531. doi: 10.1534/g3.119.400064
Morais-Júnior, O. P., Duarte, J. B., Breseghello, F., Coelho, A. S. G., and Magalhães, A. M. Jr. (2018). Single-step reaction norm models for genomic prediction in multienvironment recurrent selection trials. Crop Sci. 58, 592–607. doi: 10.2135/cropsci2017.06.0366
Perkins, J. M., and Jinks, J. L. (1968). Environmental and genotype-environmental components of variability. 3. Multiple lines and crosses. Heredity (Edinb). 23, 339–356. doi: 10.1038/hdy.1968.48
Resende, R. T., Piepho, H. P., Rosa, G. J. M., Silva-Junior, O. B., Silva, F. F. E., de Resende, M. D. V., et al. (2020). Enviromics in breeding: applications and perspectives on envirotypic-assisted selection. Theor. Appl. Genet. 134, 95–112. doi: 10.1007/s00122-020-03684-z
Rincent, R., Kuhn, E., Monad, H., Oury, F. X., Rousset, M., Allard, V., et al. (2017). Optimization of multi - environment trials for genomic selection based on crop models. Theor. Appl. Genet. 130, 1735–1752. doi: 10.1007/s00122-017-2922-4
Rincent, R., Malosetti, M., Ababaei, B., Touzy, G., Mini, A., Bogard, M., et al. (2019). Using crop growth model stress covariates and AMMI decomposition to better predict genotype-by-environment interactions. Theor. Appl. Genet. 132, 3399–3411. doi: 10.1007/s00122-019-03432-y
Robert, P., Le Gouis, J., and Rincent, R. (2020). Combining crop growth modeling with trait-assisted prediction improved the prediction of genotype by environment interactions. Front. Plant Sci. 11, 1–11. doi: 10.3389/fpls.2020.00827
Rogers, A. R., Dunne, J. C., Romay, M. C., Bohn, M., Buckler, E. S., Ciampitti, I. A., et al. (2021). The importance of dominance and genotype-by-environment interactions on grain yield variation in a large-scale public cooperative maize. G3:Genes|Genomes|Genetics 1:jkaa050.doi: 10.1093/g3journal/jkaa050
Roorkiwal, M., Rathore, A., Das, R. R., Singh, M. K., Jain, A., Srinivasan, S., et al. (2016). Genome-enabled prediction models for yield related traits in Chickpea. Front. Plant Sci. 7:1666. doi: 10.3389/fpls.2016.01666
Vargas, M., Crossa, J., Sayre, K., Reynolds, M., Ramirez, M. E., and Talbot, M. (1998). Interpreting genotype X environment interaction using partial least squares regression. Crop Sci. 38, 679–689. doi: 10.2135/cropsci1998.0011183X003800030010x
Vivek, B. S., Krishna, G. K., Vengadessan, V., Babu, R., Zaidi, P. H., Kha, L. Q., et al. (2017). Use of genomic estimated breeding values results in rapid genetic gains for drought tolerance in maize (Zea mays L.). Plant Genome 10, 1–8, doi: 10.3835/plantgenome2016.07.0070
Wolfe, M. D., Del Carpio, D. P., Alabi, O., Ezenwaka, L. C., Ikeogu, U. N., Kayondo, I. S., et al. (2017). Prospects for Genomic Selection in Cassava Breeding. Plant Genome 10:15. doi: 10.3835/plantgenome2017.03.0015
Wood, J. T. (1976). The use of environmental variables in the interpretation of genotype-environment interaction. Heredity (Edinb). 37, 1–7. doi: 10.1038/hdy.1976.61
Keywords: genomics, phenomics, enviromics, high throughput phenotype, multi-trait, multi-environment
Citation: Crossa J, Fritsche-Neto R, Montesinos-Lopez OA, Costa-Neto G, Dreisigacker S, Montesinos-Lopez A and Bentley AR (2021) The Modern Plant Breeding Triangle: Optimizing the Use of Genomics, Phenomics, and Enviromics Data. Front. Plant Sci. 12:651480. doi: 10.3389/fpls.2021.651480
Received: 09 January 2021; Accepted: 11 February 2021;
Published: 16 April 2021.
Edited by:
Brian Gardunia, Bayer Crop Science, United StatesReviewed by:
Flavio Breseghello, Brazilian Agricultural Research Corporation (EMBRAPA), BrazilCopyright © 2021 Crossa, Fritsche-Neto, Montesinos-Lopez, Costa-Neto, Dreisigacker, Montesinos-Lopez and Bentley. This is an open-access article distributed under the terms of the Creative Commons Attribution License (CC BY). The use, distribution or reproduction in other forums is permitted, provided the original author(s) and the copyright owner(s) are credited and that the original publication in this journal is cited, in accordance with accepted academic practice. No use, distribution or reproduction is permitted which does not comply with these terms.
*Correspondence: Alison R. Bentley, QS5CZW50bGV5QGNnaWFyLm9yZw==
Disclaimer: All claims expressed in this article are solely those of the authors and do not necessarily represent those of their affiliated organizations, or those of the publisher, the editors and the reviewers. Any product that may be evaluated in this article or claim that may be made by its manufacturer is not guaranteed or endorsed by the publisher.
Research integrity at Frontiers
Learn more about the work of our research integrity team to safeguard the quality of each article we publish.