- 1Department of Plant Sciences, University of California, Davis, Davis, CA, United States
- 2College of Bioscience and Biotechnology, Chubu University, Kasugai, Japan
- 3ARS Crops Pathology and Genetics Unit, United States Department of Agriculture, Davis, CA, United States
We showed previously that gallic acid is produced in walnut from 3-dehydroshikimate by a shikimate dehydrogenase (JrSkDH). This study focuses on the next step in the hydrolysable tannin pathway, the formation of 1-O-galloyl-β-D-glucose from the phenolic gallic acid and UDP glucose by a glycosyltransferase. JrGGT1 (UGT84A73) and JrGGT2 (UGT84A74) are predicted to be two such glycosyltransferases, which we expressed in tobacco plants. GC-MS analysis of the transgenic tobacco revealed moderate, yet significant alterations in plant secondary metabolism, such as depleted phenolic acids, including gallic acid. We postulate that these effects are due to JrGGT1 and JrGGT2 activity, as JrGGT orthologs glycosylate these phenolic compounds in vitro. Moreover, JrGGT expression in tobacco caused upregulation of shikimic acid pathway metabolites and differing responses in phenylpropanoids, such as phenolic acids and flavonoids. In transcriptome analysis of walnut pellicle tissues, both JrGGTs showed substantial and significant expression correlations with the gallic acid-producing JrSkDHs and were highly coexpressed with the genetic circuits constituting the shikimic acid and phenylpropanoid biosynthetic pathways. Verification of JrGGT gene expression by transcriptome analysis of 20 walnut tissues revealed striking similarities with that of the pellicle data, with the greatest expression in roots, wood, buds, and leaves of Juglans regia cv. Chandler: tissues that typically accumulate hydrolysable tannins. Like the transgenic tobacco, pellicle metabolomic analyses revealed that many phenylpropanoids correlated negatively with JrGGT expression, while shikimic acid pathway metabolites correlated positively with JrGGT expression. This research supports the hypothesis that JrGGT1 and JrGGT2 play non-trivial roles in metabolism of phenolic acids, flavonoids, and ostensibly, tannins.
Introduction
Phenolic compounds are a large class of plant secondary metabolites that include phenolic acids, flavonoids, and tannins (Abbas et al., 2017). Phenolic acids and flavonoids are considered “simple” compared to the more “complex” tannins, since the latter are usually polymers of the former. These phytochemicals help plants endure stresses due to water, heat, physical damage, pathogens, and herbivory (Sharma et al., 2019). Phenolic compounds are bioactive, highly bioavailable, and beneficial to human health, protecting against the adverse effects of hyperlipidemia and hyperglycemia and showing anticancer activities (Khan and Mukhtar, 2019).
Biosynthesis of phenylpropanoids like phenolic acids and flavonoids begins at the shikimic acid trunk. The irreversible condensation of erythrose-4-phosphate (E-4-P) from the pentose phosphate pathway and phosphoenolpyruvate (PEP) from glycolysis by 3-deoxy-D-arabinoheptulosonate 7-phosphate synthase (DAHPS) forms the seven-carbon 3-deoxy-D-arabinoheptulosonate 7-phosphate (Widhalm and Dudareva, 2015) (Figure 1). Cyclization of deoxyarabinoheptulosonate by a dehydroquinate synthase (DHQS) results in the formation of 3-dehydroquinate (3-DHQ). Catalysis of the third and fourth reactions of the shikimic acid trunk in J. regia occurs by a bifunctional shikimate dehydrogenase (JrSkDH) (Muir et al., 2011) (Figure 1). JrSkDH facilitates a two-step reaction in which 3-DHQ is converted to 3-dehydroshikimate (3-DHS) by the dehydratase subunit and is subsequently acted on by the NADP+/(H)-dependent dehydrogenase subunit to produce shikimate (Muir et al., 2011). This assembly of two enzymes is different than in bacteria, where dehydratase and dehydrogenase are separate enzymes (Michel et al., 2003; Muir et al., 2011; Maeda and Dudareva, 2012).
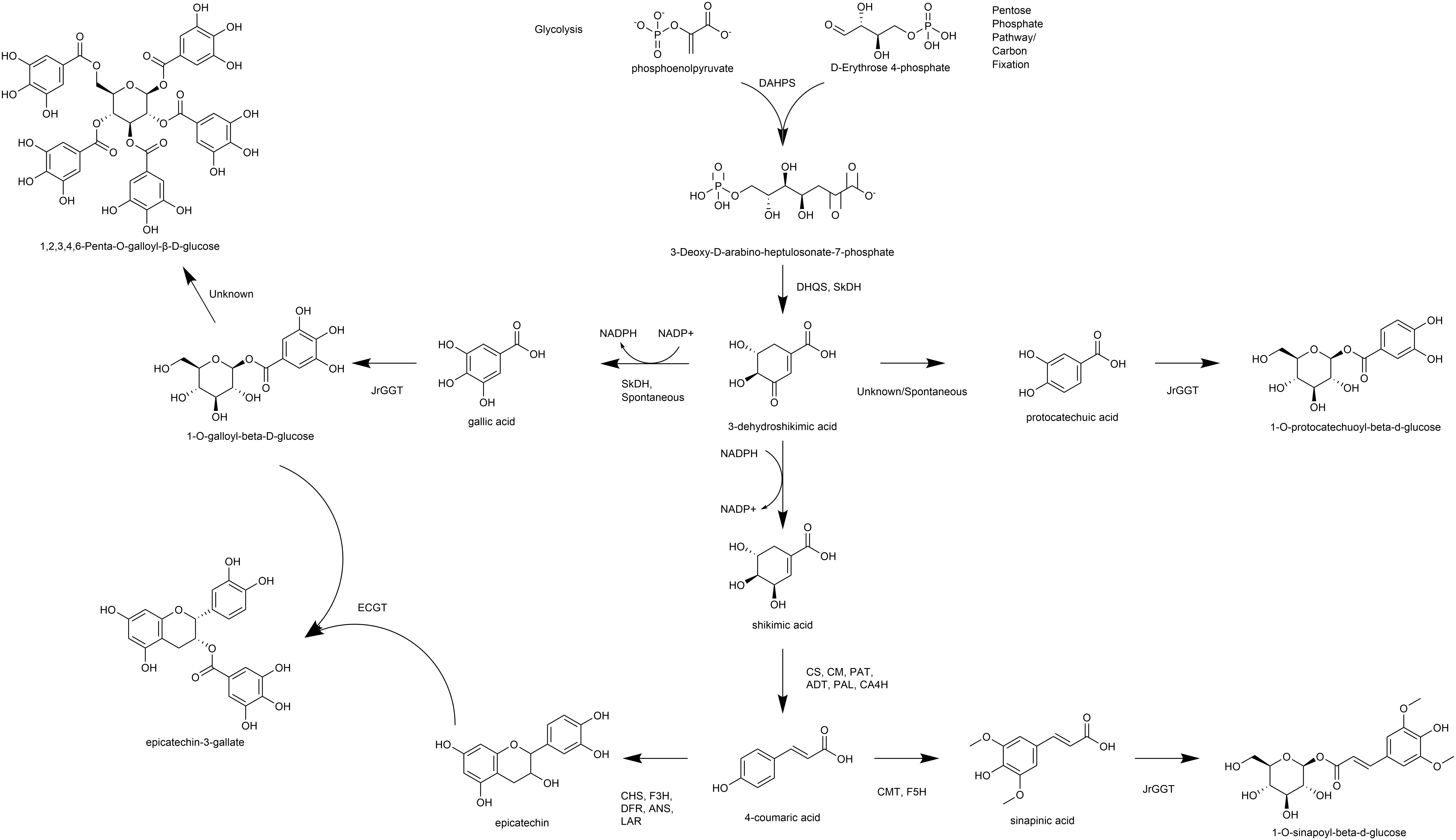
Figure 1. Overview of pathways, enzymes, and metabolites primarily associated with HBA/HCA UGT activity. DAHPS, 3-deoxy-D-arabinoheptulosonate 7-phosphate synthase; DHQS, dehydroquinate synthase; SkDH, bifunctional dehydroquinate dehydratase/shikimate dehydrogenase; CS, chorismate synthase; CM, chorismate mutase; PAT, bifunctional aspartate aminotransferase and glutamate/aspartate-prephenate aminotransferase; ADT, arogenate dehydratase/prephenate dehydratase; PAL, phenylalanine ammonia lyase; CA4H, cinnamic acid-4-hydroxylase; CMT, caffeic acid 3-O-methyltransferase; F5H, ferulate-5-hydroxylase; CHS, chalcone synthase; F3H, naringenin, 2-oxoglutarate 3-dioxygenase; DFR, dihydroflavonol-4-reductase; LAR, leucoanthocyanidin reductase; ANS, leucoanthocyanidin dioxygenase; UGT, uridine diphosphate glycosyltransferase.
The first committed step of phenylpropanoid metabolism begins past the shikimic acid trunk and encompasses the biosynthesis of hydroxycinnamic acids (HCA) and flavonoids. Phenylalanine ammonia-lyase (PAL) deaminates phenylalanine to produce ammonia and the HCA cinnamic acid (Blount et al., 2000). Cinnamic acid is then hydroxylated to produce 4-HCA (also p-coumaric acid) by cinnamic acid 4-hydroxylase (C4H), which is followed by 4-coumarate-CoA ligase (4CL) to produce p-coumaroyl CoA (Deng and Lu, 2017). At this step, p-coumaroyl CoA can commit either to the flavonoid pathway via chalcone synthase (CHS) or to the HCA pathway via hydroxycinnamoyl-CoA: shikimate/quinate hydroxycinnamoyl transferase (HCT) (Deng and Lu, 2017). HCT acts on p-coumaroyl CoA to produce caffeoyl shikimic acid and caffeoyl quinic acid (QA; chlorogenic acid). Caffeoyl shikimic acid can then be converted to caffeic acid by coumaroylshikimate esterase (CSE). Alternatively, 4-HCA can be converted to caffeic acid directly by C4H (Chen et al., 2011). Ferulic and sinapinic are biosynthesized successively by CMT with the methyl group being incorporated by S-adenosylmethionine (Urban et al., 1994; Wang et al., 2018, 2019). CHS constitutes the first committed step of flavonoid biosynthesis by condensation of 4-coumaroyl-CoA (from 4-HCA) and malonyl-CoA and thus is linked tightly to phenylpropanoid metabolism (Nakayama et al., 2019) (Figure 1). Subsequently, the flavanones, including naringenin, are formed by chalcone isomerase (CHI), which are acted on by flavanone 3-hydroxylase (F3H) to produce the flavanols. Dihydroflavonol-4-reductase (DFR) acts on the flavonols to make the leucoanthocyanidins, which can be channeled to the flavan-3-ols by leucoanthocyanidin reductase (LAR) or to the anthocyanidins by anthocyanidin synthase (ANS) (Nakayama et al., 2019).
The polyphenolic hydrolysable tannins (HTs) consist of a central glucose moiety that is esterified with at least five galloyl residues (Grundhöfer et al., 2001) (Figure 1). The HTs constitute a class of polyphenolic compounds found abundantly in the oak family that includes walnut (Order Fagales). HTs are used for tanning leather because of their unique property to precipitate proteins, which may also be related to their ability to provide pest, pathogen, and metal toxicity resistance.
We were the first to show that gallic acid (GA), a key intermediate in the biosynthesis of HTs, is made enzymatically from a shikimate dehydrogenase (SkDH) via the shikimic acid pathway (Muir et al., 2011) (Figure 1). This enzyme represents a branching point in the pathway, where 3-DHS can either be oxidized to the hydroxybenzoic acid (HBA) GA (NADP+-dependent), spontaneously converted to the HBA GA and PCA, or be reduced to shikimic acid (NADPH-dependent) (Muir et al., 2011; Bontpart et al., 2016) (Figure 1).
The first committed step in HT biosynthesis is formation of 1-O-galloyl-β-D-glucose from GA and UDP-glucose (UDPG) by a UDP-glycosyltransferase (UGT) (Gross, 2008) (Figure 1). Experiments in oak (Quercus robur), pomegranate (Punica granatum), grape (Vitis vinifera), strawberry (Fragaria × ananassa), and tea (Camellia sinensis) have characterized genes and enzymes with UGT activity toward phenolic acids and flavonoids, with particular emphasis on GA (Lunkenbein et al., 2006; Khater et al., 2012; Mittasch et al., 2014; Cui et al., 2016; Ono et al., 2016). Double RNAi knockdown of UGT84A23 and UGT84A24 in pomegranate hairy roots drastically reduced HTs in these tissues (Ono et al., 2016). Moreover, biosynthesis of the famous tea tannins, epicatechin gallate, and epigallocatechin gallate was achieved with 1-O-galloyl-β-D-glucose acting as the galloyl donor (Liu et al., 2012). Interestingly, the JrGGT orthologs display different degrees of promiscuity among phenolic compounds. For example, UGT84A13 from oak displayed a clear preference for glycosylating HBAs over HCAs by specific activity (Mittasch et al., 2014). Conversely, UGT84A23 and UGT84A24 from pomegranate did not discriminate between HBAs and HCAs and even showed activity with some flavonoids (Ono et al., 2016). Similarly, UGT84A22 from tea did not prefer HBAs over HCAs (Cui et al., 2016). Lastly, UGT84A44 (VvgGT1), VvgGT2, and VvgGT3 displayed an insignificant, slight inclination for HBAs over HCAs, with no significant activity detected with flavonoids (Khater et al., 2012).
This class of glycosyltransferases (GTs) is grouped into the 1-glycosyltransferase family (of 108 families) and relies upon uridine diphosphate (UDP) sugars as the donor substrate, according to the Carbohydrate-Active enZYmes Database (CAZy) (Lombard et al., 2014). The 1-glycosyltransferases are further organized into 16 groups (A-P) (Caputi et al., 2012). Using the group L UGT from Quercus robur UGT84A13 (Mittasch et al., 2014) and the UGT conserved PSPG motif, the Juglans regia transcriptome was queried and ∼ 130 different UGT genes were identified (Martinez-Garcia et al., 2016). In a search for enzymes with putative UGT84A activity, two genes shared the greatest similarity to the group L UGT84A13 and were named JrGGT1 and JrGGT2. While many studies have elucidated in vitro functions of UGT84As, few have examined their in vivo effects and behavior as we present in this paper (Lunkenbein et al., 2006; Khater et al., 2012; Mittasch et al., 2014; Cui et al., 2016; Ono et al., 2016). The genomics, transcriptomics, and resultant metabolomics of the putative tannin biosynthetic enzymes are presented in this study. We intend to build upon the current body of knowledge to elucidate the genetic and metabolic background of the first committed step of HT biosynthesis in Juglans regia.
Materials and Methods
J. regia Somatic Embryos and N. tabacum SR1 Plants
JrGGT1 and JrGGT2 sequences were amplified from DNA extracted from somatic embryo tissue of Juglans regia cv. Chandler. Nicotiana tabacum cv. Petit Havana (SR1 tobacco) was used in transformation experiments to express JrGGT1 & 2.
J. regia Pellicle Tissue
Three cultivars of Juglans regia were chosen for transcriptome and metabolome profiling. Walnuts were collected in the UC Davis campus research orchards. A single tree of each cultivar was identified and tagged for repeated nut harvests. Nuts were harvested from each at three developmental stages: Stage 2 (85 days post-peak bloom), Stage 3 (110 days post-peak bloom), and Stage 4 (150 days post-peak bloom). At each harvest, nuts were chosen from the lower perimeter of the tree in each cardinal direction. Nuts of similar proportions representing five biological replicates were removed, placed in a one-gallon Ziploc© bag, and transported back to the laboratory on wet ice in a cooler. Nut length and width were measured with a caliper to ensure consistent maturity within each sample set. The nuts were dissected quickly to remove pellicle tissues; excised pellicle tissue was frozen in liquid N2 and kept frozen at −80°C. Frozen, excised pellicles were ground under liquid N2, and 0.10 to 0.15 g of powdered tissue was used for transcriptome and metabolome analysis.
Gene Characterization
JrGGT1 and JrGGT2 sequences were identified previously (Martinez-Garcia et al., 2016). BLASTP (a trademark of NCBI, Basic Local Alignment Search Tool) was also performed using the NCBI-predicted, full-length amino acid sequence of JrGGT1 as a query. In addition to JrGGT2, seven functionally characterized UGTs and one non-functionally characterized UGT were selected from these results.
Cloning of JrGGT1 and JrGGT2 and Expression in Tobacco
Genomic DNA was extracted from somatic embryos of Juglans regia cv. Chandler using NucleoSpin Plant II (TaKaRa Bio, Japan). Four primer sets were designed according to the Juglans regia genome database in NCBI to clone JrGGTs (JrGGT1 and JrGGT2) from walnut (Supplementary Table 1). PCR reactions were conducted using genomic DNA as a template. Primers “FW: JrGGT1” and “Rv: JrGGT1” were used to clone JrGGT1, while “FW: JrGGT2” and “Rv: JrGGT2” were used to clone JrGGT2. PCR reactions were as follows: one cycle of 98°C for 1 min followed by 36 cycles of 98°C for 10 s, 60°C for 15 s, and 68°C for 90 s. To avoid carryover of template DNA in the cloning reactions, all PCR fragments were purified by 0.8% (w/v) agarose gel electrophoresis and subsequent spin column recovery using NucleoSpin Gel and PCR Clean-up (TaKaRa Bio, Japan). The amplicons were subsequently cloned into the pCR2.1-TOPO vector using a TOPO TA Cloning Kit (Thermo Fisher Scientific, United States). The resultant plasmids were transformed into E. coli by heat shock using TOP10 competent cells (Thermo Fisher Scientific, United States). Colonies containing each plasmid (JrGGT1 and JrGGT2) were screened by PCR using primers “FW: JrGGT1,” “Rv: JrGGT1,” “FW: JrGGT2,” and “Rv: JrGGT2.” Plasmid DNA from selected colonies was isolated (QIAprep Spin Miniprep Kit, Qiagen, Germany) and sequenced (Quintara Biosciences, United States).
After confirmation of native JrGGT sequences, we synthesized JrGGT1 and JrGGT2 coding sequences (CDS) to include an encoded six-histidine tag at the 3′ end (Supplementary Figure 1). These synthesized fragments were inserted into a binary vector with In-Fusion HD Cloning (Clontech, United States) to generate JrGGT1 and JrGGT2 overexpressing vectors (Supplementary Figure 1). The resultant plasmids, pDH17.0301 (JrGGT1) and pDH17.0401 (JrGGT2), were transformed into E. coli HST08 by heat shock. After proper selection, DNA was extracted from Stellar competent cells containing pDH17.0301 (JrGGT1) and pDH17.0401 (JrGGT2), then electroporated into Agrobacterium tumefaciens strain EHA 105 PCH32 according to a protocol adapted from a previously published one (Shen and Forde, 1989). Then, pDH17.0301 and pDH17.0401 were used to transform N. tabacum cv. SR1 using Agrobacterium-mediated plant transformation at the Ralph M. Parsons Plant Transformation Facility1.
RNA Extraction of Pellicle Tissue and cDNA Library Preparation
Total mRNA was extracted from pellicles representing five independent biological replicates (each nut being a biological replicate) for each of three genotypes and three time stages for 45 samples total. The PureLink Plant RNA Reagent (Invitrogen; Carlsbad, CA, United States) was used for the extraction per manufacturer’s protocol, with modifications. Due to the low water content and high lipid concentration in pellicles, the ratio of PureLink Reagent: tissue was increased from 500 μL PureLink Reagent: 0.1 g tissue to 500 μL PureLink Reagent: 0.025 ground pellicle, a four-fold increase in the extraction buffer. RNA pellets were resuspended in 30 μL nuclease-free water (Ambion; Austin, TX, United States) and suspended overnight on wet ice in a 4°C chamber for 16 h. The construction of cDNA libraries was performed in accordance with a published protocol (Illumina TruSeq Stranded Sample Preparation Guide 2014). Total RNA molecules were chemically fragmented in the presence of Fragment, Prime Finish Buffer for 8 min at 94°C, followed by a 4°C incubation to arrest the chemical cleavage of mRNA molecules. These fragmentation patterns yield, on average, a cDNA insert size of 120 bp. A BIOO Scientific NEXTflexTM Rapid Directional RNA-seqTM Library Prep Kit Adapter kit containing 48 unique adapter sequences was used to generate uniquely labeled samples. Thirty microliters of uniquely barcoded cDNA libraries were transferred to non-stick, RNAse-free 0.5 mL microfuge tubes (Ambion, Austin, TX, United States) and stored at −20°C prior to sequencing by synthesis.
Sequencing by Synthesis—RNA-Seq and Bioinformatics Analysis of RNA-Seq Data
cDNA sequencing was conducted at the UC Davis Expression Analysis Core Facility (UCD EACF) using an Illumina HiSeq 3000 machine. Each cDNA library was sequenced in one direction for 50 nucleotides (NT). The quality of the raw reads was checked using FastQC2, and the low-quality bases were trimmed using a custom-made Perl script. The adapters were removed using Cutadapt Version 1.8.1 (Martin, 2011). To remove the rRNAs, the cleaned reads were aligned to the SILVA rRNA database3 using Bowtie Version 2.2.8 (Langmead et al., 2009) and only the unaligned reads were considered for further analysis. The preprocessed reads were then mapped to the walnut (Juglans regia) reference genome downloaded from NCBI4 using “splicing-aware” aligner HISAT (hierarchical indexing for spliced alignment of transcripts; Version 2.0.5) (Kim et al., 2015). The normalized gene expression levels in FPKM (fragments/kb transcript per million mapped reads) of all samples were estimated using Cufflinks Version 2.2.1 (Trapnell et al., 2013). The RNA-Seq data analysis workflow is illustrated in Supplementary Figure 2.
Purification of Recombinant Protein
The protein was extracted by weighing 400 mg frozen leaf tissue into 2-mL microcentrifuge tubes. A 3-mm stainless steel bead was then added to each tube along with 1 mL binding buffer (Milli-Q water, 50 mM Tris–HCl, 20 mM imidazole, 500 mM NaCl, and 15% (v/v) glycerol; pH adjusted to 7.6). The samples were lysed in a Qiagen Retsch MM300 TISSUELYSER (Haan, Germany) at maximum frequency for 90 s and subsequently centrifuged at 20,000 × g for 20 min. The supernatant was then purified using the Zymo His-Spin Protein Miniprep kit (Irvine, CA, United States). The purified eluted protein was then added to an Amicon Ultra-0.5 Centrifugal Filter Unit (30 kDa) and centrifuged at 14,500 × g until 40 μL of volume was achieved. Then, 450 μL dilution buffer (Milli-Q water, 50 mM Tris–HCl and 20% (v/v) glycerol; pH 7.6) was added to 40 μL protein (total volume 490 μL) and centrifuged until 40 μL was achieved for a second time. This step was repeated twice to remove most of the imidazole and NaCl and to concentrate the protein. The protein was then quantified using Qubit Fluorometric Quantification (Thermo Scientific, Waltham, MA, United States).
Metabolomic Analysis
JrGGT1- and JrGGT2-Overexpressing Tobacco
Leaf tissue samples representing six independent biological replicates, each from JrGGT1- and JrGGT2-overexpressing tobacco lines, and leaf tissue samples representing seven independent biological replicates from UT lines were sent to the West Coast Metabolomics Center (WCMC) in the Genome Center at UC Davis for primary metabolism analysis by gas chromatography/time-of-flight mass spectrometry (GCTOF). Twenty ±5-mg fresh weight of tobacco leaves were harvested and frozen immediately in liquid nitrogen. Tissue was homogenized under liquid nitrogen using a Retsch mill. Two mL of a single-phase solvent mixture of methanol/chloroform/water 2.5:1:1 v/v/v kept at −20°C was added to the tissue and thoroughly mixed at 4°C for 30 min to precipitate proteins and DNA/RNA and to disassociate metabolites from membrane and cell wall components. After centrifugation, the remaining pellet consisting of DNA/RNA, proteins, starch, membranes, and cell wall components was extracted in a second step with 1 mL methanol/chloroform 1:1 v/v at −20°C. The organic solvent extracts were combined and used for metabolite analysis via GC-TOF.
For GC-TOF MS (Leco Pegasus IV GC-TOF mass spectrometer; Leco, St. Joseph, MO, United States) analysis, the organic phase was dried and dissolved in 50 mL methoxamine hydrochloride (20 mg/mL pyridine) and incubated at 30°C for 90 min with continuous shaking. Then, 80 mL N-methyl-N-trimethylsilyl trifluoroacetamide (MSTFA) was added at 37°C for 30 min to derivatize polar functional groups. The derivatized samples were stored at room temperature for 120 min before injection. GC-TOF analysis was performed on an HP 5890 gas chromatograph with tapered, deactivated split/splitless liners containing glass wool (Agilent, Böblingen, Germany) and 1 mL split-less injection at 230°C injector temperature. The GC was operated at a constant flow of one mL/min helium with a 40 m 0.25 mm id 0.25 mm RTX-5 column with 10 m integrated precolumn. The temperature gradient started at 80°C, was held isocratic for 2 min, and subsequently ramped at 15°C/min to a final temperature of 330°C, which was held for 6 min. Twenty spectra per second were recorded between m/z 85 and 500. Peak identification and quantification were performed using the Pegasus software package (Leco, St. Joseph, MO, United States).
Reference chromatograms were defined that had a maximum of detected peaks over a signal/noise threshold of 20 and were used for automated peak identification based on mass spectral comparison to a standard NIST98 library (Mclafferty et al., 1999). Automated assignments of unique fragment ions for each individual metabolite were taken as default as quantifiers and manually corrected where necessary. All artifactual peaks caused by column bleeding or phthalates and polysiloxanes derived from MSTFA hydrolyzation were identified manually and removed from the results table.
Metabolite data were then normalized using the mTIC method by calculating the sum of all peak heights for all identified metabolites (but not the unknowns) in each sample. Such peak sums are called “mTIC” in analogy to the term TIC used in mass spectrometry (for “total ion chromatogram”), but with the notification “mTIC” to indicate that only genuine metabolites (identified compounds) were used to avoid using potential non-biological artifacts for the biological normalizations, such as column bleed, plasticizers, or other contaminants.
Subsequently, it was determined whether the mTIC averages are significantly different between treatment groups or cohorts. If these averages were different by p < 0.05, data was normalized to the average mTIC of each group. If averages between treatment groups were not different, data was normalized to the total average mTIC.
Metabolite Analysis of J. regia Pellicle Tissue
Pellicles representing five biological replicates (each nut being a biological replicate) for each of the three genotypes at each of the three time stages, for a total of 45 samples, were shipped to Metabolon (Research Triangle Park, NC, United States) for sample preparation and analysis. Pellicle samples were prepared using the automated MicroLab STAR® system from Hamilton Company (Reno, NV, United States) with the addition of recovery standards. To disassociate protein-bound metabolites, the samples were mixed with methanol and shaken vigorously for 2 min using a Glen Mills GenoGrinder 2000 (SPEX Sample Prep; Metuchen, NJ, United States); proteins were pelleted by centrifugation. Sample extracts were dried, then reconstituted in solvents compatible with four methods: two for analysis on two separate reversed-phase (RP) ultraperformance liquid chromatography (UPLC)–mass spectroscopy (MS/MS) with positive ion electrospray ionization (ESI) platforms; one for analysis on an RP/UPLC-MS/MS with negative ESI platform; and one for analysis by hydrophilic interaction chromatography (HILIC)/UPLC-MS/MS with negative ion mode ESI. One sample was reserved for backup. Each solvent contained a series of standards at fixed concentrations to ensure injection and chromatographic consistency.
A global, unbiased metabolic profiling of J. regia pellicles was performed using a Waters (Milford, MA, United States) ACQUITY ultra-performance liquid chromatography (UPLC) and a Thermo Scientific (Waltham, MA, United States) Q-Exactive high-resolution/accurate mass spectrometer interfaced with a heated electrospray ionization (HESI-II) source with an Orbitrap mass analyzer for metabolite separation and identification. The MS analysis alternated between MS and data-dependent MSn scans using dynamic exclusion. The scan range varied slightly between methods but covered 70 to 1000 m/z. Raw data files were archived and extracted as described below.
Compounds were identified by comparison to a library of >3300 purified, authenticated standards containing the retention time/index (RI), m/z, and chromatographic data (including MS/MS spectra) of each molecule. Identifications were based on three criteria: retention index, accurate mass match to the library, and a comparison of MS/MS forward and reverse scores. Artifactual peaks were identified and removed by comparing experimental samples with process (ultra-pure water) and solvent blanks. Peaks were quantified using area-under-the-curve. To correct for minor variations, overall process variance (which includes MS peak quantitation variance) was established by running a pool of technical replicates made from a pool of experimental replicates interspersed evenly among the experimental samples throughout each run day. Data normalization was performed to correct instrument inter-day tuning differences. Essentially, each compound was corrected in run-day blocks by registering the medians to equal one (1.00) and normalizing each data point proportionately.
Free Amino Acid Analysis
Extraction
Free amino acids were extracted from tobacco leaf tissue samples representing six independent biological replicates each for JrGGT1 and JrGGT2 expressing and UT lines based as described (Hacham et al., 2002). One hundred and fifty milligrams of frozen tissue was weighed into two-mL Eppendorf tubes and cooled in liquid nitrogen. Six hundred microliters of water:chloroform:methanol (3:5:12 v/v) and a metal lysing bead were added to each tube. The samples were lysed in a Qiagen Retsch MM300 TISSUELYSER at maximum frequency for 90 s and sonicated in a Sonicor for 15 min. After sonication, the lysates were centrifuged at 21,000 × g for 10 min. The supernatants were collected, and the pellets were extracted once more. After combining supernatants, 300 μL chloroform and 450 μL water were added to each sample and centrifuged for 2 min. The resultant samples were separated into a chloroform phase at the bottom and water/methanol phase on top. The upper water/methanol phase was collected into new two-mL Eppendorf tubes and evaporated to dryness at room temperature using a speed-vac. The samples were then stored at −20°C. The Molecular Structure Facility at UC Davis conducted the analysis as described below.
Quantitative Analyses of Free Amino Acids in Physiological Fluids
Extracted sample pellets from JrGGT1- and JrGGT2-expressing and UT tobacco were dissolved in 100 nmol/mL AE-Cys (internal standard) Li (lithium) diluent before the 50-μL injection. Free amino acids were separated using strong cation-exchange chromatography with a post-column ninhydrin reaction for detection. Hitachi (Chiyoda, Tokyo, Japan) supplied column and buffers, and Wako (Richmond, Virginia) supplied ninhydrin. Calibration of the Hitachi 8900 was performed using amino acid standards (Sigma-Aldrich, St. Louis, MO, United States). Absorbance was recorded at both 570 nm and 440 nm after the reaction with ninhydrin to determine the response factor for each amino acid and to quantify relative to the known amino acid standards. The included internal standard (AE-Cys) was used to correct for any variance in injection volume due to the auto-sampler.
Statistical Analysis
Feature Correlation Analysis of RNAs and Metabolites of J. regia Pellicle Tissue
RNA and metabolite data from pellicle tissue were analyzed using MetaboAnalystR (Xia et al., 2015). The “peak intensities” option was selected for data preprocessing. No data filtering was conducted. No normalization was conducted as Spearman correlation is a non-parametric analysis. MetaboAnalyst R function, FeatureCorrelation, was used with the argument, method = “spearman,” to return all RNAs and metabolites associated with JrGGT1 and JrGGT2 expression. The significance threshold was set at P < 0.05. Features meeting this threshold will be termed “significant” throughout this paper. Significance for metabolome pellicle (Mp) and transcriptome pellicle (Tp) were calculated using 45 independent biological replicates. Significance for T20 was calculated using 20 independent biological replicates.
Metabolomics Analysis of JrGGT1 and JrGGT2 Overexpressing Tobacco
The metabolomics data were analyzed using MetaboAnalystR. The “peak intensities” option was selected for data preprocessing, and no IQR filtering was performed. Normalization by auto-scaling and general logarithm transformation was used to achieve normal distributions. Principal component analysis was performed to observe unbiased sample clustering, while partial least-square discriminant analysis (PLS-DA) was used to determine differentially expressed metabolites. Metabolites were only considered “significant” or “differentially expressed” if they had a variable importance in projection (VIP) score of one or higher. Features meeting this threshold are termed “significant” throughout this paper.
Free Amino Acid Analysis of JrGGT1- and JrGGT2-Overexpressing Tobacco
The free amino acid data were analyzed using MetaboAnalystR. The “peak intensities” option was selected for data preprocessing and IQR filtering was performed. Normalization by auto-scaling and general logarithm transformation was used to achieve a more normal distribution. Partial least-square discriminant analysis (PLS-DA) was used to determine differentially expressed metabolites. Metabolites were only considered “significant” or “differentially expressed” if they had a variable importance in projection (VIP) score of one or higher. Features meeting this threshold are termed “significant” throughout this paper.
Results
Cloning of JrGGTs
In an earlier study, we identified two genes, JrGGT1 and JrGGT2, based on their significant homology to QrUGT84A13 and postulated that these two genes were likely candidates involved in the synthesis of 1-O-galloyl-β-D-glucose, an essential precursor for synthesis of hydrolysable tannins (HTs) (Martinez-Garcia et al., 2016). There was close structural similarity of JrGGT1, located on chromosome 16, and JrGGT2, present on chromosome 13, to enzymes from oak, pomegranate, grapevine, and tea (Table 1). In the present study, we performed a functional analysis of these two genes expressed in tobacco, combined with RNA-seq and metabolomics analyses of walnut tissues. Based on the recommendation of the UNC, JrGGT1 was named UGT84A73 and JrGGT2 was named UGT84A74 (Mackenzie et al., 2005). We cloned full-length protein-encoding regions corresponding to JrGGT1 and JrGGT2 directly from DNA extracted from Juglans regia cv. Chandler using the primers shown in Supplementary Table 1 since neither gene has introns. The coding sequences for both were contiguous in the walnut genome.
JrGGT1 and JrGGT2 Coexpressed With Genes and Metabolites Responsible for HBA, HCA, and Flavonoid Biosynthesis
To investigate in natura the relationships between the JrGGTs and HBA, HCA, and flavonoid metabolism, we examined RNAs and metabolites associated with JrGGT1 and JrGGT2 expression. We used transcriptome data from 20 walnut tissues (T20) (Chakraborty et al., 2016) and transcriptome (Tp) and metabolome (Mp) data of pellicle tissue from three varieties of walnut at three different stages of maturity for our analyses. Tp and Mp data sets were merged according to their respective sample ID to conduct bivariate analysis. Like UGT84A13, both JrGGTs’ highest expression was in roots, wood, buds, and leaves: tissues that typically accumulate HTs and phenolic acid glycosides (Mittasch et al., 2014) (Figure 2). JrGGT1 had relatively low expression (<100 FPKM) in most tissues except for in packing tissue mature (PTM), pistillate flower (PF), vegetative bud (VB), transition wood (TW), and root (R) (Figure 2 and Supplementary Table 2). JrGGT2 was expressed at >100 FPKM in more tissues, including PTM, leaf early (LE), hull (H), hull dehiscing (HD), VB, leaf (L), catkin (C), leaf mature (LM), PF, TW, and R (Supplementary Table 2). Expression of JrGGT1 in pellicle tissue was about three times less than that of JrGGT2 in T20 (Figure 2 and Supplementary Table 2). Tp data corroborates that JrGGT1 expression on average is lower than JrGGT2 (Figure 2). JrGGT2 displayed uniquely high expression in leaf tissues compared to JrGGT1. Spearman feature correlation analysis resulted in 12,321 RNAs (Tp), 97 metabolites (Mp), and 14,320 RNAs (T20) that correlated significantly with JrGGT1 expression (Table 2). JrGGT2 expression correlated with 8,253 RNAs (Tp), 27 metabolites (Mp), and 17,093 RNAs (T20).
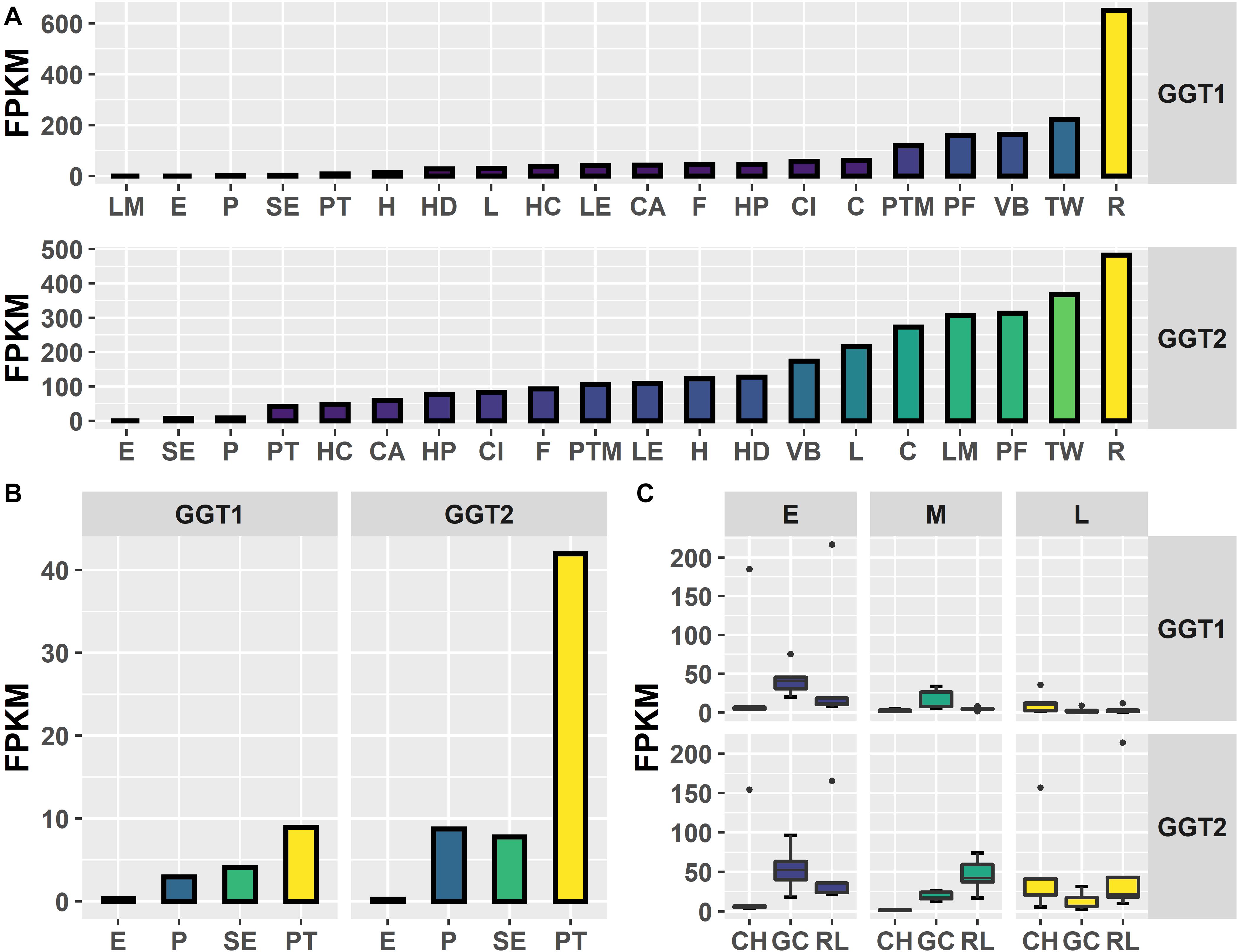
Figure 2. Expression of JrGGT1 and JrGGT2. (A) Expression by tissue type of three independent biological replicates pooled together. LM, leaf mature; E, embryo; P, pellicle; SE, somatic embryo; PT, packing tissue; H, hull; HD, hull dehiscing; L, leaf; HC, hull cortex; LE, leaf early; CA, callus exterior; F, fruit; HP, hull peel; CI, callus interior; C, catkin; PTM, packing tissue mature; PF, pistillate flower; VB, vegetative bud; TW, transition wood; R, root. Note that tissue type is ordered by expression level. (B) Scaled view of E, P, SE, and PT from (A). (C) Expression by genotype and stage. CH, Chandler; GC, Gayle’s Caramel; RL, Robert Livermore; E, early; M, middle; L, late; FPKM, fragments per kilobase of transcript per million mapped reads. Each box and whiskers (genotype/stage) consists of five independent biological replicates.
Transcripts Correlated With JrGGT1
In T20 and Tp RNA-seq data sets, JrGGT1 positively correlated with enzymes in the shikimic acid pathway, deoxy-D-arabinoheptulosonate 7-phosphate synthase (JrDAHPS) (NCBI 109007614 and 109007613), and shikimate dehydrogenase (JrSkDH) (NCBI 109011053 and 109011052) (Figure 1 and Table 3). Both Tp and T20 correlation analyses identified the same JrDAHPSs and JrSkDHs (Table 3). Genes of the phenylpropanoid pathway also correlated with JrGGT expression. JrGGT1 expression mirrored RNAs encoding phenylalanine ammonia-lyase (JrPAL) (NCBI 108997102), bifunctional aspartate aminotransferase and glutamate/aspartate–prephenate aminotransferase (JrPAT) (NCBI 109013250), cinnamic acid-4-hydroxylase (JrCA4H) (NCBI 108996955), and caffeic acid 3-O-methyltransferase (JrCMT) (NCBI 109007527 and 109013104). The JrCMTs did not correlate with JrGGT1 in T20 (Table 3). JrGGT1 displayed robust correlations only in the Tp data with expression of leucoanthocyanidin reductase (JrLAR) (NCBI 108994581), flavanone 3-hydroxylase (JrF3H) (NCBI 108997708), chalcone synthase (JrCHS) (NCBI 108995889), dihydroflavonol-4-reductase (JrDFR) (NCBI 108991381), and anthocyanidin synthase (JrANS) (NCBI 109010746) of the flavonoid biosynthetic pathway (Figure 1 and Table 4).
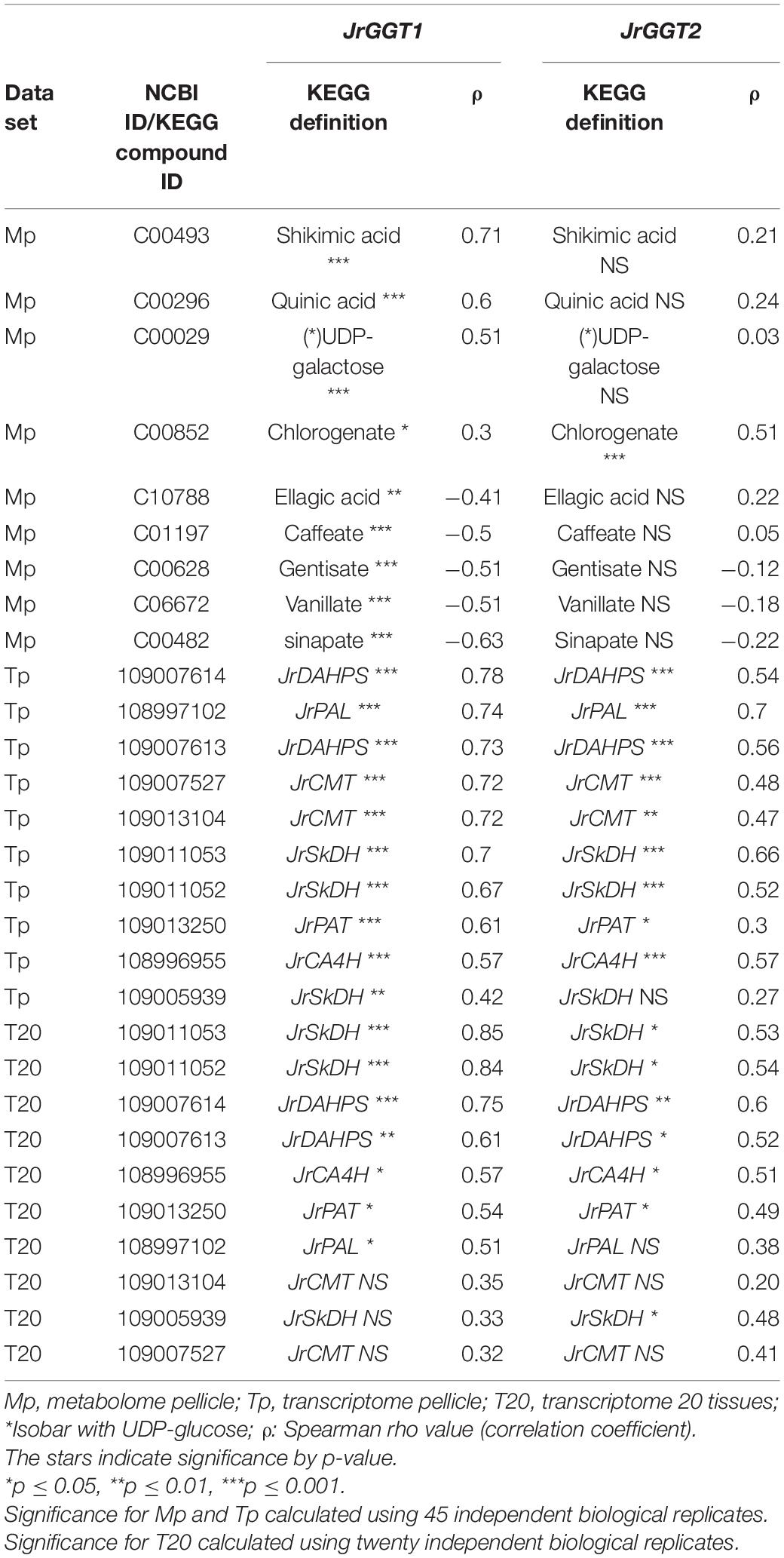
Table 3. Selected RNAs and metabolites related to HBA/HCA UGT activity from Spearman feature correlation of JrGGT1 and JrGGT2.
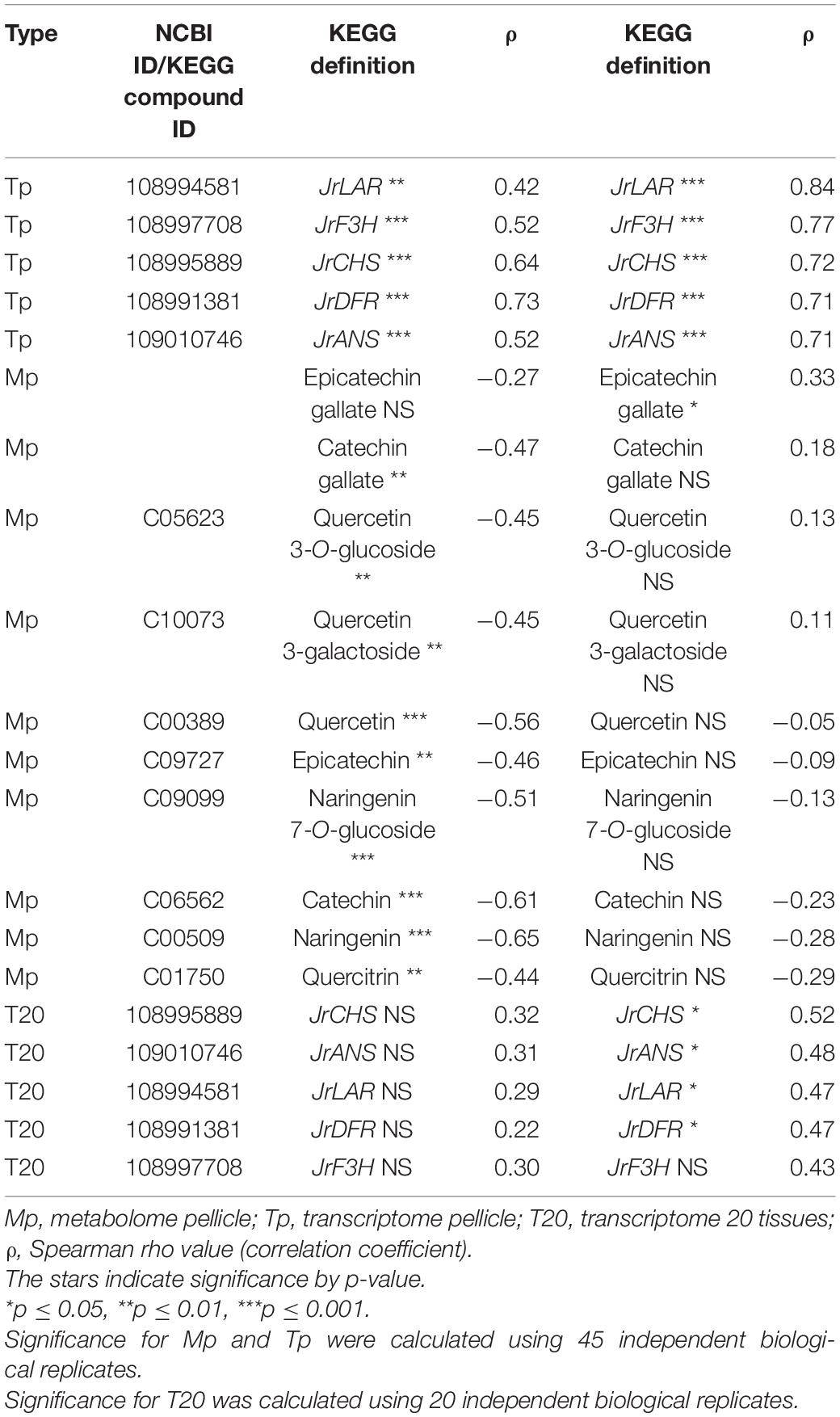
Table 4. Selected RNAs and metabolites related to flavonoid UGT activity from Spearman feature correlation of JrGGT1 and JrGGT2.
Metabolites Correlated With JrGGT1
1-O-Galloyl-β-D-glucose was not detected in Mp, despite being in the compound library. Several phenolic metabolites and precursors involved in HBA/HCA UGT activity correlated strongly with JrGGT1 expression. Shikimate, QA, UDP-galactose (isobar with UDPG), and chlorogenate correlated positively with JrGGT1 (Table 3). Many phenolic acids correlated negatively with JrGGT1, including HBAs like vanillate, gentisate, and the HCAs caffeic acid and sinapinic acid. The ellagitannin by-product, ellagic acid, also correlated negatively with JrGGT1. Surprisingly, the correlation between GA and JrGGT1 was negative but not significant. This pattern continued into the flavonoids, where JrGGT1 expression correlated negatively with many flavonoid aglycones and glycones (Table 4). JrGGT1 did not correlate with epicatechin gallate.
Transcripts Correlated With JrGGT2
JrGGT2 correlated less with the shikimic acid pathway enzymes JrDAHPSs and JrSkDHs than JrGGT1 in Tp and T20 (Table 3). The phenylpropanoid pathway enzymes JrPAL, JrPAT, JrCA4H, and JrCMTs correlated well with JrGGT2 expression but weaker than JrGGT1. JrPAL and the two JrCMTs did not correlate with JrGGT2 expression in T20. In Tp and T20, JrGGT2 correlated better than JrGGT1 with JrCHS, JrF3H, JrDFR, JrANS, and JrLAR of flavonoid biosynthesis. All correlations in flavonoid biosynthetic genes were weaker in T20 than in Tp.
Metabolites Correlated With JrGGT2
A superior correlation was seen between JrGGT2 and chlorogenate than with JrGGT1. However, JrGGT2 did not correlate with shikimic acid, QA, or UDP-galactose. Moreover, unlike JrGGT1, no significant association was seen between JrGGT2 expression and any of the HBAs, HCAs, and flavonoids, except for a positive correlation with epicatechin gallate (Table 4).
Expression of JrGGTs in Tobacco Plants Altered Metabolites Responsible for HBA, HCA, and Flavonoid Biosynthesis
We used an anti-his tag antibody for transgenic tobacco screening to select two highest-expressing lines: one for JrGGT1 and one for JrGGT2 (Figure 3). Seeds from these T0 plants and untransformed tobacco were germinated on selective and non-selective media, respectively, to create plants grown in the greenhouse. Twelve seedlings, each from untransformed tobacco (UT; grown without kanamycin) and from tobacco plants overexpressing JrGGT1 and JrGGT2, were allowed to grow 4 weeks. Then, leaf tissue was harvested for metabolite and amino acid analysis.
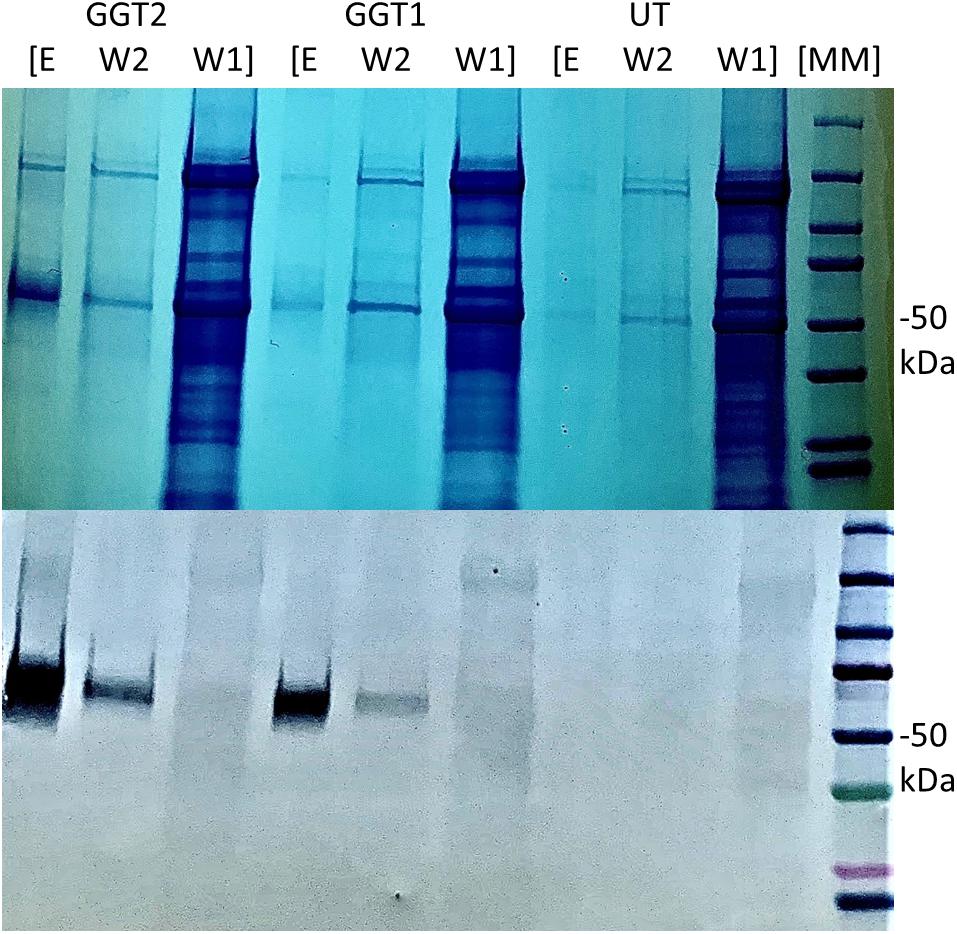
Figure 3. Expression of recombinant JrGGT1 and JrGGT2 protein in tobacco leaf tissue. Two gels for SDS-PAGE (top) and Western blot (bottom) were run in tandem and the same samples were used for both. “E, W2, and W1” stand for elution, wash two, and wash one, respectively, from the His-purification process. UT served as an untransformed control. Molecular weight marker (MM) of 50 kDa is denoted by “–50 kDa” on the right side of the figure. Note that the elution step was concentrated using a centrifugal filter and, thus, is an inflated representation of protein expression compared to the other steps of the His-purification process.
Transgenic expression of JrGGTs in tobacco produced similar metabolic profiles compared to UT. In principal component analysis scores, tobacco lines expressing JrGGT1 and JrGGT2 clustered in the same direction and space compared to UT, but with different magnitudes. This result indicates they had similar global metabolomes (Figure 4). JrGGT1-expressing samples separated well from UT samples. JrGGT2-expressing tobacco lines did not separate well from UT samples or JrGGT1 samples. Partial least-square-discriminant analysis (PLS-DA) cross-validation performance measures Q2 and accuracy were lower in JrGGT2-expressing lines than in JrGGT1-expressing tobacco lines. This finding indicates that JrGGT2-expressing lines were not as different from UT as JrGGT1-expressing lines (Supplementary Figure 3). Compared to UT lines, PLS-DA resulted in 41 metabolites increased and 23 metabolites decreased in JrGGT1-expressing tobacco. In JrGGT2-expressing lines, this analysis resulted in 44 metabolites increased and 18 metabolites decreased (Table 5). 1-O-Galloyl-β-D-glucose was not detected in this study, despite being in the compound library.
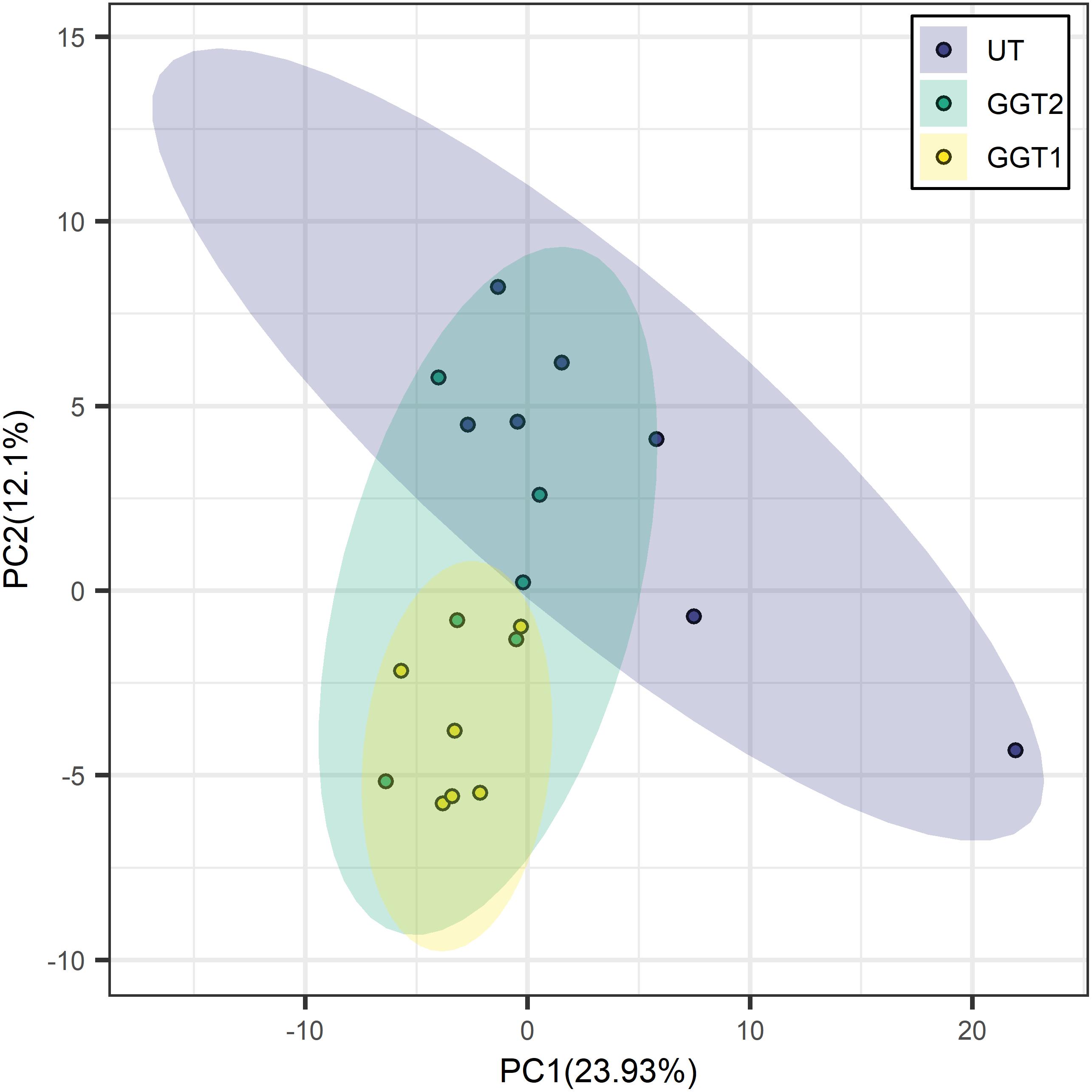
Figure 4. Principal component analysis score clustering of JrGGT1- and JrGGT2-expressing and UT tobacco lines by GC-MS metabolomics. UT, untransformed. The circles surrounding each class represent 95% confidence intervals. Six independent biological replicates were used for JrGGT1- and JrGGT2-expressing lines, while seven were used for UT lines.
JrGGT1-Expressing Lines vs. UT Lines
JrGGT1 tobacco lines had 22% more shikimic acid and 25% more UDP-galactose than UT lines. Only JrGGT1-expressing tobacco lines were enriched in chlorogenic acid by 59%. QA was not affected. All differentially expressed HBAs detected were reduced in JrGGT-expressing tobacco lines. The HBA plant hormone salicylic acid (SA), the methoxybenzoic acid p-anisic acid, 3,4-dihydroxybenzoic acid (PCA), and GA were reduced by 18%, 19%, 21%, and 31%, respectively, in JrGGT1-expressing tobacco lines (Table 6 and Figure 5A). Expectedly, benzoic acid remained unchanged. Only JrGGT1-expressing tobacco lines had less 4-HBA (p-hydroxybenzoic acid), by 10%. There were no differences in levels of 4-HCA or cis-caffeic acid. JrGGT1-expressing tobacco lines had 45% more of the sinapinic acid precursor ferulic acid and 24% less sinapinic acid (Figure 5B). Neither line showed significant changes in the HCA cis-caffeic acid. Catechin was reduced only in JrGGT1-expressing tobacco lines, by 42%. Levels of a flavonoid glycone, 4′,5-dihydroxy-7-glucosyloxyflavanone (prunin), were not changed by JrGGT1 expression. Only JrGGT1-expressing tobacco lines had significantly reduced tyrosine in GC-MS analysis, by 17%. Amino acid analysis of JrGGT1-expressing tobacco lines also showed that only tyrosine was reduced by 23% (Figure 5C). Phenylalanine was depleted in JrGGT1-expressing tobacco lines by 27% and ammonia reduced by 30%.
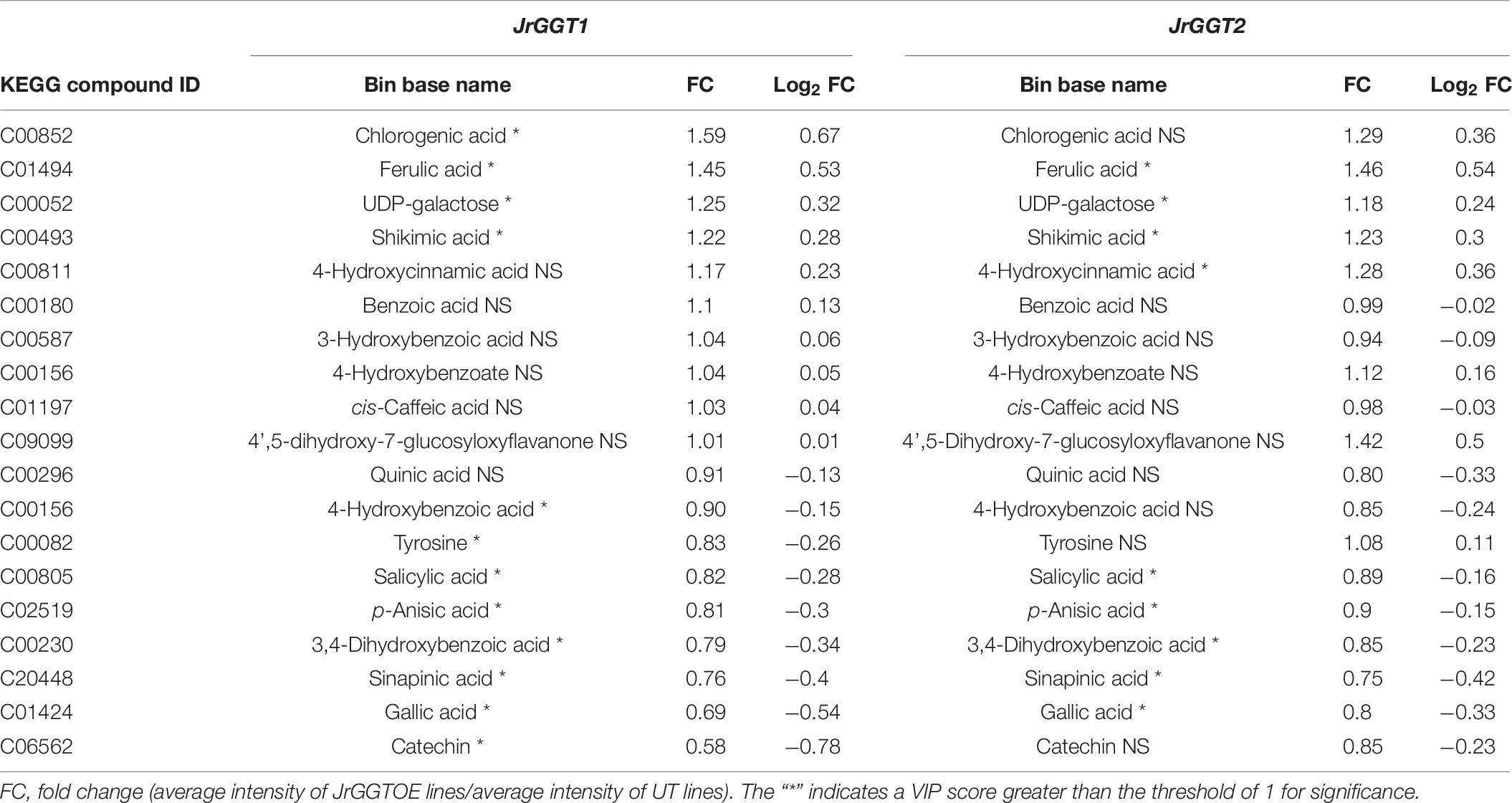
Table 6. Selected metabolites related to HBA/HCA UGT activity from GC-TOF-MS analysis of JrGGT1- and JrGGT2-expressing tobacco lines.
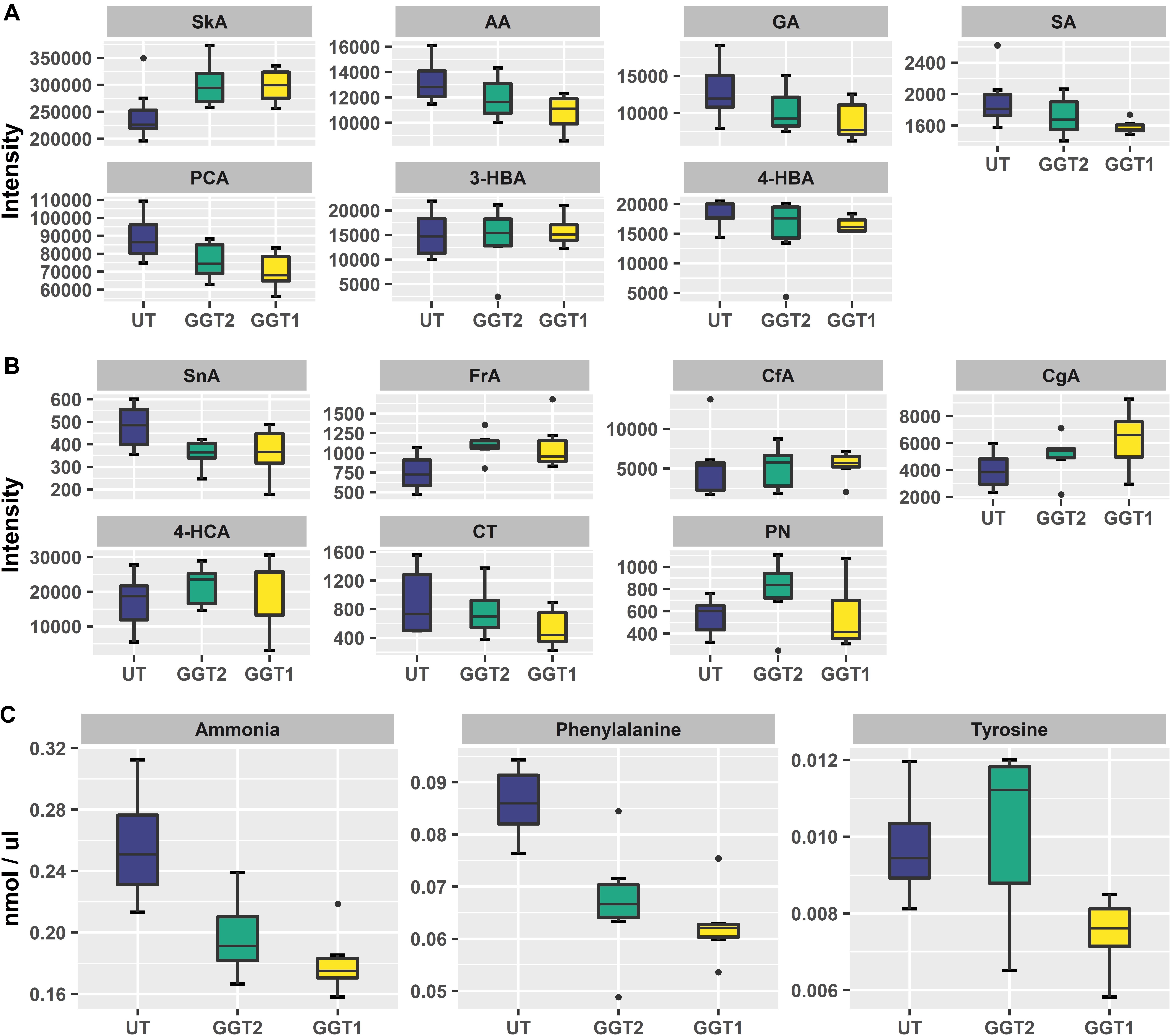
Figure 5. Boxplots of relevant HBAs, HCAs, flavonoids, and amino acids. (A) SkA, shikimic acid; AA, p-anisic acid; GA, gallic acid; SA, salicylic acid; PCA, protocatechuic acid; 3-HBA, 3-hydroxybenzoic acid; 4-HBA, 4-hydroxybenzoic acid. (B) SnA, sinapinic acid; FrA, ferulic acid; CfA, cis-caffeic acid; CgA, chlorogenic acid; 4-HCA, 4-hydroxycinnamic acid; CT, catechin; PN, prunin. Six independent biological replicates were used for JrGGT1- and JrGGT2-expressing lines, while seven were used for UT lines. (C) Boxplots of ammonia, phenylalanine, and tyrosine from free amino acid analysis of six independent biological replicates each for JrGGT1- and JrGGT2-expressing lines and UT lines.
JrGGT2-Expressing Lines vs. UT Lines
Similar to JrGGT1-expressing tobacco lines, JrGGT2-expressing lines had 23% more shikimic acid and 18% more UDP-galactose. QA was also unchanged. SA, p-anisic acid, PCA, and GA were also reduced by 11%, 10%, 15%, and 20%, respectively, in JrGGT2-expressing tobacco lines. Benzoic acid was also not affected. JrGGT2-expressing lines showed increases in 4-HCA by 28%, while cis-caffeic acid remained unchanged. Like JrGGT1-expressing lines, JrGGT2 expression increased ferulic by 48%, while sinapinic acid decreased by 25%. Catechin remained unchanged; however, JrGGT2-expressing lines had 42% more prunin, with a variable importance in projection (VIP) score just below the significance threshold (Figure 5B). JrGGT2 expression also reduced the amino acid phenylalanine by 22% and ammonia by 27%.
Discussion
By the convention of the UDP-glycosyltransferase (UGT) Nomenclature Committee (UNC), JrGGT1 and JrGGT2 belong to the UGT84A class of enzymes: HBA, HCA, and flavonoid UGTs that derive from the shikimic acid and phenylpropanoid pathways (Lunkenbein et al., 2006; Khater et al., 2012; Mittasch et al., 2014; Cui et al., 2016; Ono et al., 2016). Sequence identity shared by the JrGGTs and all functionally characterized UGT84As (Table 1) vastly exceeds 60%, as stated by UNC’s nomenclature guidelines (Mackenzie et al., 2005). Moreover, JrGGT1, and to a lesser extent, JrGGT2, coexpress tightly with a suite of genes and metabolites of the shikimic acid and phenylpropanoid pathways required for biosynthesis of HBAs, HCAs, and flavonoids (Figure 1 and Tables 3, 4). Non-coincidentally, JrGGT expression in tobacco created similar patterns in shikimic acid and phenylpropanoid metabolites. The two transgenic groups produced comparable phenolic and global metabolic profiles (Figures 4, 5 and Tables 6, 7). Together, the genomic, transcriptomic, and metabolomic evidence presented here suggests that JrGGT1 and JrGGT2 play important roles in the metabolism of HBAs, HCAs, and flavonoids.
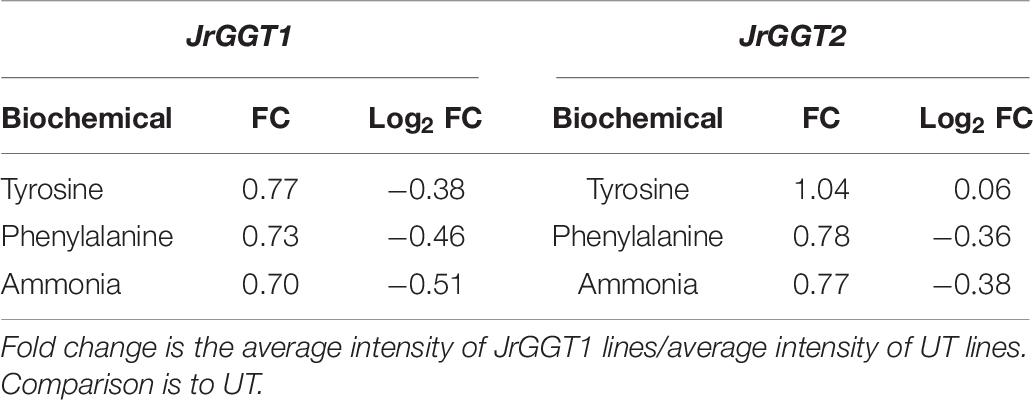
Table 7. Tyrosine, phenylalanine, and ammonia from free amino acid analysis of JrGGT1- and JrGGT2-expressing SR1 lines.
As the UGT84As tend to show high affinity toward GA and PCA in vitro, it was not surprising to see that JrGGT1 and JrGGT2 positively correlated with the JrDAHPSs and JrSkDHs in vivo (Khater et al., 2012; Mittasch et al., 2014; Ono et al., 2016) (Figure 1 and Table 3). JrGGT1, in particular, showed robust positive correlations with two JrDAHPSs, while JrGGT2’s correlations with these genes were weaker. JrGGT2 also did not correlate as strongly with the JrSkDHs (Table 3). Perhaps these results indicate that the two JrGGTs have different biochemical functions. The unique relationship between the JrGGTs, JrDAHPSs, and JrSkDHs in Table 3 may indicate they evolved to work together, as many JrDAHPSs and JrSkDHs did not correlate with the JrGGTs. Both JrGGTs’ correlations with JrSkDH (NCBI 109005939) were far weaker than that of the JrSkDHs (NCBI 109011053 and 109011052). This finding was intriguing, given that JrSkDH (NCBI 109005939) was described as responsible for GA biosynthesis in Juglans regia (Muir et al., 2011). The strong positive correlations between JrGGT1 expression, shikimic acid, and QA corroborate the relationships between JrGGT1, the JrDAHPSs, the JrSkDHs, and thus, the shikimic acid trunk (Figure 1 and Table 3). The lack of correlations between JrGGT2 expression, shikimic acid, and QA is somewhat consistent with this gene’s weaker association with the JrDAHPS variants and the JrSkDHs compared to JrGGT1. Despite the correlation results, JrGGT2-expressing tobacco lines accumulated more shikimic acid than UT (Table 6). JrGGT1-expressing tobacco lines also accumulated more shikimic acid, contributing to our hypothesis that these genes are involved in shikimic acid metabolism (Figure 5A and Table 6). These findings were interesting, given that we predicted that JrGGT overexpression would deplete free shikimic acid. In a similar case of confusion, SkDH silencing in tobacco increased concentrations of the substrate (3-DHQ) and its product, shikimic acid (Ding et al., 2007). Since the SkDHs are NADP+/(H) dependent, it is possible that altered metabolism along the shikimic acid pathway could shift the NADP+: NADPH ratio near the enzyme. Redox homeostasis could regulate the shikimic acid concentration, with NADP+ favoring oxidation to GA and NADPH favoring reduction to shikimic acid (Figure 1). Similarly, cofactor balance may necessitate that any additional carbon flowing toward the GA pathway would require a commensurate amount of carbon to flux toward shikimic acid biosynthesis. The generation of GA can result in NADPH production (Figure 1), which may then be used to facilitate reduction of shikimic acid and subsequent generation of NADP+, which can again drive the oxidation of 3-DHS to GA, forming a small cycle. Put shortly, GA and shikimic acid biosynthesis may be inexorably linked, as suggested previously (Muir et al., 2011). Similar to the shikimic acid results, JrGGT1 correlated strongly with UDP-galactose, and its expression in tobacco resulted in accumulation of UDP-galactose, indicating that it is also involved in UDP sugar metabolism (Table 3). JrGGT2 expression did not correlate with UDP-galactose, yet JrGGT2-expressing lines accumulated more UDP-galactose than UT. Perhaps JrGGT2 is less active than JrGGT1.
Evidence that JrGGT1 regulates HBAs was provided by the negative correlation between JrGGT1 expression and the HBAs vanillate and gentisate (Table 3). This negative relationship between enzyme and substrate is sensible if JrGGT1 is responsible for glycosylation of vanillate and gentisate. Increased expression of HBA-glycosylating enzymes (JrGGT1) would reduce aglycones of vanillate and gentisate, while decreased expression of HBA-glycosylating enzymes would favor their increase. Echoing JrGGT2’s effects in tobacco, JrGGT2 expression also correlated negatively with vanillate and gentisate. However, this relationship was not significant (Figure 5A and Tables 3, 6). Indeed, JrGGT orthologs have high substrate affinity with vanillate (Lunkenbein et al., 2006; Mittasch et al., 2014). Vanillate could be a preferred HBA for JrGGT1 in pellicle tissue. Surprisingly, JrGGT expression did not correlate significantly with GA in pellicle metabolite data, despite GA being a famous metabolite in the field of UGT84A research. This finding may indicate that GA is not a principal substrate for the JrGGTs in natura. JrGGT overexpression in tobacco either reduced or did not significantly change the relative abundance of all HBAs, supporting the correlations with HBAs in Mp (Figure 5A and Table 6). The most significantly reduced HBAs in both lines were GA, PCA, and the plant hormone SA. Surprisingly, the effects of JrGGT expression on GA were inconsistent with the correlation data, given that GA did not correlate with JrGGT expression. Perhaps this result was somehow confounded by JrGGT expression in tobacco and not walnut. Also reduced was 4-HBA, but this was only significant in JrGGT1-expressing lines. In both lines, the order of magnitude of reduction was GA>PCA>SA>4-HBA (Table 6). Intriguingly, this echoes similarity with the order-specific activity of VvGT1-3 for PCA and 4-HBA, but not that of UGT84A23-24, and suggests a preference for GA over PCA, and for PCA over SA and 4-HBA in vivo when expressed in tobacco (Khater et al., 2012; Ono et al., 2016).
Both JrGGT1 and JrGGT2 expression mirrored that of JrPAL (Table 3). Coupled with this observation is the fact that JrGGT1-expressing tobacco lines showed reductions in tyrosine, phenylalanine, and ammonia, respectively, while in JrGGT2-expressing tobacco lines, only phenylalanine and ammonia were reduced (Figure 5C and Table 7). This finding suggests that the JrGGTs are intimately connected with the PAL reaction. Both JrGGTs correlated strongly with JrCA4H and JrCMT RNAs in Tp data (Table 3). However, these relationships tended to be weaker in JrGGT2 than in JrGGT1. JrGGT1 also correlated negatively with the HCAs caffeate and sinapate (also sinapinic acid), while this relationship with JrGGT2 was not significant (Table 3). Associations with JrGGT expression and genes and metabolites of the phenylpropanoid pathway were verified to be causal in metabolomics of JrGGT-expressing tobacco. 4-HCA and ferulic acid were enriched, caffeic acid was unchanged, and sinapinic acid was reduced in JrGGT1- and JrGGT2-expressing tobacco lines (Figure 5B and Table 6). The reductions in sinapinic acid suggest that it may be the preferred HCA for JrGGT1 and JrGGT2 activity in vivo. Indeed, sinapinic acid concentration and JrGGT1 expression also had a negative relationship in Mp data (Table 3). Moreover, sinapinic acid was glycosylated by UGTs from strawberry, pomegranate, tea, and grape (Lunkenbein et al., 2006; Khater et al., 2012; Cui et al., 2016; Ono et al., 2016). The reductions in preceding metabolites phenylalanine and ammonia, increases in 4-HCA and ferulic acid, and reductions in sinapinic acid could indicate some form of feedback regulation at PAL, as in the phenylpropanoid pathway (Blount et al., 2000).
In contrast to the shikimic acid and phenylpropanoid pathways, JrGGT2 correlated better with genes and metabolites of flavonoid metabolism than JrGGT1. Both JrGGTs displayed similar correlations with JrCHS and JrDFR in Tp; however, this similarity diminished in T20, with JrGGT2 alone retaining significance (Table 4). The relationships between JrF3H, JrLAR, JrANS, and JrGGT2 were highly robust in Tp data, while that of JrGGT1 was less compelling. This difference may indicate that JrGGT2 is more involved in the metabolism of flavanols, leucoanthocyanidins, flavan-3-ols, and anthocyanidins. Indeed, JrGGT2 showed a positive relationship with the flavan-3-ol epicatechin gallate, while JrGGT1 showed no correlation with these flavonoids (Table 4). Biosynthesis of epicatechin gallate in tea requires the HBA glucoside 1-O-galloyl-β-D-glucose to donate a galloyl moiety in a galloyltransferase reaction (Liu et al., 2012). JrGGT2 may be involved in supporting the available pool of 1-O-galloyl-β-D-glucose required to synthesize epicatechin gallate. Moreover, JrGGT2-expressing, but not JrGGT1-expressing, tobacco lines had 42% more of the flavanone glucoside prunin, with a VIP score just below the threshold (Table 6). Similarly, only JrGGT2-expressing tobacco lines had 28% more of the flavonoid precursor 4-HCA (Table 6). Perhaps this was to provide more carbon to serve the pathway through CHS. Alternatively, it was JrGGT1-expressing and not JrGGT2-expressing tobacco lines that were reduced in the flavan-3-ol catechin (Table 6). The reduction of catechin in JrGGT2-expressing tobacco lines was not significant, which is curious as JrGGT2 correlated strongly with JrLAR in the Tp and T20 data sets, while JrGGT1 was not so convincing (Table 4). Confirming this observation was that the catechin fluctuations correlated negatively with JrGGT1 expression, and not with JrGGT2 expression.
Conclusion
Experimental evidence from genomic, transcriptomic, and metabolomic perspectives suggests the JrGGTs are UDP84A-type glycosyltransferases and are involved in shikimic acid and phenylpropanoid metabolism of phenolic acids and flavonoids. While JrGGT1 and JrGGT2 share high sequence similarity at the nucleotide and amino acid levels, their correlations within plant secondary metabolism and gene expression appear different. Considering the evidence provided by Tp, Mp, T20, and JrGGT overexpressing tobacco lines, JrGGT1 seems more involved in the metabolism of HBAs, HCAs, and some flavonoids than JrGGT2. Alternatively, these results suggest that JrGGT2 may be more involved in the metabolism of specific flavonoids. Further adding to this point are the differential expression profiles of JrGGT1 and JrGGT2 in the T20 expression data.
This study focused on the smaller molecular weight compounds detectable by GC-MS methods and deepened our understanding of JrGGT1 and JrGGT2 and their unique roles in plant secondary metabolism. However, only one phenol glycoside was detected in GC-MS profiling of JrGGT-expressing tobacco. Due to their greater molecular weight, tannins and phenolic glycosides are typically not detectable using a GC-MS platform. However, 1-O-galloyl-β-D-glucose was detectable yet not observed in the GC-MS tobacco metabolomics. Interestingly, the Mp data generated using a combination of LC-MS and GC-MS techniques also detected no HBA glycosides, despite 1-O-galloyl-β-D-glucose being detectable. Therefore, an untargeted LC-MS approach focused on polyphenolics may shed light on the potential effects of JrGGT expression on the HTs, tea tannins, and phenolic glycosides in vivo. Moreover, the GC-MS tobacco data lacked an empty vector control. These results would be strengthened by adding a PDH17 vector lacking JrGGT CDS to provide additional confidence that these effects were not due to transgene insertion.
Data Availability Statement
Raw metabolite data for of JrGGT1 and JrGGT2 overexpressing tobacco were deposited at https://www.ebi.ac.uk/metabolights/search as study MTBLS2042. The raw reads for the RNA-seq analysis of walnut pellicle tissue were deposited to the Sequence Read Archive, https://www.ncbi.nlm.nih.gov/sra, under the BioProject ID: PRJNA663110. Detailed information on the walnut pellicle data can be found in a dissertation (Butterfield, 2017).
Author Contributions
HS and AD conceived and designed the experiments. HS coordinated and performed the experiments and functional analysis, and wrote the manuscript, which was then edited by TB, TH, NF, BB, and AD. TH performed cloning experiments. TB and BB acquired and processed pellicle RNA-seq and metabolite data. NF and CZ performed the experiments and helped with data collection. AJ maintained plant material. AD and all other authors revised the final manuscript. All authors contributed to the article and approved the submitted version.
Funding
This work was funded by grants received from the California Walnut Board. HS acknowledges receipt of a Henry A. Jastro Research Scholarship Award.
Conflict of Interest
The authors declare that the research was conducted in the absence of any commercial or financial relationships that could be construed as a potential conflict of interest.
Acknowledgments
We thank Emilio A. Laca for helping improve the author’s understanding of the statistical analyses used in this study.
Supplementary Material
The Supplementary Material for this article can be found online at: https://www.frontiersin.org/articles/10.3389/fpls.2021.626483/full#supplementary-material
Supplementary Figure 1 | Schematic of binary vectors pDH17.0301 and pDH17.0401, used to express JrGGT1 and JrGGT2, respectively, in transgenic Nicotiana tabacum.
Supplementary Figure 2 | Schematic of bioinformatics of RNA-seq data.
Supplementary Figure 3 | Cross-validation of (A) JrGGT1 and (B) JrGGT2 PLS-DA, displaying three model performance measures: the sum of squares captured by the model (R2), the cross-validated R2 (also known as Q2), and the prediction accuracy (Accuracy). The default criterion is Q2 (marked by a red star), which indicates the optimal number of components to use during modeling.
Supplementary Table 1 | Primers used in cloning and vector construction.
Supplementary Table 2 | Expression of JrGGT1 and JrGGT2 across 20 tissues in fragments per kilobase per million mapped reads (FPKM) of walnut.
Abbreviations
3-DHQ, 3-dehydroquinate; 3-DHS, 3-dehydroshikimate; AA, p-anisic acid; ADT, arogenate dehydratase/prephenate dehydratase; ANS, leucoanthocyanidin dioxygenase, anthocyanidin synthase; CA4H, cinnamic acid-4-hydroxylase; CHS, chalcone synthase; CMT, caffeic acid 3-O-methyltransferase; CS, chorismate synthase; CSE, caffeoylshikimate esterase; DAHPS, 3-deoxy-D-arabinoheptulosonate 7-phosphate synthase; DFR, dihydroflavonol-4-reductase; DHQS, dehydroquinate synthase; E-4-P, erythrose-4-phosphate; F3H, naringenin2-oxoglutarate 3-dioxygenase flavanone 3-hydroxylase; F5H, ferulate-5-hydroxylase; GA, gallic acid; GGT, galloyl glycosyltransferase; HBA, hydroxybenzoic acid; HCA, hydroxycinnamic acid; HCT, hydroxycinnamoyl-CoA: shikimate/quinate hydroxycinnamoyl transferase; LAR, leucoanthocyanidin reductase; Mp, metabolome pellicle; PAL, phenylalanine ammonia lyase; PAT, bifunctional aspartate aminotransferase and glutamate/aspartate-prephenate aminotransferase; PCA, protocatechuic acid, 3,4-dihydroxybenzoic acid; PEP, phosphoenolpyruvate; QA, quinic acid; SA, salicylic acid; SkA, shikimic acid; SkDH, bifunctional dehydroquinate dehydratase/shikimate dehydrogenase; T20, transcriptome 20 tissues; Tp, transcriptome pellicle; UDPG, UDP-glucose; UGT, uridine diphosphate glycosyltransferase; UNC, UGT Nomenclature Committee.
Footnotes
- ^ https://ptf.ucdavis.edu/
- ^ http://www.bioinformatics.babraham.ac.uk/projects/fastqc/
- ^ http://www.arb-silva.de
- ^ ftp://ftp.ncbi.nlm.nih.gov/genomes/all/GCF/001/411/555/GCF_001411555.1_wgs.5d/GCF_001411555.1_wgs.5d_genomic.fna.gz
References
Abbas, M., Saeed, F., Anjum, F. M., Afzaal, M., Tufail, T., Bashir, M. S., et al. (2017). Natural polyphenols: an overview. Int. J. Food Prop. 20, 1689–1699. doi: 10.1080/10942912.2016.1220393
Blount, J. W., Korth, K. L., Masoud, S. A., Rasmussen, S., Lamb, C., and Dixon, R. A. (2000). Altering expression of cinnamic acid 4-hydroxylase in transgenic plants provides evidence for a feedback loop at the entry point into the phenylpropanoid pathway. Plant Physiol. 122, 107–116. doi: 10.1104/pp.122.1.107
Bontpart, T., Marlin, T., Vialet, S., Guiraud, J., Pinasseau, L., Meudec, E., et al. (2016). Two shikimate dehydrogenases, VvSDH3 and VvSDH4, are involved in gallic acid biosynthesis in grapevine. J. Exp. Bot. 67, 3537–3550. doi: 10.1093/jxb/erw184
Butterfield, T. S. (2017). Identifying Genetic Determinants of Pellicle Pigmentation in Juglans regia. Ph. D. Dissertation, University of California, Davis, Davis, CA.
Caputi, L., Malnoy, M., Goremykin, V., Nikiforova, S., and Martens, S. (2012). A genome-wide phylogenetic reconstruction of family 1 UDP- glycosyltransferases revealed the expansion of the family during the adaptation of plants to life on land. Plant J. 69, 1030–1042. doi: 10.1111/j.1365-313X.2011.04853.x
Chakraborty, S., Britton, M., Martínez-García, P. J., and Dandekar, A. M. (2016). Deep RNA-Seq profile reveals biodiversity, plant–microbe interactions and a large family of NBS-LRR resistance genes in walnut (Juglans regia) tissues. AMB Express 6, 1–13. doi: 10.1186/s13568-016-0182-3
Chen, H. C., Li, Q., Shuford, C. M., Liu, J., Muddiman, D. C., Sederoff, R. R., et al. (2011). Membrane protein complexes catalyze both 4- and 3-hydroxylation of cinnamic acid derivatives in monolignol biosynthesis. Proc. Natl. Acad. Sci. U.S.A. 108, 21253–21258. doi: 10.1073/pnas.1116416109
Cui, L., Yao, S., Dai, X., Yin, Q., Liu, Y., Jiang, X., et al. (2016). Identification of UDP-glycosyltransferases involved in the biosynthesis of astringent taste compounds in tea (Camellia sinensis). J. Exp. Bot. 67, 2285–2297. doi: 10.1093/jxb/erw053
Deng, Y., and Lu, S. (2017). Biosynthesis and regulation of phenylpropanoids in plants. CRC Crit. Rev. Plant Sci. 36, 257–290. doi: 10.1080/07352689.2017.1402852
Ding, L., Hofius, D., Hajirezaei, M. R., Fernie, A. R., Börnke, F., and Sonnewald, U. (2007). Functional analysis of the essential bifunctional tobacco enzyme 3-dehydroquinate dehydratase/shikimate dehydrogenase in transgenic tobacco plants. J. Exp. Bot. 58, 2053–2067. doi: 10.1093/jxb/erm059
Gross, G. G. (2008). From lignins to tannins: forty years of enzyme studies on the biosynthesis of phenolic compounds. Phytochemistry 69, 3018–3031. doi: 10.1016/j.phytochem.2007.04.031
Grundhöfer, P., Niemetz, R., Schilling, G., and Gross, G. G. (2001). Biosynthesis and subcellular distribution of hydrolyzable tannins. Phytochemistry 57, 915–927. doi: 10.1016/S0031-9422(01)00099-1
Hacham, Y., Avraham, T., and Amir, R. (2002). The N-terminal region of Arabidopsis cystathionine γ-synthase plays an important regulatory role in methionine metabolism. Plant Physiol. 128, 454–462. doi: 10.1104/pp.010819
Khan, N., and Mukhtar, H. (2019). Tea polyphenols in promotion of human health. Nutrients 11, 1–39. doi: 10.3390/nu11010039
Khater, F., Fournand, D., Vialet, S., Meudec, E., Cheynier, V., and Terrier, N. (2012). Identification and functional characterization of cDNAs coding for hydroxybenzoate/hydroxycinnamate glucosyltransferases coexpressed with genes related to proanthocyanidin biosynthesis. J. Exp. Bot. 63, 1201–1214. doi: 10.1093/jxb/err340
Kim, D., Langmead, B., and Salzberg, S. L. (2015). HISAT: a fast spliced aligner with low memory requirements. Nat. Methods 12, 357–360. doi: 10.1038/nmeth.3317
Langmead, B., Trapnell, C., Pop, M., and Salzberg, S. L. (2009). Ultrafast and memory-efficient alignment of short DNA sequences to the human genome. Genome Biol. 10:R25. doi: 10.1186/gb-2009-10-3-r25
Liu, Y., Gao, L., Liu, L., Yang, Q., Lu, Z., Nie, Z., et al. (2012). Purification and characterization of a novel galloyltransferase involved in catechin galloylation in the tea plant (Camellia sinensis). J. Biol. Chem. 287, 44406–44417. doi: 10.1074/jbc.M112.403071
Lombard, V., Golaconda Ramulu, H., Drula, E., Coutinho, P. M., and Henrissat, B. (2014). The carbohydrate-active enzymes database (CAZy) in 2013. Nucleic Acids Res. 42, 490–495. doi: 10.1093/nar/gkt1178
Lunkenbein, S., Bellido, M. L., Aharoni, A., Salentijn, E. M. J., Kaldenhoff, R., Coiner, H. A., et al. (2006). Cinnamate metabolism in ripening fruit. Characterization of a UDP-glucose:Cinnamate Glucosyltransferase from strawberry. Plant Physiol. 140, 1047–1058. doi: 10.1104/pp.105.074955
Mackenzie, P. I., Bock, K. W., Burchell, B., Guillemette, C., Ikushiro, S., Iyanagi, T., et al. (2005). Nomenclature update for the mammalian UDP glycosyltransferase (UGT) gene superfamily. Pharmacogenet. Genomics 15, 677–685. doi: 10.1097/01.fpc.0000173483.13689.56
Maeda, H., and Dudareva, N. (2012). The shikimate pathway and aromatic amino acid biosynthesis in plants. Annu. Rev. Plant Biol. 63, 73–105. doi: 10.1146/annurev-arplant-042811-105439
Martin, M. (2011). CutAdapt removes adapter sequences from high-throughput sequencing reads. EMBnet. J. 17, 10–12. doi: 10.14806/ej.17.1.200
Martinez-Garcia, P. J., Paul, R., Butterfield, T. S., Britton, M. T., Reagan, R. L., Chakraborty, S., et al. (2016). The walnut (Juglans regia) genome sequence reveals diversity in genes coding for the biosynthesis of non-structural polyphenols. Plant J. 87, 507–532. doi: 10.1111/tpj.13207
Mclafferty, F. W., Stauffer, D. A., and Loh, S. Y. (1999). Unknown identification using reference mass spectra. Quality evaluation of databases. J. Am. Soc. Mass Spectrom 10, 1229–1240. doi: 10.1016/s1044-0305(99)00104-x
Michel, G., Roszak, A. W., Sauvé, V., Maclean, J., Matte, A., Coggins, J. R., et al. (2003). Structures of shikimate dehydrogenase AroE and its paralog YdiB: a common structural framework for different activities. J. Biol. Chem. 278, 19463–19472. doi: 10.1074/jbc.M300794200
Mittasch, J., Böttcher, C., Frolova, N., Bönn, M., and Milkowski, C. (2014). Identification of UGT84A13 as a candidate enzyme for the first committed step of gallotannin biosynthesis in pedunculate oak (Quercus robur). Phytochemistry 99, 44–51. doi: 10.1016/j.phytochem.2013.11.023
Muir, R. M., Iba, A. M., Joseph, J., Jemmis, E. D., and Dandekar, A. M. (2011). Mechanism of gallic acid biosynthesis in bacteria (Escherichia coli) and walnut (Juglans regia). Plant Mol. Biol. 75, 555–565. doi: 10.1007/s11103-011-9739-3
Nakayama, T., Takahashi, S., and Waki, T. (2019). Formation of flavonoid metabolons: functional significance of protein-protein interactions and impact on flavonoid chemodiversity. Front. Plant Sci. 10:821. doi: 10.3389/fpls.2019.00821
Ono, N. N., Qin, X., Wilson, A. E., Li, G., and Tian, L. (2016). Two UGT84 family glycosyltransferases catalyze a critical reaction of hydrolyzable tannin biosynthesis in pomegranate (Punica granatum). PLoS One 11:e0156319. doi: 10.1371/journal.pone.0156319
Sharma, A., Shahzad, B., Rehman, A., Bhardwaj, R., Landi, M., and Zheng, B. (2019). Response of phenylpropanoid pathway and the role of polyphenols in plants under abiotic stress. Molecules 24, 1–22. doi: 10.3390/molecules24132452
Shen, W. J., and Forde, B. G. (1989). Efficient transformation of Agrobacterium spp. by high voltage electroporation. Nucleic Acids Res. 17:8385. doi: 10.1093/nar/17.20.8385
Trapnell, C., Hendrickson, D. G., Sauvageau, M., Goff, L., Rinn, J. L., and Pachter, L. (2013). Differential analysis of gene regulation at transcript resolution with RNA-seq. Nat. Biotechnol. 31, 46–53. doi: 10.1038/nbt.2450
Urban, P., Werck-Reichhart, D., Teutsch, H. G., Durst, F., Regnier, S., Kazmaier, M., et al. (1994). Characterization of recombinant plant cinnamate 4-hydroxylase produced in yeast: kinetic and spectral properties of the major plant P450 of the phenylpropanoid pathway. Eur. J. Biochem. 222, 843–850. doi: 10.1111/j.1432-1033.1994.tb18931.x
Wang, M., Zhu, X., Wang, K., Lu, C., Luo, M., Shan, T., et al. (2018). A wheat caffeic acid 3-O-methyltransferase TaCOMT-3D positively contributes to both resistance to sharp eyespot disease and stem mechanical strength. Sci. Rep. 8:6543. doi: 10.1038/s41598-018-24884-0
Wang, X., Chao, N., Zhang, M., Jiang, X., and Gai, Y. (2019). Functional characteristics of Caffeoyl shikimate esterase in Larix kaempferi and monolignol biosynthesis in gymnosperms. Int. J. Mol. Sci. 20:6071. doi: 10.3390/ijms20236071
Widhalm, J. R., and Dudareva, N. (2015). A familiar ring to it: biosynthesis of plant benzoic acids. Mol. Plant 8, 83–97. doi: 10.1016/j.molp.2014.12.001
Keywords: glucosyltransferase, hydrolysable tannin, UGT84A, gallic acid, walnut, phenol metabolism, transcriptomics, metabolomics
Citation: Saxe HJ, Horibe T, Balan B, Butterfield TS, Feinberg NG, Zabaneh CM, Jacobson AE and Dandekar AM (2021) Two UGT84A Family Glycosyltransferases Regulate Phenol, Flavonoid, and Tannin Metabolism in Juglans regia (English Walnut). Front. Plant Sci. 12:626483. doi: 10.3389/fpls.2021.626483
Received: 06 November 2020; Accepted: 25 January 2021;
Published: 24 February 2021.
Edited by:
Giorgio Gambino, National Research Council (CNR), ItalyReviewed by:
Prashant Shidram Swamy, Washington State University, United StatesKamil Marcin Kostyn, University of Wrocław, Poland
Copyright © 2021 Saxe, Horibe, Balan, Butterfield, Feinberg, Zabaneh, Jacobson and Dandekar. This is an open-access article distributed under the terms of the Creative Commons Attribution License (CC BY). The use, distribution or reproduction in other forums is permitted, provided the original author(s) and the copyright owner(s) are credited and that the original publication in this journal is cited, in accordance with accepted academic practice. No use, distribution or reproduction is permitted which does not comply with these terms.
*Correspondence: Abhaya M. Dandekar, YW1kYW5kZWthckB1Y2RhdmlzLmVkdQ==