- 1Department of Biological Systems Engineering, University of Nebraska–Lincoln, Lincoln, NE, United States
- 2Department of Agronomy and Horticulture, University of Nebraska–Lincoln, Lincoln, NE, United States
Phenotyping plants is an essential component of any effort to develop new crop varieties. As plant breeders seek to increase crop productivity and produce more food for the future, the amount of phenotype information they require will also increase. Traditional plant phenotyping relying on manual measurement is laborious, time-consuming, error-prone, and costly. Plant phenotyping robots have emerged as a high-throughput technology to measure morphological, chemical and physiological properties of large number of plants. Several robotic systems have been developed to fulfill different phenotyping missions. In particular, robotic phenotyping has the potential to enable efficient monitoring of changes in plant traits over time in both controlled environments and in the field. The operation of these robots can be challenging as a result of the dynamic nature of plants and the agricultural environments. Here we discuss developments in phenotyping robots, and the challenges which have been overcome and others which remain outstanding. In addition, some perspective applications of the phenotyping robots are also presented. We optimistically anticipate that autonomous and robotic systems will make great leaps forward in the next 10 years to advance the plant phenotyping research into a new era.
Introduction: Robotic Technology Is Vital for High-Throughput Plant Phenotyping
Agriculture must produce enough food, feed, fiber, fuel, and fine chemicals in next century to meet the needs of a growing population worldwide. Agriculture will face multiple challenges to satisfy these growing human needs while at the same time dealing with the climate change, increased risk for drought and high temperatures, heavy rains, and degradation of arable land and depleting water resources. Plant breeders seek to address these challenges by developing high yielding and stress-tolerance crop varieties adapted to future climate conditions and resistant to new pests and diseases (Fischer, 2009; Furbank and Tester, 2011; Rahaman et al., 2015). However, the rate of crop productivity needs to be increased to meet projected future demands. Advances in DNA sequencing and genotyping technologies have relieved a major bottleneck in both marker assisted selection and genomic prediction assisted plant breeding, the determination of genetic information for newly developed plant varieties. Dense genetic marker information can aid in the efficiency and speed of the breeding process (Wang et al., 2018; Happ et al., 2019; Moeinizade et al., 2019). However, large and high quality plant phenotypic datasets are also necessary to dissect the genetic basis of quantitative traits which are related to growth, yield and adaptation to stresses (McMullen et al., 2009; Jannink et al., 2010; Phillips, 2010; Fahlgren et al., 2015; Tripodi et al., 2018; Chawade et al., 2019).
Plant phenotyping is the quantitative and qualitative assessment of the traits of a given plant or plant variety in a given environment. These traits include the biochemistry, physiology, morphology, structure, and performance of the plants at various organizational scales. Plant traits are determined by both genetic and environmental factors as well as non-additive interactions between the two. In addition, variation in one phenotypic trait (e.g., leaf characteristics) can result in variation in other plant traits (e.g., plant biomass or yield). Therefore, phenotyping large numbers of plant varieties for multiple traits across multiple environments is an essential task for plant breeders as they work to select desirable genotypes and identify genetic variants which provide optimal performance in diverse and changing target environments (Granier and Tardieu, 2009; Dhondt et al., 2013; Li et al., 2014; Foix et al., 2015; Walter et al., 2015; Costa et al., 2019; Pieruschka and Schurr, 2019).
Traditionally plant traits are quantified using manual and destructive sampling methods. These methods are usually labor-intensive, time-consuming, and costly. In addition, manual sampling and analysis protocols generally involve many steps requiring human intervention, with each step increasing the chances of introducing mistakes. Often the plant and its organ is cut at fixed time points or at particular phenological stages in order to measure its phenotypic traits. This method destroys or damages the plant at one time point, disallowing the temporal examination of the traits for individual plants during the growing season. For example, yield measurement (such as plant biomass and grain weight) is invasive and more labor intensive compare to the measurement of plant height and leaf chlorophyll content (measured by a handheld sensor). As a result of the labor and resource intensive nature of plant phenotyping, many plant breeders rely solely on a single measurement most critical to their efforts: yield. However, yield is considered as one of the most weakly inherited phenotypes in crop breeding (Richards et al., 2010; Furbank and Tester, 2011). The measurement of other traits in addition to yield can increase the accuracy with which yield can be predicted across diverse environments. Enabling high-throughput and non-destructive measurements of plant traits from large numbers of plants in multiple environments would therefore lead to increases in breeding efficiency (McMullen et al., 2009; Andrade-Sanchez et al., 2013; Fahlgren et al., 2015; Foix et al., 2018; Vijayarangan et al., 2018; Ge et al., 2019; Hassanijalilian et al., 2020a).
In recent years, high-throughput systems and workflows have been developed to monitor and measure large populations of plants rapidly in both greenhouse and field environments. These systems combine modern sensing and imaging modalities with the sensor deployment technologies (including conveyor belts, ground and aerial vehicles, and field gantries) to enable fast measurement and wide area coverage (Busemeyer et al., 2013; Ge et al., 2016; Virlet et al., 2017; Hassan et al., 2019). Although not fully autonomous, these systems represent the state of the art in modern plant phenotyping with several advantages over the traditional, manually collected phenotypic traits.
Robotic systems have been playing a more significant role in modern agriculture and considered as an integral part of precision agriculture or digital farming (Wolfert et al., 2017; Chlingaryan et al., 2018; Zhang et al., 2019; Hassanijalilian et al., 2020b; Jin et al., 2020; Pandey et al., 2021). The robots are fully autonomous and do not need experienced operators to accomplish farming tasks. This is the biggest advantage of the robots compared to tractor-based systems (White et al., 2012). Autonomous robots have taken over a wide range of farming operations including harvesting [Arad et al., 2020 (sweet pepper); Hemming et al., 2014 (sweet pepper); Lili et al., 2017 (tomato); van Henten et al., 2002 (cucumber); Hayashi et al., 2010; Xiong et al., 2020 (strawberry); Silwal et al., 2017 (apple)], pest and weed control [Raja et al., 2020 (tomato and lettuce); Oberti et al., 2016 (grape); Åstrand and Baerveldt, 2002 (sugar beet); Blasco et al., 2002 (lettuce)], spraying [Hejazipoor et al., 2021 (Anthurium); Gonzalez-de-Soto et al., 2016 (wheat); Adamides et al., 2017 (grape)], and pruning [Zahid et al., 2020 (apple); Chonnaparamutt et al., 2009; Ishigure et al., 2013 (cedar and hinko trees)]. Together with imaging and sensing, autonomous robotic systems are also deemed essential and integral parts for high-throughput plant phenotyping, as they will enhance substantially the capacity, speed, coverage, repeatability, and cost-effectiveness of plant trait measurements.
In this paper, we reviewed the latest development of robotic technologies in high-throughput plant phenotyping. We define the robotic technologies as a system having three components: (1) a sensing module that senses the target (plants or crops) and its environment, (2) a computational module to interpret the sensed information and form adaptive (or context-specific) decisions, and (3) an actuation module to complete certain desired operations (e.g., robotic probing, trait measurements, and navigation). For example, the robot makes decision based on the existing status of environment, obstacles, and plant geometry to manipulate a robotic arm to locate an imaging system with less occlusion and collision free close to plant organs, find appropriate target point on the leaf and control the end-effector based on the leaf angle for effective grasping, or accurately navigate the ground-based vehicles between crop rows. With this definition, systems like LemnaTec’s conveyor-based phenotyping platform (Fahlgren et al., 2015; Ge et al., 2016) was not considered in the review, because the plant movement usually follows a pre-defined schedule and no adaptive decision is made during phenotyping. Also not considered in this review are self-propelled ground vehicles or unmanned aerial vehicles (Bai et al., 2016; Han et al., 2018) that are merely used as a sensor deployment platform with no automated path planning or navigation.
Different artificial intelligence (AI) technologies such as deep learning, fuzzy logic, and genetic algorithms are actively used for control of the phenotyping robots. In recent years, deep learning techniques has gained increased interest to guide robotic manipulators and mobile platforms. In this regard, deep neural networks (DNNs) are commonly used to detect different objects in images such as crop rows, plant organs, soil, and obstacles. DNNs are typically operate directly on raw images and actively learn a variety of filter parameters during the training of a model (Pound et al., 2017; Irshat et al., 2018). Aguiar et al. (2020) presented a DNN models to detect the vine trunks as reliable features and landmarks to navigate a mobile robot in a vineyard. Parhar et al. (2018) used variation of Generative Adversarial Network (GAN) to detect the stalk of sorghum in the field and grasp it by a robotic manipulator.
There are three motivations behind writing this review paper. Firstly, robotic technologies in agriculture have seen rapid advancement recently with many emerging applications in plant phenotyping. A timely review of the literature is warranted to summarize the newest development in the field. Secondly, there is large and growing interest from the plant breeding and plant science communities in how these new technologies can be integrated into research and breeding programs to improve phenotyping throughput and capacity (Furbank and Tester, 2011; Fiorani and Schurr, 2013; Araus and Cairns, 2014). Thirdly, robotic phenotyping has advanced through cross-disciplinary collaborations between engineers and plant scientists. Outlining capabilities, goals and interests across these two very different disciplines may help readers to identify research gaps and challenges as well as provide insight into the future directions of the plant phenotyping robotic technologies.
Review: Many Indoor and Outdoor Robots Were Developed to Measure a Wide Range of Plant Traits
Phenotyping robotic systems have emerged to automate the phenotyping process in different aspects. The robotic manipulators and ground-based vehicles are used as platforms to attach different sensors to collect data rapidly and with higher repeatability. Robotic systems are deployed to collect and measure the human-defined phenotypic traits (such as plant height, and leaf area). Additionally, in some cases it is needed to collect repeated measurements of plant traits within large populations at several time points during a growing season. Robotic systems are highly desirable in this scenario as they provide the necessary speed and accuracy for this kind of phenotyping tasks.
Robotic platforms for plant phenotyping applications can be divided into two categories: those developed for indoor or controlled environments (greenhouse or laboratory), and those for outdoor environments (field) (Shafiekhani et al., 2017). In controlled environment, plants are either placed in a fixed position and the robot moves around the facility to interact with the plants, or the plants are moved by conveyor belts or other automated systems to a fixed location where the robot operates. Often the robotic system does not need to touch the plants. The robotic arm is equipped with RGB cameras or depth sensors [Time of Flight (TOF) cameras or 3D laser scanners] to acquire visible images or point cloud data. The morphological traits of the plants are then estimated from the reconstructed 3D model of the plants. Stem height and leaf length of corn seedlings were measured using a robotic arm at a fixed position and a TOF camera (Lu et al., 2017). Chaudhury et al. (2017) developed a gantry robot system consisted of a 3D laser scanner installed on the end-effector of a seven Degree of Freedom (DOF) robotic arm to compute the surface area and volume of Arabidopsis and barley. The settings of both robotic systems were unable to position the vision system to capture images from the leaves hidden by other leaves or the stem. This occlusion problem is common in image-based phenotyping (Das Choudhury et al., 2019). Even with imaging from multiple views (e.g., enabled by rotating plants during image acquisition), occlusion can still be substantial. The use of imaging systems carried by a robotic manipulator can provide viable solution to this issue, due to the flexibility of the robotic manipulator to position and orient cameras at the best intended viewpoints. Wu et al. (2019) proposed an automated multi-robot system, which comprised of three robotic arms each equipped with a depth camera to obtain the point cloud data of the plant (Figure 1A). Deep learning based next-best view (NBV) planning pipeline was presented to evaluate and select the next-best viewpoints to maximize the information gain from the plant in data acquisition process. The robotic arms then were manipulated based on the determined optimal viewpoints. Their system was more efficient and flexible compared to other robotic systems to address the occlusion issue. The ability of the system to find the optimal viewpoints, however, can be challenging, because its performance depends upon the predictions produced by the trained deep networks. This means that the best view-points may not be determined by the system if the deep networks can not generate accurate predictions.
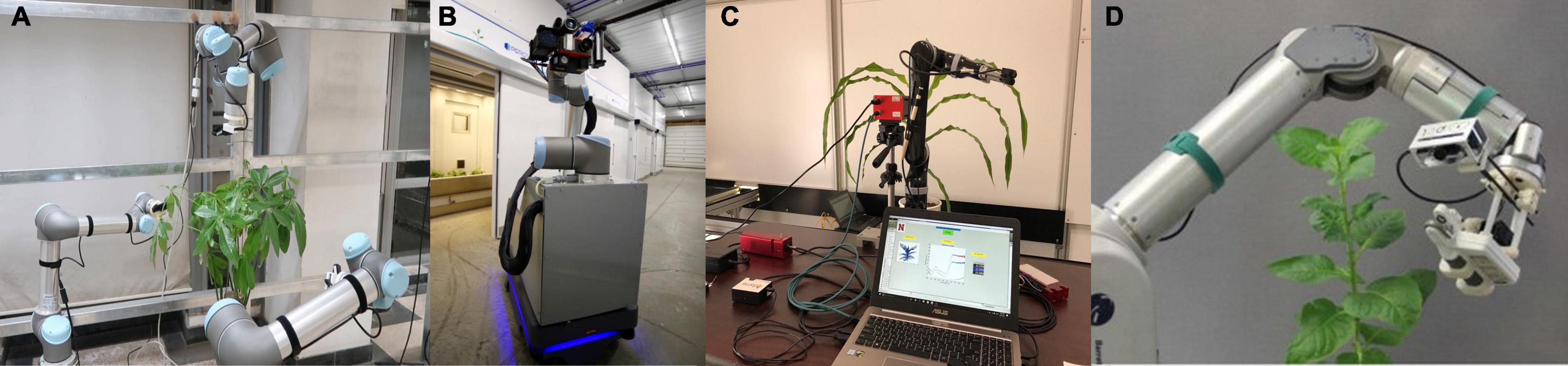
Figure 1. Plant phenotyping robotic systems for indoor environment: (A) A multi-robot system equipped with deep learning technique to determine optimal viewpoints for 3D model reconstruction (Wu et al., 2019), (B) Sensor-equipped robot to measure the reflectance spectra, temperature, and fluorescence of leaf (Bao et al., 2019c), (C) Robotic system to measure leaf reflectance and leaf temperate (Atefi et al., 2019), and (D) Robotic system for direct measurement of leaf chlorophyll concentrations (Alenyá et al., 2014).
A second group of indoor plant phenotyping robots sought to touch or probe plants or plant organs, in order to extend the ability of robotic phenotyping from plant’s outward morphological traits to innate physiological and biochemical traits (Schulz and Baranska, 2007; Biskup et al., 2009). In this sense, the phenotyping robot was designed to mimic humans to manipulate plants and measure certain traits from targeted plant organs (Figure 2). This type of the robotic systems usually included a robotic gripper designed to attach specialized plant sensors, and a vision module to segment the plant from the background and find an appropriate point on the organs for probing [Alenyà et al., 2011; Shah et al., 2016; Bao et al., 2017 (Ficus plant)] or grasping process [Alenya et al., 2013; Ahlin et al., 2016 (Anthurium, Pothos, and Dieffenbachia)]. A sensor-equipped robot was presented to measure physiological parameters of the plant (Bao et al., 2019c). The sensor unit including RGB, hyperspectral, thermal, and TOF cameras, and a fluorometer were attached to a robotic arm. The robot measured the reflectance spectra, temperature, and fluorescence by imaging the leaf or placing probes with millimeter distance from the leaf surface (Figure 1B). Two different plant phenotyping robotic systems were introduced to measure leaf and stem properties of maize and sorghum plants (Atefi et al., 2019, 2020). The systems consisted of a TOF camera, a four DOF robotic manipulator, and custom-designed grippers to integrate the sensors to the robotic manipulator. Image-processing and deep-learning based algorithms were presented to find the grasping point on leaves and stem. An optical fiber cable (attached to a spectrometer) and a thermistor were used to collect leaf hyperspectral reflectance and leaf temperature simultaneously. The stem diameter was measured by a linear potentiometer sensor. Leaf hyperspectral reflectance was used to build predictive models for leaf chlorophyll content, water content, N (nitrogen), P (phosphorus), and K (potassium) concentrations (Figure 1C). Alenyà Ribas et al. (2012) mounted a SPAD meter to a robotic arm to directly measure leaf chlorophyll concentrations of Anthurium White, Anthurium Red, and Pothus (Figure 1D). Quadratic surface models were applied to segment leaves from infrared-intensity images and depth maps captured by a TOF camera. The estimation issues of probing point caused by poor leaf-fitting model reduced the probing success rate of the robotic system (82%).
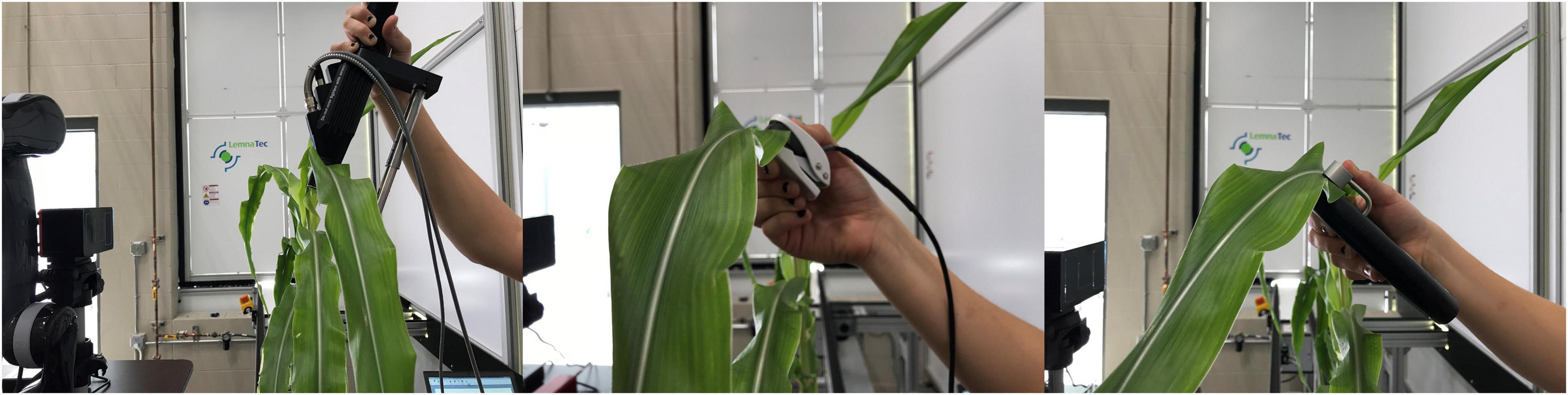
Figure 2. Manual measurements of leaf reflectance (left), leaf temperature (middle), and chlorophyll content (right) (Atefi et al., 2019).
Although controlled environments can make it easier to grow plants and quantify their phenotypic traits, because environment plays a large role in determining plant traits, plants grown in controlled environments show many differences from plants grown in field conditions. Therefore, with the exception of a growing range of horticultural crops where production occurs in control environments, for many crops the assessment of phenotypic responses in field conditions provides more directly actionable information for crop improvement. A wide range of platforms have been developed for field-based high-throughput plant phenotyping [Montes et al., 2007; White et al., 2012; Gao et al., 2018 (soybean); Weiss and Biber, 2011 (detection and mapping of maize plants); Jenkins and Kantor, 2017 (stalk detection of sorghum); Iqbal et al., 2020 (plant volume and height); Smitt et al., 2020 (fruit counting of sweet pepper and tomato)]. These robotic systems are guided between crop rows and moved toward plants. This creates several new challenges for both navigation and data collection which are absent when robotic phenotyping is conducted in control conditions. Factors like temperature, sunlight, wind, and unevenness of soil surface, can negatively impact the performance of the system. Therefore, the hardware and software of the robotic system must be designed to be resilient to the unique challenges of operating in field conditions. In the field plants are always stationary, necessitating that (1) phenotyping robots move to the plants rather than vice versa, (2) all components of the phenotyping robot including the vision system, robotic arm, and sensors as well as power supplies be carried by a robotic mobile platform, and (3) this platform be capable of navigation whether through global positioning system (GPS) data and/or employing sensors to perceive its local environment to guide navigation.
Unmanned ground vehicle (UGV) robotic systems employ a range of sensor types including light detection and ranging (LIDAR) and cameras [RGB, TOF, near infrared (NIR), and stereo vision] for data collection. They can be installed on a fixed stand within the overall mobile platform, or affixed a robotic arm to increase the number of diversity of positions from which sensor data can be collected. Different techniques such as 3D reconstruction, image processing, and machine learning are used for data analysis and quantify morphological traits. Existing UGV robotic systems have been employed to measure plant height, plant orientation, leaf angle, leaf area, leaf length, leaf and stem width, and stalk count of various species such as maize, and sorghum, sunflower, savoy cabbage, cauliflower, and Brussels sprout (Jay et al., 2015; Fernandez et al., 2017; Baweja et al., 2018; Choudhuri and Chowdhary, 2018; Vázquez-Arellano et al., 2018; Vijayarangan et al., 2018; Bao et al., 2019b; Breitzman et al., 2019; Qiu et al., 2019; Young et al., 2019; Zhang et al., 2020), count the cotton bolls (Xu et al., 2018), architectural traits and density of peanut canopy (Yuan et al., 2018), berry size and color of grape (Kicherer et al., 2015), and shape, volume, and yield estimation of vineyard (Lopes et al., 2016; Vidoni et al., 2017). A compact and autonomous TerraSentia rover equipped with three RGB cameras and a LIDAR was demonstrated to acquire in-field LIDAR scans of maize plants to extract their Latent Space Phenotypes (LSPs) (Gage et al., 2019). They were inferred from the images using machine learning methods (Ubbens et al., 2020) and contained information about plant architecture and biomass distribution. Shafiekhani et al. (2017) introduced a robotic system (Vinobot) including a six DOF robotic arm with a 3D imaging sensor mounted on a mobile platform. The Vinobot collected data in order to measure plant height and leaf area index (LAI) of maize and sorghum (Figure 3A). The authors reported that the use of a semi-autonomous approach created most of challenges for navigation of the system. In this approach, the alignment of the robot with the crop rows was required before autonomously moving between the rows and collecting data from the plants.
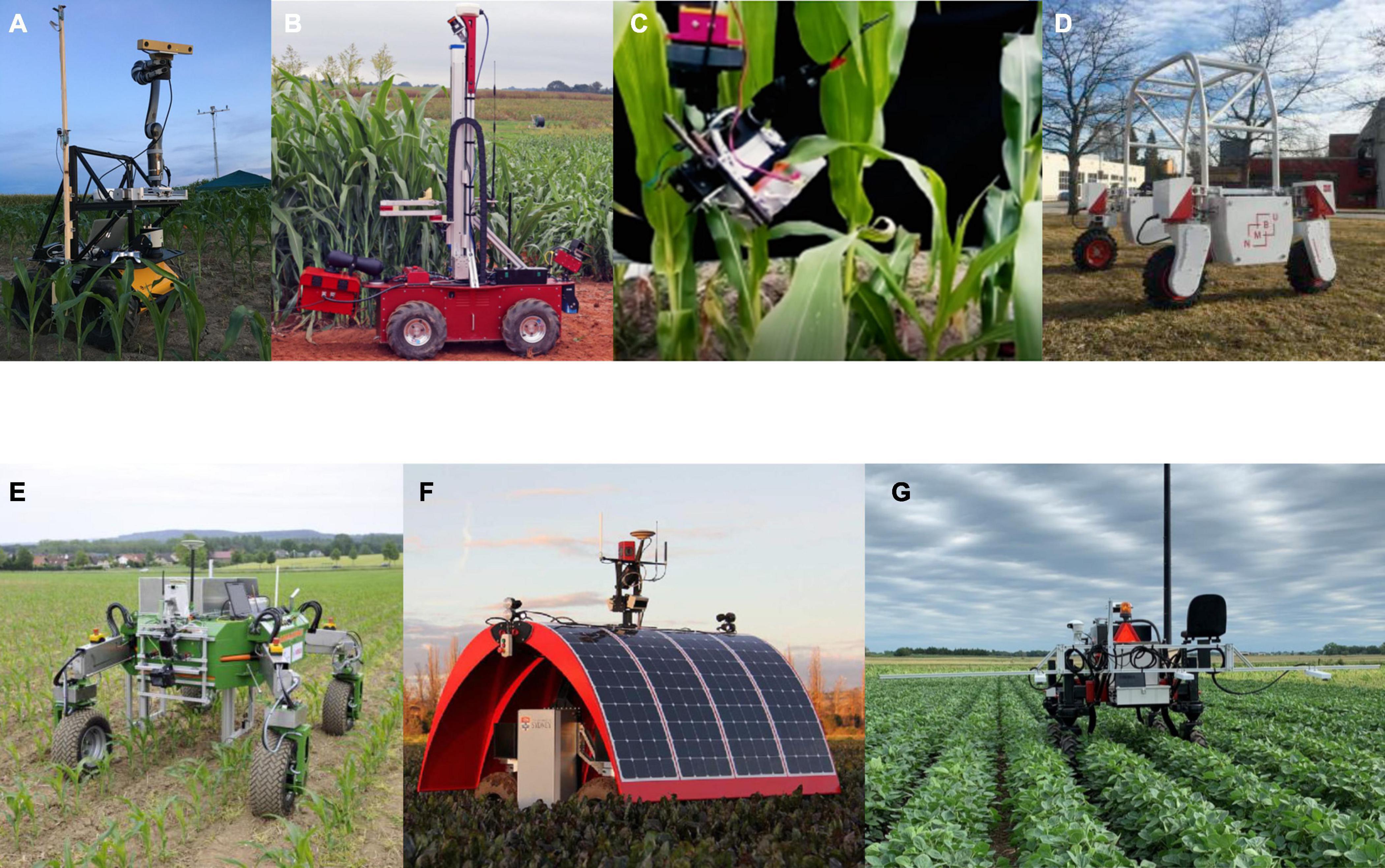
Figure 3. Plant phenotyping systems for outdoor environment: (A) Vinobot: robotic system including six DOF robotic manipulator and a 3D imaging sensor mounting on a mobile platform to measure plant height and LAI (Shafiekhani et al., 2017), (B) Robotanist: UGV-based robotic system equipped with a three DOF robotic manipulator and a force gauge for stalk strength measurement (Mueller-Sim et al., 2017), (C) A robotic system to slide LeafSpec across entire leaf to collect its hyperspectral images (Chen et al., 2021), (D) Thorvald II: VIS/NIR multispectral camera mounted on a mobile robot to measure NDVI (Grimstad and From, 2017), (E) BoniRob: autonomous robot platform using spectral imaging and 3D TOF cameras to measure plant height, stem thickness, biomass, and spectral reflection (Biber et al., 2012), (F) Ladybird: ground-based system consisted of a hyperspectral camera, a stereo camera, a thermal camera, and LIDAR to measure crop height, crop closure, and NDVI (Underwood et al., 2017), and (G) Flex-Ro: high-throughput plant phenotyping system equipped with a passive fiber optic, a RGB camera, an ultrasonic distance sensor, and an infrared radiometer for the measurement of NDVI, canopy coverage, and canopy height (Murman, 2019).
Measurements of some biochemical and physiological properties require a direct contact between sensors and plants. Measuring these properties therefore requires a robot capable of grasping or touching plant organs. Grasping plant organs in turn requires a dexterous robotic arm as well as onboard sensors and algorithms capable of reconstructing the 3D geometry of the target plant. Robotanist, a UGV equipped with a custom stereo camera, was established to measure stalk strength of sorghum (Mueller-Sim et al., 2017; Figure 3B). A three DOF robotic arm along with a special end-effector was mounted on Robotanist. The end-effector consisted of a rind penetrometer that was modified by attaching a force gauge and a needle. When the stalk was grasped by the end-effector, the needle and force gauge were pushed into the stalk to accomplish the measurement. The authors suggested to develop algorithms using laser scan and navigation camera data to improve the performance of the navigation system to reliably work under taller sorghum canopy and throughout the entire growing season. Abel (2018) attached a spectrometer to the robotic manipulator of Robotanist to capture spectral reflectance measurements of leaves and stems of sorghum. Random sample consensus (RANSAC) method was used for leaf and stem detection. A machine learning approach was applied to estimate the chlorophyll content of leaves, and moisture and starch contents of stems from reflectance spectra. Two factors reduced the grasping success rate of leaves (68%). First, the grasping process was failed because the wind moved the leaves and changed the position of the grasping point. Second, the occlusion and overlapping affected the performance of the segmentation algorithms to detect more leaves in the images. Chen et al. (2021) developed a robotic system including LeafSpec (invented at Purdue University) attached to a robotic manipulator to collect hyperspectral images of maize leaves in the field (Figure 3C). The robot slid the LeafSpec across the leaf from the beginning to tip to acquire hyperspectral images of entire leaf. The system predicted leaf nitrogen content with R2 = 0.73.
Other autonomous ground-based systems were presented to measure both morphological and biochemical/physiological attributes. A visible and near infrared (VIS/NIR) multispectral camera was mounted on a mobile robot called “Thorvald I” to measure the normalized difference vegetation index (NDVI) of wheat from multispectral images (Burud et al., 2017). The robot then modified to a new version called “Thorvald II” to have better performance for phenotyping tasks (Grimstad and From, 2017; Figure 3D). BoniRob was proposed as an autonomous robot platform including spectral imaging and 3D TOF cameras which can be used to measure plant parameters such as plant height, stem thickness, biomass, and spectral reflection (Ruckelshausen et al., 2009; Biber et al., 2012; Figure 3E). Underwood et al. (2017) introduced a ground-based system (Ladybird) for row phenotyping of grain and legume crops (wheat, faba bean, lentil, barley, chickpea, and field pea) (Figure 3F). Crop height, crop closure, and NDVI were determined after processing the data from the LIDAR and the hyperspectral camera. Flex-Ro, a multi-purpose field robotic platform was used for high-throughput plant phenotyping to measure phenotyping traits of soybean (Murman, 2019; Figure 3G). Three sets of sensors were installed on Flex-Ro to collect data from crop rows. For each set, a passive fiber optic cable, a RGB camera, an ultrasonic distance sensor, and an infrared radiometer were used to measure NDVI, canopy coverage, canopy temperature, and height.
Table 1 summarizes the indoor and outdoor robotic systems which could successfully measure plant traits for different crops.
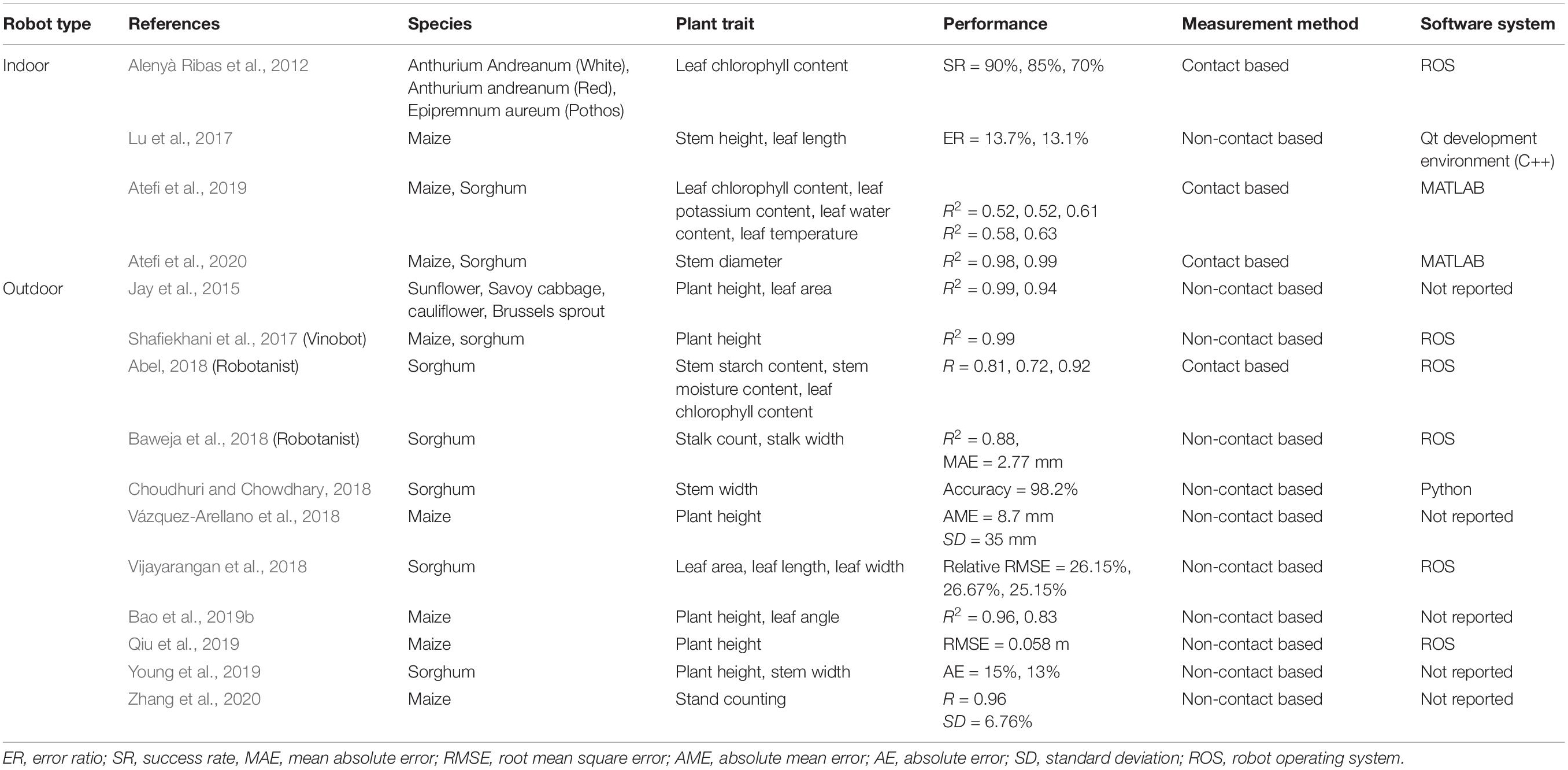
Table 1. Summary of indoor and outdoor robotic systems that successfully measured plant properties for different crops.
Figure 4 gives summary statistics regarding the plant phenotyping robotic systems that is discussed in this section. It can be seen that the robotic phenotyping research targeted maize and sorghum more than other species (soybean, wheat, barley, chickpea, pea, faba bean, lentil, cabbage, cauliflower, cotton, peanut, sunflower, grape, tomato, sweet pepper, and Arabidopsis) (Figure 4A). Maize and sorghum are two of the most economically important and highly diverse cereal crops with vast numbers of accessions (Zhao et al., 2016; Bao et al., 2019b). Therefore, more attention was devoted to breed Maize and sorghum to produce food, animal fodder, and biofuel. Moreover, the available genetic resources for these crops required the phenotyping data to map their genotypes to phenotypes and thus crop yield improvement. Accordingly, there has been an emerging need for phenotyping robots to automatically measure the phenotypic traits. Regarding the plant structure, maize, and sorghum have similar morphology. Their leaves are arranged alternately on each side of the stem that has cylindrical/elliptic-cylinder shape and is positioned in the middle part of the plant. This plant structure provides less complexity for the robotic system to distinguish between the stem and leaves and extract their features. Figure 4B shows that the height, width, and volume of plant/canopy are three main (morphological) traits that more frequently measured by the robotic systems than other traits, each of them being ≤ 5% (leaf length, leaf width, leaf angle, leaf area, leaf reflectance, leaf chlorophyll content, leaf/canopy temperature, LAI, plant/canopy NDVI, stem reflectance, stalk strength, stalk count, berry size, and fruit count). Two reasons can be considered for the frequent measurements of these phenotypic traits. Firstly, the plant architectural traits (such as plant height) are the most common and important parameters for field plant phenotyping since they have significant effects on light interception for photosynthesis, nitrogen availability, and yield (Barbieri et al., 2000; Andrade et al., 2002; Tsubo and Walker, 2002). Consequently, by studying and then manipulation of the plant architecture, the crop productivity will be increased. Secondly, as it was discussed in this section, the robot just needs non-contact based sensors (RGB camera or depth sensor) to collect data from the plants. Then, by analyzing the 2D images or creating plant 3D models, the aforementioned plant traits can be estimated in either ways: (1) the correlation between the pixel counts in the images and the ground truth measurements, or (2) extracting the distance/volume in real world from the depth sensor data. Hence, the measurement of these morphological properties is less challenging for the phenotyping robots using simple sensors and algorithms. In addition to the more frequent measurements of stem height and width (of maize and sorghum), these properties were also measured more accurately by the robotic systems because they are less affected by the plant morphology (Figure 4C). The first step to extract the stem height and width is to detect the stem and segment it from other plant organs. The morphology of maize and sorghum (alternately arranged leaves, and cylindrical-shaped stem in the middle) provides more hints for stem detection and segmentation. Moreover, the height and width can be measured as linear measurements. Accordingly, these two stem properties can be measured with less complexity and higher accuracy. Figure 4D illustrates that non-contact based sensing systems (such as RGB, stereo vision, and multispectral cameras, and LIDAR) were used more in phenotyping robots compared to contact-based sensors (chlorophyll meters, spectrometers, thermistors, and linear potentiometers). This can be explained by the fact that the majority of the robotic systems were developed to measure the morphological traits or some physiological properties. To achieve these goals, the phenotyping robots are required to use the 2D images/3D models of plants using non-contact based sensors. Among the non-contact sensors, the sensor-fusion based systems (including RGBD/stereo vision cameras, RGB camera + LIDAR, RGB + TOF cameras, spectral imaging + TOF camera) and depth sensors (TOF camera, LIDAR, laser scanner, and ultrasonic sensor) were commonly used as vision/sensing systems for the phenotyping robots. The key is to acquire depth information as a vital parameter to manipulate a robotic arm to grasp the plant organs, navigate a mobile robot between crop rows, and measure plant properties (such as height, width, and volume). Sensor-fusion based systems were employed by phenotyping robots more often than depth sensors. The reason would be that these sensors prepare the plant color/spectral information along with the depth information. Consequently, by acquiring more information, the plant can be effectively segmented from the background and the plant properties can be effectively measured. Regarding the robot software system, it can be found that Robot Operating System (ROS) is one the most popular systems to develop the software of the phenotyping robots (Figure 4E). ROS is an open source system that provides services, libraries, and tools for sensors-actuators interface, software components communication, and navigation and path planning1. Different manufacturers of robot’s hardware provide ROS drivers for their products such as imagery systems, sensors, actuators, robotic manipulators, and mobile platforms. This allows the researchers to develop the phenotyping robotic systems more efficiently.
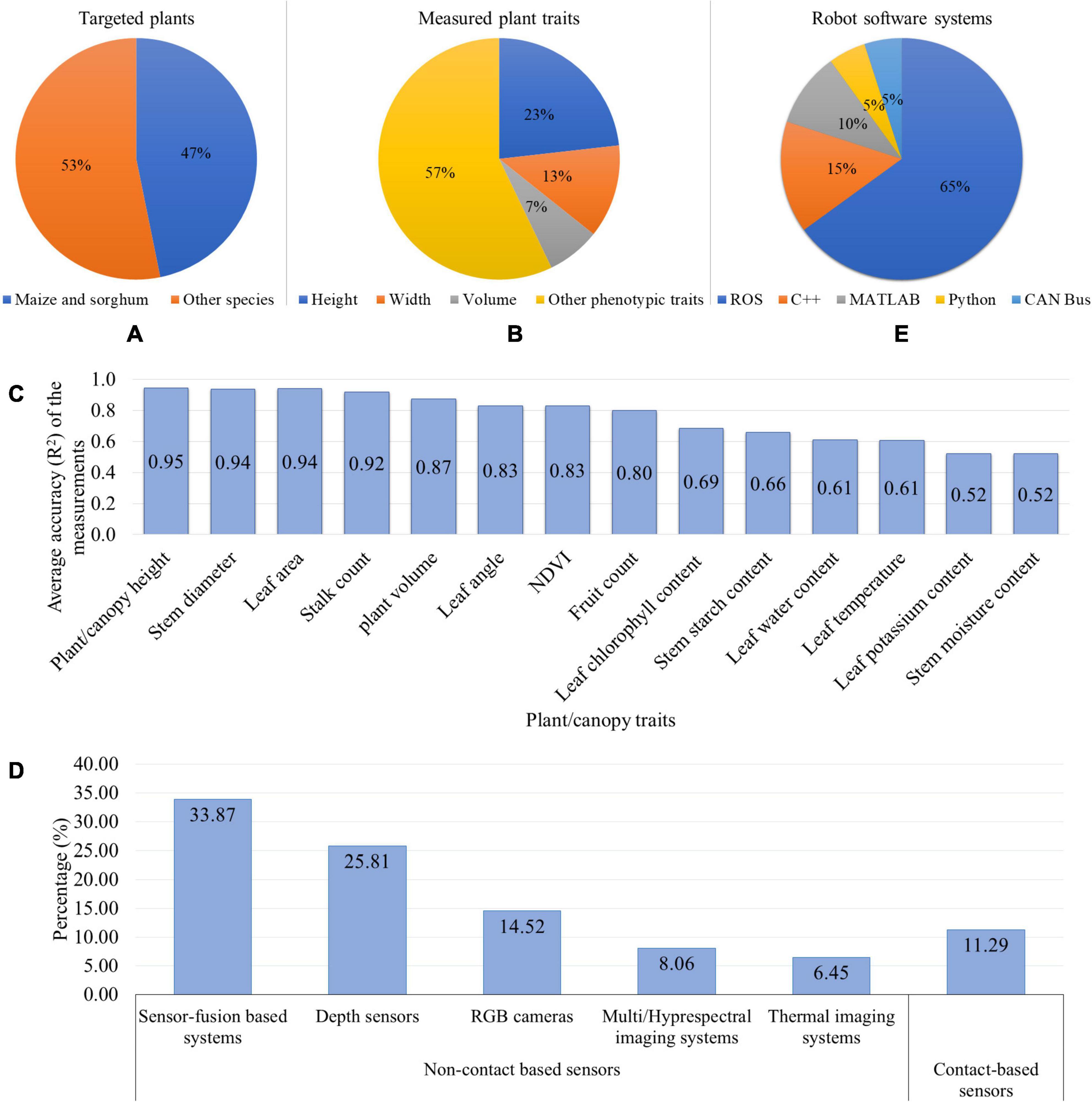
Figure 4. Summary statistics of the phenotyping robotic systems: (A) Targeted plants, (B) Plant/canopy traits measured by the robots, (C) Average accuracy (R2) to measure the phenotypic traits, (D) Robot vision/sensing systems, and (E) Robot software systems.
Regarding the platform of the mobile phenotyping robots, most of the mobile platforms were developed by researchers (86%) and few off-the-shelf robots were used (14%). The custom-designed platform offers potential to meet specific conditions regarding soil, weather, and plant which vary with experimental site and phenotyping task. Moreover, the researcher has more control on modifying the hardware and software. Based on the reviewed papers, most of the mobile platforms used four wheels/legged-wheels (88%) as their driving system compared with tracked mechanism (12%). In the wheeled-vehicles, the wheels can be independently steered (good maneuverability) which provides high flexibility with respect to control and navigation (desired orientation angle and rotation speed) of the vehicles between the crop rows in the field. Moreover, the vehicle can move faster using (legged) wheels and has high ground adaptability (crop height, and irregular and sloped terrain) using legged-wheels. However, the tracked-vehicles create more traction and less pressure on soil (work better in wet soil and less soil compaction), and can drive over rough terrain and obstacles easier than the wheeled-vehicles (Bruzzone and Quaglia, 2012). Accordingly, a hybrid locomotion system can be developed with the combination of legged-wheels and tracked systems. Therefore, this platform can use the advantages of the both driving systems to accomplish phenotyping tasks more effectively and efficiently.
Phenotyping Robots Face Several Challenges
There are several outstanding challenges in the development of robotic systems for plant phenotyping. Some of these challenges related to segmentation (vision systems) and grasping (robotic manipulators) are shared or at least similar for both indoor and outdoor phenotyping robots. Other challenges, particularly those related to navigation are specific to outdoor robotic phenotypic applications.
Complex and Deformable Nature of Plants Represents a Major Issue for Robot’s Vision and Sensing System
The UGV or robotic manipulator equipped with contact/non-contact based sensing systems offer a great potential to measure plant phenotypic data compare to non-autonomous robotic sensing systems. For example, the UGV equipped with stereo vision camera can move between crop rows and collect images from the canopy or individual plant. The image data can be analyzed immediately or can be processed later to extract plant properties. The long-term measurement of the plant traits can provide useful knowledge for crop modeling purposes over time (Duckett et al., 2018). Another example would be the robotic manipulator equipped with a hyperspectral imaging system. The robotic arm can move around the plant to locate the sensor close to the plant organs. With this proximal sensing, more phenotyping information can be acquired about the organs. However, the robotic vision/sensing technologies for the phenotyping task encounter different challenges.
Various imaging technologies are utilized as vision systems of the robots. Visible imaging/RGBD camera are commonly used technologies that rely on the color/texture information of an object. Images are processed to segment plant organs and identify desirable targets for grasping. The identification and localization of different plant organs (such as leaves, stems, flowers, and fruits) is one of the major problems in computer vision, due to complex structure and deformable nature of plants. The overlap between the adjacent leaves or leaf-stem causes occlusion; even though leaf and stem have different architecture, they share similarities in color and texture. Accordingly, it is difficult to distinguish occluded leaves or stem in the image. The morphology of plants (shape and size) varies dramatically across different plant species and even within a single species different varieties or the same variety grown in different conditions may exhibit radically different morphology. In this regard, the software of the robotic system should cover a wide range of scenarios and possibilities to be able to respond and adapt appropriately to day-to-day changes in the same plant or differences between plants within the same experiment. Additionally, non-uniform imaging conditions (lighting and background) make it more complex to find an appropriate color space and optimal approach for the segmentation purposes (Zhang et al., 2016; Narvaez et al., 2017; Qiu et al., 2018; Bao et al., 2019a).
Multispectral/hyperspectral and thermal imaging systems are sensitive to illumination since the reflectance from the plant organ is depend on its distance and orientation toward the light source/incident radiation and camera. Moreover, multiple reflectance and also shade will occur due to the curvature nature and complex geometry of plant (Li et al., 2014; Mishra et al., 2017; Qiu et al., 2018). To deal with these issues, researchers introduced different technical solutions. Behmann et al. (2016) combined the hyperspectral image with 3D point cloud (using a laser scanner) of sugar beet to create hyperspectral 3D model. Then, it was used to quantify and model the effects of plant geometry and sensor configuration. Finally, the geometry effects in hyperspectral images were weakened or removed using reflectance models. Shahrimie et al. (2016) used inverse square law and Lambert’s cosine law along with Standard Normal Variate (SNV) for maize plants to remove the distance and orientation effects.
Robotic Control System Needs to Deal With Dynamic and Unstructured Environment
The size and orientation of the plant organs are constantly changing across their growth stages. Therefore, the lack of needed DOF or enough workspace of the robotic manipulator are the limitations for the robots to grasp the plant organs and sense their properties successfully. The robotic arm cannot reach the organs if they are out of its workspace. In addition, a robot arm with less flexibility (DOF) might not able to properly adjust the angle of its end-effector in grasping process.
Field-based robots need to navigate between crop rows and then turned to the next row safely and autonomously. To achieve this task, the crop rows and obstacles should be detected to build a map of the surrounding area. Then, their position and orientation relative to the vehicle will be found to compute an optimal path and avoid unexpected obstacles. Finally, adequate action will be determined to steer the wheels and guide the system around the field. However, the uncontrolled and unstructured field environment creates challenges for accurate navigation and control of the robot (De Baerdemaeker et al., 2001; Nof, 2009; Bechar and Vigneault, 2016; Shamshiri et al., 2018). GPS is a common method for robot navigation. However, the tall plant canopy affects on the accuracy of GPS for navigation purposes as the canopy blocks the satellite signals to the GPS receiver. Hence, the information provided from other sensors along with GPS is also required to detect the obstacles, precisely guide the phenotyping robot, and minimize the damage to the robot and plants. The UGV based phenotyping robots can facilitate the data fusion of GPS and other sensors since the robot can equipped with various sensors (such as LIDAR, RGB/stereo vision cameras) and also precisely control their location (Duckett et al., 2018). Nonetheless, varying ambient light conditions, changing crop growth stages (size, shape, and color), and similar appearance between crops and weeds are common factors that fail visual navigation. In these situations, RGB sensor-based systems usually cannot find a stable color-space or plant features to detect different objects. Incomplete rows and missing plants can cause errors to compute distance between the robot and plants using range sensors. Different soil properties (soil types and moisture) and terrain variation (even, uneven, flat, slope) are other factors that influence robot dexterous manipulation, wheel-terrain interaction, wheel slip, and steering control algorithms (Åstrand and Baerveldt, 2005; Grift et al., 2008; Li et al., 2009; Shalal et al., 2013).
After navigating the robot between the rows, a suitable path should be selected for the robotic manipulator with minimum collisions inside a plant or canopy to reach and grasp the targets delicately. However, robots operate in extremely complex, dynamic, uncertain, and heterogenous real world condition. In this situation, visual occlusion of a plant by others caused by high plant density should be taken into account for target identification and segmentation. In addition, the target information will be affected by sunlight and wind. For instance, TOF/RGBD cameras use infrared light to measure distance. Since the sunlight has infrared wavelengths and wind moves the targets, the location of the target in 3-dimensional space might not be accurately measured (Andújar et al., 2017; Narvaez et al., 2017; Qiu et al., 2018; Li et al., 2020a). Consequently, the obstacle-avoidance path-planning algorithm cannot be determined correctly. Another example would be when the targets are seen shinier or darker because of specular reflection or shade.
Issues With Robot Software for Phenotyping Robotic System Development
Two main drawbacks present in many robot software are: (1) the lack of support for certain functional packages (of open source software) and (2) real-time constraints (Barth et al., 2014; Park et al., 2020). For the first issue, it can be supposed that a phenotyping robot is developed by researchers to accomplish a phenotyping task. They create the robot library and share their codes with the (open source) software community. However, by ending the project, there is no guarantee to fix the bugs and update the codes. In the case of other researchers might start similar research using the shared codes, it might be problematic to make the research forward because of the lack of support for the robot library. The second challenge is the real-time constraints that causes system malfunction due to latency. One example would be when a UGV moves between crop rows to measure plant traits. If the robot cannot satisfy the real-time constraints, the robot will have delay to identify the obstacles or adjust its position relative to the crop rows. Accordingly, the robot could hit the obstacles and the plants and this causes the physical damage to the robot or plants. Regarding ROS, although ROS1 has real-time constraints, however the community is actively working on software improvement. For example, RT-ROS supports the real-time communication that leads to performance enhancement of ROS1 (Wei et al., 2016). It is obvious that by growing the ROS community, sophisticated libraries and packages will be developed for more plant phenotyping applications.
Other Challenges: Managing Big Data, Reliable Power Source, Durability Under Harsh Environment, and High Cost
The phenotyping robot collects massive volumes and various types of data (such as images, multi/hyperspectral data) taken by different sensors from large population of plants. The robot needs to analyze large quantities of data in real-time for suitable action/decision-making process. In addition, the large-scale phenotypic data could be stored properly for the benefit of future research. Therefore, managing and analyzing the big data as a result of high-throughput, robotically collected plant traits is an emerging issue for the phenotyping robot.
The field-based mobile robots need to be equipped with reliable power sources to provide energy for the vehicle carriage weight, distance traveled, and different electrical components such as sensors for data collection. Batteries are commonly used for this purpose. The problems with batteries are: (1) limited operating time that prevents the robots to work for long time and accomplish large-scale missions, and (2) need to recharge which typically takes a long time.
Another challenge is the durability and stability of these robotic systems under harsh outside environment caused by extreme temperature, high humidity, strong sunlight, and dust. These harsh conditions can cause damages for the components of the robotic system and accordingly will have negative effects on the robot’s performance.
The cost of phenotyping robots (in general agricultural robots) is still high and this makes limitations for wide-spread use of the robots. In most cases the phenotyping robotic systems are developed for research purposes and the robots are not commercially available yet. Both the hardware and software systems were restricted to a very specific condition and could not be transferred to a different scenario. This leads to high R&D (research and development) cost that can not be spread over multiple units. However, more general purpose phenotyping robots can be developed and commercialized in the future and their cost will be reduced substantially. Moreover, with the consistent trend of price reduction of electronics, sensors, and computers, the robotic systems will become cost-effective enough to be more widely used for phenotyping tasks.
Potential Improvements of Phenotyping Robots
Sensors and Controllers Fusion Technique Can Improve the Performance of Robot
Sensing-reasoning, and task planning-execution are two essential functions for autonomous phenotyping robots. They sense the environment, apply an appropriate control algorithms, make decision, and act in real-time to perform the phenotyping tasks (Grift et al., 2008; Bechar and Vigneault, 2016). The design of the phenotyping robot and its control algorithm needs to be optimized to achieve successful operation in continuously changing environment. To reach this purpose, the phenotyping robots need to employ advanced technology to cope with the dynamic and unstructured environment. The emerging sensor technologies such as sensor fusion increase the robot capabilities and yield better results (Grift et al., 2008). Sensor fusion allows the robot to combine information from a variety of sensing modules to form better decision for navigation and path planning, as well as increase the capacity of sensing to gather more information from the plants. For example, Choudhuri and Chowdhary (2018) measured the stem width of sorghum with 92.5% accuracy using RGB data. However, they achieved higher accuracy (98.2%) after combining RGB + LIDAR data. Kim et al. (2012) could successfully navigate an unmanned weeding robot using sensor fusion of a laser range finder (LRF) and an inertial measurement unit (IMU). The robot also needs more sophisticated and intelligent algorithms to accomplish different subtasks such as sensing, navigation, path-planning, and control. Different control strategies such as genetic algorithm (GA), fuzzy logic (FL), neural network (NN), reinforcement learning (RL), and transfer learning (TL) can be integrated to develop such robot algorithms (Shalal et al., 2013). Therefore, a robust controller will be provided for the phenotyping robot since the robot control system can use the merits of both technologies (combining two control strategies). Batti et al. (2020) studied the performance of fuzzy logic and neuro-fuzzy (NN + FL) approaches to guide a mobile robot moving between the static obstacles. The authors found that neuro-fuzzy controller provide better results for robot navigation compare to fuzzy logic controller. Although several different autonomous phenotyping robots were developed, more research is needed to adapt and improve the advanced technologies to overcome the robot limitations to accomplish the phenotyping tasks, and also increase the autonomy level of the phenotyping robots.
Internet of Robotic Things (IoRT): Technology to Manage Big Data for Phenotyping Robots
Internet of Things (IoT) technologies are helpful to send lots of data collected by different sensors over Internet in a real-time manner. The Internet-of-Robotic-Things (IoRT) is the confluence of autonomous robotic systems with IoT which is an emerging paradigm that can be employed for phenotyping robots (Grieco et al., 2014; Ray, 2016; Batth et al., 2018; Saravanan et al., 2018; Afanasyev et al., 2019). Mobile robots can use IoT to transfer and store a large amount of phenotypic datasets to a central server. By sending the data via IoT, the robots do not need to frequently move to a place and physically upload the collected data to a local server/computer. Moreover, plant breeders/scientists can visualize the data using a mobile device (a tablet or a smartphone) or an office computer and therefore the performance of plants and changes in crop growth and development can be remotely inspected in different regions of the field in a real-time fashion. Another attractive aspect of using IoRT is to send commands to robots to accomplish phenotyping tasks. For instance, an operator can remotely control the greenhouse robotic manipulator systems via Internet any time from his home/office to collect phenotypic data. Another example is when the close inspection of an area in a field is necessary after analyzing the drone-based image data; therefore, commands can be sent via Internet to deploy mobile robots in this regard. Several mobile robots can work together to operate more efficiently to achieve a specific task.
Solar Panels and Hydrogen Fuel Cell: Renewable Power Sources for Phenotyping Robots
Solar panels and hydrogen fuel cell are two technologies that produce clean, renewable, and sustainable energy. A solar panel consists of many small units called photovoltaic cells which convert sunlight into electricity. The maintenance cost of the solar panel is low since it does not have moving parts (no wear) and it just need to clean the cells. The hydrogen fuel cell comprised a pressurized container to store hydrogen. The fuel cell is an electrochemical device that takes oxygen from the air and combines hydrogen with oxygen to produce electricity. Re-fueling time of a hydrogen fuel cell is very short (5 min or less) and its cells are fairly durable.
Based on the advantages of solar panels and hydrogen fuel cell, both technologies can be used as renewable power sources for different components of the phenotyping robots (Underwood et al., 2017; Quaglia et al., 2020). However, there is not a wide range of application of these technologies for the phenotyping robots. The cost of both technologies is high. For solar panels, the efficiency of the system drops in cloudy and rainy days. In addition, more solar panels are needed to produce more electricity which requires a lot of space. For hydrogen fuel cell, there are relatively few places to re-fuel the cell. Nevertheless, both technologies are constantly developing which can be assumed to reduce their cost and improve their efficiency to produce electricity.
Perspective Applications of Robotic Phenotyping
Phenotyping Robots Has Great Potential to Measure Other Plant Properties
Section “Review: Many Indoor and Outdoor Robots Were Developed to Measure a Wide Range of Plant Traits” introduced the robotic systems for indoor and outdoor applications to measure several different plant traits. However, other leaf/stem characteristics are also reliable indicators to detect the symptoms of biotic/abiotic stresses and monitor the plant health during a growing season. Stomatal conductance, gas exchange, and chlorophyll fluorescence of leaves are indicative of their water status, photosynthesis, and chlorophyll content (Castrillo et al., 2001; Ohashi et al., 2006; Li et al., 2020b). Stem sap flow and lodging resistance can provide useful information about plant water use and stem strength (Cohen et al., 1990; Kong et al., 2013). These aforementioned phenotypic traits are still measured manually. On the other hand, new clip-on sensor system can be presented to measure them automatically. The system includes a custom-designed gripper/clip combined with novel sensor(s) (Afzal et al., 2017; Palazzari et al., 2017). The design of these sensing systems is important since the accuracy and robustness of trait prediction models depend on the phenotypic data quality (Würschum, 2019). The design of the gripper and DOF of the robotic manipulator should allow a good and gentle contact between the sensing unit and the leaf/stem. Sometimes a vacuum mechanism attached to a soft gripper can hold the leaf/stem and help the sensing unit for effective contact and collect accurate data with less damage to the plant organs (Hayashi et al., 2010; Hughes et al., 2016; Zhang et al., 2020). Moreover, autonomous robots should gather data with minimum error (high signal to noise ratio). Therefore, sensors with high signal to noise ratio should be selected and accurately calibrated. In addition to the accuracy, the robots should rapidly (short execution time) accomplish their missions. Deep reinforcement learning (DRL) technique is an accurate and reliable method to find an optimal path with nearest and collision avoidance route. This technique can be adopted by phenotyping robots to manipulate a robotic arm for grasping process or to navigate a mobile robot between crop rows (Zhang et al., 2015; Zhang et al., 2019; Duguleana and Mogan, 2016; Franceschetti et al., 2018; Taghavifar et al., 2019). Although the robotic phenotyping is mainly focusing on leaf and stem, it can be utilized for other plant organs such as inflorescences (spike, panicle, and tassel), flowers, fruits, and roots.
The morphometric parameters of inflorescence are highly correlated with yield and grain quality (Leilah and Al-Khateeb, 2005; Gegas et al., 2010). Several studies discussed about using image-based techniques (2D images/3D reconstruction) to extract architectural traits such as length and width of inflorescence, inflorescence volume (weight), grain shape and size, grain angle, and number of grains, and number of flowers (Faroq et al., 2013; Crowell et al., 2014; Gage et al., 2017; Rudolph et al., 2019; Sandhu et al., 2019; Xiong et al., 2019; Zhou et al., 2019). In such applications to measure the morphological traits, a robot with LIDAR/camera can be useful to automatically take images/point cloud data from different views of the inflorescence. The physiological traits are indicator for stress or disease. For instance, the temperature of the spikes was used for detecting the plant under the water stress (Panozzo et al., 1999). Conceivably, a robotic arm equipped with a temperature sensor can grasp the spike and insert the sensor into spikelets to record their temperature.
Several properties of fruits such as water content, sugar content, chlorophyll, carotenoid, soluble solid, acidity, and firmness are measured for fruit quality assessment. The spectroscopy/spectral imagery are non-destructive and high-throughput methods to estimate these qualitative parameters (Berardo et al., 2004; ElMasry et al., 2007; Shao and He, 2008; Wu et al., 2008; Nishizawa et al., 2009; Penchaiya et al., 2009; Ecarnot et al., 2013; Guo et al., 2013; Dykes et al., 2014; Wang et al., 2015; Mancini et al., 2020). However, a robotic system can be presented to monitor the dynamics of these attributes for hundreds of growing fruits per day. For example, a portable spectrometer can be attached to the robot’s end-effector. After detecting the fruit on the plant, the robot can grasp the fruit and gather its spectral data to further infer its quality parameters.
Since the root has functional roles in resource acquisition, the characteristics of root provide valuable information about plant physiological and ecosystem functioning (Mishra et al., 2016). In traditional root phenotyping, two different methods are used to acquire images from root (in the soil or soil-free or transparent media). In first method, a camera is mounted on a tripod and moved by a human around the root, and in the second method camera(s)/sensor(s) are set in fixed point(s) and root (plant) is rotated (Atkinson et al., 2019). This is a tedious task and some root information (such as fine branches) might be lost due to less flexibility of the system to take up close images from the complex architecture of root. Consequently, automated root phenotyping systems can facilitate and improve the traditional root phenotyping in terms of efficiency and effectiveness with acquiring fast and precise measurements (Wu et al., 2019). Here, the “plant to sensor” system can be used to examine vast number of roots (or plants) without the need of huge space of greenhouse facility. In this system, the root (or plant) is moved toward a robotic manipulator (equipped with camera/sensor) and located on a rotation table. In each step angle of the table, the root is rotated and stopped in front of the robotic system. Then, the robotic manipulator moves the camera around the root and gather close proximity data from different views (positions and angles). Therefore, more detailed information of root can be captured due to high resolution sensing offered by the robotic system.
Robots in Greenhouses Complement the Image-Based Phenotyping
Automatic greenhouses such as LemnaTec (LemnaTec GmbH, Aachen, Germany) monitor plants using image-based technique. While it has shown great potential to measure and predict the plant traits, many hurdles cannot be handled by this technology. It needs to efficiently manage “big data” problems and also postprocess images to characterize the plant traits. Moreover, this approach is not sufficient for early detection of stress/disease with internal symptoms. Furthermore, this method requires direct measurements using sensors to calibrate and validate of its models to extract the phenotypic traits from images (Madden, 2012; Mutka and Bart, 2015; Singh et al., 2016; Lee et al., 2018). Hence, several robotic arms with different sensors can be integrated to the greenhouse for real-time and direct measurement of the chemical/physiological traits. Basically, plants are transported by an automatic conveyor belt and stopped in front of each robotic system. Then, the system uses “sensor-to-plant” concept (Lee et al., 2018) in which the robot moves toward the plant to take measurements before sending it through the imaging chambers. These stationary robotic systems are designed to operate in indoor environment. Moreover, several robots can be presented to collect data from a specific plant. It is difficult to develop a general prototype that are broadly applicable for different conditions (Mutka and Bart, 2015; Wu et al., 2019). However, the software and hardware of the robots should be adapted to other species and field-phenotyping applications. The challenge for both type of robots (indoor/outdoor) would be continuously collect and save large amount of data.
Swarm Robot Is a New Frontier to Efficiently Accomplish Complex Phenotyping Tasks
Swarm robotics is a new frontier technology which has potential application for proximal sensing of plants, and data/sample collection in a large field. A swarm robotics system composed of large numbers of autonomous robots that are coordinated with local sensing and communication, and a decentralized control system (Brambilla et al., 2013; Bayındır, 2016; Blender et al., 2016; Chamanbaz et al., 2017; Figure 5A). The application of swarm robots has some advantages which is suitable for large scale tasks. Since swarm robotics has large population size, the tasks can be decomposed using parallelism and can be completed efficiently and consequently it would save time significantly. Moreover, the swarm robots can achieve the distributed sensing that means they can have a wide range of sensing in different places at the same time (Navarro and Matía, 2012; Tan and Zheng, 2013).
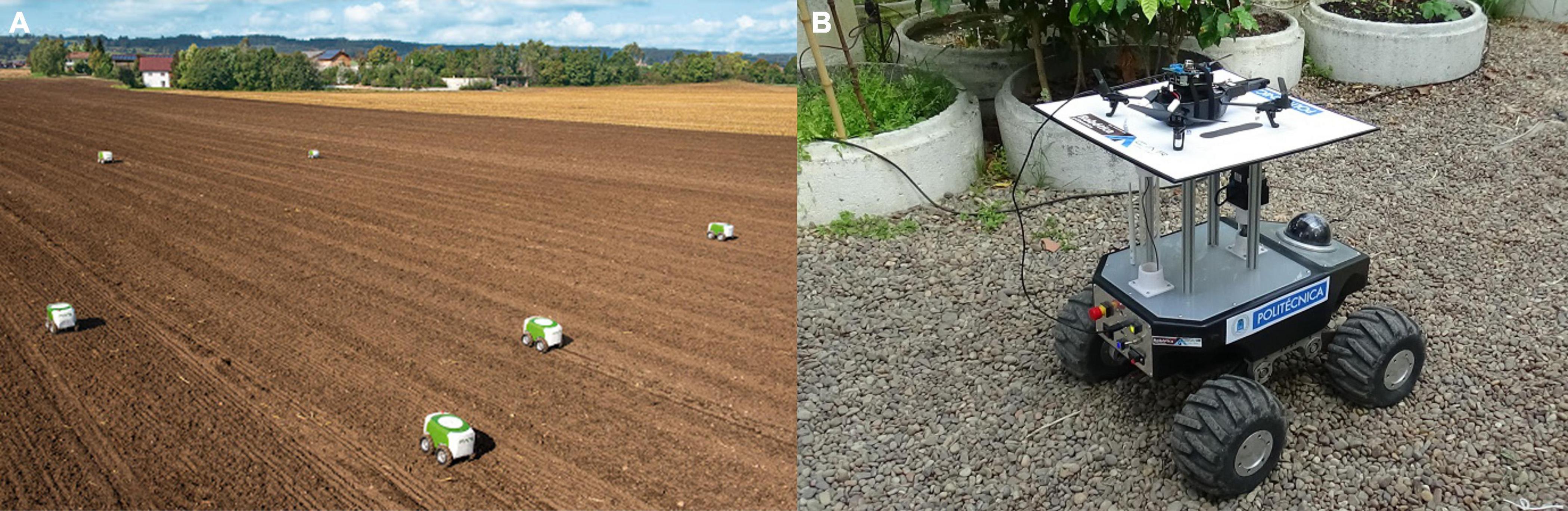
Figure 5. (A) Mobile Agricultural Robot Swarms (MARS) for seeding process (The European Coordination Mobile Agricultural Robot Swarms (MARS). PDF file. November 11, 2016. http://echord.eu/public/wp-content/uploads/2018/01/Final-Report-MARS.pdf), (B) UAV-UGV cooperative system to measure environmental variables in greenhouse (Roldán et al., 2016).
Both UAV and UGV by itself have been successfully employed in plant phenotyping tasks. The coordination between UAV and UGV enables a new breakthrough application of UAV/UGV cooperative systems to achieve a common goal more effectively and efficiently (Arbanas et al., 2018; Vu et al., 2018). Both vehicles in this cooperative team share complementarities according to their capabilities that allow them to operate in the same field and work together to fulfill phenotyping missions. In this manner, the UAV can fly to quickly obtain overview of the fields beyond the obstacles; whereas the UGV can continuously patrols in the field with large payload capabilities of different sensors and robotic arms (Chen et al., 2016; Roldán et al., 2016; Figure 5B). In the context of UAV-UGV cooperation, an obstacle map of the field will be provided by the UAV for UGV path planning. Based on their communication and the map, the UGV can move rapidly between the crop rows for up-close plant investigation.
Concluding Remarks
Autonomous robotic technologies have the potential to substantially increase the speed, capacity, repeatability, and accuracy of data collection in plant phenotyping tasks. Many robotic systems are successfully developed and deployed in both greenhouse and field environments, tested on a variety of plant species (row crops, specialty crops, and vineyards), and capable of measuring many traits related to morphology, structure, development, and physiology. Many technical challenges remain to be addressed regarding sensing, localization, path planning, object detection, and obstacle avoidance. Intensive research is needed to overcome these limitations of phenotyping robots and improve their speed, accuracy, safety, and reliability. Collaborations among different disciplines (such as plant science, agricultural engineering, mechanical and electrical engineering, and computer science) are imperative. With this transdisciplinary research, more efficient and robust sensing and control systems will be developed for intelligent plant phenotyping robots. Sophisticated sensor modules can be developed using sensor-fusion techniques. Regarding the control systems, multiple intelligent algorithms (such as different AI algorithms) can be combined to design more powerful controllers. These developments can potentially overcome the issues caused by changing environmental parameters, and complex structure of plants. Moreover, the suitable sensing and control systems yield better performance for accurate object detection (mainly for plants and crops, but also for humans, animals and other obstacles coexisting in the environments), path planning, and navigation. Sufficient funding from the public and private sources is the key to fuel the high-risk research in intelligent phenotyping robots in a sustainable way. We are optimistic that, in the next 10 years, we will see great leaps forward in autonomous and robotic technologies in plant phenotyping, enabled by the confluence of the rapid advancements in sensing, controllers, and intelligent algorithms (AIs).
Author Contributions
AA and YG provided the conceptualization of the manuscript. AA drafted the manuscript. YG, SP, and JS substantially edited the manuscript. All the authors contributed to the article and approved the submitted version.
Funding
The funding for this work was provided by USDA-NIFA under Grant No. 2017-67007-25941 and National Science Foundation under Grant No. OIA-1557417.
Conflict of Interest
The authors declare that the research was conducted in the absence of any commercial or financial relationships that could be construed as a potential conflict of interest.
Footnotes
References
Abel, J. (2018). In-Field Robotic Leaf Grasping and Automated Crop Spectroscopy. Pittsburgh, PA: Carnegie Mellon University.
Adamides, G., Katsanos, C., Parmet, Y., Christou, G., Xenos, M., Hadzilacos, T., et al. (2017). HRI usability evaluation of interaction modes for a teleoperated agricultural robotic sprayer. Appl. Ergon. 62, 237–246. doi: 10.1016/j.apergo.2017.03.008
Afanasyev, I., Mazzara, M., Chakraborty, S., Zhuchkov, N., Maksatbek, A., Kassab, M., et al. (2019). Towards the internet of robotic things: analysis, architecture, components and challenges. arXiv [Preprint]. Avaliable online at: https://arxiv.org/abs/1907.03817 (accessed April 23, 2020).
Afzal, A., Duiker, S. W., Watson, J. E., and Luthe, D. (2017). Leaf thickness and electrical capacitance as measures of plant water status. Trans. ASABE 60, 1063–1074.
Aguiar, A. S., Dos Santos, F. N., De Sousa, A. J. M., Oliveira, P. M., and Santos, L. C. (2020). Visual trunk detection using transfer learning and a deep learning-based coprocessor. IEEE Access 8, 77308–77320.
Ahlin, K., Joffe, B., Hu, A.-P., McMurray, G., and Sadegh, N. (2016). Autonomous leaf picking using deep learning and visual-servoing. IFAC PapersOnLine 49, 177–183. doi: 10.1016/j.ifacol.2016.10.033
Alenya, G., Dellen, B., Foix, S., and Torras, C. (2013). Robotized plant probing: leaf segmentation utilizing time-of-flight data. IEEE Robot. Autom. Mag. 20, 50–59. doi: 10.1109/MRA.2012.2230118
Alenyà, G., Dellen, B., and Torras, C. (2011). “3D modelling of leaves from color and ToF data for robotized plant measuring,” in 2011 IEEE International Conference on Robotics and Automation, Shanghai, 3408–3414. doi: 10.1109/ICRA.2011.5980092
Alenyà Ribas, G., Dellen, B., Foix Salmerón, S., and Torras, C. (2012). “Robotic leaf probing via segmentation of range data into surface patches,” in Proceedings of the 2012 IROS Workshop on Agricultural Robotics: Enabling Safe, Efficient, Affordable Robots for Food Production, Vilamoura, 1–6.
Alenyá, G., Foix, S., and Torras, C. (2014). ToF cameras for active vision in robotics. Sensors Actuators A Phys. 218, 10–22. doi: 10.1016/j.sna.2014.07.014
Andrade, F. H., Calvino, P., Cirilo, A., and Barbieri, P. (2002). Yield responses to narrow rows depend on increased radiation interception. Agron. J. 94, 975–980.
Andrade-Sanchez, P., Gore, M. A., Heun, J. T., Thorp, K. R., Carmo-Silva, A. E., French, A. N., et al. (2013). Development and evaluation of a field-based high-throughput phenotyping platform. Funct. Plant Biol. 41, 68–79. doi: 10.1071/FP13126
Andújar, D., Dorado, J., Bengochea-Guevara, J. M., Conesa-Muñoz, J., Fernández-Quintanilla, C., and Ribeiro, Á (2017). Influence of wind speed on RGB-D images in tree plantations. Sensors 17:914. doi: 10.3390/s17040914
Arad, B., Balendonck, J., Barth, R., Ben- Shahar, O., Edan, Y., Hellström, T., et al. (2020). Development of a sweet pepper harvesting robot. J. F. Robot. 37, 1027–1039. doi: 10.3390/s16081222
Araus, J. L., and Cairns, J. E. (2014). Field high-throughput phenotyping: the new crop breeding frontier. Trends Plant Sci. 19, 52–61. doi: 10.1016/j.tplants.2013.09.008
Arbanas, B., Ivanovic, A., Car, M., Orsag, M., Petrovic, T., and Bogdan, S. (2018). Decentralized planning and control for UAV–UGV cooperative teams. Auton. Robots 42, 1601–1618.
Åstrand, B., and Baerveldt, A.-J. (2002). An agricultural mobile robot with vision-based perception for mechanical weed control. Auton. Robots 13, 21–35. doi: 10.1023/A:1015674004201
Åstrand, B., and Baerveldt, A.-J. (2005). A vision based row-following system for agricultural field machinery. Mechatronics 15, 251–269. doi: 10.1016/j.mechatronics.2004.05.005
Atefi, A., Ge, Y., Pitla, S., and Schnable, J. (2019). In vivo human-like robotic phenotyping of leaf traits in maize and sorghum in greenhouse. Comput. Electron. Agric. 163:104854. doi: 10.1016/j.compag.2019.104854
Atefi, A., Ge, Y., Pitla, S., and Schnable, J. (2020). Robotic detection and grasp of maize and sorghum: stem measurement with contact. Robotics 9:58.
Atkinson, J. A., Pound, M. P., Bennett, M. J., and Wells, D. M. (2019). Uncovering the hidden half of plants using new advances in root phenotyping. Curr. Opin. Biotechnol. 55, 1–8. doi: 10.1016/j.copbio.2018.06.002
Bai, G., Ge, Y., Hussain, W., Baenziger, P. S., and Graef, G. (2016). A multi-sensor system for high throughput field phenotyping in soybean and wheat breeding. Comput. Electron. Agric. 128, 181–192. doi: 10.1016/j.compag.2016.08.021
Bao, Y., Tang, L., Breitzman, M. W., Salas Fernandez, M. G., and Schnable, P. S. (2019a). Field-based robotic phenotyping of sorghum plant architecture using stereo vision. J. F. Robot. 36, 397–415.
Bao, Y., Tang, L., Srinivasan, S., and Schnable, P. S. (2019b). Field-based architectural traits characterisation of maize plant using time-of-flight 3D imaging. Biosyst. Eng. 178, 86–101. doi: 10.1016/j.biosystemseng.2018.11.005
Bao, Y., Zarecor, S., Shah, D., Tuel, T., Campbell, D. A., Chapman, A. V. E., et al. (2019c). Assessing plant performance in the Enviratron. Plant Methods 15, 1–14. doi: 10.1186/s13007-019-0504-y
Bao, Y., Tang, L., and Shah, D. (2017). “Robotic 3D plant perception and leaf probing with collision-free motion planning for automated indoor plant phenotyping,” in 2017 ASABE Annual International Meeting, Spokane, WA, 1. doi: 10.13031/aim.201700369
Barbieri, P. A., Rozas, H. n. R. S., Andrade, F. H., and Echeverria, H. n. E. (2000). Row spacing effects at different levels of nitrogen availability in maize. Agron. J. 92, 283–288.
Barth, R., Baur, J., Buschmann, T., Edan, Y., Hellström, T., Nguyen, T., et al. (2014). “Using ROS for agricultural robotics-design considerations and experiences,” in Proceedings of the Second International Conference on Robotics and Associated High-Technologies and Equipment for Agriculture and Forestry, 509–518.
Batth, R. S., Nayyar, A., and Nagpal, A. (2018). “Internet of robotic things: driving intelligent robotics of future-concept, architecture, applications and technologies,” in 2018 4th International Conference on Computing Sciences (ICCS), Jalandhar: IEEE, 151–160.
Batti, H., Ben Jabeur, C., and Seddik, H. (2020). Autonomous smart robot for path predicting and finding in maze based on fuzzy and neuro- Fuzzy approaches. Asian J. Control 23:2345.
Baweja, H. S., Parhar, T., Mirbod, O., and Nuske, S. (2018). in StalkNet: A Deep Learning Pipeline for High-Throughput Measurement of Plant Stalk Count and Stalk Width BT - Field and Service Robotics, eds M. Hutter and R. Siegwart (Cham: Springer International Publishing), 271–284.
Bayındır, L. (2016). A review of swarm robotics tasks. Neurocomputing 172, 292–321. doi: 10.1016/j.neucom.2015.05.116
Bechar, A., and Vigneault, C. (2016). Agricultural robots for field operations: concepts and components. Biosyst. Eng. 149, 94–111. doi: 10.1016/j.biosystemseng.2016.06.014
Behmann, J., Mahlein, A.-K., Paulus, S., Dupuis, J., Kuhlmann, H., Oerke, E.-C., et al. (2016). Generation and application of hyperspectral 3D plant models: methods and challenges. Mach. Vis. Appl. 27, 611–624.
Berardo, N., Brenna, O. V., Amato, A., Valoti, P., Pisacane, V., and Motto, M. (2004). Carotenoids concentration among maize genotypes measured by near infrared reflectance spectroscopy (NIRS). Innov. food Sci. Emerg. Technol. 5, 393–398.
Biber, P., Weiss, U., Dorna, M., and Albert, A. (2012). “Navigation system of the autonomous agricultural robot Bonirob,” in in Workshop on Agricultural Robotics: Enabling Safe, Efficient, and Affordable Robots for Food Production (Collocated with IROS 2012), Vilamoura.
Biskup, B., Scharr, H., Fischbach, A., Wiese-Klinkenberg, A., Schurr, U., and Walter, A. (2009). diel growth cycle of isolated leaf discs analyzed with a novel, high-throughput three-dimensional imaging method is identical to that of intact leaves. Plant Physiol. 149, 1452–1461. doi: 10.1104/pp.108.134486
Blasco, J., Aleixos, N., Roger, J. M., Rabatel, G., and Moltó, E. (2002). AE—Automation and emerging technologies: robotic weed control using machine vision. Biosyst. Eng. 83, 149–157. doi: 10.1006/bioe.2002.0109
Blender, T., Buchner, T., Fernandez, B., Pichlmaier, B., and Schlegel, C. (2016). “Managing a Mobile Agricultural Robot Swarm for a seeding task,” in IECON 2016 - 42nd Annual Conference of the IEEE Industrial Electronics Society, Florence, 6879–6886. doi: 10.1109/IECON.2016.7793638
Brambilla, M., Ferrante, E., Birattari, M., and Dorigo, M. (2013). Swarm robotics: a review from the swarm engineering perspective. Swarm Intell. 7, 1–41.
Breitzman, M. W., Bao, Y., Tang, L., Schnable, P. S., and Salas-Fernandez, M. G. (2019). Linkage disequilibrium mapping of high-throughput image-derived descriptors of plant architecture traits under field conditions. F. Crop. Res. 244:107619. doi: 10.1016/j.fcr.2019.107619
Bruzzone, L., and Quaglia, G. (2012). Locomotion systems for ground mobile robots in unstructured environments. Mech. Sci. 3, 49–62.
Burud, I., Lange, G., Lillemo, M., Bleken, E., Grimstad, L., and Johan From, P. (2017). Exploring robots and UAVs as phenotyping tools in plant breeding. IFAC-PapersOnLine 50, 11479–11484. doi: 10.1016/j.ifacol.2017.08.1591
Busemeyer, L., Mentrup, D., Möller, K., Wunder, E., Alheit, K., Hahn, V., et al. (2013). BreedVision — A multi-sensor platform for non-destructive field-based phenotyping in plant breeding. Sensors 13, 2830–2847. doi: 10.3390/s130302830
Castrillo, M., Fernandez, D., Calcagno, A. M., Trujillo, I., and Guenni, L. (2001). Responses of ribulose-1, 5-bisphosphate carboxylase, protein content, and stomatal conductance to water deficit in maize, tomato, and bean. Photosynthetica 39, 221–226.
Chamanbaz, M., Mateo, D., Zoss, B. M., Tokić, G., Wilhelm, E., Bouffanais, R., et al. (2017). Swarm-enabling technology for multi-robot systems. Front. Robot. AI 4:12. doi: 10.3389/frobt.2017.00012
Chaudhury, A., Ward, C., Talasaz, A., Ivanov, A. G., Brophy, M., Grodzinski, B., et al. (2017). Machine vision system for 3D plant phenotyping. arXiv [Preprint]. arXiv1705.00540.
Chawade, A., van Ham, J., Blomquist, H., Bagge, O., Alexandersson, E., and Ortiz, R. (2019). High-throughput field-phenotyping tools for plant breeding and precision agriculture. Agronomy 9:258.
Chen, J., Zhang, X., Xin, B., and Fang, H. (2016). Coordination between unmanned aerial and ground vehicles: a taxonomy and optimization perspective. IEEE Trans. Cybern. 46, 959–972. doi: 10.1109/TCYB.2015.2418337
Chen, Z., Wang, J., Wang, T., Song, Z., Li, Y., Huang, Y., et al. (2021). Automated in-field leaf-level hyperspectral imaging of corn plants using a Cartesian robotic platform. Comput. Electron. Agric. 183:105996.
Chlingaryan, A., Sukkarieh, S., and Whelan, B. (2018). Machine learning approaches for crop yield prediction and nitrogen status estimation in precision agriculture: a review. Comput. Electron. Agric. 151, 61–69. doi: 10.1016/j.compag.2018.05.012
Chonnaparamutt, W., Kawasaki, H., Ueki, S., Murakami, S., and Koganemaru, K. (2009). “Development of a timberjack-like pruning robot: climbing experiment and fuzzy velocity control,” in 2009 ICCAS-SICE, Fukuoka, 1195–1199.
Choudhuri, A., and Chowdhary, G. (2018). “Crop stem width estimation in highly cluttered field environment,” in Proc. Comput. Vis. Probl. Plant Phenotyping (CVPPP 2018), Newcastle, 6–13.
Cohen, Y., Huck, M. G., Hesketh, J. D., and Frederick, J. R. (1990). Sap flow in the stem of water stressed soybean and maize plants. Irrig. Sci. 11, 45–50.
Costa, C., Schurr, U., Loreto, F., Menesatti, P., and Carpentier, S. (2019). Plant phenotyping research trends, a science mapping approach. Front. Plant Sci. 9:1933.
Crowell, S., Falcão, A. X., Shah, A., Wilson, Z., Greenberg, A. J., and McCouch, S. R. (2014). High-resolution inflorescence phenotyping using a novel image-analysis pipeline, PANorama. Plant Physiol. 165, 479–495. doi: 10.1104/pp.114.238626
Das Choudhury, S., Samal, A., and Awada, T. (2019). Leveraging image analysis for high-throughput plant phenotyping. Front. Plant Sci. 10:508. doi: 10.3389/fpls.2019.00508
De Baerdemaeker, J., Munack, A., Ramon, H., and Speckmann, H. (2001). Mechatronic systems, communication, and control in precision agriculture. IEEE Control Syst. Mag. 21, 48–70. doi: 10.1016/j.aca.2020.11.008
Dhondt, S., Wuyts, N., and Inzé, D. (2013). Cell to whole-plant phenotyping: the best is yet to come. Trends Plant Sci. 18, 428–439. doi: 10.1016/j.tplants.2013.04.008
Duckett, T., Pearson, S., Blackmore, S., Grieve, B., Chen, W. H., Cielniak, G., et al. (2018). Agricultural robotics: The future of robotic agriculture. arXiv [Preprint]. arXiv1806.06762.
Duguleana, M., and Mogan, G. (2016). Neural networks based reinforcement learning for mobile robots obstacle avoidance. Exp. Syst. Appl. 62, 104–115. doi: 10.1016/j.eswa.2016.06.021
Dykes, L., Hoffmann, L. Jr., Portillo-Rodriguez, O., Rooney, W. L., and Rooney, L. W. (2014). Prediction of total phenols, condensed tannins, and 3-deoxyanthocyanidins in sorghum grain using near-infrared (NIR) spectroscopy. J. Cereal Sci. 60, 138–142.
Ecarnot, M., Ba̧czyk, P., Tessarotto, L., and Chervin, C. (2013). Rapid phenotyping of the tomato fruit model, Micro-Tom, with a portable VIS–NIR spectrometer. Plant Physiol. Biochem. 70, 159–163. doi: 10.1016/j.plaphy.2013.05.019
ElMasry, G., Wang, N., ElSayed, A., and Ngadi, M. (2007). Hyperspectral imaging for nondestructive determination of some quality attributes for strawberry. J. Food Eng. 81, 98–107.
Fahlgren, N., Feldman, M., Gehan, M. A., Wilson, M. S., Shyu, C., Bryant, D. W., et al. (2015). A versatile phenotyping system and analytics platform reveals diverse temporal responses to water availability in setaria. Mol. Plant 8, 1520–1535. doi: 10.1016/j.molp.2015.06.005
Faroq, A.-T., Adam, H., Dos Anjos, A., Lorieux, M., Larmande, P., Ghesquière, A., et al. (2013). P-TRAP: a panicle trait phenotyping tool. BMC Plant Biol. 13:122. doi: 10.1186/1471-2229-13-122
Fernandez, M. G. S., Bao, Y., Tang, L., and Schnable, P. S. (2017). A high-throughput, field-based phenotyping technology for tall biomass crops. Plant Physiol. 174, 2008–2022. doi: 10.1104/pp.17.00707
Fiorani, F., and Schurr, U. (2013). Future scenarios for plant phenotyping. Annu. Rev. Plant Biol. 64, 267–291. doi: 10.1146/annurev-arplant-050312-120137
Fischer, G. (2009). “World food and agriculture to 2030/50,” in Technical Paper From the Expert Meeting on How to Feed the World in, Rome, 24–26.
Foix, S., Alenyà, G., and Torras, C. (2015). “3D Sensor planning framework for leaf probing,” in 2015 IEEE/RSJ International Conference on Intelligent Robots and Systems (IROS), Hamburg, 6501–6506. doi: 10.1109/IROS.2015.7354306
Foix, S., Alenyà, G., and Torras, C. (2018). Task-driven active sensing framework applied to leaf probing. Comput. Electron. Agric. 147, 166–175. doi: 10.1016/j.compag.2018.01.020
Franceschetti, A., Tosello, E., Castaman, N., and Ghidoni, S. (2018). Robotic Arm Control and Task Training through Deep Reinforcement Learning.
Furbank, R. T., and Tester, M. (2011). Phenomics – technologies to relieve the phenotyping bottleneck. Trends Plant Sci. 16, 635–644. doi: 10.1016/j.tplants.2011.09.005
Gage, J. L., Miller, N. D., Spalding, E. P., Kaeppler, S. M., and de Leon, N. (2017). TIPS: a system for automated image-based phenotyping of maize tassels. Plant Methods 13:21. doi: 10.1186/s13007-017-0172-8
Gage, J. L., Richards, E., Lepak, N., Kaczmar, N., Soman, C., Chowdhary, G., et al. (2019). In-field whole plant maize architecture characterized by latent space phenotyping. bioRxiv [Preprint]. doi: 10.1101/763342
Gao, T., Emadi, H., Saha, H., Zhang, J., Lofquist, A., Singh, A., et al. (2018). A novel multirobot system for plant phenotyping. Robotics 7:61. doi: 10.3390/robotics7040061
Ge, Y., Atefi, A., Zhang, H., Miao, C., Ramamurthy, R. K., Sigmon, B., et al. (2019). High-throughput analysis of leaf physiological and chemical traits with VIS–NIR–SWIR spectroscopy: a case study with a maize diversity panel. Plant Methods 15:66. doi: 10.1186/s13007-019-0450-8
Ge, Y., Bai, G., Stoerger, V., and Schnable, J. C. (2016). Temporal dynamics of maize plant growth, water use, and leaf water content using automated high throughput RGB and hyperspectral imaging. Comput. Electron. Agric. 127, 625–632. doi: 10.1016/j.compag.2016.07.028
Gegas, V. C., Nazari, A., Griffiths, S., Simmonds, J., Fish, L., Orford, S., et al. (2010). A genetic framework for grain size and shape variation in wheat. Plant Cell 22, 1046–1056. doi: 10.1105/tpc.110.074153
Gonzalez-de-Soto, M., Emmi, L., Perez-Ruiz, M., Aguera, J., and Gonzalez-de-Santos, P. (2016). Autonomous systems for precise spraying – Evaluation of a robotised patch sprayer. Biosyst. Eng. 146, 165–182. doi: 10.1016/j.biosystemseng.2015.12.018
Granier, C., and Tardieu, F. (2009). Multi-scale phenotyping of leaf expansion in response to environmental changes: the whole is more than the sum of parts. Plant. Cell Environ. 32, 1175–1184. doi: 10.1111/j.1365-3040.2009.01955.x
Grieco, L. A., Rizzo, A., Colucci, S., Sicari, S., Piro, G., Di Paola, D., et al. (2014). IoT-aided robotics applications: technological implications, target domains and open issues. Comput. Commun. 54, 32–47.
Grift, T., Zhang, Q., Kondo, N., and Ting, K. C. (2008). A review of automation and robotics for the bio-industry. J. Biomechatronics Eng. 1, 37–54.
Grimstad, L., and From, P. J. (2017). Thorvald II - a modular and re-configurable agricultural robot. IFAC PapersOnLine 50, 4588–4593. doi: 10.1016/j.ifacol.2017.08.1005
Guo, Z., Huang, W., Chen, L., Wang, X., and Peng, Y. (2013). “Nondestructive evaluation of soluble solid content in strawberry by near infrared spectroscopy,” in Piageng 2013: Image Processing and Photonics for Agricultural Engineering, (Bellingham: International Society for Optics and Photonics), 87610O.
Han, L., Yang, G., Yang, H., Xu, B., Li, Z., and Yang, X. (2018). Clustering field-based maize phenotyping of plant-height growth and canopy spectral dynamics using a UAV remote-sensing approach. Front. Plant Sci. 9:1638. doi: 10.3389/fpls.2018.01638
Happ, M. M., Wang, H., Graef, G. L., and Hyten, D. L. (2019). Generating high density, low cost genotype data in soybean [Glycine max (L.) Merr.]. G3 Genes Genomes Genet. 9, 2153–2160. doi: 10.1534/g3.119.400093
Hassan, M. A., Yang, M., Rasheed, A., Yang, G., Reynolds, M., Xia, X., et al. (2019). A rapid monitoring of NDVI across the wheat growth cycle for grain yield prediction using a multi-spectral UAV platform. Plant Sci. 282, 95–103. doi: 10.1016/j.plantsci.2018.10.022
Hassanijalilian, O., Igathinathane, C., Bajwa, S., and Nowatzki, J. (2020a). Rating iron deficiency in soybean using image processing and decision-tree based models. Remote Sens. 12:4143.
Hassanijalilian, O., Igathinathane, C., Doetkott, C., Bajwa, S., Nowatzki, J., and Haji Esmaeili, S. A. (2020b). Chlorophyll estimation in soybean leaves infield with smartphone digital imaging and machine learning. Comput. Electron. Agric. 174:105433. doi: 10.1016/j.compag.2020.10543
Hayashi, S., Shigematsu, K., Yamamoto, S., Kobayashi, K., Kohno, Y., Kamata, J., et al. (2010). Evaluation of a strawberry-harvesting robot in a field test. Biosyst. Eng. 105, 160–171. doi: 10.1016/j.biosystemseng.2009.09.011
Hejazipoor, H., Massah, J., Soryani, M., Vakilian, K. A., and Chegini, G. (2021). An intelligent spraying robot based on plant bulk volume. Comput. Electron. Agric. 180:105859.
Hemming, J., Bac, C. W., van Tuijl, B. A. J., Barth, R., Bontsema, J., Pekkeriet, E. J., et al. (2014). “A robot for harvesting sweet-pepper in greenhouses,” in Paper Presented at AgEng 2014, Zurich.
Hughes, J., Culha, U., Giardina, F., Guenther, F., Rosendo, A., and Iida, F. (2016). Soft manipulators and grippers: a review. Front. Robot. AI 3:69.
Iqbal, J., Xu, R., Halloran, H., and Li, C. (2020). Development of a Multi-Purpose Autonomous Differential Drive Mobile Robot for Plant Phenotyping and Soil Sensing. Electronics 9:1550.
Irshat, K., Petr, R., and Irina, R. (2018). “The selecting of artificial intelligence technology for control of mobile robots,” in 2018 International Multi-Conference on Industrial Engineering and Modern Technologies (FarEastCon), Vladivostok: IEEE, 1–4.
Ishigure, Y., Hirai, K., and Kawasaki, H. (2013). “A pruning robot with a power-saving chainsaw drive,” in 2013 IEEE International Conference on Mechatronics and Automation, Takamatsu, 1223–1228. doi: 10.1109/ICMA.2013.6618088
Jannink, J.-L., Lorenz, A. J., and Iwata, H. (2010). Genomic selection in plant breeding: from theory to practice. Brief. Funct. Genomics 9, 166–177. doi: 10.1093/bfgp/elq001
Jay, S., Rabatel, G., Hadoux, X., Moura, D., and Gorretta, N. (2015). In-field crop row phenotyping from 3D modeling performed using structure from Motion. Comput. Electron. Agric. 110, 70–77. doi: 10.1016/j.compag.2014.09.021
Jenkins, M., and Kantor, G. (2017). “Online detection of occluded plant stalks for manipulation,” in Proceedings of the 2017 IEEE/RSJ International Conference on Intelligent Robots and Systems (IROS), 5162–5167. doi: 10.1109/IROS.2017.8206404
Jin, X., Zarco-Tejada, P., Schmidhalter, U., Reynolds, M. P., Hawkesford, M. J., Varshney, R. K., et al. (2020). High-throughput estimation of crop traits: a review of ground and aerial phenotyping platforms. IEEE Geosci. Remote Sens. Mag. 2, 1–33.
Kicherer, A., Herzog, K., Pflanz, M., Wieland, M., Rüger, P., Kecke, S., et al. (2015). An automated field phenotyping pipeline for application in grapevine research. Sensors 15, 4823–4836. doi: 10.3390/s150304823
Kim, G.-H., Kim, S.-C., Hong, Y.-K., Han, K.-S., and Lee, S.-G. (2012). “A robot platform for unmanned weeding in a paddy field using sensor fusion,” in Proceedings of the 2012 IEEE International Conference on Automation Science and Engineering (CASE), (Piscataway, NJ: IEEE), 904–907.
Kong, E., Liu, D., Guo, X., Yang, W., Sun, J., Li, X., et al. (2013). Anatomical and chemical characteristics associated with lodging resistance in wheat. Crop J. 1, 43–49. doi: 10.1016/j.cj.2013.07.012
Lee, U., Chang, S., Putra, G. A., Kim, H., and Kim, D. H. (2018). An automated, high-throughput plant phenotyping system using machine learning-based plant segmentation and image analysis. PLoS One 13:e0196615. doi: 10.1371/journal.pone.0196615
Leilah, A. A., and Al-Khateeb, S. A. (2005). Statistical analysis of wheat yield under drought conditions. J. Arid Environ. 61, 483–496. doi: 10.1016/j.jaridenv.2004.10.011
Li, L., Zhang, Q., and Huang, D. (2014). A review of imaging techniques for plant phenotyping. Sensors 14, 20078–20111. doi: 10.3390/s141120078
Li, M., Imou, K., Wakabayashi, K., and Yokoyama, S. (2009). Review of research on agricultural vehicle autonomous guidance. Int. J. Agric. Biol. Eng. 2, 1–16.
Li, Z., Guo, R., Li, M., Chen, Y., and Li, G. (2020a). A review of computer vision technologies for plant phenotyping. Comput. Electron. Agric. 176:105672.
Li, Z., Zhang, Q., Li, J., Yang, X., Wu, Y., Zhang, Z., et al. (2020b). Solar-induced chlorophyll fluorescence and its link to canopy photosynthesis in maize from continuous ground measurements. Remote Sens. Environ. 236:111420. doi: 10.1016/j.rse.2019.111420
Lili, W., Bo, Z., Jinwei, F., Xiaoan, H., Shu, W., Yashuo, L., et al. (2017). Development of a tomato harvesting robot used in greenhouse. Int. J. Agric. Biol. Eng. 10, 140–149.
Lopes, C. M., Graça, J., Sastre, J., Reyes, M., Guzmán, R., Braga, R., et al. (2016). “Vineyard yeld estimation by VINBOT robot-preliminary results with the white variety Viosinho,” in Proceedings 11th Int. Terroir Congress, eds G. Jones and N. Doran (Ashland, USA: Southern Oregon University), 458–463.
Lu, H., Tang, L., Whitham, A. S., and Mei, Y. (2017). A robotic platform for corn seedling morphological traits characterization. Sensors 17:2082. doi: 10.3390/s17092082
Mancini, M., Mazzoni, L., Gagliardi, F., Balducci, F., Duca, D., Toscano, G., et al. (2020). Application of the non-destructive NIR technique for the evaluation of strawberry fruits quality parameters. Foods 9:441. doi: 10.3390/foods9040441
McMullen, M. D., Kresovich, S., Villeda, H. S., Bradbury, P., Li, H., Sun, Q., et al. (2009). Genetic properties of the maize nested association mapping population. Science 325, 737–740. doi: 10.1126/science.1174320
Mishra, K. B., Mishra, A., Klem, K., and Govindjee. (2016). Plant phenotyping: a perspective. Indian J. Plant Physiol. 21, 514–527. doi: 10.1007/s40502-016-0271-y
Mishra, P., Asaari, M. S. M., Herrero-Langreo, A., Lohumi, S., Diezma, B., and Scheunders, P. (2017). Close range hyperspectral imaging of plants: a review. Biosyst. Eng. 164, 49–67.
Moeinizade, S., Hu, G., Wang, L., and Schnable, P. S. (2019). Optimizing selection and mating in genomic selection with a look-ahead approach: an operations research framework. G3 Genes, Genomes, Genet. 9, 2123–2133.
Montes, J. M., Melchinger, A. E., and Reif, J. C. (2007). Novel throughput phenotyping platforms in plant genetic studies. Trends Plant Sci. 12, 433–436. doi: 10.1016/j.tplants.2007.08.006
Mueller-Sim, T., Jenkins, M., Abel, J., and Kantor, G. (2017). “The robotanist: a ground-based agricultural robot for high-throughput crop phenotyping,” in 2017 IEEE International Conference on Robotics and Automation (ICRA), Singapore, 3634–3639. doi: 10.1109/ICRA.2017.7989418
Mutka, A. M., and Bart, R. S. (2015). Image-based phenotyping of plant disease symptoms. Front. Plant Sci. 5:734. doi: 10.3389/fpls.2014.00734
Narvaez, F. Y., Reina, G., Torres-Torriti, M., Kantor, G., and Cheein, F. A. (2017). A survey of ranging and imaging techniques for precision agriculture phenotyping. IEEE ASME Trans. Mechatronics 22, 2428–2439.
Nishizawa, T., Mori, Y., Fukushima, S., Natsuga, M., and Maruyama, Y. (2009). Non-destructive analysis of soluble sugar components in strawberry fruits using near-infrared spectroscopy. Nippon Shokuhin Kagaku Kogaku KaishiJ. Japanese Soc. Food Sci. Technol. 56, 229–235.
Oberti, R., Marchi, M., Tirelli, P., Calcante, A., Iriti, M., Tona, E., et al. (2016). Selective spraying of grapevines for disease control using a modular agricultural robot. Biosyst. Eng. 146, 203–215. doi: 10.1016/j.biosystemseng.2015.12.004
Ohashi, Y., Nakayama, N., Saneoka, H., and Fujita, K. (2006). Effects of drought stress on photosynthetic gas exchange, chlorophyll fluorescence and stem diameter of soybean plants. Biol. Plant. 50, 138–141.
Palazzari, V., Mezzanotte, P., Alimenti, F., Fratini, F., Orecchini, G., and Roselli, L. (2017). Leaf compatible “eco-friendly” temperature sensor clip for high density monitoring wireless networks. Wirel. Power Transf. 4, 55–60.
Pandey, P., Dakshinamurthy, H. N., and Young, S. N. (2021). Autonomy in detection, actuation, and planning for robotic weeding systems. Trans. ASABE.
Panozzo, J. F., Eagles, H. A., Cawood, R. J., and Wootton, M. (1999). Wheat spike temperatures in relation to varying environmental conditions. Aust. J. Agric. Res. 50, 997–1006. doi: 10.1371/journal.pone.0189594
Park, J., Delgado, R., and Choi, B. W. (2020). Real-time characteristics of ROS 2.0 in multiagent robot systems: an empirical study. IEEE Access 8, 154637–154651.
Parhar, T., Baweja, H., Jenkins, M., and Kantor, G. (2018). “A deep learning-based stalk grasping pipeline,” in 2018 IEEE International Conference on Robotics and Automation (ICRA), Brisbane, QLD: IEEE, 6161–6167.
Penchaiya, P., Bobelyn, E., Verlinden, B. E., Nicolaï, B. M., and Saeys, W. (2009). Non-destructive measurement of firmness and soluble solids content in bell pepper using NIR spectroscopy. J. Food Eng. 94, 267–273.
Pieruschka, R., and Schurr, U. (2019). Plant phenotyping: past, present, and future. Plant Phenomics 2019, 1–6.
Pound, M. P., Atkinson, J. A., Townsend, A. J., Wilson, M. H., Griffiths, M., Jackson, A. S., et al. (2017). Deep machine learning provides state-of-the-art performance in image-based plant phenotyping. Gigascience 6:gix083.
Qiu, Q., Sun, N., Bai, H., Wang, N., Fan, Z., Wang, Y., et al. (2019). Field-based high-throughput phenotyping for maize plant using 3D LiDAR point cloud generated with a “Phenomobile.”. Front. Plant Sci. 10:554. doi: 10.3389/fpls.2019.00554
Qiu, R., Wei, S., Zhang, M., Li, H., Sun, H., Liu, G., et al. (2018). Sensors for measuring plant phenotyping: a review. Int. J. Agric. Biol. Eng. 11, 1–17.
Quaglia, G., Visconte, C., Scimmi, L. S., Melchiorre, M., Cavallone, P., and Pastorelli, S. (2020). Design of a UGV powered by solar energy for precision agriculture. Robotics 9:13.
Raja, R., Nguyen, T. T., Slaughter, D. C., and Fennimore, S. A. (2020). Real-time robotic weed knife control system for tomato and lettuce based on geometric appearance of plant labels. Biosyst. Eng. 194, 152–164.
Rahaman, M. M., Chen, D., Gillani, Z., Klukas, C., and Chen, M. (2015). Advanced phenotyping and phenotype data analysis for the study of plant growth and development. Front. Plant Sci. 6:619. doi: 10.3389/fpls.2015.00619
Ray, P. P. (2016). Internet of robotic things: concept, technologies, and challenges. IEEE Access 4, 9489–9500.
Richards, R. A., Rebetzke, G. J., Watt, M., Condon, A. G., Spielmeyer, W., and Dolferus, R. (2010). Breeding for improved water productivity in temperate cereals: phenotyping, quantitative trait loci, markers and the selection environment. Funct. Plant Biol. 37, 85–97. doi: 10.1071/FP09219
Roldán, J. J., Garcia-Aunon, P., Garzón, M., De León, J., Del Cerro, J., and Barrientos, A. (2016). Heterogeneous multi-robot system for mapping environmental variables of greenhouses. Sensors 16:1018. doi: 10.3390/s16071018
Ruckelshausen, A., Biber, P., Dorna, M., Gremmes, H., Klose, R., Linz, A., et al. (2009). BoniRob–an autonomous field robot platform for individual plant phenotyping. Precis. Agric. 9, 841–847. doi: 10.3390/s17010214
Rudolph, R., Herzog, K., Töpfer, R., and Steinhage, V. (2019). Efficient identification, localization and quantification of grapevine inflorescences and flowers in unprepared field images using Fully Convolutional Networks. Vitis 58, 95–104.
Sandhu, J., Zhu, F., Paul, P., Gao, T., Dhatt, B. K., Ge, Y., et al. (2019). PI-Plat: a high-resolution image-based 3D reconstruction method to estimate growth dynamics of rice inflorescence traits. Plant Methods 15:162. doi: 10.1186/s13007-019-0545-2
Saravanan, M., Perepu, S. K., and Sharma, A. (2018). “Exploring collective behavior of internet of robotic things for indoor plant health monitoring,” in 2018 IEEE International Conference on Internet of Things and Intelligence System (IOTAIS), Bali: IEEE, 148–154.
Schulz, H., and Baranska, M. (2007). Identification and quantification of valuable plant substances by IR and Raman spectroscopy. Vib. Spectrosc. 43, 13–25. doi: 10.1016/j.vibspec.2006.06.001
Shafiekhani, A., Kadam, S., Fritschi, B. F., and DeSouza, N. G. (2017). Vinobot and vinoculer: two robotic platforms for high-throughput field phenotyping. Sensors 17:214. doi: 10.3390/s17010214
Shah, D., Tang, L., Gai, J., and Putta-Venkata, R. (2016). Development of a mobile robotic phenotyping system for growth chamber-based studies of genotype x environment interactions. IFAC PapersOnLine 49, 248–253. doi: 10.1016/j.ifacol.2016.10.046
Shahrimie, M. A. M., Mishra, P., Mertens, S., Dhondt, S., Wuyts, N., and Scheunders, P. (2016). “Modeling effects of illumination and plant geometry on leaf reflectance spectra in close-range hyperspectral imaging,” in 2016 8th Workshop on Hyperspectral Image and Signal Processing: Evolution in Remote Sensing (WHISPERS), Los Angeles, CA: IEEE, 1–4.
Shalal, N., Low, T., McCarthy, C., and Hancock, N. (2013). A Review of Autonomous Navigation Systems in Agricultural Environments.
Shamshiri, R. R., Weltzien, C., Hameed, I. A., Yule, I. J., Grift, T. E., Balasundram, S. K., et al. (2018). Research and development in agricultural robotics: a perspective of digital farming. Int. J. Agric. Biol. Eng. 11, 1–14.
Shao, Y., and He, Y. (2008). Nondestructive measurement of acidity of strawberry using Vis/NIR spectroscopy. Int. J. Food Prop. 11, 102–111.
Silwal, A., Davidson, J. R., Karkee, M., Mo, C., Zhang, Q., and Lewis, K. (2017). Design, integration, and field evaluation of a robotic apple harvester. J. F. Robot. 34, 1140–1159. doi: 10.1002/rob.21715
Singh, A., Ganapathysubramanian, B., Singh, A. K., and Sarkar, S. (2016). Machine learning for high-throughput stress phenotyping in plants. Trends Plant Sci. 21, 110–124.
Smitt, C., Halstead, M., Zaenker, T., Bennewitz, M., and McCool, C. (2020). PATHoBot: A Robot for Glasshouse Crop Phenotyping and Intervention. arXiv [Preprint]. arXiv2010.16272.
Taghavifar, H., Xu, B., Taghavifar, L., and Qin, Y. (2019). Optimal path-planning of nonholonomic terrain robots for dynamic obstacle avoidance using single-time velocity estimator and reinforcement learning approach. IEEE Access 7, 159347–159356.
Tan, Y., and Zheng, Z. (2013). Research advance in swarm robotics. Def. Technol. 9, 18–39. doi: 10.1016/j.dt.2013.03.001
Tripodi, P., Massa, D., Venezia, A., and Cardi, T. (2018). Sensing technologies for precision phenotyping in vegetable crops: current status and future challenges. Agronomy 8:57.
Tsubo, M., and Walker, S. (2002). A model of radiation interception and use by a maize–bean intercrop canopy. Agric. For. Meteorol. 110, 203–215.
Ubbens, J., Cieslak, M., Prusinkiewicz, P., Parkin, I., Ebersbach, J., and Stavness, I. (2020). Latent space phenotyping: automatic image-based phenotyping for treatment studies. Plant Phenomics 2020:5801869. doi: 10.34133/2020/5801869
Underwood, J., Wendel, A., Schofield, B., McMurray, L., and Kimber, R. (2017). Efficient in-field plant phenomics for row-crops with an autonomous ground vehicle. J. F. Robot. 34, 1061–1083.
van Henten, E. J., Hemming, J., van Tuijl, B. A. J., Kornet, J. G., Meuleman, J., Bontsema, J., et al. (2002). An autonomous robot for harvesting cucumbers in greenhouses. Auton. Robots 13, 241–258. doi: 10.1023/A:1020568125418
Vázquez-Arellano, M., Paraforos, D. S., Reiser, D., Garrido-Izard, M., and Griepentrog, H. W. (2018). Determination of stem position and height of reconstructed maize plants using a time-of-flight camera. Comput. Electron. Agric. 154, 276–288.
Vidoni, R., Gallo, R., Ristorto, G., Carabin, G., Mazzetto, F., Scalera, L., et al. (2017). “ByeLab: An agricultural mobile robot prototype for proximal sensing and precision farming,” in ASME International Mechanical Engineering Congress and Exposition, (New York, NY: American Society of Mechanical Engineers), V04AT05A057.
Vijayarangan, S., Sodhi, P., Kini, P., Bourne, J., Du, S., Sun, H., et al. (2018). in High-Throughput Robotic Phenotyping of Energy Sorghum Crops BT - Field and Service Robotics, eds M. Hutter and R. Siegwart (Cham: Springer International Publishing), 99–113.
Virlet, N., Sabermanesh, K., Sadeghi-Tehran, P., and Hawkesford, M. J. (2017). Field scanalyzer: an automated robotic field phenotyping platform for detailed crop monitoring. Funct. Plant Biol. 44, 143–153. doi: 10.1071/FP16163
Vu, Q., Raković, M., Delic, V., and Ronzhin, A. (2018). “Trends in development of UAV-UGV cooperation approaches,” in Precision Agriculture BT - Interactive Collaborative Robotics, eds A. Ronzhin, G. Rigoll, and R. Meshcheryakov (Cham: Springer International Publishing), 213–221.
Walter, A., Liebisch, F., and Hund, A. (2015). Plant phenotyping: from bean weighing to image analysis. Plant Methods 11:14. doi: 10.1186/s13007-015-0056-8
Wang, H., Peng, J., Xie, C., Bao, Y., and He, Y. (2015). Fruit quality evaluation using spectroscopy technology: a review. Sensors 15, 11889–11927.
Wang, L., Zhu, G., Johnson, W., and Kher, M. (2018). Three new approaches to genomic selection. Plant Breed. 137, 673–681.
Wei, H., Shao, Z., Huang, Z., Chen, R., Guan, Y., Tan, J., et al. (2016). RT-ROS: a real-time ROS architecture on multi-core processors. Futur. Gener. Comput. Syst. 56, 171–178. doi: 10.1016/j.future.2015.05.008
Weiss, U., and Biber, P. (2011). Plant detection and mapping for agricultural robots using a 3D LIDAR sensor. Rob. Auton. Syst. 59, 265–273. doi: 10.1016/j.robot.2011.02.011
White, J. W., Andrade-Sanchez, P., Gore, M. A., Bronson, K. F., Coffelt, T. A., Conley, M. M., et al. (2012). Field-based phenomics for plant genetics research. F. Crop. Res. 133, 101–112. doi: 10.1016/j.fcr.2012.04.003
Wolfert, S., Ge, L., Verdouw, C., and Bogaardt, M.-J. (2017). Big data in smart farming – a review. Agric. Syst. 153, 69–80. doi: 10.1016/j.agsy.2017.01.023
Wu, C., Zeng, R., Pan, J., Wang, C. C. L., and Liu, Y. (2019). Plant phenotyping by deep-learning-based planner for multi-robots. IEEE Robot. Autom. Lett. 4, 3113–3120. doi: 10.1109/LRA.2019.2924125
Wu, G. F., Huang, L. X., and He, Y. (2008). Research on the sugar content measurement of grape and berries by using Vis/NIR spectroscopy technique. Guang pu xue yu Guang pu fen xiGuang pu 28, 2090–2093.
Würschum, T. (2019). “Modern field phenotyping opens new avenues for selection,” in Applications of Genetic and Genomic Research in Cereals, eds T. Miedaner and V. Korzun (Amsterdam: Elsevier), 233–250.
Xiong, Y., Ge, Y., Grimstad, L., and From, P. J. (2020). An autonomous strawberry- harvesting robot: Design, development, integration, and field evaluation. J. F. Robot. 37, 202–224.
Xiong, B., Wang, B., Xiong, S., Lin, C., and Yuan, X. (2019). 3D morphological processing for wheat spike phenotypes using computed tomography images. Remote Sens. 11:1110.
Xu, R., Li, C., and Mohammadpour Velni, J. (2018). Development of an autonomous ground robot for field high throughput phenotyping. IFAC-PapersOnLine 51, 70–74. doi: 10.1016/j.ifacol.2018.08.063
Young, S. N., Kayacan, E., and Peschel, J. M. (2019). Design and field evaluation of a ground robot for high-throughput phenotyping of energy sorghum. Precis. Agric. 20, 697–722. doi: 10.1007/s11119-018-9601-6
Yuan, H., Wang, N., Bennett, R., Burditt, D., Cannon, A., and Chamberlin, K. (2018). Development of a ground-based peanut canopy phenotyping system. IFAC PapersOnLine 51, 162–165. doi: 10.1016/j.ifacol.2018.08.081
Zahid, A., Mahmud, M. S., He, L., Choi, D., Heinemann, P., and Schupp, J. (2020). Development of an integrated 3R end-effector with a cartesian manipulator for pruning apple trees. Comput. Electron. Agric. 179:105837.
Zhang, F., Leitner, J., Milford, M., Upcroft, B., and Corke, P. (2015). Towards vision-based deep reinforcement learning for robotic motion control. arXiv [Preprint]. arXiv1511.03791.
Zhang, J., Gong, L., Liu, C., Huang, Y., Zhang, D., and Yuan, Z. (2016). Field phenotyping robot design and validation for the crop breeding. IFAC PapersOnLine 49, 281–286. doi: 10.1016/j.ifacol.2016.10.052
Zhang, Q., Karkee, M., and Tabb, A. (2019). The use of agricultural robots in orchard management. arXiv [Preprint]. arXiv1907.13114.
Zhang, Z., Kayacan, E., Thompson, B., and Chowdhary, G. (2020). High precision control and deep learning-based corn stand counting algorithms for agricultural robot. Auton. Robots 44, 1289–1302.
Zhao, J., Mantilla Perez, M. B., Hu, J., and Salas Fernandez, M. G. (2016). Genome- wide association study for nine plant architecture traits in sorghum. Plant Genome 9, 1–14. doi: 10.3835/plantgenome2015.06.0044
Keywords: autonomous robotic technology, agricultural robotics, phenotyping robot, high-throughput plant phenotyping, computer vision
Citation: Atefi A, Ge Y, Pitla S and Schnable J (2021) Robotic Technologies for High-Throughput Plant Phenotyping: Contemporary Reviews and Future Perspectives. Front. Plant Sci. 12:611940. doi: 10.3389/fpls.2021.611940
Received: 29 September 2020; Accepted: 14 May 2021;
Published: 25 June 2021.
Edited by:
Ankush Prashar, Newcastle University, United KingdomReviewed by:
Simon Pearson, University of Lincoln, United KingdomKatja Herzog, Institut für Rebenzüchtung, Julius Kühn-Institut, Germany
Rui Xu, University of Georgia, Georgia
Copyright © 2021 Atefi, Ge, Pitla and Schnable. This is an open-access article distributed under the terms of the Creative Commons Attribution License (CC BY). The use, distribution or reproduction in other forums is permitted, provided the original author(s) and the copyright owner(s) are credited and that the original publication in this journal is cited, in accordance with accepted academic practice. No use, distribution or reproduction is permitted which does not comply with these terms.
*Correspondence: Yufeng Ge, eWdlMkB1bmwuZWR1