- 1Institute of Animal Science, Chinese Academy of Agricultural Sciences, Beijing, China
- 2Institute of Animal Science, Ningxia Academy of Agricultural and Forestry Sciences, Yinchuan, China
Forage quality determined mainly by protein content and fiber composition has a crucial influence on digestibility and nutrition intake for animal feeding. To explore the genetic basis of quality traits, we conducted QTL mapping based on the phenotypic data of crude protein (CP), neutral detergent fiber (NDF), acid detergent fiber (ADF), and lignin of an F1 alfalfa population generated by crossing of two alfalfa parents with significant difference in quality. In total, 83 QTLs were identified with contribution to the phenotypic variation (PVE) ranging from 1.45 to 14.35%. Among them, 47 QTLs interacted significantly with environment and 12 QTLs were associated with more than one trait. Epistatic effect was also detected for 73 pairs of QTLs with PVE of 1.08–14.06%. The results suggested that the inheritance of quality-related traits was jointly affected by additive, epistasis and environment. In addition, 83.33% of the co-localized QTLs were shared by ADF and NDF with the same genetic direction, while the additive effect of crude protein-associated QTLs was opposite to that fiber composition on the same locus, suggesting that the loci may antagonistically contribute to protein content and fiber composition. Further analysis of a QTL related to all the three traits of fiber composition (qNDF1C, qADF1C-2, and qlignin1C-2) showed that five candidate genes were homologs of cellulose synthase-like protein A1 in Medicago truncatula, indicating the potential role in fiber synthesis. For the protein-associated loci we identified, qCP4C-1 was located in the shortest region (chr 4.3 39.3–39.4 Mb), and two of the seven corresponding genes in this region were predicted to be E3 ubiquitin-protein ligase in protein metabolism. Therefore, our results provide some reliable regions significantly associated with alfalfa quality, and identification of the key genes would facilitate marker-assisted selection for favorable alleles in breeding program of alfalfa quality improvement.
Introduction
Crude protein and fiber components are two key indicators of forage quality, which affects digestibility and nutrition intake for animal feeding. Perennial legume alfalfa (Medicago sativa L.) has become an important forage in the diet of ruminants due to its high protein content (15–20%), as well as vitamins and minerals (Annicchiarico et al., 2015). Improvement of alfalfa’s protein content lowers the cost of raising of ruminants. Generally, fiber component of alfalfa is consisted of neutral detergent fiber (NDF), acid detergent fiber (ADF), and lignin. Among them, NDF is negatively correlated with feed intake of animals (Oba and Allen, 1999), and high content of ADF or lignin lowers digestibility (Sarwar et al., 1999). Therefore, breeding alfalfa new varieties with improved quality has been a major focus of the forage breeders worldwide.
Forage quality is affected by both genetic and environmental factors (Griffin et al., 1994; Palmonari et al., 2014; Liu et al., 2018; Yang et al., 2019). A lot of progress has been made in improving alfalfa quality by genetic manipulation and optimization of cultivation strategy. For example, protein content in alfalfa was increased by overexpressing of glutamate synthase or glutamine synthase, two enzymes involving in ammonium assimilation (Vance et al., 1995; Kaur et al., 2019). Reduction of lignin content was achieved by down-regulating lignin synthesis genes such as, hydroxycinnamoyl -CoA:shikimate hydroxycinnamoyl transferase (HCT), coumarate 3-hydroxylase (C3H), cinnamyl alcohol dehydrogenase (CAD), caffeic acid 3-O-methyltransferase (COMT), and caffeoyl CoA 3-O-methyltransferase (CCoAOMT) (Baucher et al., 1999; Marita et al., 2003; Nakashima et al., 2008; Tong et al., 2015; Gallego-Giraldo et al., 2016). In addition, suitable field management of seasonal fertilization and irrigation, is beneficial for alfalfa growth and enables a better balance of protein and fiber content (Bacenetti et al., 2018).
Since the proposal of QTL interval mapping (IM) in 1989 (Lander and Botstein, 1989), QTL mapping has become a focus of quantitative genetics research. There have been a number of QTL studies in other crops, such as rice and soybean, for these quality-related traits (Zhang et al., 2008, 2018; Warrington et al., 2015; Bazrkarkhatibani et al., 2019). For example, 47 QTLs related to fiber were identified in a corn RIL population (Li et al., 2017), and 14 QTLs related to grain protein content were mapped in a rice BC3F4 population, and gluten family genes were identified from qGPC1.1 (Chattopadhyay et al., 2019).
QTL research in alfalfa is rather slow primarily due to its nature of heterozygosity and autotetraploidy which hampers the construction of genetic maps. It has been documented that the linkage distance between molecular markers could be estimated using single dose alleles (SDA) with a separation ratio of 1:1 in alfalfa (Brouwer and Osborn, 1999). Based on SDA, using this method, a series of alfalfa genetic maps containing eight chromosomes have been constructed (Sledge et al., 2005). In 2014, a saturated genetic linkage map was developed using 3,591 single nucleotide polymorphism (SNP) markers of alfalfa (Li et al., 2014). Some of the linkage maps have been applied to analyze the agriculturally important traits especially yield, fall dormancy and stress tolerance to advert environmental conditions (Ray et al., 2015; Adhikari et al., 2018; Zhang et al., 2019). Used the method described by Zhang et al. (2020), we previously also constructed an alfalfa linkage map containing 3,818 SNP markers obtained by genotyping-by-sequencing (GBS) of an F1 population of about 392 individual plants. To identified the key QTLs or candidate genes associated with alfalfa quality, here we conducted QTL mapping based on our 3-year data of crude protein content and fiber composition of the F1 population. Our results provide useful information on reliable candidate locus for alfalfa quality improvement.
Materials and Methods
Plant Materials and Growth Conditions
Alfalfa materials used in this study were an F1 population of 392 individuals generated by crossing of a landrace “Cangzhou” (the paternal parent) with Zhongmu No.1 (the maternal parent) as described previously (Zhang et al., 2020).
The field trials were conducted at the research base of the Chinese Academy of Agricultural Sciences in Langfang, Hebei province. The average temperature annually is 11.9°C, the average temperature in the coldest month (January) is −4.7°C, and in the hottest month (July) is 26.2°C. The annual precipitation is 554.9 mm. The soil is medium loam with 1.69% organic matter and a pH of 7.37.
Phenotyping
Seedlings including F1 population and the parental plants were cultured in greenhouse under conditions of 16 h day/8 h night, 22°C, and 40% relative humidity. Clones were generated via stem cuttings and transplanted into field in early April of 2014 with three clones as replicates randomly planted in three adjacent blocks. Row spacing is 100 cm and column spacing 80 cm. Plants were clipped late fall with the ground retention height of 5 cm. No application of fertilizer or irrigation was carried out for the field.
The first cut of 2016, 2019, and 2020 with a height of ground stubble at 5 cm was used for phenotypic data collection. Samples were oven-dried (60°C, 24 h), and ground to pass through a 1-mm sieve. The content of crude protein, NDF, ADF, and lignin was measured using near-infrared reflectance spectroscopy (Foss NIRS 1650) with an analytical model corrected by chemically measured data of 20 alfalfa samples. SAS 9.4 was performed to analyze the mean comparison of the parental phenotypic data and the normal distribution test (variation range, mean, variance, skewness, and kurtosis) and correlation analysis for the crude protein, NDF, ADF, and lignin of F1 population. The frequency distribution of the F1 population phenotypic data was plotted using SPSS 18.0 (Baarda and van Dijkum, 2019). The broad sense heritability (H2) of each trait was calculated using the ANOVA function of the software QTL-IciMapping (Meng et al., 2015).
QTL Analysis
Raw data of Genotyping-by-Sequencing (GBS) were submitted to the NCBI Sequence Read Archive with bioproject ID: PRJNA5228871.
The genetic linkage map was constructed as has been described by our previous study (Zhang et al., 2020). Since our population is a pseudo-testcross F1 population, we constructed linkage map separately in maternal and paternal parents (Grattapaglia, 1997).
For environmental effect, best linear unbiased prediction (BLUP) was calculated by ANOVA model of IciMapping (ICIM) (Malosetti et al., 2004; Messmer et al., 2009). Additive QTLs were detected using Biparental Populations (BIP) function of ICIM and mapped by ICIM-ADD with Likehood of odd (LOD) threshold of 2.5 for significance. The additive QTL result was graphically represented using MapChart (Voorrips, 2002). Additive QTLs interacting with environment were identified by MET (QTL by Environment Interactions for Multi-Environment Trials) model of ICIM, the threshold was set to 2.5. For epistatic QTLs, BIP-ICIM-EPI model was used, the LOD threshold was set to 5.0 (Li et al., 2007; Meng et al., 2015).
Results
Analysis of the Phenotypic Variations
For evaluation of alfalfa quality, phenotypic data including crude protein and fiber composition of F1 population and its two parental plants were measured in 2016, 2019, and 2020. According to the t-test, the crude protein content of the parental parent was significantly higher than that of the maternal parent (21.19 vs. 18.83%; 22.32 vs. 19.27%) in 2016 and 2019 (P < 0.01). In contrast, the content of NDF, ADF, and lignin of the paternal parent was significantly lower than that in the maternal parent tested in the 3 years (P < 0.01) except lignin in 2020 (Supplementary Table 1). The results demonstrated that the paternal parent was superior to the maternal one in quality. A frequency distribution histogram based on the F1 population quality data showed transgressive segregation in the F1 population with a continuous distribution (Figure 1), indicating that the quality indexes used here were quantitative traits. The phenotypic variation we observed is consistent with the previous reports (Julier et al., 2000; Wang et al., 2016; Biazzi et al., 2017). The variation over years suggested that these traits are affected by the environment.
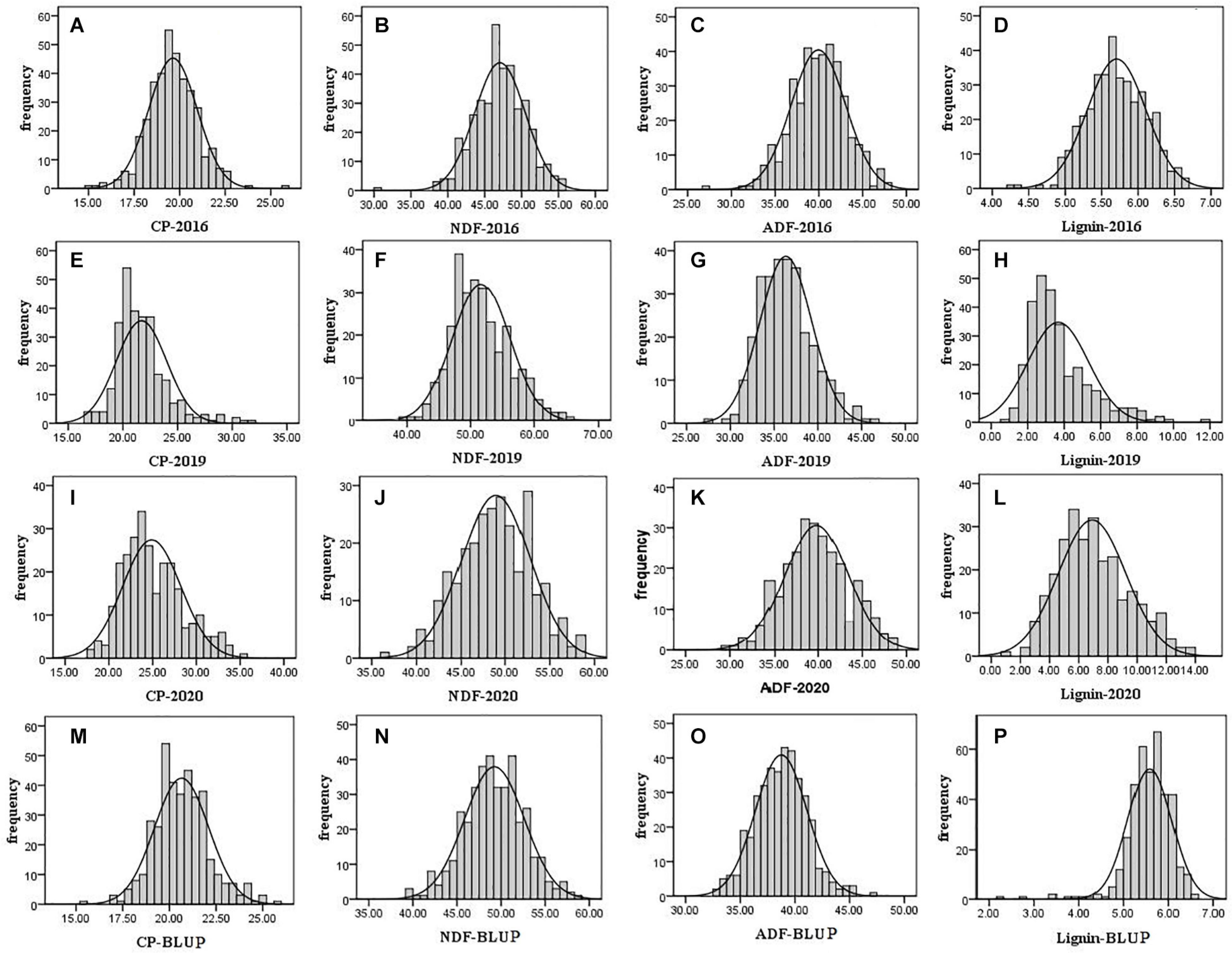
Figure 1. Frequency distribution histogram of F1 population quality traits in 2016, 2019, and 2020. The black solid line is the normal distribution curve. The traits are include the following: (A) CP in 2016; (B) NDF in 2016; (C) ADF in 2016; (D) lignin in 2016; (E) CP in 2019; (F) NDF in 2019; (G) ADF in 2019; (H) lignin in 2019; (I) CP in 2020; (J) NDF in 2020; (K) ADF in 2020; (L) lignin in 2020; (M) CP of BLUP; (N) NDF of BLUP; (O) ADF of BLUP; and (P) lignin of BLUP.
Analysis of Correlation Coefficiency and the Broad-Sense Heritability in F1 Population
The correlation coefficient was calculated using the BLUP value. As shown in Table 1, the three indexes for fiber composition were positively correlated and the correlation coefficient ranged from 0.40–0.68 (P < 0.01) with the highest between NDF and ADF. Crude protein was negatively correlated with both ADF (−0.49) and Lignin (−0.17) for P < 0.01, but not with NDF (P > 0.05). The calculation of the broad-sense heritability showed a relatively higher value for NDF (0.56) and lower one for lignin (0.46) (Table 1).
Mappping of QTLs Associated With Crude Protein and Fiber Composition
Based on the data of crude protein and fiber composition of the F1 population and its parental plants collected in 2016, 2019, and 2020, QTL mapping was performed using the alfalfa linkage map we previously constructed (Zhang et al., 2020). In total, we detected 83 QTLs related to either protein content or fiber composition with 31, 15, and 17 loci from the year of 2016, 2019, and 2020, respectively, and the rest were detected using BLUP values.
For crude protein, a total of 23 QTLs were mapped including seven QTLs identified using BLUP values (Table 2 and Supplementary Table 2). The LOD values ranged from 2.62 to 10.24, the percentage of phenotypic variation explained by QTL (PVE) was 1.45–11.34%, and the additive effect value of −0.87 to 1.32. QTL qCP7D, had the highest LOD value (Supplementary Table 2), and QTL located on the chromosome 5B (qCP5B) had the highest PVE and additive effect values (Table 2).
QTLs associated with fiber composition were separately identified based on our evaluation of NDF, ADF, and lignin content. For NDF-related QTLs, the 29 loci with 12 mapped in the paternal and 17 in the maternal parent were located on 18 linkage groups with PVE varying from 2.18–6.27% (Table 2 and Supplementary Table 2). The number of QTLs we discovered varied in the test years with a double amount mapped in 2016 compared with the 2019 and 2020. Additive effect of half the loci (14 out of 29) was positive and the other half (15 out of 29) negative, suggesting they were inherited from the maternal and paternal parent, respectively.
For QTLs associated with ADF, in total, 18 QTLs locating on 12 different linkage groups were discovered to be associated with ADF (Table 2 and Supplementary Table 2). In 2016, 8 QTLs were mapped, and additive effect of five loci was negative with PVE of 4.06 and 4.12%. Using, BLUP values of ADF, four QTLs were detected with three from the maternal parent.
For lignin-associated QTLs, a total of 13 QTLs were discovered on 10 linkage groups (Table 2 and Supplementary Table 2). Their LOD values ranged from 2.55 to 11.75. PVE of 3.01–14.35%, and additive effect of −0.72 to 0.63. QTL qlignin6D-2 had the highest LOD and PVE of 11.75 and 14.35, respectively. QTL qlignin5B locating on chromosome 5B had the highest additive effect.
QTLs Associated With Multiple Traits
Our results showed that 83 QTLs were detected to be associated with the content of protein or fiber in alfalfa. Among them, 12 QTLs were found to be associated with more than one trait, and seven loci were distributed on the maternal linkage map and five on the paternal one (Figure 2 and Supplementary Figure 1). QTL qlignin6D-2, which was associated with both lignin and crude protein, has the biggest LOD and PVE (Table 2), suggesting this QTL may contain key genes that can significantly affect lignin content.
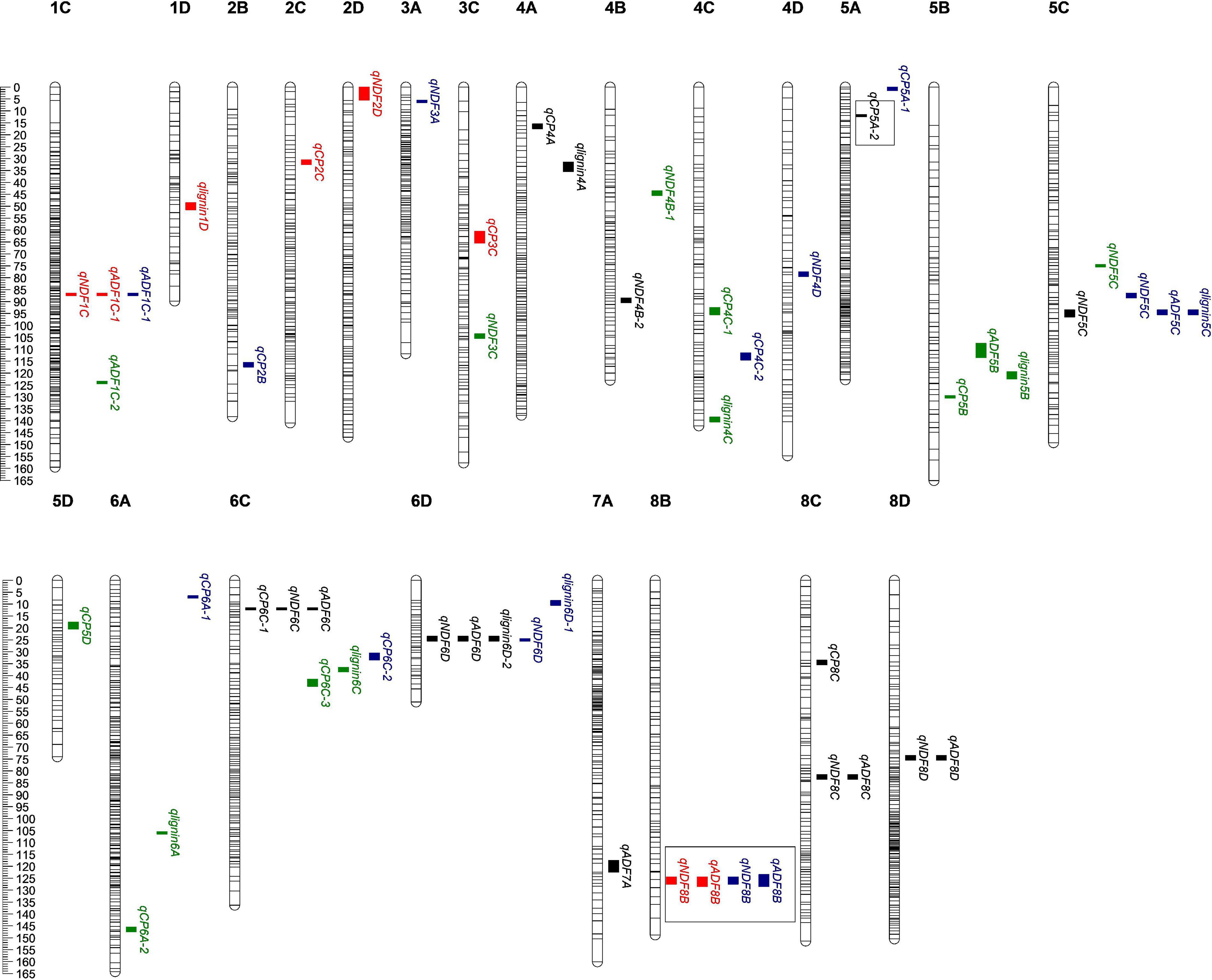
Figure 2. Quality-related QTL across the 32 linkage groups from a genetic map of maternal parent. The ruler on the left side represents the relative position of SNP on the chromosome. The small colored blocks represent QTL intervals. Black, QTLs in 2016; red, QTLs in 2019; green, QTLs in 2020; BLUP, QTLs of BLUP. The QTL in the box means it has been reported, qCP5A-2 (Biazzi et al., 2017); qNDF8B and qADF8B (Sakiroglu and Brummer, 2017).
83.33% (10 out of 12) of the QTLs were co-localized by ADF and NDF (Figure 2 and Supplementary Figure 1), indicating that both indexes contribute to fiber connect in alfalfa. Among them, three loci were identified to be associated with ADF, NDF, and lignin simultaneously, suggesting the higher reliability of these loci as candidates for further investigation of alfalfa fiber composition. The rest two co-localized QTLs were related to crude protein and NDF (Figure 2 and Supplementary Figure 1). Interestingly, the values of addition effect for individual QTL co-localized by fiber composition were unanimously negative or positive, suggesting that thy shared the same genetic direction. In contrast, the additive effect for QTLs associated with both crude protein and fiber composition were opposite, suggesting that the loci may antagonistically contribute to the two traits.
Analysis of Additive QTLs Interacted With the Environment
We also analyzed effect of environment by measuring the interaction effects. Among the 83 quality related QTLs, 47 were identified as interacting with the environment (Table 2 and Supplementary Table 2). The additive QTL × environment interaction effect (AE) of 24 loci was positive (0.05 ∼ 0.49), suggesting an increase of the phenotypic value of the corresponding trait, and the rest 23 were negative (−0.02 ∼ −0.55). PVE of AE was ranged from 0.10 ∼ 3.84%, suggesting these interaction effects have less effect on the phenotype.
Analysis of Epistatic QTLs
To explore the interaction between QTLs, we analyzed epistasis effect. In total, 73 epistatic QTL pairs (epQTLs) were detected with PVE ranging from 1.08 to 14.06%, and 31 pairs were identified using the BLUP values. No additive QTLs were found to be affected by other QTLs. 73 epQTLs all independently affected the phenotype, and these loci were distributed on 29 linkage groups except 2B, 3B, and 5A (Supplementary Tables 3, 4, Figure 3, and Supplementary Figure 2). Eleven pairs were associated with crude protein, eight with NDF, 17 with ADF, and 37 with lignin, respectively. A total of 25 epQTLs showed positive effects (0.12 ∼ 1.62), indicated that they could increase the phenotypic value independently from additive QTL, and 37 pairs showed negative effect (−0.11 ∼ −3.06), showed that they could reduce the phenotypic value independently. ep-QTL (eqNDF1C-eqNDF5B) associated with NDF had the highest PVE of 11.18%. In addition, 43 pairs of epistatic QTLs were identified at similar positions on the same chromosome, suggesting that most quality-related gene regulatory factors exist nearby. Moreover, we also identified the co-localized regions of two epistatic QTLs for different traits, which showed these loci may contribute to the two traits. The co-localized regions: (1) The region between markers TP6403 and TP71156 and the region between markers TP71156 and TP57346 on chromosome 1 (linkage group 1C) were associated with crude protein and lignin. (2) The region (TP49934-TP25688) on chromosome 1 (linkage group 1C) and the region (TP19263-TP27443) on chromosome 4 (linkage group 4D) were associated with NDF and ADF.
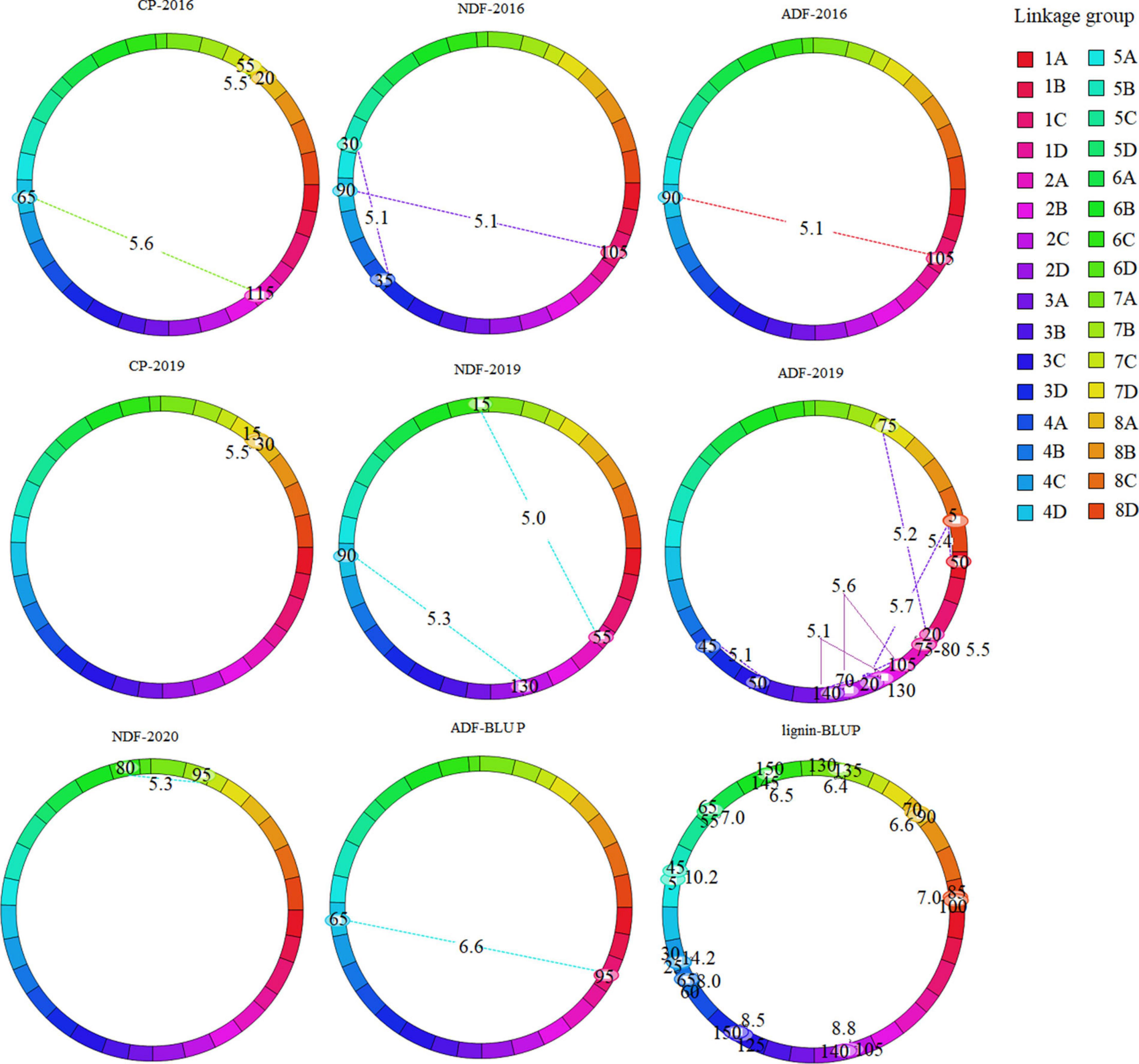
Figure 3. Cyclic diagram of epistatic QTLs for quality-related traits of the maternal parent. The dotted lines indicate the interacting SNP marker pairs mapped on the same or different linkage group with corresponding LOD scores owing to their epistatic effects. Marker position (cM) is mentioned inside the oval located on linkage group.
Analysis of Potential Candidate Genes
To search candidate genes, sequences of the markers flanking our additive QTLs were used to perform BLAST against the Medicago truncatula genome. Because of the genetic difference between Medicago truncatula and Medicago sativa, limited markers were matched. Among them, the region of qNDF1C, which co-localized with qADF1C and qlignin1C, contains eight related genes, five annotated as cellulose synthase-like protein A1 (Table 3). Consistently, a NDF-associated SNP was detected on this locus by Li et al. (2011). Genetic manipulation of the candidate genes would help to elucidate their role in alfalfa quality formation.
We also referenced the alfalfa genome (Chen et al., 2020) using TBtools to do BLAST. Six QTLs were matched on six chromosomal regions and the corresponding genes within these regions were listed (Supplementary Tables 5–10). The narrowest region (qCP4C-1) encoded seven genes, and two were predicted to be E3 ubiquitin-protein ligase SDIR1 and E3 ubiquitin-protein ligase RDUF2 (Table 3), which have been proven to be closely related to the degradation of plant proteins (Holdsworth et al., 2020). More effort are needed to align the QTLs in future.
Discussion
Alfalfa (Medicago sativa L.) known as the “queen of forage” has been cultivated worldwide due to its high nutritional quality as animal feed, and become the fourth most valuable field crop in the United States. Alfalfa quality determined mainly by protein content and fiber composition affects the digestibility and nutrition intake for animal feeding. Improving alfalfa quality by increasing crude protein or reducing fiber content has benefited animal husbandry (Koçer et al., 2018). Using QTL mapping combined with high throughput genotyping technology, we identified 83 regions associated with crude protein, fiber or lignin based on the phenotypic data of an F1 population established by crossing two alfalfa parents with significant difference in quality.
It has been documented that analysis of multiple effects including additive effect, epistatic effect, and QTL × environment interaction effect helps to avoid an underestimation of the total genetic impact of a trait (Patil et al., 2013; Chattopadhyay and Behera, 2019). We found that 60.26% (47 out of 83) of additive QTLs interacted with the environment, and 73 pairs regions had epistatic effect, which suggested the complexity of alfalfa quality traits. This phenomenon was also found in the QTL identification of rice grain protein content (Chattopadhyay et al., 2019). Future research of different mapping groups with phenotypic data from multiple temporal and spatial collection would be effective.
Previous study revealed that the QTLs of NDF, ADF, and lignin in alfalfa have co-localizations on chromosomes 1 and 3 (Wang et al., 2016). We identified 12 QTLs associated with multiple traits and they distribute on chromosomes 1, 4, 5, 6, 7, and 8. The poor continuity of QTL mapping has been reported in crop yield studies of rice (Agrama et al., 2007; Zhang et al., 2014), probably due to the difference of populations used for the studies, as well as the number of markers available. Colocalization and pleiotropic associations have been reported to help reveal important genomic regions or genes associated with the target traits (Huang et al., 2015). Although the 12 co-localized regions scattered on six linage groups, the traits for fiber composition, including ADF, NDF, and lignin, were found unanimously sharing either negative or positive values of addition effect, indicating the similar genetic contribution of the loci to these traits. In contrast, the two QTLs co-localized by crude protein and NDF/ADF had the opposite additive effect. For example, the additive effect of a QTL associated with crude protein, NDF, and ADF in linkage group 6C, was 0.3391, −0.8079, and −0.6446, respectively, implying that the QTL antagonistically contributes to protein content and fiber composition. The findings are consistent with the correlation analysis between these traits. Supportively, down-regulation of lignin synthesis gene hydroxycinnamoyl CoA: shikimate hydroxycinnamoyl transferase in transgenic alfalfa could reduce NDF and ADF (Shadle et al., 2007). The colocalized QTLs may be applied in improvement of multiple traits simultaneously.
Four of the 83 QTLs we identified here have been documented to be associated with alfalfa quality (Li et al., 2011; Espinoza and Julier, 2013; Biazzi et al., 2017; Sakiroglu and Brummer, 2017). For example, A SNP associated with NDF in Li’s report shared the same region with qNDF1C, and the QTL was co-localized by qADF1C-2 and qlignin1C-2. The shortest region of a QTL related to protein content has two E3 ubiquitin-protein ligases, which mediate the polyubiquitination of lysine and cysteine residues on target proteins, and have been proven to play an important role as regulators of protein trafficking and degradation. These findings are in supportive of our association analysis of alfalfa quality QTLs, and the loci are worthy of further investigation. Narrow down of the regions covered by our QTLs would specify more candidates potentially contributing to alfalfa quality formation. An increase of sequencing coverage, enrichment of markers and application of higher density linkage maps would facilitate marker-assisted selection for favorable alleles in breeding alfalfa varieties with improved quality.
Conclusion
The identified QTLs associated with quality-related traits provide important information for understanding the genetic controls of alfalfa quality. The results of this study could be used for molecular marker-assisted selection, dramatically improving the quality of alfalfa by molecular means.
Data Availability Statement
The original contributions presented in the study are publicly available. This data can be found here: https://www.ncbi.nlm.nih.gov/bioproject/?term=PRJNA522887.
Author Contributions
JK and QY designed the experiments and developed mapping population. CY, ZW, and JK performed the data analysis, and wrote the manuscript. FZ, LC, and RL performed the genotyping and sequencing, and constructed genetic maps. XY, XJ, FH, ZW, TY, CW, and TG managed field work and investigated phenotypic data. All authors read and approved the final manuscript.
Funding
The authors acknowledge the financial support for this study provided by Breeding forage and grain legumes to increase China’s and EU’s protein self-sufficiency, collaborative research key project between China and EU, granted by the Ministry of Science and Technology of China (2017YFE0111000) and granted by European Commission (EUCLEG 727312) and the earmarked fund for China Agriculture Research System (CARS-35-04) and the key research project of Ningxia province for alfalfa breeding program (2019NYY203) and Agricultural Science and Technology Innovation Program (ASTIP-IAS14).
Conflict of Interest
The authors declare that the research was conducted in the absence of any commercial or financial relationships that could be construed as a potential conflict of interest.
Acknowledgments
The authors hope to express their appreciation to the reviewers for this manuscript.
Supplementary Material
The Supplementary Material for this article can be found online at: https://www.frontiersin.org/articles/10.3389/fpls.2021.608940/full#supplementary-material
Supplementary Figure 1 | Quality-related QTLs across the 32 linkage groups from a genetic map of the paternal parent. The ruler on the left side represents the relative position of SNP on the chromosome. The small colored blocks represent QTL intervals. Black, QTLs in 2016; red, QTLs in 2019; green, QTLs in 2020; BLUP, QTLs of BLUP.
Supplementary Figure 2 | Cyclic diagram of epistatic QTLs for quality-related traits of the paternal parent. The dotted lines indicate the interacting SNP marker pairs mapped on the same or different linkage group with corresponding LOD scores owing to their epistatic effects. Marker position (cM) is mentioned inside the oval located on linkage group. The QTL in the box means it has been reported, qNDF1C, qADF1C-2, and qlignin1C-2 (Li et al., 2011); qNDF7D and qADF7D (Espinoza and Julier, 2013).
Footnotes
References
Adhikari, L., Lindstrom, O. M., Markham, J., and Missaoui, A. M. (2018). Dissecting key adaptation traits in the polyploid perennial Medicago sativa using GBS-SNP mapping. Front. Plant Sci. 9:934. doi: 10.3389/fpls.2018.00934
Agrama, H., Eizenga, G., and Yan, W. (2007). Association mapping of yield and its components in rice cultivars. Mol. Breed. 19, 341–356. doi: 10.1007/s11032-006-9066-6
Annicchiarico, P., Barrett, B., Brummer, E. C., Julier, B., and Marshall, A. H. (2015). Achievements and Challenges in Improving Temperate Perennial Forage Legumes. Critic. Rev. Plant Sci. 34, 327–380. doi: 10.1080/07352689.2014.898462
Bacenetti, J., Lovarelli, D., Tedesco, D., Pretolani, R., and Ferrante, V. (2018). Environmental impact assessment of alfalfa (Medicago sativa L.) hay production. Sci. Total Environ. 635, 551–558. doi: 10.1016/j.scitotenv.2018.04.161
Baucher, M., Bernard-Vailhé, M. A., Chabbert, B., Besle, J.-M., Opsomer, C., Van Montagu, M., et al. (1999). Down-regulation of cinnamyl alcohol dehydrogenase in transgenic alfalfa (Medicago sativa L.) and the effect on lignin composition and digestibility. Plant Mol. Biol. 39, 437–447. doi: 10.1046/j.1467-7652.2003.00040.x
Bazrkarkhatibani, L., Fakheri, B. A., Hosseinichaleshtori, M., Mahender, A., Mahdinejad, N., and Ali, J. (2019). Genetic Mapping and Validation of Quantitative Trait Loci (QTL) for the Grain Appearance and Quality Traits in Rice (Oryza sativa L.) by Using Recombinant Inbred Line (RIL) Population. Comparat. Funct. Genomics 2019, 1–13. doi: 10.1155/2019/3160275
Biazzi, E., Nazzicari, N., Pecetti, L., Brummer, E. C., Palmonari, A., Tava, A., et al. (2017). Genome-wide association mapping and genomic selection for alfalfa (Medicago sativa) forage quality traits. PLoS One 12:e0169234. doi: 10.1371/journal.pone.0169234
Brouwer, D., and Osborn, T. (1999). A molecular marker linkage map of tetraploid alfalfa (Medicago sativa L.). Theoret. Appl. Genet. 99, 1194–1200. doi: 10.1007/s001220051324
Chattopadhyay, K., and Behera, E. (2019). Detection of stable QTLs for grain protein content in rice (Oryza sativa L.) employing high throughput phenotyping and genotyping platforms. Sci. Rep. 9, 1–16.
Chattopadhyay, K., Behera, L., Bagchi, T. B., Sardar, S. S., Moharana, N., Patra, N. R., et al. (2019). Detection of stable QTLs for grain protein content in rice (Oryza sativa L.) employing high throughput phenotyping and genotyping platforms. Sci. Rep. 9, 1–16.
Chen, H., Zeng, Y., Yang, Y., Huang, L., Tang, B., Zhang, H., et al. (2020). Allele-aware chromosome-level genome assembly and efficient transgene-free genome editing for the autotetraploid cultivated alfalfa. Nat. Commun. 11, 1–11.
Espinoza, L. D. C. L., and Julier, B. (2013). QTL detection for forage quality and stem histology in four connected mapping populations of the model legume Medicago truncatula. Theoret. Appl. Genet. 126, 497–509. doi: 10.1007/s00122-012-1996-2
Gallego−Giraldo, L., Shadle, G., Shen, H., Barros−Rios, J., Fresquet Corrales, S., Wang, H., et al. (2016). Combining enhanced biomass density with reduced lignin level for improved forage quality. Plant Biotechnol. J. 14, 895–904. doi: 10.1111/pbi.12439
Grattapaglia, D. (1997). “Pseudo-testcross mapping strategy using RAPD markers,” in Fingerprinting methods based on arbitrarily primed PCR, eds R. Bova and M. R. Micheli, (Berlin: Springer), 201–218. doi: 10.1007/978-3-642-60441-6_23
Griffin, T. S., Cassida, K. A., Hesterman, O. B., and Rust, S. R. (1994). Alfalfa Maturity and Cultivar Effects on Chemical and In Situ Estimates of Protein Degradability. Crop Sci. 34:1654. doi: 10.2135/cropsci1994.0011183x003400060043x
Holdsworth, M. J., Vicente, J., Sharma, G., Abbas, M., and Zubrycka, A. (2020). The plant N-degron pathways of ubiquitinmediated proteolysis. J. Integr Plant Biol. 62, 70-89. doi: 10.1111/jipb.12882
Huang, Y., Sun, C., Min, J., Chen, Y., Tong, C., and Bao, J. (2015). Association mapping of quantitative trait loci for mineral element contents in whole grain rice (Oryza sativa L.). J. Agricult. Food Chem. 63, 10885–10892. doi: 10.1021/acs.jafc.5b04932
Julier, B., Huyghe, C., and Ecalle, C. (2000). Within-and among-cultivar genetic variation in alfalfa: forage quality, morphology, and yield. Crop Sci. 40, 365–369. doi: 10.2135/cropsci2000.402365x
Kaur, H., Peel, A., Acosta, K., Gebril, S., Ortega, J. L., and Sengupta−Gopalan, C. (2019). Comparison of alfalfa plants overexpressing glutamine synthetase with those overexpressing sucrose phosphate synthase demonstrates a signaling mechanism integrating carbon and nitrogen metabolism between the leaves and nodules. Plant Direct 3:e00115. doi: 10.1002/pld3.115
Koçer, B., Bozkurt, M., Ege, G., Tüzün, A., Konak, R., and Olgun, O. (2018). Effects of a meal feeding regimen and the availability of fresh alfalfa on growth performance and meat and bone quality of broiler genotypes. Br. Poultry Sci. 59, 318–329. doi: 10.1080/00071668.2018.1440378
Lander, E. S., and Botstein, D. (1989). Mapping mendelian factors underlying quantitative traits using RFLP linkage maps. Genetics 121, 185–199. doi: 10.1093/genetics/121.1.185
Li, H., Ye, G., and Wang, J. (2007). A modified algorithm for the improvement of composite interval mapping. Genetics 175, 361–374. doi: 10.1534/genetics.106.066811
Li, K., Wang, H., Hu, X., Ma, F., Wu, Y., Wang, Q., et al. (2017). Genetic and quantitative trait locus analysis of cell wall components and forage digestibility in the Zheng58× HD568 maize RIL population at anthesis stage. Front. Plant Sci. 8:1472. doi: 10.3389/fpls.2017.01472
Li, X., Wei, Y., Acharya, A., Jiang, Q., Kang, J., and Brummer, E. C. (2014). A saturated genetic linkage map of autotetraploid alfalfa (Medicago sativa L.) developed using genotyping-by-sequencing is highly syntenous with the Medicago truncatula genome. G3 4, 1971–1979. doi: 10.1534/g3.114.012245
Li, X., Wei, Y., Moore, K. J., Michaud, R., Viands, D. R., Hansen, J. L., et al. (2011). Association mapping of biomass yield and stem composition in a tetraploid alfalfa breeding population. Plant Genome J. 4, 24–35.
Liu, Y., Wu, Q., Ge, G., Han, G., and Jia, Y. (2018). Influence of drought stress on afalfa yields and nutritional composition. BMC Plant Biol. 18:13. doi: 10.1186/s12870-017-1226-9
Malosetti, M., Voltas, J., Romagosa, I., Ullrich, S., and Van Eeuwijk, F. (2004). Mixed models including environmental covariables for studying QTL by environment interaction. Euphytica 137, 139–145. doi: 10.1023/b:euph.0000040511.46388.ef
Marita, J. M., Ralph, J., Hatfield, R. D., Guo, D., Chen, F., and Dixon, R. A. (2003). Structural and compositional modifications in lignin of transgenic alfalfa down-regulated in caffeic acid 3-O-methyltransferase and caffeoyl coenzyme A 3-O-methyltransferase. Phytochemistry 62, 53–65. doi: 10.1016/s0031-9422(02)00434-x
Meng, L., Li, H., Zhang, L., and Wang, J. (2015). QTL IciMapping: integrated software for genetic linkage map construction and quantitative trait locus mapping in biparental populations. Crop J. 3, 269–283. doi: 10.1016/j.cj.2015.01.001
Messmer, R., Fracheboud, Y., Bänziger, M., Vargas, M., Stamp, P., and Ribaut, J.-M. (2009). Drought stress and tropical maize: QTL-by-environment interactions and stability of QTLs across environments for yield components and secondary traits. Theoret. Appl. Genet. 119, 913–930. doi: 10.1007/s00122-009-1099-x
Nakashima, J., Chen, F., Jackson, L., Shadle, G., and Dixon, R. A. (2008). Multi−site genetic modification of monolignol biosynthesis in alfalfa (Medicago sativa): effects on lignin composition in specific cell types. N. Phytol. 179, 738–750. doi: 10.1111/j.1469-8137.2008.02502.x
Oba, M., and Allen, M. S. (1999). Evaluation of the Importance of the Digestibility of Neutral Detergent Fiber from Forage: Effects on Dry Matter Intake and Milk Yield of Dairy Cows. J. Dairy Sci. 82, 589–596. doi: 10.3168/jds.s0022-0302(99)75271-9
Palmonari, A., Fustini, M., Canestrari, G., Grilli, E., and Formigoni, A. (2014). Influence of maturity on alfalfa hay nutritional fractions and indigestible fiber content. J. Dairy Sci. 97, 7729–7734. doi: 10.3168/jds.2014-8123
Patil, R., Tamhankar, S., Oak, M., Raut, A., Honrao, B., Rao, V., et al. (2013). Mapping of QTL for agronomic traits and kernel characters in durum wheat (Triticum durum Desf.). Euphytica 190, 117–129. doi: 10.1007/s10681-012-0785-y
Ray, I. M., Han, Y. E. L., Meenach, C. D., Santantonio, N., Sledge, M. K., et al. (2015). Identification of quantitative trait loci for alfalfa forage biomass productivity during drought stress. Crop Sci. 55, 2012–2033. doi: 10.2135/cropsci2014.12.0840
Sakiroglu, M., and Brummer, E. C. (2017). Identification of loci controlling forage yield and nutritive value in diploid alfalfa using GBS-GWAS. Theoret. Appl. Genet. 130, 261–268. doi: 10.1007/s00122-016-2782-3
Sarwar, N., Mahmood, S., Nisa, M., and Bilal, M. (1999). Factors affecting digestibility of feeds in ruminants. Int. J. Agric. Biol. 1, 366–372.
Shadle, G., Chen, F., Reddy, M. S., Jackson, L., Nakashima, J., and Dixon, R. A. (2007). Down-regulation of hydroxycinnamoyl CoA: shikimate hydroxycinnamoyl transferase in transgenic alfalfa affects lignification, development and forage quality. Phytochemistry 68, 1521–1529. doi: 10.1016/j.phytochem.2007.03.022
Sledge, M., Ray, I., and Jiang, G. (2005). An expressed sequence tag SSR map of tetraploid alfalfa (Medicago sativa L.). Theoret. Appl. Genet. 111, 980–992. doi: 10.1007/s00122-005-0038-8
Tong, Z., Li, H., Zhang, R., Ma, L., Dong, J., and Wang, T. (2015). Co-downregulation of the hydroxycinnamoyl-CoA: shikimate hydroxycinnamoyl transferase and coumarate 3-hydroxylase significantly increases cellulose content in transgenic alfalfa (Medicago sativa L.). Plant Sci. 239, 230–237. doi: 10.1016/j.plantsci.2015.08.005
Vance, C. P., Miller, S. S., Gregerson, R. G., Samac, D. A., Robinson, D. L., and Gantt, J. S. (1995). Alfalfa NADH−dependent glutamate synthase: structure of the gene and importance in symbiotic N2 fixation. Plant J. 8, 345–358. doi: 10.1046/j.1365-313x.1995.08030345.x
Voorrips, R. (2002). MapChart: software for the graphical presentation of linkage maps and QTLs. J. Heredity 93, 77–78. doi: 10.1093/jhered/93.1.77
Wang, Z., Qiang, H., Zhao, H., Xu, R., Zhang, Z., Gao, H., et al. (2016). Association mapping for fiber-related traits and digestibility in alfalfa (Medicago sativa). Front. Plant Sci. 7:331. doi: 10.3389/fpls.2016.00331
Warrington, C. V., Abdelhaleem, H., Hyten, D. L., Cregan, P. B., Orf, J. H., Killam, A. S., et al. (2015). QTL for seed protein and amino acids in the Benning × Danbaekkong soybean population. Theoret. Appl. Genet. 128, 839–850. doi: 10.1007/s00122-015-2474-4
Yang, S., Zu, Y., Li, B., Bi, Y., Jia, L., He, Y., et al. (2019). Response and intraspecific differences in nitrogen metabolism of alfalfa (Medicago sativa L.) under cadmium stress. Chemosphere 220, 69–76. doi: 10.1016/j.chemosphere.2018.12.101
Zhang, F., Kang, J., Long, R., Yu, L. X., Sun, Y., Wang, Z., et al. (2020). Construction of high−density genetic linkage map and mapping quantitative trait loci (QTL) for flowering time in autotetraploid alfalfa (Medicago sativa L.) using genotyping by sequencing. Plant Genome 13:e20045.
Zhang, F., Kang, J., Long, R., Yu, L.-X., Wang, Z., Zhao, Z., et al. (2019). High-density linkage map construction and mapping QTL for yield and yield components in autotetraploid alfalfa using RAD-seq. BMC Plant Biol. 19:165. doi: 10.1186/s12870-019-1770-6
Zhang, P., Liu, X., Tong, H., Lu, Y., and Li, J. (2014). Association mapping for important agronomic traits in core collection of rice (Oryza sativa L.) with SSR markers. PLoS One 9:e111508. doi: 10.1371/journal.pone.0111508
Zhang, W., Bi, J., Chen, L., Zheng, L., Ji, S., Xia, Y., et al. (2008). QTL mapping for crude protein and protein fraction contents in rice (Oryza sativa L.). J. Cereal Sci. 48, 539–547. doi: 10.1016/j.jcs.2007.11.010
Keywords: crude protein, fiber composition, quality, QTL mapping, Medicago sativa L.
Citation: Yang C, Zhang F, Jiang X, Yang X, He F, Wang Z, Long R, Chen L, Yang T, Wang C, Gao T, Kang J and Yang Q (2021) Identification of Genetic Loci Associated With Crude Protein Content and Fiber Composition in Alfalfa (Medicago sativa L.) Using QTL Mapping. Front. Plant Sci. 12:608940. doi: 10.3389/fpls.2021.608940
Received: 22 September 2020; Accepted: 27 January 2021;
Published: 18 February 2021.
Edited by:
Eric Von Wettberg, University of Vermont, United StatesReviewed by:
Maria Samsonova, Peter the Great St. Petersburg Polytechnic University, RussiaManish Kumar Pandey, International Crops Research Institute for the Semi-Arid Tropics (ICRISAT), India
Copyright © 2021 Yang, Zhang, Jiang, Yang, He, Wang, Long, Chen, Yang, Wang, Gao, Kang and Yang. This is an open-access article distributed under the terms of the Creative Commons Attribution License (CC BY). The use, distribution or reproduction in other forums is permitted, provided the original author(s) and the copyright owner(s) are credited and that the original publication in this journal is cited, in accordance with accepted academic practice. No use, distribution or reproduction is permitted which does not comply with these terms.
*Correspondence: Junmei Kang, kangjunmei@caas.cn; Qingchuan Yang, qchyang66@163.com