- 1South Australia Research and Development Institute, Adelaide, South Australia, Australia
- 2School of Agriculture, Food and Wine, The University of Adelaide, Australia
We’re in a maze, not a highway; there is nowhere that speed alone can take us … I’m not being held back by the university’s computers … I’m being held back by my own lack of insight into the problems I’m addressing…
Julie Dehghani
In the spring of 2018, the city of Adelaide hosted the 5th International Plant Phenotyping Symposium (IPPS), titled “From plant, to data, to impact”, as well as the OECD-sponsored workshop “Making science useful to agriculture”1. Here I share a personal account of salient findings of these two meetings, with a focus on more effective phenotyping, namely, phenotyping that is more likely to deliver tangible outcomes to plant breeding. To improve the effectiveness of the large phenotyping effort worldwide, I suggest asking two questions:
Are we really limited by data? Or better, to what extent we are limited by data, and to what extent we are, in the terms of Julie Dehghani, being held back by our own lack of insight into the problems we’re addressing? I would argue our theories are lagging, and the theoretical model of the phenotype has become a bottleneck.
Can we improve the matching of trait and phenotyping platform? Individual plants and populations are fundamentally different biological entities; yield is a population-level attribute where plant-plant interactions are important. I would propose that explicit consideration of scaling, density- and context-dependence can help to better match agronomic traits with phenotyping platform and method, avoid expensive distractions and improve return on R&D investment.
The underlying assumption of ‘omics’ technologies is that large datasets on genes, their expression (transcriptomics) and products (proteomics and metabolomics) would resolve the complexities of key traits (Langridge, 2018). These ‘omics’ efforts have been primarily driven by technology—it was possible and therefore done—and largely failed to resolve the agronomically relevant phenotype, particularly crop yield (Langridge, 2018). This failure was ascribed to lagging phenotyping technologies (Cobb et al., 2013; Araus and Cairns, 2014), which in turn motivated the phenotyping effort displayed in the 5th IPPS. The IPPS showed a similar technology-driven approach that leads to large datasets of variable quality and relevance. Once again, we are assuming we are limited by data—but are we? Big data (and associated technologies) is the next promise, and it might deliver in some areas. However, we need hypothesis-driven science at the very least for proper experimental design, and importantly, to match trait and phenotyping platform.
The complete lack of attention to the theoretical aspects of the construction of the phenotype in the 5th IPPS was striking, and reinforces the notion of a technology-driven effort. The oversimplified model based on the unidirectional arrow from genotype to phenotype is unjustified but remains influential (Vinocur and Altman, 2005; Pickett, 2016). More nuanced theories of the phenotype need consideration (West-Eberhard, 2003; Piersma and van Gils, 2011; Noble, 2012; Félix, 2016). The metaphors that genes “control” development, and that genomes embody “programs” for development are particularly misplaced (Noble, 2012; Félix, 2016). A unified theory of phenotypic development and evolution emphasizes that “the individual’s genotype can never be said to control development. Development depends at every step on the pre-existent structure of the phenotype, a structure that is complexly determined by a long history of both genomic and environmental influences” (West-Eberhard, 2003). This perspective is more broadly captured in the concept of downward causation (Noble, 2012; Flack, 2017; Noble, 2017). A panel discussion on what it takes for phenotypic data to be useful focused on technical aspects along the lines of the FAIR principles (Wilkinson et al., 2016). A lonely voice advanced the importance of asking questions that matter biologically and agronomically for data to be useful, but after a lukewarm collective nod the discussion reverted to technical aspects of data management. Future editions of this IPPS will benefit from sessions devoted to the theory of the phenotype.
The 5th IPPS, and the literature more broadly, show that the mismatch between trait and phenotyping approach is a major source of inefficiency, e.g. phenotyping for density-dependent traits such as yield or nutrient uptake in isolated plants in the glasshouse, or in single rows in the field. For different reasons, research in plant biology frequently simplifies to potted plants in chamber or glasshouse. If we are interested in a biological process of an isolated plant in an artificial condition lacking agronomic context, this simplification is fine and needs no justification. However, we must ask rigorous questions about scaling, density- and context-dependence if we want to achieve agronomic relevance.
How does a trait measured in an individual plant relate to the trait in a crop stand, where yield and other agronomic attributes are resolved? Sadras and Richards (2014) classified traits in three groups depending on their scaling. Some traits generally scale, like herbicide tolerance or Bt (Figure 1A). There are traits for which scaling is strictly dependent on experimental protocols; for example, leaf expansion rate seems to scale from glasshouse to field in well designed and carefully executed experiments (Reymond et al., 2003). There are traits that rarely scale, including yield (Figure 1B), photosynthesis, capture and efficiency in the use of water and nutrients, allometric relationships (Figure 1C), and architecture traits such as tillering, shoot and root branching (Sadras and Richards, 2014). Photosynthesis is strongly buffered from molecular to stand level (Pettigrew et al., 1989; Sinclair et al., 2004). Lack of both wind and canopy structure in plant-based settings (glasshouse, chamber) generates unrealistic canopy-atmosphere coupling, hence the difficulty in scaling gas exchange (Jarvis and McNaughton, 1986; Fereres et al., 2014). Artifacts from growing plants in pots are well established (Ben-Porath and Baker, 1990; Passioura, 2002; Passioura, 2006; Poorter et al., 2012; Ryan and Graham, 2018), and yet potted plants are used in phenotyping traits related to water and nutrient uptake and efficiency. Ryan and Graham (2018) critically revised the agronomic relevance of plant-mycorrhiza studies in controlled environments. Density-dependence (Donald, 1963; Harper, 1977) is a useful criterion to predict scaling; tillering responds to neighboring plants as related to both light quality and resources (Casal et al., 1986; Skinner and Simmons, 1993; Peltonen-Sainio and Järvinen, 1995; Robertson et al., 2009), hence is less likely to scale. Nitrogen-biomass allometry—the keystone for quantifying crop nitrogen status (Sadras and Lemaire, 2014; Gastal et al., 2015)—is strongly density dependent, and therefore does not scale from plant to crop (Figure 1C). Density-dependence leads to hierarchies in stands and is an artifact commonly overlooked in the glasshouse (Chen et al., 2018) and, to a lesser extent in the field (Rebetzke et al., 2014; Fischer and Rebetzke, 2018). For example, transformed tobacco plants with superior leaf-level photosynthesis were grown with a single-row buffer of shorter, untransformed wild-types leading to a gross misinterpretation of the effect of molecular manipulations on the crop phenotype (South et al., 2019). Gene expression is density-dependent in locust (Pener and Simpson, 2009), and in Arabidopsis (Geisler et al., 2012). In chickpea, Fst genome scan revealed a mismatch in the top genomic regions under selection for yield in border rows under relaxed competition and inner rows under full competition (Lake et al., 2016).
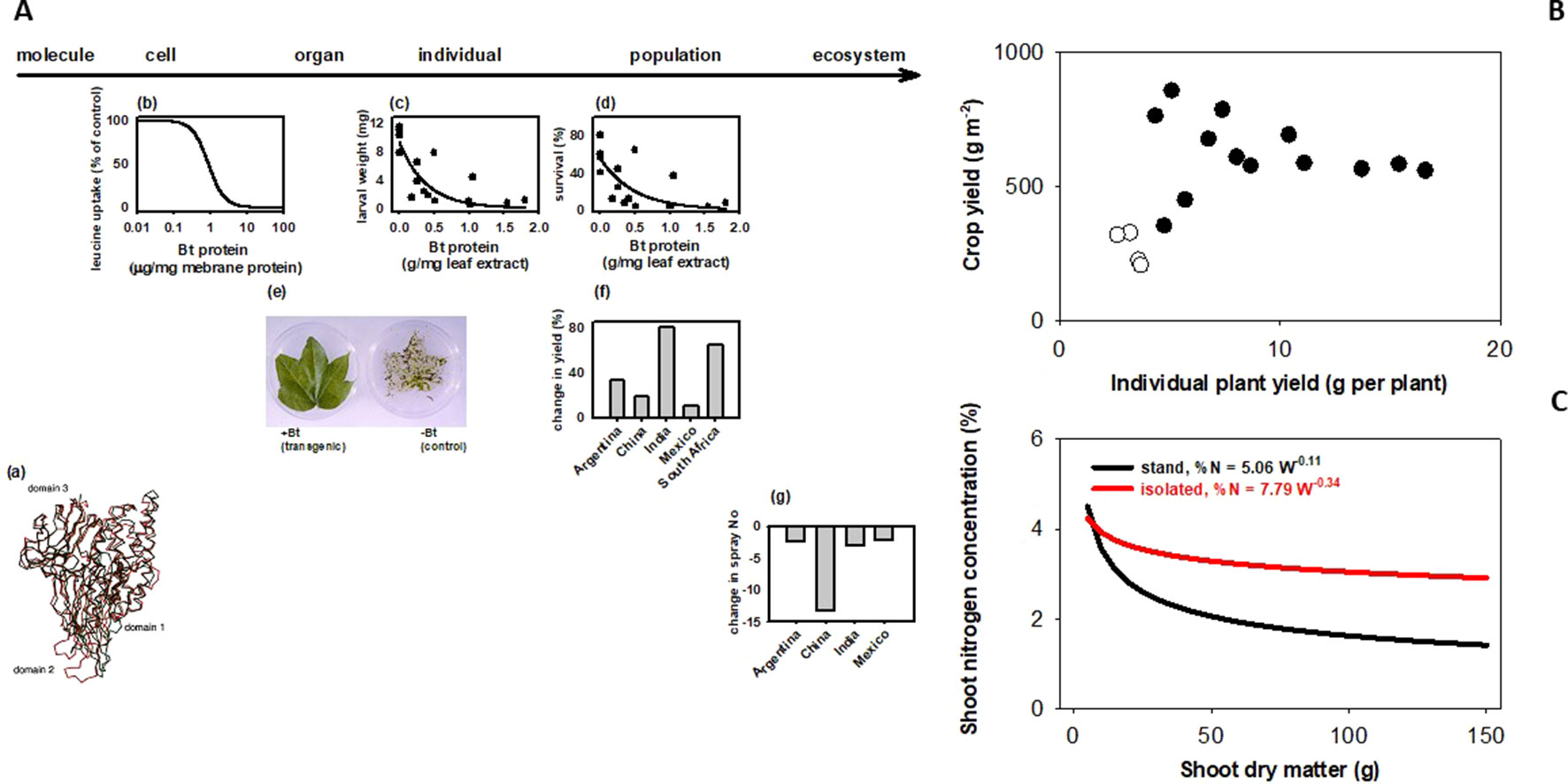
Figure 1 Bt scales, but yield and plant allometry do not because they are density dependent. (A) Bt scales from molecular to ecosystem level. (a) Bacillus turingensis (Bt) Cry proteins have deleterious effects on Lepidoptera larvae at the (b) cell, (c) individual, and (d) population levels. Bt proteins expressed in transformed plant tissues confer protection at the (e) organ and (f) population level. This protection allows for (g) less reliance on synthetic insecticides for pest control, with consequences at the ecosystem level. Sources: (a) Ca traces of individual domains of CryIA(a) (red) and CryIIIA (black) proteins (Grochulski et al., 1995); (b) inhibitory effect of CryIA(a) on leucine uptake by membrane vesicles prepared from Bombyx mori larvae midgut; (c) weight and (d) survival of 7-day-old, 3rd instar larvae of Elsmopalpus lignosellus after a week of feeding treatments with peanut leaf containing variable concentration of CryIA(c) protein (Singsit et al., 1997); (f) comparison of cotton leaves expressing CryIA(c) proteins and non-transformed controls exposed to Helicoverpa spp larva (Lewis Wilson, unpublished); differences between Bt and conventional cotton in (g) lint yield. (B) Yield does not scale from plant to population. Yield of wheat stands (200–400 plants m-2) does not correlate with yield of isolated plants (<20 plants m-2) grown under the same conditions in the field. Source: Pedró et al. (2012). (C) Nitrogen-biomass allometry does not scale from plant to population. Owing to plant-plant interactions, dilution curves relating shoot nitrogen concentration and shoot dry matter show a sharp dilution for plants in stands, and a highly buffered dilution in isolated plants. Source: Lemaire and Millard (1999).
Consideration of biological context is critical for effective phenotyping. Environmental factors such as radiation, photoperiod and temperature are spatially and temporally correlated, and plants evolved receptors coupled with molecular signaling that extract information from these joint multivariate properties of the environment. Hence responses dependent on these properties of the environment are encoded in the genome, epigenome and phenome of plants (Karban, 2015). For this reason, phenotyping in an unnatural context, i.e. where correlations between environmental variables have been unrealistically altered, are often of little agronomic relevance because biased relationships among the states of different environmental variables disturb the information decoded by the plant, and hence the phenotype. For example, diurnal profiles of carbon and nitrogen metabolites of plants grown with a step-change in radiation do not match those for plants grown with both regular (day-night sinusoidal cycle) and irregular (due to clouds) fluctuations in radiation (Annunziata et al., 2017). Sowing date trials to screen for heat adaptation in the field bias the relative state of key environmental factors (radiation, photoperiod, temperature, vapor pressure deficit), and confound developmental and thermal-stress responses (Sadras et al., 2015).
The sequence “From plant, to data, to impact” resonates with both the directional “pipeline” in biotechnology, from lab to field (Nuccio et al., 2018) and with the simplistic genotype-to-phenotype model. These directional perspectives have delivered improvements in crop protection but have largely failed to improve yield and adaptation to drought (Dalal et al., 2017; Nuccio et al., 2018). Conceptually, Denis Noble concludes that there is no privileged level of causation (Noble, 2012). Renee Laffite and colleagues have advanced a robust approach that focuses on agronomically rigorous field phenotyping of grain yield and high-level secondary traits such as anthesis-silking interval, with complementary work under controlled conditions—working from field to lab ensures agronomic relevance (Habben et al., 2014; Shi et al., 2015; Brugiere et al., 2017; Lafitte et al., 2018).
To improve the effectiveness of the large phenotyping effort worldwide, I suggest asking two questions:
Are we really limited by data? Or better, to what extent we are limited by data, and to what extent we are, in the terms of Julie Dehghani, being held back by our own lack of insight into the problems we’re addressing? I would argue our theories are lagging, and the theoretical model of the phenotype needs attention.
Can we improve the matching of trait and phenotyping platform? Individual plants and populations are fundamentally different biological entities; yield is a population attribute. I would propose that explicit consideration of scaling, density- and context-dependence can help to better match agronomic traits with phenotyping platform and method, avoid expensive distractions and improve return on R&D investment.
Author Contributions
VS wrote the paper.
Conflict of Interest
The author declares that the research was conducted in the absence of any commercial or financial relationships that could be construed as a potential conflict of interest.
Acknowledgments
The workshop “Making science useful to agriculture” was supported by OECD, Grains Research and Development Corporation, and South Australian Grains Industry Trust.
Footnotes
- ^ Papers and presentations from this meeting are at: https://msua.aweb.net.au/.
References
Annunziata, M. G., Apelt, F., Carillo, P., Krause, U., Feil, R., Mengin, V., et al. (2017). Getting back to nature: a reality check for experiments in controlled environments. J. Exp. Bot. 68 (16), 4463–4477. doi: 10.1093/jxb/erx220
Araus, J. L., Cairns, J. E. (2014). Field high-throughput phenotyping: the new crop breeding frontier. Trends Plant Sci. 19 (1), 52–61. doi: 10.1016/j.tplants.2013.09.008
Ben-Porath, A., Baker, D. N. (1990). Tap-root restriction effects on growth, earliness, and dry weight partitioning of cotton. Crop Sci. 30, 809–814. doi: 10.2135/cropsci1990.0011183X003000040009x
Brugiere, N., Zhang, W. J., Xu, Q. Z., Scolaro, E. J., Lu, C., Kahsay, R. Y., et al. (2017). Overexpression of RING domain E3 ligase ZmXerico1 confers drought tolerance through regulation of ABA homeostasis. Plant Physiol. 175 (3), 1350–1369. doi: 10.1104/pp.17.01072
Casal, J. J., Sanchez, R. A., Deregibus, V. A. (1986). The effect of plant-density on tillering - the involvement of r/fr ratio and the proportion of radiation intercepted per plant. Environ. Exp. Bot. 26 (4), 365–371. doi: 10.1016/0098-8472(86)90024-9
Chen, T.-W., Cabrera-Bosquet, L., Alvarez Prado, S., Perez, R., Artzet, S., Pradal, C., et al. (2018). Genetic and environmental dissection of biomass accumulation in multi-genotype maize canopies. J. Exp. Bot. 70, ery309–ery309. doi: 10.1093/jxb/ery309
Cobb, J. N., Declerck, G., Greenberg, A., Clark, R., McCouch, S. (2013). Next-generation phenotyping: requirements and strategies for enhancing our understanding of genotype-phenotype relationships and its relevance to crop improvement. Theor. Appl. Genet. 126 (4), 867–887. doi: 10.1007/s00122-013-2066-0
Dalal, A., Attia, Z., Moshelion, M. (2017). To produce or to survive: how plastic is your crop stress physiology? Front. Plant Sci. 8 (2067). doi: 10.3389/fpls.2017.02067
Donald, C. M. (1963). “Competition among crop and pasture plants,” in Advances in Agronomy. Ed. Norman, A. G. (Academic Press), 1–118. doi: 10.1016/S0065-2113(08)60397-1
Félix, M. A. (2016). Phenotypic evolution with and beyond genome evolution. Curr. Topics Dev. Biol. 119, 291–347. doi: 10.1016/bs.ctdb.2016.04.002
Fereres, E., Orgaz, F., Gonzalez-Dugo, V., Testi, L., Villalobos, F. J. (2014). Balancing crop yield and water productivity tradeoffs in herbaceous and woody crops. Funct. Plant Biol. 41 (11), 1009–1018. doi: 10.1071/FP14042
Fischer, R. A., Rebetzke, G. J. (2018). Indirect selection for potential yield in early-generation, spaced plantings of wheat and other small-grain cereals: a review. Crop Pasture Sci. 69 (5), 439–459. doi: 10.1071/CP17409
Flack, J. C. (2017). “Life’s information hierarchy,” in From matter to life: information and casualty. Eds. Walker, S. I., Davies, P. C. W., Ellis, G. F. R. (Cambridge: Cambridge University Press), 283–302. doi: 10.1017/9781316584200.012
Gastal, F., Lemaire, G., Durand, J. L., Louarn, G. (2015). “Quantifying crop responses to nitrogen and avenues to improve nitrogen-use efficiency,” in Crop Physiology: Applications for genetic improvement and agronomy. Eds. Sadras, V. O., Calderini, D. F.. (San Diego: Academic Press), 161–206. doi: 10.1016/B978-0-12-417104-6.00008-X
Geisler, M., Gibson, D. J., Lindsey, K. J., Millar, K., Wood, A. J. (2012). Upregulation of photosynthesis genes, and down-regulation of stress defense genes, is the response of Arabidopsis thaliana shoots to intraspecific competition. Bot. Stud. 53 (1), 85–96.
Grochulski, P., Masson, L., Borisova, S., Pusztai-Carey, M., Schwartz, J.-L., Brousseau, R., et al. (1995). Bacillus thuringiensisCrylA(a) insecticidal toxin: crystal structure and channel formation. J. Mol. Biol. 254 (3), 447–464. doi: 10.1006/jmbi.1995.0630
Habben, J. E., Bao, X. M., Bate, N. J., DeBruin, J. L., Dolan, D., Hasegawa, D., et al. (2014). Transgenic alteration of ethylene biosynthesis increases grain yield in maize under field drought-stress conditions. Plant Biotechnol. J. 12 (6), 685–693. doi: 10.1111/pbi.12172
Jarvis, P. G., McNaughton, K. (1986). Stomatal control of transpiration: scaling up from leaf to region. Adv. Ecol. Res. 15 (1), 49. doi: 10.1016/S0065-2504(08)60119-1
Karban, R. (2015). Plant sensing and communication. (Chicago: The University of Chicago Press). doi: 10.7208/chicago/9780226264844.001.0001
Lafitte, H. R., Habben, J. E., Simmons, C. R. (2018). Searching for transgenes that improve yield: promise and reality. Making Sci. Useful Agric. Adelaide Nov. 26-29 2018. https://msua.aweb.net.au/.
Lake, L., Li, Y., Casal, J. J., Sadras, V. O. (2016). Negative association between chickpea response to competition and crop yield: phenotypic and genetic analysis. Field Crops Res. 196, 409–417. doi: 10.1016/j.fcr.2016.07.021
Langridge, P. (2018). State-of-the-art in genetic resources. Making Sci. Useful Agric. Adelaide Nov. 26-29 2018; https://msua.aweb.net.au/.
Lemaire, G., Millard, P. (1999). An ecophysiological approach to modelling resource fluxes in competing plants. J. Exp. Bot. 50 (330), 15–28. doi: 10.1093/jexbot/50.330.15
Noble, A. D. (2017). “Digital and analogue information in organisms,” in From matter to life: information and casualty. Eds. Walker, S. I., Davies, P. C. W., Ellis, G. F. R. Cambridge: (Cambridge University Press), 114–129. doi: 10.1017/9781316584200.006
Noble, D. (2012). A theory of biological relativity: no priviliged level of causation. Interface Focus 2, 55–64. doi: 10.1098/rsfs.2011.0067
Nuccio, M. L., Paul, M., Bate, N. J., Cohn, J., Cutler, S. R. (2018). Where are the drought tolerant crops? An assessment of more than two decades of plant biotechnology effort in crop improvement. Plant Sci. 273, 110–119. doi: 10.1016/j.plantsci.2018.01.020
Passioura, J. B. (2002). Soil conditions and plant growth. Plant Cell Environ. 25 (2), 311–318. doi: 10.1046/j.0016-8025.2001.00802.x
Passioura, J. B. (2006). The perils of pot experiments. Funct. Plant Biol. 33 (12), 1075–1079. doi: 10.1071/FP06223
Pedró, A., Savin, R., Slafer, G. A. (2012). Crop productivity as related to single-plant traits at key phenological stages in durum wheat. Field Crops Res. 138 (0), 42–51. doi: 10.1016/j.fcr.2012.09.016
Peltonen-Sainio, P., Järvinen, P. (1995). Seeding rate effects on tillering, grain yield, and yield components of oat at high latitude. Field Crops Res. 40, 49–56. doi: 10.1016/0378-4290(94)00089-U
Pener, M. P., Simpson, S. J. (2009). “Locust Phase Polyphenism: an update,” in Advances in Insect Physiology, Vol 36. Eds. Simpson, S. J., Casas, J.. (Academic Press), 1–272. doi: 10.1016/S0065-2806(08)36001-9
Pettigrew, W. T., Hesketh, J. D., Peters, D. B., Woolley, J. T. (1989). Characterisation of canopy photosynthesis of chlorophyll-deficient soybean isolines. Crop Sci. 29, 1025–1029. doi: 10.2135/cropsci1989.0011183X002900040040x
Pickett, J. A. (2016). The essential need for GM crops. Nat. Plants 2 (6), 2. doi: 10.1038/nplants.2016.78
Poorter, H., Bühler, J., van Dusschoten, D., Climent, J., Postma, J. A. (2012). Pot size matters: a meta-analysis of the effects of rooting volume on plant growth. Funct. Plant Biol. 39, 839–850. doi: 10.1071/FP12049
Rebetzke, G. J., Fischer, R. A., van Herwaarden, A. F., Bonnett, D. G., Chenu, K., Rattey, A. R., et al. (2014). Plot size matters: interference from intergenotypic competition in plant phenotyping studies. Funct. Plant Biol. 41, 107–118. doi: 10.1071/FP13177
Reymond, M., Muller, B., Leonardi, A., Charcosset, A., Tardieu, F. (2003). Combining quantitative trait loci analysis and an ecophysiological model to analyze the genetic variability of the responses of maize leaf growth to temperature and water deficit. Plant Physiol. 131 (2), 664–675. doi: 10.1104/pp.013839
Robertson, D., Zhang, H., Palta, J. A., Colmer, T., Turner, N. C. (2009). Waterlogging affects the growth, development of tillers, and yield of wheat through a severe, but transient, N deficiency. Crop Pasture Sci. 60 (6), 578–586. doi: 10.1071/CP08440
Ryan, M. H., Graham, J. H. (2018). Little evidence that farmers should consider abundance or diversity of arbuscular mycorrhizal fungi when managing crops. New Phytol. 220, 1092–1107. doi: 10.1111/nph.15308
Sadras, V. O., Lemaire, G. (2014). Quantifying crop nitrogen status for comparisons of agronomic practices and genotypes. Field Crops Res. 164, 54–64. doi: 10.1016/j.fcr.2014.05.006
Sadras, V. O., Richards, R. A. (2014). Improvement of crop yield in dry environments: benchmarks, levels of organisation and the role of nitrogen. J. Exp. Bot. 65 (8), 1981–1995. doi: 10.1093/jxb/eru061
Sadras, V. O., Vadez, V., Purushothaman, R., Lake, L., Marrou, H. (2015). Unscrambling confounded effects of sowing date trials to screen for crop adaptation to high temperature. Field Crops Res. 177, 1–8. doi: 10.1016/j.fcr.2015.02.024
Shi, J., Habben, J. E., Archibald, R. L., Drummond, B. J., Chamberlin, M. A., Williams, R. W., et al. (2015). Overexpression of ARGOS genes modifies plant sensitivity to ethylene, leading to improved drought tolerance in both Arabidopsis and maize. Plant Physiol. 169 (1), 266–26+. doi: 10.1104/pp.15.00780
Sinclair, T. R., Purcell, L. C., Sneller, C. H. (2004). Crop transformation and the challenge to increase yield potential. Trends Plant Sci. 9 (2), 70–75. doi: 10.1016/j.tplants.2003.12.008
Singsit, C., Adang, M. J., Lynch, R. E., Anderson, W. F., Wang, A., Cardineau, G., et al. (1997). Expression of a Bacillus thuringiensis cryIA(c) gene in transgenic peanut plants and its efficacy against lesser cornstalk borer. Transgenic Res. 6 (2), 169–176. doi: 10.1023/A:1018481805928
Skinner, R., Simmons, S. R. (1993). Modulation of leaf elongation, tiller appearance and tiller senescence in spring barley by far-red light. Plant Cell Environ. 16, 555–562. doi: 10.1111/j.1365-3040.1993.tb00903.x
South, P. F., Cavanagh, A. P., Liu, H. W., Ort, D. R. (2019). Synthetic glycolate metabolism pathways stimulate crop growth and productivity in the field. Science 363, eaat9077. doi: 10.1126/science.aat9077
Vinocur, B., Altman, A. (2005). Recent advances in engineering plant tolerance to abiotic stress: achievements and limitations. Curr. Opin. Biotechnol. 16 (2), 123–132. doi: 10.1016/j.copbio.2005.02.001
West-Eberhard, M. J. (2003). Developmental plasticity and evolution. New York: Oxford University Press.
Keywords: Scaling, yield, density-dependence, phenotype, context-dependence
Citation: Sadras VO (2019) Effective Phenotyping Applications Require Matching Trait and Platform and More Attention to Theory. Front. Plant Sci. 10:1339. doi: 10.3389/fpls.2019.01339
Received: 15 May 2019; Accepted: 25 September 2019;
Published: 22 October 2019.
Edited by:
Michelle Watt, Julich Research Centre, GermanyReviewed by:
Richard Richards, Commonwealth Scientific and Industrial Research Organisation, AustraliaCopyright © 2019 Sadras. This is an open-access article distributed under the terms of the Creative Commons Attribution License (CC BY). The use, distribution or reproduction in other forums is permitted, provided the original author(s) and the copyright owner(s) are credited and that the original publication in this journal is cited, in accordance with accepted academic practice. No use, distribution or reproduction is permitted which does not comply with these terms.
*Correspondence: Victor O. Sadras, dmljdG9yLnNhZHJhc0BzYS5nb3YuYXU=