- 1Department of Plant Sciences, Faculty of Biosciences, Norwegian University of Life Sciences, Ås, Norway
- 2Institute of Biological, Environmental and Rural Sciences, Aberystwyth University, Aberystwyth, United Kingdom
Survivor populations of red clover (Trifolium pratense L.) from plots in a field experiment in southern Norway were genetically characterized using genotyping by sequencing, and compared with the original population and each other. Genetic differentiation between populations was characterized on the basis of allele frequencies of single nucleotide polymorphisms (SNPs), using principal component analysis. SNPs that had been under selection, i.e., SNPs with significantly different allele frequencies in survivor populations relative to the original population, or between survivor populations that had received different treatments, were identified by analysis of FST values, using BayeScan and a simple and stringent FST-based test utilizing replicate populations from the field experiment. In addition, we tested the possibility of pooling DNA samples prior to sequencing, and pooling leaf samples prior to DNA extraction and sequencing, followed by allele frequency estimation on the basis of number of variant reads. Overall, survivor populations were more different from each other than from the original population, indicating random changes in allele frequency, selection in response to local variation in conditions between plots in the field experiment, or sampling error. However, some differentiation was observed between plots sown as pure stands or species mixtures, plots sown at different densities, and plots subjected to different harvesting regimes. Allele frequencies could be accurately estimated from pooled DNA, and SNPs under selection could be identified when leaf samples were pooled prior to DNA extraction. However, substantial sampling error required replicate populations and/or a high number of sampled individuals. We identified a number of chromosomal loci that had been under selection in pure stand plots relative to the original sown population, and loci that had been under differential selection in pure stands of red clover vs. red clover grown in species mixtures. These are all candidate loci for establishment success or persistence in red clover.
Introduction
Red clover (Trifolium pratense L.) is a perennial legume used extensively in production of silage and hay in temperate regions, where it is cultivated in mixture with grasses such as timothy, meadow fescue and perennial ryegrass. It has high yields, can largely replace nitrogen fertilization through its symbiosis with nitrogen-fixing rhizobia, and it contributes to protein-rich forage and high intake (Frame et al., 1998; Abberton and Marshall, 2005). However, although red clover is a perennial, its persistence is limited, and it is often the species that first disappears from a species mixture (Ms). Improving persistence is therefore, a major breeding goal in most breeding programs (Taylor, 2008; Boller et al., 2010). Red clover is an outbreeding species with a gametophytic self-incompatibility system (Taylor, 1982), and thus there is a considerable amount of genetic variation within cultivars, which are usually synthetic populations with a large number of parents. Red clover has a genome size of 420 Mb (Sato et al., 2005). Sequences of the red clover genome have been published (Ištvánek et al., 2014; De Vega et al., 2015), but only the latter of which represents a draft genome at pseudomolecule level, covering 309 Mb of the genome. This facilitates particularly the identification of genomic regions potentially under selection.
Persistence is a complex trait controlled by many different genetic and environmental factors. It may be defined purely as survival over years, or alternatively, as maintenance of annual yield over years. These two measures can be correlated (e.g., Herrmann et al., 2008), but there may also be negative associations between harvestable biomass produced and survival during a subsequent stressful period, such as a winter (Therrien and Smith, 1960; Ergon, 2017). Biotic and abiotic stresses such as fungal pathogens, nematodes and insect herbivores, abiotic stresses, and competition from grasses under high N fertilization levels, are factors that can cause reduced persistence of red clover (Lager and Gerhardson, 2002; Abberton and Marshall, 2005; Taylor, 2008; Boller et al., 2010; Annicchiarico et al., 2015). Cutting frequency has been found to be of lesser importance (Coulman and Kielly, 1988; Wiersma et al., 1998). Positive correlations have been found between persistence and stem height or leaf size (Herrmann et al., 2008) and adventitious root formation (Montpetit and Coulman, 1991a,b). The ability to regrow after repeated defoliation is likely to be related to presence of leaves low in the canopy, level of root energy reserves and number of crown buds, as indicated for lucerne (Brummer and Bouton, 1991, 1992). Little is known about the genetic control of persistence, but it is likely that interaction with environmental factors are important. To our knowledge only one report on QTLs for persistence (Herrmann et al., 2008), and very few reports on QTLs for related traits such as winter survival and disease resistance (Klimenko et al., 2010) and vigor (Herrmann et al., 2008) are published.
In agricultural fields in Norway, seed mixtures, often containing around 10% (weight) of red clover, are commonly sown at a rate of 20–30 kg ha-1. With a 1000 seed weight of 2 g (diploid red clover), this equates to a red clover seeding rate of around 1 × 106 – 1.5 × 106 seeds ha-1. Only a fraction of the sown plants will survive the first years due to competition and stress. For example, in pure red clover stands sown at a rate of 18 kg ha-1, 40, 27 and 18% of the number of plants expected to germinate according to a germination test, had survived by the end of the first (establishment year), second and third growing season, respectively (Marley et al., 2003). However, the initial survival rate is likely higher in species mixtures because intraspecific competition is usually higher than interspecific competition.
Natural selection can guide breeding (Henry and Nevo, 2014), particularly when it comes to a trait like the survival component of persistence. Selection of high persistence in breeding programs is usually done by selecting plants that have survived under field conditions for around 3 years. Such survivor populations of both red and white clover have been found to have experienced a shift in the genetic composition of the populations relative to the original populations, measured either with molecular markers or with phenotyping of offspring (Annicchiarico and Piano, 1997; Collins et al., 2001, 2002, 2012; Dalmannsdóttir et al., 2001; Frankow-Lindberg, 2001; Helgadóttir et al., 2001; Göransson et al., 2012; Ergon and Bakken, 2016). In white clover grown under Nordic conditions, such shifts have been associated with improved winter survival or related traits (Dalmannsdóttir et al., 2001; Frankow-Lindberg, 2001; Göransson et al., 2012).
In this paper, we utilized survivor populations to investigate whether non-random selection could be detected within one generation of red clover growing in the field for 2.5 years, and to what extent any such selection had acted on the population structure or on individual loci. We first aimed to identify loci controlling persistence by detecting loci with significantly altered allele frequencies in survivor populations compared to the originally sown population (study 1). We based our analyses on single nucleotide polymorphism (SNP) data obtained from genotyping by sequencing (GBS) of individuals from the original population and survivor populations. We then tested whether reliable allele frequencies could be obtained by sequencing pools of individual DNA samples rather than sequencing the individual samples themselves (study 2). Finally, we used GBS-derived SNP data from pools of leaf samples to investigate whether different loci had been selected in red clover survivors grown in pure stands as compared to red clover survivors grown in species mixtures (study 3).
Materials and Methods
Plant Material and Genotyping
The diploid red clover cultivar “Lea” (Graminor, Norway) was included in a larger field experiment, sown in two replicates at Ås, Norway, in June 2010 (Ergon et al., 2016, Supplementary Figure S1). The plot size was 7.5 m2, seeds were sown at a total seed rate of 10 (low) or 20 (high) kg ha-1, either as red clover pure stand (Ps) or as mixed stands (Ms) sown with equal amounts (seed weight) of red clover, white clover, perennial ryegrass and tall fescue. With an approximate thousand seed weight of diploid red clover of 2 g, this equals approximately 3750 or 7500 seeds per Ps plot, and 938 or 1875 seeds per Ms plot. Plots had been harvested either 3 or 5 times a year (3H and 5H). Leaf blades were sampled from survivor populations (i.e., plots) in October 2012, and stored at -80°C.
In 2013, DNA was extracted from leaves of 48 or 47 survivor plants randomly selected from Ps survivor populations sown at high seeding rate and harvested 3 or 5 times a year (two plots from each harvesting regime, sample set 1, Table 1), and from leaf samples of 88 individuals of the original population seeded in the greenhouse (sample set 2), using DNeasy 96 Plant Kit (Qiagen). GBS libraries were made for each of the 278 individuals. In order to test how well allele frequencies can be estimated from DNA pools, equal amounts of DNA from the 88 individuals of the original population were pooled and distributed among seven tubes from which 7 replicate GBS libraries were made (sample set 3). GBS library preparation and sequencing, as well as SNP calling, was done at the Institute for Genomic Diversity, Cornell University, according to Elshire et al. (2011). The enzyme ApeK1 was used for digestion of genomic DNA, and the GBS UNEAK analysis pipeline, an extension to the Java program TASSEL (Bradbury et al., 2007), was used to call bi-allelic SNPs from the sequenced GBS libraries.
In 2017, DNA was also extracted from leaf samples of some of the other survivor populations that had been kept at -80°C. This time, DNA was extracted from pools of leaves (one leaf from each of 100 random individuals from each population, sample set 4, Table 1), using DNeasy Plant Maxi Kit (Qiagen). Leaves were sampled from four red clover Ps survivor populations, and four Ms survivor populations, all which had been harvested 3 times a year. Two plots of each stand type had been sown at high seeding rate (H) and two at low seeding rate (L). From the Ps H populations, three replicate samples, each consisting of one leaf from each of 100 random individuals, were sampled in order to evaluate the reproducibility of the sampling. GBS library preparation and sequencing, as well as SNP calling for the resulting 12 samples, was done at Beijing Genomic Institute, using ApeK1 as the restriction enzyme. Sequences were aligned to the red clover genome using SOAP (Li et al., 2009b), and bi-allelic SNPs were detected with SOAPsnp (Li et al., 2009a).
Analysis of Genotype Data
Study 1: Genetic Changes in Survivor Populations as Compared to the Originally Sown Population, Based on Genotyping of Individuals
For the libraries made from individuals in sample set 1 and 2 (Table 1), a minimum of ten reads in total, and, in the case of heterozygotes, a minimum of two reads for each of the alleles, was required to maintain the genotype for each genotype and SNP combination. For analysis of the changes in survivor populations (sample set 1) as compared to the original population (sample set 2), SNPs with an established genotype for a minimum of 25 individuals in all five populations, and minor allele frequency (MAF) > 0.05 in the original population, were used (4966 SNPs).
Principal component analyses (PCAs) were performed in The Unscrambler X v.10.3 (Camo Software, Norway) in order to visualize differentiation between populations as well as population structure. For the visualization of population differentiation MAF of each SNP in each population were used as input data. For visualization of population structure the genotype of each SNP and all individuals were used as input data.
In order to identify SNP loci that had been under selection two different methods were used. With the first method, a simple FST-based method, pairwise FST-values (original population vs. each of the four survivor populations) were calculated for each SNP and pair of populations as , where q is the allele frequency of a SNP variant and all the averages are calculated over the two populations compared. Secondly, a chi-square test was used to identify SNPs with significant FST’s at different significance levels, using the test statistic X2 = 2NFST, where 2N = the sum of genotyped gametes in the two populations (Hedrick, 2011). Thirdly, SNPs with significant FST in all four survivor populations relative to the original population, were identified. Only these SNPs were regarded as having different allele frequency in survivor populations as compared to the original population. In order to control the high rate of false positives that can occur in multiple testing, corresponding overall estimates of the false discovery rate (FDR) were calculated for each significance level as , where l = number of SNP loci tested (4966), P = the significance level of the individual chi-square tests, n = the number of survivor populations tested against the original population (4), and d = the number of SNP loci identified with a significant FST in all n population pairs. For each SNP with a significant FST in all four survivor populations relative to the original population, the significance of the difference between the allele frequency in the original population and the combined population of 190 genotyped survivors (all four plots) was confirmed with Fishers exact test, using the tool at http://lh3lh3.users.sourceforge.net/fisher.shtml. The 64 bp tag sequences containing significant SNPs were blasted against the red clover draft genome (De Vega et al., 2015) at https://legumeinfo.org/, in order to identify map locations (defined as the best hit with at least 57 bp aligned and a maximum e-value of 1 e-18) and surrounding candidate genes.
Single nucleotide polymorphism outliers were also detected with BayeScan v 2.1 (Foll and Gaggiotti, 2008), using default input parameters. The allele frequency dataset described above was converted to allele numbers using the number of haploid genomes that had been genotyped for each population. BayeScan uses logistic regression to decompose locus-population FST-values into population-specific and SNP-specific components. Population-specific FST-values are based on the comparison between each population and the pool of all populations in the analysis. Outlier SNPs are identified as those, where the SNP-specific component is necessary to explain the observed variation. We ran the analysis with five defined populations (four survivor populations and the original population) and three defined populations (data from 3H populations pooled, data from 5H populations pooled, original population).
Study 2: The Use of Pooled Samples to Predict Allele Frequencies in Populations
For the seven libraries made from pools of individual DNA samples (sample set 3, Table 1), the sequence reads were filtered for minimum 10 and maximum 126 reads per SNP and replicate library. Reads were subsequently pooled across libraries, and allele frequencies were calculated based on number of reads. For comparison of allele frequencies obtained from GBS of individuals (sample set 2) vs. pooled DNA (sample set 3), SNPs with an established genotype for a minimum of 25 individuals and MAF > 0.05 among individuals in the original population, were used (8218 SNPs).
Study 3: Differential Selection in Pure Stands vs. Mixed Stands, Based on Genotyping of Pooled Tissue Samples
Sequencing of libraries made from the DNA extracted from leaf pools in sample set 4 (Table 1) generated 8294 SNPs with 100–499 reads and MAF > 0.05 within all 12 samples. We discarded those that mapped to scaffolds not yet assigned a chromosomal locations, leaving 4556 SNPs for analysis. Allele frequencies were estimated on the basis of the number of reads. Differentiation between populations and replicate samples was analyzed with PCA as in experiment 1.
In order to identify SNPs potentially differentially selected in plots receiving different treatments, we used the same two methods as in study 1. For the simple FST-based method, pairwise FST values between each of the four Ps populations and the average of the Ms populations, and between each of the four Ms populations and the average of the Ps populations, were calculated for each SNP. For the two plots with replicate samples, the average allele frequencies for each plot were used. Allele frequency differences were tested for significance as described in study 1. For a SNP locus to be identified as having been under differential selection, it was required that all eight FST values were significant. Estimates of FDR were calculated as in study 1, with l = 4556 and n = 4, because there are only four independent FST values. Fishers exact test was not performed due to the lack of individual genotypes.
BayeScan compares each population with all the other populations in the analysis. An identified SNP outlier may therefore not necessarily vary consistently between, e.g., all Ps and Ms populations. We therefore tested the effect of stand type, seeding density and the interaction for each significant SNP with a two-way analysis of variance using the GLM procedure in SAS Enterprise Guide v. 6.1, in order to identify SNPs that had significantly different allele frequencies in either different stand types or in different seeding densities. We also ran a BayeScan analysis in which the data from each of the eight populations were combined in two main groups, Ps and Ms.
Results
Study 1: Genetic Changes in Survivor Populations as Compared to the Originally Sown Population, Based on Genotyping of Individuals
In study 1, the set of SNPs with at least 25 genotyped individuals in each of the five population samples and MAF > 0.05 in the original population (4966 SNPs), were used to characterize the genetic changes that had occurred in the field over the two and a half years. A PCA of MAF for each SNP and population showed that along the two first axes, the survivor populations had diverged from the original population in different directions (Figure 1). The two populations from the 5H harvesting regime were more similar to each other than the two populations from the 3H harvesting regime. The first axis, explaining 29% of the variation, separated the 3H populations from the 5H populations. The second axis (28% of the variation) separated the two 3H populations, and the third axis (25% of the variation) separated the two 5H populations. These results suggest that the survivor populations had diverged from the original population in different directions, and that most of the observed difference in allele frequencies was random.
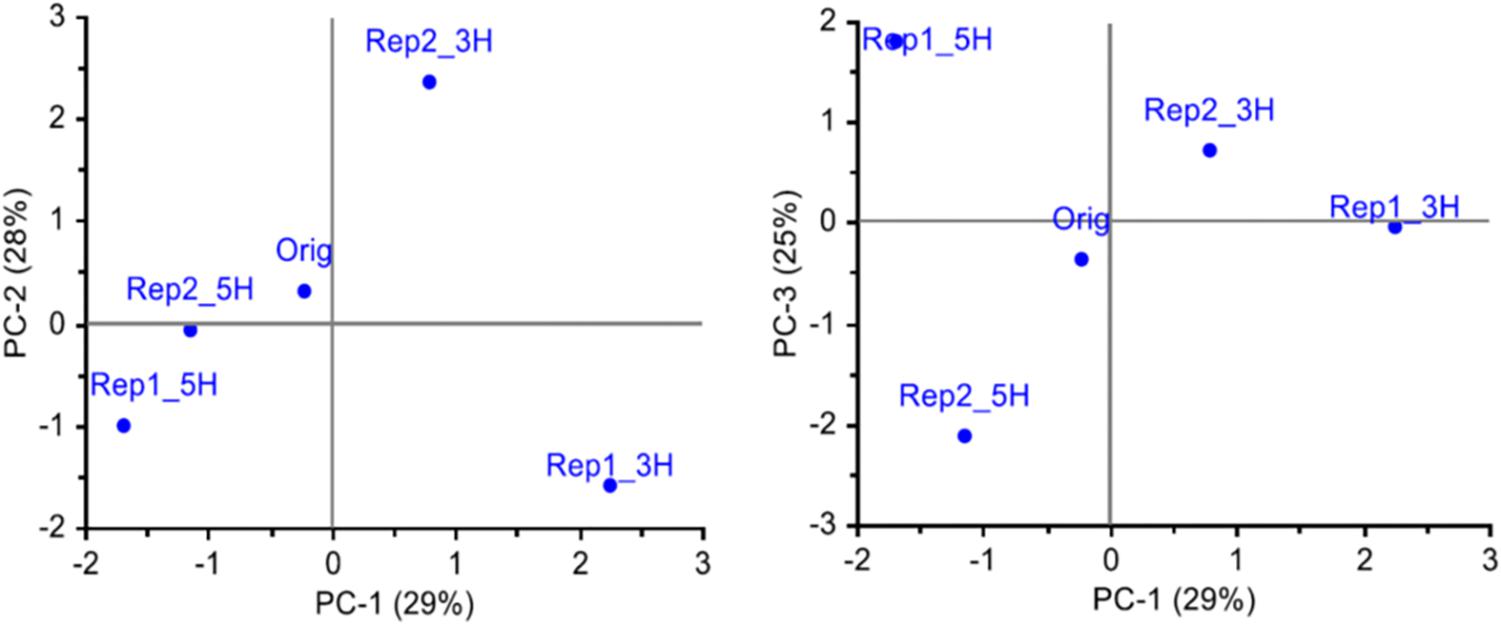
Figure 1. Genetic differentiation between the original population (Orig, 88 individuals) and four survivor populations sampled from four plots (47–48 individuals per population) in a field experiment 2.5 years after sowing, i.e., all plants are in the same generation (study 1). The survivor populations had been harvested three (3H) or five (5H) times a year, and there were two replicate plots of each harvesting regime. All SNPs that were successfully genotyped for a minimum of 25 individuals from each population, and had a MAF > 0.05 in the original population, were included (4966 SNPs). The three first principal components explaining the variation in allele frequencies are shown.
Population structure was analyzed with PCA of all individuals and the 4966 SNPs. The same weak structure observed in the original population, with one major and one minor subpopulation, was observed in each of four survivor populations (Figure 2). The two first principal components only explained 2 and 1% of the variation, respectively. The proportion of individuals in the minor cluster were 0.17 and 0.15 – 0.23 for the original population and the four survivor populations, respectively, indicating that the survival/mortality that had occurred in the field had not favored one subpopulation over the other.
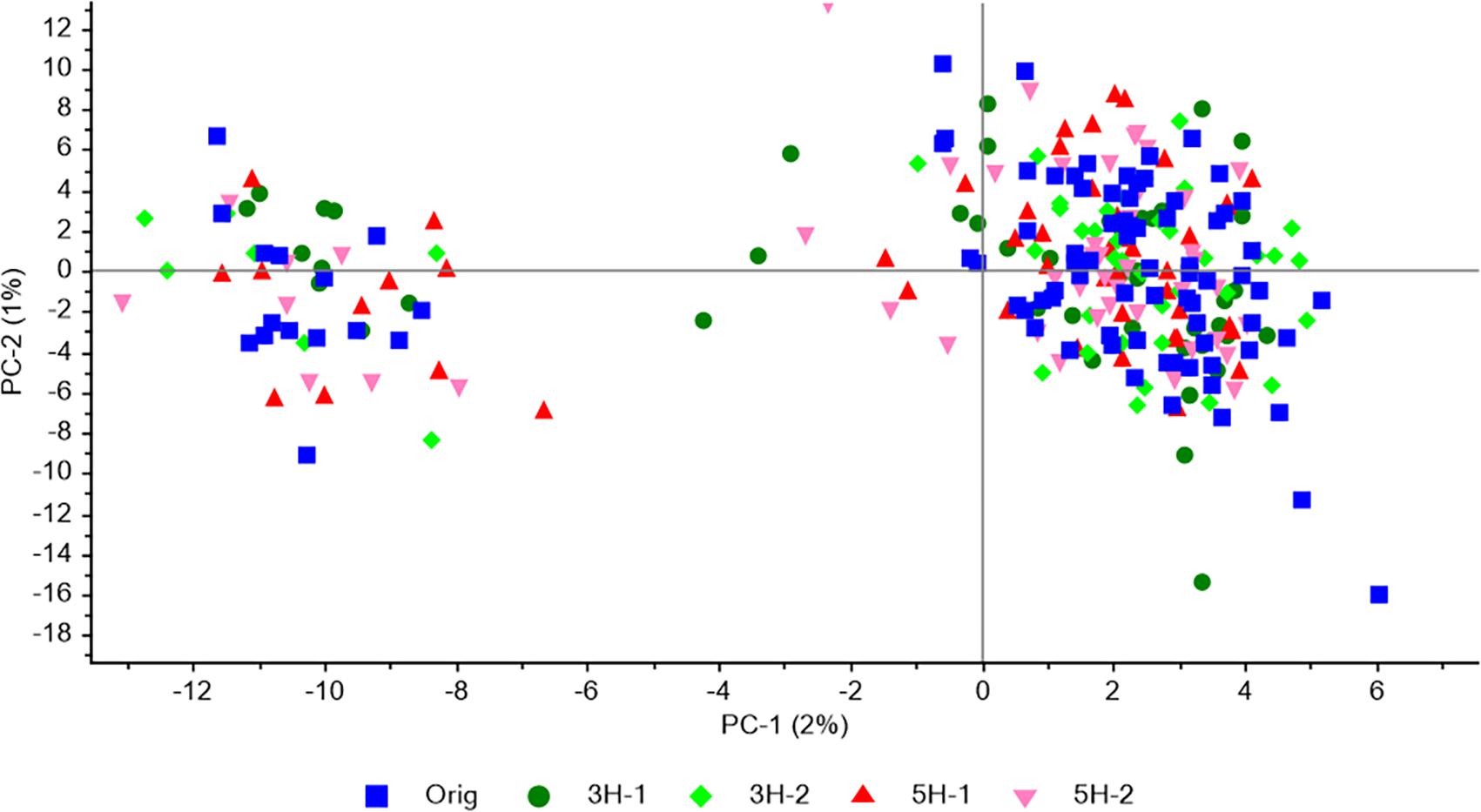
Figure 2. Population structure revealed by PCA of SNP genotype data for 88 individuals from the original population together with 47 or 48 individuals from each of the four survivor populations in study 1. All SNPs that were successfully genotyped in a minimum of 25 individuals from each population, and had a MAF > 0.05 in the original population, were included (4966 SNPs).
The 4966 SNP loci were first screened for significant shifts in allele frequency between the original population and survivor populations with a simple test using FST values and a chi square test combined with FDR. When testing individual survivor populations separately, or when combining all survivors in one common population, significant FDR values could not be obtained; they were in the range of 0.4 – 0.8. Thus, the number of SNP loci identified was only about twice of what could be expected from chance alone. However, it is very unlikely that a locus has a shift in allele frequency due to chance alone in all four survivor populations. We therefore looked for SNP loci that had a significant shift in allele frequency in all four populations, and calculated a modified FDR accounting for this reduced number of expected false positives (see section “Materials and Methods”). Twenty-seven SNPs had a shift in allele frequency at P < 0.1 in all four survivor populations with an overall corresponding FDR of 0.018. Thirteen of these were also significant at P < 0.05 in all survivor populations with an overall FDR of 0.002 (Supplementary Table S1). The average shifts in allele frequency of the 27 SNPs across the four survivor populations ranged from 0.09 to 0.22 and the average FST values ranged from 0.017 to 0.061 (Table 2). For comparison, the FST value averaged across survivor populations and all 4966 loci was 0.005. When we tested the allele frequency difference of the 27 SNPs between all 190 survivors and the original population using Fishers exact test, all SNPs were significant at P < 0.01 - 0.000008 (Table 2). Sequence tags of 20 of the 27 SNPs could be mapped onto the red clover genome. Fourteen SNPs mapped to chromosomes (between two and four SNPs on each of chromosome 1, 2, 3, 4, and 7) (Table 2 and Figure 3), and six mapped to scaffolds not yet assigned to chromosomes (Supplementary Table S2).
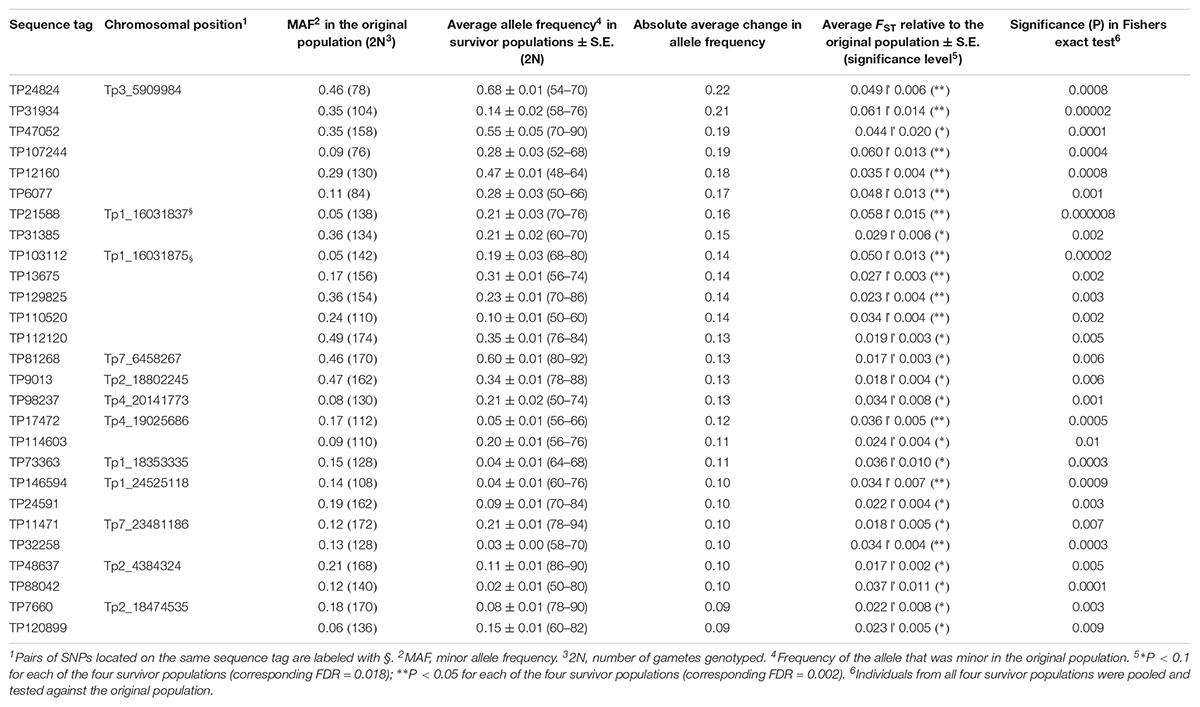
Table 2. Sequence tags containing SNPs with a significant shift in allele frequency in four red clover pure stand survivor populations relative to the originally sown population (study 1), identified using a simple FST-based method.
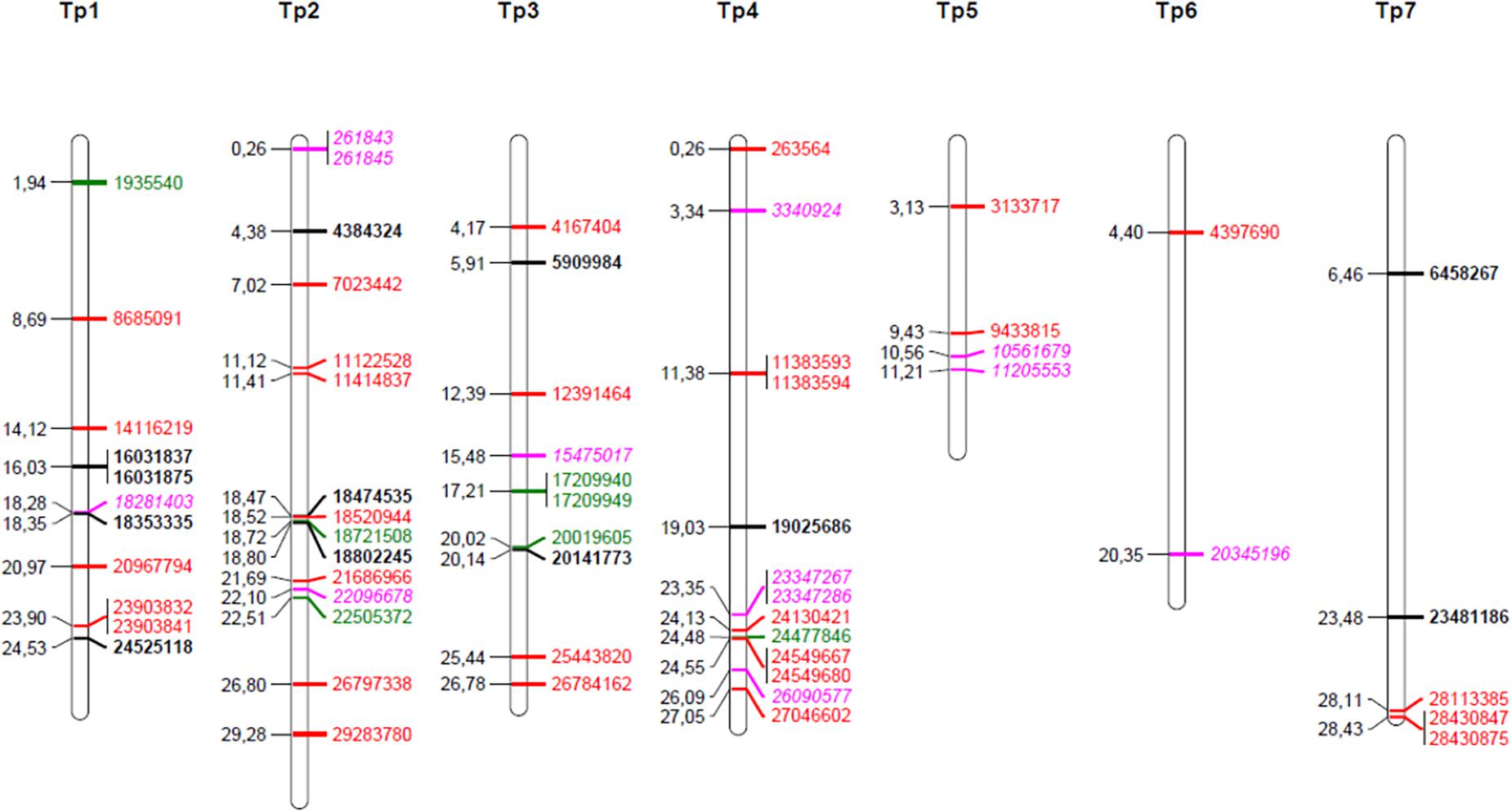
Figure 3. Map positions of chromosomal SNPs found to have been under selection. Black bold; selected in four survivor populations (pure stand, sown at high density) as compared to the original, sown population (study 1), red; differentially selected in survival populations from pure stand as compared to survival populations from species mixtures (study 3), green; differentially selected in populations as compared to populations sown at low seeding density (study 3), pink italic, selection affected by both stand type and seeding density (study 3).
BayeScan did not detect any significant SNP outliers (FDR 0.05) when all five populations were defined, but when we combined the two 3H populations into one population, and the two 5H populations into another, in addition to the original population, one significant SNP was identified. This SNP, Tp3_16031875, was also identified with the simple FST-based method (Table 2). We also attempted to identify SNP loci that had been under specific selection in either of the two harvesting regimes by looking for significant allele frequency differences between the 3H and the 5H survivor populations, using the simple FST-based method. No such SNP loci could be detected, possibly due to the fact that there were only two replicate plots of each harvesting regime, and thus less power in the test.
Study 2: The Use of Pooled Samples to Predict Allele Frequencies in Populations
A comparison was made between the allele frequencies obtained by genotyping individuals from the original population, with the allele frequency estimates obtained when sequencing a pool of equal amounts of DNA from each individual (sample set 2 and 3). The inaccuracy of the allele frequency estimates based on GBS of pooled DNA was higher for SNPs with less than 50 reads or more than 600 reads from the sequencing of the pool (Table 3). The best accuracy was obtained between 150 and 399 reads. When only SNPs in this range were included (2313 SNPs), the average deviation in allele frequency was 0.036 and the correlation 0.98. Expanding the range to 100–499 reads resulted in a much higher number of SNPs (3726), an average deviation of 0.039 and a correlation of 0.97 (Figure 4).
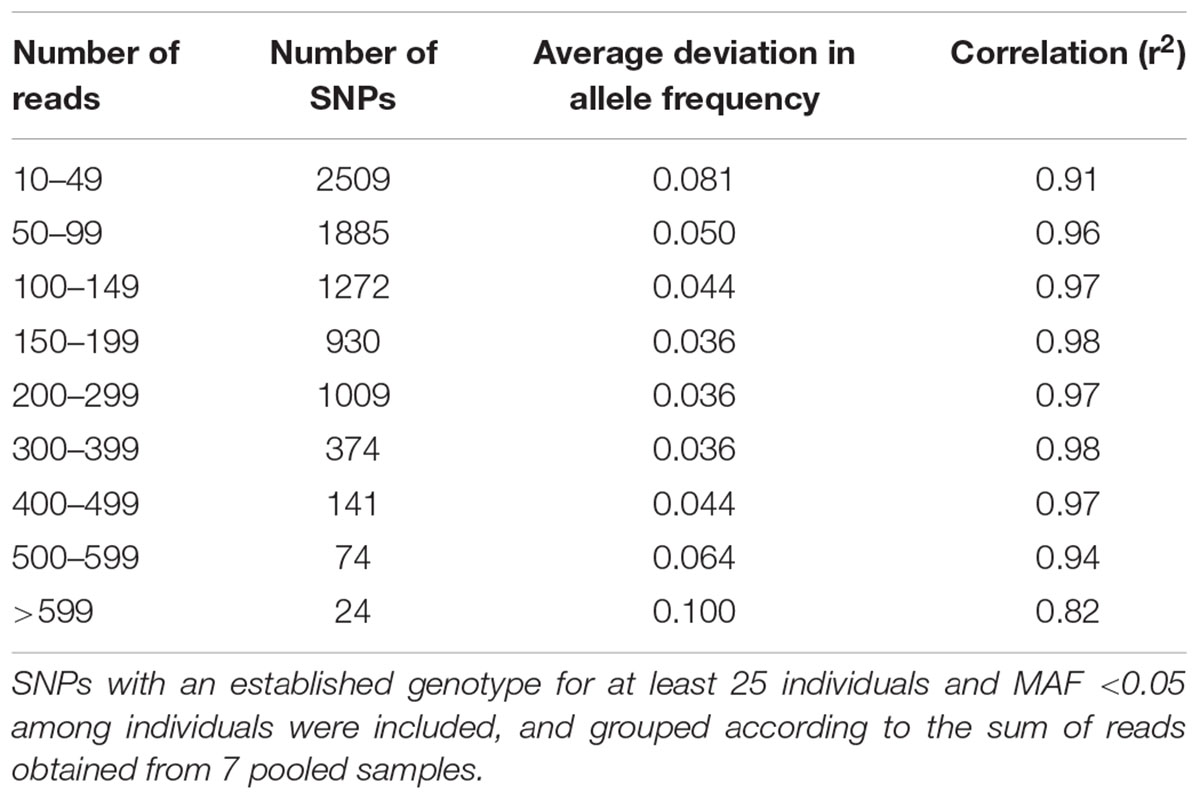
Table 3. Average deviation in allele frequency estimates obtained when genotyping pooled samples consisting of equal amounts of 88 individuals as compared to genotyping the individuals separately (study 1).
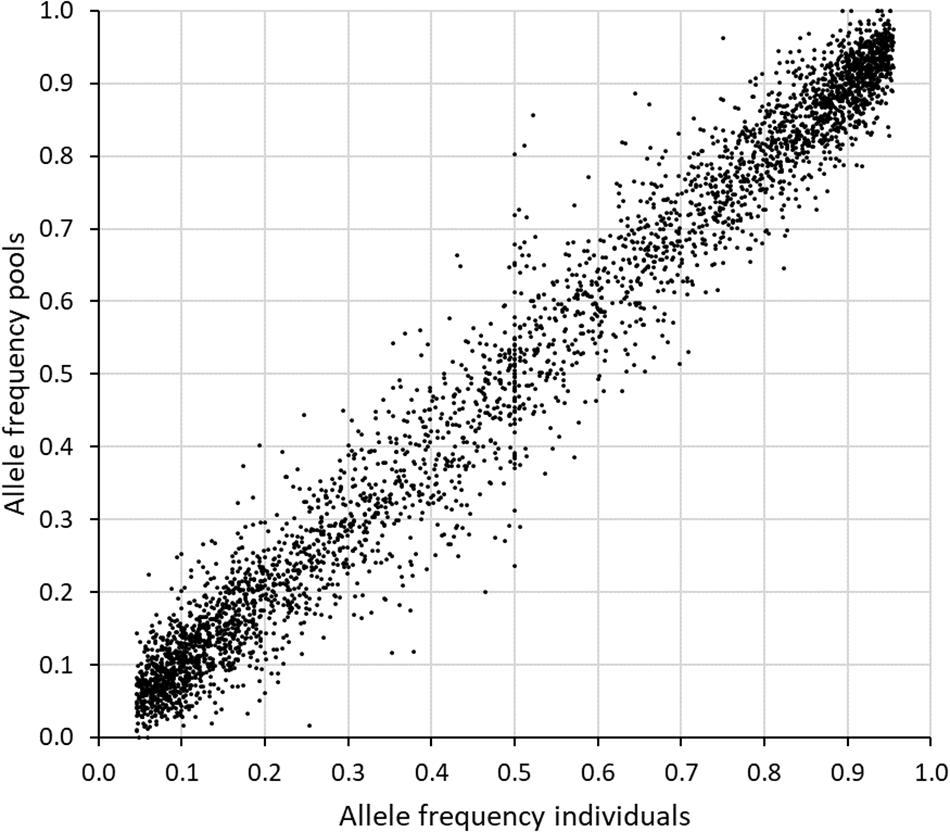
Figure 4. Comparison of the allele frequencies obtained when genotyping 88 individuals separately (x-axis) with those obtained when genotyping pools of equal amount of DNA from each individual (y-axis) in study 2. Seven GBS libraries were made from the pooled sample and the reads from these were combined prior to calculation of allele frequencies. The 3716 SNPs with an established genotype for at least 25 individuals, MAF > 0.05 among individuals, and 100–499 reads in the pooled sample, were included here.
Study 3: Differential Selection in Pure Stands vs. Mixed Stands, Based on Genotyping of Pooled Tissue Samples
Study 3 served both to investigate the possibility of pooling individual leaf samples prior to DNA extraction and GBS, and to investigate the possible differential selection that had occurred in survivor populations as a result of different stand types and seeding densities. A comparison was made between allele frequencies obtained from genotyping of DNA extracted from three replicate pools of leaf tissue from each of two Ps H populations, each pool consisting of 100 leaves. The average pairwise deviation in allele frequency between replicates was 0.056–0.057, while the average pairwise correlation of allele frequencies (r2) was 0.90 in both populations. Principal component analysis (PCA) (Figure 5A) revealed that the random variation between replicate samples from the same population was at least as large as the variation between samples from different populations. When allele frequencies were averaged across the three replicates this random variation appeared to be reduced (Figure 5B), indicating that a large part of the variation between samples was due to random variation which was reduced with the averaging of the three replicates.
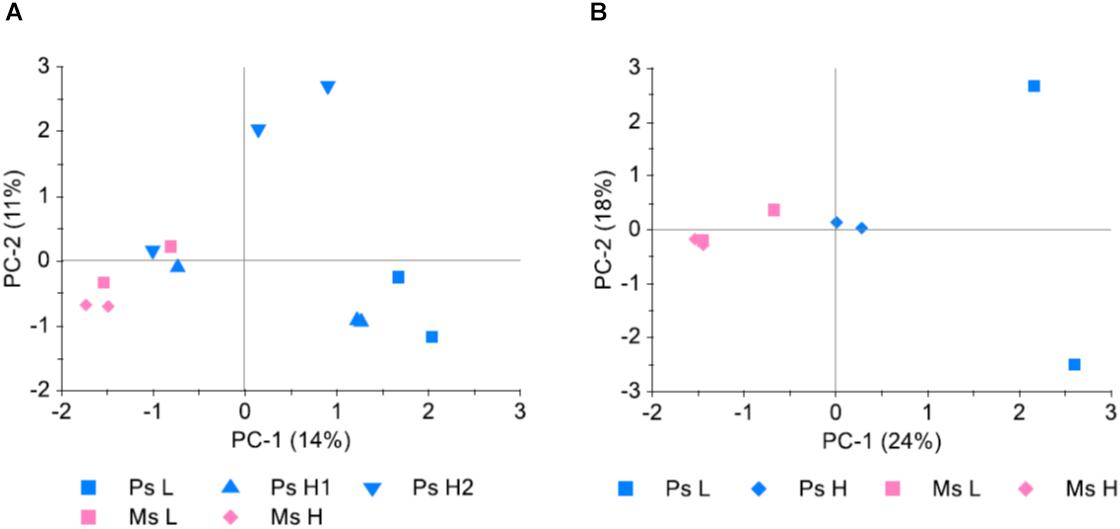
Figure 5. Genetic differentiation between survivor populations sampled from four pure stand plots (Ps, red clover only) and four mixed stand plots (Ms, red clover growing in mixture with white clover, perennial ryegrass and tall fescue), sown at high (H) or low (L) seeding density (study 3). Hundred leaves were collected from each plot and pooled prior to DNA extraction and GBS. For the Ps H plots (Ps H1 and Ps H2), three replicate samples, each consisting of 100 leaves, were sampled. SNPs which could be mapped to chromosomes and had 100–499 reads and MAF >0.05 were included (4556 SNPs). (A) Principal component analysis (PCA) of minor allele frequencies (MAF) in all 12 samples. (B) PCA of MAF in the 8 different populations. MAF for Ps H populations was the average of the three replicate samples.
The first principal component, explaining 24% of the variation in MAF between populations, separated Ms, Ps H, and Ps L populations (Figure 5B), suggesting differential selection as a result of the different treatments in the field experiment. The largest differences were between populations belonging to different stand types. There was also a difference between Ps populations sown at different seeding densities, possibly only detectable in Ps due to the higher number of individuals genotyped in Ps H populations.
The simple FST-based method identified 11 SNPs at P < 0.1 (FDR 0.04), six SNPs at P < 0.05 (FDR 0.005) and one SNP at P < 0.01 (FDR 0.00005) (Figure 3, Table 4, and Supplementary Table S3). The difference in allele frequency between the average Ps population and the average Ms population for the 11 SNPs ranged from 0.17 to 0.37, and the average FST-values ranged between 0.037 and 0.150, as opposed to a value of 0.005 across all 4556 SNPs. Thus, the differentiation between survival populations in this study was larger than the differentiation between the original population and survivor populations in study 1. BayeScan identified 156 SNPs with deviating allele frequency in at least one of the eight populations sampled (FDR 0.05). Testing each of these SNPs with analysis of variance showed that stand type had a significant effect on the allele frequency of 42 of these SNPs, while seeding density had an effect on 19, and 12 were affected by both factors (P < 0.05, Table 4). BayeScan analysis with only two defined populations – Ps and Ms – identified 59 significant SNPs. Thirty-three of these were among those 42 that had been identified as being affected by stand type. The 11 SNPs identified with the simple FST-based method were also identified in both BayeScan analyses, and among those for which there was a significant effect of stand type.
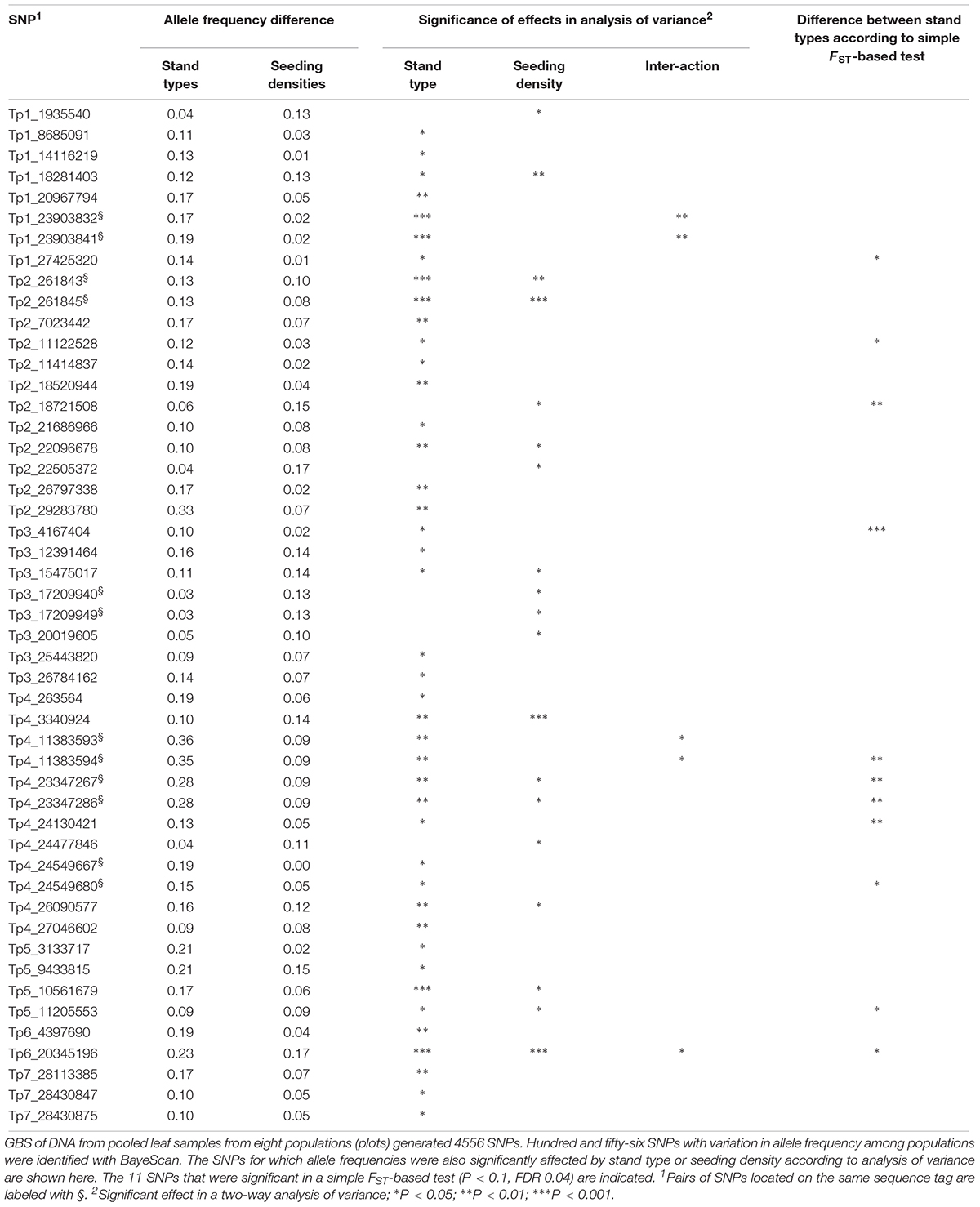
Table 4. Single nucleotide polymorphisms (SNPs) with different allele frequencies in red clover populations 2.5 years after being sown in Ps orMs, and with low or high seeding density (study 3).
Chromosomal Regions of Identified SNPs Under Selection
The identified SNPs under selection were spread across all seven red clover chromosomes, and were in some cases closely or moderately linked (Figure 3). We took a closer look at the chromosomal regions around the SNPs with the largest allele frequency differences between populations (further details are found in Supplementary Tables S2, S4). The SNP with the largest allele frequency difference, a difference of 0.36 between Ps and Ms populations, was Tp4_11383593/11383594 located on chromosome 4. This SNP was not located in a known gene. There were also several SNPs located toward the distal end of chromosome 4. Among these, Tp4_23347367/23347286 and Tp4_24549667 had allele frequency differences between Ps and Ms populations of 0.28 and 0.19, respectively. The former one was located near an annexin and the latter one was located in an oxygenase and close to a transcription factor. The SNP with the second largest allele frequency difference between Ps and Ms (0.33) was Tp2_29283780 on chromosome 2. It was located in a stress-induced phosphoprotein and close to a syntaxin. Further up on chromosome 2 there was a region with many SNPs with moderate allele frequency differences between populations. Among these, Tp2_18474535, Tp2_18520944 and Tp2_18802245, were located in a WRKY family transcription factor, close to an LRR-like protein kinase, and in a RNA-binding protein, respectively. The SNP with the largest shift in allele frequency between the original population and the four survivor populations in study 1 was Tp3_5909984, with an average change in allele frequency of 0.22. This SNP was located in one of three adjacent membrane transport protein-like genes.
Discussion
Methodology
Several statistical methods have been developed to scan large numbers of loci across many individuals and link patterns of genetic variation to environmental variation (Holderegger et al., 2008; Schoville et al., 2012; Pannell and Fields, 2014). These methods identify outlier loci – loci with stronger differentiation in allele frequencies between populations than can be expected to occur due to random processes only, and which are, therefore, assumed to have been under selection. Statistically significant associations between genetic variation in outlier loci and variation in environmental variables indicate a role of the outlier loci in local adaptation. Adaptive outlier loci may represent new beneficial mutations that have increased in frequency and eventually become fixed in the population (hard sweeps). Alternatively, outlier loci represent alleles or haplotypes that have increased in frequency, but where some polymorphism is maintained (soft sweeps) (Barrett and Schluter, 2007). Soft sweeps can occur when selection on standing variation acts on multiple haplotypes in the genome simultaneously. Studies of local adaptation usually compare populations that have been exposed to contrasting conditions over many generations, and, in spite of migration, have evolved through repeated cycles of recombination and selection (e.g., Freeland et al., 2010; Turner et al., 2010; Gould et al., 2014). In some cases, such studies include replicates of populations that have started out from a common pool and been exposed to the same conditions; these replicates can be used to separate consistent signs of selection from random changes like genetic drift (Wiberg et al., 2017). The present study is different from these studies in the way that we characterize the selection (mortality/survival) that occurs within one generation only, with no reproduction or migration occurring. This allows for the use of a simple FST-based test of changes in allele frequencies resulting from selection. We show that in spite of a large proportion of random mortality/survival, the use of several replicate survivor populations, sampled from replicate plots in a field experiment, improves the power of the test substantially, and makes it possible to remove these random effects and identify loci that have been under selection in all replicates. In study 1, BayeScan identified one of the SNP outliers identified by the simple FST-based method, after combining the 3H and 5H replicates. In contrast, in study 3, where a higher number of individuals were pooled in each population sample and the differentiation between populations was larger than in study 1, BayeScan identified more potential outliers than the simple FST-based method. Testing these SNPs further with analysis of variance made it possible to identify differential selection due to stand type and/or seeding density. In study 3, all outliers identified by the simple FST-based method were included among those identified by BayeScan.
In order to be able to detect all loci with differences in allele frequency, it is necessary to have a sufficient coverage of the genome, i.e., a sufficiently high SNP density. A high SNP density can be achieved by using a restriction enzyme in the GBS protocol which is a frequent cutter (i.e., ApeK1, which we used), combining several restriction enzymes, and by sequencing to a sufficient read depth to be able to call SNPs and determine allele frequencies for the majority of restriction sites. The required SNP density depends on the linkage disequilibrium (LD) of the population. The lower the LD, the higher the SNP density needed in order for all genes to be in some degree of linkage with at least one nearby SNP. Red clover has a relatively small genome (approximately 420 Mb), facilitating good read depth relative to the sequencing effort, but varieties tend to have limited LD. The LD along the different chromosomes in the original population studied here has previously been characterized by De Vega et al. (2015), who found that the average LD, measured as R2, at distances of 100 Kb, ranged between 0.19 and 0.25 for the different chromosomes. At 500 Kb LD had decayed completely to background levels (R2 0.02–0.05). The likelihood of detecting a locus with significantly different allele frequency in different populations depends on the magnitude of the allele frequency difference, the distance between the gene conferring the effect on survival and a linked SNP, and the LD in that specific region. Here, we obtained an average density of one SNP per 85 kb or 37 kb in study 1 and in study 3, respectively. The studied variety is a synthetic population with several possible haplotypes at any given chromosomal segment, thus all nearby SNPs might not necessarily be diagnostic, that is, distinguish between alleles with different effects on survival. Therefore, with the SNP densities obtained in our study, we are likely to pick up a substantial amount of loci affecting survival, but not all, particularly not in study 1.
Pooling of individual DNA samples, or of individual leaf samples prior to DNA extraction, can increase the allele frequency information obtained per sequencing effort, and allow for comparison of a large number of populations (Turner et al., 2010; Byrne et al., 2013; Wiberg et al., 2017). While sequencing of individuals requires a certain read depth in order to call SNPs and distinguish between homozygotes and heterozygotes, sequencing pools requires an even higher read depth for allele frequencies to be estimated accurately. Moreover, information about haplotypes and population structure is lost when sequencing pools. In our study, a very good correlation was obtained between allele frequencies obtained from a DNA pool of 88 individuals and allele frequencies obtained from genotyping of individuals (Figure 4 and Table 3). Read depth was increased only 7 times in the pool relative to the 88 individual samples (i.e., >10x reduction in sequencing effort), and a similar number of SNPs were obtained. At a MAF > 0.05 and a read depth in pools of 100-499, R2 was 0.97, while it was somewhat lower at lower and higher read depth. At the same MAF and read depth range, pooling of leaves of 100 plants prior to DNA extraction led to an average correlation of 0.87 and 0.90 in two sets of three replicates. This is slightly lower than that reported by Byrne et al. (2013), who obtained a correlation of R = 0.91 (R2 = 0.93) at MAF > 0.05 and read depth above 20x in replicate samples of leaves from around 200 perennial ryegrass seedlings. Pooling of individual leaf samples prior to DNA extraction reduces costs, but the accuracy of the allele frequency estimates is also reduced. Estimates could possibly have been improved if we had used more uniform leaf material and taken more care in sampling equal amounts of tissue from each individual. However, the use of several replicate populations compensates to some extent for the reduced accuracy of allele frequency estimates. The replicate samples from two of the populations in study 3 showed that there was considerable sampling error in our method. This could be overcome by sampling more individuals and/or by including replicate samples or populations in the study.
Selection Occurring in the Field Within One Generation
The 88 plants in the original population sample represent the sown populations while the survivor populations represent subsets remaining in each plot after selection (survival) during 2.5 years of exposure to the prevailing field conditions and management. Such selection within one generation represents the environmental flexibility that the genetic variation within populations of outcrossing species can provide (Charles, 1964; Crossley and Bradshaw, 1968). Some alleles may contribute to yield in some environments, while other alleles contribute in other environments, making the population or cultivar robust to environmental variation. Our analyses of the genetic variation in the survivor populations as compared to the original population that was sown (study 1) showed that the survivor populations in four different plots had diverged from the original population in different directions. Thus, although the first PC-axis separated the two harvesting regimes (Figure 1), most of the allele frequency variation was random. This may reflect a response to unintended variation in the environment among plots, random selection of alleles at the majority of loci, or sampling error. The original population had only a very weak genetic structure, which remained in the survivor populations, indicating that there was no selection acting on the structure (Figure 2). In study 3, the first PC-axis separated Ps from Ms, and within Ps it separated the two seeding densities, suggesting that differential selection had occurred due to the different treatments (Figure 5).
If the original population has high genetic diversity and low LD (typical of forage cultivars), it cannot be expected that selection acting on a relatively limited number of loci will affect average genetic distance measured across the genome. In order to identify such selection, each individual locus must be considered. Indeed, by looking for allelic shifts of individual SNPs in several replicate survivor populations we identified loci that had been systematically selected under the prevailing conditions in the investigated field experiment (Figure 3). These are candidate loci for establishment success or persistence. In study 1, 12 SNPs, representing 11 loci, had significantly altered allele frequencies, measured as FST, in Ps survivor populations (high seeding rate) relative to the original population. These SNPs represent loci with alleles conferring a higher likelihood for survival under the conditions that are common to all four plots. They may be related to, e.g., establishment, competition in Ps, winter survival and the general environmental and management conditions. The absolute average allele frequency changes detected ranged from 0.22 to 0.09. Tp3_5909984 was the SNP with the largest allele frequency shift from the original population to the survivor populations in study 1. It is located in the middle of the proximal half of Tp3. Interestingly, this is also the approximate location of the only QTL for persistence detected in a red clover mapping population of red clover by Herrmann et al. (2008).
In study 3, survivor populations were not compared with the originally sown population. Instead, survivors from Ps populations were compared to survivors from Ms populations, and survivors from populations sown at high seeding density was compared to survivors from populations sown at low density. A number of loci with allele frequencies indicating differential selection in Ps and Ms were identified. The absolute allele frequency changes detected were up to 0.36, suggesting that stand type exerted a relatively strong differential selection pressure. Red clover in mixture with perennial ryegrass and tall fescue experience earlier competition for light and possibly other resources, as the grasses grow and elongate earlier in the summer. Indeed, we have previously shown that offspring of survivor populations from Ms have earlier stem elongation than offspring from survivor populations from Ps (Ergon and Bakken, 2016), suggesting differential selection for earliness. Later in the summer, red clover plants are likely to experience stronger competition in Ps than in Ms, as individual red clover plants grow very large. Another condition that may vary between Ps and Ms is a stronger dependence of red clover plants on nitrogen fixation in Ms, as grasses have a more efficient nitrogen uptake and less is left for the clover.
Breeding, variety testing and seed multiplication of red clover occurs in Ps. Although seeding rates used usually are much lower (2–4 kg ha-1) than those in our experiment, our results suggest that unintended selection occurring in Ps during breeding and seed multiplication may not necessarily be in favor of good persistence in practical farming, were Ms are used.
Conclusion
Making use of replicate populations and a simple FST-based test, it was possible to identify loci that had been under selection within one generation in a red clover variety grown in a field experiment for two and a half years. Pooling of individual DNA samples or leaf samples before sequencing and estimation of allele frequencies reduce costs substantially, allowing analysis of multiple populations and treatments simultaneously. Sampling error must be controlled, e.g., by sampling a large number of individuals and/or sampling from several replicate populations. Characterization of genomic changes in survival experiments may be utilized in identification of genomic regions, genes and alleles conferring survival in red clover and other species under various environmental conditions, which again can be utilized in breeding. In addition to identifying loci associated with survival under the conditions prevailing in our field experiment, we have shown that there is differential selection occurring in pure stands of red clover as compared to red clover growing in species mixtures, suggesting that the use of pure stands in breeding might not identify the best genotypes for development of varieties to be used in species mixtures.
Author Contributions
OR initiated the research. ÅE designed the experiments and wrote the manuscript. ÅE and VS conducted the experiments. ÅE, LS, and VS analyzed the data. All authors corrected and approved the final version.
Funding
This work has received funding from the European Community’s Seventh Framework Programme (FP7/2007–2013) under the grant agreement number FP7-244983 (MULTISWARD).
Conflict of Interest Statement
The authors declare that the research was conducted in the absence of any commercial or financial relationships that could be construed as a potential conflict of interest.
Acknowledgments
We acknowledge the technical assistance of Sylvia Sagen Johnsen and Øyvind Jørgensen.
Supplementary Material
The Supplementary Material for this article can be found online at: https://www.frontiersin.org/articles/10.3389/fpls.2019.00718/full#supplementary-material
References
Abberton, M. T., and Marshall, A. H. (2005). Progress in breeding perennial clovers for temperate agriculture. J. Agr. Sci. 143, 117–135. doi: 10.1017/s0021859605005101
Annicchiarico, P., Barrett, B., Brummer, E. C., Julier, B., and Marshall, A. H. (2015). Achievements and challenges in improving temperate perennial forage legumes. Crit. Rev. Plant Sci. 34, 327–380. doi: 10.1080/07352689.2014.898462
Annicchiarico, P., and Piano, E. (1997). Effect of selection under cultivation on morphological traits and yield of ladino white clover landraces. Gen. Res. Crop Evol. 44, 405–410.
Barrett, R. D. H., and Schluter, D. (2007). Adaptation from standing genetic variation. Trends Ecol. Evol. 23, 38–44. doi: 10.1016/j.tree.2007.09.008
Boller, B., Schubiger, F. X., and Kölliker, R. (2010). “Red clover,” in Fodder Crops and Amenity Grasses, Handbook of Plant Breeding, eds B. Boller, et al. (Berlin: Springer Science+Business Media).
Bradbury, P. J., Zhang, Z., Kroon, D. E., Casstevens, T. M., Ramdoss, Y., and Buckler, E. S. (2007). TASSEL: software for association mapping of complex traits in diverse samples. Bioinformatics 23, 2633–2635. doi: 10.1093/bioinformatics/btm308
Brummer, E. C., and Bouton, J. H. (1991). Plant traits associated with grazing-tolerant alfalfa. Agronomy J. 83, 996–1000. doi: 10.2134/agronj1991.00021962008300060014x
Brummer, E. C., and Bouton, J. H. (1992). Physiological traits associated with grazing-tolerant alfalfa. Agronomy J. 84, 138–143. doi: 10.2134/agronj1992.00021962008400020003x
Byrne, S., Czaban, A., Studer, B., Panitz, F., Bendixen, C., and Asp, T. (2013). Genome wide allele frequency fingerprints (GWAFFs) of populations via genotyping by sequencing. PLoS One 8:e57438. doi: 10.1371/journal.pone.0057438
Charles, A. H. (1964). Differential survival of plant types in swards. Grass For. Sci. 19, 198–204. doi: 10.1111/j.1365-2494.1964.tb01162.x
Collins, R. P., Helgadóttir,Á, Fothergill, M., and Rhodes, I. (2001). Variation amongst survivor populations of two white clover cultivars collected from sites across Europe: morphological and reproductive traits. Ann. Bot. 88, 761–770. doi: 10.1006/anbo.2001.1462
Collins, R. P., Helgadóttir,Á, Fothergill, M., and Rhodes, I. (2002). Variation amongst survivor populations of white clover collected from sites across Europe: growth attributes and physiological responses to low temperature. Ann. Bot. 89, 283–292. doi: 10.1093/aob/mcf037
Collins, R. P., Helgadóttir,Á, Frankow-Lindberg, B. E., Skøt, L., Jones, C., and Skøt, K. (2012). Temporal changes in population genetic diversity and structure in red and white clover grown in three contrasting environments in northern Europe. Ann. Bot. 110, 1341–1350. doi: 10.1093/aob/mcs058
Coulman, B. E., and Kielly, A. (1988). The effect of seeding rate and cutting frequency on the yield and persistence of red clover. Can. J. Plant Sci. 68, 733–742. doi: 10.4141/cjps88-086
Crossley, G. K., and Bradshaw, A. D. (1968). Differences in response to mineral nutrients of populations of ryegrass, Lolium perenne L. and Orchardgrass, Dactylis glomerata L. Crop Sci. 8, 383–387.
Dalmannsdóttir, S., Helgadóttir,Á, and Gudleifsson, B. E. (2001). Fatty acid and sugar content in white clover in relation to frost tolerance and ice-encasement tolerance. Ann. Bot. 88, 753–759. doi: 10.1006/anbo.2001.1465
De Vega, J. J., Ayling, S., Hegarty, M., Kudrna, D., Goicoechea, L., Ergon, Å, et al. (2015). Red clover (Trifolium pratense L.) draft genome provides a platform for trait improvement. Sci. Rep. 5:17394. doi: 10.1038/srep17394
Elshire, R. J., Glaubitz, J. C., Sun, Q., Poland, J. A., Kawamoto, K., Buckler, E. S., et al. (2011). A robust, simple genotyping-by-sequencing (GBS) approach for high diversity species. PLoS One 6:e19379. doi: 10.1371/journal.pone.0019379
Ergon, Å (2017). Optimal regulation of the balance between productivity and overwintering of perennial grasses in a warmer climate. Agronomy 7:19. doi: 10.3390/agronomy7010019
Ergon,Å, and Bakken, A. K. (2016). Red clover traits under selection in mixtures with grasses versus pure stands. Grassl. Sci. Eur. 21, 811–813.
Ergon,Å, Kirwan, L., Bleken, M., Skjelvåg, A. O., Collins, R. P., and Rognli, O. A. (2016). Species interactions in a grassland mixture under low nitrogen fertilization and two cutting frequencies: I. dry matter yield and dynamics of species composition. Grass Forage Sci. 71, 667–682. doi: 10.1111/gfs.12250
Foll, M., and Gaggiotti, O. E. (2008). A genome scan method to identify selected loci appropriate for both dominant and codominant markers: a bayesian perspective. Genetics 180, 977–993. doi: 10.1534/genetics.108.092221
Frame, J., Charlton, J. F. L., and Laidlaw, A. S. (1998). Temperate Forage Legumes. Wallingford: CAB International.
Frankow-Lindberg, B. E. (2001). Adaptation to winter stress in nine white clover populations: changes in non-structural carbohydrates during exposure to simulated winter conditions and spring regrowth potential. Ann. Bot. 88, 745–751. doi: 10.1006/anbo.2001.1432
Freeland, J. R., Biss, P., Conrad, K. F., and Silvertown, J. (2010). Selection pressures have caused genome-wide population differentiation of anthoxanthum odoratum despite potential for high gene flow. J. Evol. Biol. 23, 776–782. doi: 10.1111/j.1420-9101.2010.01947.x
Göransson, M., Kristjánsdóttir, T. A., Dalmannsdóttir, S., and Helgadóttir, Á (2012). Genetic shift in white clover (Trifolium repens) after natural selection in a marginal area. Icel. Agric. Sci. 25, 41–50.
Gould, B., McCough, S., and Geber, M. (2014). Variation in soil aluminium tolerance genes associated with local adaptation to soils at the parl grass experiment. Mol. Ecol. 23, 6058–6072. doi: 10.1111/mec.12893
Hedrick, P. W. (2011). Genetics of Populations, 4th Edn. Burlington, MA: Jones and Bartlett Publishers.
Helgadóttir,Á, Dalmannsdóttir, S., and Collins, R. P. (2001). Adaptational changes in white clover populations selected under marginal conditions. Ann. Bot. 88, 771–780. doi: 10.1006/anbo.2001.1438
Henry, R. J., and Nevo, E. (2014). Exploring natural selection to guide breeding for agriculture. Plant Biotech. J. 12, 655–662. doi: 10.1111/pbi.12215
Herrmann, D., Boller, B., Studer, B., Widmer, F., and Köllier, R. (2008). Improving persistence in red clover: insights form QTL analysis and comparative phenotypic evalutaion. Crop Sci. 48, 269–277.
Holderegger, R., Herrmann, D., Poncet, B., Gugerli, F., Thuiller, W., Taberlet, P., et al. (2008). Land ahead: using genome scans to identify molecular markers of adaptive relevance. Plant Ecol. Div. 1, 273–283. doi: 10.1080/17550870802338420
Ištvánek, J., Jaroš, M., Křenek, A., and Řepková, J. (2014). Genome assembly and annotation for red clover (Trifolium pratense; Fabaceae). Am. J. Bot. 101, 327–337. doi: 10.3732/ajb.1300340
Klimenko, I., Razgulajeva, N., Gau, M., Okomura, K., Nakaya, A., Tabata, S., et al. (2010). Mapping candidate QTLs related to plant persistency in red clover. Theor. Appl. Gen. 120, 1253–1263. doi: 10.1007/s00122-009-1253-5
Lager, J., and Gerhardson, B. (2002). Pathogenicity of clover root pathogens to pea, bean and lucerne. Zeitschrift für Pflanzenkrankenheiten unf Pflanzenschutz 109, 142–151.
Li, R., Li, Y., Fang, X., Yang, H., Wang, J., Kristiansen, K., et al. (2009a). SNP detection for massively parallel whole-genome resequencing. Genome Res. 19, 1124–1132. doi: 10.1101/gr.088013.108
Li, R., Yu, C., Li, Y., Lam, T.-W., Yiu, S.-M., Kristiansen, K., et al. (2009b). SOAP2: an improved ultrafast tool for short read alignment. Bioinformatics 25, 1966–1967. doi: 10.1093/bioinformatics/btp336
Marley, C. L., Fychan, R., Fraser, M. D., Winters, A., and Jones, R. (2003). Effect of sowing ratio and stage of maturity at harvest on yield, persistency and chemical composition of fresh and ensiled red clover/Lucerne bi-crops. Grass Forage Sci. 58, 397–406. doi: 10.1111/j.1365-2494.2003.00392.x
Montpetit, J. M., and Coulman, B. E. (1991a). Responses to divergent selection for adventitious root growth in red clover (Trifolium pratense L.). Euphytica 58, 119–127. doi: 10.1007/bf00022812
Montpetit, J. M., and Coulman, B. E. (1991b). Relationship between spring vigor and the presence of adventitious roots in established stands of red clover (Trifolium pratense L.). Can. J. Plant Sci. 71, 749–754. doi: 10.4141/cjps91-108
Pannell, J. R., and Fields, D. (2014). Evolution in subdivided plant populations: concepts, recent advances and future directions. New Phytol. 201, 417–432. doi: 10.1111/nph.12495
Sato, S., Isobe, S., Asamizu, E., Ohmido, N., Kataoka, R., Nakamura, Y., et al. (2005). Comprehensive structural analysis of the genome of red clover (Trifolium pratense, L.). DNA Res. 12, 301–364. doi: 10.1093/dnares/dsi018
Schoville, S. D., Bonin, A., Franc, O., Lobreaux, S., Melodelima, C., and Manel, S. (2012). Adaptive genetic variation on the landscape: methods and cases. Ann. Rev. Ecol. Evol. Syst. 43, 23–43. doi: 10.1146/annurev-ecolsys-110411-160248
Taylor, N. L. (1982). Stability of S alleles in a doublecross hybrid of red clover. Crop Sci. 22, 1222–1225.
Taylor, N. L. (2008). A century of clover breeding developments in the United States. Crop Sci. 48, 1–13.
Therrien, H. P., and Smith, D. (1960). The association of flowering habit with winter survival in red and alsike clover during the seedling year of growth. Can. J. Plant Sci. 40, 335–344. doi: 10.4141/cjps60-045
Turner, T. L., Bourne, E. C., Von Wettberg, E. J., Hu, T. T., and Nuzhdin, S. V. (2010). Population resequencing reveals local adaptation of Arabidopsis lyrata to serpentine soils. Nat. Genet. 42, 260–263. doi: 10.1038/ng.515
Wiberg, R. A. W., Gaggiotti, O. E., Morrissey, M. B., and Ritchie, M. G. (2017). Identifying consistent allele frequency differences in studies of stratified populations. Methods Ecol. Evol. 8, 1899–1909. doi: 10.1111/2041-210X.12810
Keywords: establishment success, genetic shift, population genomics, SNP, GBS, FST, persistence, pool
Citation: Ergon Å, Skøt L, Sæther VE and Rognli OA (2019) Allele Frequency Changes Provide Evidence for Selection and Identification of Candidate Loci for Survival in Red Clover (Trifolium pratense L.). Front. Plant Sci. 10:718. doi: 10.3389/fpls.2019.00718
Received: 31 October 2018; Accepted: 15 May 2019;
Published: 11 June 2019.
Edited by:
Soren K. Rasmussen, University of Copenhagen, DenmarkReviewed by:
Denise Brigitte Herbert, University of Giessen, GermanyAna Butron, Spanish National Research Council (CSIC), Spain
Francesca Taranto, Research Centre for Industrial Crops (CREA), Italy
Copyright © 2019 Ergon, Skøt, Sæther and Rognli. This is an open-access article distributed under the terms of the Creative Commons Attribution License (CC BY). The use, distribution or reproduction in other forums is permitted, provided the original author(s) and the copyright owner(s) are credited and that the original publication in this journal is cited, in accordance with accepted academic practice. No use, distribution or reproduction is permitted which does not comply with these terms.
*Correspondence: Åshild Ergon, ashild.ergon@nmbu.no