- 1Department of Pomology, Estación Experimental de Aula Dei-CSIC, Zaragoza, Spain
- 2Centro de Estudios Avanzados en Fruticultura, Rengo, Chile
- 3Genome Center, University of California, Davis, Davis, CA, United States
The identification of genes involved in variation of peach fruit quality would assist breeders to create new cultivars with improved fruit quality. Peach is a genetic and genomic model within the Rosaceae. A large quantity of useful data suitable for fine mapping using Single Nucleotide Polymorphisms (SNPs) from the peach genome sequence was used in this study. A set of 94 individuals from a peach germplasm collection was phenotyped and genotyped, including local Spanish and modern cultivars maintained at the Experimental Station of Aula Dei, Spain. Phenotypic evaluation based on agronomical, pomological and fruit quality traits was performed at least 3 years. A set of 4,558 out of a total of 8,144 SNPs markers developed by the Illumina Infinium BeadArray (v1.0) technology platform, covering the peach genome, were analyzed for population structure analysis and genome-wide association studies (GWAS). Population structure analysis identified two subpopulations, with admixture within them. While one subpopulation contains only modern cultivars, the other one is formed by local Spanish and several modern cultivars from international breeding programs. To test the marker trait associations between markers and phenotypic traits, four models comprising both general linear model (GLM) and mixed linear model (MLM) were selected. The MLM approach using co-ancestry values from population structure and kinship estimates (K model) identified a maximum of 347 significant associations between markers and traits. The associations found appeared to map within the interval where many candidate genes involved in different pathways are predicted in the peach genome. These results represent a promising situation for GWAS in the identification of SNP variants associated to fruit quality traits, potentially applicable in peach breeding programs.
Introduction
Peach [Prunus persica (L.) Batsch] is a model plant inside the family Rosaceae mainly due to its relatively small genome (∼230 Mb; Verde et al., 2017) and a low diploidy level (2n = 2x = 16), as well as its self-compatible mating system (Arús et al., 2012) and a relatively short juvenile period (2–4 years). In addition, it is one of the best genetically characterized Prunus species, with well-known genes controlling important traits that display Mendelian inheritance patterns, such as fruit flesh color (Y), stone adhesion-flesh texture (F-M), fruit shape (S) or sub-acidity (D; Dirlewanger and Arús, 2004). These traits have been studied in commercial and traditional peach cultivars (Cevallos-Casals et al., 2006; Reig et al., 2013, 2015; Font i Forcada et al., 2014) as well as in peach breeding progenies (Cantín et al., 2010a,b). From the market standpoint, world peach production exceeds more than twenty million tons of fruit per year, being one of the most important fruit grown in the world. The main producer countries in the world are China, Italy, Spain, and United States1.
In the last decades, peach diversity has been drastically reduced by the use of modern cultivars that share a few common ancestors (Aranzana et al., 2010). Thus, peach has a narrower genetic base (Scorza et al., 1985) in comparison with other species such as grape (Barnaud et al., 2006) or maize (Remington et al., 2001), where several studies (Yan et al., 2009) suggest that single nucleotide polymorphisms (SNPs) estimate a much lower decay of linkage disequilibrium (LD) than microsatellite (SSRs). However, the greater frequency of SNPs over SSRs makes the former more useful when the polymorphism within specific genes is desired for targeted investigations. The number and marker type used for investigating population structure has a significant effect on the rate of significant associations (Matthies et al., 2012; Cappa et al., 2013).
As an alternative to analysis in controlled crosses, association mapping (AM) is the non-random association of alleles at distinct loci in a sample population. AM is now being largely applied, using SSRs, to many crops, such as maize (Krill et al., 2010), potato (Pajerowska-Mukhtar et al., 2009), wheat (Breseghello and Sorrells, 2006), but only few studies have been carried out in fruit tree crops, such as peach (Font i Forcada et al., 2013), apple (Cevik et al., 2010), or pear (Oraguzie et al., 2010). This approach relies on the strength of association between genetic markers and phenotype (Mackay and Powell, 2007) and delimited genomic regions, which is helpful in locating and selecting candidate genes controlling the studied trait. In crop plants, the potential of exploiting LD in population-based association mapping, with the objective of estimating the position of a gene conferring a specific trait or phenotype by using LD between alleles of genetically mapped markers, has become a focus of considerable interest. As spurious associations between phenotypes and marker loci may be caused by population structure (Mariette et al., 2010; Ganopoulos et al., 2011), the structure and extent of LD within a sample population must be known before selecting an appropriate association mapping strategy (Lander and Schork, 1994). The number of markers available, their format, and cost currently limits whole-genome association studies in crop plants. In peach, different studies have been carried out using SSRs markers in cultivars with different genetic origin indicating that LD in this crop is quite high (Aranzana et al., 2010; Cao et al., 2012; Font i Forcada et al., 2013). In recent years, it has become increasingly common to use SNPs markers to get saturated genetic maps. SNPs have started to be used to study the whole-genome scans for diversity analysis, germplasm management, genetic fingerprinting, parentage verification, candidate genes and gene mapping in the Rosaceae family (Wu et al., 2008; Verde et al., 2012; Guajardo et al., 2015; Fresnedo-Ramírez et al., 2016; Zeballos et al., 2016; Hernández Mora et al., 2017). Multiplex SNP genotyping enables cost effective marker-assisted selection strategies, whole genome fingerprinting and genome-wide association studies (GWAS). Molecular markers are now widely employed in plant breeding for the acceleration of plant selection gains through marker-assisted selection (MAS) on the basis of individual genes or at the whole genome level through the selection of entire chromosomal segments (Cao et al., 2016; Zeballos et al., 2016; Hernández Mora et al., 2017). The ideal marker system should be highly polymorphic and evenly distributed across the genome, as well as provide codominant, accurate and reproducible data which can be generated in a high-throughput and cost-effective manner. In association mapping, a dense set of SNP markers covering the entire genome is needed for finding a casual mutation or a SNP that it is in linkage disequilibrium (Flint-Garcia et al., 2005). Association mapping studies requires genotyping platforms capable of producing multi-locus genotypes in a large panel of individuals. Several high-throughput platforms have been developed that allow rapid and simultaneous genotyping of hundreds of thousands of SNPs. The Illumina’s Infinium BeadArray Technology is used for genetic analysis in several crop species, such as barley (Rostoks et al., 2006), soybean (Hyten et al., 2008), and maize (McMullen et al., 2009). Furthermore, high-throughput genotyping arrays using the GoldenGate®Assay (Illumina, Inc., San Diego, CA, United States) have previously been used for SNP genotyping in soybean (Hyten et al., 2008), wheat (Akhunov et al., 2009), and maize (Yan et al., 2010). The International Peach SNP Consortium (IPSC) has pursued a genome-scale SNP discovery in peach using next generation sequencing platforms to develop and characterize a high-throughput Illumina Infinium®SNP genotyping array platform. The IPSC peach 9 K SNP array v1.0 achieved an average spacing of 26.7 kb between SNPs and distributed over all eight peach chromosomes (Verde et al., 2012). The SNP array has been successfully used in other association mapping studies in peach (Fresnedo-Ramírez et al., 2016; Zeballos et al., 2016; Hernández Mora et al., 2017). The first aim of the present work was to identify genetic regions associated with the most important agronomical and pomological traits in a peach germplasm collection using a medium-size SNP panel covering the peach genome. In previous works, this collection was phenotyped and screened with 40 SSRs markers spanning the peach genome (Font i Forcada, 2012; Font i Forcada et al., 2013, 2014), and significant associations with pomological traits were assessed. Consequently, the second objective for this study was to compare both associations showed in the two different studies and using the same peach germplasm collection.
Materials and Methods
Plant Material, Fruit Sampling and Evaluation of Fruit Quality Traits
A germplasm collection of 94 peach and nectarine [P. persica (L.) Batsch] cultivars (43 native local Spanish cultivars and 51 modern cultivars mostly from United States, France, Italy, New Zealand, and South Africa) were used in this study, as described by Font i Forcada et al. (2013). Genotypes were grown under typical Mediterranean soil and climate conditions at the Experimental Station of Aula Dei (CSIC) located at Zaragoza (northern of Spain).
The evaluation of the traits was performed over a period of at least 3 years, based on 20 fruits randomly harvested from each cultivar and year at commercial maturity. The entire fruit sample protocol was described by Font i Forcada et al. (2013, 2014). The agronomical and fruit quality traits evaluated were blooming and harvest date (Julian’s day), yield (kg/tree), vigor (TCSA; trunk cross-sectional area; cm2), yield efficiency (kg/cm2), fruit weight (g), flesh firmness (N), soluble solids content (SSC; Brix), titratable acidity (TA; g malic acid/100 g FW), and ripening index (RI; SSC/TA). Also, biochemical traits as vitamin C (mg of ascorbic acid, AsA per 100 g of FW), anthocyanin content (mg of cyanidin-3-glucoside equivalents, C3GE, per kg of FW), total phenolics (mg of gallic acid, 3,4,5-trihydroxy-benzoic acid, equivalents GAE per 100 g FW), flavonoid content (mg of catechin equivalents per 100 g of FW), relative antioxidant capacity (1,1-diphenyl-2-picrylhydrazyl, DPPH, g of Trolox equivalents per g of FW), and sucrose, glucose, fructose, sorbitol, and total sugars (g/kg FW) were evaluated. All the procedures used in this study were previously described (Font i Forcada et al., 2013, 2014). Phytochemical analyses were performed using a spectrophotometer (Beckman Coulter DU 800) and the individual and total sugars were purified and analyzed using a HPLC (Waters 515, Milford, MA, United States) as described by Font i Forcada et al. (2014).
DNA Isolation and SNP Analysis
Young leaves were collected from each cultivar, frozen immediately in liquid nitrogen, and stored at -20°C. DNA was isolated using the DNeasy Plant Mini Kit (Qiagen, Dusseldorf, Germany) following the manufacturer’s instructions. DNA concentration and quality was checked using PicoGreen®dye and measured in a fluorospectrometer. Then, the 94 cultivars were genotyped using a panel of IPSC 9K peach SNP array v1.0, using the single-base extension assay (Steemers et al., 2006) and Illumina®Infinium®HD Assay ultra protocol (Illumina, San Diego, CA, United States). The platform was developed by the International Peach SNP Consortium (IPSC) (Verde et al., 2012), which included a set of 8,144 SNPs. The analysis was performed by the ‘Instituto de Investigación Sanitaria (INCLIVA)’ of Valencia (Spain).
The SNP array was developed for use on worldwide breeding germplasm and includes Sanger-based SNPs from genome sequence of ‘Lovell’ generously provided by the International Peach Genome Initiative2.They also include SNPs identified from Illumina 80 bp paired-end genome sequencing of 22 important founder peach accessions (‘Admiral Dewey,’ ‘Slappey,’ ‘Babcock,’ ‘Elberta,’ ‘Carmen,’ ‘Chinese Cling,’ ‘Mayflower,’ ‘Bolinha,’ ‘Yellow St. John,’ ‘J. H. Hale,’ ‘Rio Oso Gem,’ ‘Diamante,’ ‘Dixon,’ ‘Early Crawford,’ ‘Florida Prince,’ ‘Dr. Davis,’ ‘O’Henry,’ ‘Okinawa,’ ‘Nemaguard,’ ‘Lovell,’ ‘Georgia Belle,’ and ‘Oldmixon Free’) and the almond cultivar ‘Nonpareil’ (Verde et al., 2012).
Markers with missing data that are non-polymorphic, redundant, or deviated from the expected segregation proportion were excluded. The workflow for SNP detection, filtering and final choice employed for association analysis are described in Table 1. When markers had the same segregation pattern, only one marker was included to improve computational algorithm efficiency (Van Ooijen, 1992). For the segregation deviation test, a chi-square test was performed with p = 0.05 as the threshold.
Statistical Analysis
Population Structure Analysis
The program STRUCTURE v2.3.4 (Pritchard et al., 2000) was used for identification of population structure by clustering individuals into genetically distinguishable groups based on allele frequencies. Structure analysis was performed using 4,558 SNPs on the whole dataset. Analysis was carried out for a range of K values from 1 to 10, with 10 runs for each K. A burn-in of 5,000 and 50,000 MCMC replications were implemented for each run. The optimal number of K clusters was estimated using the ΔK parameter of Evanno et al. (2005) in Structure Harvester (Earl and vonHoldt, 2012). Genotypes were subdivided into different populations according to their maximum membership probability among the populations with a threshold of 0.80. Results were displayed graphically in a bar graph/chart.
Association Mapping Analysis
Association analysis between DNA marker and agronomical and fruit quality traits was conducted using the software TASSEL v3.0 (Yu and Buckler, 2006). Different models comprising General Linear Model (GLM, Q) and Mixed Linear Model (MLM, Q + K) were selected to calculate P-values and to examine association between quality traits and molecular markers. The following models were compared: (a) Naïve-model (GLM without any correction for population structure); (b) Q-model (GLM with Q-matrix as correction for population structure); (c) QK-model (MLM with Q-matrix and K-matrix as correction for population structure and kinship relationships); (d) K-model (MLM with K-matrix as correction for kinship relationships structure). A structured association approach could avoid spurious associations and the results were compared to determine the best model. The significance of marker-traits associations was declared at P < 0.05 and a standard correction was performed by applying Bonferroni procedure at P < 0.00001097 (Schulze and McMahon, 2002). Alleles with a frequency (MAF) lower than 5% were removed (Wilson et al., 2004). Among the different models, the best model was selected based on the smallest mean square difference (MSD) between the observed and the expected P-values, since the random marker P-values follow an uniform distribution (Yu et al., 2005). To detect significant markers, the phenotypic variation (r2) was calculated using a simple regression equation implemented in GLM procedure in TASSEL.
All the bioinformatics analysis were performed on local servers at the UC Davis Genome Center (Davis, CA, United States). Reads were mapped to the reference sequences from the peach genome (Peach v1.0).
Power of Detection of QTLs
Computer simulations were used to determine the power of detection of QTLs with the current population and marker set. For this analysis, a modified version of the phenosym R program (Maruki and Lynch, 2015) was used to enable simulations across a complete panel of heritability values and QTL sizes. The simulations were performed iteratively in combination of heritability values and QTL sizes from 0 to 0.9 in 0.1 increments (in total 100 simulations) with 1000 iterations per simulation to determine the power of detection. After identifying the detection power of the population additional simulations were performed with varying distance between the predicted QTL and the SNP to study LD values.
Results
Genotyping of Peach and Nectarine Cultivars Using SNP Array
The Tassel pipeline initially identified 8,144 SNP loci distributed across all major scaffolds of the peach genome. Data were filtered to remove SNPs with low read support. Data for individual SNPs were retained only if they possessed polymorphic markers (6,232 SNPs remained), and with the gene train score lower than 0.4 (5,180 SNPs remained). In addition, data were filtered after removing the markers with similar pattern and with minor allele frequency (lower than 5%). After discovery and amplification on the Infinium HD BeadChips Illumina, 4,558 high quality SNPs remained for the final analysis of genetic structure and association studies, as summarized in Table 1. The final number of SNPs was distributed as follow: 653 SNPs on the scaffold 1; 735 on the scaffold 2; 499 on the scaffold 3; 842 on the scaffold 4; 348 on the scaffold 5; 576 on the scaffold 6; 410 on the scaffold 7; and 495 on the scaffold 8.
Genetic Structure Among Peach and Nectarine Cultivars
The population genetic structure determination among 94 peach and nectarine cultivars using genotyping data of genome-wide SNPs markers classified the cultivars in two distinct subpopulations at a K value of 2 (Figure 1). The main group (subpopulation A) was formed by 67 cultivars, with 38 Spanish local cultivars and 29 modern cultivars. The second group (subpopulation B) was formed by 18 modern cultivars, with USA as the origin of most of them. Six out seven nectarines analyzed in this study were grouped in subpopulation B. By considering admixed cultivars (seven genotypes), most of them have a European origin (Spain and Italy) and there is not available information about their parentage. Cultivars with known parentage information, such as ‘Andora’ and ‘Carolyn,’ which come from the ‘Libbee’ x ‘Lovell’ cross, were grouped in population A, while ‘Baby Gold 5,’ ‘Baby Gold 8,’ ‘Baby Gold 9,’ ‘Mountain Gold’ and ‘Suncling’ (which share ‘PI35201’ as the maternal genotype), were grouped in a same subpopulation (subpopulation B).

Figure 1. Population structure at K = 2 for the 94 peach/nectarine cultivars analyzed in this study.
Population Structure Analysis
Association analysis was performed in the germplasm collection of 94 peach and nectarine cultivars. Marker-trait associations were obtained for agronomical, fruit quality and biochemical traits. The mean phenotypic data obtained during 3 years of evaluation (described in Font i Forcada et al., 2014) was used to test the association analysis with the 4,558 polymorphic SNPs markers.
We tested four models in TASSEL software to determine associations and to account for the influence of population structure by comparing their ability to reduce the inflation of false positive associations. The P-values were plotted in a cumulative fashion for each model and the distribution examined. According to Stich et al. (2008) the distribution of P-values ideally should follow an uniform distribution with less deviation from the expected P-values.
The association analysis using the GLM approach (being the naïve model) and Q-model, detected a large number of associations between the markers and phenotypes which after Bonferroni correction for multiple testing of accessions, reduced down the number of associations. The Q-model showed 66 associations for the evaluated traits. It appears that these models may not have accounted for the heterogeneity of the genetic background, which may have resulted in false positive associations.
Identification of SNPs Associated With Fruit Quality Traits
The K-model and QK-model showed good fit for the P-values (P < 0.00001298), while the other models were characterized by the excess of small P-values (abundance of spurious associations; Figure 2). These two later models showed high uniform distribution of P-values. Taking into account the performance of the different models, only results from the K-model will be presented and discussed here since this appeared to have controlled population structure and kinship relationships better. A total of 347 associations (Table 2, Figure 3, and Supplementary File 1) in different scaffolds were found with blooming date (scaffolds: 1, 3, and 4), harvest date (scaffolds: 1, 2, 3, 4, 5, 6, 7, and 8), ripening index (scaffold: 7), anthocyanins (scaffolds: 1, 2, and 4), flavonoids (scaffolds: 1 and 6), RAC (scaffolds: 1 and 3), sorbitol (scaffolds: 2, 4, 6, and 8), and total sugars (scaffolds: 4, 6, and 8). Only harvest date showed associations in all scaffolds. The maximum number of associations was found in scaffold 2 (164), followed by scaffold 1 (55) and scaffold 4 (42), and the minimum number of associations (5) were found on scaffold 3. Only eight out the seventeen quality traits used, showed associations with the SNPs based on the 9k SNP array (v1.0). Figure 3 represents the associations by trait and by scaffold. It is interesting to note that some associations were also observed in the same regions where several QTLs using SSR markers had previously been identified (Tables 2, 3). The percentage of phenotypic variation detected by the associations ranged between 21.62 and 87.52%. We have detected four SNPs in agreement with the position of the SSRs markers reported by Font i Forcada et al. (2013) (Table 3). These markers are: CPPCT028 SSR marker associated with harvest date and sorbitol linkage group (LG) 4 and near to SNP_IGA_450711 in the position 20,165.259 bp; UDP96-003 SSR marker associated with anthocyanin content (LG4) and near to SNP_IGA_395202 in the position 6,168.570 bp; the CPPCT030 associated with harvest date and sorbitol (LG6) and near to SNP_IGA_700469 in the position 28,045.174 bp; and the UDP96-001 SSR marker associated with harvest date and flavonoids (LG6) and near to SNP_IGA_630302 in the position 8,238.299 bp.3 Comparing both studies, with SSRs (Font i Forcada et al., 2013) and SNPs markers, significant associations were also found with the same traits when using 40 SSRs markers and covering all the peach genome. The previous work showed association between SSR markers and harvest date in LG4 and LG6, RI in LG2, LG5 and LG6, flavonoids in LG2 and LG4, anthocyanins in LG2, LG3, LG4, LG5, LG6, and LG8, RAC in LG4 and LG6, sorbitol in LG4 and LG5, and total sugars in LG2 and LG4. The position on the physical map for the UDP98-410, endoPG1 and BPPCT015 SSR markers, where several associations were found, are unknown. The four transcripts corresponding to the SNP_IGA_450711, SNP_IGA_395202, SNP_IGA_700469, and SNP_IGA_630302 were identified as ppa023762m (Prupe.4G257400), ppa002262m (Prupe.4G114800), ppa006828m (Prupe.6G350900), and ppa019744m (Prupe.6G114400), respectively. Among them, the common associations found in both studies were obtained with the UDP98-410, BPPCT015, endoPG1, CPPCT028, UDP96-003, CPPCT030, and UDP96-001 SSR markers associated with anthocyanin content, harvest date, total sugars, and sorbitol content.
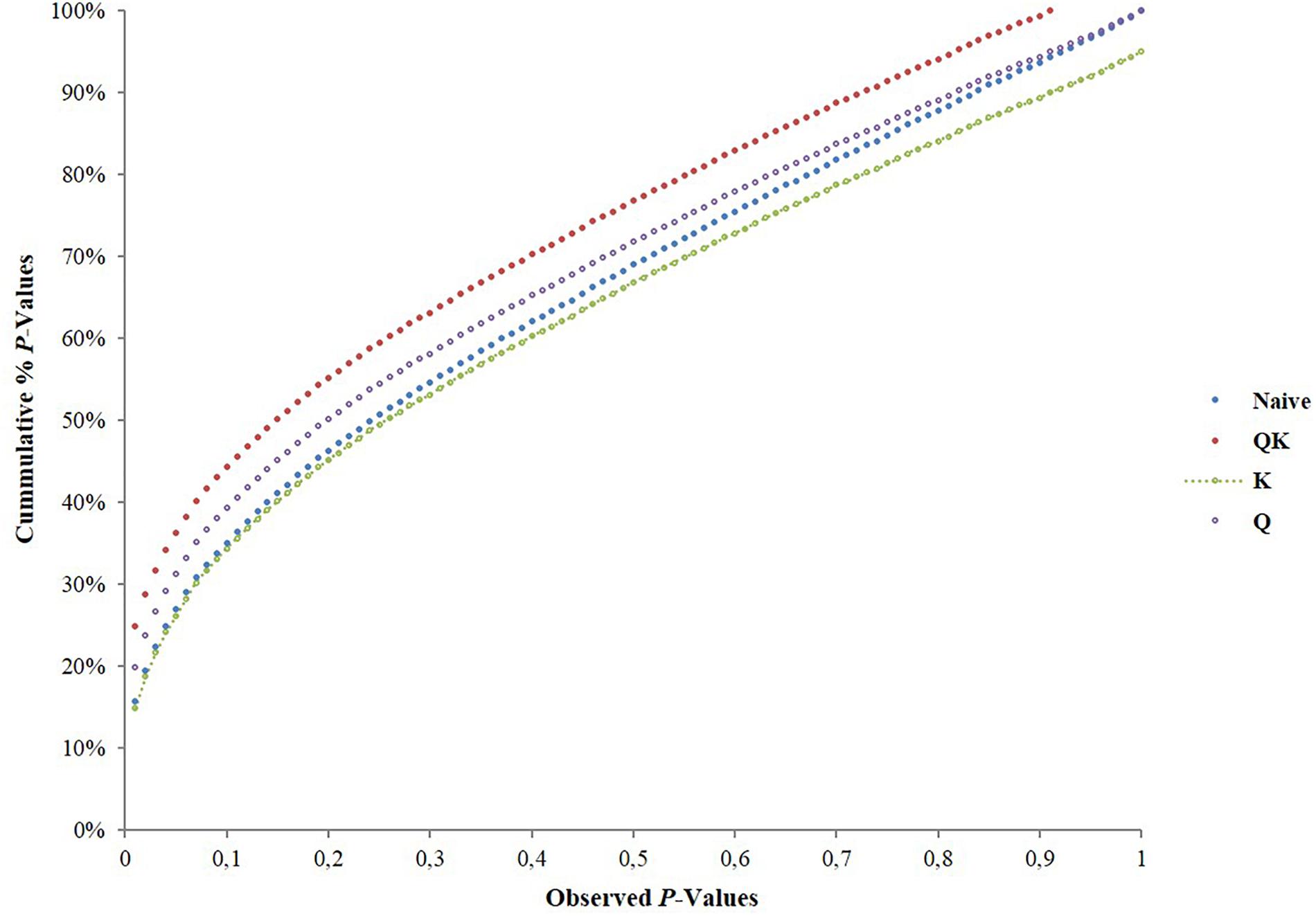
Figure 2. Comparison of four genomes wide association study model: naïve-model (GLM without any correction for population structure); Q-model (GLM with Q-matrix as correction for population structure); QK-model (MLM with Q-matrix and K-matrix as correction for population structure and kinship relationships); and K-model (MLM with K-matrix as correction for kinship relationships structure). Cumulative distribution of P-values was computed from the DNA markers and phenotypes for the different association models.
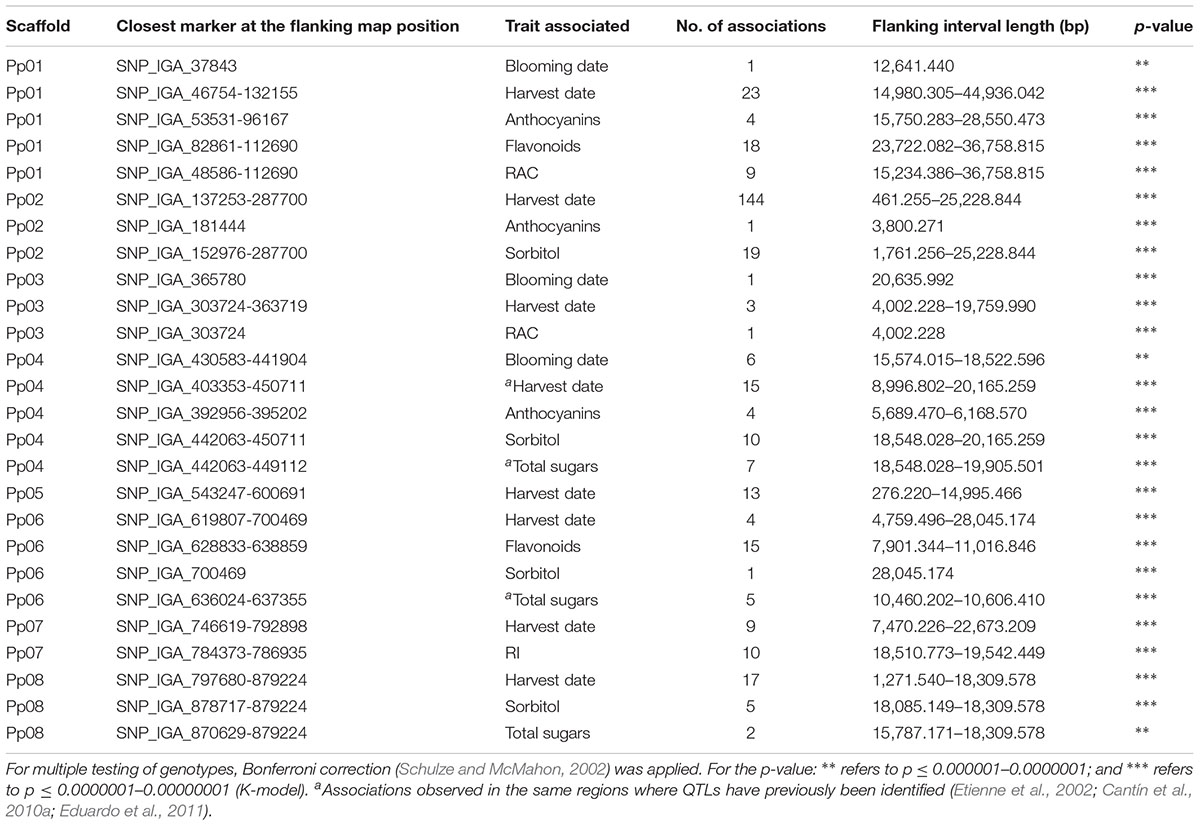
Table 2. Short list of the SNPs associated with different pomological traits, closest markers at the flanking map position, and their p-value.
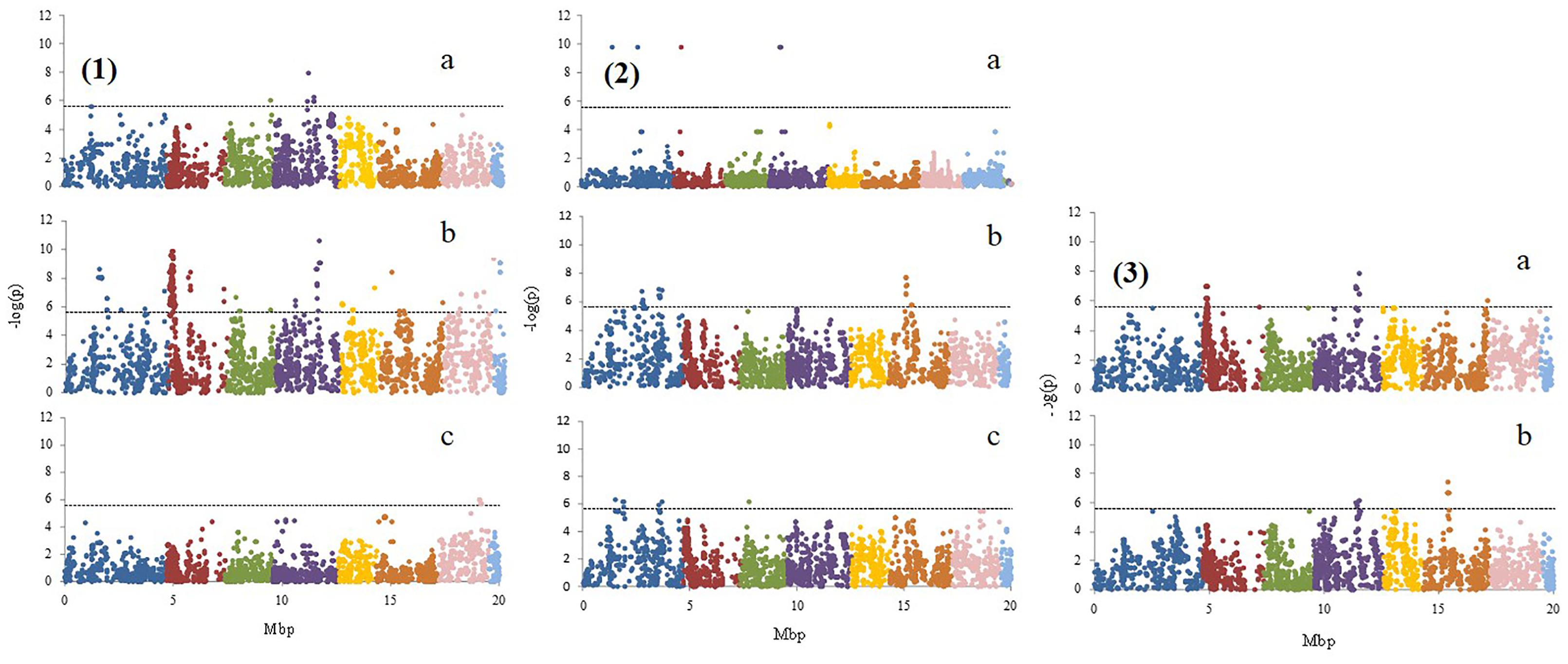
Figure 3. Genome scan showing –log (p) value for marker associations (K-model) with 1: (a) blooming date, (b) harvest date, and (c) ripening index; 2: (a) anthocyanins, (b) flavonoids, and (c) relative antioxidant capacity; 3: (a) sorbitol and (b) total sugars. The different colors represent the different linkage groups from 1 to 8.
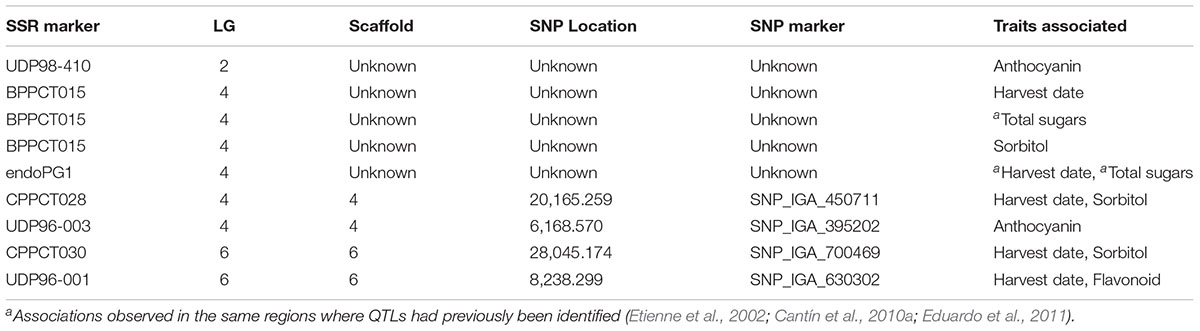
Table 3. List of SSR markers, linkage group (LG), scaffolds, SNP location, SNP marker, and traits associated comparing both studies, with SSRs (Font i Forcada et al., 2013) and SNP markers.
The power of detection of QTLs showed that, as expected, the effect of the QTL size is inversely proportional to the heritability. We can see, as shown in Figure 4 for high heritability values (over 0.5), the prediction power tends to be constant across the QTL sizes. For the lower heritability values, a linear correlation can be seen between QTL size and prediction power.
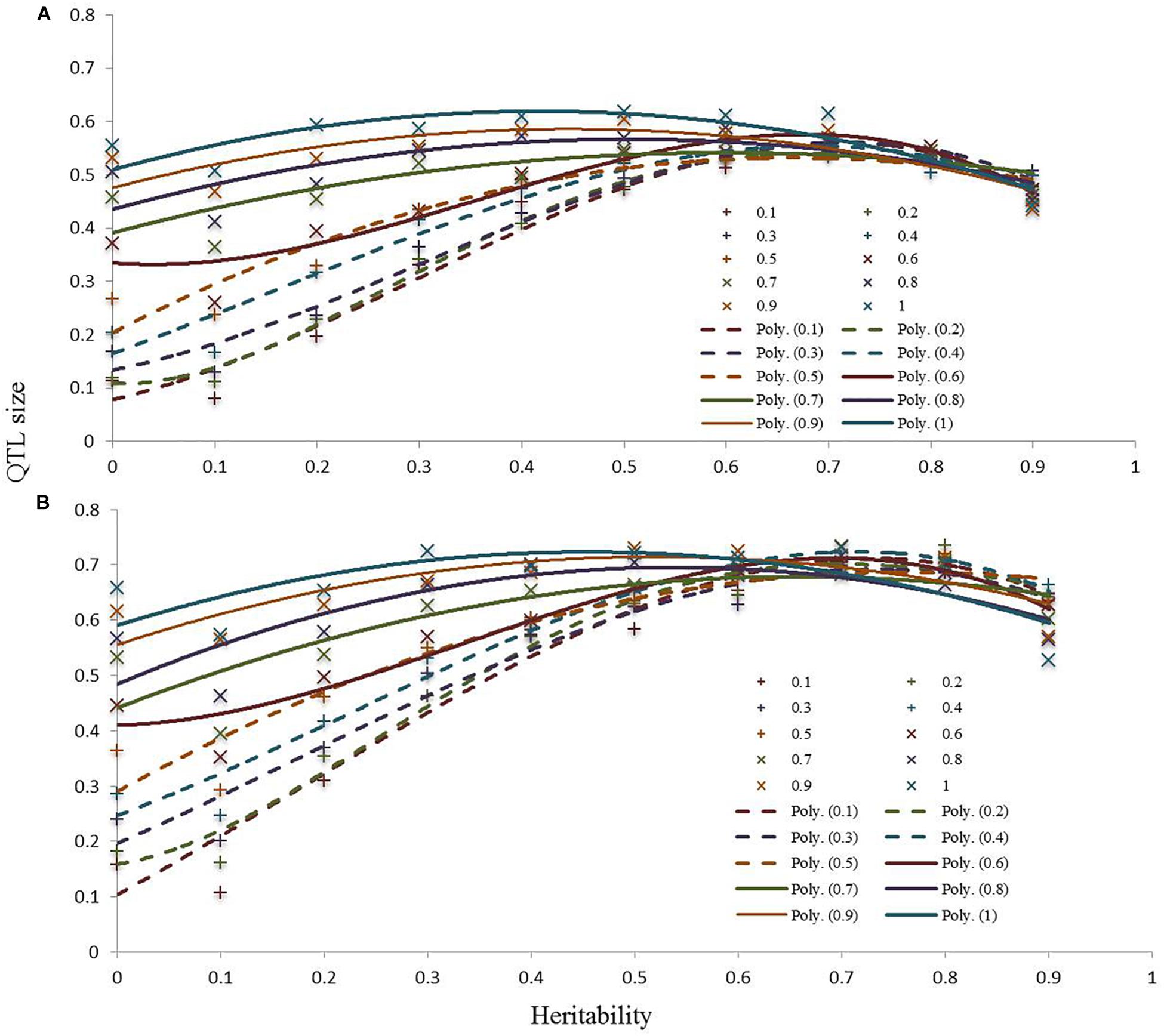
Figure 4. Computer simulations at 250 kb (A) and 500 kb (B) to determine the power of detection of QTLs.
Discussion
Population Structure
By using the STRUCTURE program to analyze the population structure of 94 peach and nectarine cultivars used in this study, the maximum ΔK-value was observed for K = 2. This result suggests that cultivars were grouped in two main subpopulations, plus a group of admixed cultivars. Peaches and nectarines were separated in two different subpopulations and cultivars with known parentage information were grouped with their parental genotypes. In a previous study (Font i Forcada et al., 2013) for the same group of cultivars, but using SSRs markers for association mapping in peach/nectarine cultivars, the maximum ΔK-value was observed for K = 3. This difference between both studies could be generated because of the different markers used in the previous work (SSRs) and those used in the present analysis (SNPs). Although SNPs are less polymorphic than SSR markers because of their biallelic nature, they easily compensate this drawback by being abundant, ubiquitous, and amenable to high- and ultra-high-throughput automation (Mammadov et al., 2012). In addition, the availability and stability of SNPs in comparison to SSRs provide better prospects for cultivar identification and assessment of genetic diversity (Fernández i Martí et al., 2012). In the present study, the main subpopulation grouped local Spanish and foreign modern cultivars, the latter from international breeding programs that are maintained at the Experimental Station of Aula Dei, Spain. It is known that after the dispersion of peach from China through Persia to Europe, a much more recent (16th-19th century) introduction of peach to the United States is represented by a few cultivars that have subsequently served as the genetic foundation of the modern breeding germplasm (Scorza et al., 1985). It is probable that several of the modern cultivars used in this study, without information about their parentage, have local European or Spanish cultivars as their parental genotypes and this could be the reason because an important group of local Spanish cultivars grouped together with modern cultivars, most of them from USA breeding programs.
Association Analysis
Association mapping is increasingly being utilized to detect marker-QTL linkage associations using plant material developed in breeding programs (Zeballos et al., 2016). Association mapping could be a more practical approach for cultivar development, considering that markers linked to major QTLs may immediately be utilized in marker assisted selection, once new QTLs are identified (Oraguzie et al., 2007).
High-density SNP phenotyping arrays are powerful tools for studying genomic patterns of diversity and marker-trait associations in mapping experiments (Muranty et al., 2014). The IPSC 9k SNP array v1.0 (Verde et al., 2012) consisted of 8,144 working SNPs, among which 80% were polymorphic across 94 cultivars of peach and 5% failed. In our study, 4,558 high quality SNPs remained for the final analysis of association studies (55.96%). These percentage of polymorphic SNPs is slightly lower comparing the study reported by Verde et al. (2012) for SNPs with MAF >0.1 (71.4%) and by Lambert et al. (2016) with MAF >0.1 (64.5%) suggesting that only 2/3 of the SNPs distributed in the 9K array v1.0 were finally useful.
Checking the literature, it exists more information in Prunus species on QTLs based on agronomical, morphological or basic fruit quality traits than QTLs based on biochemical traits. Important QTLs that control fruit quality traits have been found for organic acid content, fruit weight, sub-acidity fruit (D), and blooming and harvest dates (Etienne et al., 2002); blooming and ripening dates (Eduardo et al., 2011; Zeballos et al., 2016) and chilling injury susceptibility (Cantín et al., 2010a). However, the availability of SNP genotyping resources has assisted in fine mapping of peach (Zhebentyayeva et al., 2014). Several QTLs that control traits such as chilling and heat requirements (Romeu et al., 2014), maturity date or other pomological traits, such as fruit weight, soluble solid content, or pH (Eduardo et al., 2013; Fresnedo-Ramírez et al., 2015; Zeballos et al., 2016), have been mapped. Different studies for QTL identification in one apricot population (Salazar et al., 2016) identified major QTLs for ripening date (or harvest date) in LG4, showing that the nearest marker to major ripening date were the UDP96-003 marker. These results are in agreement with those found in our study. They showed the SNP_IGA_450711 associated with harvest date is in LG4 (Table 2 and Supplementary File 1). In addition, the marker closest to the SNP_IGA_395202 (Table 3) was the SSR marker UDP96-003 but associated with anthocyanin content instead of ripening date. However, more studies are needed in this area to facilitate QTL co-localization and/or synteny analysis among the Prunus species, with a view to undergoing candidate gene identification and fine mapping.
This genome-wide association study based on a panel of 94 peach cultivars and the ISPC 9K SNP array has identified a set of SNPs associated with different traits. Comparing this study with the previous one using SSRs (Font i Forcada et al., 2013), similar significant associations were found between the fruit traits and the different markers covering all the peach genome. The common associations found in both studies were obtained with the UDP98-410, BPPCT015, CPPCT028, UDP96-003, CPPCT030, endoPG1 and UDP96-001 SSR markers with harvest date, anthocyanin, sorbitol and total sugars. A different association mapping study with SSRs and 104 peach landraces from China showed the CPPCT005 marker in LG4 and the UDP98-407 marker in LG6 associated with blooming date (Cao et al., 2012), in good agreement with our study. Unfortunately, the positions of these markers on the physical map are unknown4. Another study with SSRs (Fan et al., 2010) also found QTLs for blooming date in LG1, but the positions of the markers on the physical map are also unknown. It is interesting to note that, we have found associations between SNPs markers and blooming date in scaffolds 1, 3, and 4. In contrast, the study with SSRs (Font i Forcada et al., 2013) did not show any association with blooming date. These results could be very useful because many of the associated markers (both, SSRs and SNPs) were located in common regions where major genes or QTLs for fruit quality traits have already been detected and have been previously identified and mapped on the Prunus reference map (Arús et al., 2012). Guan et al. (2015) reported that several QTLs for fructose, glucose, sucrose, and sorbitol were clustered. This interesting clustering of QTLs may be caused by the tight linkage among separate controlling genes or by the pleiotropic effect of a single gene. Eduardo et al. (2011) found a cluster containing QTLs for maturity date, fructose content, sucrose content, titrable acidity, and pH, also found in Dirlewanger et al. (1999) near to marker BPPCT15 on the G4. In the same region, Quilot et al. (2004) mapped a cluster of QTLs associated with maturity date and content of sorbitol, fructose, maltose, citric acid, and quinic acid. In our case, by analyzing individuals from a peach germplasm collection using association mapping, the significant associations found between the markers BPPCT015 and endoPG1 in LG4 with harvest date (highly correlated to maturity date), total sugars and sorbitol suggest a pleiotropic effect as previously reported by using bi-parental populations and QTLs identification. It will be interesting to decipher the biological processes underlying this strong pleiotropic effect in order to allow the identification of QTLs in future works. Pomological traits associated with genotypic traits seem consistent with previous studies where QTLs were mapped on LG1 for blooming date (Fan et al., 2010; Zeballos et al., 2016), and on LG4 with harvest date (Cantín et al., 2010a; Eduardo et al., 2011; Arús et al., 2012; Zeballos et al., 2016) and sugars content (Etienne et al., 2002; Arús et al., 2012; Zeballos et al., 2016). However, some associations were not consistent with the results of other published linkage analyses. These discrepancies in marker-locus-trait associations between the different studies could be attributed to number of marker loci used. Although the small sample size can cause a loss of power or provide associations that are not of general applicability (Hackshaw, 2008), they are valid for the set of genotypes used in the present study. In fact, this research includes many peach cultivars relevant to the peach industry in addition to material developed in different international breeding programs. Nevertheless, a larger confirmatory study should be carried out in the future.
Candidate Genes
Our aim was to relate these associations to structural genes using a candidate gene/QTL approach. We have detected two SNPs (SNP_IGA_449112 and SNP_IGA_450711) in the promoter of the candidate gene ppa025636m that was associated with total sugars content and sorbitol, respectively, in LG4 and in the interval comprised between 18,085 to 20,165.259 bp. Also, the SNP_IGA_450711 position, is in agreement with the position of CPPCT028 SSR marker found in the study of Font i Forcada et al. (2013). Lambert et al. (2016) reported that fruit stone adhesion and flesh texture (F-M) co-segregated in three mapping progenies in LG4 with an interval comprised between 18,593.828 and 20,176.048 bp, similar to our results, and also, the flanking map position included the SNP_IGA_449112 and the SNP_IGA_450711, according with our results.
We have founded three other SNPs associations in common with both studies, using SSRs (Font i Forcada et al., 2013) and SNPs (Table 3 and Supplementary File 2). Firstly, the UDP96-003 SSR marker was associated with anthocyanin content and very close to SNP_IGA_395202 (candidate gene ppb015985m). This gene encodes a cyclic GMP-activated non-selective cation channel in the plasma membrane of guard cells5. Second one, the lower region of the LG6 and close to marker CPPCT030 and one gene, ppa006828m, were identified very close to the position of SNP_IGA_700469, and associated with harvest date and sorbitol content. It may be responsible for the protein involved in peroxisome biogenesis5. This gene is expressed during the flowering stage, the mature plant embryo stage, and the petal differentiation and expansion stage. This is a fatty acid oxidation process that results in the complete oxidation of a long-chain fatty acid and a fatty acid beta-oxidation begins with the addition of coenzyme A to a fatty acid, and occurs by successive cycles of reactions during each of which the fatty acid is shortened by a two-carbon fragment removed as acetyl coenzyme A5. This candidate gene is also in agreement with that proposed on almonds trees (Font i Forcada et al., 2012, 2015), including Acyl-CoA, controlling the synthesis of long-chain saturated fatty acids. In the present work, it appears to be within the interval of the association found for the ppa006828m gene. Similar function was found with the gene ppa019942m (Supplementary File 2), associated with harvest date. The UDP96-001 marker was associated with harvest date and it is close to the SNP_IGA_630302 (candidate gene ppa024155m). This gene seems to be expressed during the L mature pollen stage, M germinated pollen stage and flowering stage5.
In our study, four transcription factors related to anthocyanin biosynthesis were found. In plants, the R gene product Lc, which is involved in the control of anthocyanin synthesis in maize, was the first plant protein reported to possess a bHLH motif (Ludwig et al., 1989). The bHLH is involved in the regulation of the anthocyanin pathway and it has been identified in peach (Ravaglia et al., 2013) and some other fruit species (Hichri et al., 2010; Xie et al., 2012). Here, we identified ppa022224m and ppa021918m (LG1), ppa019868m (LG2), and ppa019552m (LG7) genes through a phylogenetic tree of Arabidopsis and peach bHLHbZIP family members (Supplementary File 2). Among them, ppa022224m and ppa021918 genes were highly correlated with the flavonoid and RAC values. These traits are also highly correlated with the anthocyanin trait as described by Font i Forcada et al. (2013, 2014). Similar results were found in other peach studies (Zhao et al., 2017).
Finally, the candidate gene, ppa021329m, on chromosome 6 (Supplementary File 2), shows similarity to lycopene cyclase at3g10203 in the Arabidopsis thaliana genome and associated with harvest date and flavonoids content. Lycopene cyclases (LYCs) play a role in the biosynthesis of lutein, which is a member of the carotenoids pathway (Giuliano et al., 2008). This candidate gene may be associated with the yellow carotenoid pigmentation in peach and correlated with flavonoid content. Although the flesh coloration in peach is determined by the locus Y (LG1), major QTLs for skin color were also found (Eduardo et al., 2011) in different chromosomes (LG4, LG6 and LG7).
Datasets Are Available on Request
The raw data supporting the conclusions of this manuscript will be made available by the authors, without undue reservation, to any qualified researcher.
Conclusion
The present study, using the IPSC peach SNP array v1.0, allowed finding associations with agronomical and pomological traits in a wide peach germplasm collection. Due to the density of markers, this work confirm and reinforce results found with different markers type (SSRs and SNPs) to know if there is any impact on the results of GWA mapping in peach. Peach association mapping is an alternative to QTL mapping based on crosses between different cultivars because of the multiple advantage compared to bi-parental populations. Finding specific regions of the genome will provide further information searching for the genes involved in peach fruit quality, and to identify new candidate genes. Additionally, this work provide promising results concerning association mapping with pomological traits that could be applied in other Prunus species because of synteny inside the Rosaceae family, and it would be very useful to make predictions of genetic progress in breeding programs.
Author Contributions
CFiF and MM designed the study and the experiments. MM provided the material from the peach germplasm collection. CFiF, VG, and SC-W performed the statistical and biochemical analysis. CFiF, VG, and MM discussed the data, wrote and reviewed the manuscript. All authors have read and approved the final manuscript.
Funding
Financial support was provided by the Spanish grants AGL 2011-24576, AGL2014-52063R, RFP 2012-00020, and RFP 2015-00019, co-funded by FEDER and the Regional Government of Aragon (A44). CFiF acknowledges the JAE-Pre fellowship from the Consejo Superior de Investigaciones Científicas (CSIC) and the ‘Juan de la Cierva-Incorporación’ grant.
Conflict of Interest Statement
The authors declare that the research was conducted in the absence of any commercial or financial relationships that could be construed as a potential conflict of interest.
Acknowledgments
We thank E. Sierra and R. Giménez for the plant management in the field and technical assistance in the laboratory, respectively. We also acknowledge the assistance of Dra. Y. Gogorcena, and the bioinformatics assistance of Dr. E. Igartua and S. Solís. Part of the reported results in the present study was included in the dissertation Ph.D. thesis of CFiF (University of Lleida, Spain) and it is the only medium this content has appeared in and its publication is in line with the University of Lleida’s policies.
Supplementary Material
The Supplementary Material for this article can be found online at: https://www.frontiersin.org/articles/10.3389/fpls.2018.02005/full#supplementary-material
Footnotes
- ^http://faostat.fao.org/
- ^www.rosaceae.org/peach/genome
- ^https://www.rosaceae.org
- ^www.rosaceae.org
- ^www.arabidopsis.org
References
Akhunov, E., Nicolet, C., and Dvorak, J. (2009). Single nucleotide polymorphism genotyping in polyploid wheat with the illumina golden gate assay. Theor. Appl. Genet. 119, 507–517. doi: 10.1007/s00122-009-1059-5
Aranzana, M. J., Abbassi, E. K., Howad, W., and Arús, P. (2010). Genetic variation, population structure and linkage disequilibrium in peach commercial varieties. BMC Genet. 11:69. doi: 10.1186/1471-2156-11-69
Arús, P., Verde, I., Sosinski, B., Zhebentyayeva, T., and Abbott, A. G. (2012). The peach genome. Tree Genet. Genomes 8, 531–547. doi: 10.1007/s11295-012-0493-8
Barnaud, A., Lacombe, T., and Doligez, A. (2006). Linkage disequilibrium in cultivated grapevine Vitis vinifera L. Theor. Appl. Genet. 112, 708–716. doi: 10.1007/s00122-005-0174-1
Breseghello, F., and Sorrells, M. E. (2006). Association mapping of kernel size and milling quality in wheat (Triticum aestivum L.) cultivars. Genetics 172, 1165–1177. doi: 10.1534/genetics.105.044586
Cantín, C. M., Crisosto, C. H., Ogundiwin, E. A., Gradziel, T., Torrents, J., Moreno, M. A., et al. (2010a). Chilling injury susceptibility in an intra-specific peach [Prunus persica (L.) Batsch] progeny. Postharvest Biol. Technol. 58, 79–87. doi: 10.1016/j.postharvbio.2010.06.002
Cantín, C. M., Gogorcena, Y., and Moreno, M. A. (2010b). Phenotypic diversity and relation-ship of fruit quality traits in peach and nectarine [Prunus persica (L.) Batsch] breeding progenies. Euphytica 171, 211–226. doi: 10.1007/s10681-009-0023-4
Cao, K., Wang, L., Zhu, G., Fang, W., Chen, C., and Luo, J. (2012). Genetic diversity, linkage disequilibrium, and association mapping analyses of peach (Prunus persica) landraces in China. Tree Genet. Genomes 8, 975–990. doi: 10.1007/s11295-012-0477-8
Cao, K., Zhou, Z., Wang, Q., Guo, J., Zhao, P., Zhu, G., et al. (2016). Genome-wide association study of 12 agronomic traits in peach. Nat. Commun. 7:13246. doi: 10.1038/ncomms13246
Cappa, E. P., El-Kassaby, Y. A., Garcia, M. N., Acuña, C., Borralho, N. M. G., Grattapaglia, D., et al. (2013). Impacts of population structure and analytical models in genome-wide association studies of complex traits in forest trees: a case study in Eucalyptus globulus. PLoS One 8:e81267. doi: 10.1371/journal.pone.0081267
Cevallos-Casals, B. A., Byrne, D., Okie, W. R., and Cisneros-Zevallos, L. (2006). Selecting new peach and plum genotypes rich in phenolic compounds and enhanced functional properties. Food Chem. 96, 273–280. doi: 10.1016/j.foodchem.2005.02.032
Cevik, V., Ryder, C. D., Popovich, A., Manning, K., King, G. J., and Seymour, G. B. (2010). A Fruitfull-like gene is associated with genetic variation for fruit flesh firmness in apple (Malus domestica Borkh.). Tree Genet. Genomes 6, 271–279. doi: 10.1007/s11295-009-0247-4
Dirlewanger, E., and Arús, P. (2004). “Markers in tree breeding: improvement of peach. Molecular marker systems in plant breeding and crop improvement’,” in Biotechnology in Agriculture and Forestry, Vol. 55, eds H. Lorz and G. Wenzel (Springer: Berlin), 279–302.
Dirlewanger, E., Moing, A., Rothan, C., Svanella, L., Pronier, V., Guye, A., et al. (1999). Mapping QTLs controlling fruit quality in peach. Theor. Appl. Genet. 98, 18–31. doi: 10.1007/s001220051035
Earl, D. A., and vonHoldt, B. M. (2012). STRUCTURE HARVESTER: a website and program for visualizing STRUCTURE output and implementing the evanno method. Conserv. Genet. Resour. 4, 359–361. doi: 10.1007/s12686-011-9548-7
Eduardo, I., Chietera, G., Pirona, R., Pacheco, I., Troggio, M., Banchi, E., et al. (2013). Genetic dissection of aroma volatile compounds from the essential oil of peach fruit: QTL analysis and identification of candidate genes using dense SNP maps. Tree Genet. Genomes 9, 189–204. doi: 10.1007/s11295-012-0546-z
Eduardo, I., Pacheco, I., Chietera, G., Bassi, D., Pozzi, C., Vecchietti, A., et al. (2011). QTL analysis of fruit quality traits in two peach intraspecific populations and importance of maturity date pleiotropic effect. Tree Genet. Genomes 7, 323–335. doi: 10.1007/s11295-010-0334-6
Etienne, C., Rothan, C., Moing, A., Plomion, C., Bodénès, C., Svanella-Dumas, L., et al. (2002). Candidate genes and QTLs for sugar and organic acid content in peach [Prunus persica (L.) Batsch]. Theor. Appl. Genet. 105, 145–159. doi: 10.1007/s00122-001-0841-9
Evanno, G., Regnaut, S., and Goudet, J. (2005). Detecting the number of clusters of individuals using the software structure: a simulation study. Mol. Ecol. 14, 2611–2620. doi: 10.1111/j.1365-294X.2005.02553.x
Fan, S., Bielenberg, D. G., Zhebentyayeva, T. N., Reighard, G. L., Okie, W. R., Holland, D., et al. (2010). Mapping quantitative trait loci associated with chilling requirement, heat requirement and bloom date in peach (Prunus persica). New Phytol. 185, 917–930. doi: 10.1111/j.1469-8137.2009.03119.x
Fernández i Martí, A., Athanson, B., Koepke, T., Font i Forcada, C., Dhingra, A., and Oraguzie, N. (2012). Genetic diversity and relatedness of sweet cherry (Prunus avium L.) cultivars based on single nucleotide polymorphic markers. Front. Plant Sci. 3:116. doi: 10.3389/fpls.2012.00116
Flint-Garcia, S. A., Thuillet, A. C., Yu, J. M., Pressoir, G., Romero, S. M., Mitchell, S. E., et al. (2005). Maize association population: a high-resolution platform for quantitative trait locus dissection. Plant J. 44, 1054–1064. doi: 10.1111/j.1365-313X.2005.02591.x
Font i Forcada, C. (2012). Evaluación Agronómica y Estudio de la Calidad del Fruto en Melocotonero [Prunus persica (L.) Batsch] Variabilidad y Genética de Asociación. Ph.D. thesis, University of Lleida, Lleida.
Font i Forcada, C., Fernández i Martí, A., and Socias i Company, R. (2012). Mapping quantitative trait loci for kernel composition in almond. BMC Genet 13:47. doi: 10.1186/1471-2156-13-47
Font i Forcada, C., Gradziel, T. M., Gogorcena, Y., and Moreno, M. A. (2014). Phenotypic diversity among local Spanish and foreign peach and nectarine [Prunus persica (L.) Batsch] accessions. Euphytica 197, 261–277. doi: 10.1007/s10681-014-1065-9
Font i Forcada, C., Oraguzie, N., Igartua, E., Moreno, M. A., and Gogorcena, Y. (2013). Population structure and marker-trait associations for pomological traits in peach and nectarine cultivars. Tree Genet. Genomes 9, 331–349. doi: 10.1007/s11295-012-0553-0
Font i Forcada, C., Oraguzie, N., Reyes-Chin-Wo, S., Espiau, M. T., Socias i Company, R., and Fernández i Martí, A. (2015). Identification of genetic loci associated with quality traits in almond via association mapping. PLoS One 10:e0127656. doi: 10.1371/journal.pone.0127656
Fresnedo-Ramírez, J., Bink, M. C. A. M., van derWeg, E., Famula, T. R., Crisosto, C. H., Frett, T. J., et al. (2015). QTL mapping of pomological traits in peach and related species breeding germplasm. Mol. Breed. 35:166. doi: 10.1007/s11032-015-0357-7
Fresnedo-Ramírez, J., Frett, T. J., Sandefur, P. J., Salgado-Rojas, A., Clark, J. R., Gasic, K., et al. (2016). QTL mapping and breeding value estimation through pedigree-based analysis of fruit size and weight in four diverse peach breeding programs. Tree Genet. Genomes 12:25. doi: 10.1007/s11295-016-0985-z
Ganopoulos, I. V., Kazantzis, K., Chatzicharisis, I., Karayiannis, I., and Tsaftaris, A. S. (2011). Genetic diversity, structure and fruit trait associations in Greek sweet cherry cultivars using microsatellite based (SSR/ISSR) and morpho-physiological markers. Euphytica 181, 237–251. doi: 10.1007/s10681-011-0416-z
Giuliano, G., Tavazza, R., Diretto, G., Beyer, P., and Taylor, M. A. (2008). Metabolic engineering of carotenoid biosynthesis in plants. Trends Biotech. 26, 139–145. doi: 10.1016/j.tibtech.2007.12.003
Guajardo, V., Solís, S., Sagredo, B., Gainza, F., Muñoz, C., Gasic, K., et al. (2015). Construction of high density sweet cherry (Prunus avium L.) linkage maps using microsatellite markers and SNPs detected by genotyping-by-sequencing (GBS). PLoS One 10:e0127750. doi: 10.1371/journal
Guan, Y., Peace, C., Rudell, D., Verma, S., and Evans, K. (2015). QTLs detected for individual sugars and soluble solids content in apple. Mol. Breed. 35:135. doi: 10.1007/s11032-015-0334-1
Hackshaw, A. (2008). Small studies: strengths and limitations. Eur. Respir. J. 32, 1141–1143. doi: 10.1183/09031936.00136408
Hernández Mora, J. R., Micheletti, D., Bink, M., Van de Weg, E., Cantín, C., Nazzicari, N., et al. (2017). Integrated QTL detection for key breeding traits in multiple peach progenies. BMC Genomics 18:404. doi: 10.1186/s12864-017-3783-6
Hichri, I., Heppel, S. C., Pillet, J., Léon, C., Czemmel, S., Delrot, S., et al. (2010). The basic helix-loop-helix transcription factor MYC1 is involved in the regulation of the flavonoid biosynthesis pathway in grapevine. Mol. Plant 3, 509–523. doi: 10.1093/mp/ssp118
Hyten, D. L., Song, Q., Choi, I. Y., Yoon, M. S., Specht, J. E., Matukumalli, L. K., et al. (2008). High-throughput genotyping with the GoldenGate assay in the complex genome of soybean. Theor. Appl. Genet. 116, 945–952. doi: 10.1007/s00122-008-0726-2
Krill, A. M., Kirst, M., Kochian, L. V., Buckler, E. S., and Hoekenga, O. A. (2010). Association and linkage analysis of aluminum tolerance genes in maize. PLoS One 5:e9958. doi: 10.1371/journal.pone.0009958
Lambert, P., Campoy, J. A., Pacheco, I., Mauroux, J. B., Da Silva, Linge, C., et al. (2016). Identifying SNP markers tightly associated with six major genes in peach [Prunus persica (L.) Batsch] using a high-density SNP array with an objective of marker-assisted selection (MAS). Tree Genet. Genomes 12:121. doi: 10.1007/s11295-016-1080-1
Lander, E. S., and Schork, N. J. (1994). The genetic dissection of complex traits. Science 265, 2037–2048. doi: 10.1126/science.8091226
Ludwig, S. R., Habera, L. F., Dellaporta, S. L., and Wessler, S. R. (1989). Lc, a member of the maize R gene family responsible for tissue specific anthocyanin production, encodes a protein similar to transcriptional activators and contains a myc-homology region. Proc. Natl. Acad. Sci. U.S.A. 86, 7092–7096. doi: 10.1073/pnas.86.18.7092
Mackay, I., and Powell, W. (2007). Methods for linkage disequilibrium mapping in crops. Trends Plant Sci. 12, 57–63. doi: 10.1016/j.tplants.2006.12.001
Mammadov, J., Aggarwal, R., Buyyarapu, R., and Kumpatla, S. (2012). SNP markers and their impact on plant Breeding. Int. J. Plant Genomics 2012:728398. doi: 10.1155/2012/728398
Mariette, S., Tavaud, M., Arunyawat, U., Capdeville, G., Millan, M., and Salin, F. (2010). Population structure and genetic bottleneck in sweet cherry estimated with SSRs and the gametophytic self-incompatibility locus. Genetics 11:77. doi: 10.1186/1471-2156-11-77
Maruki, T., and Lynch, M. (2015). Genotype-frequency estimation from high-throughput sequencing data. Genetics 201, 473–486. doi: 10.1534/genetics.115.179077
Matthies, I. E., Hintum, T. V., Weise, S., and Röder, M. S. (2012). Population structure revealed by different marker types (SSR or DArT) has an impact on the results of genome-wide association mapping in European barley cultivars. Mol. Breed. 30, 951–966. doi: 10.1007/s11032-011-9678-3
McMullen, M. M., Kresovich, S., Villeda, H. S., Bradbury, P., Li, H., Sun, Q., et al. (2009). Genetic properties of the maize nested association mapping population. Science 325, 737–740. doi: 10.1126/science.1174320
Muranty, H., Jorge, V., Bastien, C., Lepoittevin, C., Bouffier, L., and Sanchez, L. (2014). Potential for marker-assisted selection for forest tree breeding: lessons from 20 years of MAS in crops. Tree Genet. Genomes 10, 1491–1510. doi: 10.1007/s11295-014-0790-5
Oraguzie, N. C., Whitworth, C. J., Brewer, L., Hall, A., Volz, R. K., Bassett, H., et al. (2010). Relationships of PpACS1 and PpACS2 genotypes, internal ethylene concentration and fruit softening in European (Pyrus communis) and Japanese (Pyrus pyrifolia) pears during cold air storage. Plant Breed. 129, 219–226. doi: 10.1111/j.1439-0523.2009.01684.x
Oraguzie, N. C., Wilcox, P. L., Rikkerink, E. H. A., and De Silva, H. N. (2007). “‘Linkage disequilibrium’,” in Association Mapping in Plants, eds N. C. Oraguzie, E. H. A. Rikkerink, S. E. Gardiner, and H. N. De Silva (New York, NY: Springer), 11–39. doi: 10.1007/978-0-387-36011-9_2
Pajerowska-Mukhtar, K., Stich, B., Achenbach, U., Ballvora, A., Lubeck, J., Strahwald, J., et al. (2009). Single nucleotide polymorphisms in the allene oxide synthase 2 gene are associated with field resistance to late blight in populations of tetraploid potato cultivars. Genetics 181, 1115–1127. doi: 10.1534/genetics.108.094268
Pritchard, J. K., Stephens, M., Rosenberg, N. A., and Donnelly, P. (2000). Association mapping in structured populations. Am. J. Hum. Genet. 67, 170–181. doi: 10.1086/302959
Quilot, B., Wu, B. H., Kervella, J., Genard, M., Foulogne, M., and Moreau, K. (2004). QTL analysis of quality traits in an advanced backcross between Prunus persica cultivars and the wild relative species P. davidiana. Theor. Appl. Genet. 109, 884–897. doi: 10.1007/s00122-004-1703-z
Ravaglia, D., Espley, R., Henry-Kirk, R., Andreotti, C., Ziosi, V., Hellens, R. P., et al. (2013). Transcriptional regulation of flavonoid biosynthesis in nectarine (Prunus persica) by a set of R2R3 MYB transcription factors. BMC Plant Biol. 13:68. doi: 10.1186/1471-2229-13-68
Reig, G., Alegre, S., Gatius, F., and Iglesias, I. (2015). Adaptability of peach cultivars [Prunus persica (L.) Batsch] to the climatic conditions of the Ebro Valley, with special focus on fruit quality. Sci. Hortic. 190, 149–160. doi: 10.1016/j.scienta.2015.04.019
Reig, G., Iglesias, I., Gatius, F., and Alegre, S. (2013). Antioxidant capacity, quality, and anthocyanin and nutrient contents of several peach cultivars [Prunus persica (L.) Batsch] grown in Spain. J. Agric. Food Chem. 61, 6344–6357. doi: 10.1021/jf401183d
Remington, D. L., Thornsberry, J. M., Matsuoka, Y., Wilson, L. M., Whitt, S. R., Doebley, J., et al. (2001). Structure of linkage disequilibrium and phenotypic associations in the maize genome in the maize genome. Proc. Natl. Acad. Sci. U.S.A. 98, 11479–11484. doi: 10.1073/pnas.201394398
Romeu, J., Monforte, A. J., Sánchez, G., Granell, A., García-Brunton, J., Badenes, M., et al. (2014). Quantitative trait loci affecting reproductive phenology in peach. BMC Plant Biol. 14:52. doi: 10.1186/1471-2229-14-52
Rostoks, N., Ramsay, L., MacKenzie, K., Cardle, L., Bhat, P. R., Roose, M. L., et al. (2006). Recent history of artificial outcrossing facilitates whole-genome association mapping in elite inbred crop varieties. Proc. Natl. Acad. Sci. U.S.A. 103, 18656–18661. doi: 10.1073/pnas.0606133103
Salazar, J. A., Ruiz, D., Campoy, J. A., Tartarini, S., Dondini, L., and Martínez-Gómez, P. (2016). Inheritance of reproductive phenology traits and related QTL identification in apricot. Tree Genet. Genomes 12:71. doi: 10.1007/s11295-016-1027-6
Schulze, T. G., and McMahon, F. J. (2002). Genetic association mapping at the crossroads: which test and why? overview and practical guidelines. Am. J. Med. Genet. 114, 1–11. doi: 10.1002/ajmg.10042
Scorza, R., Mehlenbacher, S. A., and Lighner, G. W. (1985). Inbreeding and coancestry of freestone peach cultivars of the eastern United States and implications for peach germplasm improvement. J. Am. Soc. Hortic. Sci. 110, 547–552.
Steemers, F. J., Chang, W., Lee, G., Barker, D. L., Shen, R., and Gunderson, K. L. (2006). Whole-genome genotyping with the single-base extension assay. Nat. Methods 3, 31–33. doi: 10.1038/nmeth842
Stich, B., Mohring, J., Piepho, H. P., Heckenberger, M., Buckler, E. S., and Melchinger, A. E. (2008). Comparison of mixed model approaches for association mapping. Genetics 178, 1745–1754. doi: 10.1534/genetics.107.079707
Van Ooijen, J. W. (1992). Accuracy of mapping quantitative trait loci in autogamous species. Theor. Appl. Genet. 84, 803–811. doi: 10.1007/BF00227388
Verde, I., Bassil, N., Scalabrin, S., Gilmore, B., Lawley, C. T., Gasic, K., et al. (2012). Development and evaluation of a 9K SNP array for peach by internationally coordinated SNP detection and validation in breeding germplasm. PLoS One 7:e35668. doi: 10.1371/journal.pone.0035668
Verde, I., Jenkins, J., Dondini, L., Micali, S., Pagliarani, G., Vendramin, et al. (2017). The Peach v2.0 release: high-resolution linkage mapping and deep resequencing improve chromosome-scale assembly and contiguity. BMC Genomics 18:225. doi: 10.1186/s12864-017-3606-9
Wilson, L. M., Whitt, S. R., Ibañez, A. M., Rocheford, T. R., Goodman, M. M., and Buckler, E. S. I. V. (2004). Dissection of maize kernel composition and starch production by candidate gene association. Plant Cell 16, 2719–2733. doi: 10.1105/tpc.104.025700
Wu, S. H., Wirthensohn, M., Hunt, P., Gibson, J., and Sedgley, M. (2008). High resolution melting analysis of almond SNPs derived from ESTs. Theor. Appl. Genet. 118, 1–14. doi: 10.1007/s00122-008-0870-8
Xie, X. B., Li, S., Zhang, R. F., Zhao, J., Chen, Y. C., Zhao, Q., et al. (2012). The bHLH transcription factor MdbHLH3 promotes anthocyanin accumulation and fruit colouration in response to low temperature in apples. Plant Cell Environ. 35, 1884–1897. doi: 10.1111/j.1365-3040.2012.02523.x
Yan, J., Shah, T., Warburton, M. L., Buckle, E. S., McMullen, M. D., and Crouch, J. (2009). Genetic characterization and linkage disequilibrium estimation of a global maize collection using SNP markers. PLoS One 4:e8451. doi: 10.1371/journal.pone.0008451
Yan, J. B., Yang, X. H., Shah, T., Sanchez-Villeda, H., Li, J. S., Warburton, M., et al. (2010). High-throughput SNP genotyping with the GoldenGate assay in maize. Mol. Breed. 25, 441–451. doi: 10.1007/s11032-009-9343-2
Yu, J., and Buckler, E. S. (2006). Genetic association mapping and genome organization of maize. Curr. Opin. Biotechnol. 17, 155–160. doi: 10.1016/j.copbio.2006.02.003
Yu, J., Pressoir, G., Briggs, W. H., Bi, I. V., Yamasaki, M., Doebley, J. F., et al. (2005). A unified mixed-model method for association mapping that accounts for multiple levels of relatedness. Nat. Genet. 38, 203–208. doi: 10.1038/ng1702
Zeballos, J. L., Abidi, W., Giménez, R., Monforte, A. J., Moreno, M. A., and Gogorcena, Y. (2016). Mapping QTLs associated with fruit quality traits in peach [Prunus persica (L.) Batsch] using SNP maps. Tree Genet. Genomes 12:37. doi: 10.1007/s11295-016-0996-9
Zhao, Y., Dong, W., Wang, K., Zhang, B., Allan, A. C., Lin-Wang, K., et al. (2017). Differential sensitivity of fruit pigmentation to ultraviolet light between two peach cultivars. Front. Plant Sci. 8:1552. doi: 10.3389/fpls.2017.01552
Zhebentyayeva, T. N., Fan, S., Chandra, A., Bielenberg, D. G., Reighard, G. L., Okie, W. R., et al. (2014). Dissection of chilling requirement and bloom date QTLs in peach using a whole genome sequencing of sibling trees from an F2 mapping population. Tree Genet. Genomes 10, 35–51. doi: 10.1007/s11295-013-0660-6
Keywords: peach, germplasm, firmness, antioxidants, sugar content, single nucleotide polymorphism, candidate genes
Citation: Font i Forcada C, Guajardo V, Chin-Wo SR and Moreno MÁ (2019) Association Mapping Analysis for Fruit Quality Traits in Prunus persica Using SNP Markers. Front. Plant Sci. 9:2005. doi: 10.3389/fpls.2018.02005
Received: 15 December 2017; Accepted: 28 December 2018;
Published: 17 January 2019.
Edited by:
Fabio Marroni, Università degli Studi di Udine, ItalyReviewed by:
Igor Pacheco, Universidad de Chile, ChileYuepeng Han, Wuhan Botanical Garden (CAS), China
Copyright © 2019 Font i Forcada, Guajardo, Chin-Wo and Moreno. This is an open-access article distributed under the terms of the Creative Commons Attribution License (CC BY). The use, distribution or reproduction in other forums is permitted, provided the original author(s) and the copyright owner(s) are credited and that the original publication in this journal is cited, in accordance with accepted academic practice. No use, distribution or reproduction is permitted which does not comply with these terms.
*Correspondence: Carolina Font i Forcada, Y2Fyb2xmZm9udEBnbWFpbC5jb20=