- 1College of Plant Science and Technology, Huazhong Agricultural University, Wuhan, China
- 2Guiyang College of Traditional Chinese Medicine, Guiyang, China
- 3Biology Department, Saint Mary's University, Halifax, NS, Canada
- 4School of Veterinary and Life Sciences, Murdoch University, Murdoch, WA, Australia
- 5Hubei Collaborative Innovation Center for Grain Industry, Jingzhou, China
The agronomic traits, including morphological and yield component traits, are important in barley breeding programs. In order to reveal the genetic foundation of agronomic traits of interest, in this study 122 doubled haploid lines from a cross between cultivars “Huaai 11” (six-rowed and dwarf) and “Huadamai 6” (two-rowed) were genotyped by 9680 SNPs and phenotyped 14 agronomic traits in 3 years, and the two datasets were used to conduct multi-locus genome-wide association studies. As a result, 913 quantitative trait nucleotides (QTNs) were identified by five multi-locus GWAS methods to be associated with the above 14 traits and their best linear unbiased predictions. Among these QTNs and their adjacent genes, 39 QTNs (or QTN clusters) were repeatedly detected in various environments and methods, and 10 candidate genes were identified from gene annotation. Nineteen QTNs and two genes (sdw1/denso and Vrs1) were previously reported, and eight candidate genes need to be further validated. The Vrs1 gene, controlling the number of rows in the spike, was found to be associated with spikelet number of main spike, spikelet number per plant, grain number per plant, grain number per spike, and 1,000 grain weight in multiple environments and by multi-locus GWAS methods. Therefore, the above results evidenced the feasibility and reliability of genome-wide association studies in doubled haploid population, and the QTNs and their candidate genes detected in this study are useful for marker-assisted selection breeding, gene cloning, and functional identification in barley.
Introduction
Barley (Hordeum vulgare L, 2n = 2x = 14), one of the first domesticated grains in the Fertile Crescent (Zohary et al., 2012), has been used widely as animal feed, human health foods, and a source of beer. Its yield and quality are the most important breeding objectives in crop breeding programs.
Most agronomic traits, such as plant height- and yield-related traits, are controlled by quantitative trait loci (QTLs) in barley, so it is difficult to obtain their genetic foundation and molecular mechanism. Plant height and its component traits serve as major plant morphological traits affecting barley seed yield. An appropriate plant height is a prerequisite for obtaining the desired yield in barley-breeding programs. To date, more than 30 types of dwarfing or semi-dwarfing genes have been detected, while only a few have been successfully used in barley breeding program, such as uzu and sdw1/denso (Jia et al., 2009; Ren et al., 2010, 2013). Moreover, a large number of QTLs for plant height related traits were reported to be located on all the seven chromosomes (Sameri et al., 2006; Baghizadeh et al., 2007; Wang et al., 2010; Ren et al., 2014). Grain yield is the key trait for the breeder in barley breeding program, therefore, the yield related traits including spike number per plant (SP), grain number per plant (GP), grain weight per plant (GWP), and 1,000 grain weight (TGW), have gained more attentions in the genetic dissection of yield related traits. A huge number of QTLs for yield related traits were detected to be located across all the chromosomes (Li et al., 2005; Sameri et al., 2006; Baghizadeh et al., 2007; Wang et al., 2010, 2016a; Ren et al., 2013).
Traditionally, QTL mapping has been widely applied in the genetic dissection of quantitative traits in barley (Zhuang et al., 1997; Li et al., 2005, 2007; Peng et al., 2011). As the development of DNA sequencing technologies, it is relatively easy to obtain high-density SNP genotypes for association mapping (AM) population, which offers a huge convenience for genomic and genetic research in different species. Therefore, genome-wide association studies (GWAS) present a powerful tool to reconnect the complex quantitative traits with their genes. Due to the development of cheaper, faster and higher-throughput molecular markers, AM has been widely used for mapping QTLs and genes in many crops, such as maize, soybean, rice, barley and wheat (Huang et al., 2010; Yang et al., 2010; Pasam et al., 2012; Hu et al., 2015). In comparison with traditional QTL mapping, AM has three obvious advantages, including shorter construction time, much higher mapping resolution and a greater number of alleles (Zhang et al., 2005; Yu and Buckler, 2006). In barley, AM has been widely applied for complex traits including disease resistance (Massman et al., 2011), drought tolerance (Varshney et al., 2012; Wójcik-Jagła et al., 2018), salinity tolerance (Fan et al., 2016) and especially agronomic traits (Gawenda et al., 2015; Xu et al., 2018).
Beside for natural population, nowadays GWAS have been widely applied to the genetic analysis for complex traits in family-based populations, such as nested association mapping (NAM) and multi-parent advanced generation intercross (MAGIC) populations, and proved to be powerful tool for uncovering the basis of key agronomic traits in maize and barley (Tian et al., 2011; Cook et al., 2012; Maurer et al., 2015, 2016). Moreover, there are also successful cases that combine linkage analysis with GWAS in several bi-parental segregation populations, such as recombinant inbred line (RIL) population (Lu et al., 2010; Reif et al., 2010). For single segregating population, successful but fewer cases were performed using GWAS (Gao et al., 2015; Henning et al., 2016; Liu et al., 2018). Henning et al. (2016) conducted GWAS for downy mildew resistance in a segregating population of “Teamaker” × USDA 21422M in hop (Humulus lupulus L.), Gao et al. (2015) determined the location of TTKSK resistance in the 108 doubled haploid (DH) lines using a GWAS method implemented by R package rrBLUP (Endelman, 2011), and Liu et al. (2018) used two strategies (QTL mapping and GWAS) to reveal the genetic bases of fiber quality traits and yield components in 231 RILs. Therefore, it is feasible to use GWAS to dissect the genetic foundations of complex traits in single bi-parental segregating population. A combination of linkage and association methodologies should provide the more accurate and powerful approach for revealing the genetic bases of complex traits (Ott et al., 2011). Association mapping of drought tolerance-related traits was performed in barley to complement a traditional bi-parental QTL mapping study in Wójcik-Jagła et al. (2018).
The objectives of this study were to: (a) use GWAS to further dissect the genetic foundations for main agronomic traits in our previous studies of Ren et al. (2013, 2014) and Wang et al. (2016a), and compare the quantitative trait nucleotide (QTN) results with those in previous studies, (b) evaluate if GWAS is feasible and reliable in the genetic dissection of complex traits in DH population, and (c) mine the candidate genes in the regions of the QTNs. The outcome of this study will provide more precise and complete information for further gene cloning, and marker-assisted selection in barley breeding.
Materials and Methods
Plant Materials and Field Experiments
One hundred and twenty-two DH lines, derived from a cross between barley cultivar “Huaai 11” (six-rowed and dwarfing) and barley cultivar “Huadamai 6” (two-rowed), was used in this study. The details of the materials and field experiments were described in the previous studies of Ren et al. (2010, 2013, 2014) and Wang et al. (2016a).
Phenotyping Data
Fourteen agronomic traits for the above DH population were measured in 2008–2009, 2009–2010, and 2011–2012, and all the three datasets had been reported by Ren et al. (2013, 2014) and Wang et al. (2016a). These traits included plant height (PH), first internode length (IL1), second internode length (IL2), third internode length (IL3), fourth internode length (IL4), main spike length (MSL), spike number per plant (SP), spikelet number of main spike (SMS), spikelet number per plant (SLP), grain number per plant (GP), grain number per spike (GS), grain weight per plant (GWP), grain weight per spike (GWS), and 1,000 grain weight (TGW). All the details have been described in Ren et al. (2013, 2014).
The best linear unbiased predictions (BLUPs) for each trait of 3 years were calculated using the R package Lme4 (Bates et al., 2014) with the following model: y = lmer (Trait ~ (1|Genetype) + (1|Year)). The three single-year phenotypic values (Ren et al., 2013, 2014; Wang et al., 2016a) and their BLUP values were used for GWAS. The results of phenotype statistics of BLUP for each trait were summarized in Table S1.
Genotyping Data
All the above DH lines were genotyped by 10,367 polymorphic SNPs. After excluding low quality SNP markers, 9680 SNPs were used in this study. Base on the recent genome sequence release in barley (Ibsc, 2016; Beier et al., 2017; Mascher et al., 2017), all the SNP markers were aligned to the most reliable genome position (http://webblast.ipk-gatersleben.de/barley_ibsc/). The Vrs1 locus controlling row number of barley was integrated with SNP markers for GWAS. All the above information has been described in Ren et al. (2016).
GWAS
Q matrix was calculated by STRUCTURE software (Falush et al., 2003), and the optimal K was inferred in Figure S1. The kinship (K) matrix between the lines was calculated as previously described in Wang et al. (2016b). All the 9680 SNPs for the above 122 DH lines were used to conduct GWAS for the above 14 traits in 3 years and their BLUP values using five multi-locus GWAS methods, including mrMLM (Wang et al., 2016b), FASTmrMLM (Zhang and Tamba, 2018), FASTmrEMMA (Wen et al., 2018), pLARmEB (Zhang et al., 2017) and ISIS EM-BLASSO (Tamba et al., 2017), which were included in the R package mrMLM v3.1 (https://cran.r-project.org/web/packages/mrMLM/index.html). All parameters in GWAS were set at default values. The critical thresholds of significant association for the five methods were set as LOD = 3 (or P-value = 2 × 10−4; Wang et al., 2016b).
The significant QTNs, repeatedly detected in at least two environments or methods, were viewed as reliable. The associated regions on chromosomes, repeatedly located on same or similar traits in at least 2 years or methods, were viewed as reliable QTN clusters. These QTNs (or clusters) were named as “qtn (qtnc)” + trait name abbreviation + chromosome + detected QTL order on chromosome.
Phenotypes Difference Corresponding to QTNs
For each QTN, all the DH lines were firstly divided into two groups based on their QTN genotypes, then t-test was used to test the phenotypic difference between the two genotypes.
Identification of Candidate Genes
According to the recent genome sequence release of barley (Ibsc, 2016; Beier et al., 2017; Mascher et al., 2017) and the gene annotation information (http://plants.ensembl.org/Hordeum_vulgare/Info/Index and https://www.uniprot.org/uniprot), some genes around reliable QTNs (or clusters) were selected for each trait. By combining gene annotation information, protein domain function in database and previous reports, and expressional information (http://barlex.barleysequence.org), then, candidate genes for each trait were mined.
Results
GWAS for 14 Agronomic Traits
Using five multi-locus GWAS methods in the R package mrMLM v3.1, GWAS for 14 agronomic traits were performed. A total of 913 significant QTNs were found to be associated with the 14 agronomic traits in 3 years and their BLUP values (Table S2). The number of significant QTNs varied across various traits, ranging from 4 for 2012_IL4 to 37 for BLUP_PH (Figure 1; Table S2), the chromosomal distribution of all identified QTNs revealed that 2H had the maximum number of significant QTNs, which weren't evenly distributed on the genome, and five QTN hotspots on chromosomes 2H, 3H, 6H, and 7H were observed (Figures 1, 2).
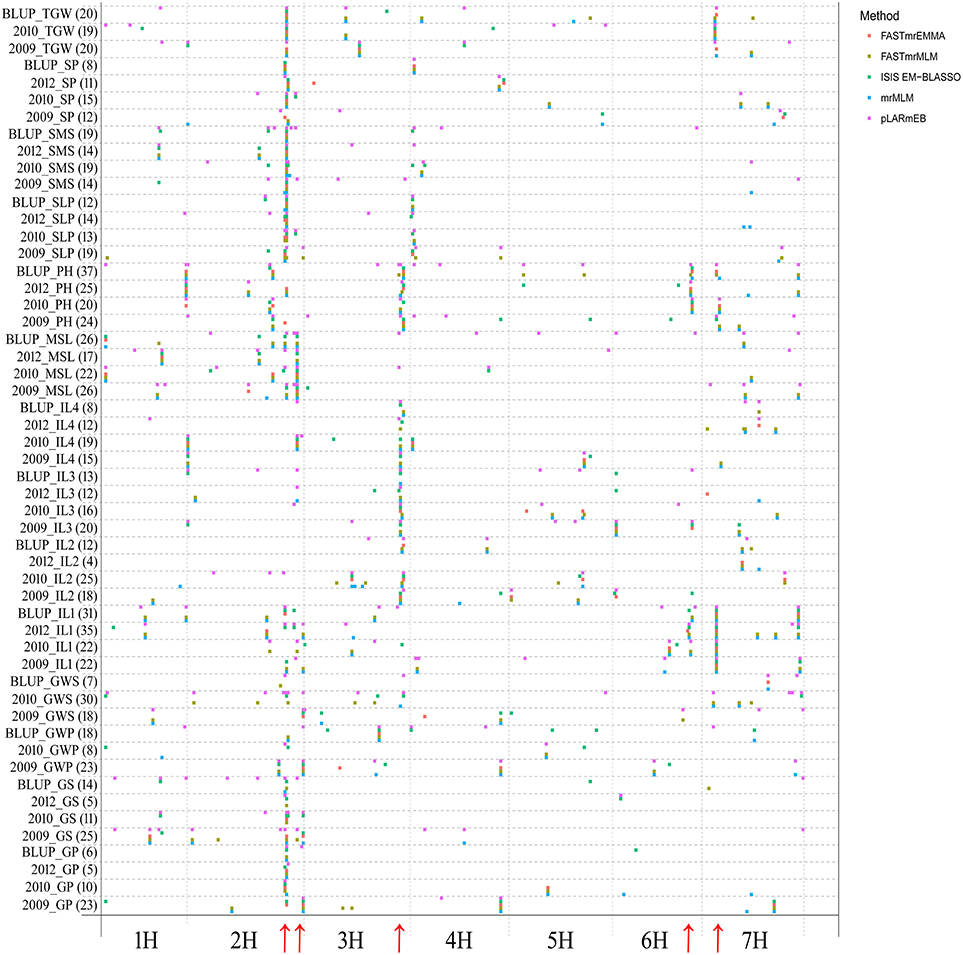
Figure 1. Chromosomal distribution of QTNs identified in this study. The x-axis indicates genomic locations by chromosomal order, and the significant QTNs are plotted against genome location. Each row represents one QTN identified by a different method. The red arrows show the QTN hotspots.
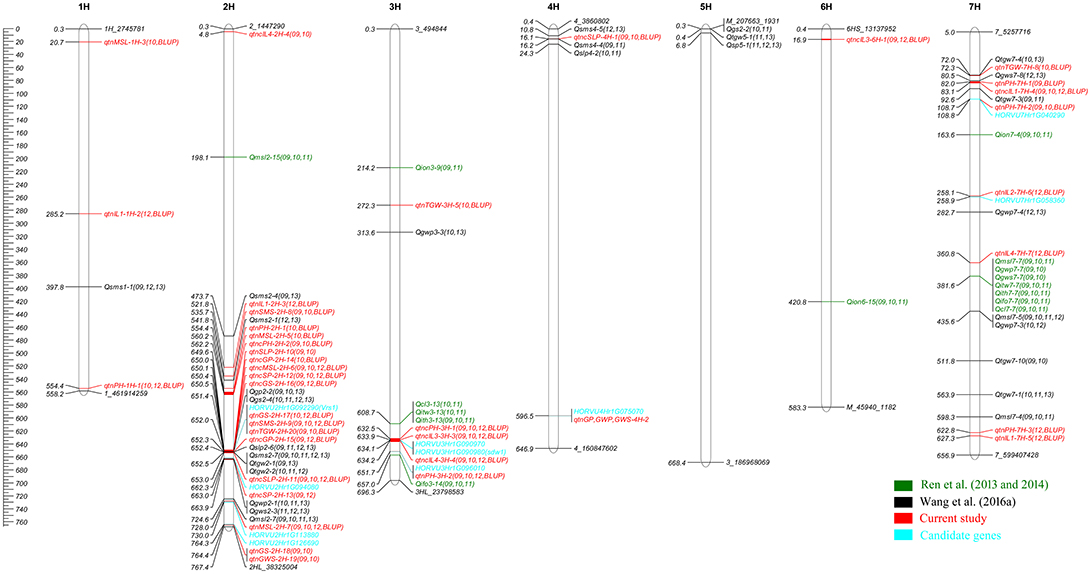
Figure 2. Chromosomes location of reliable QTLs for 14 agronomic traits in both previous studies (Ren et al., 2013, 2014; Wang et al., 2016a) and the current studies. The peak positions of previous QTLs were used for mapping, Genetic distance scale in physic position (Mb) is placed at left margin. Green is for the QTLs detected in Ren et al. (2013, 2014), black is for the QTLs Wang et al. (2016a), red is for the QTNs and QTNs clusters of current study, the region of QTNs clusters was marked with red on the bar, the cyan is for the candidate genes.
The significant QTNs repeatedly detected by multiple methods were list in Table S3. The reliable QTNs (or clusters) for 14 agronomic traits were summarized in Table 1. Totally, there were 39 reliable QTNs (or clusters) for 14 agronomic traits [8 for PH, 4 for IL1, 1 for IL2, 2 for IL3, 3 for IL4, 4 for MSL, 2 for SP, 2 for SMS, 3 for SLP, 3 for TGW, 2 for GP, 3 for GS, 1 for GWS, and 1 QTN cluster for (GP-GWP-GWS)].
The QTNs for Plant Height and Its Components Traits
Six reliable QTNs and two reliable QTN clusters, distributed on four chromosomes, were significantly associated with PH (Table 1; Figure 2). Six reliable QTNs for PH, qtnPH-1H-1 (1H: 554,371,992 bp), qtnPH-2H-1 (2H: 540,094,243 bp), qtnPH-3H-2 (3H: 651,696,476 bp), qtnPH-7H-1 (7H: 81,959,684 bp), qtnPH-7H-2 (7H:108,670,637 bp), and qtnPH-7H-3 (7H: 622,802,079 bp), located on chromosome 1H, 2H, 3H, 7H, 7H, and 7H, explained 0.63–4.07, 2.82–4.64, 3.13–9.03, 1.07–2.39, 2.31–6.07, and 0.69–2.68% of total phenotypic variation, respectively. Two reliable QTN clusters for PH, qtncPH-2H-2 (2H: 560,195,592–564,116,957 bp) and qtncPH-3H-1 (3H: 631,870,705–633,068,955 bp), were identified in at least 2 years and methods, explained 2.12–3.79 and 2.15–7.23% of total phenotypic variation, respectively (Tables 1, S3).
4, 1, 2, and 3 reliable QTNs (or clusters) were found to be associated with plant height component traits IL1, IL2, IL3, and IL4, respectively. For IL1, qtncIL1-7H-4, close to qtnPH-7H-1 (7H: 81,959,684 bp) for PH, was located on 7H (81,889,341–84,350,472 bp) accounting for a highest phenotypic variation (3.53–22.62%) among the four QTNs and QTN clusters (Table 1). For IL2, qtnIL2-7H-6 on chromosome 7H: 258,071,311 bp, explained phenotypic variation of 25.82–55.77%. For IL3, qtncIL3-3H-3 was located at 3H: 631,342,028–636,535,362 bp, close to the region of qtncPH-3H-1 (3H: 631,870,705–633,068,955 bp) for PH, accounting for 4.88–21.70% of the phenotypic variation. qtncIL3-6H-1 located on the chromosome 6H (16,165,407–17,542,081 bp), explained phenotypic variation of 1.89–3.68%. For IL4, qtncIL4-3H-4 (3H: 631,870,705–636,535,362 bp), co-located in the same region of qtncIL3-3H-3 for IL3 and qtncPH-3H-1 for PH mentioned above, explained 5.73–15.97% of phenotypic variation. Therefore, the region on chromosome 3H: 631,342,028–636,535,362 bp is a more credible QTN cluster for PH and the components traits, regulating the PH through controlling IL3 and IL4 (Table 1). Another QTN cluster for IL4, qtncIL4-2H-4 on 2H (4,629,895–4,950,022 bp) explained less (1.79–6.02%) phenotypic variation, while QTN qtnIL4-7H-7 (7H: 360793216 bp) showed a high explanation (6.10–19.57%) for IL4 (Tables 1, S3; Figure 2).
The QTNs for Spike and Yield Related Traits
Main spike length (MSL): Three reliable QTNs and one QTN cluster were detected for MSL. Among which, qtnMSL-2H-7 located on chromosome 2H: 727,985,438 bp, was repeatedly detected not only in three environments and BLUP values but also by multiple methods to be significantly associated with MSL, and explained phenotypic variation about 2.09–18.16% (LOD score: 3.29–22.92; Tables 1, S3; Figure 2).
Spikelet number of main spike (SMS): qtnSMS-2H-9 referred to Vrs1 (the morphological markers for row number of barley), located at 2H (position: 652,030,802 bp), was identified in all the situations and methods to be significantly associated with SMS (Tables 1, S3), accounting for the largest phenotypic variation (65.07–90.41%). As already known, Vrs1, a gene controlling row number of barley, was validated controlling row number in the DH population derived from a cross between the six-rowed barley cultivar “Huaai 11” and the two-rowed barley cultivar “Huadamai 6.” Thus, Vrs1 should control the spike related traits, such as SMS and SLP. Moreover, qtnSMS-2H-8 (2H: 535,680,815 bp) with minor effect was associated with SMS (Table 1).
Spikelet number per plant (SLP): One reliable QTN qtnSLP-2H-10 (2H: 649,558,019 bp) and one reliable QTN clusters qtncSLP-2H-11 (2H: 652,030,802–653,982,961 bp) close to Vrs1 (2H: 652,030,802 bp) were identified in multiple environments and by multiple methods to be significantly associated with SLP, explaining high proportions of total phenotypic variation, 14.87–55.05 and 8.97–80.30%, respectively. The reliable QTN cluster qtncSLP-4H-1 with a minor effect (2.82–4.14%), mapped on the region 15,498,372–16,168,735 bp of chromosome 4H, was detected in 2 years and the BLUP (Tables 1, S3; Figure 2).
Spike number per plant (SP): Two reliable QTN clusters for SLP were detected. qtncSP-2H-12, located on 2H: 648,821,931–652,030,802 bp overlapping with Vrs1, was detected in three environments and BLUP value and by multiple methods to be associated with SP, accounting for 7.61–52.32% of the phenotypic variation. qtncSP-2H-13, located on chromosome 2H (Position: 662,335,248–663,628,734 bp), was detected in two environments and by multiple methods to be associated with SP, explaining 6.49–11.95% of the phenotypic variation (Tables 1, S3; Figure 2).
Grain number per plant (GP) and grain number per spike (GS): The QTN cluster qtncGP-2H-14 with a high proportion of total phenotypic variation (19.56–37.44%), located at 2H: 649,558,019–650,438,830 bp, was detected in 2010 and BLUP value to be associated with GP. The qtncGP-2H-15 with a high explanation (3.41–34.59%), close to Vrs1 (2H: 652,030,802–652,604,015 bp), was detected in three environments and by multiple methods to be associated with GP (Table 1). One small-effect QTN on 4H (Position: 596,447,744 bp), was detected in 2009 to be significantly associated with GP. Meanwhile, this QTN was also detected for GWP and GWS (Table 1). One QTN cluster and two QTNs were detected for GS in at least two environments and by multiple methods. The qtncGS-2H-16 (2H: 649,558,019–651,399,477 bp), close to Vrs1, showed a high proportion of phenotypic variation (9.77–71.5%) for GS. The qtnGS-2H-17 referred to Vrs1 (2H: 652,030,802 bp) was significantly associated with GS, explaining a high percentage (49.99–72.25%) of the phenotypic variation. The reliable QTN qtnGS-2H-18, located at chromosome 2H (Position: 764,361,924 bp), was significantly associated with GS in 2009 and 2010 and multiple methods, accounting for 1.06–10.05% of the phenotypic variation (Tables 1, S3; Figure 2).
Grain weight per plant (GWP) and grain weight per spike (GWS): One QTN on 4H (Position: 596,447,744 bp) was found to be associated with GP, GWP and GWS with 3.97–9.17% proportions for the phenotypic variation. The QTN qtnGWS-2H-19, derived from the same associated SNP 2_625783669 (2H: 764,361,924 bp) as GS, was detected for GWS in 2009 and 2010 and by multiple GWAS methods with 0.74–6.25% proportion of phenotypic variation (Tables 1, S3; Figure 2).
1,000 grain weight (TGW): three reliable QTNs were detected for TGW. The qtnTGW-2H-20, located at Vrs1 (2H: 652,030,802 bp), was significantly associated with TGW in multiple environments and GWAS methods, explaining high percentage (32.77–56.66%) of the phenotypic variation. The qtnTGW-3H-5 (3H: 272,283,784 bp) and qtnTGW-7H-8 (7H: 72,344,563 bp) were significantly associated with TGW, explaining 2.19–4.39 and 4.39–11.22% of the phenotypic variation, respectively (Tables 1, S3; Figure 2).
Phenotypic Difference Corresponding to QTNs
According to the QTN genotypes, all the DH lines were divided into two different groups to test whether the significant difference of corresponding phenotypes of the QTN genotypes exist using t-test. Here, six reliable QTNs were used to underlying the phenotypes difference as an example. The details were showed in Figure 3.
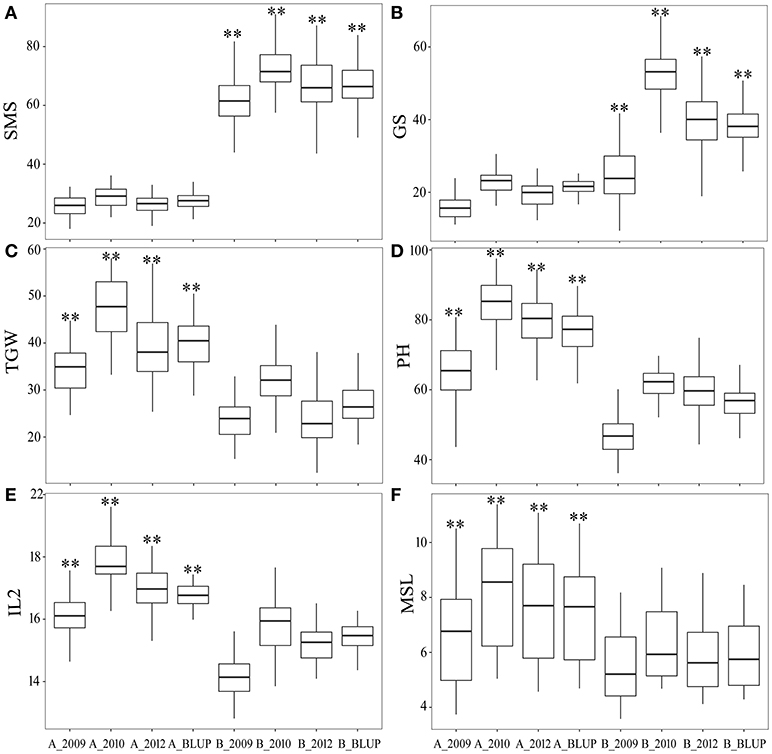
Figure 3. The difference of phenotypes between two kinds of genotypes for each of the six QTNs. (A): SMS at qtnSMS-2H-9, (B): GS at qtnGS-2H-17, (C): TGW at qtnTGW-2H-20, (D): PH at qtnPH-7H-2, (E): IL2 at qtnIL2-7H-6, (F): MSL at qtnMSL-2H-7. **Significant difference at P < 0.01.
Among six QTNs, three yield related QTNs (qtnSMS-2H-9 for SMS, qtnGS-2H-17 for GS, and qtnTGW-2H-20 for TGW) had the significant differences of phenotypic averages between their two genotypes in all four environments (Figures 3A–C), and three PH related QTNs (qtnPH-7H-2, qtnIL2-7H-6, and qtnMSL-2H-7) had the significant differences in all four environments (Figures 3D–F), indicating their reliability. It was worth noting that the qtnMSL-2H-7 and qtnSMS-2H-9 was detected in all the four environments, the qtnPH-7H-2, qtnTGW-2H-20, and qtnGS-2H-17 was only detected in three environments, and the qtnIL2-7H-6 was detected only in 2012 and the BLUP (Table 1).
Identification of Candidate Genes Around Reliable QTNs (or Clusters)
According to the recently released genome sequence of barley (Ibsc, 2016; Beier et al., 2017; Mascher et al., 2017) and the gene annotation information, ten candidate genes for the traits of interest were detected around the reliable QTNs and QTN clusters (Table 2). The chromosomal distribution of candidate genes was showed in Figure 2. Among which, two genes correspond to the previously reported genes, such as sdw1/denso and Vrs1, while eight candidate genes were new and their functions were derived from the annotated information, which need to be further validated (Table 2). Among eight new genes, HORVU3Hr1G090970, HORVU7Hr1G040290, HORVU7Hr1G058360, and HORVU3Hr1G096010 are related to plant height and its component traits, encoding SAM-dependent_Mtases, Alpha-mannosidase, DHHC-cysteine-rich domain S-acyltransferase and Homeobox-like, respectively. HORVU2Hr1G113880, HORVU2Hr1G094080, HORVU2Hr1G126690, and HORVU4Hr1G075070 are associated with spike and yield related traits, encoding AP2-like ethylene-responsive transcription factor, BTB/POZ domain protein, Acyl-CoA N-acetyltransferase and Patatin, respectively (Table 2).
Discussion
Previously Reported and Novel QTNs Detected With Multi-Locus GWAS Analysis
The comparison between the reliable QTNs (or clusters) for the main agronomic traits and the reliable QTLs in previous studies (Ren et al., 2010, 2013, 2014; Wang et al., 2016a) were conducted (Table 1; Figure 2). According to the physic positions of associated markers, the reliable QTNs (or clusters) in this study were integrated to the physic map with the reliable QTLs using MapChart 2.32 (Voorrips, 2002; Figure 2). Among 39 reliable QTNs (QTN clusters) detected by GWAS, 19 were located on the same regions of QTLs in previous studies (Sameri et al., 2009; Ren et al., 2014; Wang et al., 2016a), while 20 reliable QTNs (QTN clusters) including some minor effect QTNs were novel (Table 1). Totally, 8 of 18 QTNs (QTN clusters) associated with plant height related traits, were same as those in previous studies (Sameri et al., 2009; Ren et al., 2014), and 10 were new. For spike and yield related traits, 11 of 21 QTNs (QTN clusters) were the same as the QTLs in Wang et al. (2016a), the others were new in the current study (Table 1).
Among 8 previously reported QTNs (QTN clusters) for PH related traits, QTN clusters qtncPH-3H-1 (3H: 631,870,705–633,068,955 bp), qtncIL3-3H-3 (3H: 631,870,705–636,535,362 bp), and qtncIL4-3H-4 (3H: 631,870,705–636,535,362 bp), located on the hotspot of 3H (Figure 2), were significantly associated with PH, IL3, and IL4, respectively (Table 1). Close to the region of the QTNs clusters, Qcl3-13 for CL (the length from the ground to the collar equal PH minus MSL), Qitw3-13 for IL2, Qith3-13 for IL3, and Qifo3-14 for IL4 were detected close to the region of SSR markers Bmag13 (Position: 608,671,381 bp) and Bmag877 (Position: 657,045,459 bp) on 3H, respectively (Ren et al., 2014). Moreover, qIN6-3HL was detected to be significantly associated with IN6 (sixth internode) between Bmag13 (3H: 608,671,381 bp) and e06m30.8.3 in Sameri et al. (2009). Compared to the physical positions of the QTLs with those of QTNs, the QTN clusters for PH, IL3, and IL4 should correspond to the QTL Qcl3-13 for CL, Qith3-13 for IL3, and Qifo3-14 for IL4, respectively (Ren et al., 2014; Table 1).
The qtnPH-7H-1 (7H: 81,959,684 bp), qtnPH-7H-2 (7H: 108,670,637 bp), and qtncIL1-7H-4 (7H: 81,889,341–84,350,472 bp) were detected in same region of chromosome 7HS to be significantly associated with PH and IL1, respectively. These three QTNs are likely the same to the two QTLs qIN3-7Hs and qIN4-7Hs identified between markers HVCMA (7H: 75,227,158 bp) and ABG701 (7H: 90,406,550 bp) in Sameri et al. (2009) (Table 1). No consistent QTLs were detected in the region of these QTNs by Ren et al. (2014). It seems that GWAS can detect more minor QTNs for interesting traits than traditional QTL analysis.
Among 11 previously reported QTNs (or clusters) for spike and yield related traits, it is worth noting that most were located close to the region of Vrs1 gene. Four QTNs and three QTN clusters, such as qtnSMS-2H-9 (2H: 652,030,802 bp) for SMS, qtnGS-2H-17 (2H: 652,030,802 bp) for GS, qtnTGW-2H-20 (2H: 652,030,802 bp) for TGW, qtnSLP-2H-10 (2H: 649,558,019 bp) and qtncSLP-2H-11 (2H: 652,030,802–653,982,961 bp) for SLP, and qtncGP-2H-14 (2H: 649,558,019–650,438,830 bp) and qtncGP-2H-15 (2H: 652,030,802–652,604,015 bp) for GP were detected at Vrs1 (2H: 652,030,802 bp) and the nearby region of 2H with highly phenotypic variation (Table 1; Figure 2), which was consistent with the QTLs detected in Wang et al. (2016a), including Qsms2-7 (2H: 652,507,869 bp) for SMS, Qgs2-4 (2H: 651,436,685 bp) for GS, Qtgw2-1 (2H: 652,507,869 bp) and Qtgw2-2 (2H: 652,508,158 bp) for TGW, Qslp2-6 (2H: 651,436,685 bp) for SLP, and Qgp2-2 (2H: 651,436,685 bp) for GP (Table 1; Figure 2). Moreover, QTNs (or clusters) qtnMSL-2H-7 (2H: 727,985,438 bp), qtnSMS-2H-8 (2H: 535,680,815 bp), qtncSLP-4H-1 (4H: 15,498,372–16,761,959 bp), and qtnTGW-7H-8 (7H: 72,344,563 bp) were consistent with the QTLs Qmsl2-7 (2H: 724,577,184 bp), Qsms2-1 (2H: 541,758,123 bp), Qslp4-2 (4H: 24,332,575 bp), and Qtgw7-4 (7H: 71,957,427 bp) of Wang et al. (2016a) (Table 1; Figure 2).
For 20 novel reliable QTNs (QTN clusters) detected by multi-locus GWAS, most had minor effects (Table 1). It is worth noting that qtnIL2-7H-6 was a novel QTN associated with IL2 accounting for a higher proportion of phenotypic variation (22.85–55.77%). And the candidate gene HORVU7Hr1G058360 (7H: 258,860,422–258,866,854 bp), close to qtnIL2-7H-6 (7H: 258,071,311 bp), may involve in regulating IL2. For other minor effect QTNs (QTN clusters), three reliable candidate genes (HORVU2Hr1G094080, HORVU2Hr1G126690, and HORVU4Hr1G075070) were identified to be close to the region of qtncSP-2H-13, qtnGS-2H-18 (or qtnGWS-2H-19), and qtn(GP-GWP-GWS)-4H-2, respectively (Table 2). Therefore, the novel QTNs (QTN clusters) by multi-locus GWAS were reliable, even with minor effect. In other words, multi-locus GWAS can detect more minor effect QTNs than traditional QTL analysis.
The comparison between QTLs in previous studies (Sameri et al., 2009; Ren et al., 2013, 2014; Wang et al., 2016a) and in current study indicated that the consistent results should be much more reliable, which is valuable for further gene cloning and molecular marker assistant selection for breeding. Meanwhile, it illustrated that GWAS is feasible and reliable to detect significant associations for complex quantitative traits in DH population. Moreover, some new QTNs with minor effects were detected, suggesting that GWAS should be a good complementary to traditional QTL mapping. The combination of linkage and association analysis should provide the more accurate and powerful approach for reveling the genetic base of complex quantitative traits (Ott et al., 2011).
Two Previous Reported Genes Reveal the Reliability of Multi-Locus GWAS
HORVU3Hr1G090980 (3H: 634,077,598–634,081,600 bp) was identified on the region (3H: 631,870,705–636,535,362 bp) of three QTN clusters for plant height related traits (qtncPH-3H-1 for PH, qtncIL3-3H-3 for IL3, and qtncIL4-3H-4 for IL4; Table 2; Figure 2). This gene HORVU3Hr1G090980 correspondeds to the previously reported gene sdw1/denso, which was a semi-dwarf gene encoding a gibberellin 20-oxidase enzyme in barley (Jia et al., 2009; Xu et al., 2017). It was clarified that GA 20-oxidases encoded by sdw1/denso affected the plant height involving in the later steps of GA biosynthesis (Spielmeyer et al., 2004; Jia et al., 2009; Xu et al., 2017; Table 2). Therefore, the association between three QTN clusters and three height related traits (PH, IL3, and IL4) might be the effect of the gene sdw1/denso. Vrs1 (2H: 652,030,802 bp) was detected to be associated with SMS (qtnSMS-2H-9), GS (qtnGS-2H-17), and TGW (qtnTGW-2H-20) with high proportion of phenotypic variation in multiple environments and by multi-locus GWAS methods in current study (Tables 1, 2). Around the gene Vrs1, moreover, five QTNs (or clusters), including qtnSLP-2H-10 (2H: 649,558,019 bp) and qtncSLP-2H-11 (2H: 652,030,802–653,982,961 bp) for SLP, qtncGP-2H-14 (2H: 649,558,019–650,438,830 bp), and qtncGP-2H-15 (2H: 652,030,802–652,604,015 bp) for GP, and qtncSP-2H-12 (2H: 648,821,931–652,030,802 bp) for SP, were detected in multiple situations and by multi-locus GWAS methods with high proportion of phenotypic variation (Table 1; Figure 2). Vrs1, controlling row number of barley, encodes homeobox and profoundly affects barley spike morphology (Komatsuda et al., 2007). The identification of the reliable QTNs (or clusters) around two previously reported genes using multi-locus GWAS further revealed the reliability of multi-locus GWAS in bi-parental segregation population.
Novel Candidate Genes Reveal the Possible Molecular Basis of Plant Height- and Yield-Related Traits
Gene HORVU3Hr1G090970 (3H: 634,071,757–634,080,040 bp), encoded a S-adenosylmethionine (SAM)-dependent-MTases, which was identified on the region (3H: 631,870,705–636,535,362 bp) of three QTN clusters for plant height related traits (qtncPH-3H-1 for PH, qtncIL3-3H-3 for IL3, and qtncIL4-3H-4 for IL4; Table 2; Figure 2). It was reported that SAM biosynthetic pathways affects lignin biosynthesis in switchgrass (Panicum virgatum L.; Bai et al., 2018; Table 2). Cystathionine c-synthase (CGS) is the first committed enzyme for the biosynthesis of Met that can be metabolized to SAM, CGS-RNAi transgenic switchgrass lines showed much shorter plant height and internode length (Bai et al., 2018). Therefore, HORVU3Hr1G090970 might be a new semi-dwarf gene in barley.
HORVU7Hr1G008720, was identified at 7H:108,834,015–108,839,990 bp, which was 0.15Mb from the QTN qtnPH-7H-2 for PH, encoding Alpha-mannosidase like AMS1p (Table 2; Figure 2). Alpha-mannosidase is the component of cell wall, involving in cell wall biosynthesis or modification, which participated in the cell growth of internodes with pectinesterase and alpha-xylosidase in plant (Wu and Cao, 2008). Moreover, Alpha-mannosidase is the member of cytoplasm-to-vacuole targeting (Cvt) pathway with AuTophaGy8 (ATG8) gene, and soybean transgenic lines over-expressed GmATG8c showed higher plant height than the wild type (Xia et al., 2012). Therefore, HORVU7Hr1G008720 should be a reliable candidate gene, which affected PH by regulating the cell growth as AMS1p does.
HORVU7Hr1G058360 at 7H: 258,860,422-258,866,854 bp was close to qtnIL2-7H-6 (7H: 258,071,311 bp) for IL2, encoding a S-acyltransferase with DHHC-cysteine-rich domain (Table 2; Figure 2). DHHC-cysteine-rich domain S-acyltransferase proteins are involved in plant development and stress responses in Arabidopsis (Li et al., 2016). AtPAT10 is an S-acyl transferase, which affects the vascular development through controlling the cell division and expansion in Arabidopsis. AtPAT10 mutants are semi-dwarfed, and the reduction of plant height is due to the reduced length of the internodes, which appears to be the result of reduction in both cell number and cell size in these tissues (Qi et al., 2013). Therefore, HORVU7Hr1G058360 is a reliable candidate gene regulating the IL2 as AtPAT10 dose.
HORVU3Hr1G096010 (3H: 651,659,644–651,663,119 bp) encoding homeobox-like protein with SANT/MYB domain, was close to the QTN qtnPH-3H-2 (3H: 651,696,476 bp) for PH (Table 2; Figure 2). Homeobox gene was reported to be involved in the regulation of morphological development in plants, homeobox gene OSH15 affects the architecture of internodes resulting in d6 dwarf plants (Sato et al., 1999). Moreover, the RAD gene in Arabidopsis, encoding small plant-specific single SANT/MYB domain protein, affects the growth and development of Arabidopsis. Overexpression of the RAD gene can repress Arabidopsis growth, resulting in dwarfing and delaying flowering (Baxter et al., 2007; Zhang et al., 2011). Thus, HORVU3Hr1G096010 is a reliable candidate gene regulating plant height as the function of homeobox gene or RAD gene.
For main spike length (MSL), HORVU2Hr1G113880 corresponding to Cly1 gene, was identified at 2H:730,027,508–730,030,208 bp, which was 3 Mb from the QTN qtnMSL-2H-7 (2H: 727,985,438 bp) for MSL (Table 2; Figure 2). Cly1 encodes for an AP2-protein that inhibits development of flower (Nair et al., 2010; Terzi et al., 2017). HvAP2 regulates the length of a critical developmental window required for the elongation of the inflorescence internodes in barley (Houston et al., 2013). Therefore, the association between qtnMSL-2H-3 and MSL might be the effect of Cly1 gene.
For spike number per plant (SP), HORVU2Hr1G094080 (2H: 662,298,334–662,300,891 bp), encoding a protein with BTB/POZ domain (Broad complex, Tramtrack, Bric à brac, Pox virus and Zinc finger), was detected about 40 Kb from the QTN cluster qtncSP-2H-13 (2H: 662,335,248–663,628,734 bp; Table 2; Figure 2). HvCul4 gene encodes a BLADE-ON-PETIOLE-like (BOP-Like) protein containing BTB/POZ domain, which shares high similarity with Arabidopsis BOP1 and BOP2 (Tavakol et al., 2015; Jost et al., 2016). It was reported that HvCul4 controlled the tiller and leaf pattern in barley (Tavakol et al., 2015; Jost et al., 2016), and Arabidopsis BOP1 and BOP2 acted at boundary regions to regulate axillary development and leaf morphogenesis (Ha et al., 2004). In addition, according to the barley expression database from Barlex (http://barlex.barleysequence.org), HORVU2Hr1G094080.1 showed highest level of expression in developing inflorescences. Therefore, candidate gene HORVU2Hr1G094080 performed the similar function as HvCul4, BOP1, and BOP2 to control the spike number per plant (SP).
For grain number per spike (GS) and grain weight per spike (GWS), marker 2_625783669 (2H: 764,361,924 bp) was detected significantly associated with these 2 traits. HORVU2Hr1G126690 (2H: 764,279,329–764,290,102 bp), encoding a protein with N-acetyltransferase domain, was detected about 83 Kb from the marker 2_625783669 (Table 2; Figure 2). OsSNAT1 encodes N-acetyltransferase1, it was reported that overexpression of T2 homozygous OsSNAT1 in rice increased panicle number and seed weight per plant, while decreased spikelet numbers per panicle under paddy field conditions (Lee and Back, 2017). Moreover, the expression of HORVU2Hr1G126690.4 is much higher in developing inflorescences than in other tissues according to the barley expression database from Barlex (http://barlex.barleysequence.org). Thus, the candidate gene HORVU2Hr1G126690 may affect the GS and GWS through the similar function of OsSNAT1.
Marker 4_497278091 (4H: 596,447,744 bp) was significant associated with GP, GWP, and GWS. HORVU4Hr1G075070 (4H: 596,446,043–596,448,382 bp), encoding Patatin, was detected at 4_497278091 (Table 2). Overexpression of a patatin-like protein in Camelina sativa (Li et al., 2015) or in Arabidopsis (Li et al., 2013) reduced growth and overall seed production, but increased seed oil content. Therefore, HORVU4Hr1G075070 is a reliable candidate gene which might affect GP, GWP and GWS as the function of patatin-like gene.
Among the above ten candidate genes, two were previously reported, such as sdw1/denso and Vrs1, eight were new, which were derived from the annotated information. Based on the annotations of these candidate genes, homologous genes or proteins with same function or function domain were reported to be regulated the corresponding traits in barley, Arabidopsis and rice. The reliable QTNs and QTN clusters for these traits may be the effect of the candidate genes with similar function as the homologous genes or proteins does. The functions of eight reliable candidate genes need to be further validated. In summary, it is feasible and reliable to use multi-locus GWAS in bi-parental segregation populations.
The New Multi-Locus GWAS for Bi-Parental Segregation Population
Traditionally, segregation populations were used for QTL analysis, and GWAS are commonly used in natural populations. Nowadays, as the development of high-throughput SNP markers and high-throughput phenotypes, GWAS have been widely applied to the genetic analysis for complex traits in family-based populations (such as NAM and MAGIC populations) and proved to be powerful tool for uncovering the basis of key agronomic traits in maize and barley (Tian et al., 2011; Cook et al., 2012; Maurer et al., 2015, 2016). However, for single segregating population, successful but fewer cases were performed using GWAS (Gao et al., 2015; Henning et al., 2016; Liu et al., 2018). It indicated that GWAS for segregating population are feasible. However, high false positive rate is an obvious problem in the traditional single-locus GWAS using general linear models (GLMs) and mixed linear models (MLMs) (Zhang et al., 2010; Pace et al., 2015). And the P threshold (P = 0.05/n, n is the number of SNPs) leads to missing many significant QTNs, particularly small-effect QTNs (Wang et al., 2016b). Some multi-locus GWAS methodologies, such as mrMLM (Wang et al., 2016b), FASTmrMLM (Zhang and Tamba, 2018), FASTmrEMMA (Wen et al., 2018), ISIS EM-BLASSO (Tamba et al., 2017), pLARmEB (Zhang et al., 2017), and pKWmEB (Ren et al., 2018) have been developed to remedy the shortcomings mentioned above. These multi-locus GWAS methods have been used to analyze the published data, indicated that these methods constituted effective approaches with high detection power and less stringent criteria (Wang et al., 2016b; Tamba et al., 2017; Zhang et al., 2017; Wen et al., 2018). Totally, five multi-locus GWAS methods were used in our study, which improved the detection power and accuracy of QTNs for interesting traits. Moreover, the QTNs (QTN clusters), which were repeatedly detected in multiple environments and GWAS methods, were selected as reliable QTNs (QTN clusters). This greatly improved the accuracy of the association results and reduced its false positive, and more small-effect QTNs were detected within a certain rate of false positive. In addition, the t-test results of the phenotypic difference corresponding to QTNs, demonstrated that GWAS have the more stringent threshold of significance than t-test, and the advantages of accuracy and false positive controlling. In our study, 39 reliable QTNs and QTN clusters were detected, among which 10 reliable candidate gene were identified. Meanwhile, several new reliable QTNs with small-effect were also detected, which were different from the previous reports (Table 1). There was a limitation to identify candidate genes for all the reliable QTNs and QTN clusters, especially the small-effect ones, based on the imperfect annotation database of barley. However, these results indicated that multi-locus GWAS methods are feasible and reliable for DH population, and good complementary to traditional QTL mapping for the detection of new reliable QTNs even with small-effect. which will provide more useful information for future works.
Conclusions
Available online at: In this study, five multi-locus GWAS methods were performed for 14 main agronomic traits in 122 doubled haploid (DH) lines. Thirty-nine reliable QTNs and/or QTN clusters were repeatedly detected in multiple environments and methods 10 candidate genes for the interest traits were detected, 19 QTNs and two genes (sdw1/denso and Vrs1) were previously reported, and eight candidate genes need to be further validated. The results validated the feasibility and reliability of GWAS in DH population and the good complementary to traditional QTL analysis. All the results will facilitate elucidating genetic basis of agronomic traits and improving marker-assisted selection breeding in barley.
Author Contributions
DS and XR conceived and designed the experiments. XH, XR, JW, and LL conducted the experiments and phenotyping measurements. XH and JZ performed the analysis. XH and JZ wrote the paper. GS, XR, and DS modified the manuscript. CL produced the Huaai 11 and Huadamai 6 DH population. All the authors read and approved the final version of this manuscript.
Funding
This project was supported by the earmarked fund for China Agriculture Research System (CARS-5).
Conflict of Interest Statement
The authors declare that the research was conducted in the absence of any commercial or financial relationships that could be construed as a potential conflict of interest.
Supplementary Material
The Supplementary Material for this article can be found online at: https://www.frontiersin.org/articles/10.3389/fpls.2018.01683/full#supplementary-material
References
Baghizadeh, A., Taleei, A. R., and Naghavi, M. R. (2007). QTL analysis for some agronomic traits in barley (Hordeum vulgare L.). Int. J. Agric. Biol. 9, 372–374.
Bai, Z., Qi, T., Liu, Y., Wu, Z., Ma, L., Liu, W., et al. (2018). Alteration of S-adenosylhomocysteine levels affects lignin biosynthesis in switchgrass. Plant Biotechnol. J. 16, 2016–2026. doi: 10.1111/pbi.12935
Bates, D., Maechler, M., Bolker, B., and Walker, S. (2014). lme4: Linear mixed-effects models using Eigen and S4. R package version. J. Stat. Softw. 1, 1–23.
Baxter, C. E., Costa, M. M. R., and Coen, E. S. (2007). Diversification and co-option of RAD-like genes in the evolution of floral asymmetry. Plant J. 52, 105–113. doi: 10.1111/j.1365-313X.2007.03222.x
Beier, S., Himmelbach, A., Colmsee, C., Zhang, X. Q., Barrero, R. A., Zhang, Q., et al. (2017). Construction of a map-based reference genome sequence for barley, Hordeum vulgare L. Sci. Data 4, 170044. doi: 10.1038/sdata.2017.44
Cook, J. P., McMullen, M. D., Holland, J. B., Tian, F., Bradbury, P., Ross-Ibarra, J., et al. (2012). Genetic architecture of maize kernel composition in the nested association mapping and inbred association panels. Plant Physiol. 158, 824–834. doi: 10.1104/pp.111.185033
Endelman, J. B. (2011). Ridge regression and other kernels for genomic selection with R package rrBLUP. Plant Genome 4, 250–255. doi: 10.3835/plantgenome2011.08.0024
Falush, D., Stephens, M., and Pritchard, J. K. (2003). Inference of population structure using multilocus genotype data: linked loci and correlated allele frequencies. Genetics 164, 1567–1587. doi: 10.3410/f.1015548.197423
Fan, Y., Zhou, G., Shabala, S., Chen, Z.-H., Cai, S., Li, C., et al. (2016). Genome-wide association study reveals a new QTL for salinity tolerance in barley (Hordeum vulgare L.). Front. Plant Sci. 7:946. doi: 10.3389/fpls.2016.00946
Gao, L. L., Kielsmeier-Cook, J., Bajgain, P., Zhang, X. F., Chao, S. M., Rouse, M. N., et al. (2015). Development of genotyping by sequencing (GBS)- and array-derived SNP markers for stem rust resistance gene Sr42. Mol. Breeding 35, 207. doi: 10.1007/s11032-015-0404-4
Gawenda, I., Thorwarth, P., Günther, T., Ordon, F., and Schmid, K. J. (2015). Genome-wide association studies in elite varieties of German winter barley using single-marker and haplotype-based methods. Plant Breeding 134, 28–39. doi: 10.1111/pbr.12237
Ha, C. M., Jun, J. H., Nam, H. G., and Fletcher, J. C. (2004). BLADE-ON-PETIOLE1 encodes a BTB/POZ domain protein required for leaf morphogenesis in Arabidopsis thaliana. Plant Cell Physiol. 45, 1361–1370. doi: 10.1093/pcp/pch201
Henning, J. A., Gent, D. H., Twomey, M. C., Townsend, M. S., Pitra, N. J., and Matthews, P. D. (2016). Genotyping-by-sequencing of a bi-parental mapping population segregating for downy mildew resistance in hop (Humulus lupulus L.). Euphytica 208, 545–559. doi: 10.1007/s10681-015-1600-3
Houston, K., McKim, S. M., Comadran, J., Bonar, N., Druka, I., Uzrek, N., et al. (2013). Variation in the interaction between alleles of HvAPETALA2 and microRNA172 determines the density of grains on the barley inflorescence. Proc. Natl. Acad. Sci. U.S.A. 110, 16675–16680. doi: 10.1073/pnas.1311681110
Hu, X., Ren, J., Ren, X., Huang, S., Sabiel, S. A., Luo, M., et al. (2015). Association of agronomic traits with SNP markers in durum wheat (Triticum turgidum L. durum (Desf.)). PLoS ONE 10:e0130854. doi: 10.1371/journal.pone.0130854
Huang, X., Wei, X., Sang, T., Zhao, Q., Feng, Q., Zhao, Y., et al. (2010). Genome-wide association studies of 14 agronomic traits in rice landraces. Nat. Genet. 42, 961–967. doi: 10.1038/ng.695
Ibsc (2016). Pseudomolecules of the map-based reference genome assembly of barley cv. Morex. doi: 10.5447/ipk/2016/34
Jia, Q. J., Zhang, J. J., Westcott, S., Zhang, X. Q., Bellgard, M., Lance, R., et al. (2009). GA-20 oxidase as a candidate for the semidwarf gene sdw1/denso in barley. Funct. Integr. Genomic. 9, 255–262. doi: 10.1007/s10142-009-0120-4
Jost, M., Taketa, S., Mascher, M., Himmelbach, A., Yuo, T., Shahinnia, F., et al. (2016). A homolog of Blade-On-Petiole 1 and 2 (BOP1/2) controls internode length and homeotic changes of the barley inflorescence. Plant Physiol. 171, 1113–1127. doi: 10.1104/pp.16.00124
Komatsuda, T., Pourkheirandish, M., He, C., Azhaguvel, P., Kanamori, H., Perovic, D., et al. (2007). Six-rowed barley originated from a mutation in a homeodomain-leucine zipper I-class homeobox gene. Proc. Natl. Acad. Sci. U.S.A. 104, 1424–1429. doi: 10.1073/pnas.0608580104
Lee, K., and Back, K. (2017). Overexpression of rice serotonin N-acetyltransferase 1 in transgenic rice plants confers resistance to cadmium and senescence and increases grain yield. J. Pineal. Res. 62:e12392. doi: 10.1111/jpi.12392
Li, J., Huang, X., Heinrichs, F., Ganal, M., and Röder, M. (2005). Analysis of QTLs for yield, yield components, and malting quality in a BC3-DH population of spring barley. Theor. Appl. Genet. 110, 356–363. doi: 10.1007/s00122-004-1847-x
Li, M., Bahn, S. C., Fan, C., Li, J., Phan, T., Ortiz, M., et al. (2013). Patatin-related phospholipase pPLAIIIä increases seed oil content with long-chain fatty acids in Arabidopsis. Plant Physiol. 162, 39–51. doi: 10.1104/pp.113.216994
Li, M., Wei, F., Tawfall, A., Tang, M., Saettele, A., and Wang, X. (2015). Overexpression of patatin-related phospholipase AIIIδ altered plant growth and increased seed oil content in camelina. Plant Biotechnol. J. 13, 766–778. doi: 10.1111/pbi.12304
Li, S. S., Jia, J. Z., Wei, X. Y., Zhang, X. C., Li, L. Z., Chen, H. M., et al. (2007). A intervarietal genetic map and QTL analysis for yield traits in wheat. Mol. Breeding 20, 167–178. doi: 10.1007/s11032-007-9080-3
Li, Y. X., Lin, J. Z., Li, L., Peng, Y. C., Wang, W. W., Zhou, Y. B., et al. (2016). DHHC-cysteine-rich domain S-acyltransferase protein family in rice: organization, phylogenetic relationship and expression pattern during development and stress. Plant. Syst. Evol. 302, 1405–1417. doi: 10.1007/s00606-016-1339-x
Liu, R., Gong, J., Xiao, X., Zhang, Z., Li, J., Liu, A., et al. (2018). GWAS analysis and QTL identification of fiber quality traits and yield components in upland cotton using enriched high-density SNP markers. Front. Plant Sci. 9:1067. doi: 10.3389/fpls.2018.01067
Lu, Y., Zhang, S., Shah, T., Xie, C., Hao, Z., Li, X., et al. (2010). Joint linkage–linkage disequilibrium mapping is a powerful approach to detecting quantitative trait loci underlying drought tolerance in maize. Proc. Natl. Acad. Sci. U.S.A. 107, 19585–19590. doi: 10.1073/pnas.1006105107
Mascher, M., Gundlach, H., Himmelbach, A., Beier, S., Twardziok, S. O., Wicker, T., et al. (2017). A chromosome conformation capture ordered sequence of the barley genome. Nature 544, 427–433. doi: 10.1038/nature22043
Massman, J., Cooper, B., Horsley, R., Neate, S., Dill-Macky, R., Chao, S., et al. (2011). Genome-wide association mapping of Fusarium head blight resistance in contemporary barley breeding germplasm. Mol. Breeding 27, 439–454. doi: 10.1007/s11032-010-9442-0
Maurer, A., Draba, V., Jiang, Y., Schnaithmann, F., Sharma, R., Schumann, E., et al. (2015). Modelling the genetic architecture of flowering time control in barley through nested association mapping. BMC Genomics 16:290. doi: 10.1186/s12864-015-1459-7
Maurer, A., Draba, V., and Pillen, K. (2016). Genomic dissection of plant development and its impact on thousand grain weight in barley through nested association mapping. J. Exp. Bot. 67, 2507–2518. doi: 10.1093/jxb/erw070
Nair, S. K., Wang, N., Turuspekov, Y., Pourkheirandish, M., Sinsuwongwat, S., Chen, G., et al. (2010). Cleistogamous flowering in barley arises from the suppression of microRNA-guided HvAP2 mRNA cleavage. Proc. Natl. Acad. Sci. U.S.A. 107, 490–495. doi: 10.1073/pnas.0909097107
Ott, J., Kamatani, Y., and Lathrop, M. (2011). Family-based designs for genome-wide association studies. Nat. Rev. Genet. 12, 465–474. doi: 10.1038/nrg2989
Pace, J., Yu, X., and Lubberstedt, T. (2015). Genomic prediction of seedling root length in maize (Zea mays L.). Plant J. 83, 903–912. doi: 10.1111/tpj.12937
Pasam, R. K., Sharma, R., Malosetti, M., van Eeuwijk, F. A., Haseneyer, G., Kilian, B., et al. (2012). Genome-wide association studies for agronomical traits in a world wide spring barley collection. BMC Plant Biol. 12:16. doi: 10.1186/1471-2229-12-16
Peng, B., Li, Y., Wang, Y., Liu, C., Liu, Z., Tan, W., et al. (2011). QTL analysis for yield components and kernel-related traits in maize across multi-environments. Theor. Appl. Genet. 122, 1305–1320. doi: 10.1007/s00122-011-1532-9
Qi, B., Doughty, J., and Hooley, R. (2013). A Golgi and tonoplast localized S-acyl transferase is involved in cell expansion, cell division, vascular patterning and fertility in Arabidopsis. New Phytol. 200, 444–456. doi: 10.1111/nph.12385
Reif, J. C., Liu, W., Gowda, M., Maurer, H. P., Mohring, J., Fischer, S., et al. (2010). Genetic basis of agronomically important traits in sugar beet (Beta vulgaris L.) investigated with joint linkage association mapping. Theor. Appl. Genet. 121, 1489–1499. doi: 10.1007/s00122-010-1405-7
Ren, W. L., Wen, Y. J., Dunwell, J. M., and Zhang, Y. M. (2018). pKWmEB: integration of Kruskal-Wallis test with empirical Bayes under polygenic background control for multi-locus genome-wide association study. Heredity 120, 208–218. doi: 10.1038/s41437-017-0007-4
Ren, X., Sun, D., Guan, W., Sun, G., and Li, C. (2010). Inheritance and identification of molecular markers associated with a novel dwarfing gene in barley. BMC Genet. 11:89. doi: 10.1186/1471-2156-11-89
Ren, X., Wang, J., Liu, L., Sun, G., Li, C., Luo, H., et al. (2016). SNP-based high density genetic map and mapping of btwd1 dwarfing gene in barley. Sci. Rep. 6:31741. doi: 10.1038/srep31741
Ren, X. F., Sun, D. F., Dong, W. B., Sun, G. L., and Li, C. D. (2014). Molecular detection of QTL controlling plant height components in a doubled haploid barley population. Genet. Mol. Res. 13, 3089–3099. doi: 10.4238/2014.April.17.5
Ren, X. F., Sun, D. F., Sun, G. L., Li, C. D., and Dong, W. B. (2013). Molecular detection of QTL for agronomic and quality traits in a doubled haploid barley population. Aust. J. Crop Sci. 7, 878–886.
Sameri, M., Nakamura, S., Nair, S. K., Takeda, K., and Komatsuda, T. (2009). A quantitative trait locus for reduced culm internode length in barley segregates as a Mendelian gene. Theor. Appl. Genet. 118, 643–652. doi: 10.1007/s00122-008-0926-9
Sameri, M., Takeda, K., and Komatsuda, T. (2006). Quantitative trait loci controlling agronomic traits in recombinant inbred lines from a cross of oriental- and occidental-type barley cultivars. Breeding Sci. 56, 243–252. doi: 10.1270/jsbbs.56.243
Sato, Y., Sentoku, N., Miura, Y., Hirochika, H., Kitano, H., and Matsuoka, M. (1999). Loss-of-function mutations in the rice homeobox gene OSH15 affect the architecture of internodes resulting in dwarf plants. EMBO J. 18, 992–1002. doi: 10.1093/emboj/18.4.992
Spielmeyer, W., Ellis, M., Robertson, M., Ali, S., Lenton, J. R., and Chandler, P. M. (2004). Isolation of gibberellin metabolic pathway genes from barley and comparative mapping in barley, wheat and rice. Theor. Appl. Genet. 109, 847–855. doi: 10.1007/s00122-004-1689-6
Tamba, C. L., Ni, Y. L., and Zhang, Y. M. (2017). Iterative sure independence screening EM-Bayesian LASSO algorithm for multi-locus genome-wide association studies. PLoS Comput. Biol. 13:e1005357. doi: 10.1371/journal.pcbi.1005357
Tavakol, E., Okagaki, R., Verderio, G., Shariati, J. V., Hussien, A., Bilgic, H., et al. (2015). The barley Uniculme4 gene encodes a BLADE-ON-PETIOLE-like protein that controls tillering and leaf patterning. Plant Physiol. 168, 164–174. doi: 10.1104/pp.114.252882
Terzi, V., Tumino, G., Pagani, D., Rizza, F., Ghizzoni, R., Morcia, C., et al. (2017). Barley developmental mutants: The high road to understand the cereal spike morphology. Diversity 9:21. doi: 10.3390/d9020021
Tian, F., Bradbury, P. J., Brown, P. J., Hung, H., Sun, Q., Flint-Garcia, S., et al. (2011). Genome-wide association study of leaf architecture in the maize nested association mapping population. Nat. Genet. 43, 159–162. doi: 10.1038/ng.746
Varshney, R. K., Paulo, M. J., Grando, S., van Eeuwijk, F. A., Keizer, L. C. P., Guo, P., et al. (2012). Genome wide association analyses for drought tolerance related traits in barley (Hordeum vulgare L.). Field Crop. Res. 126, 171–180. doi: 10.1016/j.fcr.2011.10.008
Voorrips, R. E. (2002). MapChart: software for the graphical presentation of linkage maps and QTLs. J. Hered. 93, 77–78. doi: 10.1093/jhered/93.1.77
Wang, J., Sun, G., Ren, X., Li, C., Liu, L., Wang, Q., et al. (2016a). QTL underlying some agronomic traits in barley detected by SNP markers. BMC Genet. 17:103. doi: 10.1186/s12863-016-0409-y
Wang, J., Yang, J., McNeil, D. L., and Zhou, M. (2010). Identification and molecular mapping of a dwarfing gene in barley (Hordeum vulgare L.) and its correlation with other agronomic traits. Euphytica 175, 331–342. doi: 10.1007/s10681-010-0175-2
Wang, S. B., Feng, J. Y., Ren, W. L., Huang, B., Zhou, L., Wen, Y. J., et al. (2016b). Improving power and accuracy of genome-wide association studies via a multi-locus mixed linear model methodology. Sci. Rep. 6:19444. doi: 10.1038/srep19444
Wen, Y. J., Zhang, H., Ni, Y. L., Huang, B., Zhang, J., Feng, J. Y., et al. (2018). Methodological implementation of mixed linear models in multi-locus genome-wide association studies. Brief Bioinform. 19, 700–712. doi: 10.1093/bib/bbw145
Wójcik-Jagła, M., Fiust, A., Koscielniak, J., and Rapacz, M. (2018). Association mapping of drought tolerance-related traits in barley to complement a traditional biparental QTL mapping study. Theor. Appl. Genet. 131, 167–181. doi: 10.1007/s00122-017-2994-1
Wu, T., and Cao, J. S. (2008). Differential gene expression of tropical pumpkin (Cucurbita moschata Duchesne) bush mutant during internode development. Sci. Hortic. 117, 219–224. doi: 10.1016/j.scienta.2008.04.002
Xia, T., Xiao, D., Liu, D., Chai, W., Gong, Q., and Wang, N. N. (2012). Heterologous expression of ATG8c from soybean confers tolerance to nitrogen deficiency and increases yield in Arabidopsis. PLoS ONE 7:e37217. doi: 10.1371/journal.pone.0037217
Xu, X., Sharma, R., Tondelli, A., Russell, J., Comadran, J., Schnaithmann, F., et al. (2018). genome-wide association analysis of grain yield-associated traits in a Pan-European barley cultivar collection. Plant Genome 11:170073. doi: 10.3835/plantgenome2017.08.0073
Xu, Y., Jia, Q., Zhou, G., Zhang, X. Q., Angessa, T., Broughton, S., et al. (2017). Characterization of the sdw1 semi-dwarf gene in barley. BMC Plant Biol. 17:11. doi: 10.1186/s12870-016-0964-4
Yang, X., Yan, J., Shah, T., Warburton, M. L., Li, Q., Li, L., et al. (2010). Genetic analysis and characterization of a new maize association mapping panel for quantitative trait loci dissection. Theor. Appl. Genet. 121, 417–431. doi: 10.1007/s00122-010-1320-y
Yu, J., and Buckler, E. S. (2006). Genetic association mapping and genome organization of maize. Curr. Opin. Biotechnol. 17, 155–160. doi: 10.1016/j.copbio.2006.02.003
Zhang, F., Liu, X., Zuo, K., Sun, X., and Tang, K. (2011). Molecular cloning and expression analysis of a novel SANT/MYB gene from Gossypium barbadense. Mol. Biol. Rep. 38, 2329–2336. doi: 10.1007/s11033-010-0366-x
Zhang, J., Feng, J. Y., Ni, Y. L., Wen, Y. J., Niu, Y., Tamba, C. L., et al. (2017). pLARmEB: integration of least angle regression with empirical Bayes for multilocus genome-wide association studies. Heredity 118, 517–524. doi: 10.1038/hdy.2017.8
Zhang, Y. M., Mao, Y., Xie, C., Smith, H., Luo, L., and Xu, S. (2005). Mapping quantitative trait loci using naturally occurring genetic variance among commercial inbred lines of maize (Zea mays L.). Genetics 169, 2267–2275. doi: 10.1534/genetics.104.033217
Zhang, Y.-M., and Tamba, C. L. (2018). A fast mrMLM algorithm for multi-locus genome-wide association studies. bioRxiv [preprint] 341784. doi: 10.1101/341784
Zhang, Z., Ersoz, E., Lai, C. Q., Todhunter, R. J., Tiwari, H. K., Gore, M. A., et al. (2010). Mixed linear model approach adapted for genome-wide association studies. Nat. Genet. 42, 355–360. doi: 10.1038/ng.546
Zhuang, J. Y., Lin, H. X., Lu, J., Qian, H.-R., Hittalmani, S., Huang, N., et al. (1997). Analysis of QTL × environment interaction for yield components and plant height in rice. Theor. Appl. Genet. 95, 799–808. doi: 10.1007/s001220050628
Keywords: genome-wide association study, barley, multi-locus model, doubled haploid population, quantitative trait nucleotide, candidate gene
Citation: Hu X, Zuo J, Wang J, Liu L, Sun G, Li C, Ren X and Sun D (2018) Multi-Locus Genome-Wide Association Studies for 14 Main Agronomic Traits in Barley. Front. Plant Sci. 9:1683. doi: 10.3389/fpls.2018.01683
Received: 30 July 2018; Accepted: 29 October 2018;
Published: 20 November 2018.
Edited by:
Zhenyu Jia, University of California, Riverside, United StatesCopyright © 2018 Hu, Zuo, Wang, Liu, Sun, Li, Ren and Sun. This is an open-access article distributed under the terms of the Creative Commons Attribution License (CC BY). The use, distribution or reproduction in other forums is permitted, provided the original author(s) and the copyright owner(s) are credited and that the original publication in this journal is cited, in accordance with accepted academic practice. No use, distribution or reproduction is permitted which does not comply with these terms.
*Correspondence: Dongfa Sun, sundongfa1@mail.hzau.edu.cn
Xifeng Ren, renxifeng@mail.hzau.edu.cn
†These authors have contributed equally to this work