- 1Department of Genomics and Biology of Fruit Crops, Research and Innovation Centre, Fondazione Edmund Mach, San Michele all′Adige, Italy
- 2Unit of Computational Biology, Research and Innovation Centre, Fondazione Edmund Mach, San Michele all′Adige, Italy
- 3Department of Information Engineering and Computer Science, University of Trento, Trento, Italy
- 4Consiglio Nazionale delle Ricerche-Institute of Materials for Electronics and Magnetism, Trento, Italy
In recent years the scientific community has been heavily engaged in studying the grapevine response to climate change. Final goal is the identification of key genetic traits to be used in grapevine breeding and the setting of agronomic practices to improve climatic resilience. The increasing availability of transcriptomic studies, describing gene expression in many tissues and developmental, or treatment conditions, have allowed the implementation of gene expression compendia, which enclose a huge amount of information. The mining of transcriptomic data represents an effective approach to expand a known local gene network (LGN) by finding new related genes. We recently published a pipeline based on the iterative application of the PC-algorithm, named NES2RA, to expand gene networks in Escherichia coli and Arabidopsis thaliana. Here, we propose the application of this method to the grapevine transcriptomic compendium Vespucci, in order to expand four LGNs related to the grapevine response to climate change. Two networks are related to the secondary metabolic pathways for anthocyanin and stilbenoid synthesis, involved in the response to solar radiation, whereas the other two are signaling networks, related to the hormones abscisic acid and ethylene, possibly involved in the regulation of cell water balance and cuticle transpiration. The expansion networks produced by NES2RA algorithm have been evaluated by comparison with experimental data and biological knowledge on the identified genes showing fairly good consistency of the results. In addition, the algorithm was effective in retaining only the most significant interactions among the genes providing a useful framework for experimental validation. The application of the NES2RA to Vitis vinifera expression data by means of the BOINC-based implementation is available upon request (valter.cavecchia@cnr.it).
Introduction
Climate change represents a major challenge for modern and future agriculture, in particular for grapevine cultivation and wine production due its wide geographical distribution, and economic relevance. A significant warming has already been observed in most grape-growing areas of the world affecting the varietal choice as well as grape quality (Mozell and Thach, 2014). The constant temperature increase trend has been triggering an advanced phenology, anticipating the ripening phase to warmer periods and affecting grape composition and development (Teixeira et al., 2013). An increased intensity of visible and UV solar radiation is also severely impacting on leaf morphological and biochemical parameters such as area, pigment, and antioxidant composition (Castagna et al., 2017), and berry characteristics, such as sugar-acid ratio and polyphenol abundance and composition (Bobeica et al., 2015). Finally, water deficit will be a major concern, not only in hotspots already facing seasonal drought, but also where rare heavy rains will alternate with drought during the same season. The scientific community is thus deploying great efforts to study the grapevine response to environmental changes (extensively reviewed in Geròs et al., 2015), with the aim to improve disease and yield prediction models and to identify key genetic traits to be used in grapevine breeding. Transcriptomic studies could be very useful to investigate plant adaptation as tuning of gene expression represents a major adaptive response of the plant. The increasing availability of grapevine transcriptomic data has prompted the creation of Vitis-gene expression compendia, such as VTCdb which included only microarray data (Wong et al., 2013) and Vespucci which comprises both microarray and RNA-seq data (Moretto et al., 2016, see Table 1). These databases contain a considerable amount of information that requires proper tools to be explored and interpreted. NES2RA algorithm (Asnicar et al., 2016) has been developed to expand known LGNs in model organisms, such as E. coli and A. thaliana, from transcriptomic data. It showed better performance than other approaches such as ARACNE (Margolin et al., 2006) and NESRA (Asnicar et al., 2015).
In this work we present the application of NES2RA to expand four grapevine LGNs related to the response to climate changes using the Vespucci compendium. To cope with the large computational requirement, NES2RA was run on the gene@home project developed on the Berkeley Open Infrastructure for Network Computing (BOINC) platform TN-Grid, which manages the distribution of the calculation among computers made available by the volunteers participating in the gene@home project (Asnicar et al., 2015). Here, we show that this approach can provide reliable results also in a non-model species such as V. vinifera, for which a much more limited amount of data is available. NES2RA final output consists in an expansion gene list and in an interaction file – defining the relationships among the genes – which are used to reconstruct the expansion network. This represents a helpful tool for biologists to expand LGNs based on a priori knowledge and/or experimental evidence on a system biology level providing novel candidates for further characterization. We focused on four grapevine networks possibly involved in the climate change response. Two networks are related to the secondary metabolic pathways for anthocyanin and stilbenoid synthesis, involved in the response to solar radiation, the remaining two are signaling networks, related to the hormones abscisic acid (ABA) and ethylene, involved in the regulation of cell water balance and cuticle transpiration. The network named “Anthocyanins,” i.e., the red pigments of grapes, comprises VvUFGT, which codes for the main enzyme responsible for anthocyanin biosynthesis (Ford et al., 1998), a cluster of four VvMYBA genes (Kobayashi et al., 2002; Walker et al., 2007) and VvMYC1, coding for transcription factors known to physically interact and regulate VvUFGT expression (Hichri et al., 2010). The network named “Stilbenoids,” i.e., grapevine secondary metabolites with plant defense properties (Mattivi et al., 2011), is composed by 13 genes: four VvSTS genes encoding for different stilbene synthase isoforms, the enzymes catalyzing the synthesis of resveratrol, VvMYB14 and VvMYB15, coding for two characterized regulators of stilbene biosynthesis in grapevine (Höll et al., 2013) and seven genes encoding for peroxidases, enzymes potentially involved in the oligomerization of stilbene monomers. These enzymes have been included even if they have not been proved yet to be part of the stilbenoids regulatory network. The network named “ERFs” is formed by three genes coding for ethylene responsive transcription factors: VvERF045 is up-regulated during berry ripening, while VvERF042 and VvERF044 are down-regulated and belong to the SHINE clade of AP2/ERF transcription factors that in Arabidopsis have been associated to cuticle structure, leaf and flower composition, and water loss (Aharoni et al., 2004; Shi et al., 2011; Leida et al., 2016). Finally, the network named “ABA” consists of five genes related to ABA signaling: three encoding for transcription factors, the ABA responsive element binding factor AREB2, the homeobox HB7, and VvNAC26; one for an ABA receptor homologous to the Arabidopsis ABA insensitive ABI1 gene, and one for a receptor kinase LRK10. These genes are up-regulated by ABA treatment in grapevine cell cultures and pre-véraison berry skin samples (Nicolas et al., 2014; Pilati et al., 2017). VvNAC26 expression affects berry size, likely by positively regulating ABA biosynthesis (Tello et al., 2015); HB7 and ABI1 are strictly interdependent in the ABA response in Arabidopsis (Soderman et al., 1996), whereas LRK10 is related to ABA signaling and drought resistance in Arabidopsis (Lim et al., 2015).
Materials and Methods
Data Pre-processing of Vespucci Compendium
The Vespucci grapevine transcriptomic compendium (Moretto et al., 2016) available at http://vitis.colombos.fmach.it/, is composed by 29,090 genes and 1,565 contrasts, which refer to the comparisons of condition vs. reference within each experiment. The pre-processing procedure comprised three steps: (1) removal of contrasts with more than 55% of missing values; (2) removal of genes with more than 55% of missing values; (3) for each gene, replacement of the remaining missing values with the median of its contrasts values. This procedure reduced the original dataset to 28,013 genes and 701 contrasts (Supplementary Table S1).
Gene Network Expansion by NES2RA Algorithm Within gene@home Project
The expansion lists were computed applying the NES2RA algorithm (Asnicar et al., 2016) to the pre-processed Vespucci dataset. NES2RA is based on the PC-algorithm, named after its authors Peter and Clark (Spirtes and Glymour, 1991), a gaussian graphical model (GGM) that finds causal relationships from observational data. Other GGM have been successfully applied to reconstruct plant regulatory gene networks (Ma et al., 2007), but the PC-algorithm has been shown to be the most promising for biological applications compared to Neighborhood selection, G-Lasso, and Shrinkage estimation (Albieri and Didelez, 2014).
The PC-algorithm is based on a systematic test for conditional independence to retain significant relations between pairs of genes. It starts from a fully connected network and removes interactions between genes, if it finds a set of genes that supports that interaction (i.e., separation set). From a mathematical point of view, the test for statistical independence between the genes a, b conditioned by a set of genes S is driven by the estimation of the partial correlation ρ (a,b∖S). The example reported in Figure 1 shows how the estimated partial-correlation allows the identification of spurious interactions, with respect to the simple correlation. On the other hand, the exhaustive exploration of all the subsets of conditioning genes is computational impossible, therefore the PC-algorithm takes into consideration only a limited number of those sets, as described in Algorithm 3 in Asnicar et al. (2016). At the same time, the NES2RA algorithm, developed to cope with this computational complexity, randomly divides the Vespucci dataset into tiles of equal number of genes (subsetting), where each tile always includes all the genes of the LGN, to be then processed by the PC-algorithm. The random subsettings of all the genes in the genome are repeated for a given numbers of iterations. The NES2RA algorithm currently runs as part of the gene@home project which aims to systematically expand gene regulatory networks based on available public gene expression data. The gene@home project relies on thousands of volunteers’ computers by means of the BOINC system (Anderson, 2004) within the TN-Grid platform (Asnicar et al., 2015).
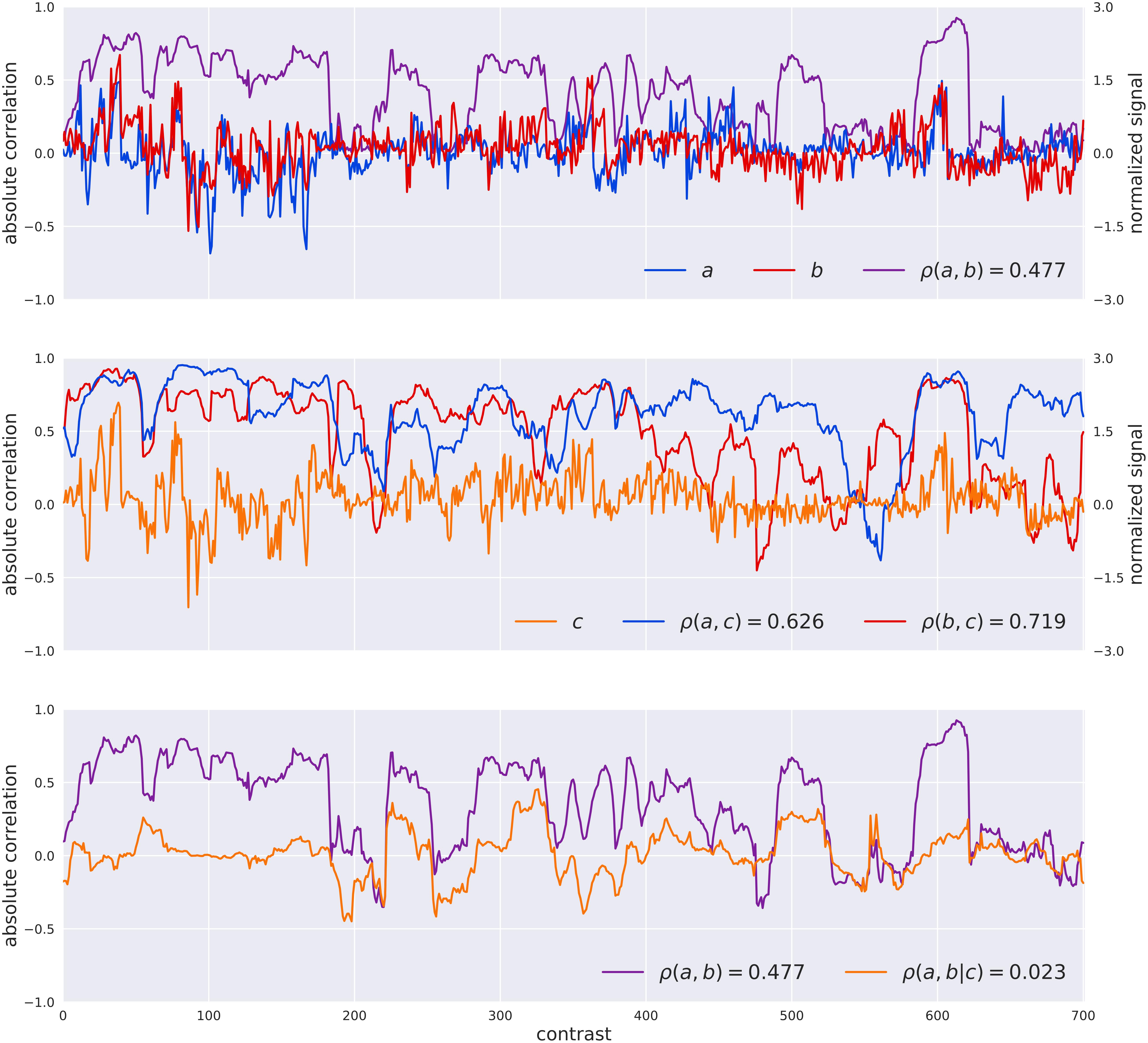
FIGURE 1. Visualization of the difference between the Pearson correlation [ρ(A,B)] of two genes A and B and their partial correlation given the gene C [ρ(A,B| C)] as computed by the PC-algorithm. The top plot reports the normalized raw signals of A and B, their windowed Pearson correlation (window-size of 31 experiments for visualization sake), and the value of their Pearson correlation coefficient in the legend. In the middle plot the signal of C is introduced as well as the windowed correlations between A,C and B,C and the values of correlation. The bottom plot shows the simple correlation between A,B and the partial correlation of A,B given C. In this case the PC-algorithm, that systematically search for variables for separating pair of variables, would have considered the correlation between A and B as completely explained in terms of C (a separation set of dimension one) and removed the interaction between them for the successive steps.
The networks found by the PC-algorithm are then post-processed off-line to determine and aggregate the final expansion gene lists. List aggregation is done with the ranking aggregation method Markov Chain 4 (MC4) (Dwork et al., 2001), since it has been shown to yield the most precise results (Asnicar et al., 2016). For the MC4 ranking aggregation we decided to consider the first 1,000 genes of each expansion output list (K = 1,000).
Gene Network Expansion by Simple Correlation
To better show the difference between NES2RA and simple correlation results, the input networks were expanded computing the Pearson correlation coefficient between each input gene and all the other genes in the dataset, in pairs. The mean correlation of each gene respect to all the input genes was computed and sorted to provide the final expansion list.
Networks Visualization
For each expanded gene network a limited number of genes were graphically depicted using Cytoscape (Shannon et al., 2003). In the case of the NES2RA networks, the putative interactions were determined using the interaction files produced by NES2RA as an intermediate output.
In the case of the simple correlation networks, an interaction between two genes was depicted if the Pearson coefficient passed the Z-test for correlation (P-value < 0.05).
Validation Methods
Output genes were annotated according to the 12X PN40024 reference genome version 1 (V1) gene prediction annotation (Supplementary Table S2 in Grimplet et al., 2012 and available at http://genomes.cribi.unipd.it/DATA/V1/ANNOTATION/), except for few transcription factor gene families recently characterized in grapevine, for which we referred to the specific published annotations (Ford et al., 1998; Gomez et al., 2009, 2011; Hichri et al., 2010; Licausi et al., 2010; Vannozzi et al., 2012; Wang et al., 2013, 2014; Liu et al., 2014; Cavallini et al., 2015; Wong et al., 2016). Two of the four networks produced by NES2RA were validated with published experimental datasets by computing a Precision curve to estimate the overlap between the two gene sets. Precision has been calculated as the intersection of the first k genes of the expansion lists calculated by NES2RA with the set of published experimental data, normalized on k. Additionally, two statistical approaches were applied to all the four networks: (i) the Gene Ontology functional category enrichment analysis, using the topGO Bioconductor package (Alexa and Rahnenfuehrer, 2015) coupled with the goslim_plant.obo available at http://www.geneontology.org, and (ii) motif enrichment promoter analysis using DREME (MEME suite, Bailey et al., 2009) and DAP motif database (O’Malley et al., 2016).
Code Availability
A stand-alone version of the NES2RA pipeline is publicly available at https://github.com/lucamasera/NESSRA. In addition to the code, the repository contains the raw results presented in this paper before the aggregation step and the input files (local gene networks and the expression dataset) required to reproduce them. Note that, due to the random nature of the subsetting step, the results of the stand-alone version may differ from the ones computed by gene@home project presented in this work.
Results
Expansion of Four Grapevine Gene Networks
The four grapevine gene networks, namely “Anthocyanins,” “Stilbenoids,” “ERFs,” and “ABA,” were expanded using NES2RA in order to identify additional genes causally related to them. The overall flow of analysis is depicted in Figure 2A, where the case of “ERFs” LGN is reported as an example. To reduce computing complexity, the Vespucci normalized expression dataset was divided into either 29 tiles of 1,000 gene (t = 1000) or 57 tiles of 500 genes (t = 500). This step was repeated 2,000 times (i = 2000) or 1,000 times (i = 1000). The three input genes of “ERFs” were present in each tile. The gene@home project allowed the distribution of the computation by the PC-algorithm as tiles to volunteers’ computers. Then, the partial results were post-processed to obtain four ranked lists of candidate genes and the corresponding interactions. The first 1,000 genes of each list were then aggregated using MC4 (K = 1000, see section “Material and Methods”). The first 22 genes of the aggregated list and their interactions with the “ERFs” network were used to depict the expanded network, whereas a larger list consisting of the top 100 genes was validated using Gene Ontology functional class enrichment, promoter analysis, and literature mining.
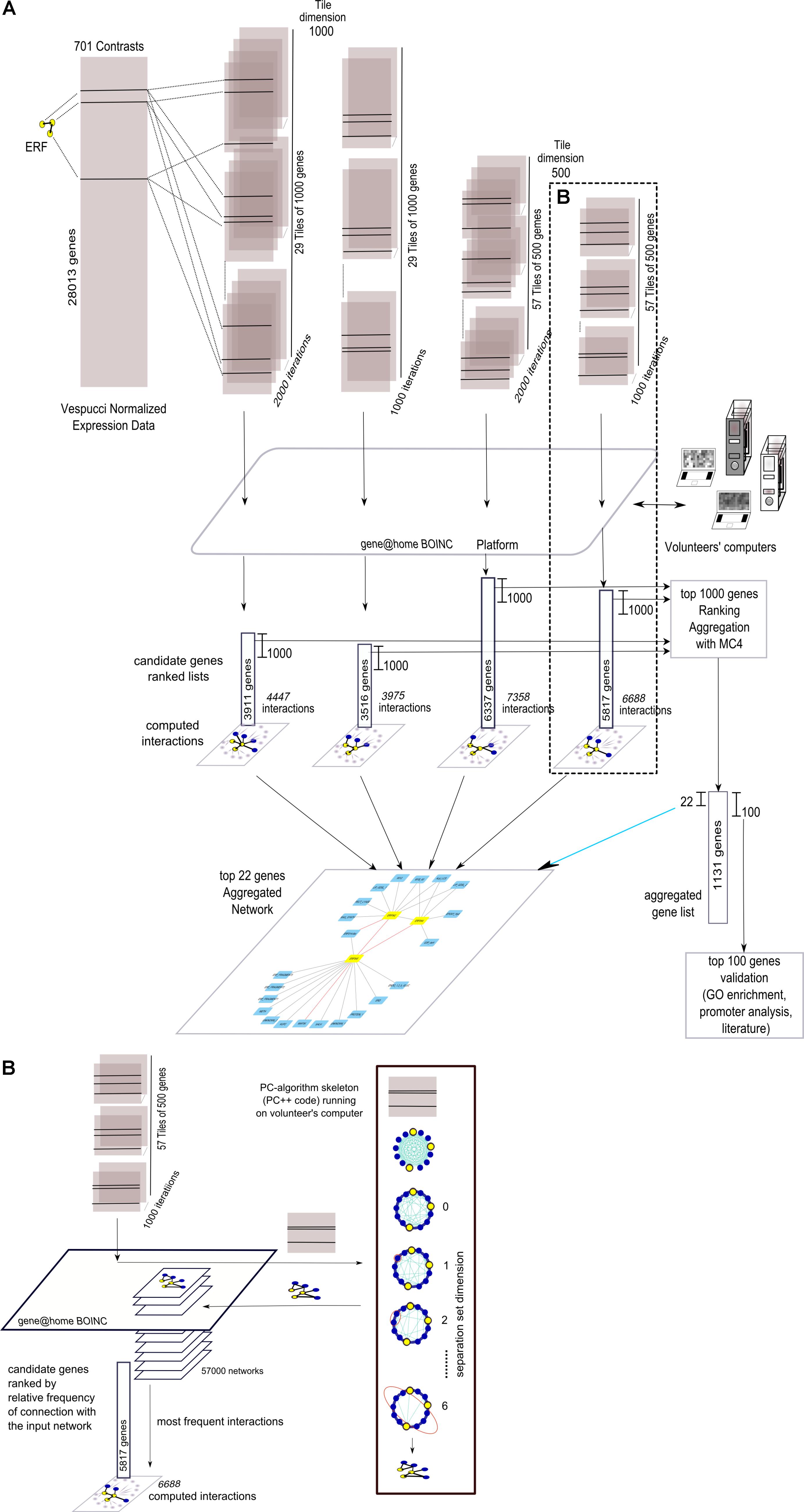
FIGURE 2. Overall view of the network expansion method. (A) Summary graphical visualization of the application (using four parameters settings) of NES2RA to the ERFs LGN using data from the Vespucci compendium. Each tile, including all the genes of the LGN, generated during the subsetting step, is analysed using the PC-algorithm that runs on the volunteers’ computers, made available through the gene@home BOINC project. The post-processing takes as input all the resulting networks and ranks the genes using their relative frequency, measured as the number of times a gene was found connected to the input LGN. In the final expansion network, the nodes are the LGN (in yellow) and the top-ranking genes of the aggregated list (in light blue), whereas the edges derive from the union of all the found interactions. (B) Details on the internal steps of the PC-algorithm that, starting from a complete graph, iteratively searches separation sets that allows the cut of an edge. For each tile, the result of the application of the PC-algorithm is a network.
More in detail, as depicted in Figure 2B, given a tile, the PC-algorithm produced a network that represents the putative causal interactions between the genes. The PC-algorithm, starting from the complete correlation network and given a pair of interacting genes, searched for a growing-dimension separation set of genes within the genes interacting with the pair. The 57,000 networks thus obtained were post-processed, that is, interactions between genes, and “ERFs” genes were counted over the iterations and ranked accordingly. Finally, the candidate genes were ranked with respect to the overall relative frequency of interaction with the input network.
The results of the expansions of the four grapevine networks are presented in Table 1, where, for each set of parameters, the number of genes found and the number of interactions among them is reported. There is an evident dependence of the output gene number on the parameter settings. The first 1,000 genes of each of the four output lists were aggregated in order to have a refined final list, reported in the last column of Table 1.
Expansion Networks Produced by NES2RA and Simple Correlation
In the attempt to highlight the difference between the expansion gene lists obtained using NES2RA and the simple correlation, both analyses have been performed on the same networks and the top 100 genes were considered for the comparison (Supplementary Tables S2, S3). One more network was computed, made of just one gene, the transcription factor AREB2 included in the ABA network, because it represents the simplest network possible. The networks produced by the two approaches have been visualized, considering only the top 20–30 genes of the lists for clarity (Figure 3 and Supplementary Table S4). While in the case of the simple correlation networks the genes are almost fully connected, the number of interactions retained by NES2RA is considerably reduced. A clear effect of the latter approach is the removal of not significant interactions, possibly those due to noisy or redundant information. This allows to reduce the complexity of the network and focus on the network topology and the most likely gene interactions. Jaccard similarity index curves were calculated to compare the expansion gene lists obtained. Quite interestingly, the expansions of the single-gene network obtained with NES2RA and simple correlation resulted the most similar ones, with a rather constant high value of Jaccard index (corresponding to about 60% overlap). Conversely, the curves for the LGN expansions showed different trends and in general a lower similarity, suggesting that when a network rather than a single gene is expanded, the two approaches identify different sets of genes.
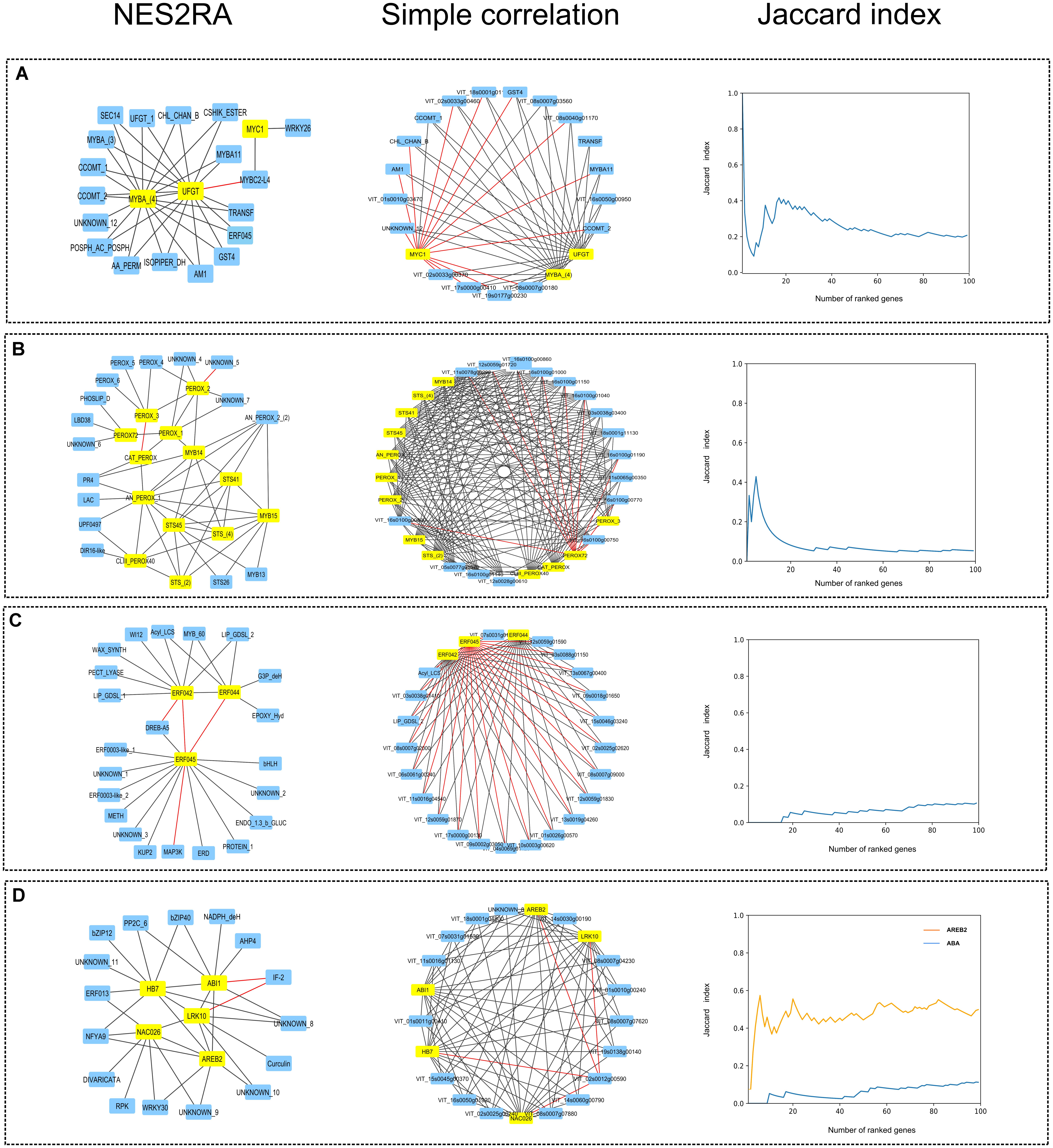
FIGURE 3. Comparison between expansion networks obtained with NES2RA and simple correlation. First column: visualization of the four gene networks obtained with NES2RA. Second column: visualization of the four gene networks obtained by computing simple correlation. Input genes have a yellow background, expansion genes a blue one. Gene names are abbreviations (see Supplementary Table S4 for the complete description). Edges represent gene interactions: black and red lines link positively and negatively correlated genes, respectively. Third column: graphical representation of the Jaccard similarity index, calculated between the top-100 genes of the NES2RA and simple correlation expansion lists. (A) “Anthocyanins”, (B) “Stilbenoids,” (C) “ERFs,” and (D) “ABA” (and AREB2, in the Jaccard graph, orange line) networks.
In the “Anthocyanins” network (Figure 3A), all the genes of the expansion except VvMYBC2-L4 were positively correlated with both VvMYBA cluster (including VvMYBA1 and VvMYBA2) and VvUFGT, highlighting the intimate relationship of this pair in regulating anthocyanin biosynthesis. VvMYC1 was positively correlated exclusively with VvWRKY26 and VvMYBC2-L4, which is in turn negatively correlated to VvUFGT. Concerning the “Stilbenoids” network (Figure 3B), characterized by a higher number of genes not fully confirmed by previous evidences, NES2RA analysis provided first of all an interesting analysis of the LGN, showing its lower homogeneity. Indeed, VvMYB14 and VvMYB15 were found interacting with all the stilbene synthases and three out of seven peroxidases, suggesting a minor involvement of the remaining four peroxidases in this pathway. Moreover, here we can appreciate the power of the PC-algorithm in eliminating spurious relationships in the case of AN_PEROX, PEROX1, and VvMYB14. Indeed, they are all highly co-expressed, but only the interactions between the two peroxidases and VvMYB14 were retained. VvMYB14 is indeed sufficient to explain the co-expression of the two enzymes, thus suggesting it could regulate the transcription of both. Widening to the expansion network, NES2RA identified one STS (VvSTS26), one MYB (VvMYB13) and a cluster of two peroxidase genes (AN_PEROX_2) related to the VvMYB14-15 system and then other peroxidases and stress related genes connected to the other peroxidases. The topology of the “ERFs” expansion network (Figure 3C) revealed a bipartite structure with two ERFs anti-correlated to the third one and two groups of related genes, one enriched in transcription factors (lower group) and the other in genes related to lipid and wax metabolism (upper group). Finally, “ABA” network (Figure 3D) was characterized by a rather homogeneous distribution of the interactions both in the input and in the expanded gene sets. The interaction between HB7_2 and ABI1 was confirmed. In general, the three transcription factors were connected to many other regulators, including a Nuclear Factor Y, whereas the two kinases interacted with other signaling proteins (DBPPP2C_6, AHP4).
Validation of the Expanded Gene Networks
To estimate the biological relevance of the genes identified by NES2RA, we searched for public experimental data – not included in Vespucci compendium – with an experimental design suited to validate our networks and we could find them only for “ABA” and “Anthocyanins” networks. The dataset for “ABA” consisted in the genes modulated by ABA treatment in grapevine berry skins and in cell cultures (Nicolas et al., 2014; Pilati et al., 2017); for the Anthocyanins network the dataset consisted in the differentially modulated genes in grapevine hairy roots overexpressing VvMYBA1 (Cutanda-Perez et al., 2009). As shown in Supplementary Figure S1 precision is initially very high and then decreases with the number of genes, suggesting that genes are ranked in a biologically relevant way. Considering the top 100 genes precision decreases to 40 and 20% in the case of “ABA” and “Anthocyanins,” respectively, suggesting that the top 100 genes of the expansion lists represents a reasonable trade-off between focusing on the strongest candidates of the expanded networks and preserving enough statistical power for the other analyses. Thus, this threshold was applied to the other two networks too.
The four gene expansion lists were analyzed with TopGO (Fisher’s exact test) to get a general overview of the more represented functional categories (Supplementary Table S5). Despite the high number of unknown or non-annotated genes, this analysis showed that the top 100 genes in the NES2RA output were functionally related to the input genes: the “Anthocyanins” and “Stilbenoids” expansions were populated by genes related to secondary metabolism and defense, while the “ERFs” and “ABA” expansions were populated by many regulators and genes involved in the lipid biosynthesis and in the hormonal and abiotic stress responses, respectively.
To verify if the expanded networks included co-regulated genes, sharing common regulatory motifs in their promoters, the 1 Kb promoter regions of the top 100-gene lists were retrieved and analyzed with DREME (Bailey et al., 2009). Results are presented in Supplementary Table S6.
Promoter analysis produced different outputs for the four networks. The promoters of the genes of the “Anthocyanins” network were enriched in two 8-nt motifs, which were not present in the reference annotation database (O’Malley et al., 2016), meaning that these sequences have not been already characterized and therefore cannot be associated yet to a transcription factor. “ABA” network was highly enriched in three 8-nt motifs well annotated in the reference databases: two of them (CACBTGTC and CCACGTGK) matched with the motifs recognized by bHLH and/or bZIP AREB transcription factors and one (ACVCTCCT) by MYB88. “Stilbenoids” network retrieved a sequence of just 5-nt (ACGYG), rather unspecific since matching to 72 possible transcription factors belonging to several transcription factor families. Finally, in the “ERFs” network no enriched motifs could be found. Overall, this analysis suggests that despite the starting networks were composed, partly or exclusively, by transcription factors, the expansion sets not necessarily are enriched in their direct targets. Further experiments would be necessary to come to a more general conclusion.
Discussion
In the field of viticulture, growing attention is dedicated to investigate plant adaptation and stress responses to climate change, as this represents the main challenge for the near future (Geròs et al., 2015). Here we present an in silico approach to extend our knowledge on gene networks related to plant-environment interaction, relevant for berry quality and water stress response, with the final aim to identify novel candidates to drive experimental work. Anthocyanin and stilbenoid synthesis are known to be deeply affected by changes in external conditions, such as air temperature, water availability and solar radiation type and intensity (Teixeira et al., 2013). Besides, specific ethylene responsive factors are reported to be involved in cuticle metabolism thus regulating water transpiration (Aharoni et al., 2004; Shi et al., 2011) and ABA is intimately related to plant water balance and drought stress tolerance (Fujita et al., 2011; Golldack et al., 2014). Despite the role of these cellular processes and/or factors has been well ascertained at the physiological level, much remains to be elucidated at the molecular level.
Gene network computation based on large datasets of expression data represents an interesting approach to unveil the complex interactions between transcripts inside the cell. Here we present the application of a PC-based algorithm NES2RA (Asnicar et al., 2016) to the grapevine transcriptomic compendium Vespucci (Moretto et al., 2016) in order to expand LGNs (Figure 2). NES2RA was initially set up to analyze model organisms transcriptomic data, such as E. coli and A. thaliana, where a large number of experimental conditions are available. However, considering NES2RA ability to scale to lower data dimensions, we were interested in testing its performance on a non-model species such as V. vinifera. Compared to simple correlation approaches, NES2RA applies a statistical criterion of conditional independence which, starting from a fully connected network, eliminates spurious and/or redundant relationships (Figure 1). This simplifies the network and highlights significant relationships between genes, named interactions. The same challenge has been addressed in other works by calculating a co-expression index [based on highest reciprocal ranks (HRR) and mutual ranks (MR)] within the VTCdb (Wong et al., 2013) and ad-hoc datasets in specific studies, also including promoter cis-regulatory elements analysis (Wong et al., 2016, 2017; Vannozzi et al., 2018). While expanding a single gene produces similar results with all the approaches, the power of NES2RA becomes more evident when expanding LGNs (Figure 3). Moreover, the expansions obtained with NES2RA strictly depend on the nature of the LGN for two reasons: firstly, the input genes define the separation set during the PC-algorithm run, secondly, the post-processing pipeline top-ranks genes with the largest number of connections to the whole LGN. Therefore NES2RA preferentially identifies highly connected central nodes rather than one-to-one direct gene interactions, such as the case of a transcription factor and its target. The comparison of NES2RA expansions with experimental datasets found in literature confirmed that the top-ranking genes were modulated upon ABA stimulus or VvMYBA1 over-expression supporting they belong to these networks (Supplementary Figure S1). The Gene Ontology enrichment highlighted for all the networks functional categories related to the LGNs (Supplementary Table S5). Conversely, promoter motif enrichment analysis produced more case-specific results (Supplementary Table S6): the “Anthocyanins” and “ABA” expansions genes were enriched in motifs, uncharacterized in the former case and recognized by ABA responsive transcription factors in the latter; “Stilbenoids” and “ERFs” expansions genes didn’t show any significant co-regulation, despite the fact that the second network included exclusively transcription factors. We can speculate that this result might be ascribed to the heterogeneity of the input network and/or to the amplification of the signaling cascade in the expansion network.
Going into the detail of each expanded network, the VvMYBA cluster (including VvMYBA1 and VvMYBA2) was found to interact with VvUFGT and both genes were positively correlated with VvAM1 and VvGST4, known to be involved in vacuolar sequestration of anthocyanins (Gomez et al., 2009, 2011). Interestingly, VvMYBA and VvUFGT were found interacting with VvERF045, supporting the recently suggested role of this factor in regulating genes of the anthocyanin pathway (Leida et al., 2016), and with other five MYBA regulators and enzymes of the general phenylpropanoid pathway (CCOMT and CSHIK_ESTER). The MYC1 interaction with the two transcription factors VvWRKY26 and VvMYBC2-L4, recently characterized as regulators of both Anthocyanin and PAs biosynthesis (Cavallini et al., 2015; Amato et al., 2017), confirms its involvement in both pathways. Apparently, NES2RA is not able to clearly solve the cases of multifactors-mediated regulation such as the case of the known MBW mode of action. However, we cannot appreciate whether this is due to the mining approach or to the intrinsic multilevel nature of the regulation which could be not purely transcriptional.
Considering the “Stilbenoids” expansion network, the already known interactions within the input gene set were confirmed: VvMYB14 and VvMYB15 were found to interact with eight stilbene synthases (VvSTS25/27/29/31, VvSTS41, VvSTS45, and VvSTS35/48) as supported by the recent evidences by Wong et al. (2016) and Vannozzi et al. (2018), and with two peroxidases, AN_PEROX_1 and CLIII_PEROX40, both co-expressed with VvSTS41 and VvSTS45 in VTCdb database. In addition, NES2RA identified another MYB factor (VvMYB13), which shares close similarity in sequence and expression with VvMYB14 and VvMYB15 (Wong et al., 2016), and additionally a STS (VvSTS26) and a cluster of two peroxidases (AN_PEROX_2) which will be tested for their ability to catalyze stilbenoids synthesis and oligomerization during the grapevine response to abiotic and biotic stresses. The group of PEROX_1, _2, _3, interacting with VvMYB14, is found related to other peroxidases (PEROX_4, _5, _6), which could participate to stilbenoid metabolisms, as well.
Interestingly, among the top-100 genes of the “Stilbenoids” LGN expansion (Supplementary Table S2), there is a Group II WRKY transcription factor, VvWRKY18, which represents a potential new interesting candidate for the regulation of this pathway. On the other hand, the VvWRKY genes proposed by Vannozzi et al. (2018) as involved in the regulation of VvSTS genes are not present in the NES2RA output. The approach of Gene Co-expression Network analysis based on the MR index of co-expression proved to be more suited to identify direct interactions, such as the regulator of a target. Nonetheless, NES2RA expansion of VvSTS29 actually identifies VvWRKY03 (not shown), confirming that when applied to a single gene, the two approaches produce similar results.
“ERFs” expansion network reflected the bipartite structure of the input set, in which the two SHINE genes (VvERF042/44) were inversely related to VvERF045. The only common gene is represented by a DREB transcription factor. VvERF042/44, uncharacterized in grapevine, were found correlated with numerous genes involved in cuticle metabolism such as Acyl-LCS, WAX SYNTH, LIP-GDSL, EPOXY-Hyd (Yeats and Rose, 2013) and one gene linked to stomatal closure regulation, VvMYB60, (Galbiati et al., 2011), supporting the hypothesis that also in grapevine they could play a role in the control of transpiration. Interestingly, VvERF042 and VvERF044 are the putative orthologs of the Arabidopsis SHINE1 and SHINE3 genes, whose silencing leads to a decrease in cutin load and to changes in cell wall structure (Shi et al., 2011), and they resulted down-regulated in grapevine transgenic lines overexpressing VvERF045 (Leida et al., 2016). VvERF045 which is specifically induced at véraison (Leida et al., 2016), seems to interact with other transcription factors (ERF0003-like, bHlH) and genes involved in signaling, suggesting it could be a higher level regulator of berry ripening. “ABA” input network showed a complete connection between ABI1, HB7, AREB2 and LRK10, thus representing a very co-expressed module, VvNAC026 instead was exclusively connected to AREB2 and LRK10 and could represent a second-order, more specialized, level of regulation. This interaction supports the involvement of VvNAC026 in berry size determination via positive regulation of ABA synthesis, as recently proposed by Tello et al. (2015). The expanded network was highly enriched in signaling components, such as transcription factors (VvbZIP12, VvbZIP40, VvWRKY30, Divaricata, VvERF13, NF-YA9) and kinases/phosphorilases (PP2C_6, AHP4, RPK), thus proposing novel candidates to expand ABA regulatory network. Interestingly, the Nuclear Factor YA9 is reported to be induced by salt and PEG treatments and possibly related to seed maturation and dehydration signaling in grapevine (Ren et al., 2016).
Conclusion
In conclusion, we have shown that NES2RA algorithm can be successfully applied to a non-model plant species transcriptomic data to expand LGNs, not necessarily fully characterized. It is meant to be used on a complete database including RNA-seq and microarray data - such as Vespucci - without losing the ability of identifying local specialized networks, in this study the flavonoid-related LGNs. Indeed, it represents a useful tool to mine the entire transcriptome to extend a LGN, on a system biology perspective, as it searches for genes highly connected to the whole LGN. For instance, it seems to find points of convergence between pathways, such as the case of the VvMYC1 gene bridging the Anthocyanin and Proanthocyanidin synthesis pathways. In the case of the ABA expansion, NES2RA identifies many genes known to be related to this signaling network and many other transcription factors and genes representing interesting candidates for experimental characterization. However, we observed that NES2RA does not represent the best tool to find direct targets of transcription factors unless the single gene is analyzed.
Since this approach is computationally demanding, we have overcome this problem by exploiting the BOINC platform, with the drawback of not providing a user-web resource. We are currently developing alternative strategies to solve this problem.
Author Contributions
EB and CM conceived, developed, and coordinated the research. SP, GM, and CM identified the networks and analyzed the expansion results. MM and PS helped with the expression compendium. SV and FA contributed to the whole data analysis, to results aggregation and networks drawing. LM and VC assisted with the BOINC application. GM and SP contributed substantially to manuscript preparation. All authors read and approved the final version of the manuscript.
Funding
For FEM the research was funded by the Autonomous Province of Trento (PAT-ADP 2017-2019).
Conflict of Interest Statement
The authors declare that the research was conducted in the absence of any commercial or financial relationships that could be construed as a potential conflict of interest.
Acknowledgments
The authors would like to thank Gianluca Bortoli, Martin Brugnara, Giulia Gangi, Federica Lago, Gil Amir Mizrahi, Viola Nervini, Anna Saini, and Agnese Zardini for their contribution with networks expansion experiments and their validation.
Supplementary Material
The Supplementary Material for this article can be found online at: https://www.frontiersin.org/articles/10.3389/fpls.2018.01385/full#supplementary-material
References
Aharoni, A., Dixit, S., Jetter, R., Thoenes, E., van Arkel, G., and Pereira, A. (2004). The SHINE clade of AP2 domain transcription factors activates wax biosynthesis, alters cuticle properties, and confers drought tolerance when overexpressed in Arabidopsis. Plant Cell 16, 2463–2480. doi: 10.1105/tpc.104.022897
Albieri, V., and Didelez, V. (2014). Comparison of statistical methods for finding network motifs. Stat. Appl. Genet. Mol. Biol. 13, 403–422. doi: 10.1515/sagmb-2013-0017
Alexa, A., and Rahnenfuehrer, J. (2015). Gene Set Enrichment Analysis With topGO. Available at: https://www.bioconductor.org/packages/devel/bioc/vignettes/topGO/inst/doc/topGO.pdf
Amato, A., Cavallini, E., Zenoni, S., Finezzo, L., Begheldo, M., Ruperti, B., et al. (2017). A grapevine TTG2-like WRKY Transcription factor is involved in regulating vacuolar transport and flavonoid biosynthesis. Front. Plant Sci. 7:1979. doi: 10.3389/fpls.2016.01979
Anderson, D. P. (2004). “BOINC: a system for public-resource computing and storage,” in Proceedings of the 5th IEEE/ACM International Workshop on Grid Computing GRID ’04, (Washington, DC: IEEE Computer Society), 4–10. doi: 10.1109/GRID.2004.14
Asnicar, F., Masera, L., Coller, E., Gallo, C., Sella, N., Tolio, T., et al. (2016). NES 2 RA: network expansion by stratified variable subsetting and ranking aggregation. Int. J. High Perform. Comput. Appl. 32, 380–392. doi: 10.1177/1094342016662508
Asnicar, F., Sella, N., Masera, L., Morettin, P., Tolio, T., Semeniuta, S., et al. (2015). “TN-grid and gene@home project: volunteer computing for bioinformatics,” in International Conference BOINC:FAST 2015, ed. E. Ivashko (Petrozavodsk: Karelian Research Centre of Russian Academy of Sciences).
Bailey, T. L., Boden, M., Buske, F. A., Frith, M., Grant, C. E., Clementi, L., et al. (2009). MEME SUITE: tools for motif discovery and searching. Nucleic Acids Res. 37, W202–W208. doi: 10.1093/nar/gkp335
Bobeica, N., Poni, S., Hilbert, G., Renaud, C., Gomès, E., Delrot, S., et al. (2015). Differential responses of sugar, organic acids and anthocyanins to source-sink modulation in cabernet sauvignon and sangiovese grapevines. Front. Plant Sci. 6:382. doi: 10.3389/fpls.2015.00382
Castagna, A., Csepregi, K., Neugart, S., Zipoli, G., Večeřová, K., Jakab, G., et al. (2017). Environmental plasticity of Pinot noir grapevine leaves; a trans-European study of morphological and biochemical changes along a 1500 km latitudinal climatic gradient. Plant. Cell Environ. 40, 2790–2805. doi: 10.1111/pce.13054
Cavallini, E., Matus, J. T., Finezzo, L., Zenoni, S., Loyola, R., Guzzo, F., et al. (2015). The phenylpropanoid pathway is controlled at different branches by a set of R2R3-MYB C2 repressors in grapevine. Plant Physiol. 167, 1448–1470. doi: 10.1104/pp.114.256172
Cutanda-Perez, M.-C., Ageorges, A., Gomez, C., Vialet, S., Terrier, N., Romieu, C., et al. (2009). Ectopic expression of VlmybA1 in grapevine activates a narrow set of genes involved in anthocyanin synthesis and transport. Plant Mol. Biol. 69, 633–648. doi: 10.1007/s11103-008-9446-x
Dwork, C., Kumar, R., Naor, M., and Sivakumar, D. (2001). “). Rank aggregation methods for the Web,” in Proceedings of the 10th International Conference on World Wide Web, New York, NY, 613–622. doi: 10.1145/371920.372165
Ford, C. M., Boss, P. K., and Hoj, P. B. (1998). Cloning and characterization of Vitis vinifera UDP-glucose: flavonoid 3-O-glucosyltransferase, a homologue of the enzyme encoded by the maize Bronze-1 locus that may primarily serve to glucosylate anthocyanidins in vivo. J. Biol. Chem. 273, 9224–9233. doi: 10.1074/JBC.273.15.9224
Fujita, Y., Fujita, M., Shinozaki, K., and Yamaguchi-Shinozaki, K. (2011). ABA-mediated transcriptional regulation in response to osmotic stress in plants. J. Plant Res. 124, 509–525. doi: 10.1007/s10265-011-0412-3
Galbiati, M., Matus, J., Francia, P., Rusconi, F., Cañón, P., Medina, C., et al. (2011). The grapevine guard cell-related VvMYB60 transcription factor is involved in the regulation of stomatal activity and is differentially expressed in response to ABA and osmotic stress. BMC Plant Biol. 11:142. doi: 10.1186/1471-2229-11-142
Geròs, H. Ã, Chaves, M. M., Gil, H. M., and Delrot, S. (2015). Grapevine in a Changing Environment: A Molecular and Ecophysiological Perspective. Hoboken, NJ: John Wiley & Sons. doi: 10.1002/9781118735985
Golldack, D., Li, C., Mohan, H., and Probst, N. (2014). Tolerance to drought and salt stress in plants: unraveling the signaling networks. Front. Plant Sci. 5:151. doi: 10.3389/fpls.2014.00151
Gomez, C., Conejero, G., Torregrosa, L., Cheynier, V., Terrier, N., and Ageorges, A. (2011). In vivo grapevine anthocyanin transport involves vesicle-mediated trafficking and the contribution of anthoMATE transporters and GST. Plant J. 67, 960–970. doi: 10.1111/j.1365-313X.2011.04648.x
Gomez, C., Terrier, N., Torregrosa, L., Vialet, S., Fournier-Level, A., Verriès, C., et al. (2009). Grapevine MATE-type proteins act as vacuolar H + -dependent acylated anthocyanin transporters. Plant Physiol. 150, 402–415. doi: 10.1104/pp.109.135624
Grimplet, J., Van Hemert, J., Carbonell-Bejerano, P., Díaz-Riquelme, J., Dickerson, J., Fennell, A., et al. (2012). Comparative analysis of grapevine whole-genome gene predictions, functional annotation, categorization and integration of the predicted gene sequences. BMC Res. Notes 5:213. doi: 10.1186/1756-0500-5-213
Hichri, I., Heppel, S. C., Pillet, J., Léon, C., Czemmel, S., Delrot, S., et al. (2010). The basic helix-loop-helix transcription factor MYC1 is involved in the regulation of the flavonoid biosynthesis pathway in grapevine. Mol. Plant 3, 509–523. doi: 10.1093/MP/SSP118
Höll, J., Vannozzi, A., Czemmel, S., D’Onofrio, C., Walker, A. R., Rausch, T., et al. (2013). The R2R3-MYB transcription factors MYB14 and MYB15 regulate stilbene biosynthesis in Vitis vinifera. Plant Cell 25, 4135–4149. doi: 10.1105/tpc.113.117127
Kobayashi, S., Ishimaru, M., Hiraoka, K., and Honda, C. (2002). Myb -related genes of the Kyoho grape (Vitis labruscana ) regulate anthocyanin biosynthesis. Planta 215, 924–933. doi: 10.1007/s00425-002-0830-5
Leida, C., Dal Rì, A., Dalla Costa, L., Gómez, M. D., Pompili, V., Sonego, P., et al. (2016). Insights into the role of the berry-specific ethylene responsive factor VviERF045. Front. Plant Sci. 7:1793. doi: 10.3389/fpls.2016.01793
Licausi, F., Giorgi, F. M., Zenoni, S., Osti, F., Pezzotti, M., and Perata, P. (2010). Genomic and transcriptomic analysis of the AP2/ERF superfamily in Vitis vinifera. BMC Genomics 11:719. doi: 10.1186/1471-2164-11-719
Lim, C. W., Yang, S. H., Shin, K. H., Lee, S. C., and Kim, S. H. (2015). The AtLRK10L1.2, Arabidopsis ortholog of wheat LRK10, is involved in ABA-mediated signaling and drought resistance. Plant Cell Rep. 34, 447–455. doi: 10.1007/s00299-014-1724-2
Liu, J., Chen, N., Chen, F., Cai, B., Dal Santo, S., Tornielli, G. B., et al. (2014). Genome-wide analysis and expression profile of the bZIP transcription factor gene family in grapevine (Vitis vinifera). BMC Genomics 15:281. doi: 10.1186/1471-2164-15-281
Ma, S., Gong, Q., and Bohnert, H. J. (2007). An Arabidopsis gene network based on the graphical Gaussian model. Genome Res. 17, 1614–1625. doi: 10.1101/gr.6911207
Margolin, A. A., Nemenman, I., Basso, K., Wiggins, C., Stolovitzky, G., Favera, R. D., et al. (2006). ARACNE: an algorithm for the reconstruction of gene regulatory networks in a mammalian cellular context. BMC Bioinformatics 7:1471–2105. doi: 10.1186/1471-2105-7-S1-S7
Mattivi, F., Vrhovsek, U., Malacarne, G., Masuero, D., Zulini, L., Stefanini, M., et al. (2011). Profiling of resveratrol oligomers, important stress metabolites, accumulating in the leaves of hybrid Vitis vinifera (Merzling × Teroldego) genotypes infected with Plasmopara viticola. J. Agric. Food Chem. 59, 5364–5375. doi: 10.1021/jf200771y
Moretto, M., Sonego, P., Pilati, S., Malacarne, G., Costantini, L., Grzeskowiak, L., et al. (2016). VESPUCCI: exploring patterns of gene expression in grapevine. Front. Plant Sci. 7:633. doi: 10.3389/fpls.2016.00633
Mozell, M. R., and Thach, L. (2014). The impact of climate change on the global wine industry: challenges & solutions. Wine Econ. Policy 3, 81–89. doi: 10.1016/J.WEP.2014.08.001
Nicolas, P., Lecourieux, D., Kappel, C., Cluzet, S., Cramer, G., Delrot, S., et al. (2014). The basic leucine zipper transcription factor ABSCISIC ACID RESPONSE ELEMENT-BINDING FACTOR2 is an important transcriptional regulator of abscisic acid-dependent grape berry ripening processes. Plant Physiol. 164, 365–383. doi: 10.1104/pp.113.231977
O’Malley, R. C., Huang, S. C., Song, L., Lewsey, M. G., Bartlett, A., Nery, J. R., et al. (2016). Cistrome and epicistrome features shape the regulatory DNA landscape. Cell 165, 1280–1292. doi: 10.1016/j.cell.2016.04.038
Pilati, S., Bagagli, G., Sonego, P., Moretto, M., Brazzale, D., Castorina, G., et al. (2017). Abscisic acid is a major regulator of grape berry ripening onset: new insights into ABA signaling network. Front. Plant Sci. 8:1093. doi: 10.3389/fpls.2017.01093
Ren, C., Zhang, Z., Wang, Y., Li, S., and Liang, Z. (2016). Genome-wide identification and characterization of the NF-Y gene family in grape (Vitis vinifera L.). BMC Genomics 17:605. doi: 10.1186/s12864-016-2989-3
Shannon, P., Markiel, A., Ozier, O., Baliga, N. S., Wang, J. T., Ramage, D., et al. (2003). Cytoscape: a software environment for integrated models of biomolecular interaction networks. Genome Res. 13, 2498–2504. doi: 10.1101/gr.1239303
Shi, J. X., Malitsky, S., De Oliveira, S., Branigan, C., Franke, R. B., Schreiber, L., et al. (2011). SHINE transcription factors act redundantly to pattern the archetypal surface of Arabidopsis flower organs. PLoS Genet. 7:e1001388. doi: 10.1371/journal.pgen.1001388
Soderman, E., Mattsson, J., and Engstrom, P. (1996). The Arabidopsis homeobox gene ATHB-7 is induced by water deficit and by abscisic acid. Plant J. 10, 375–381. doi: 10.1046/j.1365-313X.1996.10020375.x
Spirtes, P., and Glymour, C. (1991). An algorithm for fast recovery of sparse causal graphs. Soc. Sci. Comput. Rev. 9, 62–72. doi: 10.1177/089443939100900106
Teixeira, A., Eiras-Dias, J., Castellarin, S. D., and Gerós, H. (2013). Berry phenolics of grapevine under challenging environments. Int. J. Mol. Sci. 14, 18711–18739. doi: 10.3390/ijms140918711
Tello, J., Torres-Pérez, R., Grimplet, J., Carbonell-Bejerano, P., Martínez-Zapater, J. M., and Ibáñez, J. (2015). Polymorphisms and minihaplotypes in the VvNAC26 gene associate with berry size variation in grapevine. BMC Plant Biol. 15:253. doi: 10.1186/s12870-015-0622-2
Vannozzi, A., Dry, I. B., Fasoli, M., Zenoni, S., and Lucchin, M. (2012). Genome-wide analysis of the grapevine stilbene synthase multigenic family: genomic organization and expression profiles upon biotic and abiotic stresses. BMC Plant Biol. 12:130. doi: 10.1186/1471-2229-12-130
Vannozzi, A., Wong, D. C. J., Höll, J., Hmmam, I., Matus, J. T., Bogs, J., et al. (2018). Combinatorial regulation of stilbene synthase genes by WRKY and MYB transcription factors in grapevine (Vitis vinifera L.). Plant Cell Physiol. 59, 1043–1059. doi: 10.1093/pcp/pcy045
Walker, A. R., Lee, E., Bogs, J., McDavid, D. A. J., Thomas, M. R., and Robinson, S. P. (2007). White grapes arose through the mutation of two similar and adjacent regulatory genes. Plant J. 49, 772–785. doi: 10.1111/j.1365-313X.2006.02997.x
Wang, M., Vannozzi, A., Wang, G., Liang, Y.-H., Tornielli, G. B., Zenoni, S., et al. (2014). Genome and transcriptome analysis of the grapevine (Vitis vinifera L.) WRKY gene family. Hortic. Res. 1:14016. doi: 10.1038/hortres.2014.16
Wang, N., Zheng, Y., Xin, H., Fang, L., and Li, S. (2013). Comprehensive analysis of NAC domain transcription factor gene family in Vitis vinifera. Plant Cell Rep. 32, 61–75. doi: 10.1007/s00299-012-1340-y
Wong, D. C. J., Lopez Gutierrez, R., Gambetta, G. A., and Castellarin, S. D. (2017). Genome-wide analysis of cis-regulatory element structure and discovery of motif-driven gene co-expression networks in grapevine. DNA Res. 24, 311–326. doi: 10.1093/dnares/dsw061
Wong, D. C. J., Schlechter, R., Vannozzi, A., Höll, J., Hmmam, I., Bogs, J., et al. (2016). A systems-oriented analysis of the grapevine R2R3-MYB transcription factor family uncovers new insights into the regulation of stilbene accumulation. DNA Res. 23, 451–466. doi: 10.1093/dnares/dsw028
Wong, D. C. J., Sweetman, C., Drew, D. P., and Ford, C. M. (2013). VTCdb: a gene co-expression database for the crop species Vitis vinifera (grapevine). BMC Genomics 14:882. doi: 10.1186/1471-2164-14-882
Keywords: gene network, NES2RA, Vitis vinifera, climate change, abscisic acid ABA, flavonoids, stilbenoids, ERF
Citation: Malacarne G, Pilati S, Valentini S, Asnicar F, Moretto M, Sonego P, Masera L, Cavecchia V, Blanzieri E and Moser C (2018) Discovering Causal Relationships in Grapevine Expression Data to Expand Gene Networks. A Case Study: Four Networks Related to Climate Change. Front. Plant Sci. 9:1385. doi: 10.3389/fpls.2018.01385
Received: 17 February 2018; Accepted: 31 August 2018;
Published: 21 September 2018.
Edited by:
Laurent Deluc, Oregon State University, United StatesReviewed by:
Atsushi Fukushima, RIKEN, JapanJosé Tomás Matus, Centre for Research in Agricultural Genomics (CRAG), Spain
Copyright © 2018 Malacarne, Pilati, Valentini, Asnicar, Moretto, Sonego, Masera, Cavecchia, Blanzieri and Moser. This is an open-access article distributed under the terms of the Creative Commons Attribution License (CC BY). The use, distribution or reproduction in other forums is permitted, provided the original author(s) and the copyright owner(s) are credited and that the original publication in this journal is cited, in accordance with accepted academic practice. No use, distribution or reproduction is permitted which does not comply with these terms.
*Correspondence: Claudio Moser, claudio.moser@fmach.it
†These authors have contributed equally to this work