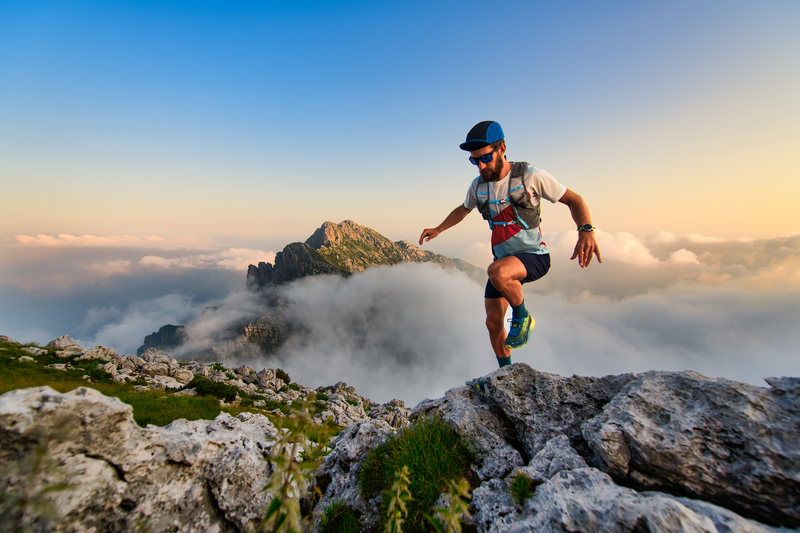
95% of researchers rate our articles as excellent or good
Learn more about the work of our research integrity team to safeguard the quality of each article we publish.
Find out more
HYPOTHESIS AND THEORY article
Front. Plant Sci. , 21 November 2017
Sec. Computational Genomics
Volume 8 - 2017 | https://doi.org/10.3389/fpls.2017.01943
Abiotic stress is one of the major factors responsible for huge yield loss in crop plants. MicroRNAs play a key role in adaptive responses of plants under abiotic stress conditions through post-transcriptional gene regulations. In present study, 95 potential miRNAs were predicted in Brassica juncea using comparative genomics approach. It was noted that these miRNAs, target several transcription factors (TFs), transporter family proteins, signaling related genes, and protease encoding genes. Nineteen distinct miRNA-target regulatory networks were observed with significant involvement in regulation of transcription, response to stimulus, hormone and auxin mediated signaling pathway related gene ontology (GO) term. The sucrose-starch metabolism and pentose-gluconate interconversion pathways were found significantly enriched for these target genes. Molecular markers such as Simple Sequence Repeats (SSR) and Single Nucleotide Polymorphism (SNPs) were identified on miRNAs (miR-SSRs and miR-SNPs) and their target genes in B. juncea. Notably, one of the miR-SNP (C/T) was found at the 5th position on mature region of miR2926. This C/T transition led to the distorted and unstable hairpin structure of miR2926, consequently complete loss of target function. Hence, findings from this study will lay a foundation for marker assisted breeding for abiotic stress tolerant varieties of B. juncea.
Brassica belongs to Brassicaceae family is important sources of edible oil, vegetables, and condiments across the globe. Among various Brassica species, Brassica juncea is a major oilseed crop in South Asia (Woods et al., 1991). In India, B. juncea is grown on ~6–7 million hectares of land during the winter season under rain-fed cropping system. During various developmental stages of growth crops are exposed to several abiotic stresses such as salinity, drought, and high temperature (Zhang et al., 2014). These abiotic stresses often cause adverse effects on plant growth and development leading to substantial yield penalties (Dolferus et al., 2011).
To cope with such environmental conditions, plants have evolved with several physiological and molecular mechanisms to respond and adapt to adverse conditions. These adaptive mechanisms in plants causes altered gene expression, wherein arrays of regulatory elements are intricately involved. microRNAs (miRNAs) are key regulatory elements in various cellular responses under stress conditions (Sanan-Mishra et al., 2009; Singh and Shah, 2014). MiRNAs regulate gene expressions at post-transcriptional level by suppression of the target mRNA translation or direct cleavage (Bej and Basak, 2014). Various stress-regulated miRNAs have been identified and characterized in plants (Ramesh et al., 2015; Zhang, 2015; Ohama et al., 2016), whereas only limited reports are available in B. juncea. (Kanwar et al., 2012; Srivastava et al., 2013; Bhardwaj et al., 2014).
Additionally, recent evidences suggest that presence of SSRs and SNPs markers on miRNAs have potential role in the expression of quantitative traits (Ganie and Mondal, 2015). Polymorphisms as SSR or SNP on mature miRNA, may lead to disruption of interaction between putative target gene and miRNA (Ferrão et al., 2015). A line of evidence shows that SNPs are important functional sequence variations that occur at miRNA loci may have profound effects during the evolution of a species (Ehrenreich and Purugganan, 2008; Guo et al., 2008). Consequently, the expression patterns of genes could be significantly affected, sometimes leading to the appearance of transgressive phenotypes in plants (Meng et al., 2011; Liu et al., 2013; Formey et al., 2014). Therefore, identification of miRNAs and their predicted target genes together with SSRs and SNPs would be useful for designing more comprehensive studies on evaluation of the effectiveness of these markers in plant breeding.
Over the years, different approaches have been adapted to identify potential miRNAs in plants. The conventional approaches largely involve cloning of size-fractionated RNAs followed by Sanger sequencing and validation through experimentation (Chaudhuri and Chatterjee, 2007). However, by the virtue of highly conserved nature and similar secondary structure of the majority of miRNAs, their new homologs can be easily predicted in species of interest with the help of proper miRNA prediction algorithms and computational tools. In present study, we identified potential miRNA homologs and their target genes in B. juncea using available transcriptome data, ESTs and genomic sequences (GSS) following a conceptual pipeline (Figure 1). Functional classification and pathway enrichment analysis of the miRNA targeted genes was performed to understand the biological function of the predicted miRNAs. In addition, we examined the occurrence and distribution of potential SSRs and SNPs in the predicted miRNAs; miR-markers (SSRs/SNPs), and their target genes for their possible applications in marker-assisted breeding in B. juncea.
Figure 1. Schematic representation of the study design for miRNA, their target prediction, and miR marker identification.
Transcriptome contig sequences (GSE73201 and GSE66389), ESTs (5656), and GSS (33) of B. juncea were retrieved from NCBI (www.ncbi.nlm.nih.gov). These EST sequences were pre-processed by EST-trimmer (http://pgrc.ipk-gatersleben.de/misa/download/) and polyA-tails were removed. It insures that no stretches of (T)5 or (A)5 were present on 5′ or 3′ ends of trimmed sequences in a range of 50 bp. To exclude low quality sequences, ESTs with less than 100 bp and more than 800 bp were discarded and clipped at 3′ end (Thiel et al., 2003). The processed and filtered sequences were then assembled using CAP3 (Huang and Madan, 1999) program. Transcriptome data encompassing 2,148,658 and 933,376 transcript sequences respectively from GSE73201and GSE66389, 799 assembled ESTs, 2774 ESTs singleton from EST and GSS were further subjected in miRNA prediction pipeline.
All available plant miRNAs and their precursor sequences were retrieved from miRBase v 20.0 repository; a database of published miRNA (Griffiths-Jones, 2004). Non-redundant set of miRNA sequences were used for BLASTn (mismatch < 1, with no gap and E-Value 0.01) homology search against total transcriptome contigs, assembled EST contigs, singleton and GSS sequences. Further BLASTx was performed against non-redundant (NR) protein database to remove protein-coding sequences (Altschul et al., 1997).
The secondary structures of non-redundant miRNA sequences were generated using Zuker folding algorithm implemented in Mfold (http://mfold.rna.albany.edu/?q$=$mfold/download-mfold/) with default parameters (Zuker, 2003). Predicted miRNA secondary structure should have higher Minimal Free Energy Index (MFEI) folding and negative Minimal Folding Free Energy (MFE) to differentiate it from other small RNAs. Sequences satisfying Meyers rules were considered as potential miRNA (Meyers et al., 2008). The predicted mature miRNAs had no more than one nucleotide substitutions compared with a known reference mature miRNAs, (i) it must fold into proper stem-loop hairpin structures, (ii) it should be present on one of the arm of hairpin, (iii) no more than four mismatch in predicted miRNA and the miRNA* (on opposite arm of mature region), (iv) it must not contain more than one bulges, (v) the size of bulges are not more than two, (vi) the predicted secondary structure must contain high negative MFE and high MFEI values.
In order to understand the biological functions of miRNAs, putative target genes were identified by using psRNA Target server (http://plantgrn.noble.org/psRNATarget/) against available B. juncea transcript sequences and B. rapa unigenes, which is close to juncea (as it contains AA genome) (Mun et al., 2009). Functional annotations of target genes were retrieved through BRAD Database (http://brassicadb.org/brad/) (Cheng et al., 2011). Based on UPE (unpaired energy) energy and inhibition process, miRNA-target network were built among miRNA, and their target genes using cytoscape software (Shannon et al., 2003). Gene Ontology enrichment analysis was performed using agriGO (Du et al., 2010) by Singular Enrichment Analysis (SEA) with hypergeometric statistical test method and Bonferroni multi-test adjustment method (significance level < 0.05).
Simple sequence repeats (SSR) mining was performed using MISA (microsatellite search module) with 2–6 bp repeats as described by Thiel et al. (2003).
Putative SNP/indels were extracted by using AutoSNP pipeline for SNP discovery (Barker et al., 2003). The programme first makes a cluster of sequences followed by contigs formation of highly homologous sequences. Each of the contig was analyzed for presence of SNP sites. For identification of miR-SNP, precursor miRNA sequences were subjected for SNPs detection with low E-value and occurrence in more than five sequences. SNPs on target genes were identified by comparing it with reported SNPs from B. juncea (AABB) (Paritosh et al., 2014) and B. napus (AACC) (Trick et al., 2009). Further, we utilized Protein variation effect analyser (PROVEAN) (Choi and Chan, 2015) and PANTHER (Thomas et al., 2003) tool to predict the change in the biological functions of a target protein due to an amino acid substitution or indel based on the sequence clustering and alignment-based scoring. In PROVEAN, variants with scores less than −2.5 were considered deleterious and greater than −2.5 as a neutral variant (Choi and Chan, 2015).
Homology search against miRBase as well as a series of manual inspection, evaluation, and filtration processes was performed. Total of 95 unique potential miRNAs (miR1114, miR156h, miR2926, miR3434-3p, miR408, miR5015, miR5021, miR536f, miR5658, miR854a-e), sequences belonging to 32 miR-families were identified in B. juncea (Table S1A). All miRNA sequences were 19–24 nt long, with Watson-Crick or G/U base pairing and stable minimal folding free energy (MFE). Precursor sequences of predicted miRNA from ESTs were mapped to B. juncea genome and transcriptome to validate they are real miRNAs (Table S1B).
Target gene prediction is a key step for determining biological function of miRNAs. A total of 906 unique potential target genes were predicted for identified miRNAs using B. juncea transcript sequences and B. rapa unigenes (Tables S2A–C). Many of the identified potential miRNA targets were member of several transcription factor (TFs) family, signaling related genes and growth regulating factors. Especially, miR156, miR171 miR5021, miR2926, miR393, miR164, miR408, and miR854 displayed a striking inclination to TFs target genes (Table S2).
Widely studied miR156 predicted to target many squamosa promoter binding protein-like (SBP domain) TF, ATP binding cassette subfamily, F-box family protein, F-box/RNI-like/FBD-like domains-containing proteins. MiR160 family was predicted to target auxin response factors (10, 16, and 17). Similarly miR393 targeted auxin signaling F-box (2 and 3) and targets of miR5021 were WRKY, CPK2 (calmodulin-domain protein kinase), ARR2 (Arabidopsis response regulator 2), MYB101, heat shock protein binding, TIR1, and NAC. MiR408 targeted 22 genes, including chloroplast heat shock protein 70-1, ASPARTATE KINASE 3, F-box family protein, and SWAP (Suppressor-of-White-Apricot) (Table S2).
MiR2926 targeted ATP binding, kinase, ATGWD3, elongation factor 1-beta/EF-1-beta, APUM5 (Arabidopsis Pumilio 5), peroxisomal membrane protein (PMP36), DNAJ heat shock family protein, zinc finger, and sterile alpha motif (SAM). The miR5015 received four target genes such as, SPX (SYG1/Pho81/XPR1), zinc finger (C3HC4-type RING finger) protein-related, PGIP1 (Polygalacturonase Inhibiting Protein 1), and PHD finger family protein, PHYC (phytochrome defective c) protein histidine kinase. All these miRNA-target annotation showed their involvement in various biological processes such as TF mediated and/or signaling related pathways.
The miRNA-target genes ontology (GO) enrichment showed their association to signal transduction and several cellular and metabolic processes. We observed the regulation of transcription, developmental process, nitrogen compound metabolic process, macromolecule biosynthetic, hormone stimulus, and auxin mediated signaling pathway related biological processes GO term with significant enrichment (Figure 2). Moreover, transcription regulator activity binding and DNA binding were noted to be significantly enriched molecular function GO categories. Enrichment of nucleus, intracellular membrane-bounded organelle, integral to membrane, and intrinsic to membrane were observed with significance cellular component GO terms (Figures S1A–C).
Figure 2. Snapshot of significantly enriched biological process Gene Ontology terms for predicted target genes of miRNA in B. juncea.
Pathway analysis of miRNA target genes showed significantly enriched pathways under environmental information processing such as signal transduction, carbohydrate metabolism, starch and sucrose metabolism and pentose and glucuronate inter-conversions pathways (Figures S2A,B). Exploring the results further showed, target of miR396, miR5658, miR854e, miR854a miR408, miR5021 were involved in starch and sugar metabolism. While miR408 and miR5021 regulated targets genes annotated as glycosyl hydrolase family protein were also involved in pentose and gluconate inter-conversion pathway. SPL target predicted for miR156 were found involved in plant hormone signal transduction pathways.
SSR motifs on 95 pre-miRNA sequences were shown three type of SSR motifs were co-localized on 10 pre-miRNAs (Table S3A). These SSRs on 10 pre-miRNAs were from five miR families such as miR824, miR396, miR5021, miR156, and miR5015. Total of six SSR motifs were overlapped on mature miRNA region. Most of the SSR motifs were trinucleotide with frequent CTT and one of the C* from miR156h (Figure S3A).
Mining of SSR motifs on target genes showed 700 SSR motifs in 621 target genes with maximum frequency of trinucleotide repeats (Tables S3B,C). Results depicted that 4 mononucleotide repeats, 5 dinucleotide repeats, 656 trinucleotide repeats, 12 tetranucleotide repeats, 1 pentanucleotide repeats, and 22 nucleotide repeats Figure S3B were present on targets. It is observed that 17 of the miRNA target sequences have more than one SSR motifs. Total of 14 compound SSR (C* and C) motifs were identified from miRNA target genes.
Reliable SNPs were retrieved on 95 pre-miRNAs and mature miRNA using integrated AutoSNP pipeline with ESTs mapped from more than one genotype (Barker et al., 2003). Total 34 SNPs were found, with greatest number of transition i.e., 14 (46.47%) (Table S4A). Indels were localized on three precursor sequences of miR854, miR824, and miR171 (Table S4A). The SNPs identified in miR854 were located on flanking to the downstream of mature region. However, SNPs on miR2926 were located on the precursor as well as mature region. MiR-SNP (snpID: rs54273) with transition (C/T) type at 5th from 5′ end of mature region of pre-miR2926 were noted (Figure 3A).
Figure 3. (A) miR-SNP (C-to-T transition at 5th position) on mature region of miRNA-2926 in B. juncea. (B) Secondary structure of miRNA-2926 without SNP, ΔG = −178.90 kcal/mol. (C) Secondary structure of miRNA-2926 with miR-SNP (C/T) (ΔG = −174.10 kcal/mol). The blue circle shows SNP position.
Further, effect of miR-SNP was evaluated on modified structure of miR2926 due to base transition (C to T) at 5th position (Figure 3B). Evaluating the possible impact of SNP on pre-miRNA secondary structure, ΔG, and MFEI were also calculated. Loss of stability has been observed with increased ΔG from −178.90 to −174.10 as well MFEI from −0.5 to −0.476 of the secondary structure with this base transition from C to T. Figure 3C clearly shows the structural variation due to the transition i.e., loss of miRNA-miRNA* duplex. This exercise demonstrated that presence of SNP on miR2926 destabilized pre-miRNA structure. This consequently affects their target genes. Hence, with the aim to better understand the alteration in target spectrum due to SNP, putative targets of miRNA with transitioned nucleotide (C to T) were searched using psRNA Target tool. Notably, no target genes were found for miR2926 with miR-SNP; reflecting as complete loss of function of target genes. These results justifying our in-silico finding of distorted secondary structure.
To identify SNPs on target genes of our predicted miRNAs in B. juncea (AABB), they were mapped to the already observed genes with SNPs in B. juncea (AABB) (Paritosh et al., 2014) and B. napus (AACC) (Trick et al., 2009). Total of 100 targets (79 matched Ids with B. napus and 21 with B. juncea) were found having SNPs, where C-to-T substitution with a greatest frequency (Tables S4B,C). These targets with SNPs mapped have been found to be regulated by miR854 (mostly), miR171, miR408, miR156, miR2926, miR5658, miR5015, miR3434, and miR5021. The functional annotation of 100 target genes with SNPs revealed association to signal-enzymes, followed by TF family genes that known to play important role in abiotic stress condition (Figure 4). Further, deleterious consequences of SNPs found on target genes from B. juncea were investigated and found 13 synonymous and 5 non-synonymous SNPs. Notably, two SNPs on Bra039026 (V208G) and Bra020200 (S330Y) were predicted having deleterious effect with PROVAEN score −6.6 and −3.3, respectively and also “probably damaging” and “probably benign” respectively from PANTHER.
Figure 4. Functional classification of miRNA-target genes with identified SNPs. Most of the genes belong to signaling enzymes such as KCS20, CYP94D2, and FAD (22%).
In order to explore the relationship among the miRNAs and their targets, miRNA-mediated regulatory networks were constructed. Nineteen independent networks showing multiplicity behavior of miRNA i.e., one miRNA can target more than one genes (Figure 5). Exploring these results revealed miR156 sharing target genes with miR157 and miR529. MiR854 regulatory network connected with miR5658 network with common target genes (Bra006283, Bra011043, Bra030266, Bra034179, Bra005011, and Bra001582) involved in transmembrane transport and zinc ion binding. Common target genes (Bra027592, Bra035905, Bra040355, Bra020820, Bra001710, and Bra014818) with functions as aspartic-type endopeptidase activity, lipid glycosylation, and proteolysis for miR5658 and miR5021. It indicates the co-operative behavior of these target genes, where one gene can be controlled by more than one miRNA (John et al., 2004). Many other miR-networks were found to be independent to each other, where one miRNA regulate multiple targets showing their multiplicity behavior. Further, mapping of the target genes reported bearing SNPs on the network was done. Interestingly, network showed maximum of SNPs on miR854 regulated target genes (green circle nodes on network in Figure 5), followed by miR5021 and miR5658 reported in B. juncea (Paritosh et al., 2014).
Figure 5. MiRNA-target genes regulatory network identified in B. juncea. Red triangle nodes in network represents miRNAs. Nodes in diamond shape denote target genes having SNP mapped from B. juncea (green nodes) and B. napus (blue nodes).
Computational prediction of miRNAs using transcriptome, EST, and GSS sequences is rather a prompt and cost effective approach to identify potential miRNAs (Frazier et al., 2010; Kim et al., 2011; Katiyar et al., 2012; Srivastava et al., 2013; Bhardwaj et al., 2015). In current study, 95 potential mature miRNAs in B. juncea, utilizing comparative genomics-based approach were predicted. A notable aspect of the present study was addition of few new B. juncea miRNAs sequences variants for miR396, miR408, miR160, and miR166 as compared to the results mentioned in previous studies (Yang et al., 2013; Bhardwaj et al., 2014) (Table S5).
The complementarities between the miRNA and their target genes provided the hierarchy of biological process regulation. The predicted miRNA targets genes coding of proteins such as, TFs, transporters, defense, and stress responsive signaling pathways. The expression of important TF families like MYB, BHLH, and zinc finger in relation to miRNAs has been reported to be involved in hormonal homeostasis (Sorin et al., 2005; Siemens et al., 2006). We compared the expression of target genes using Arabidopsis homologs with transcriptome data available for B. juncea (Table S6) (Sharma et al., 2015; Sinha et al., 2015; Srivastava et al., 2015). We observed genes encode nuclear response regulator that acts as a negative regulator in cytokinin-mediated signal transduction were down regulated under salinity stress (Table S6A). Whereas, number of target genes were observed down regulated under arsenic stress (Table S6B). This may indicate the upregulation of upstream miRNAs under these stress condition.
The target genes of miR156 such as CAT, ABCB, CBS, and SPL showed their involvement in stress response related biological processes. SPL has been studied in response to stress and temporal expression changes during leaf development in B. juncea and other plants (Wang et al., 2009; Xie et al., 2012; Stief et al., 2014). Other target genes such as ABCB, CAT5, and CBS reported to regulate signaling pathways under stress conditions (Kushwaha et al., 2009). Role of miR156h has been reported to be involved in hormonal homeostasis, abiotic stress responses, tissue development and cytoplasmic male-sterility in B. juncea as well as other plants (Achard et al., 2004; Sunkar and Zhu, 2004). It is reported that miR156 also expressed during club root formation in canola (Verma et al., 2014) and turnip mosaic virus infection in Arabidopsis (Kasschau et al., 2003; Navarro et al., 2008) Overexpression of miR156b and miR156h resulted in dwarfism as well as reduced panicle size in rice (Chen et al., 2015). These findings are obvious true as phytohormones functions to coordinate plant growth and development as well as for mitigating the effect of stress as studied in B. juncea and several others (Hasanuzzaman et al., 2013; Wilson et al., 2014).
MiR854 target networks are mostly involved in signaling pathways, which is in consistent with previous study. Srivastava et al. reported high expression of miR854 in response to arsenic stress in roots (Srivastava et al., 2013) and low expression under jasmonic acid supplementation (Srivastava et al., 2013) in B. juncea. They described that under arsenic stress, the altered expression of miR854, led to the interplay of JA, IAA, and miRNAs, where suppressing its expression lead to the increased expression of its target genes in B. juncea. Notably, LSD1 was reported to be target gene of miR854, functions as marker for JA signaling, which acts as antagonistic transcriptional regulators to control attenuation of cell death through regulation of ROS (reactive oxygen species) (Tamaoki et al., 2008).
Most of the targets of miR5021 such as WRKY, MYB, and ARR2 have regulatory function under stress condition. Previous studies have shown MYB protein was targeted by miR5021 in Catharanthus roseus (Pani and Mahapatra, 2013) and it has proven role in abiotic and boron stress response (Katiyar et al., 2012; Ozhuner et al., 2013; Smita et al., 2015). Further, miR1114 has been studied for their role during seed development in B. napus (Huang et al., 2013) and their target genes such as kinase and F-box have studied to play role in seed development in B. juncea and B. napus (Fei et al., 2007; Mandal et al., 2011). The target genes of miR5015 such as leucine zipper, HSF, PGIP1 etc. were also observed in carrot, which are having significant role in response to abiotic stress and in defense response (Berger et al., 2000; Barozai et al., 2013).
The pathway enrichment analysis of target genes showed starch—sucrose metabolism and pentose-glucuronate inter-conversions pathways with highest significance.
These targets were majorly annotated as glycosyl hydrolase family protein and targeted by five of the miRNA (miR5658, miR854e, miR854a, miR408, and miR5021) (Figure 6). These pathways are part of carbohydrate metabolism, which is an important biochemical processes in plant growth and stress tolerance (Zhou et al., 2010). Previous study showed that the coding protein β-fructofuranosidase target genes down-regulated by miR408, takes part in starch and sucrose metabolism in rice (Zhou et al., 2010). Additionally, miR3434 have been reported for its role in starch metabolism in Arabidopsis leaf (Ingkasuwan et al., 2012). Consistent to our findings discussed above it may be noted that these miRNAs targeted genes and their regulatory cascade showing their significant involvement in starch and sucrose metabolism pathway during abiotic stress response.
Figure 6. Relationship of miRNAs and target genes in starch-sucrose pathway and pentose and glucuronate interconversions in B. juncea. Nodes in red colors are miRNAs; rectangular boxes are pathways and in purple are significantly enriched target genes. Nodes in gray color are with “glycosyl related terms.”
Heat stress at seedling stage is crucial in India, whereas, it is a major problem during flowering stage in major mustard growing countries such as China, Australia, Canada (Angadi et al., 2000; Sharma and Sardana, 2013). Therefore, heat stress in B. juncea has been a major problem especially in Asian countries.
MiR408 and their target genes such as heat shock protein 70-1 and ASPARTATE KINASE etc. were well studied in response to heat stress in checkpea and rice (Mutum et al., 2013; Hajyzadeh et al., 2015). These results are also consistent with previous study in wheat and Populus euphratica, where, it was observed that miR408 have differential expression in response to heat stress also (Ozhuner et al., 2013). These results indicated that heat stress drive change in expression of many TFs and kinases which serve as key components of signal transduction pathways (Bhardwaj et al., 2015). Also, miR408 were identified and checked for their response in zinc deficiency in B. juncea roots (Shi et al., 2013) playing important role in heat stress. Most of the targets of miR2926 such as APUM5, DNAJ, zinc finger, TPR, and elongation factor were reported to be involved in response to heat stress (Bekh-Ochir et al., 2013; Bogamuwa and Jang, 2014; Huh and Paek, 2014) (Figure 7). Interestingly, miR2926 has been studied in response to heat stress in Saccharina japonica (Liu et al., 2015). In general, on the basis of earlier functional analysis we hypothesized, that miRNA target networks consist of miR408 and miR2926 were predominantly related to heat stress responses.
Figure 7. Network consists of miR2926 and their target genes. Nodes in orange color are known to be involved in heat stress. Nodes in diamond with blue color denotes that target genes having SNP mapped from B. napus. Edges in pink and green color, respectively showing translation and cleavage type of inhibition.
It has been reported that markers present in UTRs and introns can modulate gene expression (Li et al., 2002). Therefore, in the present study co-localization of SSR and SNP markers were discovered on the precursor as well mature region of identified miRNAs (miR-SSR and miR-SNPs) in B. juncea. We observed, miR5021, miR5015, and miR156h were found with trinucleotide SSR repeats in highest frequency. Therefore, these miRNAs with traits (abiotic stress) specific SSRs may be utilized further in marker assisted breeding program in B. juncea. In a recent study, trait-specific SSRs were identified showing salt response in rice (Ganie and Mondal, 2015). Although, the development of EST-SSR markers was reported in plant species and Brassica genotype for marker assisted breeding (Hopkins et al., 2007; Gupta et al., 2014). But limited information is available till date about the miR-SSR in B. juncea, despite it is being a major oilseed crop (Ganie and Mondal, 2015). A preliminary analysis to verify the presence of SSR motifs in primary-miRNAs of black pepper was reported (Joy and Soniya, 2012). A very good attempt was taken by a group for analyzing SSR motifs in 8619 premature-miRNAs from 87 species, mostly were from 3 to 4 bp repeats and suggested SSRs are an important component of pre-miRNAs (Chen et al., 2010). These results can be further utilized for marker assisted breeding programme, as it directly or indirectly affect the expression of miRNA and subsequently its target expression (Li et al., 2002).
Additionally, functional SNPs can cause apparent phenotype changes or trait variations (Shastry, 2009), which mainly dispersed in sequence regions with less conservation, such as intronic and intergenic regions, but fewer in functional regions, such as coding sequences (CDSs) and regulatory elements (Castle, 2011). In current study, high frequency of C/T transition noted in consistent with previous study (Low et al., 2014; Rawlings-Goss et al., 2014) (Table S4A). It has been also reported that, C/T transition at the target site enhances the repression of TGFBR2 by hsa-miR-34b* (Ahluwalia et al., 2012). We identified two SNPs with deleterious effect on genes encoding methyltransferase and phosphoglycerate mutase targeted by miR3434 and miR5658. Methyltransferases such as HEN1 has been studied to involve in plant miRNA biogenesis (Tkaczuk et al., 2006; Baranauske et al., 2015). Phosphoglycerate mutase studied to have critical roles in vegetative growth in Arabidopsis thaliana (Zhao and Assmann, 2011). One SNP on mature region of miR2926 that de-stabilizes the secondary structure and consequently the complete loss of function (Figure 3). Our results showed miR2926 role in heat stress, in consistent with Liu et al. reported the role of miR2926 in response to heat stress in S. Japonica (Liu et al., 2015). Therefore, identified SNP marker on mature miR2926 region is a researchable area and seeks further validation to achieve trait specific goal. These SNPs can be utilized further for fine mapping of important agronomic traits and will shed light on the diversification of Brassica species.
Identified miRNAs and their target genes are potentially involved in abiotic stress response, developmental stages and sugar-starch metabolic pathway in B. juncea. The functional regulatory networks of miRNAs provided insight into the details of particular miRNA functional comprehensions. Conclusively, this modular dissection and analysis unravel several functional and their molecular decoding. Co-localization of miR markers (miR-SSR and miR-SNP) on mature miRNA and their target genes associated to abiotic stress in B. juncea revealed their utility for trait specific marker assisted breeding program. Occurrence of SNP at mature miR2926 had changed the stability of miRNAs secondary structures as well as the miRNA-target interactions. Thereby affecting the miRNAs biogenesis and causing the complete loss of function. Overall, the finding from present study will contribute to further deepen understanding of the effect of markers on the evolution of miRNAs in B. juncea. This will lead to abiotic stress varietal development through marker assisted breeding and mitigation of faster changing climatic conditions.
IS and SS initiated the research, performed the downstream analyses, interpreted the results and drafted the manuscript. IS, SS, SK, DM and AR interpreted the results. SK, BS, and DM conceived the idea of the study and drafting the manuscript. All authors have read and approved the manuscript for publication.
The authors declare that the research was conducted in the absence of any commercial or financial relationships that could be construed as a potential conflict of interest.
Authors acknowledge Project “National project on agricultural bioinformatics and computational biology,” Indian Agricultural Statistics Research Institute, PUSA, New Delhi for providing all facility to conduct this study.
The Supplementary Material for this article can be found online at: https://www.frontiersin.org/articles/10.3389/fpls.2017.01943/full#supplementary-material
Figure S1. Gene ontology (A) biological process, (B) cellular component, (C) molecular function enrichment analysis of target genes for predicted miRNA in B. juncea. Darker the color more significant is the term.
Figure S2. Enriched pathway (A) starch and sucrose metabolism, (B) pentose and glucuronate interconversions for target genes for predicted miRNA in B. juncea.
Figure S3. Distribution of simple sequence Repeats (SSRs) on (A) miRNA precursor sequences and (B) target genes in B. juncea.
Achard, P., Herr, A., Baulcombe, D. C., and Harberd, N. P. (2004). Modulation of floral development by a gibberellin-regulated microRNA. Development 131, 3357–3365. doi: 10.1242/dev.01206
Ahluwalia, J. K., Soni, K., Sivasubbu, S., and Brahmachari, V. (2012). Modeling SNP mediated differential targeting of homologous 3′UTR by microRNA. RNA Biol. 9, 351–360. doi: 10.4161/rna.19318
Altschul, S. F., Madden, T. L., Schaffer, A. A., Zhang, J., Zhang, Z., Miller, W., et al. (1997). Gapped BLAST and PSI-BLAST: a new generation of protein database search programs. Nucleic Acids Res. 25, 3389–3402. doi: 10.1093/nar/25.17.3389
Angadi, S. V., Cutforth, H. W., Miller, P. R., McConkey, B. G., Entz, M. H., Brandt, S. A., et al. (2000). Response of three Brassica species to high temperature stress during reproductive growth. Can. J. Plant Sci. 80, 693–701. doi: 10.4141/P99-152
Baranauske, S., Mickute, M., Plotnikova, A., Finke, A., Venclovas, C., Klimašauskas, S., et al. (2015). Functional mapping of the plant small RNA methyltransferase: HEN1 physically interacts with HYL1 and DICER-LIKE 1 proteins. Nucleic Acids Res. 43, 2802–2812. doi: 10.1093/nar/gkv102
Barker, G., Batley, J., O' Sullivan, H., Edwards, K. J., and Edwards, D. (2003). Redundancy based detection of sequence polymorphisms in expressed sequence tag data using autoSNP. Bioinformatics 19, 421–422. doi: 10.1093/bioinformatics/btf881
Barozai, M. Y. K., Kakar, S. U. R., and Sarangza, A. M. (2013). Profiling the carrot (Daucus carota L.) microRNAs and their targets. Pak. J. Bot. 45, 353–358.
Bej, S., and Basak, J. (2014). MicroRNAs: the potential biomarkers in plant stress response. Am. J. Plant Sci. 5, 748–759. doi: 10.4236/ajps.2014.55089
Bekh-Ochir, D., Shimada, S., Yamagami, A., Kanda, S., Ogawa, K., Nakazawa, M., et al. (2013). A novel mitochondrial DnaJ/Hsp40 family protein BIL2 promotes plant growth and resistance against environmental stress in brassinosteroid signaling. Planta 237, 1509–1525. doi: 10.1007/s00425-013-1859-3
Berger, D. K., Oelofse, D., Arendse, M. S., Du Plessis, E., and Dubery, I. A. (2000). Bean polygalacturonase inhibitor protein-1 (PGIP-1) inhibits polygalacturonases from Stenocarpella maydis. Physiol. Mol. Plant Pathol. 57, 5–14. doi: 10.1006/pmpp.2000.0274
Bhardwaj, A. R., Joshi, G., Kukreja, B., Malik, V., Arora, P., Pandey, R., et al. (2015). Global insights into high temperature and drought stress regulated genes by RNA-Seq in economically important oilseed crop Brassica juncea. BMC Plant Biol. 15:9. doi: 10.1186/s12870-014-0405-1
Bhardwaj, A. R., Joshi, G., Pandey, R., Kukreja, B., Goel, S., Jagannath, A., et al. (2014). A genome-wide perspective of mirnaome in response to high temperature, salinity and drought stresses in Brassica juncea (Czern) L. PLoS ONE 9:e92456. doi: 10.1371/journal.pone.0092456
Bogamuwa, S. P., and Jang, J. C. (2014). Tandem CCCH zinc finger proteins in plant growth, development and stress response. Plant Cell Physiol. 55, 1367–1375. doi: 10.1093/pcp/pcu074
Castle, J. C. (2011). SNPs occur in regions with less genomic sequence conservation. PLoS ONE 6:e20660. doi: 10.1371/journal.pone.0020660
Chaudhuri, K., and Chatterjee, R. (2007). MicroRNA detection and target prediction: integration of computational and experimental approaches. DNA Cell Biol. 26, 321–337. doi: 10.1089/dna.2006.0549
Chen, M., Tan, Z., Zeng, G., and Peng, J. (2010). Comprehensive analysis of simple sequence repeats in pre-miRNAs. Mol. Biol. Evol. 27, 2227–2232. doi: 10.1093/molbev/msq100
Chen, Z., Gao, X., and Zhang, J. (2015). Alteration of osa-miR156e expression affects rice plant architecture and strigolactones (SLs) pathway. Plant Cell Rep. 34, 767–781. doi: 10.1007/s00299-015-1740-x
Cheng, F., Liu, S., Wu, J., Fang, L., Sun, S., Liu, B., et al. (2011). BRAD, the genetics and genomics database for Brassica plants. BMC Plant Biol. 11:136. doi: 10.1186/1471-2229-11-136
Choi, Y., and Chan, A. P. (2015). PROVEAN web server: a tool to predict the functional effect of amino acid substitutions and indels. Bioinformatics 31, 2745–2747. doi: 10.1093/bioinformatics/btv195
Dolferus, R., Ji, X., and Richards, R. A. (2011). Abiotic stress and control of grain number in cereals. Plant Sci. 181, 331–341. doi: 10.1016/j.plantsci.2011.05.015
Du, Z., Zhou, X., Ling, Y., Zhang, Z., and Su, Z. (2010). agriGO: a GO analysis toolkit for the agricultural community. Nucleic Acids Res. 38, W64–W70. doi: 10.1093/nar/gkq310
Ehrenreich, I., and Purugganan, M. (2008). Sequence variation of MicroRNAs and their binding sites in Arabidopsis. Plant Physiol. 146, 1974–1982. doi: 10.1104/pp.108.116582
Fei, H., Tsang, E., and Cutler, A. J. (2007). Gene expression during seed maturation in Brassica napus in relation to the induction of secondary dormancy. Genomics 89, 419–428. doi: 10.1016/j.ygeno.2006.11.008
Ferrão, L. F. V., Caixeta, E. T., Pena, G., Zambolim, E. M., Cruz, C. D., Zambolim, L., et al. (2015). New EST–SSR markers of Coffea arabica: transferability and application to studies of molecular characterization and genetic mapping. Mol. Breed. 35, 1–5. doi: 10.1007/s11032-015-0247-z
Formey, D., Sallet, E., Lelandais-Brière, C., Ben, C., Bustos-Sanmamed, P., Niebel, A., et al. (2014). The small RNA diversity from Medicago truncatula roots under biotic interactions evidences the environmental plasticity of the miRNAome. Genome Biol. 15:457. doi: 10.1186/s13059-014-0457-4
Frazier, T. P., Xie, F., Freistaedter, A., Burklew, C. E., and Zhang, B. (2010). Identification and characterization of microRNAs and their target genes in tobacco (Nicotiana tabacum). Planta 232, 1289–1308. doi: 10.1007/s00425-010-1255-1
Ganie, S. A., and Mondal, T. K. (2015). Genome-wide development of novel miRNA-based microsatellite markers of rice (Oryza sativa) for genotyping applications. Mol. Breed. 35:51. doi: 10.1007/s11032-015-0207-7
Griffiths-Jones, S. (2004). The microRNA registry. Nucleic Acids Res. 32, D109–D111. doi: 10.1093/nar/gkh023
Guo, X., Gui, Y., Wang, Y., Zhu, Q. H., Helliwell, C., and Fan, L. (2008). Selection and mutation on microRNA target sequences during rice evolution. BMC Genomics 9:454. doi: 10.1186/1471-2164-9-454
Gupta, N., Zargar, S. M., Gupta, M., and Gupta, S. (2014). Assessment of genetic variation in Indian mustard (Brassica juncea L.) using PCR based markers. Mol. Plant Breed. 5, 10–17. doi: 10.5376/mpb.2014.05.0003
Hajyzadeh, M., Turktas, M., Khawar, K. M., and Unver, T. (2015). miR408 overexpression causes increased drought tolerance in chickpea. Gene 555, 186–193. doi: 10.1016/j.gene.2014.11.002
Hasanuzzaman, M., Nahar, K., Alam, M. M., Roychowdhury, R., and Fujita, M. (2013). Physiological, biochemical, and molecular mechanisms of heat stress tolerance in plants. Int. J. Mol. Sci. 14, 9643–9684. doi: 10.3390/ijms14059643
Hopkins, C. J., Cogan, N. O. I., Hand, M., Jewell, E., Kaur, J., Li, X. I., et al. (2007). Sixteen new simple sequence repeat markers from Brassica juncea expressed sequences and their cross-species amplification. Mol. Ecol. Notes 7, 697–700. doi: 10.1111/j.1471-8286.2007.01681.x
Huang, D., Koh, C., Feurtado, J. A., Tsang, E. W., and Cutler, A. J. (2013). MicroRNAs their putative targets in Brassica napus seed maturation. BMC Genomics 14:140. doi: 10.1186/1471-2164-14-140
Huang, X., and Madan, A. (1999). CAP3: a DNA sequence assembly program. Genome Res. 9, 868–877. doi: 10.1101/gr.9.9.868
Huh, S. U., and Paek, K. H. (2014). APUM5, encoding a Pumilio RNA binding protein, negatively regulates abiotic stress responsive gene expression. BMC Plant Biol. 14:75. doi: 10.1186/1471-2229-14-75
Ingkasuwan, P., Netrphan, S., Prasitwattanaseree, S., Tanticharoen, M., Bhumiratana, S., Meechai, A., et al. (2012). Inferring transcriptional gene regulation network of starch metabolism in Arabidopsis thaliana leaves using graphical Gaussian model. BMC Syst. Biol. 6:100. doi: 10.1186/1752-0509-6-100
John, B., Enright, A. J., Aravin, A., Tuschl, T., Sander, C., and Marks, D. S. (2004). Human MicroRNA targets. PLoS Biol. 2:e363. doi: 10.1371/journal.pbio.0020363
Joy, N., and Soniya, E. V. (2012). Identification of an miRNA candidate reflects the possible significance of transcribed microsatellites in the hairpin precursors of black pepper. Funct. Integr. Genomics 12, 387–395. doi: 10.1007/s10142-012-0267-2
Kanwar, M. K., Bhardwaj, R., Arora, P., Chowdhary, S. P., Sharma, P., and Kumar, S. (2012). Plant steroid hormones produced under Ni stress are involved in the regulation of metal uptake and oxidative stress in Brassica juncea L. Chemosphere 86, 41–49. doi: 10.1016/j.chemosphere.2011.08.048
Kasschau, K. D., Xie, Z., Allen, E., Llave, C., Chapman, E. J., Krizan, K. A., et al. (2003). P1/HC-Pro, a viral suppressor of RNA silencing, interferes with Arabidopsis development and miRNA function. Dev. Cell 4, 205–217. doi: 10.1016/S1534-5807(03)00025-X
Katiyar, A., Smita, S., Chinnusamy, V., Pandey, D. M., and Bansal, K. (2012). Identification of miRNAs in sorghum by using bioinformatics approach. Plant Signal. Behav. 7, 246–259. doi: 10.4161/psb.18914
Kim, H. J., Baek, K. H., Lee, B. W., Choi, D., and Hur, C. G. (2011). In silico identification and characterization of microRNAs and their putative target genes in Solanaceae plants. Genome 54, 91–98. doi: 10.1139/G10-104
Kushwaha, H. R., Singh, A. K., Sopory, S. K., Singla-Pareek, S. L., and Pareek, A. (2009). Genome wide expression analysis of CBS domain containing proteins in Arabidopsis thaliana (L.) Heynh and Oryza sativa L. Reveals their developmental and stress regulation. BMC Genomics 10:200. doi: 10.1186/1471-2164-10-200
Li, Y. C., Korol, A. B., Fahima, T., Beiles, A., and Nevo, E. (2002). Microsatellites: genomic distribution, putative functions and mutational mechanisms: a review. Mol. Ecol. 11, 2453–2465. doi: 10.1046/j.1365-294X.2002.01643.x
Liu, F., Wang, W., Sun, X., Liang, Z., and Wang, F. (2015). Conserved and novel heat stress-responsive microRNAs were identified by deep sequencing in Saccharina japonica (Laminariales, Phaeophyta). Plant Cell Environ. 38, 1357–1367. doi: 10.1111/pce.12484
Liu, Q., Wang, H., Zhu, L., Hu, H., and Sun, Y. (2013). Genome-wide identification and analysis of miRNA-related single nucleotide polymorphisms (SNPs) in rice. Rice 6:10. doi: 10.1186/1939-8433-6-10
Low, E.-T. L., Rosli, R., Jayanthi, N., Mohd-Amin, A. H., Azizi, N., Chan, K.-L., et al. (2014). Analyses of hypomethylated Oil Palm gene space. PLoS ONE 9:e86728. doi: 10.1371/journal.pone.0086728
Mandal, S., Bose, P., Dawar, S., Rajarani, A. P., and Santha, I. M. (2011). Kinetic and expression profiling of cytosolic pyruvate kinase enzyme during seed development of Indian mustard (Brassica juncea). J. Plant Biochem. Biotechnol. 20, 102–109. doi: 10.1007/s13562-010-0034-1
Meng, Y., Shao, C., and Chen, M. (2011). Toward microRNA-mediated gene regulatory networks in plants. Brief. Bioinformatics 12, 645–659. doi: 10.1093/bib/bbq091
Meyers, B. C., Axtell, M. J., Bartel, B., Bartel, D. P., Baulcombe, D., Bowman, J. L., et al. (2008). Criteria for annotation of plant MicroRNAs. Plant Cell 20, 3186–3190. doi: 10.1105/tpc.108.064311
Mun, J.-H., Yu, H.-J., Park, S., and Park, B.-S. (2009). Genome-wide identification of NBS-encoding resistance genes in Brassica rapa. Mol. Genet. Genomics 282, 617–631. doi: 10.1007/s00438-009-0492-0
Mutum, R. D., Balyan, S. C., Kansal, S., Agarwal, P., Kumar, S., Kumar, M., et al. (2013). Evolution of variety-specific regulatory schema for expression of osa-miR408 in indica rice varieties under drought stress. FEBS J. 280, 1717–1730. doi: 10.1111/febs.12186
Navarro, L., Jay, F., Nomura, K., He, S. Y., and Voinnet, O. (2008). Suppression of the MicroRNA pathway by bacterial effector proteins. Science 321, 964–967. doi: 10.1126/science.1159505
Ohama, N., Sato, H., Shinozaki, K., and Yamaguchi-Shinozaki, K. (2016). Transcriptional regulatory network of plant heat stress. Trends Plant Sci. 22, 53–65. doi: 10.1016/j.tplants.2016.08.015
Ozhuner, E., Eldem, V., Ipek, A., Okay, S., Sakcali, S., Zhang, B., et al. (2013). Boron stress responsive MicroRNAs and their targets in Barley. PLoS ONE 8:e59543. doi: 10.1371/journal.pone.0059543
Pani, A., and Mahapatra, R. K. (2013). Computational identification of microRNAs and their targets in Catharanthus roseus expressed sequence tags. Genomics Data 1, 2–6. doi: 10.1016/j.gdata.2013.06.001
Paritosh, K., Gupta, V., Yadava, S. K., Singh, P., Pradhan, A. K., and Pental, D. (2014). RNA-seq based SNPs for mapping in Brassica juncea (AABB): synteny analysis between the two constituent genomes A (from B. rapa) and B (from B. nigra) shows highly divergent gene block arrangement and unique block fragmentation patterns. BMC Genomics 15:396. doi: 10.1186/1471-2164-15-396
Ramesh, S., Ratnaparkhe, M., Husain, S., and Bhatia, V. (2015). DViral Micro RNA Transcriptomics (miRNAomics). Transcriptomics 3:108. doi: 10.4172/2329-8936.1000108
Rawlings-Goss, R. A., Campbell, M. C., and Tishkoff, S. A. (2014). Global population-specific variation in miRNA associated with cancer risk and clinical biomarkers. BMC Med. Genomics 7:53. doi: 10.1186/1755-8794-7-53
Sanan-Mishra, N., Kumar, V., Sopory, S. K., and Mukherjee, S. K. (2009). Cloning and validation of novel miRNA from basmati rice indicates cross talk between abiotic and biotic stresses. Mol. Genet. Genomics 282, 463–474. doi: 10.1007/s00438-009-0478-y
Shannon, P., Markiel, A., Ozier, O., Baliga, N. S., Wang, J. T., Ramage, D., et al. (2003). Cytoscape: a software environment for integrated models of biomolecular interaction networks. Genome Res. 13, 2498–2504. doi: 10.1101/gr.1239303
Sharma, P., and Sardana, V. (2013). Screening of Indian mustard (Brassica juncea) for thermo tolerance at seedling and terminal stages. J. Oilseed Brass. 4, 61–67.
Sharma, R., Mishra, M., Gupta, B., Parsania, C., Singla-Pareek, S. L., and Pareek, A. (2015). De novo assembly and characterization of stress transcriptome in a salinity-tolerant variety CS52 of Brassica juncea. PLoS ONE 10:e0126783. doi: 10.1371/journal.pone.0126783
Shastry, B. S. (2009). SNPs: impact on gene function and phenotype. Methods Mol. Biol. 578, 3–22. doi: 10.1007/978-1-60327-411-1_1
Shi, L., Shi, T., Broadley, M. R., White, P. J., Long, Y., Meng, J., et al. (2013). High-throughput root phenotyping screens identify genetic loci associated with root architectural traits in Brassica napus under contrasting phosphate availabilities. Ann. Bot. 112, 381–389. doi: 10.1093/aob/mcs245
Siemens, J., Keller, I., Sarx, J., Kunz, S., Schuller, A., Nagel, W., et al. (2006). Transcriptome analysis of Arabidopsis clubroots indicate a key role for cytokinins in disease development. Mol. Plant Microbe Int. 19, 480–494. doi: 10.1094/MPMI-19-0480
Singh, I., and Shah, K. (2014). Exogenous application of methyl jasmonate lowers the effect of cadmium-induced oxidative injury in rice seedlings. Phytochemistry 108(Suppl. C), 57–66. doi: 10.1016/j.phytochem.2014.09.007
Sinha, S., Raxwal, V. K., Joshi, B., Jagannath, A., Katiyar-Agarwal, S., Goel, S., et al. (2015). De novo transcriptome profiling of cold-stressed siliques during pod filling stages in Indian mustard (Brassica juncea L.). Front. Plant Sci. 6:932. doi: 10.3389/fpls.2015.00932
Smita, S., Katiyar, A., Chinnusamy, V., Pandey, D. M., and Bansal, K. C. (2015). Transcriptional Regulatory Network Analysis of MYB transcription factor family genes in rice. Front. Plant Sci. 6:1157. doi: 10.3389/fpls.2015.01157
Sorin, C., Bussell, J. D., Camus, I., Ljung, K., Kowalczyk, M., Geiss, G., et al. (2005). Auxin and light control of adventitious rooting in Arabidopsis require ARGONAUTE1. Plant Cell Online 17, 1343–1359. doi: 10.1105/tpc.105.031625
Srivastava, S., Srivastava, A. K., Sablok, G., Deshpande, T. U., and Suprasanna, P. (2015). Transcriptomics profiling of Indian mustard (Brassica juncea) under arsenate stress identifies key candidate genes and regulatory pathways. Front. Plant Sci. 6:646. doi: 10.3389/fpls.2015.00646
Srivastava, S., Srivastava, A. K., Suprasanna, P., and D'Souza, S. F. (2013). Identification and profiling of arsenic stress-induced microRNAs in Brassica juncea. J. Exp. Bot. 64, 303–315. doi: 10.1093/jxb/ers333
Stief, A., Altmann, S., Hoffmann, K., Pant, B. D., Scheible, W. R., and Baurle, I. (2014). Arabidopsis miR156 regulates tolerance to recurring environmental stress through SPL transcription factors. 26, 1792–1807. doi: 10.1105/tpc.114.123851
Sunkar, R., and Zhu, J.-K. (2004). Novel and stress-regulated microRNAs and other small RNAs from Arabidopsis. Plant Cell Online 16, 2001–2019. doi: 10.1105/tpc.104.022830
Tamaoki, M., Freeman, J. L., and Pilon-Smits, E. A. H. (2008). Cooperative ethylene and jasmonic acid signaling regulates selenite resistance in Arabidopsis. Plant Physiol. 146, 1219–1230. doi: 10.1104/pp.107.110742
Thiel, T., Michalek, W., Varshney, R. K., and Graner, A. (2003). Exploiting EST databases for the development and characterization of gene-derived SSR-markers in barley (Hordeum vulgare L.). Theor. Appl. Genet. 106, 411–422. doi: 10.1007/s00122-002-1031-0
Thomas, P. D., Kejariwal, A., Campbell, M. J., Mi, H., Diemer, K., Guo, N., et al. (2003). PANTHER: a browsable database of gene products organized by biological function, using curated protein family and subfamily classification. Nucleic Acids Res. 31, 334–341. doi: 10.1093/nar/gkg115
Tkaczuk, K. L., Obarska, A., and Bujnicki, J. M. (2006). Molecular phylogenetics and comparative modeling of HEN1, a methyltransferase involved in plant microRNA biogenesis. BMC Evol. Biol. 6:6. doi: 10.1186/1471-2148-6-6
Trick, M., Long, Y., Meng, J., and Bancroft, I. (2009). Single nucleotide polymorphism (SNP) discovery in the polyploid Brassica napus using Solexa transcriptome sequencing. Plant Biotechnol. J. 7, 334–346. doi: 10.1111/j.1467-7652.2008.00396.x
Verma, S. S., Rahman, M. H., Deyholos, M. K., Basu, U., and Kav, N. N. V. (2014). Differential expression of miRNAs in Brassica napus root following infection with Plasmodiophora brassicae. PLoS ONE 9:e86648. doi: 10.1371/journal.pone.0086648
Wang, J. W., Czech, B., and Weigel, D. (2009). miR156-regulated SPL transcription factors define an endogenous flowering pathway in Arabidopsis thaliana. Cell 138, 738–749. doi: 10.1016/j.cell.2009.06.014
Wilson, R. A., Sangha, M. K., Banga, S. S., Atwal, A. K., and Gupta, S. (2014). Heat stress tolerance in relation to oxidative stress and antioxidants in Brassica juncea. J. Environ. Biol. 35, 383–387.
Woods, D., Capcara, J., and Downey, R. (1991). The potential of mustard (Brassica juncea (L.) Coss) as an edible oil crop on the canadian Prairies. Can. J. Plant Sci. 71, 195–198.
Xie, K., Shen, J., Hou, X., Yao, J., Li, X., Xiao, J., et al. (2012). Gradual increase of miR156 regulates temporal expression changes of numerous genes during leaf development in rice. Plant Physiol. 158, 1382–1394. doi: 10.1104/pp.111.190488
Yang, J., Liu, X., Xu, B., Zhao, N., Yang, X., and Zhang, M. (2013). Identification of miRNAs and their targets using high-throughput sequencing and degradome analysis in cytoplasmic male-sterile and its maintainer fertile lines of Brassica juncea. BMC Genomics 14:9. doi: 10.1186/1471-2164-14-9
Zhang, B. (2015). MicroRNA: a new target for improving plant tolerance to abiotic stress. J. Exp. Bot. 66, 1749–1761. doi: 10.1093/jxb/erv013
Zhang, X., Lu, G., Long, W., Zou, X., Li, F., and Nishio, T. (2014). Recent progress in drought and salt tolerance studies in Brassica crops. Breed. Sci. 64, 60–73. doi: 10.1270/jsbbs.64.60
Zhao, Z., and Assmann, S. M. (2011). The glycolytic enzyme, phosphoglycerate mutase, has critical roles in stomatal movement, vegetative growth, and pollen production in Arabidopsis thaliana. J. Exp. Bot. 62, 5179–5189. doi: 10.1093/jxb/err223
Zhou, L., Liu, Y., Liu, Z., Kong, D., Duan, M., and Luo, L. (2010). Genome-wide identification and analysis of drought-responsive microRNAs in Oryza sativa. J. Exp. Bot. 61, 4157–4168. doi: 10.1093/jxb/erq237
Keywords: miRNA, target genes, miR-SSR, miR-SNP, miRNA-target regulatory network, Brassica
Citation: Singh I, Smita S, Mishra DC, Kumar S, Singh BK and Rai A (2017) Abiotic Stress Responsive miRNA-Target Network and Related Markers (SNP, SSR) in Brassica juncea. Front. Plant Sci. 8:1943. doi: 10.3389/fpls.2017.01943
Received: 31 May 2017; Accepted: 30 October 2017;
Published: 21 November 2017.
Edited by:
Raya Khanin, Memorial Sloan Kettering Cancer Center, United StatesReviewed by:
Mehar Hasan Asif, National Botanical Research Institute (CSIR), IndiaCopyright © 2017 Singh, Smita, Mishra, Kumar, Singh and Rai. This is an open-access article distributed under the terms of the Creative Commons Attribution License (CC BY). The use, distribution or reproduction in other forums is permitted, provided the original author(s) or licensor are credited and that the original publication in this journal is cited, in accordance with accepted academic practice. No use, distribution or reproduction is permitted which does not comply with these terms.
*Correspondence: Dwijesh C. Mishra, dcmishra@iasri.res.in
†These authors have contributed equally to this work.
Disclaimer: All claims expressed in this article are solely those of the authors and do not necessarily represent those of their affiliated organizations, or those of the publisher, the editors and the reviewers. Any product that may be evaluated in this article or claim that may be made by its manufacturer is not guaranteed or endorsed by the publisher.
Research integrity at Frontiers
Learn more about the work of our research integrity team to safeguard the quality of each article we publish.