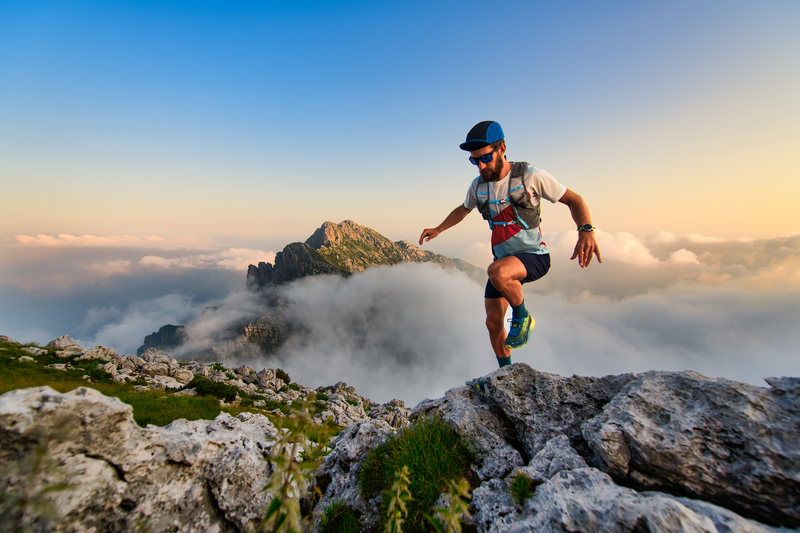
95% of researchers rate our articles as excellent or good
Learn more about the work of our research integrity team to safeguard the quality of each article we publish.
Find out more
ORIGINAL RESEARCH article
Front. Plant Sci. , 22 March 2017
Sec. Plant Breeding
Volume 8 - 2017 | https://doi.org/10.3389/fpls.2017.00367
This article is part of the Research Topic Advances in legume research View all 45 articles
Red clover (Trifolium pratense) is an important forage plant worldwide. This study was directed to broadening current knowledge of red clover's coding regions and enhancing its utilization in practice by specific reanalysis of previously published assembly. A total of 42,996 genes were characterized using Illumina paired-end sequencing after manual revision of Blast2GO annotation. Genes were classified into metabolic and biosynthetic pathways in response to biological processes, with 7,517 genes being assigned to specific pathways. Moreover, 17,727 enzymatic nodes in all pathways were described. We identified 6,749 potential microsatellite loci in red clover coding sequences, and we characterized 4,005 potential simple sequence repeat (SSR) markers as generating polymerase chain reaction products preferentially within 100–350 bp. Marker density of 1 SSR marker per 12.39 kbp was achieved. Aligning reads against predicted coding sequences resulted in the identification of 343,027 single nucleotide polymorphism (SNP) markers, providing marker density of one SNP marker per 144.6 bp. Altogether, 95 SSRs in coding sequences were analyzed for 50 red clover varieties and a collection of 22 highly polymorphic SSRs with pooled polymorphism information content >0.9 was generated, thus obtaining primer pairs for application to diversity studies in T. pratense. A set of 8,623 genome-wide distributed SNPs was developed and used for polymorphism evaluation in individual plants. The polymorphic information content ranged from 0 to 0.375. Temperature switch PCR was successfully used in single-marker SNP genotyping for targeted coding sequences and for heterozygosity or homozygosity confirmation in validated five loci. Predicted large sets of SSRs and SNPs throughout the genome are key to rapidly implementing genome-based breeding approaches, for identifying genes underlying key traits, and for genome-wide association studies. Detailed knowledge of genetic relationships among breeding material can also be useful for breeders in planning crosses or for plant variety protection. Single-marker assays are useful for diagnostic applications.
Fabaceae is among the most studied of plant families. The third-largest plant family, it includes many food and industrial plants and stands second only to Poaceae among the most important plant families from economic and nutritional perspectives (Graham and Vance, 2003). This importance results not only from the species' economic and nutritive values, but also from their unique capability for fixing atmospheric nitrogen. In the past decade, the extent of genomic information available on legumes has been broadened substantially. Such model species as Medicago truncatula Gaertn. (Young et al., 2011) and Lotus japonicus L. (Sato et al., 2008), as well as the crops soybean (Glycine max [L.] Merrill.; Schmutz et al., 2010), pigeon pea (Cajanus cajan [L.] Millsp; Varshney et al., 2012), and chickpea (Cicer arietinum L.; Varshney et al., 2013) have been sequenced. Several other sequencing projects are under way which encompass a broad range of agronomically and horticulturally important plants (www.phytozome.net).
Red clover (Trifolium pratense L.) belongs to the tribe Trifolieae, together with another 240 annual and perennial herb species, both wild and cultivated. It is an important forage plant worldwide, serving as a temporary cover crop or manure crop as well as for silage production and grazing. Like other legumes, it is capable of fixing atmospheric nitrogen via symbiosis with Rhizobium leguminosarum bv. trifolii (Sprent, 2009). Its breeding and related research have been complicated, however, by the species' outcrossing nature with gametophytic self-incompatibility. The resulting heterozygosity has hampered intensive genetic and genomic analysis. Nonetheless, with the rising availability of sequencing technology, red clover has been a target of several genomic studies in recent years.
Red clover's nuclear genome is divided into seven chromosomes (x = 7) with size estimated to be 418 Mbp (1C = 0.43 pg; Vižintin et al., 2006). The first consensus high-density linkage map contained 1,414 simple sequence repeats (SSRs), 181 amplified fragment length polymorphisms, and 228 restriction fragment length polymorphisms (Isobe et al., 2009). The structure of the red clover genome has been investigated using fluorescence in situ hybridization (Sato et al., 2005; Kataoka et al., 2012). The genome also has been compared with those of related species (white clover, M. truncatula and L. japonicus) using DNA markers (Isobe et al., 2012). DNA markers, too, can be used in various research and practical approaches. For example, two studies used DNA markers to identify quantitative trait loci (QTLs) related to persistence (Herrmann et al., 2008), disease resistance, and winter hardiness (Klimenko et al., 2010) in full sib mapping families. Recently, great insight into red clover genomics has been achieved through application of next-generation sequencing (NGS) technology. Both whole-genome sequencing (WGS; Ištvánek et al., 2014; De Vega et al., 2015) and RNA sequencing (Yates et al., 2014) have been carried out in red clover. While WGS focused on describing red clover's genome, RNA sequencing described transcriptome differences in conditions of drought stress. Concurrently, studies of both types identified a great number of DNA markers which can be of great value in practical applications.
As a consequence of the outcrossing, both natural ecotypes and varieties that may be morphologically similar are likely to be highly heterogeneous genetically. Strategies for genetic diversity analysis based on DNA profiling must address this issue and enable quantification of variation within and among populations. Evaluation of genetic variation for outcrossing forage species is important for the processes of cultivar identification and seed purity analysis, ecological analysis of pasture populations, and selection of genetically divergent parents for genetic mapping studies (Forster et al., 2001). The genetic divergence of some genotypes ensures a high level of genetic polymorphism in crosses. Breeding methods for cross-pollinated forage crops, including red clover, require strategies for genotyping. Genetic markers assaying variation in transcribed regions of genes with known functions will be useful for developing trait-linked markers. NGS has shown great potential for large-scale production of functional genes and molecular markers at the whole-genome level, especially in non-model organisms. Two important tasks for NGS are identifying expression patterns in biochemical processes and classifying genes into specific pathways. Legumes can produce more secondary metabolites (especially cyanogenic glucosides, glucosinolates, amines, and alkaloids) than can other plants which are not nitrogen fixers. Most secondary metabolites exhibit some biological, pharmacological, or toxicological activity (Teuscher and Lindequist, 2010; Wink, 2013). In this respect, the Fabaceae are distinguished by isoflavones, which function as antioxidants, phytoestrogens, and antimicrobial compounds. The benefits of protecting plant proteins from degradation in the rumen by means of polyphenol oxidases (PPOs) have been established in some fodder crops, and red clover contains PPOs in significant quantities (Jones et al., 1995; Jakešová et al., 2015). There is also an increasing need to develop molecular markers for resistance genes or components relating to nitrogen fixation.
Based on previously published genome assembly (Ištvánek et al., 2014), this study aims to elucidate red clover genes involved within complex biosynthetic pathways in response to biological processes. Special attention is given to specific secondary metabolites inasmuch as they can significantly influence the final variety's breeding strategy and purpose. Gene-specific SSR and single nucleotide polymorphism (SNP) markers are reported and described with a view to enhancing marker-assisted breeding in outcrossing species of red clover. Finally, we developed and validated sets of polymorphic microsatellites and SNPs for the analysis of genetic relationships among red clover varieties and individuals. The findings of this study can be useful in investigating genetic diversity and red clover breeding. These markers will contribute to enriching the current reference red clover map, generating more informative genetic and genomic tools, and enabling genome synteny analysis.
Sequencing, de novo assembly, gene prediction, and initial annotation is described in Ištvánek et al. (2014). Whole Genome Shotgun project has been deposited at DDBJ/ENA/GenBank under the accession ASHM00000000. The version used in this paperis ASHM01000000. Detailed inspection of gene annotation was completed manually. Protein-encoding genes were classified into functional categories according to Gene Ontology (GO) annotation, and the results were summarized in plant GOslim functional categories. Each gene was aligned against KEGG (release 67.1) proteins, and the pathway in which that gene might be involved was determined. Genes were also sorted by the pathways.
Homologous gene sequences were analyzed among red clover, M. truncatula, and G. max. Predicted genes in these species were mapped and searched for homology on the basis of red clover (DDBJ/EMBL/NCBI accession numbers: LT555306.1–LT555312.1; De Vega et al., 2015), M. truncatula, and G. max chromosome sequences. The best hits from a TBLASTX (e ≤ 1e−15) search with at least 70% identity were mapped on these chromosomes and gene densities for every 100 kbp were counted. Homologous sequences for these species were determined using the best hits from a reciprocal TBLASTX search. Circos software (Krzywinski et al., 2009) was used to visualize the top half hits of the data through a circular concentric ideograms layout. Gene densities were displayed as histogram plots and homologous sequences for the three species as lines.
SSR Locator (da Maia et al., 2008) was used to mine SSRs in the red clover genes as well as for primer design. Uniform melting temperature at Tm = 55°C was set for all predicted SSR sites, which were defined as a monomer occurring at least 12 times, a dimer occurring at least 6 times, tri- and tetramers occurring at least 4 times, and penta- and hexamers occurring at least 3 times. The number of polymerase chain reaction (PCR) products was predicted for each primer pair.
Probable SNPs in genes were discovered by aligning Tatra reads onto predicted genes using bwa v0.7.5 (Li and Durbin, 2010). Samtools v0.1.19 (Li et al., 2009) and Picard v1.80 (http://broadinstitute.github.io/picard/) were used in subsequent steps of marking PCR duplicates, sorting, and indexing. GATK v2.7 (https://www.broadinstitute.org/gatk/) was used to remap the reads near the InDels, recalibrate base quality scores, and identify SNPs. Only sites with SNP calling quality scores of 30 or higher and with read depths of at least 10 reads were marked as high-quality SNPs. For subsequent filtration, custom perl scripts were used.
SSR marker validation was carried out for 50 red clover varieties, 34 of which were Czech varieties and 16 from other countries (Table 1). SNP marker validation was performed for 5 varieties (Amos, Fresko, Start, Tatra, Tempus). Leaves were collected from plants 30 days old which had been grown in a greenhouse. For SSR analysis, genomic DNA was isolated from 16 pooled plants per variety from ~1 g of young leaves using the modified protocol by Dellaporta et al. (1983). Concentration and purity of extracted genomic DNA was assessed using NanoDrop (Thermo Scientific, Waltham, MA, USA).
To validate SSRs, we randomly selected 96 SSR loci in coding sequences. SSR primers (Table S1) were predicted by SSR Locator. Validations of predicted SSRs were performed via PCR and electrophoretic separation. PCRs were carried out in a volume of 10 μl with 1x reaction buffer, 0.2 mM of each dNTP (10 mM; Sigma-Aldrich, Steinheim, Germany), 10 pmol of each primer, 0.5 U of GoTaq® polymerase (Promega, Madison, WI, USA), and 30 ng of genomic DNA template.
Cycling conditions were set as follows: a preliminary step at 94°C for 3 min, 58°C for 1 min, 72°C for 1 min; 30 cycles of 94°C for 30 s, 58°C for 30 s, and 72°C for 30 s; and an elongation step at 72°C for 5 min. PCR-amplified fragments were separated by electrophoresis on either a 3% agarose gel or a 10% polyacrylamide gel and visualized by ethidium bromide staining.
The validation of predicted SNP variants was performed by SNP array (Arrayit Corporation, CA, USA) with 8,623 genome-wide distributed SNPs. We examined intra-variety genetic heterogeneity using SNP genotyping for a set of 20 DNA samples of individual red clover plants of the variety Tatra. Probes, 15-mer oligonucleotides, were designed with the SNP at the center position without overlap (two probes per SNP; Table S2). The fluorescent dyes Alexa Fluor® 555 and Alexa Fluor® 647 (Invitrogen, CA, USA) were used for labeling. Capture agents were printed into 1–48 microarrays per 25 × 76 mm glass substrate slide, each probe 3 times. Hybridization was performed with genomic DNA isolated from individual plants using the modified protocol by Dellaporta et al. (1983). Variance stabilizing normalization was used to evaluate fluorescence intensities of the reference and alternative alleles by RStudio software (RStudio Team, 2015) with limma package (Ritchie et al., 2015) and the log2 intensity data were processed.
Single-marker SNP polymorphisms were also validated by the modified technique of temperature switch PCR (Tabone et al., 2009) whereby two PCRs were carried out for each locus, one for a reference (R) allele and the other for an alternative (A) allele. Due to this technique's specific requirements, only SNP sites having no other SNP in their vicinity for 30 bp were included within the validation. Genomic DNA was isolated from 100 mg of leaves of individual plants using the CTAB method (Rogers and Bendich, 1989). SNP validation was performed for five candidate SNPs from Tatra coding sequences used as a reference. Primers (Table S3) for SNPs were predicted using Primer3 and OligoCalc. For an R allele, two primer pairs were used (LS—locus specific and NLS—nested locus specific) with different melting temperatures. PCR amplification using these four primers provides 4 amplicons, while only the amplicon emerging from the NLS primers (with NLS_F primer directly binding to the SNP position) confirms the presence of an R allele in a selected sample. For reliable detection of an A allele, we performed a simplified PCR reaction with two primers, a forward LS primer and a reverse primer (labeled as reverse primer SNP; SNP_R) which binds with its 3′ end to a SNP nucleotide. The presence of a PCR product of a predicted length confirms the existence of an A allele. All expected products with their predicted lengths are listed in Table S4. The PCR components used for both PCRs were the same as for the SSR validation, with minor modifications: for detection of the R allele, 5 pmol of each NLS primer and 1 pmol of each LS primer were used. Also, PCR was enriched with 1% bovine serum albumin. To detect the A allele, 5 pmol of both primers were used. Both PCRs used GoTaq® polymerase in a concentration of 0.25 U. Cycling conditions for the R allele were used according to Tabone et al. (2009). Cycling conditions for the A allele were shortened to a denaturation step at 95°C for 5 min; 30 cycles of 95°C for 30 s, 62°C for 30 s, and 72°C for 30 s; then 72°C for 5 min as a final elongation step. PCR-amplified fragments were separated by electrophoresis on a 10% polyacrylamide gel and visualized by ethidium bromide staining.
Evaluation of individual polymorphic fragments was inferred from agarose or polyacrylamide gels with separated PCR-amplified fragments and was performed for each SSR marker manually. Pooled polymorphism information content (pPIC) was then calculated for each SSR marker, expressing the probability of detecting a polymorphism between genotypes of two randomly drawn red clover varieties. pPIC was calculated for all 50 red clover varieties. Calculations were performed for each SSR marker m separately as follows:
1) Genotypes were divided into two groups (G1 and G0) according to the presence/absence of any PCR-amplified product for the marker m (i.e., G1 contained all genotypes with at least one PCR-amplified product and G0 contained the rest).
2) pPIC for the subgroup G1 was calculated as:
where i is one particular band from n possible bands of this marker; Π is the product operator, i.e., the symbol denoting product of a sequence in a similar manner as ∑ denotes summation; and fi is the frequency of the i-th band among all genotypes in G1. pPIC1 is thus the probability that the marker m can distinguish any two random genotypes whose probabilities for the presence of each band come from the same distribution as in the sample G1.
3) pPIC for the subgroup G0 was pPIC0 = 0, because the genotypes in G0 cannot be distinguished from each other (they did not have any PCR-amplified products).
4) pPIC for comparison of two genotypes, one from each group, was pPIC1 × 0 = 1, because any genotype from G1 can be distinguished from any G0 genotype by having at least one product.
5) Overall pPIC for the marker m was then calculated by weighting the values acquired in previous steps according to the proportionate sizes of G1 and G0:
where , are proportions of genotypes in the two groups G1 and G0 (N1 and N0 are counts of genotypes in G1 and G0 for the marker m).
The polymorphic information content (PIC) of the SNP loci was calculated according to Botstein et al. (1980).
The similarity between each pair of T. pratense varieties was assessed according to the presence or absence of individual separated PCR-amplified fragments using the Jaccard (1901) and Sørensen–Dice (Dice, 1945; Sørenson, 1948) indices for each SSR marker. The Jaccard and Sørensen–Dice indices were calculated as nxy/(nx + ny – nxy) and 2nxy/(nx + ny), respectively, where nxy represents the number of bands which are present simultaneously in both compared varieties, nx represents the number of all bands of one of the compared varieties, and ny represents the number of all bands of the other compared variety.
Separately for each of the indices, these coefficients of similarity were used for calculating a pairwise distance matrix for each marker, where the distance between two selected varieties was computed as 1—the corresponding similarity coefficient. Finally, an averaged distance matrix was created by averaging distance matrices of all markers. Thus, two pairwise distance matrices—one based on the Jaccard index and one on the Sørensen–Dice index—were created, describing the averaged dissimilarity between each pair of red clover varieties. Two phylogenetic trees based on the averaged distance matrices were calculated in MATLAB (version R2015a, http://www.mathworks.com) using the unweighted pair group method with arithmetic mean (UPGMA) clustering method then manually edited and visualized in FigTree (version 1.4.2, http://tree.bio.ed.ac.uk/software/figtree/).
As described in Ištvánek et al. (2014), 243.6 million reads were obtained by sequencing. After filtering out low-quality reads and sequencing adapter relics, genome coverage of ~55.4x was achieved. A total of 64,761 genes were predicted in red clover (Ištvánek et al., 2014) and after manual revision of Blast2GO annotation, 42,996 genes were characterized. These included 1,316 genes related to repetitive elements (Table 2). One of the main annotation steps was based on finding the sequence homology with accessions in the RefSeq database (BLASTP search). The results of this part are summarized in Figure 1 in the form of Blast Top-Hits, showing the degree of relationship to other sequenced plant model species. All predicted genes with their annotations are displayed in Table S5.
Annotated genes were assigned to appropriate biological process, molecular function, and cell component subclasses based on their annotation (Figure 2). Within the sequences associated with biological processes, GO terms associated with primary and secondary metabolism were the most prevalent. In this respect, primary metabolites are known to be essential for plant survival while secondary metabolites play important roles in plant protection and have a broad spectrum of utilization. We also found genes associated with the GO term “response to stimulus” to occur very frequently. This category includes mainly genes involved in responses to stress, biotic stress, and endogenous as well as extracellular stimuli. In molecular function, almost one-half of genes are associated with the GO term “binding,” in which the binding functions of nucleotides or DNA form the majority. “Catalytic activity,” as the second most prevalent term, comprises enzymatic activities of kinases, hydrolases, nucleases, etc. In cellular component, the most frequent GO terms were associated with functions within the plant cell, organelles (plastids and mitochondria), and plasma membrane. In short, these relate to the main cellular compartments of plant cells.
Figure 2. Assignment of red clover genes into Gene Ontology (GO) terms in level 2 belonging to: (A) Biological Process, (B) Molecular Function, (C) Cellular Component.
Annotated genes were also classified into metabolic and biosynthetic pathways. A total of 7,517 genes were characterized and assigned to specific pathways. Because many genes figure in multiple biosynthetic or metabolic pathways, a total of 17,727 enzymatic nodes were described in all pathways. Among the largest metabolic pathways (each involving more than 1,000 genes) were purine metabolism and starch and sucrose metabolism. The 20 largest biosynthetic pathways are summarized in Table 3. Table S6 presents a complete list of genes assigned to specific metabolic and biosynthetic pathways. Each enzyme was also assigned to one of the main enzyme classes (Figure 3). In red clover, almost one-half of enzymes belong to transferases (43.44%), and more than 89% of enzymes consist of transferases, hydrolases, and oxidoreductases.
Table 3. Twenty largest biosynthetic and metabolic pathways in red clover based on number of genes (enzymes) involved.
A TBLASTX search was performed to evaluate the distribution of all red clover genes along recently published chromosomes of red clover, chromosomes of the model legume species M. truncatula (8 chromosomes), and chromosomes of G. max (20 chromosomes). The results were plotted using a window size of 100 kb through genomic sequences (Figure 4). Repetitive element and gene densities in each species were distributed along all chromosomes of T. pratense, M. truncatula, and G. max. Distribution patterns were similar in both T. pratense and M. truncatula. Under the specified criteria, 41,607 red clover genes were found to be homologs in comparison with M. truncatula and 32,737 genes were homologs with G. max. In G. max, the genes were concentrated in subtelomeric and telomeric regions. These are regions with low density of repetitive elements, unlike centromeric regions (Torales et al., 2013). This can be seen also in the central lines that show the distribution of homologous sequences to M. truncatula, G. max, and red clover. On the other hand, the gene densities in M. truncatula are the more balanced, with only a slight decrease in centromeric regions. Centromeric regions were also poorer for homologous sequences, for example in chromosome Mt6 and Mt8.
Figure 4. Comparison of gene densities and genome structure in legume model species (A) M. truncatula and (B) G. max with T. pratense. The 7 T. pratense chromosomes (DDBJ/EMBL/NCBI accession numbers: LT555306.1 - LT555312.1) are shown in orange, 8 M. truncatula chromosomes in blue, and 20 G. max chromosomes in green in the outer circles. (1) First circles represent repetitive element densities relevant to each chromosome (yellow). Gene densities (by 100 kb windows) are displayed on each chromosome as follows: (2) gene density in T. pratense (orange), M. truncatula (blue), and G. max (green) on their own chromosomes; (3) relative gene densities of T. pratense on M. truncatula and G. max chromosomes mapped on the partner's chromosomes; (4) homologous sequences and synteny regions in T. pratense with M. truncatula and T. pratense with G. max (central lines; top half is colored).
Using SSR Locator (da Maia et al., 2008), we identified 6,749 potential microsatellite loci in red clover coding sequences. For those with sufficient flanking sequences, we designed appropriate unique primers to generate PCR product preferentially within 100–350 bp. The resulting 4,005 (59.3%) potential SSR markers were characterized (Figure 5). Because 1,061 (26.5%) of these SSR markers occurred in an identical unique locus, it results that just 3,409 (5.3%) coding sequences possess at least one SSR marker. When the total length of coding sequences (49.6 Mbp) is taken into count, marker density of 1 SSR marker per 12.39 kbp was achieved. Especially noteworthy is that no SSR markers were found in the genes belonging to the isoflavonoid biosynthetic pathway, such as 2-dihydroflavonol reductase, chalcone synthase, and isoflavone synthase. All potential SSR markers are shown in Table S7.
Figure 5. Statistics for 4,005 predicted SSR markers in coding sequences of red clover. (A) Basic motif frequencies in coding regions of red clover. (B) Frequencies of mono-, di-, tri-, tetra-, penta-, and hexamers plus complex SSR motifs in red clover genes containing one to six SSRs per locus. Total number of sequences containing depicted number of SSRs is shown above each column.
As expected, the most frequently seen basic motif of microsatellite corresponded to trimeric repeat (78.68%), followed by complex (10.74%) and hexameric (8.16%) motifs (Figure 5). These motifs were also present mainly in loci with a single SSR marker. Complex motifs consisted mainly of two–five trimeric motifs, with only 55 (12.8%) exceptions containing also other motifs (mainly hexameric). Other motifs, such as dimeric and pentameric, were seen much less frequently. Only in 7 SSRs with complex motifs did the complex motif not contain a trimeric repeat.
SNPs were identified by aligning reads to predicted coding sequences. The analysis resulted in identification of 343,027 SNP markers, providing marker density of 1 SNP marker per 144.6 bp, meaning on average 5.3 SNP markers per gene. Of these SNPs, 290,905 (84.8%) SNPs were high quality. SNP markers were also divided between transitions and transversions based on the nature of the A allele. The majority (nearly two-thirds) of SNP markers were transitions. In addition, 4,065 (1.19%) of the identified SNP markers were multi-allelic, with more than one A allele. Table 4 presents a complete overview and statistics relating to SNP markers. Table S8 summarizes the complete list of SNP markers, including their positions and additional information.
Of 96 chosen SSR loci, only the SSR locus SSR-TP_g53834.t1.cds1 was not amplified. Altogether, 95 SSRs were analyzed for 50 red clover varieties. SSR markers with a PCR product are summarized in Table S1. Single monomorphic SSR marker SSR-TP_g20700.t1.cds3 was amplified in all 50 varieties. The lowest number of amplified samples/varieties was 27 (Figure 6). Fifteen varieties gave a PCR product for all 95 SSRs, and the lowest number of markers (4) was amplified in a single variety (Radegast).
Figure 6. SSR marker validation in red clover varieties. (A) Numbers of SSRs amplified in analyzed varieties. (B) Numbers of varieties amplified for individual SSRs. (C) Allele number distribution for SSR markers validated in red clover varieties. (D) pPIC distribution for SSR markers validated in red clover varieties.
Allele number ranged from 1 to 17 (Table S1, Figure 6). The pPIC of these SSR loci ranged from 0 to 0.986 with a mean of 0.679 and median of 0.693 (Table S1). The highest diversity was determined for SSR loci with trinucleotide motifs and pPIC ranging from 0.180 to 0.986 (Table 5). Twenty-two SSRs out of 95 validated were highly polymorphic and with pPIC >0.9 while 72 SSRs showed pPIC >0.5 (Figure 6).
The similarity between individual varieties of T. pratense was assessed using the Sørensen–Dice and Jaccard indices (Figure 7, Figure S1). Cluster analysis grouped the 50 red clover varieties into two clusters. Sub-cluster IA consisted of the single variety Radegast (4x), developed from landraces that were well adapted locally, from breeding varieties (Slovensky podtatransky, Chlumecky, and Horal) and a later cross with the variety Weitetra. Sub-cluster IIA comprised a group of varieties whose genomes were enriched with genotypes of European origin: Dolina (4x), Vulkan (4x), and Sigord (4x) were developed by crosses of Czech, Polish, and German varieties; Tabor (2x) was developed by mass crosses of selected resistant plants belonging to 49 varieties; and Atlantis (4x) is of German origin. Cluster IIB1 consisted of two varieties: Slavoj (2x) was developed by the selection of genotypes, and Kvarta (4x; released 1974) was developed by polyploidy of landraces and the variety Chlumecky (2x), which itself was a component of the next sub-cluster IIB2-1. Chlumecky is the earliest red clover cultivar (released 1935) developed by individual plant selection from the landrace Cesky. Sprint (4x) was obtained after the polyploidy of four newly bred genotypes of European origin.
Figure 7. Study of red clover variety divergence using SSR markers (Sørensen-Dice index, *reference genotype).
IIB2-2 was a large cluster of diploid and tetraploid Czech, European, and non-European varieties, reflecting that the varieties are often populations developed from genotypes with wide genetic variability, by targeted crosses, polycrosses, and topcrosses suitable for the selection of complex characters, with synteny of the selected genotypes. The sub-clusters of non-Czech origin varieties were as follows: IIB2-2a Grasslands Hamua from New Zealand and Hungarotetra from Hungary; IIB2-2b three varieties of non-European origin (Makimidori, Concorde, Walter); and IIB2-2c, five European varieties (Lossam, Triton, Essex Broad Red, Gibridnij Pozdnespelyj, Parka). In addition, Pavo and Astur were released in Switzerland, Nemaro, and Titus in Germany, and Vesna is of Czech origin but developed by crosses of diploid genotypes from Czech, French, Swiss, and German varieties with subsequent polyploidy by colchicine. Slovak and Swedish red clover genetic material was introgressed into the genome of Tatra, and Blizard was bred using non-Czech genotypes and recurrent phenotypic selection. Start, released in 1974, was used as a component for more recently bred varieties such as Garant, Cyklon, Spur, Trubadur, Dolly, and Tempus. The same cluster distribution was observed using the Sørensen–Dice and Jaccard indices, with only a few exceptions in cluster IIB2-2 (Figure 7, Figure S1), such as the sub-clusters Grasslands Hamua and Hungarotetra, Cyklon, Suez, Beskyd, Spur, and Trubadur.
We examined intra-variety genetic heterogeneity using genome-wide SNP genotyping. Five possible genotypes for two alleles per SNP (reference R, alternative A) were differentiated (RRRR, RRRA, RRAA, RAAA, AAAA) for tetraploid plants. Our analysis revealed 8,607 polymorphic SNP markers with PIC ranging from 0.024 to 0.375 with a mean of 0.338 and median of 0.355 (Table S9).
Single-marker polymorphism was successfully validated and confirmed in five SNP loci and homozygosity/heterozygosity was determined in 14 particular plants, 7 of which were of variety Tatra, 4 of variety Tempus, and 1 plant of each Start, Amos, and Fresco. The majority of those plants analyzed were heterozygous in the tested loci. Homozygosity for the R allele was detected in one plant (Tempus) in TP_g30014_516, four plants (Tatra, Amos, Fresco, Tempus) in TP_g30658_273, two plants (Tatra, Tempus) in TP_g33120_639, two plants (Amos, Tempus) in TP_g51879_538, and eight plants (Tatra, Start, Amos, Fresco, and all Tempus) in TP_g56325_406. Two homozygotes of Tatra were detected for the A allele in TP_g33120_639 (Figure 8). All amplified fragments corresponded to those predicted.
The number of annotated genes in this study is higher than the number of genes identified recently by RNA sequencing (34,534 genes; Yates et al., 2014), but it is very close to the number from other WGS (40,868 genes; De Vega et al., 2015). On the basis of improved annotation of red clover genes, genes were classified into biosynthetic and metabolic pathways and key enzymes were identified. We have found 1,138 genes involved in purine metabolism, which is the fundamental pathway for plant growth and development (Zrenner et al., 2006). This pathway is associated with DNA synthesis, energy sources, and synthesis of many primary and secondary metabolic products (Stasolla et al., 2003). More than 1,000 genes are also involved in starch and sucrose metabolism, which is one of the most important pathways regarding energy sources in plants. Moreover, with 257 genes involved, biosynthesis of flavonoids is among the largest metabolic and biosynthetic pathways. These genes are of particular interest to red clover breeders inasmuch as flavonoid biosynthesis is associated with isoflavonoid content in the plant. Because they are known plant estrogen analogs, high levels of isoflavonoids are undesirable in forage varieties (Adams, 1995) but these are required in varieties used in pharmaceutics (Park and Weaver, 2012).
Based on the distribution of homologous sequences among red clover, M. truncatula, and G. max, very similar patterns in gene distribution are present in red clover and M. truncatula. In contrast to G. max, gene density is rather uniform along entire chromosomes. Occasional spikes in density of red clover genes show clusters of numerous gene families, such as genes of resistance. As previously described (Kulikova et al., 2004; Isobe et al., 2012; Torales et al., 2013), M. truncatula had fewer homologous genes located on its chromosome 6 due to the abundance of heterochromatic sites and retroviral elements scattered throughout its arms. This was in contrast to red clover, where no such decrease was observed. The density of repetitive content along the chromosomes also was inspected and this provided very similar results. Also visible, however, is increased content of repetitive elements in red clover (Ištvánek et al., 2014) compared to M. truncatula (Young et al., 2011). Orthologous loci were connected and compared among species visualizing syntenic loci and rearrangements of genome structure. During speciation, red clover clearly underwent complex genome restructuring, possibly associated with reduction of the basic chromosome number from eight to seven. Results supporting this hypothesis were also found in a comparison among red clover, white clover (T. repens), and M. truncatula based on comparing DNA markers and their location in the genomes (Isobe et al., 2012). Our results are supported, too, by a recently published paper regarding WGS of red clover and construction of its physical map (De Vega et al., 2015). A slight discrepancies in locations of homologous sequences are likely the result of different methodology compared to previously published papers (Isobe et al., 2012; De Vega et al., 2015).
DNA markers have a broad spectrum of uses in both research and practical breeding. They are used in QTL mapping (Zhao et al., 2013), evolution relationship studies (Ghamkhar et al., 2012; Isobe et al., 2012), variability assessment and genotyping of breeding material (Younas et al., 2012; Cidade et al., 2013), marker-assisted selection, and even gene pyramiding (Qi et al., 2015). Based on NGS technology, we are capable of discovering thousands of SSR and many millions of SNP markers (Zalapa et al., 2012).
Searching for SSR loci within 64,761 predicted coding sequences resulted in the identification of 6,749 SSR loci. This is more than twice the number of SSR loci identified from red clover transcriptome sequencing (Yates et al., 2014). On the other hand, due to the lower number of genes and shorter length of coding sequence described by RNA sequencing, the average SSR marker frequency is very similar (1 SSR marker per 13.42 kbp; Yates et al., 2014). When multiple repeat occurrences are taken into account, 10.4% of genes on average contained an SSR locus. This frequency is comparable to that reported in Prosopis alba (11%; Torales et al., 2013) but lower than those in Nothofagus nervosa (15%; Torales et al., 2012) and oak (19%; Ueno et al., 2010 and 24%; Durand et al., 2010). In coding sequences, clear domination of trimeric motifs (78.68%) is observed when compared to the SSRs from the genome as a whole (26.9%; Ištvánek et al., 2014). Much lower frequencies were found for other motifs. Similar results have been obtained also in other species, such as N. nervosa (Torales et al., 2012) and oak (Ueno et al., 2010). This phenomenon is very likely connected to the need to preserve open reading frame within the coding sequences and negative selection pressure against those SSR loci breaking it. Even in the majority of SSR loci with non-trimeric basic motifs, therefore, the combination of motif length and its repeat number is divisible by three—e.g., (A)12, (GA)6, (ATTGG)3—and this, then, does not violate reading frame by frame-shift mutations (Metzgar et al., 2000).
SNPs were also identified in coding sequences of red clover. Their greater frequency throughout the genome (343,027 SNP markers; 1 SNP per 144.6 bp) makes them useful in such high-throughput methods as SNP arrays (Víquez-Zamora et al., 2013; Yu et al., 2014). Nevertheless, when compared to other plant species, it is clear that SNP frequency is influenced by many factors, such as the number of individual plants analyzed in the study, natural variability in the population of the studied species, etc. In P. alba, for example, 1 SNP marker was found for every 2,512 bp (Torales et al., 2013), in Capsicum annuum 1 for every 2,253 bp (Ashrafi et al., 2012), in oak 1 for every 471 bp (Ueno et al., 2010), and in Eucalyptus grandis 1 for every 192 bp (Novaes et al., 2008). In these studies, the SNP number found correlated mainly with the number of individuals analyzed (e.g., 21 individual plants in oak, more than 200 in E. grandis). Although, just 16 individual plants of the same variety were analyzed in red clover, even higher SNP frequency was obtained, likely due to the outcrossing nature of clovers. Within the identified SNPs, transitions (63.52%) showed significant dominance over transversions (35.29%). These results are consistent with those in P. alba (Torales et al., 2013) and Cucurbita pepo (Blanca et al., 2011). A large number of SNPs are now available in red clover for genome-wide association studies and SNP microarray construction, where tens of thousands of markers are required.
Outcrossing species populations are exceptionally variable and with a high level of heterozygosity. The majority of genetic analyses of such species are necessarily carried out in pooled samples in order to collect most of the population variability and also minimize costs. Results obtained from such pooled samples are, however, unsuitable for estimating the copy number of individual alleles, which precludes assessment of exact allele frequencies required to calculate polymorphic information content (PIC; Botstein et al., 1980). Recent advances in NGS technologies enable determination of allele frequencies from pooled samples (Mullen et al., 2012; Lynch et al., 2014), but these are very expensive and thus inaccessible especially for breeders working with non-model crops.
PIC is commonly used in plant genetics to assess polymorphism level for a marker locus. For leguminous plants, PIC was recently used, for example, for evaluating 48 SSR markers of Vigna radiata (Shrivastava et al., 2014), 45 SSR markers of Trifolium alexandrinum (Verma et al., 2015), and 36 SSR markers of Vicia spp. (Raveendar et al., 2015). Estimated PIC is usually directly connected with suitability for subsequent utilization, such as in variety identification or selection of suitable material for breeding purposes. In order to calculate PIC, a precise determination of allelic frequencies in the studied population is required.
To overcome the disadvantages of pooled samples, we proposed a modified PIC value termed pooled polymorphic information content (pPIC) which does not rely on determining allelic frequencies in the selected population. pPIC ranges from 0 to 1, similarly as does PIC, and it estimates the probability that two randomly collected pooled samples from the given species will differ in the given marker. Unlike PIC, pPIC works well for assessing the polymorphism level of a marker locus for pooled samples. The presented pPIC of the 95 SSR markers analyzed should, however, be taken into account only to evaluate pooled samples similar in size to that of our study. A significant decrease or increase in pooled sample size could shift pPIC and thus degrade the estimation of SSR marker discrimination power. Our results based on a pooled sample size of 16 plants should nevertheless be optimal for most potential subsequent utilizations. This is particularly important for screening gene bank accessions and large-scale analysis of cultivar identity and seed purity. For red clover, moreover, the optimal bulk size for genetic variation assessment among cultivars has been determined as 20 (Kongkiatngam et al., 1996).
This study generated a collection of 22 highly polymorphic SSRs with pPIC >0.9 and thus primer pairs for application to diversity studies in T. pratense. Seventy-two SSRs out of 95 validated showed pPIC >0.5. The single SSR marker in coding sequences SSR-TP_g20700.t1.cds3 was amplified in all 50 varieties but was also monomorphic (pPIC = 0.0). All other SSRs revealed some polymorphism in the analyzed variety populations. For the validated SSRs, the actual length range of amplified fragments corresponded with expectations with the single exception of SSR-TP_g32548.t1.cds1, whose amplified fragment was shorter (100–200 bp) than expected (237 bp).
The breeding methods in red clover include procedures suitable for outcrossing crops. Useful variation in a breeding population can be generated through hybridization and genome introgression, or by chromosome doubling (polyploidy) by colchicine. Subsequent phenotypic selection of superior individual plants or mass selection must be conducted on the progeny combining the best traits, and successive population breeding is performed. Molecular characterization of the analyzed varieties using SSRs reflects their genetic relationships, and the grouping is shown in Figure 7 and Figure S1. Tracing the breeding history revealed frequent sharing and exchange of cultivars and newly bred materials among European breeding stations. It was shown that varieties from sub-clusters IA, IIA, IIB1, and IIB2-1 had higher relatedness than varieties from sub-cluster IIB2-2. The possible reasons could be (i) introgressions from landraces and (ii) that the varieties were mostly released from 1970 to 1990, Chlumecky as early as 1935 (with the exceptions of Atlantis and Slavoj). Narrowing of the genetic base in the more recent varieties in sub-cluster IIB2-2 was also apparent.
The SSR profiling (i) differentiated varieties with possible introgressions from landraces and (ii) indicated the existence of diversity at the molecular level among different red clover varieties. The finding of inter-variety heterogeneity has important consequences for breeders who use these varieties. Cluster analysis by means of DNA profiling using the validated SSR set is suitable for such study.
Further progress in red clover breeding can be made by crosses with more distant genotypes as sources of new genetic variability, with new introgressions of important loci for resistance and quality. The identification of SSR or SNP markers in known-function genes linked to specific traits can facilitate marker-assisted selection. One important task is to develop a platform for red clover genotyping, employing genome-wide distributed SNP markers. The Tatra-derived reference sequence was initially used for the detection of the predicted 343 thousand SNPs. We used a preliminary set of 8,623 genome-wide distributed SNPs for polymorphism evaluation in individual plants. Arrayit methods provide universal microarray-based platforms for SNP genotyping (Schena et al., 1996). Sixteen of the validated SNPs were monomorphic and 8,607 were polymorphic with a mean PIC of 0.338. SNP validation confirmed the high quality of SNPs chosen for microarray. More sequenced red clover varieties/genotypes and a large set of informative SNPs are greatly needed for genotyping and association study. NGS methods such as genotyping-by-sequencing and the resequencing of targeted DNA regions from contrasting genotypes appear to be the most essential for SNP discovery and genotyping applications in red clover breeding. Temperature switch PCR can be successfully used in diagnostic applications through single-marker SNP genotyping for targeted coding sequences and for heterozygosity or homozygosity confirmation in validated loci. Large SNP sets are already available in grain legumes such as soybean (Song et al., 2013; Lee et al., 2015) and pea (Sindhu et al., 2014; Tayeh et al., 2015), or in peanut (Pandey et al., 2017). High-density SNP microarrays can significantly advance breeding applications.
JI, JN, and JŘ designed the study. JI processed sequencing data, characterized protein-coding genes, and collaboratively with JD performed detailed inspection of gene annotation manually. JI performed gene classification into metabolic and biosynthetic pathways, comparison with other legumes, and generated genome-wide SSR and SNP markers. LP and JD prepared biological material, performed DNA isolation and marker validation. JD and PD performed pPIC and PIC calculation, polymorphism evaluation and phylogenetic analysis. JR supervised all aspects of the presented analyses. All of the authors contributed to the writing of the manuscript.
The authors declare that the research was conducted in the absence of any commercial or financial relationships that could be construed as a potential conflict of interest.
The authors thank the Ministry of Agriculture of the Czech Republic (grant no. QI111A019) for financial support. Computational resources were provided by the CESNET LM2015042 and the CERIT Scientific Cloud LM2015085, provided under the programme “Projects of Large Research, Development, and Innovations Infrastructures.” Seeds were procured from the GeneBank of Crop Research Institute Ltd., Prague-Ruzyně, Czech Republic.
The Supplementary Material for this article can be found online at: http://journal.frontiersin.org/article/10.3389/fpls.2017.00367/full#supplementary-material
Adams, N. R. (1995). Detection of the effects of phytoestrogens on sheep and cattle. J. Anim. Sci. 73, 1509–1515. doi: 10.2527/1995.7351509x
Ashrafi, H., Hill, T., Stoffel, K., Kozik, A., Yao, J., Chin-Wo, S. R., et al. (2012). De novo assembly of the pepper transcriptome (Capsicum annuum): a benchmark for in silico discovery of SNPs, SSRs and candidate genes. BMC Genomics 13:571. doi: 10.1186/1471-2164-13-571
Blanca, J., Cañizares, J., Roig, C., Ziarsolo, P., Nuez, F., and Picó, B. (2011). Transcriptome characterization and high throughput SSRs and SNPs discovery in Cucurbita pepo (Cucurbitaceae). BMC Genomics 12:104. doi: 10.1186/1471-2164-12-104
Botstein, D., White, R. L., Skolnick, M., and Davis, R. W. (1980). Construction of a genetic linkage map in man using restriction fragment length polymorphisms. Am. J. Hum. Genet. 32, 314–331.
Cidade, F. W., Vigna, B. B., de Souza, F. H., Valls, J. F., Dall'Agnol, M., Zucchi, M. I., et al. (2013). Genetic variation in polyploid forage grass: assessing the molecular genetic variability in the Paspalum genus. BMC Genet. 14:50. doi: 10.1186/1471-2156-14-50
da Maia, L. C., Palmieri, D. A., De Souza, V. Q., Kopp, M. M., de Carvalho, F. I. F., and Costa de Oliveira, A. (2008). SSR Locator: tool for simple sequence repeat discovery integrated with primer design and PCR simulation. Int. J. Plant Genomics 2008:412696. doi: 10.1155/2008/412696
De Vega, J. J., Ayling, S., Hegarty, M., Kudrna, D., Goicoechea, J. L., Ergon, Å., et al. (2015). Red clover (Trifolium pratense L.) draft genome provides a platform for trait improvement. Sci. Rep. 5:17394. doi: 10.1038/srep17394
Dellaporta, S. L., Wood, J., and Hicks, J. B. (1983). A plant DNA minipreparation: version II. Plant Mol. Biol. Report. 1, 19–21. doi: 10.1007/BF02712670
Dice, L. R. (1945). Measures of the amount of ecologic association between species. Ecology 26, 297. doi: 10.2307/1932409
Durand, J., Bodénès, C., Chancerel, E., Frigerio, J.-M., Vendramin, G., Sebastiani, F., et al. (2010). A fast and cost-effective approach to develop and map EST-SSR markers: oak as a case study. BMC Genomics 11:570. doi: 10.1186/1471-2164-11-570
Forster, J. W., Jones, E. S., Kölliker, R., Drayton, M. C., Dupal, M. P., Guthridge, K. M., et al. (2001). “Application of DNA profiling to an outbreeding forage species,” in Plant Genotyping: The DNA Fingerprinting of Plants, ed R. J. Henry (Wallingford: CABI), 299–320. Available online at: http://www.cabi.org/cabebooks/ebook/20083015002 (Accessed February 9, 2016).
Ghamkhar, K., Isobe, S., Nichols, P. G. H., Faithfull, T., Ryan, M. H., Snowball, R., et al. (2012). The first genetic maps for subterranean clover (Trifolium subterraneum L.) and comparative genomics with T. pratense L. and Medicago truncatula Gaertn. to identify new molecular markers for breeding. Mol. Breed. 30, 213–226. doi: 10.1007/s11032-011-9612-8
Graham, P. H., and Vance, C. P. (2003). Legumes: importance and constraints to greater use. Plant Physiol. 131, 872–877. doi: 10.1104/pp.017004
Herrmann, D., Boller, B., Studer, B., Widmer, F., and Kölliker, R. (2008). Improving persistence in red clover: insights from QTL analysis and comparative phenotypic evaluation. Crop Sci. 48:269. doi: 10.2135/cropsci2007.03.0143
Isobe, S., Kölliker, R., Hisano, H., Sasamoto, S., Wada, T., Klimenko, I., et al. (2009). Construction of a consensus linkage map for red clover (Trifolium pratense L.). BMC Plant Biol. 9:57. doi: 10.1186/1471-2229-9-57
Isobe, S. N., Hisano, H., Sato, S., Hirakawa, H., Okumura, K., Shirasawa, K., et al. (2012). Comparative genetic mapping and discovery of linkage disequilibrium across linkage groups in white clover (Trifolium repens L.). G3 2, 607–617. doi: 10.1534/g3.112.002600
Ištvánek, J., Jaroš, M., Křenek, A., and Řepková, J. (2014). Genome assembly and annotation for red clover (Trifolium pratense; Fabaceae). Am. J. Bot. 101, 327–337. doi: 10.3732/ajb.1300340
Jaccard, P. (1901). Étude comparative de la distribution florale dans une portion des Alpes et du Jura. Bull. Soc. Vaudoise Sci. Nat. 37, 547–579. doi: 10.5169/seals-266450
Jakešová, H., Řepková, J., Nedělník, J., Hampel, D., Dluhošová, J., Soldánová, M., et al. (2015). Selecting plants with increased total polyphenol oxidases in the genus Trifolium. Czech J. Genet. Plant Breed. 51, 155–161. doi: 10.17221/107/2015-CJGPB
Jones, B. A., Hatfield, R. D., and Muck, R. E. (1995). Screening legume forages for soluble phenols, polyphenol oxidase and extract browning. J. Sci. Food Agric. 67, 109–112. doi: 10.1002/jsfa.2740670117
Kataoka, R., Hara, M., Kato, S., Isobe, S., Sato, S., Tabata, S., et al. (2012). Integration of linkage and chromosome maps of red clover (Trifolium pratense L.). Cytogenet. Genome Res. 137, 60–69. doi: 10.1159/000339509
Klimenko, I., Razgulayeva, N., Gau, M., Okumura, K., Nakaya, A., Tabata, S., et al. (2010). Mapping candidate QTLs related to plant persistency in red clover. Theor. Appl. Genet. 120, 1253–1263. doi: 10.1007/s00122-009-1253-5
Kongkiatngam, P., Waterway, M. J., Coulman, B. E., and Fortin, M. G. (1996). Genetic variation among cultivars of red clover (Trifolium pratense L.) detected by RAPD markers amplified from bulk genomic DNA. Euphytica 89, 355–361.
Krzywinski, M., Schein, J., Birol, I., Connors, J., Gascoyne, R., Horsman, D., et al. (2009). Circos: an information aesthetic for comparative genomics. Genome Res. 19, 1639–1645. doi: 10.1101/gr.092759.109
Kulikova, O., Geurts, R., Lamine, M., Kim, D.-J., Cook, D. R., Leunissen, J., et al. (2004). Satellite repeats in the functional centromere and pericentromeric heterochromatin of Medicago truncatula. Chromosoma 113, 276–283. doi: 10.1007/s00412-004-0315-3
Lee, Y. G., Jeong, N., Kim, J. H., Lee, K., Kim, K. H., Pirani, A., et al. (2015). Development, validation and genetic analysis of a large soybean SNP genotyping array. Plant J. 81, 625–636. doi: 10.1111/tpj.12755
Li, H., and Durbin, R. (2010). Fast and accurate long-read alignment with Burrows-Wheeler transform. Bioinformatics 26, 589–595. doi: 10.1093/bioinformatics/btp698
Li, H., Handsaker, B., Wysoker, A., Fennell, T., Ruan, J., Homer, N., et al. (2009). The sequence alignment/map format and SAMtools. Bioinformatics 25, 2078–2079. doi: 10.1093/bioinformatics/btp352
Lynch, M., Bost, D., Wilson, S., Maruki, T., and Harrison, S. (2014). Population-genetic inference from pooled-sequencing data. Genome Biol. Evol. 6, 1210–1218. doi: 10.1093/gbe/evu085
Metzgar, D., Bytof, J., and Wills, C. (2000). Selection against frameshift mutations limits microsatellite expansion in coding DNA. Genome Res. 10, 72–80. doi: 10.1101/gr.10.1.72
Mullen, M. P., Creevey, C. J., Berry, D. P., McCabe, M. S., Magee, D. A., Howard, D. J., et al. (2012). Polymorphism discovery and allele frequency estimation using high-throughput DNA sequencing of target-enriched pooled DNA samples. BMC Genomics 13:16. doi: 10.1186/1471-2164-13-16
Novaes, E., Drost, D. R., Farmerie, W. G., Pappas, G. J. Jr., Grattapaglia, D., Sederoff, R. R., et al. (2008). High-throughput gene and SNP discovery in Eucalyptus grandis, an uncharacterized genome. BMC Genomics 9:312. doi: 10.1186/1471-2164-9-312
Pandey, M. K., Agarwal, G., Kale, S. M., Clevenger, J., Nayak, S. N., Sriswathi, M., et al. (2017). Development and evaluation of a high density genotyping 'Axiom_Arachis' array with 58 K SNPs for accelerating genetics and breeding in groundnut. Sci. Rep. 7:40577. doi: 10.1038/srep40577
Park, C. Y., and Weaver, C. M. (2012). Vitamin D interactions with soy isoflavones on bone after menopause: a review. Nutrients 4, 1610–1621. doi: 10.3390/nu4111610
Qi, L. L., Ma, G. J., Long, Y. M., Hulke, B. S., Gong, L., and Markell, S. G. (2015). Relocation of a rust resistance gene R2 and its marker-assisted gene pyramiding in confection sunflower (Helianthus annuus L.). Theor. Appl. Genet. 128, 477–488. doi: 10.1007/s00122-014-2446-0
Raveendar, S., Lee, G.-A., Jeon, Y.-A., Lee, Y. J., Lee, J.-R., Cho, G.-T., et al. (2015). Cross-amplification of Vicia sativa subsp. sativa microsatellites across 22 other Vicia species. Molecules 20, 1543–1550. doi: 10.3390/molecules20011543
Ritchie, M. E., Phipson, B., Wu, D., Hu, Y., Law, C. W., Shi, W., et al. (2015). limma powers differential expression analyses for RNA-sequencing and microarray studies. Nucleic Acids Res. 43:e47. doi: 10.1093/nar/gkv007
Rogers, S. O., and Bendich, A. J. (1989). “Extraction of DNA from plant tissues,” in Plant Molecular Biology Manual, eds S. B. Gelvin, R. A. Schilperoort, and D. P. S. Verma (Dordrecht: Springer), 73–83. Available online at: http://www.springerlink.com/index/10.1007/978-94-009-0951-9_6 (Accessed February 9, 2016).
RStudio Team (2015). RStudio: Integrated Development for R. Boston, MA: RStudio, Inc. Available online at: http://www.rstudio.com/ (Accessed February 6, 2017).
Sato, S., Isobe, S., Asamizu, E., Ohmido, N., Kataoka, R., Nakamura, Y., et al. (2005). Comprehensive structural analysis of the genome of red clover (Trifolium pratense L.). DNA Res. 12, 301–364. doi: 10.1093/dnares/dsi018
Sato, S., Nakamura, Y., Kaneko, T., Asamizu, E., Kato, T., Nakao, M., et al. (2008). Genome structure of the legume, Lotus japonicus. DNA Res. 15, 227–239. doi: 10.1093/dnares/dsn008
Schena, M., Shalon, D., Heller, R., Chai, A., Brown, P. O., and Davis, R. W. (1996). Parallel human genome analysis: micromicroarray-based expression monitoring of 1,000 genes. Proc. Natl. Acad. Sci. U.S.A. 93, 10614–10619.
Schmutz, J., Cannon, S. B., Schlueter, J., Ma, J., Mitros, T., Nelson, W., et al. (2010). Genome sequence of the palaeopolyploid soybean. Nature 463, 178–183. doi: 10.1038/nature08670
Shrivastava, D., Verma, P., and Bhatia, S. (2014). Expanding the repertoire of microsatellite markers for polymorphism studies in Indian accessions of mung bean (Vigna radiata L. Wilczek). Mol. Biol. Rep. 41, 5669–5680. doi: 10.1007/s11033-014-3436-7
Sindhu, A., Ramsay, L., Sanderson, L. A., Stonehouse, R., Li, R., Condie, J., et al. (2014). Gene-based SNP discovery and genetic mapping in pea. Theor. Appl. Genet. 127, 2225–2241. doi: 10.1007/s00122-014-2375-y
Song, Q., Hyten, D. L., Jia, G., Quigley, C. V., Fickus, E. W., Nelson, R. L., et al. (2013). Development and evaluation of SoySNP50K, a high-density genotyping array for soybean. PLoS ONE 8:e54985. doi: 10.1371/journal.pone.0054985
Sørenson, T. (1948). A Method of Establishing Groups of Equal Amplitude in Plant Sociology Based on Similarity of Species Content and Its Application to Analyses of the Vegetation on Danish Commons. I kommission hos E. Munksgaard. Available online at: https://books.google.co.in/books?id=rpS8GAAACAAJ
Stasolla, C., Katahira, R., Thorpe, T. A., and Ashihara, H. (2003). Purine and pyrimidine nucleotide metabolism in higher plants. J. Plant Physiol. 160, 1271–1295. doi: 10.1078/0176-1617-01169
Tabone, T., Mather, D. E., and Hayden, M. J. (2009). Temperature switch PCR (TSP): robust assay design for reliable amplification and genotyping of SNPs. BMC Genomics 10:580. doi: 10.1186/1471-2164-10-580
Tayeh, N., Aluome, C., Falque, M., Jacquin, F., Klein, A., Chauveau, A., et al. (2015). Development of two major resources for pea genomics: the GenoPea 13.2K SNP array and a high-density, high-resolution consensus genetic map. Plant J. 84, 1257–1273. doi: 10.1111/tpj.13070
Teuscher, E., and Lindequist, U. (2010). Biogene Gifte: Biologie, Chemie, Pharmakologie, Toxikologie, 3 neu bearb. Stuttgart: Wiss. Verl.-Ges.
Torales, S. L., Rivarola, M., Pomponio, M. F., Fernández, P., Acuña, C. V., Marchelli, P., et al. (2012). Transcriptome survey of Patagonian southern beech Nothofagus nervosa (= N. alpina): assembly, annotation and molecular marker discovery. BMC Genomics 13:291. doi: 10.1186/1471-2164-13-291
Torales, S. L., Rivarola, M., Pomponio, M. F., Gonzalez, S., Acuña, C. V., Fernández, P., et al. (2013). De novo assembly and characterization of leaf transcriptome for the development of functional molecular markers of the extremophile multipurpose tree species Prosopis alba. BMC Genomics 14:705. doi: 10.1186/1471-2164-14-705
Ueno, S., Le Provost, G., Léger, V., Klopp, C., Noirot, C., Frigerio, J.-M., et al. (2010). Bioinformatic analysis of ESTs collected by Sanger and pyrosequencing methods for a keystone forest tree species: oak. BMC Genomics 11:650. doi: 10.1186/1471-2164-11-650
Varshney, R. K., Ribaut, J.-M., Buckler, E. S., Tuberosa, R., Rafalski, J. A., and Langridge, P. (2012). Can genomics boost productivity of orphan crops? Nat. Biotechnol. 30, 1172–1176. doi: 10.1038/nbt.2440
Varshney, R. K., Song, C., Saxena, R. K., Azam, S., Yu, S., Sharpe, A. G., et al. (2013). Draft genome sequence of chickpea (Cicer arietinum) provides a resource for trait improvement. Nat. Biotechnol. 31, 240–246. doi: 10.1038/nbt.2491
Verma, P., Chandra, A., Roy, A. K., Malaviya, D. R., Kaushal, P., Pandey, D., et al. (2015). Development, characterization and cross-species transferability of genomic SSR markers in berseem (Trifolium alexandrinum L.), an important multi-cut annual forage legume. Mol. Breed. 35, 1–14. doi: 10.1007/s11032-015-0223-7
Víquez-Zamora, M., Vosman, B., van de Geest, H., Bovy, A., Visser, R. G., Finkers, R., et al. (2013). Tomato breeding in the genomics era: insights from a SNP array. BMC Genomics 14:354. doi: 10.1186/1471-2164-14-354
Vižintin, L., Javornik, B., and Bohanec, B. (2006). Genetic characterization of selected Trifolium species as revealed by nuclear DNA content and ITS rDNA region analysis. Plant Sci. 170, 859–866. doi: 10.1016/j.plantsci.2005.12.007
Wink, M. (2013). Evolution of secondary metabolites in legumes (Fabaceae). South Afr. J. Bot. 89, 164–175. doi: 10.1016/j.sajb.2013.06.006
Yates, S. A., Swain, M. T., Hegarty, M. J., Chernukin, I., Lowe, M., Allison, G. G., et al. (2014). De novo assembly of red clover transcriptome based on RNA-Seq data provides insight into drought response, gene discovery and marker identification. BMC Genomics 15:453. doi: 10.1186/1471-2164-15-453
Younas, M., Xiao, Y., Cai, D., Yang, W., Ye, W., Wu, J., et al. (2012). Molecular characterization of oilseed rape accessions collected from multi continents for exploitation of potential heterotic group through SSR markers. Mol. Biol. Rep. 39, 5105–5113. doi: 10.1007/s11033-011-1306-0
Young, N. D., Debellé, F., Oldroyd, G. E., Geurts, R., Cannon, S. B., Udvardi, M. K., et al. (2011). The Medicago genome provides insight into the evolution of rhizobial symbioses. Nature 480, 520–524. doi: 10.1038/nature10625
Yu, H., Xie, W., Li, J., Zhou, F., and Zhang, Q. (2014). A whole-genome SNP array (RICE6K) for genomic breeding in rice. Plant Biotechnol. J. 12, 28–37. doi: 10.1111/pbi.12113
Zalapa, J. E., Cuevas, H., Zhu, H., Steffan, S., Senalik, D., Zeldin, E., et al. (2012). Using next-generation sequencing approaches to isolate simple sequence repeat (SSR) loci in the plant sciences. Am. J. Bot. 99, 193–208. doi: 10.3732/ajb.1100394
Zhao, P., Zhang, G., Wu, X., Li, N., Shi, D., Zhang, D., et al. (2013). Fine mapping of RppP25, a southern rust resistance gene in maize. J. Integr. Plant Biol. 55, 462–472. doi: 10.1111/jipb.12027
Keywords: biosynthetic pathways, genetic diversity, sequencing, SNP, specific genes, SSR
Citation: Ištvánek J, Dluhošová J, Dluhoš P, Pátková L, Nedělník J and Řepková J (2017) Gene Classification and Mining of Molecular Markers Useful in Red Clover (Trifolium pratense) Breeding. Front. Plant Sci. 8:367. doi: 10.3389/fpls.2017.00367
Received: 19 November 2016; Accepted: 01 March 2017;
Published: 22 March 2017.
Edited by:
Diego Rubiales, Instituto de Agricultura Sostenible (CSIC), SpainReviewed by:
Leif Skot, Aberystwyth University, UKCopyright © 2017 Ištvánek, Dluhošová, Dluhoš, Pátková, Nedělník and Řepková. This is an open-access article distributed under the terms of the Creative Commons Attribution License (CC BY). The use, distribution or reproduction in other forums is permitted, provided the original author(s) or licensor are credited and that the original publication in this journal is cited, in accordance with accepted academic practice. No use, distribution or reproduction is permitted which does not comply with these terms.
*Correspondence: Jana Řepková, cmVwa292YUBzY2kubXVuaS5jeg==
Disclaimer: All claims expressed in this article are solely those of the authors and do not necessarily represent those of their affiliated organizations, or those of the publisher, the editors and the reviewers. Any product that may be evaluated in this article or claim that may be made by its manufacturer is not guaranteed or endorsed by the publisher.
Research integrity at Frontiers
Learn more about the work of our research integrity team to safeguard the quality of each article we publish.