- Agriculture and Environmental Sciences, School of Biosciences, University of Nottingham, Loughborough, United Kingdom
The crop ideotype was first defined by Donald in 1968 as an idealised structural model of a plant which can theoretically attain maximum yield quantity and quality within a given environment. Many of the major crop species have proposed ideotypes. For example, in wheat (Triticum aestivum), suggested characteristics include a short strong stem, few erect, small leaves and large ears. Breeding for crop ideotypes is a continuous process as beneficial traits are updated to match current needs. Government organisations such as the Agriculture and Horticulture Development Board (AHDB) in the United Kingdom, publish a recommended list of varieties each year, and so we hypothesise that these recommended varieties will express traits of the ideotype. However, through greenhouse experiments, we show that three recent AHDB recommended wheat varieties differ to that of the ideotype and to each other. This either indicates that the concept may not be universal, or the main target for breeding programmes; that traits may not have successfully been bred yet; or, that other conflicting reasons may be preventing the expression of the ideotype. To this end, we review the factors that may influence plant structure before discussing new tools and methods that can aid ideotype design. Through this paper, we argue that whilst there are benefits to the concept of the ideotype, the ‘power’ of the concept is lost when integrating the multitude of factors that, in combination, determine plant structure and function in a given environment. Nevertheless, regardless of these limitations, we argue that there is still a role for the ideotype in crop breeding, but that this concept needs to be expanded to emphasise the genetic and environmental interactions that influence plant physiology.
Introduction
Food security continues to be one of the greatest challenges faced by humankind. This is confounded by the impacts of climate change, the COVID-19 pandemic and states of war, which have contributed to further instability in food production and export (FAO et al., 2022; Albaladejo Román, 2023). To combat these challenges, both an increase in food production and the implementation of local and sustainable food systems is needed (Albaladejo Román, 2023).
A crop ideotype is defined as an idealised structural model, combining both morphological and physiological traits, with the potential to maximise yield and quality within a defined environment (Donald, 1968). The ideotype approach was designed to overcome limitations traditionally experienced by breeders during selection. Primarily, the ideotype approach relies on defining a theoretically efficient, or ideal, plant type, and then it is bred by targeting all of the component traits that define it. Ideotype breeding can complement traditional breeding approaches resulting in an increase in genetic variation (Reynolds et al., 2009). As the end goal is defined for different locations and situations, characteristics of the ideotype are expected to vary across different environments, but generally include factors that increase the survivability of the plant such as drought resistance, resource use efficiency, root architecture and disease resistance (Arraiano et al., 2006; Greenland et al., 2017; Rezzouk et al., 2022).
Elite crop varieties have been created that combine relatively low-frequency alleles which are of known agronomic importance (Lyu et al., 2013). These elite varieties can be targeted to different main objectives, such as yield, product quality, resistance to abiotic and biotic stresses and agronomic suitability. Elite varieties are often recommended by government organisations. For example, in the United Kingdom, the Agriculture and Horticulture Development Board (AHDB) publishes yearly recommendations for cereal and oil seed crops (AHDB, 2023), whilst the British Beet Research Organisation (BBRO) publishes a recommended list for sugar beet varieties (BBRO, 2023). Over time, new varieties are added to the list while older varieties are removed. As such, recommended high yielding elite varieties are expected to exhibit traits of the ideotype, and thus, for a given environment, varieties should not display marked differences in appearance and characteristics.
Within this paper, we will first discuss the different traits that encompass the ideotype for a variety of crop species, with a predominant focus on cereals. We then present a case study using UK-recommended varieties to explore whether or not they exhibit the ideotype traits proposed by Donald (1968). We discuss conflicting factors that may impact the display of ideotype characteristics before finally discussing the future of the ideotype in agricultural production and the tools that can aid ideotype design.
Proposed crop ideotypes
Wheat (Triticum aestivum)
The first wheat ideotype was proposed by Donald (1968) and consists of seven main features: 1) a short strong stem, 2) erect leaves, 3) few and small leaves, 4) large ears, 5) erect ears, 6) the presence of awns, and 7) a single culm. The short stature of the proposed wheat and rice ideotypes arises from the introduction of dwarfing genes during the Green revolution (see section below; (Hedden, 2003; Pearce et al., 2011). Since the initial ideotype proposal, multiple studies have corroborated Donald’s previous assessment indicating that features such as leaf erectness, or reduced leaf area and height result in higher yield potential (Bugbee and Koerner, 1997; Singh et al., 2001; Srinivasan et al., 2017; Richards et al., 2019). In 2011, the Wheat Yield Consortium published an updated set of physiological traits to promote yield improvement. These traits include increased photosynthetic productivity; maximised spike fertility; improved grain filling and size; optimised partitioning to the grain and; lodging resistance (Foulkes and Murchie, 2011; Parry et al., 2011; Reynolds et al., 2011).
Rice (Oryza sativa)
Historically, rice breeding has experienced a number of key yield improvement steps. This firstly arose with the introduction of dwarfing genes in the 1950s, followed by hybrid crossing between high-yielding japonica and indica crosses (Peng et al., 2008). However following these initial breakthroughs, rice yield began to stagnate. These previous varieties were characterised by high tillering capacity with mainly unproductive tillers, small panicles and lodging susceptibility (Khush, 1995; Peng et al., 2008; Rahangdale et al., 2019). To overcome these limitations, the rice ideotype arose from the concept of breeding a New Plant Type (NPT) (Peng et al., 2008). This NPT shared some characteristics in common with the wheat ideotype including: upright leaves, a low tiller number and a strong culm, as well as large panicles that sit below the top three leaves, and a high harvest index.
Ideotypes have also been proposed depending on the type of rice under cultivation (Parthasarathi et al., 2012; Sharma et al., 2013; Paleari et al., 2017). For example, aerobic rice should combine the improved yield performance of lowland varieties, with the increase drought tolerance of highland varieties. This requires optimisation of maximum root- length, volume and thickness; weed competitiveness and seedling vigour; delayed flowering; cold tolerance; and altered soluble protein and pigment content (Parthasarathi et al., 2012). Additionally, these new ideotypes should be tailored according to local environmental conditions (Paleari et al., 2017; Rahangdale et al., 2019).
Maize (Zea mays)
One of the first maize ideotypes was proposed by Mock and Pearce (1975) targeting optimal growth environments. This encompasses adequate moisture, temperature, fertility, higher planting densities and early planting date. Under these conditions, Mock and Pearce (1975) identified traits such as a stiff, vertically orientated leaves above the ear but horizontal leaves below; short interval between pollen shed and silk emergence; small tassel size; maximised grain filling period; and slow leaf senescence. They also identified a set of physiological traits including cold tolerance; photoperiod insensitivity; maximum photosynthetic efficiency; and efficient conversion of photosynthate to the grain.
The maize ideotype has since been updated to focus both on production under diverse climates and to account for rooting traits (Mi et al., 2010; Cairns et al., 2012; Lynch, 2013; Perego et al., 2014; Mi et al., 2016; Xiao et al., 2020). To enhance both water and nitrogen use efficiency, root traits that permit rapid exploitation of deep soil levels are expected to be most beneficial. This includes features such as a large diameter primary root with few but long lateral roots; abundant branching or long root hairs; a single whorl of brace roots; and rapid response to localised soil regions of high nutrient supply (Mi et al., 2010; Lynch, 2013; Mi et al., 2016). Process-based crop models have also been used to identify beneficial traits for future climate scenarios (see section below on use of modelling approaches to aid ideotype design) (Perego et al., 2014; Xiao et al., 2020). For example, for future maize cultivation in the North China Plain, ideotypes should have a longer reproductive growing period, faster grain filling rate, high maximum grain number and high radiation use efficiency (Xiao et al., 2020).
Oilseed Brassica species
Oilseed Brassica breeding has been confounded by the complexity of polygenic inheritance of yield traits, strong genotype – environment interactions, and disparity in yield when grown under different planting densities (Thurling, 1991). Therefore, the ideotype concept provided means to focus on morphological features that confer yield improvement. Early proposed ideotypes for spring oilseed rape (OSR; Brassica napus) grown in Western Australia and Tasmania were targeted at known heritable traits including optimised flowering time, apetalous flowers, long upright pods and pod shattering resistance (Thurling, 1991). Seed number per pod (SNPP), pod number and seed weight are considered critical components of yield in OSR (Yang et al., 2017). Therefore a more recent study indicates additional ideotype traits including a limited number of inflorescences but with a good number of ovules and pods on the main inflorescence; long valves with high SNPP; and increased flower number for winter OSR varieties (Siles et al., 2020).
For late sown Indian mustard (Brassica juncea), the ideotype was proposed to consist of dark green leaves with high chlorophyll content and low proline content, large overall plant height and a high number of primary branches with moderate seed weight (Kumar and Srivastava, 2003).
Do recommended elite varieties represent the ideotype?
Case study: UK elite wheat varieties
Wheat accounts for around 8% of all global food production and consumption (FAO, 2022), therefore presents an important target for yield improvement and ideotype breeding. In the UK, wheat is the largest land user of any crop with approximately 1.8 million hectares under cultivation in 2021 (FAOSTAT, 2023). For UK production, both spring and winter wheat varieties are often chosen based on the recommended list produced by the AHDB, which features lists for all cereal and oilseed species (AHDB, 2023). The AHDB recommended list for Spring wheat features up to 10 varieties per year grouped into nabim group 1, nabim group 2 and Group 4 based upon their end use: designated for milling and baking or primarily for feed. Therefore, to test whether the recommended elite varieties exhibit morphological traits that are representative to that of the ideotype, three varieties were chosen based on accessibility to seed, previous experience of cultivation at the University of Nottingham, and period that they were featured on the recommended list. Paragon, was first recommended in 1999 and last recommended for the 2015 to 2016 period, KWS Willow, was first recommended in 2011 and last recommended for the 2019 to 2020 period, and KWS Cochise, was first recommended in 2017 and remains on the recommended for the 2024 to 2025 period (AHDB, 2023). Non-destructive measurements were taken from the above ground structure of each variety including: main shoot height, ear count, leaf and tiller count, leaf area, leaf erectness, leaf chlorophyll content and photosynthetic efficiency (see below for full methods).
There was no significant differences in average heights of the three varieties, with values ranging between ~ 63 (for Paragon) – 68 cm (for Cochise) (Figure 1). Short height (i.e. ~70 cm) is a feature of the wheat ideotype to prevent against lodging; the displacement of plant stems from their vertical orientation (Donald, 1968; Berry et al., 2007; Piñera-Chavez et al., 2016), and the heights of the three varieties of this study fall within the range recommended by the AHDB (AHDB, 2023). Similarly to plant height, there was no significant difference between the ear number of the three varieties with approximately four ears per plant on average.
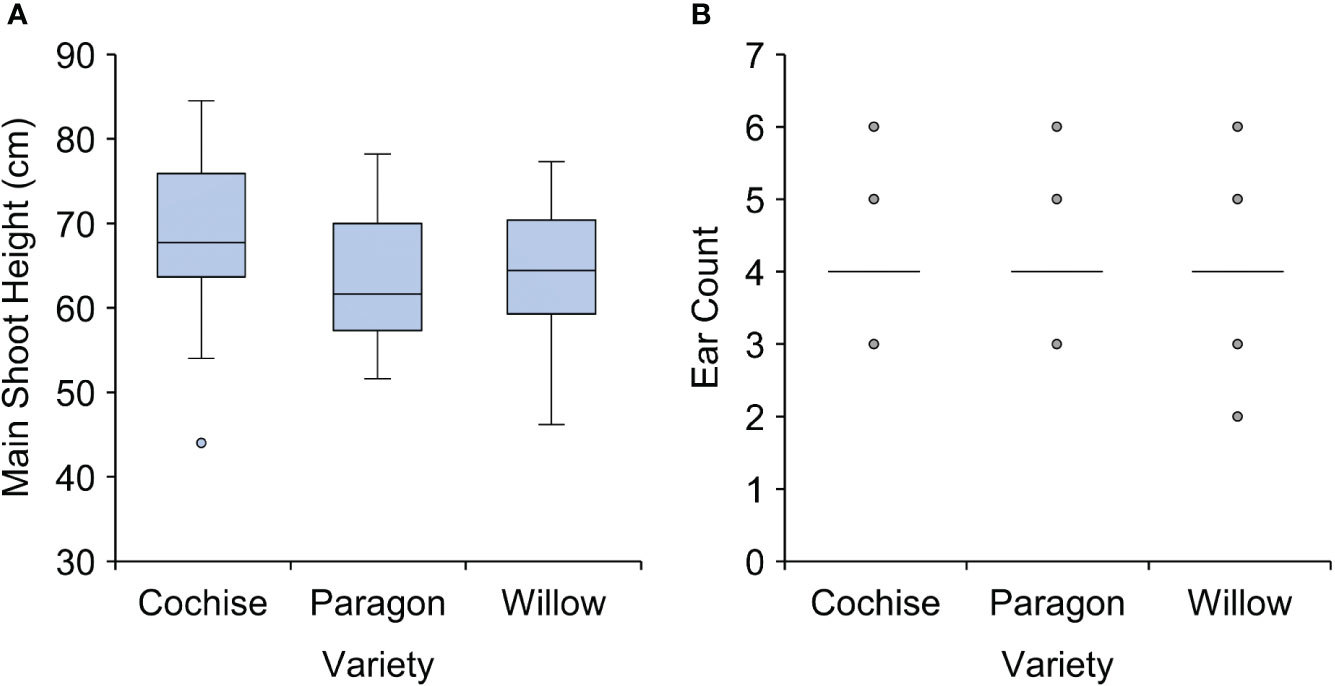
Figure 1 Plant height and ear count of three ADHB recommended elite wheat varieties. (A) main shoot height and (B) ear count. There was no statistically significant difference between the three wheat varieties for main shoot height (p = 0.084) or ear count (p = 0.964).
In contrast with plant height and ear number, leaf count and tiller count were significantly different between varieties, with Willow exhibiting higher counts for both leaves and tillers relative to the other varieties (P<0.01; Figure 2). According to the wheat ideotype presented by Donald (1968), plants should have fewer leaves to maximise light interception. Whilst tiller count is not explicitly mentioned as part of the wheat ideotype, it has been proposed as a desired characteristic for other cereals including rice (Jiao et al., 2010). Furthermore, tiller number is directly proportional to the leaf number, and a reduced tiller count is expected to be beneficial within drier environments so as to maximise yield, thus a reduced tiller count is expected to be more desirable. Out of the three varieties studied here, Cochise and Paragon are closer to the wheat ideotype in terms of leaf and tiller count.
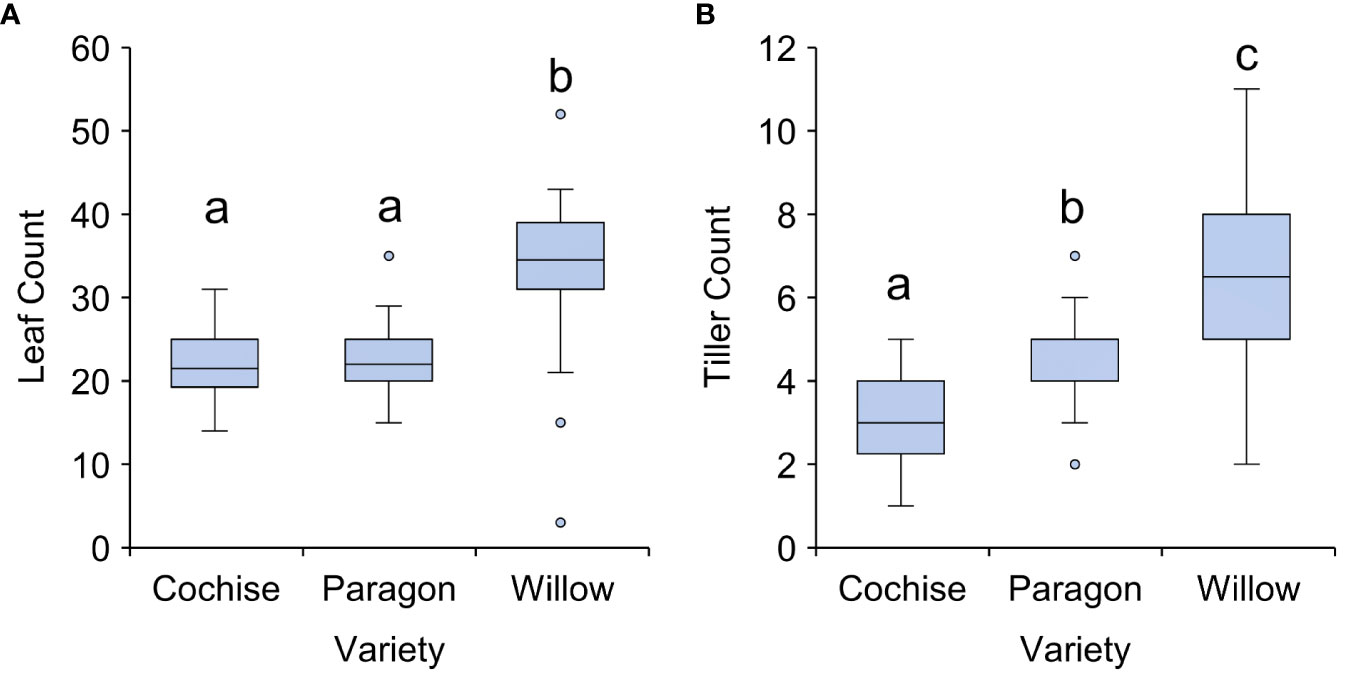
Figure 2 Leaf and tiller count of three ADHB recommended elite wheat varieties. (A) Leaf count and (B) Tiller count. There was a statistically significant difference for both leaf and tiller count (p<0.001). Letters indicate significant difference according to Tukey’s post hoc pairwise comparison test.
The wheat ideotype of Donald (1968) recommended that leaf area should be relatively small. Within this study, Cochise had the smallest mean flag leaf area at 37 cm2, relatively to 47.6 and 52.4 cm2 for Willow and Paragon, respectively (P<0.001; Figure 3A). A similar trend was seen for non-flag leaf area, with Cochise exhibiting the lowest non-flag leaf area (32.8 cm2) followed by Willow (38.9 cm2) then Paragon (46.6 cm2; P<0.001 Figure 3B). Contrary to individual leaf area, Willow exhibits the highest total leaf area relative to the other varieties (P<0.001; Figure 3C). This is a result of a higher overall leaf and tiller number (Figure 2) as leaf area per tiller is not significantly different between each line (P=0.122; Figure 3D). It is important to note that the variation in leaf area witnessed here could be a result of the lower light intensities experienced in the UK environment relative to Australia, where Donald originally defined the ideotype, and thus selective pressure to reduce leaf size is likely to differ. Nevertheless, previous experiments support the benefits of small leaf area in crop yield production (Srinivasan et al., 2017), although increasing emphasis is being placed on increasing flag leaf area to provide a direct source to the forming ears (Carmo-Silva et al., 2017; Senapati et al., 2020; Tanaka et al., 2022).
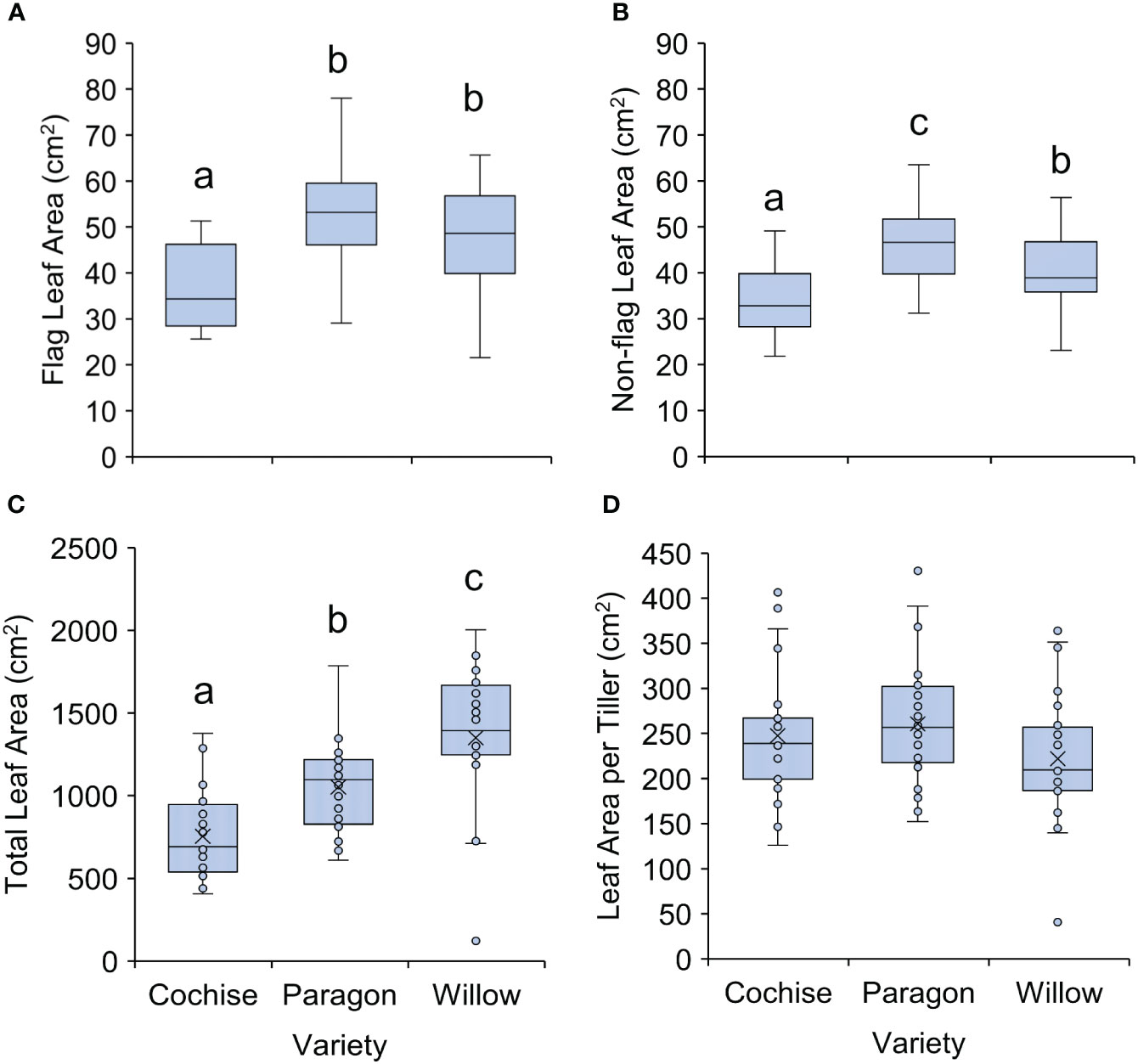
Figure 3 Leaf area of three ADHB recommended elite wheat varieties. (A) Flag leaf area, (B) Non-flag leaf area, (C) total leaf area and (D) leaf area per tiller. There was a statistically significant difference for flag leaf, non-flag leaf and total leaf areas (p<0.001) but non-significant differences in leaf area per tiller. Letters indicate significant difference according to Tukey’s post hoc pairwise comparison test.
Within cereal crops, leaf erectness is a common feature of high yielding varieties. An erect leaf structure permits greater penetration of light to lower canopy layers, unifying photosynthetic rates, and prevents excess heat loading at midday when the sun is directly overhead (Burgess et al., 2016; Richards et al., 2019; Burgess et al., 2021). Of the three tested varieties, Cochise exhibited significantly the most erect canopy (P<0.001), although only 40% of leaves were considered to be erect (Figure 4). It is expected that an erect canopy would have a leaf erectness score closer to one (see methods), however all varieties scored greater above five within this study, and Paragon exhibited the least erect, or more planophile canopy with a score approaching 10. This finding suggests that elite varieties in the UK could be improved further through a more erect leaf stature or there may be conflicting requirements for the UK environment (see section below). Furthermore, heat loading is a less pressing concern in the UK relative to other wheat growing regions.
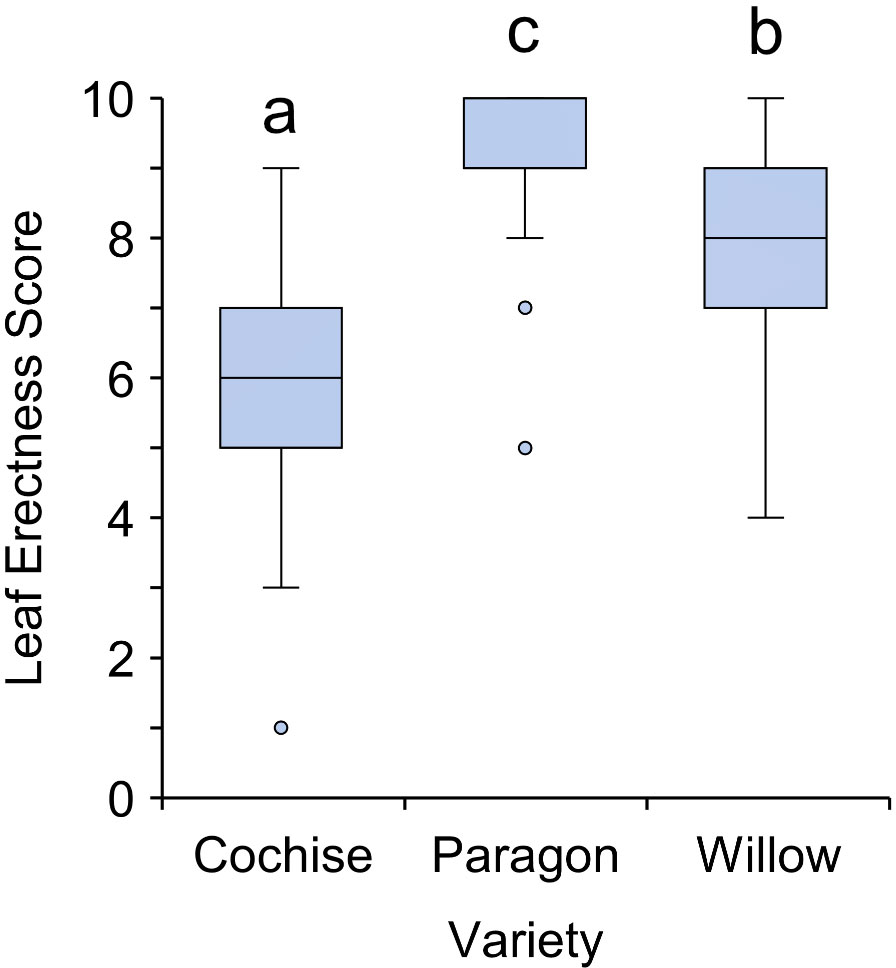
Figure 4 Leaf erectness of three ADHB recommended elite wheat varieties. There was a statistically significant difference for all three varieties (p<0.001). Letters indicate significant difference according to Tukey’s post hoc pairwise comparison test.
Although not explicitly tested within this study, observation indicate that three varieties exhibit differences in relation to susceptibility to pests and diseases. Whilst both Paragon and Cochise were highly susceptible to powdery mildew, in comparison Willow did not exhibit any visible symptoms. This is likely to be a result of genetic resistance, as the denser canopy structure of Willow is expected to generate a humid microenvironment, conducive to powdery mildew infection (Cowger et al., 2012).
Whilst not originally described as part of the wheat ideotype, one final feature of canopy physiology tested within this study was chlorophyll content. Within the last five years, studies have shown that a reduction in chlorophyll content permits a higher photosynthetic efficiency and greater radiation use efficiency whilst conserving nitrogen (Walker et al., 2018). Within this study, chlorophyll content was similar among the three varieties as measured by SPAD (Figure 5A). This translated into similar mean yet significantly different quantum yields between the three varieties with Willow exhibiting the lowest quantum yield of 0.771 and Paragon the highest at 0.786 (Figure 5B).
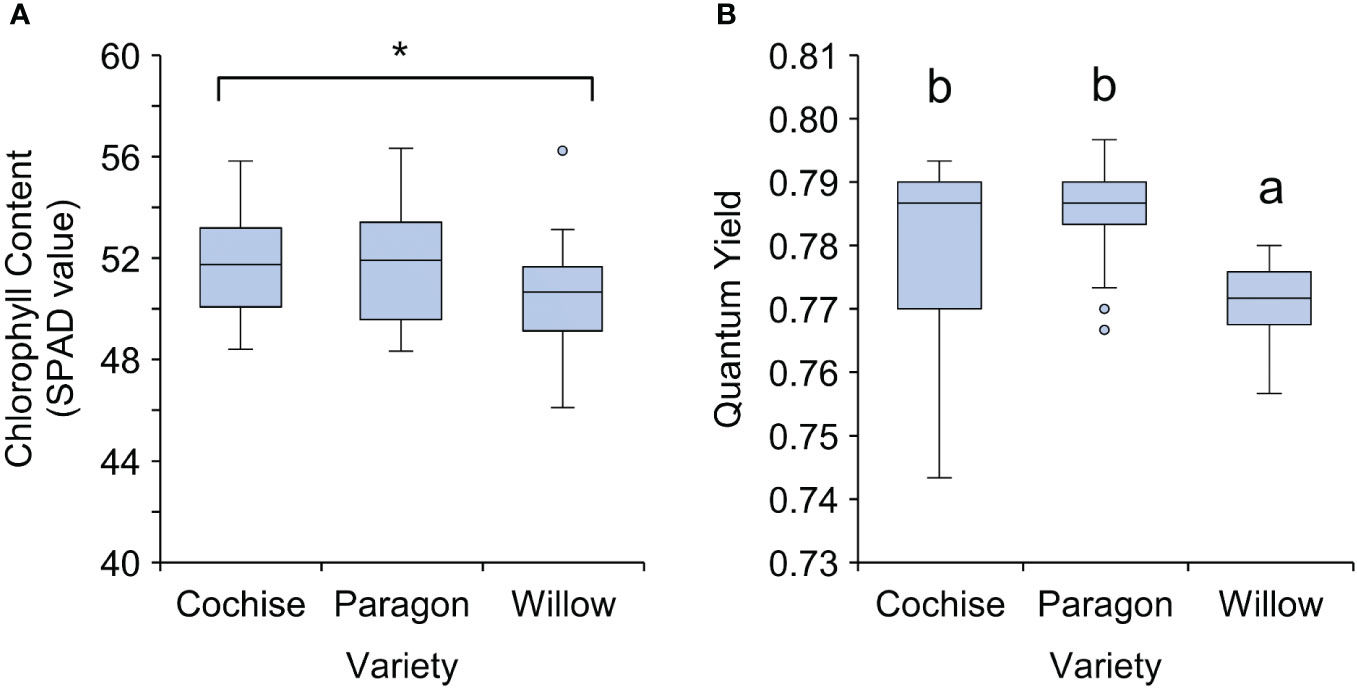
Figure 5 Chlorophyll content and quantum yield of three ADHB recommended elite wheat varieties. (A) Chlorophyll content and (B) Quantum Yield. There was a statistically significant difference for both chlorophyll content (p = 0.049) and quantum yield (p<0.001). Letters indicate significant difference according to Tukey’s post hoc pairwise comparison test. * Significant difference between all three varieties according to anova but nosignificant differences between the 3 according to Tukey’s post hoc.
Together these findings indicate that the three recommended varieties do not comply with the proposed ideotype, nor do they present the same traits as each other. Whilst some features of the ideotype are present, such as reduced plant height, other features exhibit greater variation. For example, leaf and tiller counts as well as canopy erectness exhibit significant variation and diversion away from characteristics that are known to increase yield potential (Berry et al., 2007; Bassu et al., 2011; Richards et al., 2019). Reasons for this may be diverse and some of which are discussed in greater detail in the following sections.
Discussion
Conflicting factors impacting the display of ideotype features
Multiple factors contribute to the plant phenotype. Whilst some structural and physiological traits are determined by genetics, they will also be influenced by environmental conditions and management practices. Furthermore, the genetic underpinning of traits may in themselves be constrained by the breeding process. This results in a large variation of possible crop structures and performances. The following sections will describe some of the conflicting factors that may prevent expression of ideotype features.
Breeding
The domestication process of crops may have placed restrictions on the genetic basis of certain traits. Within cereals, the domestication process targeted a core set of traits collectively known as the ‘domestication syndrome’. These traits include dormancy, seed shattering, branching angle and patterning, internode elongation and biotic and abiotic resistance (Doust, 2007; Abbo et al., 2014; Zhang and Yuan, 2014; Teichmann and Muhr, 2015; Zohary et al., 2015). In comparison to wild relatives, domesticated cereals have a lower amount of vegetative branching, which in turn influenced the structure of the vegetative and influorescent components (Doust, 2007). Whilst the cereals were domesticated independently, molecular techniques indicate that selection was performed upon similar genes or gene pathways (Paterson et al., 1995; Doust, 2007; Meyer and Purugganan, 2013). These changes often resulted in a genome-wide reduction in genetic diversity (Hyten et al., 2006; Haudry et al., 2007; Yang et al., 2019). However, within certain species, such as carrot, einkorn wheat, apples and chicory, genetic diversity has been maintained (Kilian et al., 2007; Cornille et al., 2012; Iorizzo et al., 2013).
The primary goal of most breeding programmes is to achieve an increase in crop yield. However, this does not always occur with conscious selection of traits, but rather their quantitative performance during field trials. Therefore, varieties that feature on recommended lists are not necessarily chosen based on structural or ‘ideotype’ traits but rather their overall performance. In the case of wheat, structural fearures such as reduced height (e.g. Figure 1) may comply with the ideotype as a result of breeding and selection against lodging (Berry et al., 2007; Piñera-Chavez et al., 2016). Historically, reduced plant height was achieved during the green revolution through the introduction of dwarfing genes, including the Reduced height (Rht) genes of wheat and semidwarf 1 (SD1) genes of rice (Hedden, 2003; Pearce et al., 2011). These genes target the production and action of the plant hormone gibberellin (Asano et al., 2011).
Breeding programmes also occur with additional selective pressures in the form of breeder and consumer preference. In most instances, cultural preferences for traits will have evolved under conscious selection (Knüpffer et al., 2003; Grobman et al., 2012). For example, it is a common misconception that a deep green colour is the sign of a productive, or healthy plant. Therefore farmers often prefer plants that look very green, which is an indication of high chlorophyll content. However, recent studies indicate that a reduction in chlorophyll content permits increased radiation use efficiency and photosynthetic productivity (Walker et al., 2018). Similar to greenness, farmers in the UK have continuously selected against the presence of awns in wheat despite the potential benefits associated with water use efficiency, radiation interception, and an increase in grain size (Bort et al., 1994; Motzo and Giunta, 2002; Rebetzke et al., 2016; Sanchez-Bragado et al., 2023). This may partly be a result of the promotion of fungal diseases by awns in wetter environments (Sanchez-Bragado et al., 2023). Therefore, in the UK, historically awns may have been selected against to prevent yield loss associated with fungal diseases. However, as the climate continues to change, most countries in Europe, including the UK, are expected to have dryer environments (Christidis and Stott, 2021). Therefore, awns may no longer represent an undesired characteristic, but their future presentation will depend upon farmer acceptance.
The abiotic and biotic environment
The traits expressed by a plant depend on both underlying genetics and the environment (abiotic and biotic) to which they are exposed. Variation in the local environment may lead to a plant structure that differs from a proposed ideotype. Many crop species are sensitive to environmental conditions including changes in weather patterns and extreme weather events (Porter and Semenov, 2005; Semenov et al., 2014; Senapati et al., 2019). For example, exposure to wind can lead to changes in structure including dwarfing, tissue thickening and, under high wind speeds, breakages (de Langre, 2008). Crops that are cultivated close to the equator are likely to experience similar environmental conditions year round, whereas those further away will have to adapt to seasonal variation. For areas with large variation in environmental variables, yield stability may be of greater importance than yield maximisation (Braun et al., 1992).
Whilst the ideotype concept generally refers to a process to assist with breeding, it can also account for the best crop phenotype to grow in a given environment, under a specific cropping system or for a desired end product (Martre et al., 2015). The original ideotype traits proposed by Donald (Donald, 1968) were targeted at zero- to low- stress environments. Therefore these traits are unlikely to be suitable under moderate- to severe- stress. For example, during water limitation, the impact and extent to which the plant is affected will depend upon the timing, severity and length of the stress period as well as any other interacting stressors. Under these conditions, it is therefore unlikely that a single trait will be of benefit although this is likely to require changes in both the shoot and root system, requiring adaptation of the ideotype to the given environmental conditions (Tardieu, 2012; Greenland et al., 2017; Rezzouk et al., 2022).
Similarly, biotic factors such as the presence of herbivores, weeds, pest and disease, or the risk of promoting it, may also impact the traits exhibited by elite varieties in a given area (Arraiano et al., 2006). Depending upon the type and severity of the biotic stress, this may have structural implications such as leaf curling, discolouration or stunted growth. With intense herbivory pressure, plant architecture may adapt to protect the most vulnerable parts of the plant resulting in ‘cage’ structure (Cadenasso and Pickett, 2000; Charles-Dominique et al., 2017). In regions where biotic stressors are a high risk, certain traits will provide a greater benefit than others. For example, early canopy closure can increase competitiveness against weeds (Kaur et al., 2021), however this may come at the expense of light interception (Burgess et al., 2017; Burgess et al., 2021), or provide a more humid microenvironment. Microclimate features including humidity and reduced air flow as well as increased senescence are known to facilitate the spread of bacterial and fungal disease (Chang et al., 2007; Richard et al., 2012; Tivoli et al., 2013). Therefore the selection of beneficial traits will depend upon the tradeoff between multiple stressors. The impact of biotic pressure is also dependent on the life cycle of the crop species. For example, irrigated rice has a short growth cycle and so are less impacted by weed presence and so a more erect canopy structure is suitable (Ong and Monteith, 1992).
Cultivation and management
Another conflicting factor that will impact the presence of ideotype traits is cropping system and management of a given area. Agronomic practices including soil bed preparation, planting density and the timing of external resource applications will impact the features of the crop. In some instance, this may provide an additional control point to achieve ideotype traits. For example, application of plant growth regulators (PGRs) is common to restrict plant height and achieve uniform growth (Nickell and Louis, 1982). However, in other cases, the combination of management practices used will lead to variation in traits.
Intercropping (sometimes termed mixed cropping, or polyculture) refers to an alternative cropping system in which two or more plants are grown in close proximity during at least part of their lifecycle. Intercropping systems are common to small-holder farming, but due to their potential higher yields and reduced environmental impact, they may provide a sustainable solution for wider-scale agricultural production (Lithourgidis et al., 2011; Maitra et al., 2021; Burgess et al., 2022). However, within such systems, certain negative interactions must be overcome, such as potential interspecific competition.
Most crop varieties were bred for monoculture, and thus are rarely suited to mixed species systems. Within an intercrop, the optimal combination of traits is likely to differ, and thus the corresponding ideotype will also differ (Isaacs et al., 2016; Louarn et al., 2020). Within these systems, the ideotype concept has been extended and the term ‘ideomix’ has been proposed (Litrico and Violle, 2015). To account for multi-species interactions, ideomix selection can be facilitated by participatory plant breeding (PPB), in which farmer knowledge and preferences are integrated within the variety selection process. The PPB approach was applied to an intercrop of maize and common bean (Phaseolus vulgaris L.) for cultivation in Rwanda (Isaacs et al., 2016). Through this approach, Isaacs et al. (2016) identified traits of the bean component, which constitute the ideotype for intercropping systems. These traits included restricted height, columnar plant structure, even distribution of pods, fewer leaves, and earlier maturity.
PPB programs are not only exclusive to intercrop systems but have also been applied to many monocrop species. Through client-orientated focus, it more likely that farmer criteria for genotypes are met, thus improving chance of uptake. This can therefore be used to overcome the social and cultural preferences that can impact expression of the ideotype.
The future of the ‘ideotype’
Whilst the concept of the ideotype has altered the way that we view yield improvement programmes and varietal selection, is it enough? Focusing on the ideal traits of a plant provides a simple and targeted framework for yield improvement. However, many plant traits are under control of multiple genes, some of which may be unknown. Another potential limitation to the ideotype concept is the multitude of interacting factors that determine the plant phenotype. Location, cultivation system and management all influence plant growth, and the potential combination of these features is almost infinite. Whilst the ideotype concept should, in theory, account for all of these different factors, the concept does lose some of its power when an infinite number of ideotypes are feasible.
Regardless of the complexity in achieving the ideotype, traits known to contribute to improved resource use efficiency or increased yield quantity and quality for one crop can provide a target for other, related crops. For example, Triticale (Triticum x Secale) evolved as a cross between wheat and rye and is known for high resource use efficiency and yield in dry environments (Estrada-Campuzano et al., 2012). Whilst not currently considered among the major crop species, Triticale has been shown to outcompete wheat and other cereals in environments that would not traditionally be considered dry, such as the UK. Triticale may therefore provide a blueprint for traits conveying resource use efficiency under climate change, where many cultivation zones are expected to become hotter and drier (Bassu et al., 2011; Roques et al., 2017). Beneficial traits of Triticale are associated with early vigour, a longer spike formation phase with the same duration to flowering, reduced tillering, increased remobilisation of carbohydrates to the grain and higher transpiration use efficiency (Bassu et al., 2011). For example, studies indicate that when applied to wheat, improved transpiration use efficiency is predicted to provide increased yield gains (Bassu et al., 2011).
A number of tools can assist with the generation of new, improved crop species. These will be discussed in the following section.
Tools to aid ideotype development
Improvements to phenotyping approaches
Improvements to plant phenotyping approaches have led to methods to rapidly capture detailed descriptions for a variety of plant traits. These often rely on the use of non-invasive and/or digital technologies (Costa et al., 2019). A number of recent reviews give in depth details to the advances in phenotyping approaches (e.g. (Mishra et al., 2016; Lobos et al., 2017; Ubbens and Stavness, 2017; Li et al., 2020; Pieruschka and Schurr, 2023), however a few key approaches will be given here in relation to ideotype breeding.
This desire for the creation of complex, geometrically accurate three-dimensional models of plants has led to the development of a number of different techniques in order to capture plant structure (e.g. Watanabe et al., 2005; Quan et al., 2006; Song et al., 2013; Pound et al., 2014; Gibbs et al., 2019). Applications of such models are diverse, including the study of photosynthesis for both single plants and whole canopy structures (e.g. Song et al., 2013; Burgess et al., 2017; Wang et al., 2020; Burgess et al., 2021). Image-based models, or those originating from other remote-sensing based techniques, are highly desirable (Houle et al., 2010; Santos and Oliviera, 2012; White et al., 2012; Pound et al., 2014; Gibbs et al., 2019), with the information needed to calculate a number of plant traits including leaf areas and angles, geometry or plant height. Therefore, these models are of particular importance for ideotype design and assessment, with the ability to rapidly and accurately quantify variation in structural traits.
The throughput of field data collection can be improved further through the use of gantry systems, robotics or airborne data collection (For reviews see Mogili and Deepak, 2018; Maes and Steppe, 2019; Atefi et al., 2021; Li et al., 2021; Xu and Li, 2023). In recent years, Unmanned Aerial Vehicles (UAVs), Remotely Piloted Aircrafts (RPAs) and drones have increased in popularity as a result of increases in hardware and software capabilities combined with a reduction in price. Incorporating multiple sensors within the same system can permit high spatial and temporal data collection for a wide range of plant parameters simultaneously (Mogili and Deepak, 2018; Barbedo, 2019). Together, these enable high-resolution spatial and temporal information to be collected, permitting the quantification of a large range of plant structures throughout the growth season.
Computer modelling
Generating new ideotypes is confounded by the high level of uncertainty associated with rapidly changing environmental conditions. Whilst the improvements in phenotyping approaches discussed above provide one approach to capturing plant structural traits, limitations arise as a result of access to equipment, knowledge, sufficient variation and time. To overcome limitations associated with the time and resource intensive breeding of ideotypes, tools are being continually developed to narrow down target traits. For example, designing and testing potential ideotypes in silico using simulation models enables a wider range of traits and environmental conditions to be tested. Process-based crop models have been developed to simulate interactions between genotype, environment, and management approaches. Thus, they can also be used to support plant breeding through ideotype design. Model-assisted phenotyping provides a phenotypic fingerprint that can link trait correlations to genetic information (Martre et al., 2015). Through the incorporation of global climate models, these models can also be used to predict both current and future desirable traits. Therefore whilst traditionally, breeding has focused on developing crops that are suitable for a broad variety of environments, model-assisted approaches allow varieties to be adapted to a specific set of environmental conditions. For reviews on the application of crop modelling to aid ideotype design see (Martre et al., 2015; Rötter et al., 2015; Christensen et al., 2018).
Simulation models have been applied to ideotype design for a range of crop species. The first model-aided ideotype design was accomplished within irrigated rice, leading to the production of the super hybrid rice variety ‘Lianyoupeijuu’ (Khush, 1995). Within wheat, Semenov and Stratonovitch (2013) applied the Sirius simulation model to design high-yielding ideotypes for two contrasting European sites, UK and Spain, for the 2050 A1B climate scenario (Meehl et al., 2007). This includes a year-round increase in temperature of 2 – 5°C and variation in monthly precipitation for both sites (Semenov and Stratonovitch, 2013). The authors identified that target traits predicted to achieve the greatest yield increase include improvement to the efficiency light energy conversion, optimal phenology and increased length of the grain filling period.
3-dimensional (3D) visual modelling provides an alternative route in model-assisted ideotype breeding to identify both phenotypic features and agronomic practices which can contribute to yield (Wang et al., 2019). This is facilitated by recent emphasis on the importance of crop canopy architecture as well as advances in the ways that canopies can be captured and modelled in 3D (Murchie and Burgess, 2022). Within rice (O. sativa), phenotypic plasticity was assessed to re-optimisation of physiology and yield forming processes of so called ‘super rice’ (Wang et al., 2019). Under optimal growing conditions, the super rice ideotype was predicted to have a high cumulative leaf area; erect leaf orientations, particularly within upper canopy levels; improved leaf area duration; and overall higher photosynthetic and resource use efficiency. Further potential beneficial traits include promoting early vigour and incorporating stay green phenotypes (Hoang and Kobata, 2009; Thomas and Ougham, 2014; Rebolledo et al., 2015; Zhao et al., 2019).
For ideotype breeding of multi-species cropping systems, plant growth modelling can help predict plant traits that affect both inter- and intraspecific interactions (Bourke et al., 2021). Successful intercrops arise from stronger interactions between the component crops, and thus identifying these interactions are key to ideotype breeding success. One particularly beneficial type of computer modelling approach is functional structural plant modelling (FSPM). In FSPMs, plant growth, and thus structure, is simulated in 3D over time based on the competition for growth resources (Evers et al., 2019). Therefore FSPMs are ideally suited to determining the impact of interspecies interactions under different spatial arrangements. However, for full assessment of plant performance during FSPMs, below-ground interactions must also be accounted for, thus requiring information on root systems (Evers et al., 2019).
The potential limitations associated with the use of process- based models for ideotype design are linked to model accuracy (Rötter et al., 2015). The detail and resolution at which the underlying physiological processes are presented within the model will determine their suitability for estimating beneficial traits. The resolution at which genetic information is incorporated with physiological and environmental controls is also key to progress (Bertin et al., 2010; Hammer et al., 2010; Martre et al., 2015). Incorporation of high-quality, long-term information on environmental variables and plant productivity under different scenarios will be imperative to increase the accuracy of the models going forwards (Rötter et al., 2011; Li et al., 2015). This includes the accurate representations of future weather events, including elevated carbon dioxide concentrations and the impact of elevated temperature on leaf senescence, floret abortion and grain filling (Asseng et al., 2011; Moriondo et al., 2011; Nendel et al., 2011; Rötter et al., 2011).
Genetic selection
Many desirable plant traits are quantitative and affected by multiple genes. Therefore, quantitative genetics provides a powerful framework for crop improvement. For ideotype design, parental lines could be selected for breeding programmes. Following trait recombination, genomic selection could be used as a tool to help avoid lengthy phenotypic evaluation of the progeny, while potentially achieving greater genetic gains.
When defining a genetic ideotype, the first stage is to accurately define the target environment and the corresponding impact on the phenotype (Hodson and White, 2007; Trethowan, 2014). Following this, known genetic information, gene x environment interactions and physiological response to stressors can be integrated to predict the optimal plant type. For areas of the genome where unknown genes may be located, association genetic analysis can be performed via random genotyping (Crossa et al., 2007). Once the genetic ideotype has been defined, it can then be bred for. This can be aided by methods such as marker assisted recombinant selection (Charmet et al., 1999) and through software to predict the probability of obtaining the desired genotype.
Combined approaches
Whilst computer modelling, genetic selection and new breeding approaches have all resulted in improvements to crop traits, greater improvements could be achieved by combining approaches. For example, combining simulation studies with new breeding approaches provides the platform for targeted ideotype production, significantly accelerating the generation of new cultivars.
Potential drawbacks of each individual approach may be overcome. For example, computer simulation approaches often do not consider the genetic basis of a trait of interest, not do they account for the available genetic variation in traits (Gu, 2022). In comparison, genetic- based models may be reduced in accuracy due to the complex gene x gene and gene x environment interactions. Therefore, quantitative trait loci (QTL) are often only detected under a limited set of environmental conditions (Zhou et al., 2007). Combining both approaches has therefore been proposed to overcome these issues (Letort et al., 2008; Bourke et al., 2021; Gu, 2022). When combining both approaches together, a number of factors must be addressed. For example, using phenotypic data for quantitative genetic analysis requires the growth and measurement of plants under the same environment, at the same growth stage. Conversely, accurate quantitative genetic analyses require screening of large populations to obtain sufficient genetic resolution.
The power of the combined approach comes from the application of crop simulation models in dissecting complex traits. Once dissected, it is then possible to assess genetic variation for each component and evaluate relative importance. Additionally, simulating plant growth and development using FSPMs, for example, permits the visualisation of the change in phenotype over time, and therefore provides direct information about the action of gene. This can be verified using field based phenotyping systems such as those discussed above. Due to these benefits, a combined approach has been suggested for ideotype design for both monocrops and intercrops (Bourke et al., 2021). For maximised success, several suggestions as to requirements of simulation models have been proposed, central to which is high predictability under varied environmental conditions (Hammer et al., 2002; Tardieu, 2003; Hammer et al., 2006). Some limitations may occur when using the combined approach, such as artificial pleiotropic effects, which may be a result of impact on similar primary mechanisms, but not through linked genetic bases (Yin et al., 2003).
Whilst improvements to both simulation and genetic methods will aid to increase success for ideotype breeding, combined approaches have nevertheless been applied to a number of crop species. In rice, combining ecophysiological crop models with genetic markers has been shown to provide a greater number of markers overall, whilst also allowing for prioritisation to a given environment (Gu et al., 2014). Similarly, Xu et al. (2011) were the first to combine an FSPM with a quantitative genetic model to link QTL with whole plant physiological function in rice. Whilst successful in predicting plant height, mismatch between measured and modelled dry matter production indicate further improvements are required (Xu et al., 2011). In apple trees, a FSPM was combined with genome wide information to predict tree structural information (Migault et al., 2017). Whilst the approach was sufficient at predicting phenotype for one growth season, it was less successful for another, indicating that further improvements are required to determine environmental interactions.
Conclusions
The concept of ideotype proposed by Donald (1968) suggests that there is an ideal phenotype of a crop that can produce higher and better quality yield within a given environment. This revolutionised the breeding process by providing targeted traits that are known to underlie key physiological processes. Since its proposal, the ideotype concept has been applied to a variety of different crop species, expanded to specific environments and alternative cropping systems, and applied to varying end uses. However, is the ideotype concept enough, or is it time for a new approach?
The ideotype suggests that the features of modern day elite varieties should be similar for a given habitat. Therefore, government recommended lists are expected to feature varieties that represent the ideotype, and are similar to each other. However, here we show significant variation among three elite wheat varieties featured recently on the UK recommended list (AHDB, 2023). The three varieties differed significantly in leaf and tiller numbers, leaf size, leaf erectness, chlorophyll content, photosynthetic efficiency, and mildew presence.
Multiple factors may contribute to the phenotype which an individual plant expresses. In many cases, this may result in the expression of features that are not part of the ideotype, and may have contributed to the variation we found here. Therefore the ideotype should not considered to be static, and will depend on a combination of biotic, abiotic and cultural variables. In a perfect world, these conflicting factors will have been accounted for during the ideotype selection process, and numerous advances in both computer modelling, genetic and biochemical methods are making this more feasible. However, limitations in these approaches exist, restricting their predictive capacity.
Regardless of potential limitations, we argue that there is still a role for the ideotype in crop breeding, but that this concept needs to be expanded to combine knowledge of the genetic and environmental interactions governing plant physiology.
Methods
Plant material
Three elite varieties of wheat (Triticum aestivum) were selected from AHDB recommended lists. The varieties chosen were Paragon, Willow and Cochise, which were last recommended by the AHDB for the periods of 2015 to 2016, 2019 to 2020, and 2023 to 2024, respectively (AHDB, 2023). The seeds were planted on a 96-cell module tray using John Innes No. 2 soil-based compost (pH 6.0 – 7.0; J Arthur Bower’s, Westland Horticulture Ltd., Huntingdon, UK) in the glasshouse facilities at the University of Nottingham Sutton Bonington campus. 32 seeds of each variety were planted in each tray. After three weeks, corresponding to growth stage 12 (Zadoks et al., 1974), the plants were then transplanted into 2-litre pots which were filled with the same soil-based (John Innes No. 2) compost. The experiment used a randomised block design with a total of four replicates. The first replicate was planted on 19th October 2022, the second on the 9th November 2022, the third on the 1st December 2022 and the fourth on the 20th January 2023. Glasshouse temperature was controlled to 22°C from 6:00am to 10:00pm and to 19°C from 10:00pm to 6:00am, whilst photoperiod ranged between 8 (January) and 11 (October and March) hours, in accordance with UK weather. A total of 20 plants of each variety were transplanted for the first three replicates while 24 plants of each of the Cochise and Willow varieties and 32 plants of the Paragon variety were transplanted in the fourth replicate. The pots were placed on top of benches and attached to an automated drip irrigation system.
Throughout all experimental runs, plants suffered with breakouts of both powdery mildew and aphids. These were controlled for in multiple ways. SB invigorator spray (SBPI, Stan Brouard Group, Guernsey, UK) was used for controlling both pests by applying it directly to the affected areas and also by applying it to all of the plants when the aphids or mildew were starting to spread. To control the mildew specifically, RomeoTM (Agrauxine Lesaffre Plant Care, Angers, France), a cerevisane-based biofungicide was sprayed on a weekly basis at a rate of 0.5 kg/ha. Additionally, Revystar (100 g mefentrifluconazole/l EC, BASF SE, Limburgerhof, Germany) was applied once at a rate of 1.5 x 10-3 m3/ha. To control the aphids specifically, FLiPPER® (a broad-spectrum contact bio-insecticide/acaricide, Bayer CropScience Ltd., Cambridge, UK) and Aphox (active ingredient: 50% pirimicarb; Adama Ltd., Reading, UK) were applied only when the aphid population was noticeably high at a rate of 1.6 x 10-2 m3/ha and 2.8 × 10-1 kg/ha. Additionally, biological controls in the form of aphid predators (Chrysoperla carnea) and parasitic wasps (Aphidius colemani, Aphidius ervi, and Aphelinus abdominalis) from Bioline Agrosciences (Little Clacton, UK) were applied.
Phenotypic measurements
The majority of measurements were performed on the final batch of plants which were less effected by pests and diseases. A total of twenty-four plants were measured for each of the Cochise and Willow wheat varieties while thirty-two plants were measured for the Paragon variety. All measurements taken were non-destructive and were done by either using optical devices or by manually measuring or counting the variable.
For the optical measurements, a SPAD 502 chlorophyll meter (Konica Minolta, Tokyo, Japan) was used for measuring chlorophyll content and a Photon Systems Instruments (PSI, Brno, Czech Republic) FluorPen FP 110 was used for measuring the quantum yield. Measurements were taken weekly after growth stage GS40 (Zadoks et al., 1974). The measurements were performed three times at the centre of the adaxial side of the second youngest leaf and then averaged.
For the manual measurements, main shoot height, tiller count, ear count, leaf count, leaf area and leaf erectness were measured. In addition, the presence of powdery mildew was also noted. The main shoot height was measured for each of the plants by placing a ruler with zero value on top of the soil and then measuring the height from soil surface to the top of the ear, not considering the awns if any were present. These measurements were performed after the emergence of the ear between growth stages GS60 and GS70 Leaf and tiller count were performed by manually counting the number of leaves and tillers (in addition to the main shoot) that were present in each of the plants. Counting was done on a weekly basis and the final count was done after growth stage GS60.
Flag leaf and non-flag leaf area was calculated using a non-destructive method based on the formula derived by Chanda and Singh (2002) after GS60:
For the length, the measurement was taken from the leaf base to the leaf tip. For the breadth, the measurement was taken for the widest width of the leaves at a perpendicular angle to the measurement taken for the length. To calculate the leaf erectness of the different elite varieties of wheat, the methods proposed by (Richards et al., 2019) of assigning a score from 1 (erect) to 10 (planophile) was adopted after GS70. A score of 1 was given to a canopy if all the leaves in the upper canopy had their tips positioned above the ligule while a score of 10 was given if none of the leaves had their tip positioned above the ligule. Other scores were assigned depending on the percentage of leaves that were erect (e.g., a score of 8 was given if around 20% of the leaves were erect).
Statistical analysis
All statistical analysis tests were performed using Jamovi for windows (version 2.3.21). The data was checked to see if the assumptions of homogeneity and normality were satisfied. The variables that complied with both assumptions were main shoot height, leaf count, tiller count, and chlorophyll content. The variables that did not comply with both assumptions were ear count, leaf count, tiller count, leaf erectness, and quantum yield. Analysis of variance (ANOVA) was carried for the variables that complied with both assumptions and then a post hoc Tukey’s test was used at a probability level of p< 0.05. A Kruskal-Wallis non-parametric ANOVA test was carried out for the variables that did not comply with both assumptions.
Data availability statement
The raw data supporting the conclusions of this article will be made available by the authors, without undue reservation.
Author contributions
AC: Conceptualization, Formal analysis, Investigation, Methodology, Visualization, Writing – original draft. AB: Conceptualization, Funding acquisition, Project administration, Resources, Supervision, Visualization, Writing – original draft, Writing – review & editing.
Funding
The author(s) declare that no financial support was received for the research, authorship, and/or publication of this article.
Acknowledgments
This presents part of the MSc project of AC-F, completed at the University of Nottingham, 2022-2023.
Conflict of interest
The authors declare that the research was conducted in the absence of any commercial or financial relationships that could be construed as a potential conflict of interest.
Publisher’s note
All claims expressed in this article are solely those of the authors and do not necessarily represent those of their affiliated organizations, or those of the publisher, the editors and the reviewers. Any product that may be evaluated in this article, or claim that may be made by its manufacturer, is not guaranteed or endorsed by the publisher.
References
Abbo S., Pinhasi van-Oss R., Gopher A., Saranga Y., Ofner I., Peleg Z. (2014). Plant domestication versus crop evolution: A conceptual framework for cereals and grain legumes. Trends Plant Sci. 19, 351–360. doi: 10.1016/j.tplants.2013.12.002
AHDB (2023) AHDB Recommended Lists. Available at: https://ahdb.org.uk/knowledge-library/recommended-lists-for-cereals-and-oilseeds-rl (Accessed October 4, 2023).
Albaladejo Román A. (2023) Food security in 2023: EU response to an evolving crisis. Available at: http://www.europarl.europa.eu/thinktank.
Arraiano L., Brading P., Dedryver F., Brown J. (2006). Resistance of wheat to septoria tritici blotch (Mycosphaerella graminicola) and associations with plant ideotype and the 1BL–1RS translocation. Plant Pathol. 55, 54–61. doi: 10.1111/j.1365-3059.2005.01319.x
Asano K., Yamasaki M., Takuno S., Miura K., Katagiri S., Ito T., et al. (2011). Artificial selection for a green revolution gene during japonica rice domestication. Proc. Natl. Acad. Sci. U.S.A. 108, 11034–11039. doi: 10.1073/pnas.1019490108
Asseng S., Foster I., Turner N. (2011). The impact of temperature variability on wheat yields. Glob. Chang. Biol. 17, 997–1012. doi: 10.1111/j.1365-2486.2010.02262.x
Atefi A., Ge Y., Pitla S., Schnable J. (2021). Robotic technologies for high-throughput plant phenotyping: contemporary reviews and future perspectives. Front. Plant Sci. 12. doi: 10.3389/fpls.2021.611940
Barbedo J. (2019). A review on the use of unmanned aerial vehicles and imaging sensors for monitoring and assessing plant stresses. Drones 3, 40. doi: 10.3390/drones3020040
Bassu S., Asseng S., Richards R. (2011). Yield benefits of triticale traits for wheat under current and future climates. Field Crops Res. 124, 14–24. doi: 10.1016/j.fcr.2011.05.020
BBRO (2023) BBRO: Recommended List. Available at: https://bbro.co.uk/sugar-beet-varieties/recommended-list/ (Accessed November 16, 2023).
Berry P., Sylvester-Bradley R., Berry S. (2007). Ideotype design for lodging-resistant wheat. Euphytica 154, 165–179. doi: 10.1007/s10681-006-9284-3
Bertin N., Martre P., Génard M., Quilot B., Salon C. (2010). Under what circumstances can process-based simulation models link genotype to phenotype for complex traits? Case-study of fruit and grain quality traits. J. Exp. Bot. 61, 955–967. doi: 10.1093/jxb/erp377
Bort J., Febrero A., Amaro T., Araus J. (1994). Role of awns in ear water-use efficiency and grain weight in barley. Agronomie 2, 133–139. doi: 10.1051/agro:19940209
Bourke P., Evers J., Bijma P., van Apeldoorn D., Smulders M., Kuyper T., et al. (2021). Breeding beyond monoculture: putting the “Intercrop” into crops. Front. Plant Sci. 12, 734167. doi: 10.3389/fpls.2021.734167
Braun H.-J., Pfeiffer W., Pollmer W. (1992). Environments for selecting widely adapted spring wheat. Crop Sci. 32, cropsci1992.0011183X003200060022x. doi: 10.2135/cropsci1992.0011183X003200060022x
Bugbee B., Koerner G. (1997). Yield comparisons and unique characteristics of the dwarf wheat cultivar ‘USU-Apogee.’. Adv. Space Res. 20, 1891–1894. doi: 10.1016/S0273-1177(97)00856-9
Burgess A., Correa Cano M., Parkes B. (2022). The deployment of intercropping and agroforestry as adaptation to climate change. Crop Environ. 1, 145–160. doi: 10.1016/j.crope.2022.05.001
Burgess A., Durand M., Gibbs J., Retkute R., Robson T., Murchie E. (2021). The effect of canopy architecture on the patterning of “windflecks” within a wheat canopy. Plant Cell Environ. 44, 3524–3537. doi: 10.1111/pce.14168
Burgess A., Retkute R., Herman T., Murchie E. (2017). Exploring relationships between canopy architecture, light distribution, and photosynthesis in contrasting rice genotypes using 3D canopy reconstruction. Front. Plant Sci. 8. doi: 10.3389/fpls.2017.00734
Burgess A. J., Retkute R., Preston S. P., Jensen O. E., Pound M. P., Pridmore T. P., et al. (2016). The 4-dimensional plant: effects of wind- induced canopy movement on light fluctuations and photosynthesis. Front. Plant Sci. 7. doi: 10.3389/FPLS.2016.01392
Cadenasso M. L., Pickett S. T. A. (2000). Linking forest edge structure to edge function: Mediation of herbivore damage. J. Ecol. 88, 31–44. doi: 10.1046/j.1365-2745.2000.00423.x
Cairns J., Sanchez C., Vargas M., Ordoñez R., Araus J. (2012). Dissecting maize productivity: Ideotypes associated with grain yield under drought stress and well-watered conditions. J. Integr. Plant Biol. 54, 1007–1020. doi: 10.1111/j.1744-7909.2012.01156.x
Carmo-Silva E., Andralojc P., Scales J., Driever S., Mead A., Lawson T., et al. (2017). Phenotyping of field-grown wheat in the UK highlights contribution of light response of photosynthesis and flag leaf longevity to grain yield. J. Exp. Bot. 68, 3473–3486. doi: 10.1093/jxb/erx169
Chanda S., Singh Y. (2002). Estimation of leaf area in wheat using linear measurements. Plant Breed. seed Sci. 46 (2), 75–79. Retrieved from https://ojs.ihar.edu.pl/index.php/pbss/article/view/778.
Chang K., Ahmed H., Hwang S., Gossen B., Howard R., Warkentin T., et al. (2007). Impact of cultivar, row spacing and seeding rate on ascochyta blight severity and yield of chickpea. Can. J. Plant Sci. 87, 395–403. doi: 10.4141/P06-067
Charles-Dominique T., Barczi J., Le Roux E., Chamaillé-Jammes S. (2017). The architectural design of trees protects them against large herbivores. Funct. Ecol. 31, 1710–1717. doi: 10.1111/1365-2435.12876
Charmet G., Robert N., Perretant M.-R., Gay G., Sourdille P., Groos C., et al. (1999). Marker-assisted recurrent selection for cumulating additive and interactive QTLs in recombinant inbred lines. Theor. Appl. Genet. 99, 1143–1148. doi: 10.1007/s001220051318
Christensen A., Srinivasan V., Hart J., Marshall-Colon A. (2018). Use of computational modeling combined with advanced visualization to develop strategies for the design of crop ideotypes to address food security. Nutr. Rev. 76, 332–347. doi: 10.1093/nutrit/nux076
Christidis N., Stott P. (2021). The influence of anthropogenic climate change on wet and dry summers in Europe. Sci. Bull. (Beijing) 66, 813–823. doi: 10.1016/j.scib.2021.01.020
Cornille A., Gladieux P., Smulders M., Roldán-Ruiz I., Laurens F., Le Cam B., et al. (2012). New insight into the history of domesticated apple: Secondary contribution of the European wild apple to the genome of cultivated varieties. PloS Genet. 8, 1–13. doi: 10.1371/journal.pgen.1002703
Costa C., Schurr U., Loreto F., Menesatti P., Carpentier S. (2019). Plant phenotyping research trends, a science mapping approach. Front. Plant Sci. 9. doi: 10.3389/fpls.2018.01933
Cowger C., Miranda L., Griffey C., Hall M., Murphy J., Maxwell J. (2012). ““Wheat powdery mildew,”,” in Disease resistance in wheat (UK: CABI Wallingford), 84–119.
Crossa J., Burgueno J., Dreisigacker S., Vargas M., Herrera-Foessel S., Lillemo M., et al. (2007). Association analysis of historical bread wheat germplasm using additive genetic covariance of relatives and population structure. Genetics 177, 1889–1913. doi: 10.1534/genetics.107.078659
de Langre E. (2008). Effects of wind on plants. Annu. Rev. Fluid Mech. 40, 141–168. doi: 10.1146/annurev.fluid.40.111406.102135
Doust A. (2007). Architectural evolution and its implications for domestication in grasses. Ann. Bot. 100, 941–950. doi: 10.1093/aob/mcm040
Estrada-Campuzano G., Slafer G., Miralles D. (2012). Differences in yield, biomass and their components between triticale and wheat grown under contrasting water and nitrogen environments. Field Crops Res. 128, 167–179. doi: 10.1016/j.fcr.2012.01.003
Evers J., van der Werf W., Stomph T., Bastiaans L., Anten N. (2019). Understanding and optimizing species mixtures using functional–structural plant modelling. J. Exp. Bot. 70, 2381–2388. doi: 10.1093/jxb/ery288
FAO (2022). World Food and Agriculture – Statistical Yearbook 2022 (Rome, Italy: FAO). doi: 10.4060/cc2211en
FAO, IFAD, UNICEF, WFP, WHO (2022). The State of Food Security and Nutrition in the World 2022 (Rome, Italy: FAO). doi: 10.4060/cc0639en
FAOSTAT (2023). Available at: http://www.fao.org/faostat/.
Foulkes M., Murchie E. (2011). ““Optimizing canopy physiology traits to improve the nutrient use efficiency of crops.,”,” in The Molecular and physiological basis of nutrient use efficiency in crops. Eds. Hawkesford M. J., Barraclough P. (Chichester: Wiley-Blackwell), 65–83.
Gibbs J., Pound M., French A., Wells D., Murchie E., Pridmore T. (2019). Active vision and surface reconstruction for 3D plant shoot modelling. IEEE Transactions: Comput. Biol. Bioinf. 17 (6), 1907–1917. doi: 10.1109/TCBB.2019.2896908
Greenland A., Bentley S., Jones H., Karley A., Lee D., Sherlock D., et al. (2017). New wheat root ideotypes for improved resource use efficiency and yield performance in reduced input agriculture. Available at: https://ahdb.org.uk/new-wheat-root-ideotypes-for-improved-resource-use-and-efficiency-and-yield-performance-in-reduced-input-agriculture.
Grobman A., Bonavia D., Dillehay T., Piperno D., Iriarte J., Holst I. (2012). Preceramic maize from paredones and Huaca Prieta, Peru. Proc. Natl. Acad. Sci. U.S.A. 109, 1755–1759. doi: 10.1073/pnas.1120270109
Gu J. (2022). Ecophysiological crop modelling combined with genetic analysis is a powerful tool for ideotype design. Agronomy 12 (1), 215. doi: 10.3390/agronomy12010215
Gu J., Yin X., Zhang C., Wang H., Struik P. (2014). Linking ecophysiological modelling with quantitative genetics to support marker-assisted crop design for improved yields of rice (Oryza sativa) under drought stress. Ann. Bot. 114, 499–511. doi: 10.1093/aob/mcu127
Hammer G., Cooper M., Tardieu F., Welch S., Walsh B., van Eeuwijk F., et al. (2006). Models for navigating biological complexity in breeding improved crop plants. Trends Plant Sci. 11, 587–593. doi: 10.1016/j.tplants.2006.10.006
Hammer G., Kropff M., Sinclair T., Porter J. (2002). Future contributions of crop modelling—from heuristics and supporting decision making to understanding genetic regulation and aiding crop improvement. Eur. J. Agron. 18, 15–31. doi: 10.1016/S1161-0301(02)00093-X
Hammer G., van Oosterom E., McLean G., Chapman S., Broad I., Harland P., et al. (2010). Adapting APSIM to model the physiology and genetics of complex adaptive traits in field crops. J. Exp. Bot. 61, 2185–2202. doi: 10.1093/jxb/erq095
Haudry A., Cenci A., Ravel C., Bataillon T., Brunel D., Poncet C., et al. (2007). Grinding up wheat: A massive loss of nucleotide diversity since domestication. Mol. Biol. Evol. 24, 1506–1517. doi: 10.1093/molbev/msm077
Hedden P. (2003). The genes of the green revolution. Trends Genet. 19, 5–9. doi: 10.1016/S0168-9525(02)00009-4
Hoang T. B., Kobata T. (2009). Stay-green in rice (Oryza sativa L.) of drought-prone areas in desiccated soils. Plant Prod. Sci. 12, 397–408. doi: 10.1626/pps.12.397
Hodson D., White J. (2007). Use of spatial analyses for global characterization of wheat-based production systems. J. Agric. Sci. 145, 115. doi: 10.1017/S0021859607006855
Houle D., Govindaraju D. R., Omholt S. (2010). Phenomics: the next challenge. Nat. Rev. Genet. 11, 855–866. doi: 10.1038/nrg2897
Hyten D., Song Q., Zhu Y., Choi I., Nelson R., Costa J., et al. (2006). Impact of genetic bottlenecks on soybean genome diversity. Proc. Natl. Acad. Sci. U.S.A. 103, 16666–16671. doi: 10.1073/pnas.0604379103
Iorizzo M., Senalik D., Ellison S., Grzebelus D., Cavagnaro P., Allender C., et al. (2013). Genetic structure and domestication of carrot (Daucus carota subsp. sativus) (Apiaceae). Am. J. Bot. 100, 930–938. doi: 10.3732/ajb.1300055
Isaacs K., Snapp S., Kelly J., Chung K. (2016). Farmer knowledge identifies a competitive bean ideotype for maize–bean intercrop systems in Rwanda. Agric. Food Secur. 5, 15. doi: 10.1186/s40066-016-0062-8
Jiao Y., Wang Y., Xue D., Wang J., Yan M., Liu G., et al. (2010). Regulation of OsSPL14 by OsmiR156 defines ideal plant architecture in rice. Nat. Genet. 42, 541–544. doi: 10.1038/ng.591
Kaur P., Sachan S., Sharma A. (2021). Weed competitive ability in wheat: a peek through in its functional significance, present status and future prospects. Physiol. Mol. Biol. Plants 27, 2165–2179. doi: 10.1007/s12298-021-01079-y
Khush G. (1995). Breaking the yield frontier of rice. GeoJournal 35, 329–332. doi: 10.1007/BF00989140
Kilian B., Özkan H., Walther A., Kohl J., Dagan T., Salamini F., et al. (2007). Molecular diversity at 18 loci in 321 wild and 92 domesticate lines reveal no reduction of nucleotide diversity during Triticum monococcum (einkorn) domestication: Implications for the origin of agriculture. Mol. Biol. Evol. 24, 2657–2668. doi: 10.1093/molbev/msm192
Knüpffer H., Terentyeva I., Karl H., Kolavela O., Sato K. (2003). Ecogeographical diversity - a Vavilovian approach. Dev. Plants Genet. Breed. 7, 53–76. doi: 10.1016/S0168-7972(03)80006-3
Kumar N., Srivastava S. (2003). Plant ideotype of Indian mustard (Brassica juncea) for late sown conditions. Indian J. Genet. Plant Breed. 63, 355. Available at: https://www.isgpb.org/journal/index.php/IJGPB/article/view/1757.
Letort V., Mahe P., Cournède P.-H., de Reffye P., Courtois B. (2008). Quantitative genetics and functional–structural plant growth models: simulation of quantitative trait loci detection for model parameters and application to potential yield optimization. Ann. Bot. 101, 1243–1254. doi: 10.1093/aob/mcm197
Li Z., Guo R., Li M., Chen Y., Li G. (2020). A review of computer vision technologies for plant phenotyping. Comput. Electron. Agric. 176, 105672. doi: 10.1016/j.compag.2020.105672
Li T., Hasegawa T., Yin X., Zhu Y., Boote K., Adam M., et al. (2015). Uncertainties in predicting rice yield by current crop models under a wide range of climatic conditions. Glob. Chang. Biol. 21, 1328–1341. doi: 10.1111/gcb.12758
Li D., Quan C., Song Z., Li X., Yu G., Li C., et al. (2021). High-throughput plant phenotyping platform (HT3P) as a novel tool for estimating agronomic traits from the lab to the field. Front. Bioeng. Biotechnol. 8. doi: 10.3389/fbioe.2020.623705
Lithourgidis A., Dordas C., Damalas C., Vlachostergios D. (2011). Annual intercrops: an alternative pathway for sustainable agriculture. Aust. J. Crop Sci. 5, 396–410. Available at: https://www.researchgate.net/publication/224934832_Annual_intercrops_An_alternative_pathway_for_sustainable_agriculture.
Litrico I., Violle C. (2015). Diversity in plant breeding: A new conceptual framework. Trends Plant Sci. 20, 604–613. doi: 10.1016/j.tplants.2015.07.007
Lobos G., Camargo A., Del Pozo A., Araus A., Ortiz R., Doonan J. (2017). Plant phenotyping and phenomics for plant breeding. Front. Plant Sci. 8, 2181. doi: 10.3389/fpls.2017.02181
Louarn G., Barillot R., Combes D., Escobar-Gutiérrez A. (2020). Towards intercrop ideotypes: non-random trait assembly can promote overyielding and stability of species proportion in simulated legume-based mixtures. Ann. Bot. 126, 671–685. doi: 10.1093/aob/mcaa014
Lynch J. (2013). Steep, cheap and deep: an ideotype to optimize water and N acquisition by maize root systems. Ann. Bot. 112, 347–357. doi: 10.1093/aob/mcs293
Lyu J., Zhang S., Dong Y., He W., Zhang J., Deng X., et al. (2013). Analysis of elite variety tag SNPs reveals an important allele in upland rice. Nat. Commun. 4, 2138. doi: 10.1038/ncomms3138
Maes W., Steppe K. (2019). Perspectives for remote sensing with unmanned aerial vehicles in precision agriculture. Trends Plant Sci. 24, 152–164. doi: 10.1016/j.tplants.2018.11.007
Maitra S., Hossain A., Brestic M., Skalicky M., Ondrisik P., Gitari H., et al. (2021). Intercropping—A low input agricultural strategy for food and environmental security. Agronomy 11, 343. doi: 10.3390/agronomy11020343
Martre P., Quilot-Turion B., Luquet D., Memmah M.-M.-S., Chenu K., Debaeke P. (2015). “Model-assisted phenotyping and ideotype design,” in Crop physiology (Cambridge, Massachusetts, USA: Elsevier), 349–373.
Meehl G., Covey C., Delworth T., Latif M., McAvaney B., Mitchell J., et al. (2007). The WCRP CMIP3 multimodel dataset: A new era in climate change research. Bull. Am. Meteorol. Soc. 88, 1383–1394. doi: 10.1175/BAMS-88-9-1383
Meyer R., Purugganan M. (2013). Evolution of crop species: Genetics of domestication and diversification. Nat. Rev. Genet. 14, 840–852. doi: 10.1038/nrg3605
Mi G., Chen F., Wu Q., Lai N., Yuan L., Zhang F. (2010). Ideotype root architecture for efficient nitrogen acquisition by maize in intensive cropping systems. Sci. China Life Sci. 53, 1369–1373. doi: 10.1007/s11427-010-4097-y
Mi G., Chen F., Yuan L., Zhang F. (2016). Ideotype root system architecture for maize to achieve high yield and resource use efficiency in intensive cropping systems. Adv. Agron. 139, 73–97. doi: 10.1016/bs.agron.2016.05.002
Migault V., Pallas B., Costes E. (2017). Combining genome-wide information with a functional structural plant model to simulate 1-year-old apple tree architecture. Front. Plant Sci. 7. doi: 10.3389/fpls.2016.02065
Mishra K., Mishra A., Klem K. (2016). Plant phenotyping: a perspective. Indian J. Plant Physiol. 21, 514–527. doi: 10.1007/s40502-016-0271-y
Mogili U., Deepak B. (2018). Review on application of drone systems in precision agriculture. Proc. Comput. Sci. 133, 502–509. doi: 10.1016/j.procs.2018.07.063
Moriondo M., Giannakopoulos C., Bindi M. (2011). Climate change impact assessment: the role of climate extremes in crop yield simulation. Clim. Change 104, 679–701. doi: 10.1007/s10584-010-9871-0
Motzo R., Giunta F. (2002). Awnedness affects grain yield and kernel weight in near-isogenic lines of durum wheat. Aust. J. Agric. Res. 53, 1285–1293. doi: 10.1071/AR02008
Murchie E. H., Burgess A. J. (2022). Casting light on the architecture of crop yield. Crop Environ. 1, 74–85. doi: 10.1016/J.CROPE.2022.03.009
Nendel C., Berg M., Kersebaum K., Mirschel W., Specka X., Wegehenkel M., et al. (2011). The MONICA model: Testing predictability for crop growth, soil moisture and nitrogen dynamics. Ecol. Modell. 222, 1614–1625. doi: 10.1016/j.ecolmodel.2011.02.018
Nickell L., Louis N. (1982). Plant growth regulators: Agricultural uses (Berlin, Germany: Springer).
Ong C., Monteith J. L. (1992). ““Canopy establishment: light capture and loss by crop canopies,”,” in Crop Photosynthesis: spatial and temporal determinants. Eds. Baker N., Thomas H. (Amsterdam, The Netherlands: Elsevier), 1–9.
Paleari L., Movedi E., Cappelli G., Wilson L., Confalonieri R. (2017). Surfing parameter hyperspaces under climate change scenarios to design future rice ideotypes. Glob. Chang. Biol. 23, 4651–4662. doi: 10.1111/gcb.13682
Parry M. A. J., Reynolds M., Salvucci M. E., Raines C., Andralojc P. J., Zhu X. G., et al. (2011). Raising yield potential of wheat. II. Increasing photosynthetic capacity and efficiency. J. Exp. Bot. 62, 453–467. doi: 10.1093/jxb/erq304
Parthasarathi T., Vanitha K., Lakshamanakumar P., Kalaiyarasi D. (2012). Aerobic rice-mitigating water stress for the future climate change. Int. J. Agron. Plant Prod. 3, 241–254. Available at: https://www.researchgate.net/publication/254559564_Aerobic_rice-mitigating_water_stress_for_the_future_climate_change.
Paterson A., Lin Y., Li Z., Schertz K., Doebley J., Pinson S., et al. (1995). Convergent domestication of cereal crops by independent mutations at corresponding genetic loci. Sci. (1979) 269, 1714–1718. doi: 10.1126/science.269.5231.1714
Pearce S., Saville R., Vaughan S., Chandler P., Wilhelm E., Sparks C., et al. (2011). Molecular characterization of Rht-1 dwarfing genes in hexaploid wheat. Plant Physiol. 157, 1820–1831. doi: 10.1104/pp.111.183657
Peng S., Khush G. S., Virk P., Tang Q., Zou Y. (2008). Progress in ideotype breeding to increase rice yield potential. Field Crops Res. 108, 32–38. doi: 10.1016/j.fcr.2008.04.001
Perego A., Sanna M., Giussani A., Chiodini M., Fumagalli M., Pilu S., et al. (2014). Designing a high-yielding maize ideotype for a changing climate in Lombardy plain (northern Italy). Sci. total Environ. 499, 497–509. doi: 10.1016/j.scitotenv.2014.05.092
Pieruschka R., Schurr U. (2023). Plant phenotyping: past, present, and future. Plant Phenomics 2019, 7507131. doi: 10.34133/2019/7507131
Piñera-Chavez F. J., Berry P. M., Foulkes M. J., Jesson M. A., Reynolds M. P. (2016). Avoiding lodging in irrigated spring wheat. I. Stem and root structural requirements. Field Crops Res. 196, 325–336. doi: 10.1016/j.fcr.2016.06.009
Porter J., Semenov M. (2005). Crop responses to climatic variation. Philos. Trans. R. Soc. B: Biol. Sci. 360, 2021–2035. doi: 10.1098/rstb.2005.1752
Pound M., French A., Murchie E., Pridmore T. (2014). Automated recovery of 3D models of plant shoots from multiple colour images. Plant Physiol. 144, 1688–1698. doi: 10.1104/pp.114.248971
Quan L., Tan P., Zeng G., Yuan L., Wang J., Kang S. B. (2006). Image-based plant modeling. ACM Trans. Graph 25, 599. doi: 10.1145/1141911.1141929
Rahangdale S., Singh Y., Kujur M., Koutu G. (2019). “Exploration of new plant type (NPT) rice lines for crop improvement,” in Advances in Agriculture Sciences. 19, 53. Available at: https://www.academia.edu/41340085/Exploration_of_New_Plant_Type_NPT_Rice_Lines_for_Crop_Improvement.
Rebetzke G., Bonnett D., Reynolds M. (2016). Awns reduce grain number to increase grain size and harvestable yield in irrigated and rainfed spring wheat. J. Exp. Bot. 67, 2573–2586. doi: 10.1093/jxb/erw081
Rebolledo M., Dingkuhn M., Courtois B., Gibon Y., Clément-Vidal A., Cruz D., et al. (2015). Phenotypic and genetic dissection of component traits for early vigour in rice using plant growth modelling, sugar content analyses and association mapping. J. Exp. Bot. 66, 5555–5566. doi: 10.1093/jxb/erv258
Reynolds M., Bonnett D., Chapman S., Furbank R., Manès Y., Mather D., et al. (2011). Raising yield potential of wheat. I. Overview of a consortium approach and breeding strategies. J. Exp. Bot. 62, 439–452. doi: 10.1093/jxb/erq311
Reynolds M., Foulkes M., Slafer G., Berry P., Parry M., Snape J., et al. (2009). Raising yield potential in wheat. J. Exp. Bot. 60, 1899–1918. doi: 10.1093/jxb/erp016
Rezzouk F., Gracia-Romero A., Kefauver S., Nieto-Taladriz M., Serret M., Araus J. (2022). Durum wheat ideotypes in Mediterranean environments differing in water and temperature conditions. Agric. Water Manag. 259, 107257. doi: 10.1016/j.agwat.2021.107257
Richard B., Jumel S., Rouault F., Tivoli B. (2012). Plant stage and organ age, two factors that affect pea (Pisum sativum) receptivity to ascochyta blight (Mycosphaerella pinodes). Eur. J. Plant Pathol. 132, 367–379. doi: 10.1007/s10658-011-9882-3
Richards R., Cavanagh C., Riffkin P. (2019). Selection for erect canopy architecture can increase yield and biomass of spring wheat. Field Crops Res. 244, 107649. doi: 10.1016/j.fcr.2019.107649
Roques S., Kindred D., Clarke S. (2017). Triticale out-performs wheat on range of UK soils with a similar nitrogen requirement. J. Agric. Sci. 155, 261–281. doi: 10.1017/S0021859616000356
Rötter R., Carter T., Olesen J., Porter J. (2011). Crop–climate models need an overhaul. Nat. Clim. Chang. 1, 175–177. doi: 10.1038/nclimate1152
Rötter R., Tao F., Höhn J., Palosuo T. (2015). Use of crop simulation modelling to aid ideotype design of future cereal cultivars. J. Exp. Bot. 66, 3463–3476. doi: 10.1093/jxb/erv098
Sanchez-Bragado R., Molero G., Araus J., Slafer G. (2023). Awned versus awnless wheat spikes: does it matter? Trends Plant Sci. 28, 330–343. doi: 10.1016/j.tplants.2022.10.010
Santos T., Oliviera A. (2012). “Image-based 3D digitizing for plant architecture analysis and phenotyping,” in Workshop on Industry Applications (WGARI) in SIBGRAPI 2012 (XXV Conference on Graphics, Patterns and Images) (MG, Brazil: Ouro Preto).
Semenov M., Stratonovitch P. (2013). Designing high-yielding wheat ideotypes for a changing climate. Food Energy Secur. 2, 185–196. doi: 10.1002/fes3.34
Semenov M., Stratonovitch P., Alghabari F., Gooding M. (2014). Adapting wheat in Europe for climate change. J. Cereal Sci. 59, 245–256. doi: 10.1016/j.jcs.2014.01.006
Senapati N., Griffiths S., Hawkesford M., Shewry P., Semenov M. (2020). Substantial increase in yield predicted by wheat ideotypes for Europe under future climate. Clim. Res. 80, 189–201. doi: 10.3354/CR01602
Senapati N., Stratonovitch P., Paul M., Semenov M. (2019). Drought tolerance during reproductive development is important for increasing wheat yield potential under climate change in Europe. J. Exp. Bot. 70, 2549–2560. doi: 10.1093/jxb/ery226
Sharma D., Sanghera G., Sahu P., Sahu P., Parikh M., Sharma B., et al. (2013). Tailoring rice plants for sustainable yield through ideotype breeding and physiological interventions. Afr. J. Agric. Res. 8, 5004–5019. doi: 10.5897/AJAR2013.7499
Siles L., Hassall K., Sanchis-Gritsch C., Eastmond P., Kurup S. (2020). Uncovering the ideal plant ideotype for maximising seed yield in Brassica napus. bioRxiv 2020, 12.04.411371. doi: 10.1101/2020.12.04.411371
Singh R., Huerta-Espino J., Rajaram S., Crossa J. (2001). Grain yield and other traits of tall and dwarf isolines of modern bread and durum wheats. Euphytica 119, 241–244. doi: 10.1023/A:1017541805454
Song Q., Zhang G., Zhu X.-G. (2013). Optimal crop canopy architecture to maximise canopy photosynthetic CO2 uptake under elevated CO2 – a theoretical study using a mechanistic model of canopy photosynthesis. Funct. Plant Biol. 40, 109–124. doi: 10.1071/FP12056
Srinivasan V., Kumar P., Long S. (2017). Decreasing, not increasing, leaf area will raise crop yields under global atmospheric change. Glob. Chang. Biol. 23, 1626–1635. doi: 10.1111/gcb.13526
Tanaka M., Keira M., Yoon D.-K., Mae T., Ishida H., Makino A., et al. (2022). Photosynthetic enhancement, lifespan extension, and leaf area enlargement in flag leaves increased the yield of transgenic rice plants overproducing rubisco under sufficient N fertilization. Rice 15, 10. doi: 10.1186/s12284-022-00557-5
Tardieu F. (2003). Virtual plants: modelling as a tool for the genomics of tolerance to water deficit. Trends Plant Sci. 8, 9–14. doi: 10.1016/S1360-1385(02)00008-0
Tardieu F. (2012). Any trait or trait-related allele can confer drought tolerance: just design the right drought scenario. J. Exp. Bot. 63, 25–31. doi: 10.1093/jxb/err269
Teichmann T., Muhr M. (2015). Shaping plant architecture. Front. Plant Sci. 6. doi: 10.3389/fpls.2015.00233
Thomas H., Ougham H. (2014). The stay-green trait. J. Exp. Bot. 65, 3889–3900. doi: 10.1093/jxb/eru037
Thurling N. (1991). Application of the ideotype concept in breeding for higher yield in the oilseed brassicas. Field Crops Res. 26, 201–219. doi: 10.1016/0378-4290(91)90036-U
Tivoli B., Calonnec A., Richard B., Ney B., Andrivon D. (2013). Current knowledge on plant/canopy architectural traits that reduce the expression and development of epidemics. Eur. J. Plant Pathol. 135, 471–478. doi: 10.1007/s10658-012-0066-6
Trethowan R. (2014). ““Defining a genetic ideotype for crop improvement,”,” in Crop Breeding: Methods and Protocols. Eds. Fleury D., Whitford R. (New York, NY: Springer New York), 1–20. doi: 10.1007/978-1-4939-0446-4_1
Ubbens J., Stavness I. (2017). Deep plant phenomics: a deep learning platform for complex plant phenotyping tasks. Front. Plant Sci. 8, 1190. doi: 10.3389/fpls.2017.01190
Walker B., Drewry D., Slattery R., VanLoocke A., Cho Y., Ort D. (2018). Chlorophyll can be reduced in crop canopies with little penalty to photosynthesis. Plant Physiol. 176, 1215 LP–1232. doi: 10.1104/pp.17.01401
Wang Y., Burgess S., de Becker E., Long S. (2020). Photosynthesis in the fleeting shadows: an overlooked opportunity for increasing crop productivity? Plant J. 101, 874–884. doi: 10.1111/tpj.14663
Wang D., Fahad S., Saud S., Kamran M., Khan A., Khan M., et al. (2019). Morphological acclimation to agronomic manipulation in leaf dispersion and orientation to promote “Ideotype” breeding: Evidence from 3D visual modeling of “super” rice (Oryza sativa L.). Plant Physiol. Biochem. 135, 499–510. doi: 10.1016/j.plaphy.2018.11.010
Watanabe T., Hanan J. S., Room P. M., Hasegawa T., Nakagawa H., Takahashi W. (2005). Rice morphogenesis and plant architecture: Measurement, specification and the reconstruction of structural development by 3D architectural modelling. Ann. Bot. 95, 1131–1143. doi: 10.1093/aob/mci136
White J. W., Andrade-Sanchez P., Gore M. A., Bronson K. F., Coffelt T. A., Conley M. M., et al. (2012). Field-based phenomics for plant genetics research. Field Crops Res. 133, 101–112. doi: 10.1016/j.fcr.2012.04.003
Xiao D., Li Liu D., Wang B., Feng P., Waters C. (2020). Designing high-yielding maize ideotypes to adapt changing climate in the North China Plain. Agric. Syst. 181, 102805. doi: 10.1016/j.agsy.2020.102805
Xu L., Henke M., Zhu J., Kurth W., Buck-Sorlin G. (2011). A functional–structural model of rice linking quantitative genetic information with morphological development and physiological processes. Ann. Bot. 107, 817–828. doi: 10.1093/aob/mcq264
Xu R., Li C. (2023). A review of high-throughput field phenotyping systems: focusing on ground robots. Plant Phenomics 2022, 9760269. doi: 10.34133/2022/9760269
Yang C. J., Samayoa L. F., Bradbury P. J., Olukolu B. A., Xue W., York A. M., et al. (2019). The genetic architecture of teosinte catalyzed and constrained maize domestication. Proc. Natl. Acad. Sci. U.S.A. 116, 5643–5652. doi: 10.1073/pnas.1820997116
Yang Y., Wang Y., Zhan J., Shi J., Wang X., Liu G., et al. (2017). Genetic and cytological analyses of the natural variation of seed number per pod in rapeseed (Brassica napus L.). Front. Plant Sci. 8, 1890. doi: 10.3389/fpls.2017.01890
Yin X., Stam P., Kropff M., Schapendonk A. (2003). Crop modeling, QTL mapping, and their complementary role in plant breeding. Agron. J. 95, 90–98. doi: 10.2134/agronj2003.9000a
Zadoks J., Chang T., Konzak C. (1974). A decimal growth code for the growth stages of cereals. Weed Res. 14, 415–421. doi: 10.1111/j.1365-3180.1974.tb01084.x
Zhang D., Yuan Z. (2014). Molecular control of grass inflorescence development. Annu. Rev. Plant Biol. 65, 553–578. doi: 10.1146/annurev-arplant-050213-040104
Zhao Y., Qiang C., Wang X., Chen Y., Deng J., Jiang C., et al. (2019). New alleles for chlorophyll content and stay-green traits revealed by a genome wide association study in rice (Oryza sativa). Sci. Rep. 9, 1–11. doi: 10.1038/s41598-019-39280-5
Zhou L., Wang J.-K., Yi Q., Wang Y.-Z., Zhu Y.-G., Zhang Z.-H. (2007). Quantitative trait loci for seedling vigor in rice under field conditions. Field Crops Res. 100, 294–301. doi: 10.1016/j.fcr.2006.08.003
Keywords: crop breeding, elite varieties, environment, genetics, ideotype, plant physiology, yield, cereals
Citation: Carbajal-Friedrich AAJ and Burgess AJ (2024) The role of the ideotype in future agricultural production. Front. Plant Physiol. 2:1341617. doi: 10.3389/fphgy.2024.1341617
Received: 20 November 2023; Accepted: 04 January 2024;
Published: 23 January 2024.
Edited by:
Jiaxu Li, Mississippi State University, United StatesReviewed by:
Sorin Vatca, University of Agricultural Sciences and Veterinary Medicine of Cluj-Napoca, RomaniaGiovanni Melandri, University of Arizona, United States
Copyright © 2024 Carbajal-Friedrich and Burgess. This is an open-access article distributed under the terms of the Creative Commons Attribution License (CC BY). The use, distribution or reproduction in other forums is permitted, provided the original author(s) and the copyright owner(s) are credited and that the original publication in this journal is cited, in accordance with accepted academic practice. No use, distribution or reproduction is permitted which does not comply with these terms.
*Correspondence: Alexandra J. Burgess, QWxleGFuZHJhLmJ1cmdlc3NAbm90dGluZ2hhbS5hYy51aw==