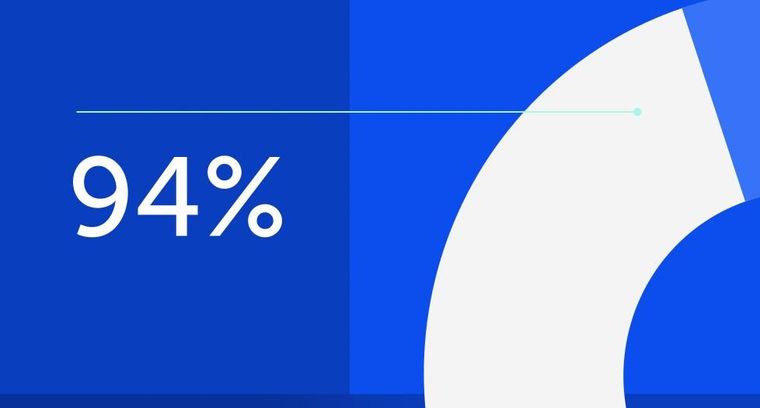
94% of researchers rate our articles as excellent or good
Learn more about the work of our research integrity team to safeguard the quality of each article we publish.
Find out more
ORIGINAL RESEARCH article
Front. Physiol.
Sec. Computational Physiology and Medicine
Volume 16 - 2025 | doi: 10.3389/fphys.2025.1581699
The final, formatted version of the article will be published soon.
Select one of your emails
You have multiple emails registered with Frontiers:
Notify me on publication
Please enter your email address:
If you already have an account, please login
You don't have a Frontiers account ? You can register here
Freezing of Gait (FoG) is a disabling symptom in Parkinson’s Disease (PD) that substantially4 affects patient ability to movement and hence quality of life. The current research aims to introduce5 a framework that is based on advanced AI techniques such as deep learning techniques that6 utilizes a more special type of deep learning models known as Convolutional Neural Networks7 (CNNs) for spatial characteristics discover and a sequence based model known as Bidirectional8 Long Short-Term Memory (BiLSTM) networks for temporal modeling, complemented by an9 attention mechanism to pinpoint essential gait features. The approach is trained with multimodal10 sensor data across sources like tDCS FoG, DeFOG, Daily Living, and Hantao’s Multimodal11 datasets in order to establish robustness and generalizability over populations of varied natures.12 Preeminent issues of sensor noise, inter-subject variation, and class imbalance are countered13 with deep preprocessing operations, i.e., sensor fusion, normalization, and data augmentation.14 The model exhibits high performance with accuracy (98%), F1-score (94%), and AUC (96%) over15 existing methods for detecting FoG episodes. The present research shows the ability of AI-aided16 analysis of wearable sensor data in enhancing diagnostic accuracy, therapy personalization, and17 monitoring of unfortunate people having neurological disability and advances the wider context of18 AI application in medicine.
Keywords: Wearable sensor, freezing of gait, deep learning, attention mechanism, Artifcial intelligence
Received: 28 Feb 2025; Accepted: 14 Apr 2025.
Copyright: © 2025 Al-Adhaileh, Wadood, Aldhyani, Khan, Uddin and Al-Nefaie. This is an open-access article distributed under the terms of the Creative Commons Attribution License (CC BY). The use, distribution or reproduction in other forums is permitted, provided the original author(s) or licensor are credited and that the original publication in this journal is cited, in accordance with accepted academic practice. No use, distribution or reproduction is permitted which does not comply with these terms.
* Correspondence: Theyazn H.H Aldhyani, King Faisal University, Al-Ahsa, Saudi Arabia
Disclaimer: All claims expressed in this article are solely those of the authors and do not necessarily represent those of their affiliated organizations, or those of the publisher, the editors and the reviewers. Any product that may be evaluated in this article or claim that may be made by its manufacturer is not guaranteed or endorsed by the publisher.
Supplementary Material
Research integrity at Frontiers
Learn more about the work of our research integrity team to safeguard the quality of each article we publish.