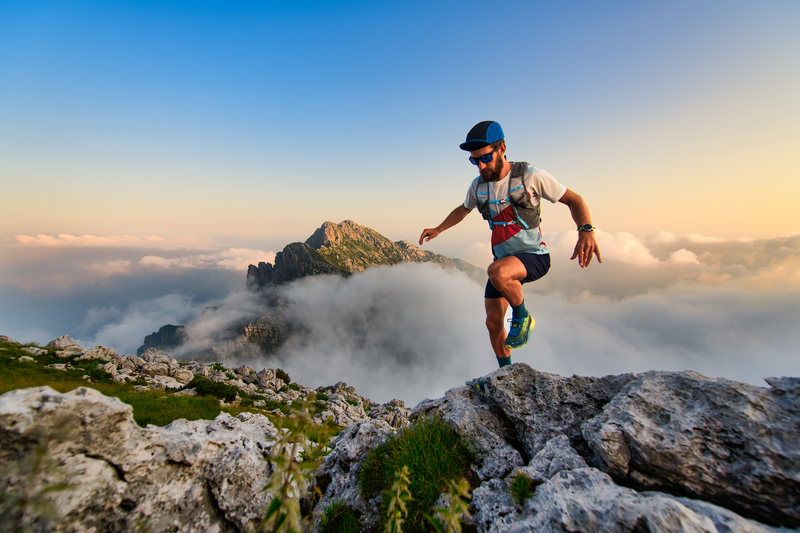
95% of researchers rate our articles as excellent or good
Learn more about the work of our research integrity team to safeguard the quality of each article we publish.
Find out more
EDITORIAL article
Front. Physiol. , 05 February 2025
Sec. Computational Physiology and Medicine
Volume 16 - 2025 | https://doi.org/10.3389/fphys.2025.1562750
This article is part of the Research Topic Integrating Machine Learning with Physics-based Modelling of Physiological Systems View all 6 articles
Editorial on the Research Topic
Integrating machine learning with physics-based modeling of physiological systems
Physics-based modeling has long been instrumental in advancing our understanding of physiological systems, including cardiovascular, neurovascular, pulmonary, and gastrointestinal systems. These models, rooted in mathematical and computational frameworks, have provided detailed insights into the underlying mechanisms of complex biological phenomena. Complementing this, machine learning (ML) has emerged as a transformative tool in computational research. By integrating these two approaches, researchers aim to overcome persistent challenges in physiological modeling and advance both scientific understanding and clinical applications.
The integration of machine learning with physics-based modeling leverages their complementary strengths: data-driven insights from ML and mechanistic understanding from physics-based models. Physics-based approaches often face bottlenecks, such as computational expense, limited grid resolution, and challenges in handling large datasets. Machine learning has shown great promise in alleviating these limitations by enhancing computational efficiency, automating data processing, and improving parameter estimation. This Research Topic explores the intersection of these methodologies, showcasing innovative applications and developments in this interdisciplinary field.
“Intracranial Pressure-Flow Relationships in Traumatic Brain Injury Patients Expose Gaps in the Tenets of Models and Pressure-Oriented Management”. In their study, Stroh et al. investigate intracranial pressure-flow relationships in patients with traumatic brain injury (TBI), critically evaluating the assumptions underlying current management protocols. By integrating physics-based hemodynamic models with multimodality monitoring data, the authors identify novel dynamical regimes, such as zero and negative pressure-flow relationships, where standard assumptions fail. While the study primarily focuses on validating these regimes and refining mechanistic models, it highlights the potential of data-driven approaches, including machine learning, for future personalization of TBI care. Their findings challenge traditional frameworks, providing a foundation for improving critical care through a deeper understanding of intracranial hemodynamics.
“Pole Balancing on the Fingertip: Model-Motivated Machine Learning Forecasting of Falls”. Depnath et al. investigate the dynamics of human pole balancing on a fingertip by combining a physics-based inverted pendulum-cart model with machine learning techniques. Using a long short-term memory (LSTM) network, the study predicts falls based on both synthetic data generated from the mathematical model and experimental human balancing data. The innovative use of transfer learning—pre-training on synthetic data and fine-tuning with real-world data—enables the model to forecast 60%–70% of falls up to 2.35 s in advance, demonstrating a notable improvement in performance. By addressing data scarcity and bridging synthetic and real-world data, the authors demonstrate how machine learning can tackle challenges in chaotic physiological systems, providing a framework applicable to domains like biomechanics and neuroscience.
“Using Dropout Based Active Learning and Surrogate Models in the Inverse Viscoelastic Parameter Identification of Human Brain Tissue”. Inverse parameter identification in biomechanics often requires computationally intensive finite element (FE) simulations. Hinrichsen et al. address this challenge by combining a generalized Maxwell viscoelastic FE model with a recurrent neural network (RNN) surrogate model. The surrogate approximates the output of FE simulations, significantly reducing computational costs while maintaining high fidelity. Dropout-based active learning further enhances efficiency by selecting the most informative training data. The surrogate model is applied to identify material parameters for human brain tissue, showcasing how machine learning can accelerate and streamline complex computational tasks. This study demonstrates the potential of ML in reducing computational costs while maintaining high fidelity in physics-based modeling.
“Neural Network-Based Estimation of Biomechanical Vocal Fold Parameters”. The biomechanics of vocal fold vibrations play a crucial role in voice physiology, yet accessing the underlying biomechanical parameters remains challenging. Donhauser et al. present a convolutional recurrent neural network (CRNN) trained on synthetic data from a six-mass model (6 MM) to estimate key biomechanical parameters, such as subglottal pressure, stiffness, and mass, from high-speed video (HSV) data. This approach replaces computationally expensive optimization with a neural network surrogate, achieving significant efficiency gains. Their work advances the field of vocal fold modeling, offering a fast and accurate method for analyzing laryngeal kinematics and improving diagnostics for voice disorders.
“MRI-MECH: Mechanics-Informed MRI to Estimate Esophageal Health”. In the final contribution, Halder et al. introduce MRI-MECH, a mechanics-informed neural network framework for assessing esophageal health non-invasively using dynamic MRI data. By integrating computational modeling with dynamic imaging, this approach estimates critical health metrics such as wall stiffness and active relaxation without invasive procedures. Physics-informed neural network enhances the framework’s ability to overcome limitations in traditional imaging, such as poor resolution or missing data, while ensuring that its predictions adhere to fundamental physical principles, including mass and momentum conservation equations. It demonstrates the potential of combining machine learning with physics-based modeling to improve gastrointestinal diagnostics, setting the stage for broader applications in non-invasive medical imaging and physiological analysis.
The five articles in this Research Topic underscore the impact of integrating machine learning with physics-based modeling across various physiological domains. They introduce innovative solutions that merge the predictive power of ML with the mechanistic insights of physics-based models.
The integration of machine learning with physics-based modeling represents a paradigm shift in computational physiology. By bridging the gap between data-driven methods and mechanistic understanding, these approaches offer transformative potential for both research and clinical practice. Future directions may include developing hybrid models that seamlessly combine ML and physics-based components, as well as standardizing methodologies and datasets for broader applicability.
As computational power continues to grow, the accessibility of these integrated approaches will expand, enabling their application to even more complex and multiscale systems, such as molecular dynamics and organ-level systems. The studies presented here exemplify how interdisciplinary collaborations can enhance our ability to model, understand, and treat physiological systems.
In conclusion, the articles in this Research Topic represent cutting-edge developments in integrating machine learning with physics-based modeling. By addressing computational inefficiencies and enhancing predictive accuracy, they not only advance the state of the art in computational physiology but also inspire new possibilities for tackling some of the most pressing challenges in biomedical research and healthcare.
JL: Writing–original draft, Writing–review and editing. HG: Writing–review and editing. MD: Writing–review and editing.
The author(s) declare that no financial support was received for the research, authorship, and/or publication of this article.
The authors declare that the research was conducted in the absence of any commercial or financial relationships that could be construed as a potential conflict of interest.
The author(s) declared that they were an editorial board member of Frontiers, at the time of submission. This had no impact on the peer review process and the final decision.
The author(s) declare that no Generative AI was used in the creation of this manuscript.
All claims expressed in this article are solely those of the authors and do not necessarily represent those of their affiliated organizations, or those of the publisher, the editors and the reviewers. Any product that may be evaluated in this article, or claim that may be made by its manufacturer, is not guaranteed or endorsed by the publisher.
The views here reflect the views of the author and should not be construed to represent U.S. FDA’s views or policies.
Keywords: physics-based modeling, modeling and simulation (CM&S), computational medicine and biology, artificial intelligence, machine learning
Citation: Lee JH, Gao H and Döllinger M (2025) Editorial: Integrating machine learning with physics-based modeling of physiological systems. Front. Physiol. 16:1562750. doi: 10.3389/fphys.2025.1562750
Received: 18 January 2025; Accepted: 21 January 2025;
Published: 05 February 2025.
Edited and reviewed by:
Raimond L. Winslow, Northeastern University, United StatesCopyright © 2025 Lee, Gao and Döllinger. This is an open-access article distributed under the terms of the Creative Commons Attribution License (CC BY). The use, distribution or reproduction in other forums is permitted, provided the original author(s) and the copyright owner(s) are credited and that the original publication in this journal is cited, in accordance with accepted academic practice. No use, distribution or reproduction is permitted which does not comply with these terms.
*Correspondence: Jae H. Lee, amVobzYzMTdAZ21haWwuY29t
Disclaimer: All claims expressed in this article are solely those of the authors and do not necessarily represent those of their affiliated organizations, or those of the publisher, the editors and the reviewers. Any product that may be evaluated in this article or claim that may be made by its manufacturer is not guaranteed or endorsed by the publisher.
Research integrity at Frontiers
Learn more about the work of our research integrity team to safeguard the quality of each article we publish.