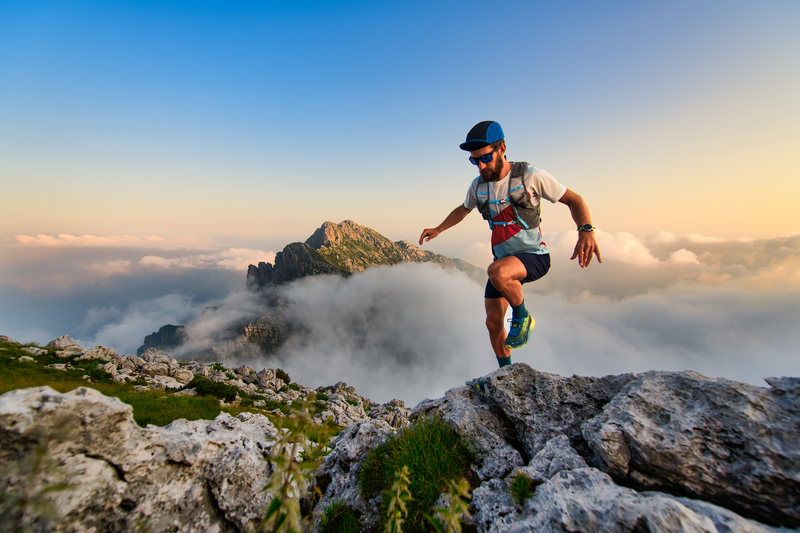
94% of researchers rate our articles as excellent or good
Learn more about the work of our research integrity team to safeguard the quality of each article we publish.
Find out more
ORIGINAL RESEARCH article
Front. Physiol.
Sec. Aquatic Physiology
Volume 16 - 2025 | doi: 10.3389/fphys.2025.1562665
The final, formatted version of the article will be published soon.
You have multiple emails registered with Frontiers:
Please enter your email address:
If you already have an account, please login
You don't have a Frontiers account ? You can register here
This study investigated the heart rate (HR) and acceleration (AC) dynamics of Atlantic salmon (Salmo salar) during a swim fitness test in a swim tunnel. Experimental fish were implanted with data loggers equipped with HR and AC sensors. These fish, and controls that were not implanted, were subjected to a swim-fitness test at incremental speeds from 0.2 to 1.0 m.s–1. Oxygen consumption (MO2) and locomotory behavior were monitored. Subsequently, these fish were subjected to a stress challenge test to further study the link between induced stress and HR and AC. When swimming from 0.2 to 1.0 m.s–1, the HR of implanted fish (N= 19) was high in the swim tunnels but remained stable between 82 to 84 beats per minute (bpm), despite significant increases in MO2, AC, tail beat frequency (TBF) and head width frequency (HWF). The stable HR observed was also reflected by HR explaining only ~15% of the variation in MO2. MO2 of implanted fish increased from 238 to 343 mg.kg–1.h–1 when swimming from 0.4 to 1.0 m.s–1. With increasing swimming speeds, AC values of implanted fish increased from 16 to 27 milli-g and explained ~40% of the variation in MO2. TBF increased linearly with swimming speed, and from 0.4 m.s–1 onward, it correlated strongly with MO2, similarly for HWF. Under controlled stress conditions, the HR values of fish were significantly higher than baseline conditions but similar during stress regardless of intensity. Also, AC showed similar stress peak patterns as HR. From these results, we conclude that the increased oxygen demand when swimming at increasing speeds is not met by increasing HR alone in Atlantic salmon. This supports the hypothesis that stroke volume plays an important role in boosting cardiac output. AC, in contrast to HR, showed a strong positive correlation with MO2 during swim-fitness tests and may serve as a reliable predictor of energy expenditure. TBF and HWF may also be useful predictors, but HR is not in Atlantic salmon. HR did show positive responses to induced stress but, similar to swimming, up to maximum values under 90 bpm.
Keywords: swimming physiology, Oxygen Consumption, sensor implants, Fish Behavior, Aquaculture
Received: 17 Jan 2025; Accepted: 05 Mar 2025.
Copyright: © 2025 Agbeti, Palstra, Black, Magnoni, Lankheet and Komen. This is an open-access article distributed under the terms of the Creative Commons Attribution License (CC BY). The use, distribution or reproduction in other forums is permitted, provided the original author(s) or licensor are credited and that the original publication in this journal is cited, in accordance with accepted academic practice. No use, distribution or reproduction is permitted which does not comply with these terms.
* Correspondence:
Wisdom Enyam Kodzo Agbeti, Animal Breeding and Genomics, Wageningen University and Research, Wageningen, 6708 PB, Netherlands
Disclaimer: All claims expressed in this article are solely those of the authors and do not necessarily represent those of their affiliated organizations, or those of the publisher, the editors and the reviewers. Any product that may be evaluated in this article or claim that may be made by its manufacturer is not guaranteed or endorsed by the publisher.
Research integrity at Frontiers
Learn more about the work of our research integrity team to safeguard the quality of each article we publish.