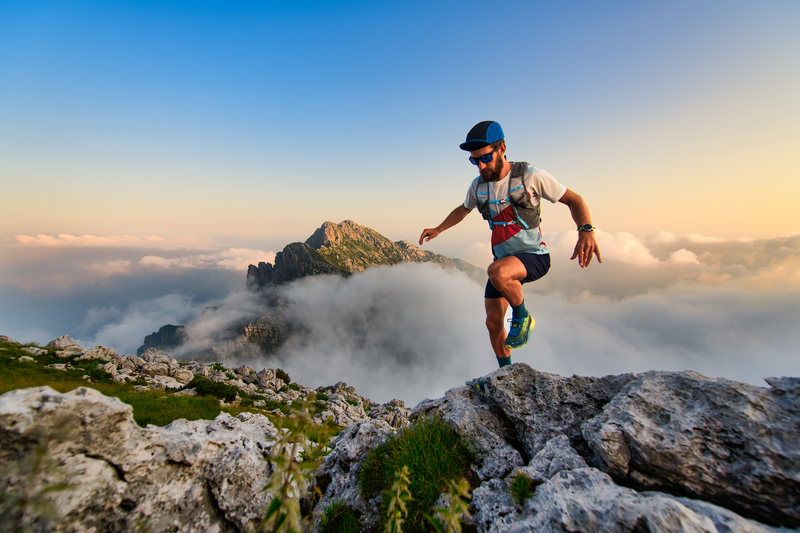
94% of researchers rate our articles as excellent or good
Learn more about the work of our research integrity team to safeguard the quality of each article we publish.
Find out more
ORIGINAL RESEARCH article
Front. Physiol.
Sec. Computational Physiology and Medicine
Volume 16 - 2025 | doi: 10.3389/fphys.2025.1558997
This article is part of the Research Topic Medical Knowledge-Assisted Machine Learning Technologies in Individualized Medicine Volume II View all 9 articles
The final, formatted version of the article will be published soon.
You have multiple emails registered with Frontiers:
Please enter your email address:
If you already have an account, please login
You don't have a Frontiers account ? You can register here
Objective: This study aimed to develop and validate a multimodal deep learning model that utilizes preoperative grayscale and contrast-enhanced ultrasound (CEUS) video data for noninvasive WHO/ISUP nuclear grading of renal cell carcinoma (RCC).In this dual-center retrospective study, CEUS videos from 100 patients with RCC collected between June 2012 and June 2021 were analyzed. A total of 6,293 ultrasound images were categorized into low-grade (G1-G2) and high-grade (G3-G4) groups. A novel model, the Multimodal Ultrasound Fusion Network (MUF-Net), integrated B-mode and CEUS modalities to extract and fuse image features using a weighted sum of predicted weights. Model performance was assessed using five-fold cross-validation and compared to single-modality models. Grad-CAM visualization highlighted key regions influencing the model's predictions.Results: MUF-Net achieved an accuracy of 85.9%, outperforming B-mode (80.8%) and CEUS-mode (81.8%, P < 0.05) models. Sensitivities were 85.1%, 80.2%, and 77.8%, while specificities were 86.0%, 82.5%, and 82.7%, respectively. The AUC of MUF-Net (0.909, 95% CI: 0.829-0.990) was superior to B-mode (0.838, 95% CI: 0.689-0.988) and CEUS-mode (0.845, 95% CI: 0.745-0.944). Grad-CAM analysis revealed distinct and complementary salient regions across modalities.Conclusions: MUF-Net provides accurate and interpretable RCC nuclear grading, surpassing unimodal approaches, with Grad-CAM offering intuitive insights into the model's predictions.
Keywords: Renal tumor, artificial intelligence, Classification, deep learning, WHO/ISUP grading system, contrast-enhanced ultrasound
Received: 11 Jan 2025; Accepted: 24 Feb 2025.
Copyright: © 2025 Zhu, Wu, Long, Li, Luo, Pang, Zhu and Luo. This is an open-access article distributed under the terms of the Creative Commons Attribution License (CC BY). The use, distribution or reproduction in other forums is permitted, provided the original author(s) or licensor are credited and that the original publication in this journal is cited, in accordance with accepted academic practice. No use, distribution or reproduction is permitted which does not comply with these terms.
* Correspondence:
Yixin Zhu, Shenzhen Hospital, Peking University, Shenzhen, 518036, Beijing Municipality, China
Disclaimer: All claims expressed in this article are solely those of the authors and do not necessarily represent those of their affiliated organizations, or those of the publisher, the editors and the reviewers. Any product that may be evaluated in this article or claim that may be made by its manufacturer is not guaranteed or endorsed by the publisher.
Research integrity at Frontiers
Learn more about the work of our research integrity team to safeguard the quality of each article we publish.