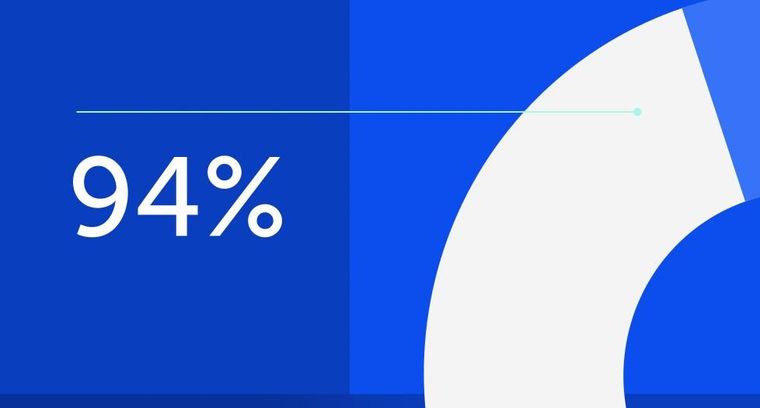
94% of researchers rate our articles as excellent or good
Learn more about the work of our research integrity team to safeguard the quality of each article we publish.
Find out more
ORIGINAL RESEARCH article
Front. Physiol.
Sec. Computational Physiology and Medicine
Volume 16 - 2025 | doi: 10.3389/fphys.2025.1558001
The final, formatted version of the article will be published soon.
Select one of your emails
You have multiple emails registered with Frontiers:
Notify me on publication
Please enter your email address:
If you already have an account, please login
You don't have a Frontiers account ? You can register here
Breast cancer (BC) is a malignant neoplasm that originates in the mammary gland's cellular structures and remains one of the most prevalent cancers among women, ranking second in cancer-related mortality after lung cancer. Early and accurate diagnosis is crucial due to the heterogeneous nature of breast cancer and its rapid progression. However, manual detection and classification are often time-consuming and prone to errors, necessitating the development of automated and reliable diagnostic approaches. Recent advancements in deep learning have significantly improved medical image analysis, demonstrating superior predictive performance in breast cancer detection using ultrasound images. Despite these advancements, training deep learning models from scratch can be computationally expensive and dataintensive. Transfer learning, leveraging pre-trained models on large-scale datasets, offers an effective solution to mitigate these challenges. In this study, we investigate and compare multiple deep-learning models for breast cancer classification using transfer learning. The evaluated architectures include modified InceptionV3, GoogLeNet, ShuffleNet, AlexNet, VGG-16, and SqueezeNet. Additionally, we propose a deep neural network model that integrates features from modified InceptionV3 to further enhance classification performance. The experimental results demonstrate that the modified InceptionV3 model achieves the highest classification accuracy of 99.10%, with a recall of 98.90%, precision of 99.00%, and an F1-score of 98.80%, outperforming all other evaluated models on the given datasets. These findings underscore the potential of the proposed approach in enhancing diagnostic precision.
Keywords: breast cancer, deep learning, InceptionV3, Ultrasound images, Transfer Learning
Received: 18 Jan 2025; Accepted: 07 Apr 2025.
Copyright: © 2025 Alnashwan, Ba Mahel, Chelloug, Rafiq, Muthanna and Aziz. This is an open-access article distributed under the terms of the Creative Commons Attribution License (CC BY). The use, distribution or reproduction in other forums is permitted, provided the original author(s) or licensor are credited and that the original publication in this journal is cited, in accordance with accepted academic practice. No use, distribution or reproduction is permitted which does not comply with these terms.
* Correspondence: Rana Alnashwan, Department of Information Technology, College of Computer and Information Sciences, Princess Nourah bint Abdulrahman University, Saudi Arabia, Riyadh, 84428, Riyadh, Saudi Arabia
Disclaimer: All claims expressed in this article are solely those of the authors and do not necessarily represent those of their affiliated organizations, or those of the publisher, the editors and the reviewers. Any product that may be evaluated in this article or claim that may be made by its manufacturer is not guaranteed or endorsed by the publisher.
Supplementary Material
Research integrity at Frontiers
Learn more about the work of our research integrity team to safeguard the quality of each article we publish.