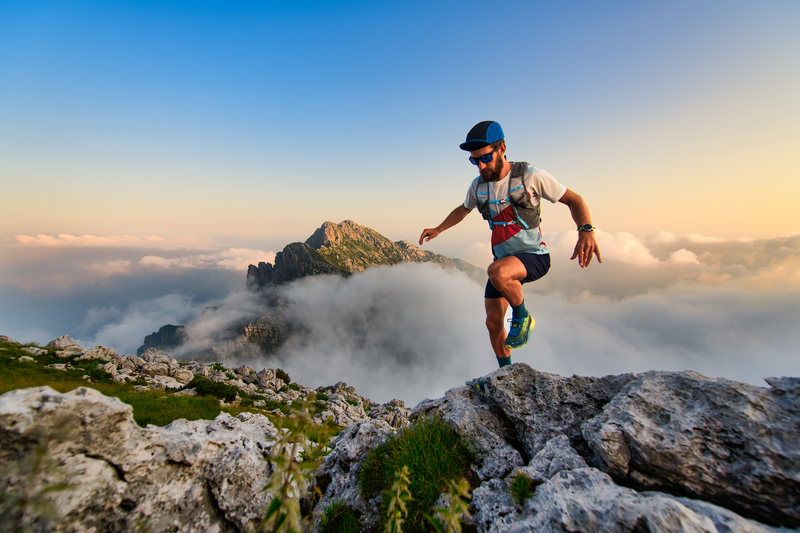
94% of researchers rate our articles as excellent or good
Learn more about the work of our research integrity team to safeguard the quality of each article we publish.
Find out more
ORIGINAL RESEARCH article
Front. Physiol.
Sec. Respiratory Physiology and Pathophysiology
Volume 16 - 2025 | doi: 10.3389/fphys.2025.1545652
This article is part of the Research Topic Multidimensional Physiology: Novel Techniques and Discoveries with Bioimpedance Measurements, Volume II View all 6 articles
The final, formatted version of the article will be published soon.
You have multiple emails registered with Frontiers:
Please enter your email address:
If you already have an account, please login
You don't have a Frontiers account ? You can register here
AbstractIntroduction: Electrical impedance tomography (EIT) enables non-invasive, continuous, bedside evaluation of ventilation/perfusion (V/Q) match. To avoid the presence of invasive monitoring for cardiac output in relative V/Q ratio calculation, we proposed a novel calibration method based on arterial blood pressure to optimize EIT V/Q match assessments.Methods: We involved 12 mechanically ventilated piglets in three experimental phases: baseline, pulmonary embolism, and atelectasis. After a thorough measurement of EIT signals, arterial blood pressure, cardiac output, and additional physiological parameters, EIT V/Q match was evaluated using existing area limited method (ALM), cardiac output calibrated method (COCM), and our proposed novel blood pressure calibrated method (BPCM). Finally, VD/VT and P/F ratio were calculated and correlated with V/Q match indicators derived from COCM and BPCM.Results: Arterial blood pressure waveform integration demonstrated strong correlation with cardiac output (R² = 0.80, p < 0.001), validating its utility for cardiac output estimation and V/Q match calibration. Both COCM and BPCM provided enhanced V/Q match region segmentation compared to ALM, yielding comprehensive diagnostic information with statistically significant differences across all three states (p < 0.05). COCM demonstrates a slightly higher correlation compared to BPCM (r = -0.63 vs -0.52) between low ventilation index (LVI) and VD/VT, while BPCM demonstrates a slightly higher correlation compared to COCM (r = 0.49 vs 0.44) between low perfusion index (LQI) and P/F ratio.Conclusion: This study described a novel calibration method for calculating corrected EIT-based V/Q match that utilized arterial blood pressure. Our method exhibited comparable capability in distinguishing V/Q mismatch areas compared to conventional cardiac output-based calibration techniques. With clinical data to establish a linear regression model, our method will ultimately enable us to calculate calibrated EIT V/Q match without cardiac output monitoring.
Keywords: electrical impedance tomography1, Pulmonary embolism2, atelectasis3, V/Q match4, arterial blood pressure5, calibration method6
Received: 15 Dec 2024; Accepted: 18 Feb 2025.
Copyright: © 2025 Han, Qin, Zhao, Yang, Liu, Li, Wei, Wei, Liu and Fu. This is an open-access article distributed under the terms of the Creative Commons Attribution License (CC BY). The use, distribution or reproduction in other forums is permitted, provided the original author(s) or licensor are credited and that the original publication in this journal is cited, in accordance with accepted academic practice. No use, distribution or reproduction is permitted which does not comply with these terms.
* Correspondence:
Yifan Liu, Air Force Medical University, Xi'an, Shaanxi, China
Feng Fu, Air Force Medical University, Xi'an, Shaanxi, China
Disclaimer: All claims expressed in this article are solely those of the authors and do not necessarily represent those of their affiliated organizations, or those of the publisher, the editors and the reviewers. Any product that may be evaluated in this article or claim that may be made by its manufacturer is not guaranteed or endorsed by the publisher.
Research integrity at Frontiers
Learn more about the work of our research integrity team to safeguard the quality of each article we publish.