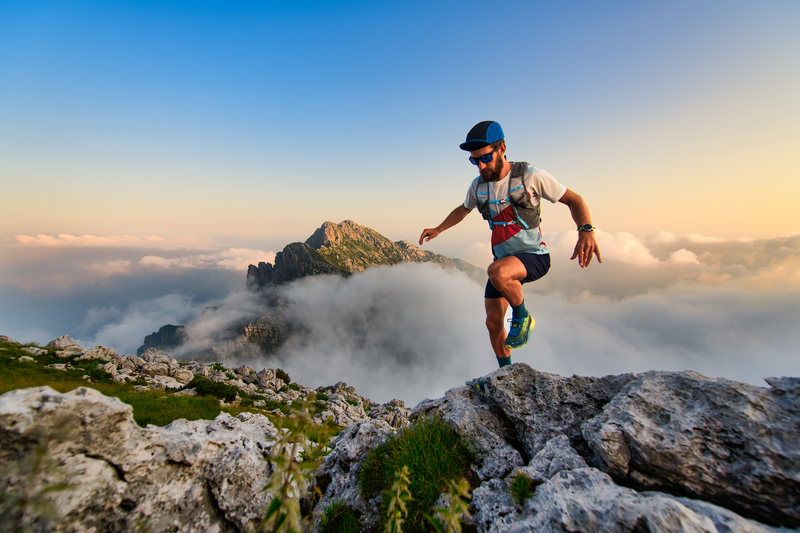
95% of researchers rate our articles as excellent or good
Learn more about the work of our research integrity team to safeguard the quality of each article we publish.
Find out more
ORIGINAL RESEARCH article
Front. Physiol.
Sec. Chronobiology
Volume 16 - 2025 | doi: 10.3389/fphys.2025.1535331
The final, formatted version of the article will be published soon.
You have multiple emails registered with Frontiers:
Please enter your email address:
If you already have an account, please login
You don't have a Frontiers account ? You can register here
Assessing real-time stress in individuals to prevent the accumulation of stress is necessary due to the adverse effects of excessive psychological stress on health. Since both stress and circadian rhythms affect the excitability of the nervous system, the influence of circadian rhythms needs to be considered during stress assessment. Most studies train classifiers using physiological data collected during fixed short time periods, overlooking the assessment of stress levels at other times. In this work, we propose a method for training a classifier that can identify stress and resting states throughout the day, based on 10 short-term heart rate variability (HRV) feature data obtained from morning, noon, and evening. Collecting and analyzing the heartbeat interval data of all volunteers over three consecutive days, we show that all HRV features exhibit 12-hour and 24-hour circadian rhythms. In addition, the circadian rhythm differences across different days for individuals are relatively small. After removing the circadian rhythm trends from the HRV feature data using the Smoothness Priors Approach (SPA), we train XGBoost models to assess stress.The results show that using detrended data as the training set can improve the overall accuracy of stress assessment across all time periods. When combining data from different time periods as the training dataset, the accuracy of the classifier trained on detrended data increases by 13.67%. These indicate that using HRV features with circadian rhythm trends removed is an effective method for assessing stress at all times throughout the day.
Keywords: stress assessment, Heart rate variability, Circadian Rhythm, feature extraction, XGBoost
Received: 27 Nov 2024; Accepted: 31 Mar 2025.
Copyright: © 2025 Shen, Fang, Zhang, Yu, Xu and Yang. This is an open-access article distributed under the terms of the Creative Commons Attribution License (CC BY). The use, distribution or reproduction in other forums is permitted, provided the original author(s) or licensor are credited and that the original publication in this journal is cited, in accordance with accepted academic practice. No use, distribution or reproduction is permitted which does not comply with these terms.
* Correspondence:
Ying Xu, Jiangsu Key Laboratory of Neuropsychiatric Diseases and Cambridge-Su Genomic Resource Center, Suzhou medical college of Soochow University, Suzhou, China
Ling Yang, School of Mathematical Sciences, Soochow University, Suzhou, Jiangsu Province, China
Disclaimer: All claims expressed in this article are solely those of the authors and do not necessarily represent those of their affiliated organizations, or those of the publisher, the editors and the reviewers. Any product that may be evaluated in this article or claim that may be made by its manufacturer is not guaranteed or endorsed by the publisher.
Research integrity at Frontiers
Learn more about the work of our research integrity team to safeguard the quality of each article we publish.