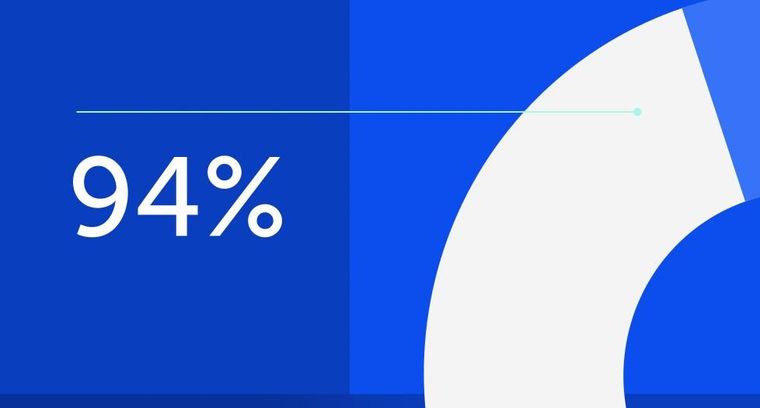
94% of researchers rate our articles as excellent or good
Learn more about the work of our research integrity team to safeguard the quality of each article we publish.
Find out more
ORIGINAL RESEARCH article
Front. Physiol.
Sec. Computational Physiology and Medicine
Volume 16 - 2025 | doi: 10.3389/fphys.2025.1527751
The final, formatted version of the article will be published soon.
Select one of your emails
You have multiple emails registered with Frontiers:
Notify me on publication
Please enter your email address:
If you already have an account, please login
You don't have a Frontiers account ? You can register here
Tongue diagnosis in Traditional Chinese Medicine (TCM) plays a crucial role in clinical practice. By observing the shape, color, and coating of the tongue, practitioners can assist in determining the nature and location of a disease. However, the field of tongue diagnosis currently faces challenges such as data scarcity and a lack of efficient multimodal diagnostic models, making it difficult to fully align with TCM theories and clinical needs. Additionally, existing methods generally lack multi-label classification capabilities, making it challenging to simultaneously meet the multidimensional requirements of TCM diagnosis for disease nature and location. To address these issues, this paper proposes TongueNet, a multimodal deep learning model that integrates tongue image data with text-based features. The model utilizes a Hierarchical Aggregation Network (HAN) and a Feature Space Projection Module to efficiently extract and fuse features while introducing consistency and complementarity constraints to optimize multimodal information fusion. Furthermore, the model incorporates a multi-scale attention mechanism (EMA) to enhance the diversity and accuracy of feature weighting and employs a Kolmogorov-Arnold Network (KAN) instead of traditional MLPs for output optimization, thereby improving the representation of complex features. For model training, this study integrates three publicly available tongue image datasets from the Roboflow platform and enlists multiple experts for multimodal annotation, incorporating multi-label information on disease nature and location to align with TCM clinical needs. Experimental results demonstrate that TongueNet outperforms existing models in both disease nature and disease location classification tasks. Specifically, in the disease nature classification task, it achieves 89.12% accuracy and an AUC of 83%; in the disease location classification task, it achieves 86.47% accuracy and an AUC of 81%. Moreover, TongueNet contains only 32.1M parameters, significantly reducing computational resource requirements 1 Sample et al. while maintaining high diagnostic performance. TongueNet provides a new approach for the intelligent development of TCM tongue diagnosis.
Keywords: Traditional Chinese medicine (TCM), multimodal fusion, Tongue diagnosis, deep learning, multi-label classification
Received: 05 Dec 2024; Accepted: 09 Apr 2025.
Copyright: © 2025 Yang, Dong, Lin and Lü. This is an open-access article distributed under the terms of the Creative Commons Attribution License (CC BY). The use, distribution or reproduction in other forums is permitted, provided the original author(s) or licensor are credited and that the original publication in this journal is cited, in accordance with accepted academic practice. No use, distribution or reproduction is permitted which does not comply with these terms.
* Correspondence: Xinliang Lü, Inner Mongolia Autonomous Region Traditional Chinese Medicine Hospital, Hohhot, Inner Mongolia Autonomous Region, China
Disclaimer: All claims expressed in this article are solely those of the authors and do not necessarily represent those of their affiliated organizations, or those of the publisher, the editors and the reviewers. Any product that may be evaluated in this article or claim that may be made by its manufacturer is not guaranteed or endorsed by the publisher.
Supplementary Material
Research integrity at Frontiers
Learn more about the work of our research integrity team to safeguard the quality of each article we publish.