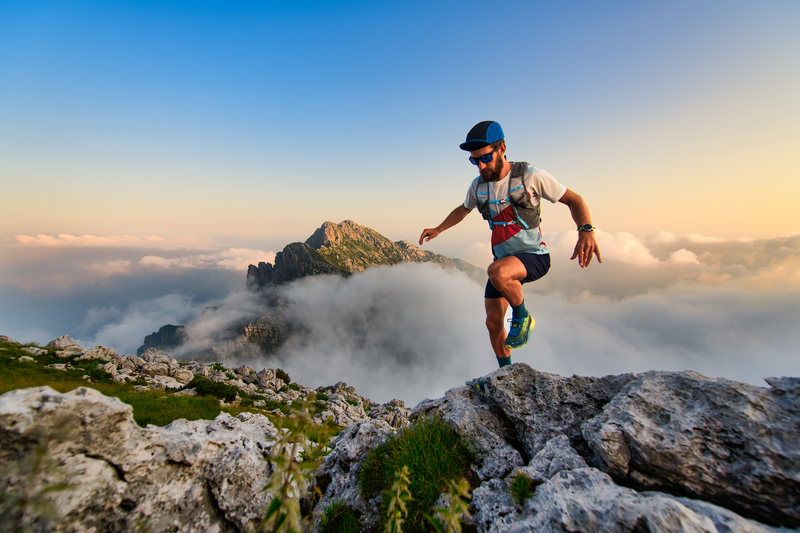
94% of researchers rate our articles as excellent or good
Learn more about the work of our research integrity team to safeguard the quality of each article we publish.
Find out more
ORIGINAL RESEARCH article
Front. Physiol.
Sec. Avian Physiology
Volume 16 - 2025 | doi: 10.3389/fphys.2025.1520826
This article is part of the Research Topic The Effect of Muscle Pathogenesis on Avian Physiology, Animal Welfare, and Quality of Muscle as a Food View all 12 articles
The final, formatted version of the article will be published soon.
You have multiple emails registered with Frontiers:
Please enter your email address:
If you already have an account, please login
You don't have a Frontiers account ? You can register here
Over the past decades, MDV has dramatically evolved towards more virulent strains and remains a persistent threat to the world's poultry industry. We performed genome-wide gene expression analysis in the spleen, thymus, and bursa tissues from MD-resistant line and susceptible line to explore the mechanism of MD resistance and susceptibility. We identified genes and pathways associated with the transcriptional response to MDV infection using the robust RNA sequencing approach. The transcriptome analysis revealed a tissue-specific expression pattern among immune organs when confronting MDV. At pathway and network levels, MDV infections influenced cytokine-cytokine receptor interaction and cellular development in resistant and susceptible chicken lines. Meanwhile, we also observed different genetic responses between the two chicken lines: some pathways like herpes simplex infection and influenza A were found in MD resistant line spleen tissues, whereas metabolic-related pathways and DNA replication could only be observed in MD susceptible line chickens. In summary, our research renders new perceptions of the MD progression mechanism and beckons further gene function studies into MD resistance.
Keywords: Marek's disease, Marek's disease virus, Poultry health, Immune organs, gene exression
Received: 31 Oct 2024; Accepted: 06 Jan 2025.
Copyright: © 2025 Song, Ding, Dunn, Zhang and Zhao. This is an open-access article distributed under the terms of the Creative Commons Attribution License (CC BY). The use, distribution or reproduction in other forums is permitted, provided the original author(s) or licensor are credited and that the original publication in this journal is cited, in accordance with accepted academic practice. No use, distribution or reproduction is permitted which does not comply with these terms.
* Correspondence:
Jiuzhou Song, Animal and Avian Sciences, University of Maryland, College Park, College Park, 20742, MD, United States
Yi Ding, Allen Institute for Brain Science, Seattle, 98109, Washington, United States
Disclaimer: All claims expressed in this article are solely those of the authors and do not necessarily represent those of their affiliated organizations, or those of the publisher, the editors and the reviewers. Any product that may be evaluated in this article or claim that may be made by its manufacturer is not guaranteed or endorsed by the publisher.
Research integrity at Frontiers
Learn more about the work of our research integrity team to safeguard the quality of each article we publish.