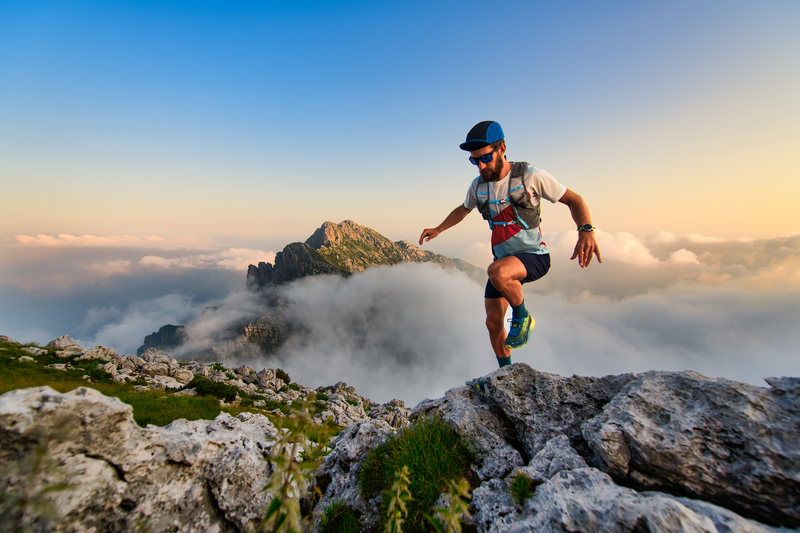
94% of researchers rate our articles as excellent or good
Learn more about the work of our research integrity team to safeguard the quality of each article we publish.
Find out more
PERSPECTIVE article
Front. Physiol. , 07 January 2025
Sec. Exercise Physiology
Volume 15 - 2024 | https://doi.org/10.3389/fphys.2024.1517355
Cardiorespiratory fitness (CRF) and muscular fitness are powerful confounders in age and sex-related comparisons. This paper provides a perspective on the benefits and limitations of matching participants by physical activity behaviour, objectively measured fitness and normative fitness percentiles. Data presented herein are a subset of a larger study, and highlight that matching by physical activity, does not necessarily match on other metrics like physical fitness, especially when age-related comparisons are being made. Our data showed that young and older adults matched by physical activity behaviours showed the expected higher CRF and muscular fitness in male and younger participants, but older adults had higher CRF percentiles. This suggests that matching by physical activity behaviour may select older adults with relatively higher CRF. Researchers must choose their matching method carefully to ensure the appropriate aspects of fitness have been matched between groups. For clarity, they should also report when certain aspects of fitness have not been accounted for and give an explanation as to why.
Confounding variables impact both dependent and independent variables in a study, potentially leading to false associations between these variables. Physical fitness is a common confounding variable in health-related outcomes and includes cardiorespiratory fitness (CRF), muscle strength, body composition and task performance. Physical activity, broadly defined as any movement requiring energy expenditure, is often used as a proxy for fitness. Both have significant effects on metabolic pathways, body systems (Fogelholm, 2010; Proper et al., 2011) and task performance, and therefore must be controlled for in research studies.
To illustrate physical fitness’ confounding role, consider a study recruiting young untrained females to assess two exercise training protocols. If training status is determined by asking participants if they engage in physical activity more than once a week, one group might be less fit due to this simplistic measure. Untrained individuals respond more to exercise training than trained individuals (Plowman et al., 1979), therefore, this could lead to a false conclusion that one exercise protocol is superior. To control for this, the baseline fitness of participants should be rigorously matched.
Matching aims to equate covariate distribution between groups and is commonly used in epidemiology (Stuart, 2010). Various forms of matching exist, such as one-to-one, paired, and propensity score matching (Stuart, 2010). The purpose is to ensure that participants in different groups are similar on average, concerning characteristics believed to be confounders in the association between the independent and dependent variables (Faresjö and Faresjö, 2010; Stuart, 2010). In the case of the two groups of females, physical fitness can be matched using questionnaires, or more accurate measures of fitness like VO2peak. VO2peak, the gold-standard measure of CRF, is measured via maximal graded exercise tests. It may also be estimated with a submaximal test, which is useful for populations that are unable to complete maximal exercise, but is more prone to error (Dugas et al., 2023).
Matching fitness is more challenging when comparing individuals of different ages and sexes, as both age and sex influence fitness. Muscular fitness and CRF decline with age (Goodpaster et al., 2006; Plowman et al., 1979), and males typically have greater CRF and muscular fitness than females (Bishop et al., 1987; Tarnopolsky, 1998). Furthermore, older adults have lower average physical activity levels than young adults (Hallal et al., 2012) and lower average fitness for a given level of physical activity (Plowman et al., 1979).
Individuals can be matched for physical activity and fitness across ages or between sexes by subjective or objective assessments. Objective matching is challenging due to age and sex effects on fitness, therefore subjective assessments are appealing. Untrained volunteers are commonly matched on subjective physical activity classifications such as self-reported weekly minutes of physical activity, or metabolic equivalent (MET) minutes. This method assumes that if physical activity is matched, so is physical fitness. For example, Hart et al., 2019, investigated age-related changes in the unfolded protein response to a single bout of resistance training, matching participants on the criterion of “not participating in routine exercise training for more than 2 days per week.” Similarly, trained volunteers are recruited based on self-reports such as participating in structured training for a minimum time (Petré et al., 2021). Self-reports are prone to error (Prince et al., 2008) and matching becomes more difficult when groups differ by age and sex.
Current matching methods have many limitations, especially when matching across age and sex. Matching individuals based on age and sex-stratified fitness percentiles (from objective fitness tests) may offer a more valid way of matching physical fitness between groups. This method involves volunteers completing objective fitness tests, and classification into age and sex-stratified percentiles. This method addresses age and sex’s confounding effects on physical fitness, removes self-report error and may offer an improved method of comparing individuals across age and sex.
The data presented herein, are a subset of a larger study that aimed to investigate age-related differences in how skeletal muscle responds to an acute bout of resistance exercise. In this larger study, both physical activity and physical fitness were matched between young and older adults. In doing so, it was observed that matching on one fitness variable (i.e., physical activity) does not necessarily match on the others (i.e., physical fitness). This perspective presents the data subset from our larger study as evidence for the importance of intentional matching when making age and sex-related comparisons in health research.
This study was approved by the University of British Columbia Research Ethics Board (H22-01203). Written informed consent was received from all participants. Healthy young (19–30 years) and older (65–85 years) male and female volunteers were recruited from the Vancouver, British Columbia, area. Participants were recreationally active, and self-reported participating in no more than 2 h per week of structured, moderate-vigorous lower-body resistance or aerobic training in the last 6 months.
Participants completed a maximal CRF ramp test on a cycle ergometer (Monark, LC6, Sweden) to measure VO2peak (mL/min/kg). They cycled to volitional fatigue and VO2peak was recorded as the highest 30-second average VO2. Maximal grip strength was tested by handgrip dynamometer (Handeful, Digital Hand Dynamometer) following methods in Hoffmann et al. (2019). Grip strength (kg) was recorded as the sum of the right and left hands. Primary outcomes were VO2peak (mL/min/kg), grip strength (kg) and VO2peak/grip strength age-sex stratified percentiles. Volunteers completed the International Physical Activity Questionnaire (IPAQ). Young adults completed the long form and older adults the elderly short form. MET-minutes per week were calculated from the IPAQ but did not determine eligibility.
To create a matching variable, age and sex-stratified fitness percentile lines of best fit were created using the findings from Kaminsky et al., 2022, for VO2peak and Hoffmann et al., 2019, for grip strength. Participants’ VO2peak (mL/min/kg) and grip strength (kg) scores were used to extrapolate their age and sex-stratified fitness percentile using these lines of best fit. If participants’ extrapolated percentile fell below 1, they were assigned the 1st percentile. If their percentile fell above 100, they were assigned the 99th percentile.
All statistical analyses were conducted using GraphPad Prism version 10.2.3 for Mac OS X (GraphPad Software, Boston, MA). Two-way ANOVA (age × sex), followed by Fisher’s LSD test was performed on all metrics. Data are presented as mean ± standard deviation.
13 young females (22.5 ± 2.9 years), 16 young males (21.5 ± 2.3 years), 10 older females (74.4 ± 3.7 years) and 9 older males (71.2 ± 2.4 years) were recruited. Males weighed more (p = <0.0001) and were taller than females (p = <0.0001). Older females (22.3 ± 2.1 kg/m2) had significantly lower BMI than older males (24.67 ± 1.94 kg/m2, p = 0.025). No other significant differences in BMI were demonstrated. No significant differences in IPAQ scores were demonstrated between groups.
Groups matched for self-reported physical activity, age, and sex, showed expected results based on the existing literature (Bishop et al., 1987; Diaz-Canestro et al., 2022). Young adults had greater VO2peak (36.86 ± 6.38 mL/min/kg, p = <0.001) (Figure 1A) and grip strength (81.51 ± 24.05 kg, p = <0.001) (Figure 1B) than older adults (25.62 ± 4.87 mL/min/kg, 65.11 ± 22.28 kg). Males had greater VO2peak (36.32 ± 6.79 mL/min/kg, p = <0.001) (Figure 1A) and grip strength (93.81 ± 15.53 kg, p = <0.001) (Figure 1B) than females (28.15 ± 7.11 mL/min/kg, 54.37 ± 12.86 kg).
Figure 1. (A) Measured VO2peak (mL/min/kg) between ages and sexes. (B) Measured grip strength (kg) between ages and sexes. (C) Comparison of age and sex-stratified cardiorespiratory fitness (VO2peak) percentiles. (D) Comparison of age and sex-stratified muscular fitness (grip strength) percentiles. Different letters above each group’s data denote statistically different post-hoc differences between groups (p < 0.05). Groups with the same letter are not statistically different from one another.
Matching relative fitness score, age, and sex showed important differences compared to matching based on self-reported data (≤ 2 hours structured lower-body exercise per week). Older adults had higher VO2peak percentiles (96.32 ± 6.7) than young adults (44.66 ± 15.98, p = <0.0001) and young females (52.77 ± 16.79) had higher VO2peak percentiles than young males (38.06 ± 11.7, p = 0.003) (Figure 1C). No differences in VO2peak percentiles were observed between older males (95.78 ± 7.11) and females (96.8 ± 6.27, p = 0.86). No differences in grip strength percentiles were observed between age groups (p = 0.6) or between sexes (p = 0.36) (Figure 1D).
As expected, recruiting individuals who engage in less than 2 hours of lower-body exercise weekly showed higher muscular (grip strength, kg) and CRF (VO2peak, mL/kg/min) in younger relative to older adults and males relative to females. When using normative data to compare fitness percentiles, however, older adults showed higher CRF but similar muscular strength, highlighting the limitations of matching CRF across age groups by self-report. Matching participants on stratified fitness percentiles can effectively control for performance and behaviour differences influenced by age. Understanding the strengths and weaknesses of each matching method (Table 1) will facilitate informed decision-making in research design.
Table 1. Advantages and disadvantages of each matching method. The focus is on comparison groups for which each method is valid. Cardiorespiratory fitness (CRF).
Objectively measuring fitness is valid for same age and sex comparisons. This method is impractical, however, when groups differ by age and/or sex. For example, a 30-year-old male with a VO2peak of 22.6 mL/min/kg falls within the 20th percentile for his age group, whereas a 70-year-old male with the same VO2peak falls between the 80th percentile, and a 70-year-old female falls above the 99th percentile (Kaminsky et al., 2022). In this case, a very unfit young male would be recruited, and a very fit older male/female would be recruited, leading to possible confounding effects of physical fitness. Similar issues arise when matching muscular fitness scores between individuals of different ages and/or sexes (Hoffmann et al., 2019). It is also important to maintain consistency in the method used to measure objective fitness scores with the methodology of the reference data. VO2peak must be measured using a maximal test, however, the results from submaximal tests are commonly extrapolated and reported as VO2peak (Hoffmann et al., 2019). Due to systemic bias in CRF estimates from submaximal protocols (Dugas et al., 2023), it is not appropriate to match objectively measured and estimated CRF data within a single study. Further, the exercise mode of the test used will influence CRF values. For example, an individual will typically achieve a higher VO2peak score on a maximal treadmill test than a maximal cycle ergometer test (McArdle et al., 1973). Thus, where possible, the CRF testing methodology should match the methods of the reference data as closely as possible.
Self-reported physical activity is advantageous for its simplicity–no physiological tests are required, facilitating recruitment. This method assumes that matching physical activity is appropriate for age and sex comparisons as individuals do not need to have the same measured fitness. Our findings challenge these assumptions. Young and older males and females who report similar weekly physical activity minutes do not fall within the same CRF percentiles (determined by VO2peak test) (Figure 1C). Young adults have significantly lower CRF percentiles than older adults and young females have significantly higher CRF percentiles than young males (Figure 1C). Self-report data is prone to error (Prince et al., 2008), therefore, older adults may underestimate and/or young adults (and to a greater extent, young males) may overestimate their physical activity levels. These findings suggest that matching physical activity minutes effectively matches CRF between sexes in older adults, but not between sexes in young adults or different age groups. These findings are specific to CRF, as individuals of different ages and sexes matched on physical activity minutes, show no differences in grip strength percentiles (Figure 1D), suggesting this matching method is valid when grip strength is a potential confounder.
A final method to be discussed is matching FFM-normalized fitness between groups. Variations in body fat percentage between males and females influence physical fitness (Tarnopolsky, 1998), thus matching FFM-normalized fitness aims to eliminate this confounding variable. This method is effective when comparing young male and female muscle (Tripp et al., 2024), however, Fleg et al., 2005, demonstrated that VO2peak/FFM declines more rapidly in older adults (like VO2peak/kg bodyweight). Thus, using this method to match between age groups might cause the same findings as matching performance-based outcomes (fitness percentiles differing significantly between groups, where older adults score higher than young adults). Matching FFM-normalized fitness assumes that body composition is the only confounder of physical fitness. However, other systemic differences contribute to overall fitness as well. Therefore, this method effectively matches physical fitness when outcomes are tissue-specific but is not necessarily valid for other outcome measures. The concern of matching whole individuals can be addressed by matching fitness percentiles. Matching fitness percentiles addresses whole-body fitness, rather than body composition differences only.
Each of the matching methods discussed has advantages and disadvantages, and their suitability depends on the comparison group, research question and research outcomes (Table 1). The challenges of matching physical fitness across multiple variables are clear and no universal strategy is optimal in all situations. Instead, it emphasizes that different aspects of physical fitness are controlled depending on the method chosen, and not all methods are valid for all population comparisons (Table 1).
Matching fitness percentiles should be considered when making age and sex comparisons where physical fitness is a confounding variable. This method, however, has limitations, including the need for normative datasets that are not always available and may be context-specific. As mentioned, different methods of collecting the same objective fitness scores have different measurement errors and biases. As objective fitness scores are needed to create fitness percentiles, it is important to match, as closely as possible, the methods used by the normative dataset to the methods used in the present study. These challenges are even greater when assessing muscular fitness as, beyond standardized measurements of grip strength, there is little consistency in measurement protocols for strength across different demographics and research areas. The use of normative data can also introduce error if its population is biased or unrepresentative. For example, the present study used normative data available from the United States, rather than Canada because Canadian normative data used a submaximal test to measure CRF (Hoffman et al., 2019), and the present study used a maximal exercise test. Given the similarities between Canadian and United States demographics, and due to the error of submaximal tests, it was decided that it was more important to match the CRF measurement method than to match the population. Researchers should be aware of the extent to which geographical characteristics affect their outcomes when choosing normative datasets. The small sample size of the present study limits the precession of the findings but still illustrates the trade-offs associated with choosing each matching method. As the current study was part of a larger study investigating age-related differences in skeletal muscle responses to exercise in Vancouver, Canada, applying these matching methods to a larger geographically diverse sample could offer better insights into age and sex-based matching.
Various methods for matching physical fitness between groups have been described, each addressing different aspects of fitness. Performance capacity can be matched with objective fitness tests (i.e., measured VO2peak), behavioural aspects with self-reports (i.e., number of physical activity minutes per week) and muscle/tissue composition differences with FFM-normalized fitness tests. Matching fitness percentiles offers the advantage of matching on average, individuals’ whole-body fitness and should be considered when age and sex comparisons are made. Each method has merits and drawbacks, researchers must understand both when choosing their matching criteria. Researchers should also explicitly acknowledge which aspects of fitness have/have not been addressed by their chosen method and justify it in their reports. Careful selection of matching criteria and transparency in reporting will enhance the validity and reliability of research outcomes.
The raw data supporting the conclusions of this article will be made available by the authors, without undue reservation.
The studies involving humans were approved by The University of British Columbia Research Ethics Board. The studies were conducted in accordance with the local legislation and institutional requirements. The participants provided their written informed consent to participate in this study.
AS: Conceptualization, Formal Analysis, Investigation, Methodology, Project administration, Writing–original draft, Writing–review and editing, Data curation. DF: Conceptualization, Writing–review and editing. MF: Investigation, Writing–review and editing. CM: Conceptualization, Funding acquisition, Investigation, Methodology, Resources, Supervision, Writing–review and editing.
The author(s) declare that financial support was received for the research, authorship, and/or publication of this article. This study was funded by the Natural Sciences and Engineering Research Council of Canada (RGPIN-2021-04259).
The authors declare that the research was conducted in the absence of any commercial or financial relationships that could be construed as a potential conflict of interest.
The authors declare that no Generative AI was used in the creation of this manuscript.
All claims expressed in this article are solely those of the authors and do not necessarily represent those of their affiliated organizations, or those of the publisher, the editors and the reviewers. Any product that may be evaluated in this article, or claim that may be made by its manufacturer, is not guaranteed or endorsed by the publisher.
Bishop P., Cureton K., Collins M. (1987). Sex difference in muscular strength in equally-trained men and women. Ergonomics 30 (4), 675–687. doi:10.1080/00140138708969760
Diaz-Canestro C., Pentz B., Sehgal A., Montero D. (2022). Sex differences in cardiorespiratory fitness are explained by blood volume and oxygen carrying capacity. Cardiovasc. Res. 118 (1), 334–343. doi:10.1093/cvr/cvab028
Dugas M. O., Paradis-Deschênes P., Simard L., Chevrette T., Blackburn P., Lavallière M. (2023). Comparison of VO2max estimations for maximal and submaximal exercise tests in apparently healthy adults. Sports 11 (12), 235. doi:10.3390/sports11120235
Faresjö T., Faresjö Å. (2010). To match or not to match in epidemiological studies—same outcome but less power. Int. J. Environ. Res. public health 7 (1), 325–332. doi:10.3390/ijerph7010325
Fleg J. L., Morrell C. H., Bos A. G., Brant L. J., Talbot L. A., Wright J. G., et al. (2005). Accelerated longitudinal decline of aerobic capacity in healthy older adults. Circulation 112 (5), 674–682. doi:10.1161/CIRCULATIONAHA.105.545459
Fogelholm M. (2010). Physical activity, fitness and fatness: relations to mortality, morbidity and disease risk factors. A systematic review. Obes. Rev. 11 (3), 202–221. doi:10.1111/j.1467-789X.2009.00653.x
Goodpaster B. H., Park S. W., Harris T. B., Kritchevsky S. B., Nevitt M., Schwartz A. V., et al. (2006). The loss of skeletal muscle strength, mass, and quality in older adults: the health, aging and body composition study. Journals Gerontology Ser. A Biol. Sci. Med. Sci. 61 (10), 1059–1064. doi:10.1093/gerona/61.10.1059
Hallal P. C., Andersen L. B., Bull F. C., Guthold R., Haskell W., Ekelund U., et al. (2012). Global physical activity levels: surveillance progress, pitfalls, and prospects. lancet 380 (9838), 247–257. doi:10.1016/S0140-6736(12)60646-1
Hart C. R., Ryan Z. C., Pfaffenbach K. T., Dasari S., Parvizi M., Lalia A. Z., et al. (2019). Attenuated activation of the unfolded protein response following exercise in skeletal muscle of older adults. Aging (Albany NY) 11 (18), 7587–7604. doi:10.18632/aging.102273
Hoffmann M. D., Colley R. C., Doyon C. Y., Wong S. L., Tomkinson G. R., Lang J. J. (2019). Normative-referenced percentile values for physical fitness among Canadians. Health Rep. 30 (10), 14–22. doi:10.25318/82-003-x201901000002-eng
Kaminsky L. A., Arena R., Myers J., Peterman J. E., Bonikowske A. R., Harber M. P., et al. (2022). Updated reference standards for cardiorespiratory fitness measured with cardiopulmonary exercise testing: data from the Fitness Registry and the Importance of Exercise National Database (FRIEND). Mayo Clin. Proc. 97 (2), 285–293. doi:10.1016/j.mayocp.2021.08.020
McArdle W. D., Katch F. I., Pechar G. S. (1973). Comparison of continuous and discontinuous treadmill and bicycle tests for max Vo2. Med. Sci. Sports 5 (3), 156–160.
Petré H., Hemmingsson E., Rosdahl H., Psilander N. (2021). Development of maximal dynamic strength during concurrent resistance and endurance training in untrained, moderately trained, and trained individuals: a systematic review and meta-analysis. Sports Med. 51, 991–1010. doi:10.1007/s40279-021-01426-9
Plowman S. A., Drinkwater B. L., Horvath S. M. (1979). Age and aerobic power in women: a longitudinal study. J. gerontology 34 (4), 512–520. doi:10.1093/geronj/34.4.512
Prince S. A., Adamo K. B., Hamel M. E., Hardt J., Gorber S. C., Tremblay M. (2008). A comparison of direct versus self-report measures for assessing physical activity in adults: a systematic review. Int. J. Behav. Nutr. Phys. activity 5, 56–24. doi:10.1186/1479-5868-5-56
Proper K. I., Singh A. S., Van Mechelen W., Chinapaw M. J. (2011). Sedentary behaviors and health outcomes among adults: a systematic review of prospective studies. Am. J. Prev. Med. 40 (2), 174–182. doi:10.1016/j.amepre.2010.10.015
Stuart E. A. (2010). Matching methods for causal inference: a review and a look forward. Stat. Sci. a Rev. J. Inst. Math. Statistics 25 (1), 1–21. doi:10.1214/09-STS313
M. Tarnopolsky (1998). Gender differences in metabolism: practical and nutritional implications (Boca Raton, FL: CRC Press), 14.
Tripp T. R., McDougall R. M., Frankish B. P., Wiley J. P., Lun V., MacInnis M. J. (2024). Contraction intensity affects NIRS-derived skeletal muscle oxidative capacity but not its relationships to mitochondrial protein content or aerobic fitness. J. Appl. Physiology 136 (2), 298–312. doi:10.1152/japplphysiol.00342.2023
Keywords: aging, exercise, matching, physical activity, self-report, sex comparisons
Citation: Schweitzer AM, Fuller D, Fliss MD and Mitchell CJ (2025) Perspective on strategies for matching across age and sex in physiology research: “recreationally active” is not good enough. Front. Physiol. 15:1517355. doi: 10.3389/fphys.2024.1517355
Received: 25 October 2024; Accepted: 10 December 2024;
Published: 07 January 2025.
Edited by:
Giancarlo Condello, University of Parma, ItalyReviewed by:
Mary Imboden, Providence St. Joseph Health, United StatesCopyright © 2025 Schweitzer, Fuller, Fliss and Mitchell. This is an open-access article distributed under the terms of the Creative Commons Attribution License (CC BY). The use, distribution or reproduction in other forums is permitted, provided the original author(s) and the copyright owner(s) are credited and that the original publication in this journal is cited, in accordance with accepted academic practice. No use, distribution or reproduction is permitted which does not comply with these terms.
*Correspondence: Cameron J. Mitchell, Y2FtZXJvbi5taXRjaGVsbEB1YmMuY2E=
Disclaimer: All claims expressed in this article are solely those of the authors and do not necessarily represent those of their affiliated organizations, or those of the publisher, the editors and the reviewers. Any product that may be evaluated in this article or claim that may be made by its manufacturer is not guaranteed or endorsed by the publisher.
Research integrity at Frontiers
Learn more about the work of our research integrity team to safeguard the quality of each article we publish.