- Department of Research & Development Management, Shanghai Aohua Photoelectricity Endoscope Co., Ltd., Shanghai, China
Cerebral aneurysms are abnormal dilations of blood vessels in the brain that have the potential to rupture, leading to subarachnoid hemorrhage and other serious complications. Early detection and prediction of aneurysm rupture are crucial for effective management and prevention of rupture-related morbidities and mortalities. This review aims to summarize the current knowledge on risk factors and predictive indicators of rupture in cerebral aneurysms. Morphological characteristics such as aneurysm size, shape, and location, as well as hemodynamic factors including blood flow patterns and wall shear stress, have been identified as important factors influencing aneurysm stability and rupture risk. In addition to these traditional factors, emerging evidence suggests that biological and genetic factors, such as inflammation, extracellular matrix remodeling, and genetic polymorphisms, may also play significant roles in aneurysm rupture. Furthermore, advancements in computational fluid dynamics and machine learning algorithms have enabled the development of novel predictive models for rupture risk assessment. However, challenges remain in accurately predicting aneurysm rupture, and further research is needed to validate these predictors and integrate them into clinical practice. By elucidating and identifying the various risk factors and predictive indicators associated with aneurysm rupture, we can enhance personalized risk assessment and optimize treatment strategies for patients with cerebral aneurysms.
Introduction
Cerebral aneurysms, pathological dilatations of the cerebral artery walls, represent a substantial health risk due to their potential for rupture, which often show localized expansions of the arterial segment, typically occurring at the bifurcations of major arteries at the base of the brain, known as the Circle of Willis (Li et al., 2023; Thompson et al., 2015; Etminan and Rinkel, 2016; Kim D. W. et al., 2019; Brisman et al., 2006; Kayembe et al., 1984; Chalouhi et al., 2013; Diagbouga et al., 2018). Currently, cerebral aneurysms have been significantly affected patients’ physical and mental conditions, which shows adverse impacts on public health concern due to their potential rupture, even leading to high mortality and disability rates (Hackenberg et al., 2020; Yang et al., 2021; Findlay and Deagle, 1998; Ajiboye et al., 2015; Wiebers et al., 1992). When rupture of cerebral aneurysms occurs, it leads to subarachnoid hemorrhage, a catastrophic event often resulting in serious conditions such as hydrocephalus, cerebral vasospasm, rebleeding, or cerebral infarction. Each of these complications brings its own devastating impact, from neurological deficits and cognitive impairments to the risk of permanent disability or death, thereby underscoring the high stakes involved in the management of cerebral aneurysms (Macdonald and Schweizer, 2017; Hop et al., 1997; Ingall et al., 2000; Tawk et al., 2021; Petridis et al., 2017; Steiner et al., 2013; Grasso et al., 2017; Biller et al., 1988).
The likelihood of cerebral aneurysm rupture is influenced by a complex interplay of nonmodifiable and modifiable factors. Nonmodifiable factors include age, gender, and genetic predisposition. For example, there is a higher prevalence of aneurysms in older individuals, females, and those with a familial history of aneurysms. Conversely, modifiable factors encompass lifestyle and health conditions such as hypertension, smoking, and alcohol consumption. Hypertension, for instance, can exacerbate the hemodynamic stress exerted on the arterial walls, while smoking and excessive alcohol consumption contribute to arterial damage and inflammation, thereby predisposing the individual to aneurysm formation and growth (Chalouhi et al., 2013; Kleinloog et al., 2018; Jin et al., 2019; Munarriz et al., 2016; Texakalidis et al., 2019; Lindner et al., 2010).
The risk of aneurysm rupture varies among individuals, and early identification of those at high risk is crucial for timely intervention and prevention of catastrophic outcomes. Cerebral aneurysms can affect individuals of any age, gender, and ethnicity, although certain demographic and clinical factors are known to increase the risk of aneurysm formation and rupture. These risk factors include advanced age, female gender, family history of aneurysms, and certain medical conditions such as hypertension and arteriosclerosis (Rinkel et al., 1998; Backes et al., 2016; Korja et al., 2014; Cho et al., 2020). Additionally, the location and morphology of the aneurysm, as well as hemodynamics acting on the vessel wall, play critical roles in determining the risk of rupture (Lall et al., 2009; Jeong et al., 2009; Signorelli et al., 2018; Waqas et al., 2020). The vessel wall at these dilated segments, often referred to as the aneurysmal dome, is characterized by the loss of elastic and muscular components, rendering it prone to rupture under hemodynamic stress (Chalouhi et al., 2013; Texakalidis et al., 2019; Signorelli et al., 2018; Sadasivan et al., 2013; Duan et al., 2016; Wang et al., 2023; Siasos et al., 2015).
In recent years, an evolving body of research has begun to pivot towards a more comprehensive understanding of the role of hemodynamic forces in the initiation, growth, and rupture of cerebral aneurysms. Hemodynamics, essentially the study of blood flow and the forces it exerts on vessel walls, is now being increasingly recognized as a critical player in the physiopathology of intracranial aneurysms (Signorelli et al., 2018; Staarmann et al., 2019). A large array of studies has attempted to elucidate the specific hemodynamic factors that contribute to aneurysm pathophysiology. Wall shear stress (WSS), for instance, is a tangential force that blood flow applies to the vessel wall, and abnormal WSS has been implicated in the development, progression, and rupture of aneurysms. A number of these studies suggest that high WSS can induce endothelial dysfunction and inflammatory responses, which contribute to aneurysm initiation, while low WSS can cause direct mechanical damage, contributing to aneurysm growth and rupture (Staarmann et al., 2019; Zhang et al., 2016; Penn et al., 2011; Meng et al., 2014). In addition to above indexes, other hemodynamic parameters such as pressure, flow velocity, and flow patterns have also been investigated for their role in aneurysm behavior (Jirjees et al., 2020; Xiang et al., 2014). For example, studies using computational fluid dynamics have modeled aneurysmal blood flow to study the complex interactions between these hemodynamic parameters and aneurysm morphology (Kleinstreuer et al., 2007; Nazri et al., 2020).
Meanwhile, there is an increasing focus on identifying predictive markers that can improve the precision of risk assessment for cerebral aneurysm rupture. These markers encompass biomolecules that mirror the biological processes that contribute to aneurysm formation and growth, such as markers of inflammation, indicators of extracellular matrix remodeling, and genetic variants (Hussain et al., 2015; Husseini and Laskowitz, 2010; Jordan and Nyquist, 2010). Advances in imaging technology, computational fluid dynamics, and machine learning algorithms have facilitated the development of innovative predictive models for assessing the risk of aneurysm rupture (Diab et al., 2023; Hosny et al., 2018; Chen et al., 2022).
Despite the progress made in understanding the risk factors and predictive indicators of rupture in cerebral aneurysms, challenges remain in accurately predicting which aneurysms will rupture. Current risk assessment primarily relies on aneurysm size, with larger aneurysms often believed to have a higher propensity for rupture. Other criteria include aneurysm location, morphology, and patient clinical history, which are all believed to have varying degrees of influence on rupture risk.
The purpose of this review is to delve into the current knowledge regarding the risk factors and predictive indicators that are associated with the rupture of cerebral aneurysms. The review underscores the significance of a holistic approach to risk assessment, taking into account a wide array of factors. Additionally, it addresses the shortcomings of the current risk assessment model, which primarily relies on aneurysm size. The review emphasizes the necessity of adopting a more multifaceted strategy that encompasses a comprehensive range of mechanical, biological, and hemodynamic factors to enhance the accuracy of predicting aneurysm rupture. By gaining a deeper understanding of the factors that contribute to aneurysm rupture, we can improve personalized risk assessment and optimize treatment strategies for patients with cerebral aneurysms, ultimately reducing the incidence of rupture-related complications and improving patient outcomes.
Clinical perspective
The clinical perspective of rupture on cerebral aneurysms encompasses a comprehensive understanding of the risk factors, management strategies, and outcomes associated with this condition (Zhu et al., 2020). Herein, an overview of the clinical aspects of cerebral aneurysms rupture is shown, which highlights the importance of timely intervention and the potential for improved patient outcomes through early diagnosis and appropriate treatment, as shown in Figure 1.
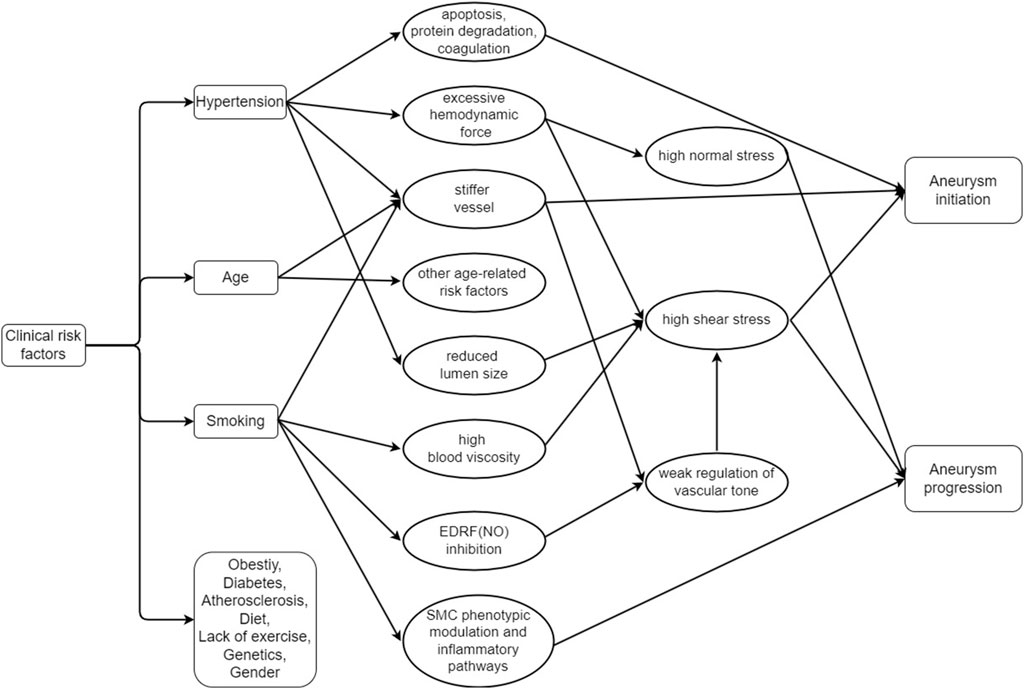
Figure 1. Relationship between the clinical risk factors and the initiation and progression of the cerebral aneurysm (EDRF: Endothelium-derived relaxation factor, NO: Nitric oxide, SMC: Smooth muscle cells).
To our knowledge, several risk factors including the age, gender, family history of aneurysms, hypertension, and arteriosclerosis are the distinguished information as the primarily routine reference from the clinicians (Kleinloog et al., 2018). Age is a nonmodifiable risk factor, with older individuals presenting a higher risk of aneurysm rupture due to the general degradation of vascular health over time (Brinjikji et al., 2016; Wermer et al., 2007). Sex is another factor, with females generally demonstrating a higher prevalence and rupture risk of intracranial aneurysms, potentially due to hormonal influences (Brinjikji et al., 2016; Turan et al., 2016). A patient’s clinical history, particularly a history of subarachnoid hemorrhage or familial history of aneurysms, significantly increases the rupture risk (Lee et al., 2021; Brown and Broderick, 2014; Rinkel, 2008).
The clinical presentation of cerebral aneurysm rupture could vary widely considering the physical diversity, but it often occurs suddenly with severe headache, neck stiffness, nauses, and vomiting (Etminan and Rinkel, 2016; Sadasivan et al., 2013; Wardlaw and White, 2000). Meanwhile, aneurysm with other symptoms may lead to altered consciousness, seizures, and focal neurological deficits. Ruptured aneurysms can occur subarachnoid hemorrhage, which can cause significant disability or death if not treated promptly.
The management of cerebral aneurysm involves a multidisciplinary approach, including the imaging, medical, and surgical interventions (Etminan and Rinkel, 2016; Brown and Broderick, 2014; Burkhardt et al., 2017). Imaging techniques play an important role in the diagnosis and monitoring of cerebral aneurysm. Medical management may include lifestyle modifications, blood pressure control, and the use of antiplatelet or anticoagulant medications. Surgical interventions including endovascular coiling or surgical clipping, is often required to prevent rupture and restore blood flow.
The outcomes of cerebral aneurysms rupture depend on various factors, including the time of diagnosis, the size and location of the aneurysm, the presence of complications, and the effectiveness of treatment (Kleinloog et al., 2018; Brinjikji et al., 2016; Ma et al., 2019). Early diagnosis and timely intervention are essential for improving outcomes and reducing the risk of disability or death. However, even with appropriate treatment, there is still risks of recurrence and the potential for long-term complications, including rebleeding and aneurysm-related stroke.
The clinical perspective on cerebral aneurysm rupture emphasizes the importance of early detection, timely intervention, and multidisciplinary management. By understanding the risk factors, clinical presentation, and management strategies, healthcare professionals can optimize patient care and improve outcomes of treatment for patients. However, the treatment of cerebral aneurysms is complex, and further research should concentrate on developing more effective prevention and treatment strategies.
Morphological risk factors
The morphological parameters of cerebral aneurysms mainly include the size, shape, location, and the ratio between various geometrical parameters, which indicates the crucial role in determining the risk of rupture (Kleinloog et al., 2018; Zhu et al., 2020; Baharoglu et al., 2010; Leemans et al., 2019; Liu et al., 2019; Tang et al., 2022). This review aims to provide a comprehensive overview of the relationship between morphological parameters and the risk of rupture in cerebral aneurysms, as shown in Figure 2.
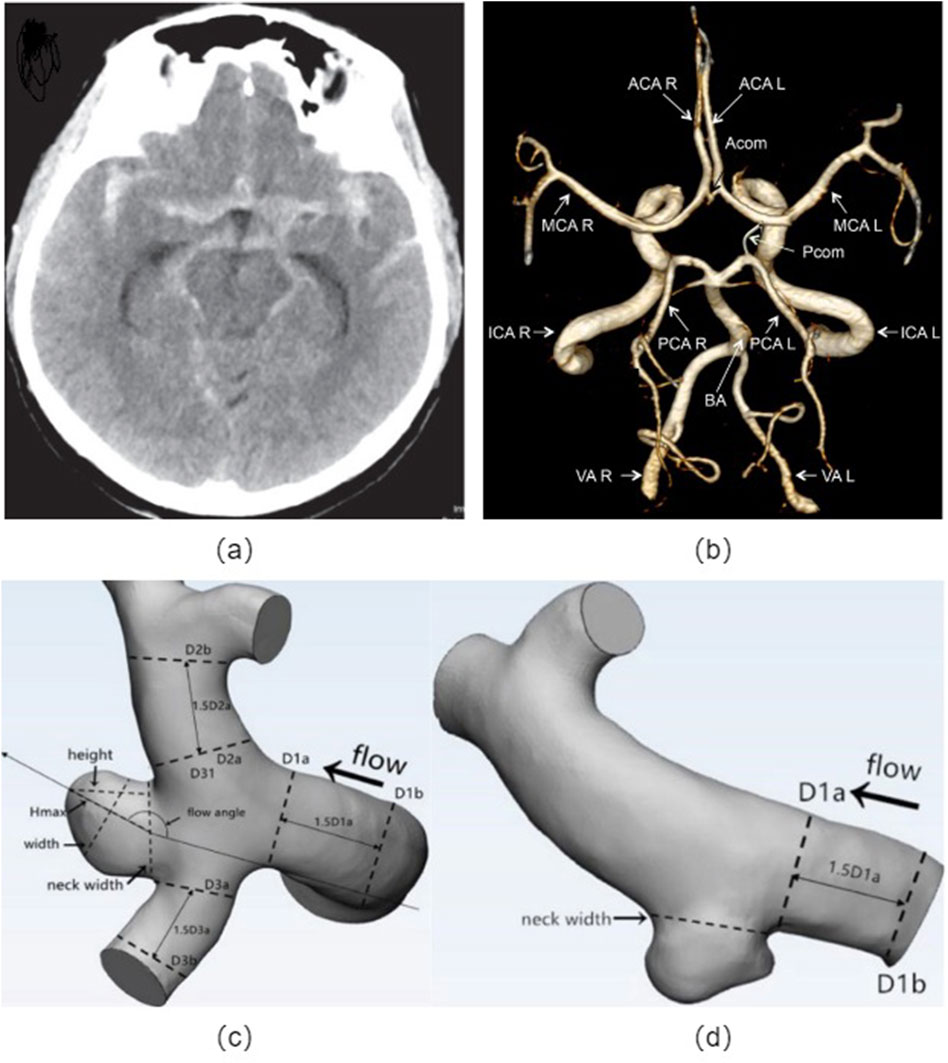
Figure 2. Cerebral artery morphology: (A) Non-contrast head CT showing subarachnoid hemorrhage from the left posterior communicating artery aneurysm; (B) Angiographic image of a human circle of Willis, indicated are: vertebral arteries (VA), right (R) and left (L), basilar artery (BA), internal carotid arteries (ICA R, ICA L), posterior cerebral arteries (PCA R, PCA L), anterior cerebral arteries (ACA R, ACA L), middle cerebral arteries (MCA R, MCA L), posterior communicating artery (Pcom) and anterior communicating artery (Acom); Definitions of morphological parameters in the bifurcation aneurysm (C) and sidewall aneurysm (D). Hmax: the maximum distance of the dome from the centroid of the neck plane, Height: the maximum perpendicular distance from the neck plane of the dome, Width: the maximum diameter perpendicular to Hmax, Neck width: the largest cross-sectional diameter of the aneurysm neck plane, aspect ratio (AR) equals to height/neck width, size ratio (SR) equals to Hmax/DV, and DV denote the average of the diameter of the cross-sectional a vessel (Da) just proximal to the neck of the aneurysm and the diameter of the cross-section (Db) at 1.5 times Da from the neck of the aneurysm.
Size: The size of the aneurysm is one of the most extensively studied morphological characteristics associated with rupture risk (Yi et al., 2016a; Merritt et al., 2021; Malhotra et al., 2017). Larger aneurysms are generally considered to have a higher risk of rupture, although the size threshold for high risk remains controversial. Some studies suggest that aneurysms larger than a certain size, such as 7 mm or 10 mm, have an increased risk of rupture, while others argue that smaller aneurysms can also be at risk (Kim H. C. et al., 2019; Xiong et al., 2022). Additionally, the rate of aneurysm expansion over time may serve as a significant determinant in assessing the likelihood of rupture.
Shape: The shape of an aneurysm is another important morphological characteristic that influences the risk of rupture (Kleinloog et al., 2018; Jirjees et al., 2020; Fung et al., 2019; Forget et al., 2001). Aneurysms with irregular shapes, such as multilocular or fusiform configurations, have been associated with an increased risk of rupture compared to those with a more spherical shape. The irregular shape may indicate areas of weakness in the aneurysm wall or increased wall stress, which can predispose the aneurysm to rupture.
Location: The location of an aneurysm within the cerebral vasculature also plays a significant role in determining the risk of rupture (Kleinloog et al., 2018; Can and Du, 2016; Park et al., 2018). Aneurysms located at the terminal ends of arteries or at bifurcations are more prone to rupture due to the increased hemodynamic forces and stress concentrations in these areas. Additionally, certain locations, such as the posterior communicating artery or the middle cerebral artery, have been identified as high-risk regions for rupture.
Intraluminal Thrombus: The presence of intraluminal thrombus within an aneurysm has been identified as a potential risk factor for rupture (Kleinloog et al., 2018; Wermer et al., 2007; Wang et al., 2019). Thrombus formation can alter the hemodynamics within the aneurysm and increase wall stress, potentially leading to rupture. The size and distribution of thrombus within the aneurysm may also influence the risk of rupture.
Aspect Ratio (AR): AR is the ratio of the perpendicular height of the aneurysm to the neck width of the aneurysm. Aneurysm with high ARs will have deep domes and small necks. An elevated AR is conducive to the recirculation of blood and the creation of low-velocity flow states, which have been detected in aneurysms that have ruptured. Such low-velocity conditions correlate with reduced WSS and an escalation in inflammatory responses within the endothelium. The expansion and rupture of aneurysms may take place in regions where wall shear stress is diminished, a situation that is probable in aneurysms characterized by a high AR and pronounced dome depths. AR of ≥1.5 is significantly increase the risk of rupture, which is regarded as strongest predictor of rupture status for aneurysms (Boussel et al., 2008; Carter et al., 2006; Sanchez et al., 2023; Dhar et al., 2008; Reneman et al., 2006).
Size Ratio (SR): Another key morphological parameter is the SR, defined as the maximum aneurysm height relative to the average parent vessel diameter (Kleinloog et al., 2018; Waqas et al., 2020; Jirjees et al., 2020; Fung et al., 2019). A high SR implies a larger aneurysm relative to the parent vessel and has been associated with increased wall tension and a greater likelihood of rupture. Aneurysms with high SRs have deep domes compared with the diameter of their parent vessels. SRs are significantly different between ruptured and unruptured aneurysms. It is previously reported that Studies have identified a SR ≥ 2.3 as a potential cutoff value for predicting aneurysm rupture (Sanchez et al., 2023). This threshold could potentially be employed to assess the rupture risk rather than only relying on the maximum diameter of the aneurysm, which could precisely predict the rupture of small aneurysms with the diameter <5 mm. However, it is important to note that while these values serve as useful indicators, the precise thresholds can vary based on the population studied and the specific methodology used in the study.
In the previous studies, the morphology of cerebral aneurysms, including size, shape, location, intraluminal thrombus, and size ratio, has played a critical role in determining the risk of rupture. However, the relationship between these morphological characteristics and rupture risk is complex and can vary among individuals. It is crucial to understand that while these measures provide valuable insights, they represent a snapshot of the aneurysm’s current state and do not fully encapsulate the dynamic nature of aneurysm growth and progression. Moreover, these morphological parameters are derived from three-dimensional structures projected onto two-dimensional images, which may not always accurately reflect the true aneurysm morphology. Therefore, while morphological parameters can help guide clinical decisions, they should be interpreted within the context of other patient-specific factors such as age, gender, and genetic predisposition.
Despite the relative simplicity and general applicability of these risk factors, they tend to overlook the intricacies of aneurysm behavior. While they provide a reasonable approximation of rupture risk, they fail to capture the complex, multifaceted nature of cerebral aneurysms, which may lead to inaccuracies in risk prediction. Currently, these traditional risk assessment methods have several limitations. Firstly, they often oversimplify the complexity of aneurysm behavior by focusing on static anatomical features, ignoring the dynamic nature of blood flow and its interaction with the vessel wall. Secondly, their predictive performance in clinical practice has shown to be inconsistent, with many small aneurysms rupturing while some large aneurysms remain stable. Finally, they do not account for individual patient variability in hemodynamics, vessel wall biology, and biomechanical properties, all of which could influence aneurysm growth and rupture.
This highlights the importance of pursuing a more comprehensive approach that factors in the intricate interplay of mechanical, biological, and hemodynamic elements in aneurysm formation, growth, and rupture. Advances in imaging techniques and computational models have improved our ability to assess morphological features and their implications for aneurysm rupture. Further research is needed to validate these findings and develop robust tools for accurate risk assessment and prediction of cerebral aneurysm rupture.
Hemodynamical risk factors
Hemodynamics is a branch of physics that concerns the principles governing blood flow in the cardiovascular system, which is fundamental to understanding the pathophysiology of numerous cardiovascular conditions, including cerebral aneurysms. Hemodynamic forces, primarily including pressure, flow velocity, and WSS, are key determinants of vascular health and function, influencing everything from vascular tone and remodeling to endothelial function and inflammatory responses (Xiang et al., 2014; Baharoglu et al., 2010; Leemans et al., 2019; Cho, 2023). Hemodynamical computation procedure for the patient-specific model is as shown in Figure 3.
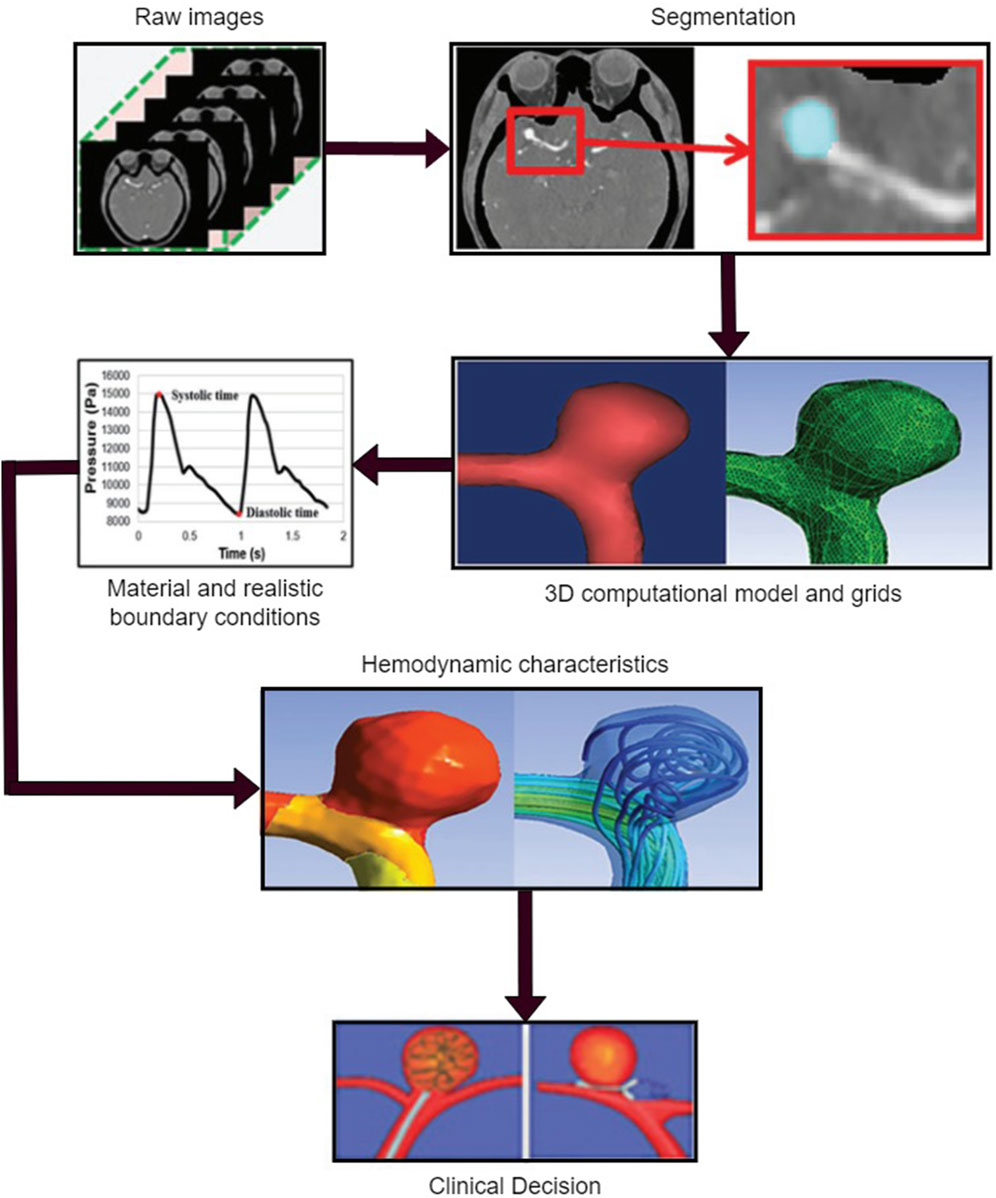
Figure 3. Hemodynamic analysis of a patient-specific aneurysmal model, including obtaining the raw data, medical segmentation concentrating on the aneurysm zone, three-dimensional (3D) reconstructed model for the computation and mesh the 3D model, define the material properties and load the realistic boundary conditions on the inlet and outlet of the computational model, obtain the flow characteristics related to the quantified hemodynamic parameters, and clinical decision for physicians.
WSS is a particular hemodynamic parameter of great importance. It is a tangential force exerted by the flowing blood on the vascular wall (Staarmann et al., 2019; Zhang et al., 2016; Meng et al., 2014; Zhou et al., 2017). Normal WSS values are crucial for maintaining vascular integrity and endothelial health. However, abnormal WSS, either too high or too low, can result in endothelial damage and dysfunction, which can pave the way for aneurysm initiation and progression. High WSS, for instance, can directly damage the vascular endothelium, leading to endothelial denudation and promoting a pro-inflammatory state that can stimulate aneurysm growth. On the other hand, areas of low WSS are often characterized by disturbed flow, which can induce endothelial cell apoptosis, inflammatory cell adhesion, and extracellular matrix degradation, all of which contribute to aneurysm formation and rupture.
Another critical hemodynamic parameter is the Oscillatory Shear Index (OSI), a dimensionless measure that quantifies the changes in WSS direction (Meng et al., 2014; Jirjees et al., 2020; Can and Du, 2016; Yamaguchi et al., 2016; Yin et al., 2018; Yin et al., 2016; Huang et al., 2021; Huang et al., 2016; Huang et al., 2018). Higher OSI values indicate a more disturbed flow, and these regions are often associated with the initiation and growth of aneurysms. Elevated OSI values cause endothelial cells to switch to a synthetic phenotype, promoting inflammation, matrix remodeling, and weakening the vascular wall, thereby contributing to aneurysm pathogenesis.
In addition to WSS and OSI, pressure (Kleinloog et al., 2018; Penn et al., 2011) and flow velocity (Can and Du, 2016; Turjman et al., 2014) also play a crucial role. High intra-aneurysmal pressure can cause the aneurysm to expand and eventually rupture. Likewise, high flow velocity within the aneurysm can result in increased WSS and turbulent flow, contributing to aneurysm growth and rupture.
The interplay of these hemodynamic forces can be influenced by several factors, including aneurysm characteristics such as size, location, and morphology. For example, larger aneurysms often exhibit slower flow and lower WSS, creating an environment conducive to aneurysm progression. Similarly, aneurysms located at bifurcations or curvatures of the cerebral arteries, where blood flow is naturally disturbed, often experience abnormal hemodynamic forces, contributing to their development and progression. Aneurysms with a high AR (more spherical) or a high SR (large relative to the parent vessel) are likely to experience unstable and high-velocity flow, which can result in increased WSS, high OSI, and elevated intra-aneurysmal pressure, all of which heighten the risk of aneurysm rupture.
Overall, the hemodynamic forces and the way they interact with the vascular wall and aneurysm characteristics play a pivotal role in the pathogenesis and progression of cerebral aneurysms. A deeper understanding of these forces and their interplay could lead to better prediction models and more effective management strategies for patients with cerebral aneurysms.
Pathology and mechanisms
To understand the reasons behind the rupture of some cerebral aneurysms, it is essential to conduct a meticulous study of their histopathological characteristics and molecular biology. Early histopathology studies through the classical histological staining techniques have confirmed that wall of cerebral aneurysms is characterized by lack of internal elastic lamina and normal intima-media-adventitia layers (Frösen et al., 2012; Hassler, 1961). These studies also showed the presence of inflammatory cells identified as polymorphonuclear leukocytes, plasma cells and small round cells (lymphocytes) (Chyatte et al., 1999; Stehbens, 1963).
From the observations of the histopathology using immunostaining techniques, it has been reported that the rupture of cerebral aneurysm is closely associated with the structural degeneration and infiltration of inflammatory cells in the wall (Frosen et al., 2004). Meanwhile, loss of endothelium, loss of mural cells, breaking down of the collagen matrix and partial hyalinization of the wall have been found in association with rupture of the wall (Kataoka et al., 1999).
Comparing the gene expression of ruptured and unruptured cerebral aneurysms with genome-wide microarrays have been investigated, which shows the overexpression of collagenases (matrix metalloproteinase 2 and 9), pro-apoptotic genes and inducible nitric oxide (NO) synthetase in ruptured cerebral aneurysms, as well as downregulation of anti-apoptotic genes. It is also found 1,426 differently regulated genes of signaling pathways associated with inflammatory cell infiltration, oxidative stress, disturbed cell homeostasis and dysfunctional endothelium were upregulated in ruptured cerebral aneurysms (Kurki et al., 2011).
Rupture of cerebral aneurysms occurs when the matrix of cerebral aneurysms wall has degenerated sufficiently fragile leading to resist the hemodynamic pressure of the cerebral arteries. Normally, collagen fibers would be repaired and maintained by a series of continuous synthesis of new collagen in case of being exposed to abnormally mechanical stress eventually leading to degenerate eventually (Sawabe and international, 2010; Bruno et al., 1998). Meanwhile, walls of ruptured cerebral aneurysm are distinguished by a significant depletion of mural cells (Frosen et al., 2004; Kataoka et al., 1999; Frösen et al., 2006; Kim et al., 1997). When mural cells are lost in the walls, the essential ‘repair and maintenance’ cycle, which relies on matrix synthesis and the proliferation of mural smooth muscle cells is impaired. The absence of mural cells, coupled with the continuous ‘wear and tear’ on collagen fibers and the ongoing proteolytic injury in some cerebral aneurysm walls, which could make the cerebral aneurysms more susceptible to rupture (Frösen et al., 2012). Consequently, the disappearance of mural cells is a pivotal factor contributing to the degeneration and ultimate rupture of cerebral aneurysm walls.
Intraluminal thrombosis, which is linked to the degeneration and rupture of cerebral aneurysms (Frosen et al., 2004), serves as a significant generator of oxidative stress. This stress arises from the activity of peroxidases released by neutrophils that become entangled within the thrombus (Houard et al., 2009). It is reported that oxidative stress may lead to mural cell death in the walls of cerebral aneurysms caused by the accumulation of cytotoxic oxidated lipids in the wall (Frösen et al., 2012). Besides, oxidative stress could indirectly induce inflammation by oxidative modification of intramural lipids of the wall, which would become immunogenic and trigger inflammatory response.
Hence, the advent of innovative techniques capable of detecting endothelial dysfunction, luminal thrombus formation, mural cell death, or inflammation holds promise for identifying cerebral aneurysms at high risk of rupture. These methods could be instrumental given the correlation between such findings and the presence of degenerate, rupture-prone walls in histopathological studies. Beyond enhancing the diagnostic capabilities for cerebral aneurysms prone to rupture, a profound understanding of the wall’s pathobiology may pave the way for the development of novel pharmaceutical or biological therapies. Such interventions could potentially tip the balance in favor of “repair and maintenance” over “cell death and wall degradation”, thereby which would reduce the ruptured risk of cerebral aneurysms instead of necessitating surgical or other invasive procedures to provide better therapeutic strategy for physicians.
Hemodynamics and vascular mechanobiology
Abnormal hemodynamics act as a trigger for vascular remodeling, which indicates that remodeling of arteries as a biological response aimed at normalizing either increased or decreased WSS to a healthy range of 15–20 dynes/cm2 (Hashimoto et al., 2006; Lu and Kassab, 2011). Specifically, the biological processes of vascular remodeling contain vascular smooth muscle cells (VSMCs) apoptosis and migration, degradation of the extracellular matrix (ECM), and inflammation, which lead to local dilation and thinning the vessel wall (Hudson et al., 2013; Penn et al., 2014; Chien and Physiology, 2007; Vasconcellos et al., 2010). Furthermore, it is important to show that the biological response of vascular remodeling in the initiation of cerebral aneurysm is a distinct process from the growth and rupture of cerebral aneurysm.
Under conditions of hemodynamic stress, the release of NO in a flow-dependent manner can inhibit the proliferation of VSMCs and trigger apoptosis by activating the caspase 3 enzyme, which results in pyknosis, karyorrhexis, and ultimately cell death (Vasconcellos et al., 2010). Additionally, mechanical stretch of the vessel wall can induce VSMC apoptosis through the activation of the tumor suppressor protein p53, which is primarily located in the medial layer of blood vessels. VSMC migration is a typical response to vascular injury and is primarily directed towards the intima, the innermost layer of the blood vessel. This migration is influenced by various chemical signals such as amines, peptide growth factors, cytokines, and components of ECM, which contributes to the thinning of the vessel wall (Penn et al., 2014). Migration of VSMCs is conducted by the release of molecules like tumor necrosis factor-alpha (TNF-α) and Kruppel-like transcription factor 4, which lead to a change in the VSMC phenotype. Hence, VSMCs start to express genes associated with upregulation of pro-inflammatory molecules, including matrix metalloproteinases (MMPs), monocyte chemoattractant protein-1 (MCP-1), vascular cell adhesion molecule-1 (VCAM-1), and interleukin (IL) (Signorelli et al., 2015). VSMCs in the intimal layer can proliferate and synthesize new matrix and fibrous tissue, which is a process known as intimal hyperplasia. Typically, in aneurysms that are prone to rupture, areas of decellularization have been identified as part of the wound-healing process, which show loss of mural cells, breakdown of matrix, and hyalinization resembling fibrinoid necrosis (Frösen et al., 2012).
The disappearance of the internal elastic lamina is one of the first changes seen in the tissue structure during aneurysmal growth, leaving the adventitia as the only layer that can withstand the pressure of blood flow. The proteins in the ECM continue to deteriorate as MMPs break down structural proteins like collagens, elastin, proteoglycans, laminin, and fibronectin. Flow-dependent NO release also enhances MMP activity through posttranslational modification (Penn et al., 2014). Specifically, an overexpression of MMP-1, -2, and -9 has been observed in aneurysm walls, with higher levels of MMP-2 and -9 found in ruptured aneurysms (Kawaguchi et al., 2012). Chronic damage caused by collagenases is further increased by the downregulation of antiapoptotic genes (Frösen et al., 2012).
Monocytes are one of the initial cell types to respond to damage to the endothelium and infiltrate the injured site, where they differentiate into macrophages. These macrophages secrete cytokines and proteinases, including MCP-1, TNF-a, and stromal cell–derived factor-1(SDF-1, also known as CXCL12). SDF-1/CXCL12 helps recruit endothelial progenitor cells and promote angiogenesis, as well as further inflammatory cell migration and infiltration (Hoh et al., 2014). Additionally, macrophages contribute to ECM degradation by secreting MMPs. CD163-positive macrophages, the primary macrophages found in cerebral aneurysms, express a specific receptor and are triggered by high levels of oxidative stress contributing to cerebral aneurysm wall degeneration and rupture (Frösen et al., 2012; Fabriek et al., 2005; Lamping et al., 2000; Fisslthaler et al., 2000). Macrophage infiltration has also been linked to intracellular lipid accumulation and apolipoproteins, such as ApoA-I (Ollikainen et al., 2016) and ApoB100 (Frösen et al., 2013).
The difference in atherosclerotic lesions between small and large aneurysms is significantly evident. In small aneurysms, the lesions are characterized by diffuse intimal thickening with mainly VSMCs and minimal macrophages and lymphocytes. However, larger aneurysms have more advanced atherosclerotic lesions with macrophages being the primary cellular infiltrate (Kosierkiewicz et al., 1994).
Humoral immune response is active in the walls of aneurysms, with complement components and antibodies (Immunoglobulin M [IgM] and [IgG]) being deposited (Frösen et al., 2012). Accumulation of C3d has been found in aneurysms, which shows a potentially involved chronic inflammatory state. Activation of the classic pathway, along with amplification of the alternative pathway, has been implicated in releasing chemokines that recruit macrophages and T cells. Membrane attack complexes are rarely found on the cell surface, but are instead located within degraded regions, suggesting complement activation may be a reaction to necrosis rather than the cause of it (Lu and Kassab, 2011).
To understand the correlation between hemodynamics and vascular remodeling associated with cerebral aneurysm rupture is of profound significance in the field of neurovascular medicine, which could predict the risk of rupture, provide appropriate strategies, and potentially guide the development of targeted therapies to stabilize vulnerable aneurysms. Moreover, understanding the interplay between hemodynamics and vascular biology provides a foundation for personalized medicine approaches, where treatment can be tailored based on an individual’s unique aneurysm characteristics and hemodynamic profile, ultimately aiming to prevent rupture and improve patient outcomes.
Imaging modality
Cerebral aneurysms are required to have early and accurate medical intervention immediately, which is crucial for effective treatment and management. In recently years, significant advancements have been made in imaging modalities, enabling healthcare professionals to diagnose cerebral aneurysms with higher accuracy and precision. Herein, there are routinely various imaging techniques used in the detection and diagnosis of cerebral aneurysms, as shown in Figure 4.
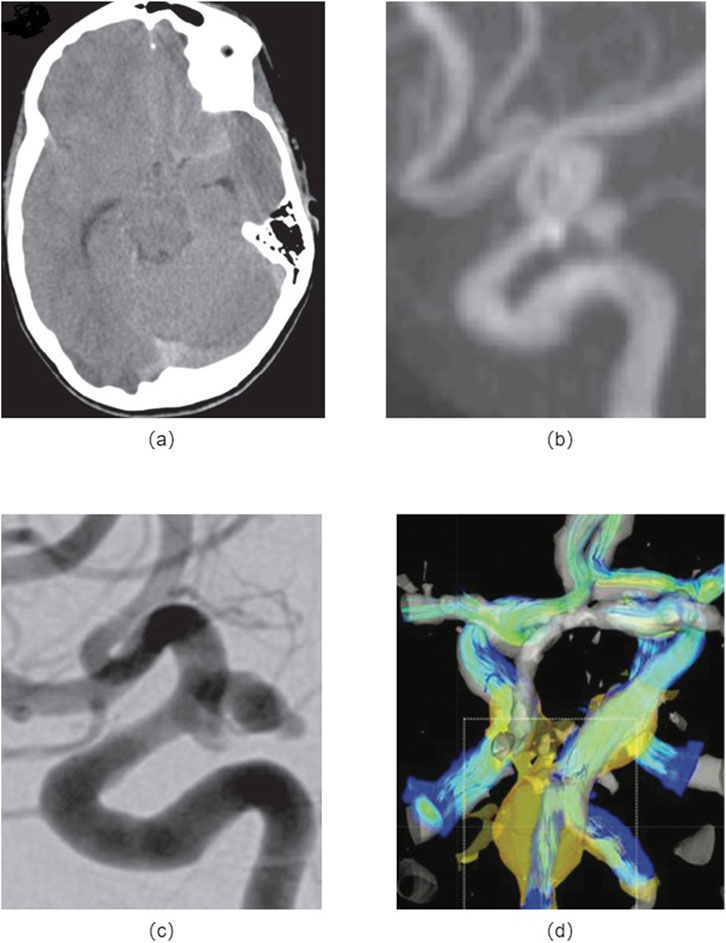
Figure 4. Medical imaging methods: (A) Non-contrast head CT images of a patient with the cerebral aneurysm; (B) MRA shows a left posterior communicating artery aneurysm; (C) DSA indicates an enlarged posterior communicating artery aneurysm; (D) 4D-MRI of the fusiform aneurysm shows the peripheral fast flow channel associated with high WSS.
Computed Tomography Angiography (CTA) has been widely used for the detection of cerebral aneurysms (Diab et al., 2023; Yi et al., 2016a; Fung et al., 2019; Yi et al., 2016b; Villablanca et al., 2013; Shi et al., 2020a; Howard et al., 2019). It involves the injection of a contrast agent into the patient’s bloodstream, followed by a series of X-ray images. CTA provides detailed images of the blood vessels in the brain, allowing for the identification of aneurysms. However, it may have limitations in detecting smaller aneurysms or those located in complex anatomical regions.
Magnetic Resonance Angiography (MRA) utilizes magnetic fields and radio waves to create detailed images of the blood vessels in the brain (Diab et al., 2023; Yi et al., 2016a; Howard et al., 2019; van Amerongen et al., 2014; Ahmed et al., 2019). It is a non-invasive procedure and does not involve the use of ionizing radiation. MRA can provide valuable information about the size, location, and characteristics of cerebral aneurysms. However, it may have limitations in visualizing smaller aneurysms or those in challenging anatomical locations.
Digital Subtraction Angiography (DSA) is considered the gold standard for diagnosing cerebral aneurysms (Fung et al., 2019; Howard et al., 2019; van Amerongen et al., 2014; Ahmed et al., 2019). It involves the injection of a contrast agent into the blood vessels, followed by X-ray imaging. DSA provides highly detailed and accurate images of the cerebral vasculature, allowing for precise detection and characterization of aneurysms. However, it is an invasive procedure and carries a slightly higher risk compared to non-invasive imaging techniques.
4D-Flow Magnetic Resonance Imaging (4D-MRI) is an advanced imaging technique that provides a comprehensive evaluation of blood flow dynamics in the brain (Castle-Kirszbaum et al., 2020; Szajer and Ho-Shon, 2018; Pereira et al., 2016; Turski et al., 2016). It can accurately measure flow velocities, directions, and volumes within the cerebral arteries, allowing for the detection of aneurysms and assessment of their hemodynamic characteristics. This technique is particularly useful in evaluating the risk of rupture and planning treatment strategies.
In clinical practice, various imaging modalities above have been conducted to obtain the medical images for the patients with cerebral aneurysms by the radiologist, it should be precisely and deliberately chosen with the appropriate imaging technique based on the individual patients’ clinical presentation and anatomical characteristics to ensure accurate diagnosis and effective treatment planning (Hesamian et al., 2019).
Blood biomarkers
Early detection and monitoring of cerebral aneurysms are crucial for timely intervention and management performed by the imaging modalities, which have been the primary method for diagnosing cerebral aneurysms. Meanwhile, recent research has focused on the identification of blood biomarkers that could complement imaging techniques (Hussain et al., 2015; Jordan and Nyquist, 2010; Nowicki et al., 2023; Wu et al., 2023). Herein, there are distinct findings of blood biomarkers in the diagnosis, prognosis, and risk assessment of cerebral aneurysms, as shown in Figure 5.
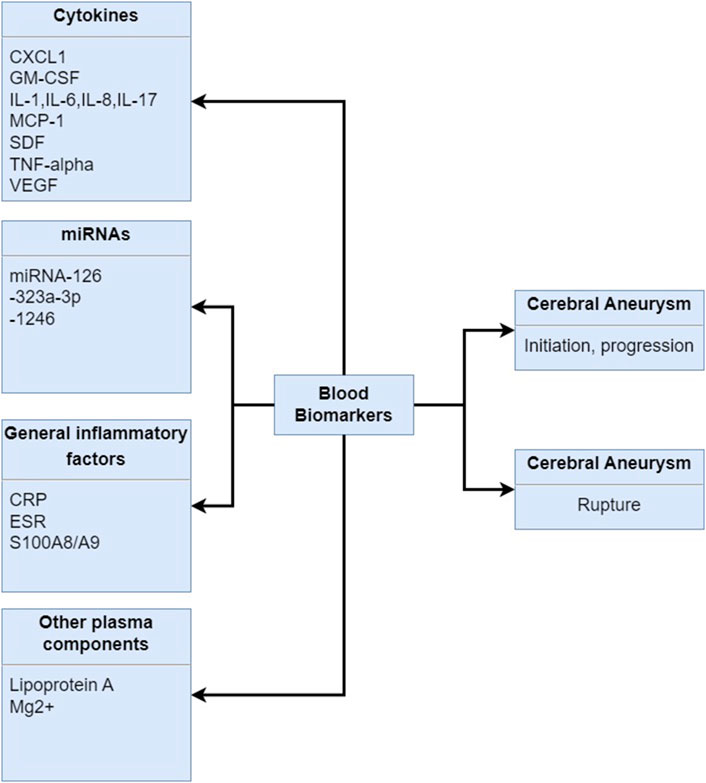
Figure 5. Blood biomarkers in human samples associated with the initiation, progression and rupture of the cerebral aneurysms. CXCL1: chemokine C-X-C motif ligand 1, GM-CSF: granulocyte-monocyte colony-stimulating factor, IL-1: interleukin-1, IL-6: interleukin-6, IL-8: interleukin-8, IL-17: interleukin-17, MCP-1: monocyte chemoattractant protein-1, SDF: stromal cell-derived factor, TNF-alpha: tumor necrosis factor--alpha, VEGF: vascular endothelial growth factor, CRP: C-reactive protein, ESR: erythrocyte sedimentation rate, S100A9/A9: S100 family of proteins.
Inflammatory and Immunological Markers: Inflammation and immune response play critical roles in the pathogenesis and progression of cerebral aneurysms. Studies have identified various inflammatory and immunological markers, such as C-reactive protein (CRP) (Wu et al., 2023; Al-Mufti et al., 2017; Przybycien-Szymanska et al., 2015), interleukin-6 (IL-6) (Wu et al., 2023; Jabbarli et al., 2020; Simon and Grote, 2021; Monsour et al., 2023; Watson et al., 2018), and tumor necrosis factor-alpha (TNF-α) (Hussain et al., 2015; Wu et al., 2023; Watson et al., 2018; Tsilimigras et al., 2018), as potential indicators of aneurysm instability and rupture risk. Elevated levels of these markers in the blood have been associated with aneurysm growth and increased risk of subarachnoid hemorrhage.
Extracellular Matrix Biomarkers: The extracellular matrix (ECM) provides structural support to the vessel walls and is subject to remodeling processes in response to hemodynamic forces and inflammation. Biomarkers of ECM remodeling, such as matrix metalloproteinases (MMPs) (Zhang et al., 2019; Li et al., 2017; Lattanzi et al., 2020), particularly MMP-2 (Mehta et al., 2013; Gareev et al., 2021) and MMP-9 (Zhang et al., 2019; Kassam et al., 2004), and tissue inhibitors of metalloproteinases (TIMPs) (Signorelli et al., 2018; Urbonavicius et al., 2008), have been implicated in aneurysm growth and rupture. Assessing the balance between ECM degradation and synthesis may offer insights into aneurysm stability and inform risk stratification.
Endothelial Dysfunction and Oxidative Stress Biomarkers: Endothelial dysfunction and increased oxidative stress contribute to vascular damage and play a role in aneurysm development (Signorelli et al., 2018). Biomarkers of endothelial dysfunction, such as von Willebrand factor (vWF) (Hussain et al., 2015; Ma et al., 2021) and asymmetric dimethylarginine (ADMA) (Siasos et al., 2015; Miao and Liao, 2014), and markers of oxidative stress (Signorelli et al., 2018; Hussain et al., 2015), such as malondialdehyde (MDA) (Husseini and Laskowitz, 2010; Rodríguez-Rodríguez et al., 2014) and 8-hydroxy-2′-deoxyguanosine (8-OHdG) (Duan et al., 2016; Korkmaz et al., 2018), have been explored as potential indicators of aneurysm risk. These markers reflect the vascular response to hemodynamic and inflammatory insults and may aid in risk assessment.
Genetic and Epigenetic Biomarkers: Advancements in genetic and epigenetic research have uncovered genetic variants and epigenetic modifications associated with aneurysm susceptibility and progression (Bakker and Ruigrok, 2021; Fernández-Pérez et al., 2024). Polymorphisms in genes involved in vascular biology, such as ACTA2 (Balmforth et al., 2019; Boileau et al., 2018), MYH11 (Wang et al., 2023; Saratzis and Bown, 2014), and COL3A1 (Kassam et al., 2004; Laurent et al., 2022), have been linked to aneurysm formation and rupture. Additionally, epigenetic changes, such as DNA methylation (Fernández-Pérez et al., 2024; Samuel and Radovanovic, 2019) and histone modifications (Fernández-Pérez et al., 2024), may regulate gene expression and contribute to aneurysm pathogenesis. These genetic and epigenetic factors hold promise as novel biomarkers for risk assessment and personalized medicine (Alg et al., 2013).
Integration of Biomarkers: The combination of multiple biomarkers may improve the diagnostic and prognostic accuracy of cerebral aneurysms (Kleinloog et al., 2018; Hussain et al., 2015). Research has suggested that a panel of biomarkers, including inflammatory, extracellular matrix, endothelial dysfunction, and genetic markers, could provide a more comprehensive assessment of aneurysm risk and aid in clinical decision-making. This approach may pave the way for personalized medicine, tailored monitoring and treatment strategies for patients with cerebral aneurysms.
Blood biomarkers offer a promising approach to complement existing imaging techniques in the detection, prognosis, and risk assessment of cerebral aneurysms. While individual biomarkers have shown potential, the integration of multiple biomarkers may enhance the diagnostic accuracy and predictive value. Future research should focus on validating these biomarkers in larger clinical studies and developing biomarker panels that can be effectively translated into clinical practice. The incorporation of blood biomarkers into routine clinical assessments could lead to more personalized and precise management strategies for patients with cerebral aneurysms.
Artificial intelligence
Recent advancements in artificial intelligence (AI) have shown great promise in enhancing the detection and management of these aneurysms (Yang et al., 2021; Zhu et al., 2020; Liu et al., 2019; Xiong et al., 2022; Larson et al., 2018; Mouridsen et al., 2020; Jin et al., 2020; Ahn et al., 2021; Detmer et al., 2020; Salman et al., 2023; Lin et al., 2022). AI has emerged as a powerful tool for enhancing the detection, risk assessment, and treatment planning of cerebral aneurysms. AI algorithms have shown great potential in improving the accuracy and efficiency of diagnosis, as well as providing valuable insights into aneurysm prognosis and treatment outcomes (Kim D. W. et al., 2019; Kim H. C. et al., 2019; Shi et al., 2020a; Hesamian et al., 2019; He et al., 2019; Wang and Summers, 2012; Liu et al., 2018; Heo et al., 2020; Nabaei, 2022; Din et al., 2023; Marasini et al., 2022). Herein, the role of AI in improving the diagnosis, risk assessment, and treatment planning for cerebral aneurysms are discussed in the following study, as shown in Figure 6.
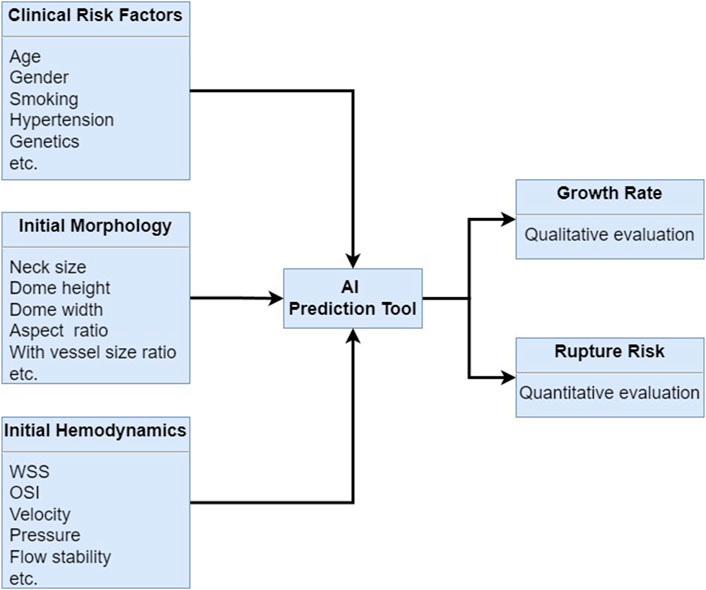
Figure 6. AI algorithm prediction for cerebral growth rate and rupture risk by combining the clinical risk factors, initial morphology and initial hemodynamics.
AI in Cerebral Aneurysm Detection: AI algorithms, particularly deep learning convolutional neural networks (CNNs), have demonstrated remarkable accuracy in detecting cerebral aneurysms from CTA and MRA images (Hosny et al., 2018). These algorithms can automatically identify and segment aneurysms, reducing the workload on radiologists and improving the efficiency of diagnosis. Several studies have shown that AI-based detection systems can achieve high sensitivity and specificity, often matching or surpassing the performance of human experts.
AI in Risk Assessment and Prognosis: AI algorithms can analyze various imaging features and clinical data to assess the risk of aneurysm rupture and predict patient outcomes. By integrating information such as aneurysm size, shape, location, hemodynamics, and blood biomarker related to the rupture of cerebral aneurysm, AI systems can provide valuable insights into the likelihood of aneurysm growth and rupture. This information can help clinicians in making informed decisions regarding the need for surgical intervention and the choice of treatment strategy.
AI in Treatment Planning: AI can also play a significant role in treatment planning for cerebral aneurysms. For instance, AI algorithms can assist in simulating blood flow dynamics within the cerebral vasculature, helping to identify regions at high risk of rupture. Additionally, AI can aid in the selection of treatment modalities, such as endovascular coiling or surgical clipping, by predicting the success rates and potential complications associated with each approach.
Despite the promising results, several challenges need to be addressed for the widespread adoption of AI in cerebral aneurysm management (Chen et al., 2022; Hesamian et al., 2019; Recht et al., 2020; Shi et al., 2020b). These include the need for large, diverse, and annotated datasets for training AI algorithms, ensuring the generalizability of these models across different populations and imaging protocols, and addressing issues related to data privacy and security. Future research should focus on developing robust AI systems that can be seamlessly integrated into routine clinical practice, as well as exploring the potential of AI in personalized medicine for cerebral aneurysm patients. Overcoming the current challenges and continued advancements in AI technology are expected to further revolutionize the field of cerebral aneurysm management, ultimately leading to better patient care and outcomes (Jiang et al., 2017).
Fluid-structure interaction
Fluid-structure interaction (FSI) analysis is a computational modeling technique that simulates the dynamic interaction between blood flow and the vessel walls, providing valuable insights into the hemodynamics and structural stresses involved in cerebral aneurysms (Kleinstreuer et al., 2007; Nazri et al., 2020; Turjman et al., 2014; Mourato et al., 2022; Bai-Nan et al., 2011). Understanding the mechanics behind aneurysm development, growth, and rupture is essential for improving diagnosis, risk assessment, and treatment strategies in clinical practice. FSI could simulate the relatively physiology conditions for patients, which considers the interaction between the vessel and blood, as shown in Figure 7.
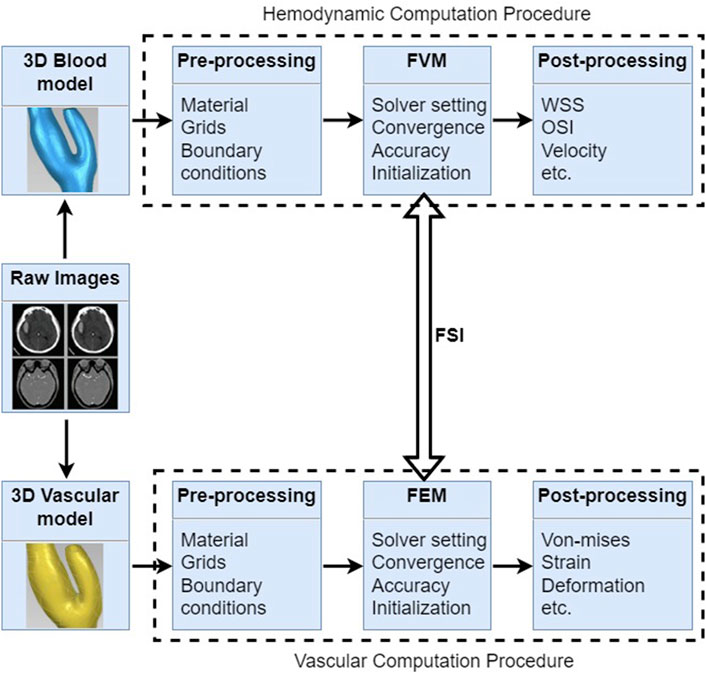
Figure 7. Fluid-structure interaction procedures for cerebral aneurysms include hemodynamic computation and vascular computation, which shows 3D reconstructed model, pre-processing, computational methods and post-processing, respectively.
FSI computation allows for the detailed examination of blood flow patterns and hemodynamics within cerebral aneurysms. Studies have shown that certain flow characteristics, such as high WSS, flow impingement (Can and Du, 2016), and high velocity jets, can contribute to aneurysm initiation and growth. By simulating these flow dynamics, FSI analysis can help identify regions at high risk of aneurysm formation and rupture, guiding clinical decision-making regarding treatment options.
The structural response of the aneurysm wall to hemodynamics is a critical factor in determining its stability and risk of rupture. FSI computation enables the evaluation of wall stress and deformation patterns, providing insights into the mechanical environment that predisposes aneurysms to rupture. By incorporating material properties of the vessel wall and studying the effects of wall remodeling, FSI analysis can enhance our understanding of the mechanical mechanisms underlying aneurysm growth and rupture.
FSI computation holds great promise for personalized medicine in the context of cerebral aneurysms. By simulating patient-specific geometries and hemodynamic conditions, FSI models can provide tailored information on the risk of aneurysm rupture and assist in treatment planning. For instance, FSI simulations can predict the distribution of WSS after endovascular coiling or surgical clipping, aiding in the selection of the most appropriate treatment strategy for individual patients.
While FSI computation has made significant contributions to our understanding of cerebral aneurysmal hemodynamics, several challenges remain to be addressed. These include the development of accurate and validated constitutive models for the vessel wall, the integration of biological factors such as inflammation and remodeling processes, and the requirement of larger-scale clinical studies to validate the predictive capabilities of FSI models. In the further study, FSI computation combined the previouslly realsitic challenges should be considered and applicable in routine clinical practice, which will promote the personalized medicine, treatment planning, and ultimately improving patient outcomes in the management of cerebral aneurysms.
Limitations and challanges
Although various risk factors have been proposed in predicting the rupture of cerebral aneurysms in the previous studies, these methods have inherent limitations and challenges that affect the predictive accuracy and clinical utility. Herein, there are limitations and challenges associated with the risk assessment of cerebral aneurysms in the previous study.
Morphological Characteristics: Morphological features, such as aneurysm size, shape, location, and aspect ratio, have been used to assess the risk of rupture. However, several limitations exist: (1) Size alone is an imperfect predictor, as small aneurysms can rupture, while larger ones may remain stable; (2) The complexity of aneurysm shapes makes it challenging to quantify risk, and subjective assessments can lead to variability among interpreters; (3) The relationship between aneurysm geometry and rupture risk is not fully understood, and the impact of geometric changes over time remains unclear.
Hemodynamical Characteristics: Hemodynamic factors, such as blood flow patterns, WSS, and pressure gradients, are crucial in aneurysm pathogenesis. However, their use in risk assessment has challenges: (1) The correlation between specific hemodynamic patterns and rupture risk is not always consistent, and the threshold values for high-risk conditions are not well-defined; (2) Hemodynamic simulations require accurate aneurysm geometry and boundary conditions, which can be difficult to obtain, especially in the presence of intraluminal thrombus or altered vascular wall properties; (3) The invasive nature of methods like phase-contrast magnetic resonance imaging (MRI) or measurement of intravascular pressure limits their applicability in routine clinical practice.
Intrasubject Variability: There is considerable intrasubject variability in aneurysm characteristics, which can change over time due to factors such as inflammation, remodeling, and changes in blood flow. This variability makes it challenging to establish a baseline for risk assessment and necessitates repeated evaluations, which may not be practical or cost-effective.
Lack of Standardization: The lack of standardized definitions and criteria for aneurysm risk assessment tools contributes to variability in interpretation and communication between clinicians and researchers. This hinders the development of consensus guidelines and the validation of risk assessment models across different studies and centers.
Integration of Multimodal Data: While morphological and hemodynamical characteristics are important, they represent only part of the complex picture of aneurysm risk. Integrating these data with other clinical and biological factors remains a challenge, and the potential of multimodal data fusion for improved risk assessment is yet to be fully realized.
In summary, advances in imaging technology, computational modeling, and data analysis techniques are required to address these issues. Standardization of definitions and criteria, development of robust validation frameworks, and integration of multimodal data are key steps toward improving the accuracy and clinical utility of risk assessment tools for cerebral aneurysms.
Conclusion
Rupture risk factors and predictors in cerebral aneurysms were performed through various methods related to clinical perspective, morphological parameters, hemodynamic parameters, pathology and mechanisms, vascular mechanobiology, imaging modalities, blood biomarkers, artificial intelligence, and fluid-structure interaction, which showed important significance in diagnosis, progression and patient-specific treatment. Meanwhile, current limitations and challenges of the previous methods to predict the rupture of cerebral aneurysms were outlined and should be enhanced to identify in the further study. By comprehending and pinpointing the diverse risk factors and predictive biomarkers linked to aneurysm rupture, we can enhance personalized risk evaluation and refine therapeutic approaches for individuals with cerebral aneurysms.
Author contributions
XW: Conceptualization, Investigation, Writing–original draft, Methodology. XH: Supervision, Writing–review and editing, Project administration, Validation.
Funding
The author(s) declare that no financial support was received for the research, authorship, and/or publication of this article.
Acknowledgments
We would like to thank all participants of the study in the Department of Mechanical and Automation Engineering at The Chinese University of Hong Kong (CUHK), Department of Mechanics and Engineering Science at Peking University (PKU), School of Intelligent Manufacturing at Nanjing University of Science and Technology (NJUST).
Conflict of interest
Authors XW and XH were employed by Shanghai Aohua Photoelectricity Endoscope Co., Ltd.
Publisher’s note
All claims expressed in this article are solely those of the authors and do not necessarily represent those of their affiliated organizations, or those of the publisher, the editors and the reviewers. Any product that may be evaluated in this article, or claim that may be made by its manufacturer, is not guaranteed or endorsed by the publisher.
References
Ahmed S. U., Mocco J., Zhang X., Kelly M., Doshi A., Nael K., et al. (2019). MRA versus DSA for the follow-up imaging of intracranial aneurysms treated using endovascular techniques: a meta-analysis. Journal of neurointerventional surgery 11 (10), 1009–1014. doi:10.1136/neurintsurg-2019-014936
Ahn J. H., Kim H. C., Rhim J. K., Park J. J., Sigmund D., Park M. C., et al. (2021). Multi-view convolutional neural networks in rupture risk assessment of small, unruptured intracranial aneurysms. J. Pers. Med. 11 (4), 239. doi:10.3390/jpm11040239
Ajiboye N., Chalouhi N., Starke R. M., Zanaty M., Bell R. J. T. S. W. J. (2015). Unruptured cerebral aneurysms evaluation and management. The Scientific World Journal, 2015.
Alg V. S., Sofat R., Houlden H., Werring D. J. J. N. (2013), Genetic risk factors for intracranial aneurysms: a meta-analysis in more than 116,000 individuals. 80(23) 2154–2165. doi:10.1212/WNL.0b013e318295d751
Al-Mufti F., Amuluru K., Smith B., Damodara N., El-Ghanem M., Singh I. P., et al. (2017). Emerging markers of early brain injury and delayed cerebral ischemia in aneurysmal subarachnoid hemorrhage. World Neurosurg. 107, 148–159. doi:10.1016/j.wneu.2017.07.114
Backes D., Rinkel G. J., Laban K. G., Algra A., Vergouwen M. D. J. S. (2016). Patient-and aneurysm-specific risk factors for intracranial aneurysm growth: a systematic review and meta-analysis. Stroke Vasc. Interv. Neurol. 47 (4), 951–957. doi:10.1161/STROKEAHA.115.012162
Baharoglu M. I., Schirmer C. M., Hoit D. A., Gao B.-L., Malek A. M. J. S. (2010). Aneurysm inflow-angle as a discriminant for rupture in sidewall cerebral aneurysms: morphometric and computational fluid dynamic analysis. Stroke Vasc. Interv. Neurol. 41 (7), 1423–1430. doi:10.1161/STROKEAHA.109.570770
Bai-Nan X., Fu-Yu W., Lei L., Xiao-Jun Z., Hai-Yue J. J. N. r. (2011). Hemodynamics model of fluid–solid interaction in internal carotid artery aneurysms. Neurosurg. Rev. 34, 39–47. doi:10.1007/s10143-010-0282-5
Bakker M. K., Ruigrok Y. M. J. S. (2021). Genetics of intracranial aneurysms. Stroke Vasc. Interv. Neurol. 52 (9), 3004–3012. doi:10.1161/strokeaha.120.032621
Balmforth D., Harky A., Adams B., Yap J., Shipolini A., Roberts N., et al. (2019). Is there a role for biomarkers in thoracic aortic aneurysm disease? Gen. Thorac. Cardiovasc. Surg. 67, 12–19. doi:10.1007/s11748-017-0855-0
Biller J., Godersky J. C., Adams H. P. J. S. (1988). Management of aneurysmal subarachnoid hemorrhage. Manag. aneurysmal subarachnoid hemorrhage 19 (10), 1300–1305. doi:10.1161/01.str.19.10.1300
Boileau A., Lindsay M. E., Michel J.-B., Devaux Y. J. A. (2018). Epigenetics in ascending thoracic aortic aneurysm and dissection. Aorta (Stamford). 6 (01), 001–012. doi:10.1055/s-0038-1639610
Boussel L., Rayz V., McCulloch C., Martin A., Acevedo-Bolton G., Lawton M., et al. (2008). Aneurysm growth occurs at region of low wall shear stress: patient-specific correlation of hemodynamics and growth in a longitudinal study. Stroke Vasc. Interv. Neurol. 39 (11), 2997–3002. doi:10.1161/STROKEAHA.108.521617
Brinjikji W., Zhu Y.-Q., Lanzino G., Cloft H., Murad M. H., Wang Z., et al. (2016). Risk factors for growth of intracranial aneurysms: a systematic review and meta-analysis. AJNR. Am. J. Neuroradiol. 37 (4), 615–620. doi:10.3174/ajnr.a4575
Brisman J. L., Song J. K.D.W.J.N.E.j.o.m. Newell (2006). Cerebral aneurysms. New England journal of medicine 355(9) 928–939.
Brown R. D., Broderick J. P. J. T. L. N. (2014). Unruptured intracranial aneurysms: epidemiology, natural history, management options, and familial screening. Lancet. Neurol. 13 (4), 393–404. doi:10.1016/S1474-4422(14)70015-8
Bruno G., Todor R., Lewis I., Chyatte D. J. J. o.n. (1998). Vascular extracellular matrix remodeling in cerebral aneurysms. J. Neurosurg. 89 (3), 431–440. doi:10.3171/jns.1998.89.3.0431
Burkhardt J.-K., Benet A., Lawton M. T. J. N. C. (2017). Management of small incidental intracranial aneurysms. Neurosurg. Clin. N. Am. 28 (3), 389–396. doi:10.1016/j.nec.2017.02.006
Can A., Du R. J. N., Association of hemodynamic factors with intracranial aneurysm formation and rupture: systematic review and meta-analysis. 78(4) (2016) 510–520. doi:10.1227/NEU.0000000000001083
Carter B. S., Sheth S., Chang E., Sethl M., Ogilvy C. S. J. N., Epidemiology of the size distribution of intracranial bifurcation aneurysms: smaller size of distal aneurysms and increasing size of unruptured aneurysms with age. 58(2) (2006) 217–223. doi:10.1227/01.NEU.0000194639.37803.F8
Castle-Kirszbaum M., Maingard J., Lim R. P., Barras C. D., Kok H. K., Chandra R. V., et al. Four-dimensional magnetic resonance imaging assessment of intracranial aneurysms: a state-of-the-art review. 87(3) (2020) 453–465. doi:10.1093/neuros/nyaa021
Chalouhi N., Hoh B. L., Hasan D. J. S. (2013). Review of cerebral aneurysm formation, growth, and rupture. Stroke Vasc. Interv. Neurol. 44 (12), 3613–3622. doi:10.1161/STROKEAHA.113.002390
Chen X., Lei Y., Su J., Yang H., Ni W., Yu J., et al. (2022). A review of artificial intelligence in cerebrovascular disease imaging: applications and challenges. Curr. Neuropharmacol. 20 (7), 1359–1382. doi:10.2174/1570159X19666211108141446
Chien S. J. A. J. o.P.-H., Physiology C., Mechanotransduction and endothelial cell homeostasis: the wisdom of the cell. 292(3) (2007) H1209–H1224.
Cho K.-C., Yang H., Kim J.-J., Oh J. H., Kim Y. B. J. S. r. (2020). Prediction of rupture risk in cerebral aneurysms by comparing clinical cases with fluid–structure interaction analyses. Sci. Rep. 10 (1), 18237. doi:10.1038/s41598-020-75362-5
Cho K.-C. J. N. (2023). The current limitations and advanced analysis of hemodynamic study of cerebral aneurysms. Neurointervention 18 (2), 107–113. doi:10.5469/neuroint.2023.00164
Chyatte D., Bruno G., Desai S., Todor D. R. J. N., Inflammation and intracranial aneurysms. 45(5) (1999) 1137, 1146. doi:10.1097/00006123-199911000-00024
Detmer F. J., Lückehe D., Mut F., Slawski M., Hirsch S., Bijlenga P., et al. (2020). Comparison of statistical learning approaches for cerebral aneurysm rupture assessment. Int. J. Comput. Assist. Radiol. Surg. 15, 141–150. doi:10.1007/s11548-019-02065-2
Dhar S., Tremmel M., Mocco J., Kim M., Yamamoto J., Siddiqui A. H., et al. Morphology parameters for intracranial aneurysm rupture risk assessment. 63(2) (2008) 185–196. doi:10.1227/01.NEU.0000316847.64140.81
Diab R., Chang D., Zhu C., Levitt M. R., Aksakal M., Zhao H.-L., et al. (2023). Advanced cross-sectional imaging of cerebral aneurysms. Br. J. Radiol. 96 (1141), 20220686. doi:10.1259/bjr.20220686
Diagbouga M. R., Morel S., Bijlenga P., Kwak B. R. J. E. j.o.c.i. (2018). Role of hemodynamics in initiation/growth of intracranial aneurysms. Eur. J. Clin. Invest. 48 (9), e12992. doi:10.1111/eci.12992
Din M., Agarwal S., Grzeda M., Wood D. A., Modat M., Booth T. C. J. J. o.N. S. (2023). Detection of cerebral aneurysms using artificial intelligence: a systematic review and meta-analysis. J. Neurointerv. Surg. 15 (3), 262–271. doi:10.1136/jnis-2022-019456
Duan X., Wen Z., Shen H., Shen M., Chen G. J. O. m., longevity c. (2016). Intracerebral hemorrhage, oxidative stress, and antioxidant therapy, 2016.
Etminan N., Rinkel G. J. J. N. R. N. (2016). Unruptured intracranial aneurysms: development, rupture and preventive management. Nat. Rev. Neurol. 12 (12), 699–713. doi:10.1038/nrneurol.2016.150
Fabriek B. O., Dijkstra C. D., van den Berg T. K. J. I. (2005). The macrophage scavenger receptor CD163. Immunobiology 210 (2-4), 153–160. doi:10.1016/j.imbio.2005.05.010
Fernández-Pérez I., Macias-Gómez A., Suárez-Pérez A., Vallverdú-Prats M., Giralt-Steinhauer E., Bojtos L., et al. (2024). The role of epigenetics in brain aneurysm and subarachnoid hemorrhage: a comprehensive review. Int. J. Mol. Sci. 25 (6), 3433. doi:10.3390/ijms25063433
Findlay J. M., Deagle G. M. J. C. j.o.n.s. (1998). Causes of morbidity and mortality following intracranial aneurysm rupture. Can. J. Neurol. Sci. 25 (3), 209–215. doi:10.1017/s031716710003403x
Fisslthaler D. H., Scandinavica B. J. A. P. (2000). Phosphorylation and activation of the endothelial nitric oxide synthase by fluid shear stress. 168(1) 81–88.
Forget T. R., Benitez R., Veznedaroglu E., Sharan A., Mitchell W., Silva M., et al. A review of size and location of ruptured intracranial aneurysms. 49(6) (2001) 1322–1325. doi:10.1097/00006123-200112000-00006
Frösen J., Litmanen S., Tulamo R., Hernesniemi J., Jääskeläinen J. E., Salminen U.-S., et al. Matrix metalloproteinase-2 and-9 expression in the wall of saccular cerebral artery aneurysm: Paper 54, 58(2) (2006) 413. doi:10.1227/01.neu.0000310209.59421.c5
Frosen J., Piippo A., Paetau A., Kangasniemi M., Niemelaa M., Hernesniemi J., et al. (2004). Remodeling of saccular cerebral artery aneurysm wall is associated with rupture: histological analysis of 24 unruptured and 42 ruptured cases. Stroke Vasc. Interv. Neurol. 35 (10), 2287–2293. doi:10.1161/01.STR.0000140636.30204.da
Frösen J., Tulamo R., Heikura T., Sammalkorpi S., Niemelä M., Hernesniemi J., et al. (2013) Lipid accumulation, lipid oxidation, and low plasma levels of acquired antibodies against oxidized lipids associate with degeneration and rupture of the intracranial aneurysm wall, 1, 1–13.
Frösen J., Tulamo R., Paetau A., Laaksamo E., Korja M., Laakso A., et al. (2012) Saccular intracranial aneurysm: pathology and mechanisms, Acta Neuropathol., 773–786. doi:10.1007/s00401-011-0939-3
Fung C., Mavrakis E., Filis A., Fischer I., Suresh M., Tortora A., et al. (2019). Anatomical evaluation of intracranial aneurysm rupture risk in patients with multiple aneurysms. Neurosurg. Rev. 42, 539–547. doi:10.1007/s10143-018-0998-1
Gareev I., Beylerli O., Yang G., Izmailov A., Shi H., Sun J., et al. (2021). Diagnostic and prognostic potential of circulating miRNAs for intracranial aneurysms. Neurosurg. Rev. 44, 2025–2039. doi:10.1007/s10143-020-01427-8
Grasso G., Alafaci C., Macdonald R. L. J. S. n.i. (2017). Management of aneurysmal subarachnoid hemorrhage: state of the art and future perspectives, Surg. Neurol. Int., 8 11. doi:10.4103/2152-7806.198738
Hackenberg K. A., Rajabzadeh-Oghaz H., Dreier R., Buchholz B. A., Navid A., Rocke D. M., et al. (2020). Collagen turnover in relation to risk factors and hemodynamics in human intracranial aneurysms. Stroke Vasc. Interv. Neurol. 51 (5), 1624–1628. doi:10.1161/STROKEAHA.120.029335
Hashimoto T., Meng H., Young W. L. J. N. r. (2006). Intracranial aneurysms: links among inflammation, hemodynamics and vascular remodeling. Neurol. Res. 28 (4), 372–380. doi:10.1179/016164106X14973
Hassler O. J. A. P. S. (1961). Morphological studies on the large cerebral arteries, with reference to the aetiology of subarachnoid haemorrhage. Acta Psychiatr. Scand. Suppl. 154, 1–145.
He J., Baxter S. L., Xu J., Xu J., Zhou X., Zhang K. J. N. m. (2019). The practical implementation of artificial intelligence technologies in medicine. Nat. Med. 25 (1), 30–36. doi:10.1038/s41591-018-0307-0
Heo J., Park S. J., Kang S.-H., Oh C. W., Bang J. S., Kim T. J. S. r. (2020). Prediction of intracranial aneurysm risk using machine learning. Mach. Learn. 10 (1), 6921. doi:10.1038/s41598-020-63906-8
Hesamian M. H., Jia W., He X., Kennedy P. J. J. o.d.i. (2019). Deep learning techniques for medical image segmentation: achievements and challenges. J. Digit. Imaging 32, 582–596. doi:10.1007/s10278-019-00227-x
Hoh B. L., Hosaka K., Downes D. P., Nowicki K. W., Wilmer E. N., Velat G. J., et al. (2014). Stromal cell–derived factor-1 promoted angiogenesis and inflammatory cell infiltration in aneurysm walls. J. Neurosurg. 120 (1), 73–86. doi:10.3171/2013.9.JNS122074
Hop J. W., Rinkel G. l.J., Algra A., Van Gijn J. J. S. (1997). Case-fatality rates and functional outcome after subarachnoid hemorrhage: a systematic review. Stroke 28 (3), 660–664. doi:10.1161/01.str.28.3.660
Hosny A., Parmar C., Quackenbush J., Schwartz L. H., Aerts H. J. J. N. R. C. (2018). Artificial intelligence in radiology. Nat. Rev. Cancer 18 (8), 500–510. doi:10.1038/s41568-018-0016-5
Houard X., Ollivier V., Louedec L., Michel J. B., Back M. J. T. F. J., Differential inflammatory activity across human abdominal aortic aneurysms reveals neutrophilderived leukotriene B4 as a major chemotactic factor released from the intraluminal thrombus, 23(5) (2009) 1376–1383. doi:10.1096/fj.08-116202
Howard B. M., Hu R., Barrow J. W., Barrow D. L. J. N. f. (2019). Comprehensive review of imaging of intracranial aneurysms and angiographically negative subarachnoid hemorrhage. Neurosurg. Focus 47 (6), E20. doi:10.3171/2019.9.FOCUS19653
Huang X., Liu D., Wang X. J. J. o.B. (2021) Morphological and hemodynamic analysis of the patient-specific renal cell carcinoma models. 126.110636
Huang X., Liu D., Yin X., Yajun E., Li Z., Tan W. (2018) Morphometry and hemodynamics of posterior communicating artery aneurysms: ruptured versus unruptured. 76, 35–44.
Huang X., Yin X., Xu Y., Jia X., Li J., Niu P., et al. (2016). Morphometric and hemodynamic analysis of atherosclerotic progression in human carotid artery bifurcations. Am. J. Physiol. Heart Circ. Physiol. 310 (5), H639–H647. doi:10.1152/ajpheart.00464.2015
Hudson J. S., Hoyne D. S., Hasan D. M. J. F. n. (2013). Inflammation and human cerebral aneurysms: current and future treatment prospects. Future Neurol. 8 (6), 663–676. doi:10.2217/fnl.13.40
Hussain S., Barbarite E., Chaudhry N. S., Gupta K., Dellarole A., Peterson E. C., et al. (2015). Search for biomarkers of intracranial aneurysms: a systematic review. World Neurosurgery 84 (5), 1473–1483. doi:10.1016/j.wneu.2015.06.034
Husseini N. E., Laskowitz D. T. J. E. r.o.n. (2010). Clinical application of blood biomarkers in cerebrovascular disease. Expert Rev. Neurother. 10 (2), 189–203. doi:10.1586/ern.09.151
Ingall T., Asplund K., Bonita R. J. S., A multinational comparison of subarachnoid hemorrhage epidemiology in the WHO MONICA stroke study. 31(5) (2000) 1054–1061.
Jabbarli R., Pierscianek D., Darkwah Oppong M., Sato T., Dammann P., Wrede K. H., et al. (2020). Laboratory biomarkers of delayed cerebral ischemia after subarachnoid hemorrhage: a systematic review. Neurosurgical Review 43, 825–833. doi:10.1007/s10143-018-1037-y
Jeong Y.-G., Jung Y.-T., Kim M.-S., Eun C.-K., Jang S.-H. J. J. o.K. N. S. (2009). Size and location of ruptured intracranial aneurysms. J. Korean Neurosurg. Soc. 45 (1), 11–15. doi:10.3340/jkns.2009.45.1.11
Jiang F., Jiang Y., Zhi H., Dong Y., Li H., Ma S., et al. (2017). Artificial intelligence in healthcare: past, present and future. Stroke and Vascular Neurology 2 (4), 230–243. doi:10.1136/svn-2017-000101
Jin D., Song C., Leng X., Han P. J. I. j.o.s. (2019). A systematic review and meta-analysis of risk factors for unruptured intracranial aneurysm growth. Int. J. Surg. 69, 68–76. doi:10.1016/j.ijsu.2019.07.023
Jin H., Geng J., Yin Y., Hu M., Yang G., Xiang S., et al. (2020). Fully automated intracranial aneurysm detection and segmentation from digital subtraction angiography series using an end-to-end spatiotemporal deep neural network. J. Neurointerv. Surg. 12 (10), 1023–1027. doi:10.1136/neurintsurg-2020-015824
Jirjees S., Htun Z. M., Aldawudi I., Katwal P. C., Khan S. J. C., Role of morphological and hemodynamic factors in predicting intracranial aneurysm rupture: a review. 12, e9178, doi:10.7759/cureus.91787) (2020).
Jordan J. D., Nyquist P. J. N. C. (2010). Biomarkers and vasospasm after aneurysmal subarachnoid hemorrhage. Neurosurg. Clin. N. Am. 21 (2), 381–391. doi:10.1016/j.nec.2009.10.009
Kassam A., Horowitz M., Chang Y.-F., Peters D. J. N., Altered arterial homeostasis and cerebral aneurysms: a review of the literature and justification for a search of molecular biomarkers. 54(5) (2004) 1199–1111. doi:10.1227/01.neu.0000119708.26886.55
Kataoka K., Taneda M., Asai T., Kinoshita A., Ito M., Kuroda R. J. S. (1999). Structural fragility and inflammatory response of ruptured cerebral aneurysms: a comparative study between ruptured and unruptured cerebral aneurysms. Stroke Vasc. Interv. Neurol. 30 (7), 1396–1401. doi:10.1161/01.str.30.7.1396
Kawaguchi T., Nishimura S., Kanamori M., Takazawa H., Omodaka S., Sato K., et al. (2012). Distinctive flow pattern of wall shear stress and oscillatory shear index: similarity and dissimilarity in ruptured and unruptured cerebral aneurysm blebs. J. Neurosurg. 117 (4), 774–780. doi:10.3171/2012.7.JNS111991
Kayembe K., Sasahara M., Hazama F. J. S. (1984). Cerebral aneurysms and variations in the circle of Willis. Stroke Vasc. Interv. Neurol. 15 (5), 846–850. doi:10.1161/01.str.15.5.846
Kim D. W., Jang H. Y., Kim K. W., Shin Y., Park S. H. J. K. j.o.r. (2019a). Design characteristics of studies reporting the performance of artificial intelligence algorithms for diagnostic analysis of medical images: results from recently published papers. Korean J. Radiol. 20 (3), 405–410. doi:10.3348/kjr.2019.0025
Kim H. C., Rhim J. K., Ahn J. H., Park J. J., Moon J. U., Hong E. P., et al. (2019b). Machine learning application for rupture risk assessment in small-sized intracranial aneurysm. J. Clin. Med. 8 (5), 683. doi:10.3390/jcm8050683
Kim S. C., Singh M., Huang J., Prestigiacomo C. J., Winfree C. J., Solomon R. A., et al. Matrix metalloproteinase-9 in cerebral aneurysms. 41(3) (1997) 642–666. doi:10.1097/00006123-199709000-00027
Kleinloog R., De Mul N., Verweij B. H., Post J. A., Rinkel G. J., Ruigrok Y. M. J. N. (2018). Risk factors for intracranial aneurysm rupture: a systematic review. Neurosurgery 82 (4), 431–440. doi:10.1093/neuros/nyx238
Kleinstreuer C., Li Z., Farber M. J. A. R. B. E. (2007) Fluid-structure interaction analyses of stented abdominal aortic aneurysms, Annu. Rev. Biomed. Eng., 169–204. doi:10.1146/annurev.bioeng.9.060906.151853
Korja M., Lehto H., Juvela S. J. S. (2014). Lifelong rupture risk of intracranial aneurysms depends on risk factors: a prospective Finnish cohort study. Stroke Vasc. Interv. Neurol. 45 (7), 1958–1963. doi:10.1161/STROKEAHA.114.005318
Korkmaz K. S., Butuner B. D., Roggenbuck D. J. J. o.L., Medicine P. (2018). Detection of 8-OHdG as a diagnostic biomarker. J. Lab. Precis. Med. 3, 95. doi:10.21037/jlpm.2018.11.01
Kosierkiewicz T. A., Factor S. M., Dickson D. W. J. J. o.N., Neurology E. (1994). Immunocytochemical studies of atherosclerotic lesions of cerebral berry aneurysms. J. Neuropathol. Exp. Neurol. 53 (4), 399–406. doi:10.1097/00005072-199407000-00012
Kurki M. I., Häkkinen S.-K., Frösen J., Tulamo R., von Und Zu Fraunberg M., Wong G., et al. Upregulated signaling pathways in ruptured human saccular intracranial aneurysm wall: an emerging regulative role of Toll-like receptor signaling and nuclear factor-κB, hypoxia-inducible factor-1A, and ETS transcription factors. 68(6) (2011) 1667–1675. doi:10.1227/NEU.0b013e318210f001
Lall R. R., Eddleman C. S., Bendok B. R., Batjer H. H. J. N. f. (2009). Unruptured intracranial aneurysms and the assessment of rupture risk based on anatomical and morphological factors: sifting through the sands of data. Neurosurg. Focus 26 (5), E2. doi:10.3171/2009.2.FOCUS0921
Lamping K. G., Nuno D. W., Shesely E. G., Maeda N., Faraci F. M. J. A. J. o.P.-H., Physiology C. (2000). Vasodilator mechanisms in the coronary circulation of endothelial nitric oxide synthase-deficient mice. Am. J. Physiol. Heart Circ. Physiol. 279 (4), H1906–H1912. doi:10.1152/ajpheart.2000.279.4.H1906
Larson D. B., Chen M. C., Lungren M. P., Halabi S. S., Stence N. V., Langlotz C. P. J. R., Performance of a deep-learning neural network model in assessing skeletal maturity on pediatric hand radiographs 287(1) (2018) 313–322. doi:10.1148/radiol.2017170236
Lattanzi S., Di Napoli M., Ricci S., Divani A. A. J. N. (2020). Matrix metalloproteinases in acute intracerebral hemorrhage. Neurotherapeutics 17 (2), 484–496. doi:10.1007/s13311-020-00839-0
Laurent D., Small C., Lucke-Wold B., Dodd W. S., Chalouhi N., Hu Y. C., et al. (2022). Neurosurgery, Understanding the genetics of intracranial aneurysms. Clinical Neurology and Neurosurgery 212.107060
Lee C.-H., Ahn C., Ryu H., Kang H.-S., Jeong S.-K., Jung K.-H. J. C. D. (2021). Clinical factors associated with the risk of intracranial aneurysm rupture in autosomal dominant polycystic kidney disease. Cerebrovasc. Dis. 50 (3), 339–346. doi:10.1159/000513709
Leemans E., Cornelissen B., Slump C., Majoie C., Cebral J., Marquering H. J. A. J. o.N. (2019). Comparing morphology and hemodynamics of stable-versus-growing and grown intracranial aneurysms. AJNR. Am. J. Neuroradiol. 40 (12), 2102–2110. doi:10.3174/ajnr.A6307
Li H., Li H., Yue H., Wang W., Yu L., Cao Y., et al. (2017). Comparison between smaller ruptured intracranial aneurysm and larger un-ruptured intracranial aneurysm: gene expression profile analysis. Neurosurg. Rev. 40, 419–425. doi:10.1007/s10143-016-0799-3
Li X., Zeng L., Lu X., Chen K., Yu M., Wang B., et al. (2023). A review of artificial intelligence in the rupture risk assessment of intracranial aneurysms: applications and challenges. Brain Sci. 13 (7), 1056. doi:10.3390/brainsci13071056
Lin S., Zou Y., Hu J., Xiang L., Guo L., Lin X., et al. (2022). Development and assessment of machine learning models for predicting recurrence risk after endovascular treatment in patients with intracranial aneurysms. Neurosurg. Rev. 45, 1521–1531. doi:10.1007/s10143-021-01665-4
Lindner S. H., Bor A. S. E., Rinkel G. J. E. (2010). Differences in risk factors according to the site of intracranial aneurysms. J. Neurol. Neurosurg. Psychiatry 81 (1), 116–118. doi:10.1136/jnnp.2008.163063
Liu J., Chen Y., Lan L., Lin B., Chen W., Wang M., et al. (2018). Prediction of rupture risk in anterior communicating artery aneurysms with a feed-forward artificial neural network. Eur. Radiol. 28, 3268–3275. doi:10.1007/s00330-017-5300-3
Liu Q., Jiang P., Jiang Y., Ge H., Li S., Jin H., et al. (2019). Prediction of aneurysm stability using a machine learning model based on PyRadiomics-derived morphological features. Stroke Vasc. Interv. Neurol. 50 (9), 2314–2321. doi:10.1161/STROKEAHA.119.025777
Lu D., Kassab G. S. J. J. o.t.r.s.i. (2011). Role of shear stress and stretch in vascular mechanobiology. J. R. Soc. Interface 8 (63), 1379–1385. doi:10.1098/rsif.2011.0177
Ma X., Lan F., Zhang Y. J. A. N. B. (2021). Associations between C-reactive protein and white blood cell count, occurrence of delayed cerebral ischemia and poor outcome following aneurysmal subarachnoid hemorrhage: a systematic review and meta-analysis. Acta Neurol. belg. 121, 1311–1324. doi:10.1007/s13760-020-01496-y
Ma X., Yang Y., Zhou Y., Jia W. J. N. r. (2019). Endovascular treatment of ruptured intracranial aneurysms in elderly patients: clinical features and treatment outcome. Neurosurg. Rev. 42, 745–751. doi:10.1007/s10143-018-1031-4
Macdonald R. L., Schweizer T. A. J. T. L., Spontaneous subarachnoid haemorrhage. 389(10069) (2017) 655–666. doi:10.1016/S0140-6736(16)30668-7
Malhotra A., Wu X., Forman H. P., Grossetta Nardini H. K., Matouk C. C., Gandhi D., et al. (2017). Growth and rupture risk of small unruptured intracranial aneurysms: a systematic review. 167 (1), 26–33. doi:10.7326/M17-0246
Marasini A., Shrestha A., Phuyal S., Zaidat O. O., Kalia J. S. J. F. i.N. (2022). Role of artificial intelligence in unruptured intracranial aneurysm: an overview. Front. Neurol. 13, 784326. doi:10.3389/fneur.2022.784326
Mehta V., Russin J., Spirtos A., He S., Adamczyk P., Amar A. P., et al. (2013). Matrix metalloproteinases in cerebral vasospasm following aneurysmal subarachnoid hemorrhage. Neurol. Res. Int. 2013, 1–4. doi:10.1155/2013/943761
Meng H., Tutino V., Xiang J., Siddiqui A. J. A. J. o.N. (2014). High WSS or low WSS? Complex interactions of hemodynamics with intracranial aneurysm initiation, growth, and rupture: toward a unifying hypothesis. Am. J. Neuroradiol. 35 (7), 1254–1262. doi:10.3174/ajnr.A3558
Merritt W. C., Berns H. F., Ducruet A. F., Becker T. A. J. S. N. I. (2021). Definitions of intracranial aneurysm size and morphology: a call for standardization, 12.
Miao Y., Liao J. K. J. E. r.o.n. (2014). Potential serum biomarkers in the pathophysiological processes of stroke. Expert Rev. Neurother. 14 (2), 173–185. doi:10.1586/14737175.2014.875471
Monsour M., Croci D. M., Grüter B. E., Taussky P., Marbacher S., Agazzi S. J. T. S. R. (2023). Cerebral aneurysm and interleukin-6: a key player in aneurysm generation and rupture or just one of the multiple factors? Transl. Stroke Res. 14 (5), 631–639. doi:10.1007/s12975-022-01079-4
Mourato A., Valente R., Xavier J., Brito M., Avril S., de Sa J. C., et al. (2022). Computational modelling and simulation of fluid structure interaction in aortic aneurysms: a systematic review and discussion of the clinical potential. Appl. Sci. (Basel). 12 (16), 8049. doi:10.3390/app12168049
Mouridsen K., Thurner P., Zaharchuk G. J. S. (2020). Artificial intelligence applications in stroke. Stroke Vasc. Interv. Neurol. 51 (8), 2573–2579. doi:10.1161/STROKEAHA.119.027479
Munarriz P. M., Gómez P. A., Paredes I., Castaño-Leon A. M., Cepeda S., Lagares A. J. W. n. (2016). Basic principles of hemodynamics and cerebral aneurysms. World Neurosurg. 88, 311–319. doi:10.1016/j.wneu.2016.01.031
Nabaei M. (2022). Cerebral aneurysm evolution modeling from microstructural computational models to machine learning. 98, 107676. doi:10.1016/j.compbiolchem.2022.107676
Nazri N. N. M., Matalif M. U., Adib M. A. H. M. (2020). “Effects of blood flow patent and cross-sectional area on hemodynamic into patient-specific cerebral aneurysm via fluid-structure interaction method: a review,” in IOP conference series: materials science and engineering. 012022.
Nowicki K. W., Mittal A. M., Abou-Al-Shaar H., Rochlin E. K., Lang M. J., Gross B. A., et al. (2023). A future blood test to detect cerebral aneurysms. Cell. Mol. Neurobiol. 43 (6), 2697–2711. doi:10.1007/s10571-023-01346-4
Ollikainen E., Tulamo R., Lehti S., Lee-Rueckert M., Hernesniemi J., Niemelä M., et al. (2016). Smooth muscle cell foam cell formation, apolipoproteins, and ABCA1 in intracranial aneurysms: implications for lipid accumulation as a promoter of aneurysm wall rupture. J. Neuropathol. Exp. Neurol. 75 (7), 689–699. doi:10.1093/jnen/nlw041
Park Y. K., Yi H.-J., Choi K.-S., Lee Y.-J., Chun H.-J. J. W. N. (2018) Intraprocedural rupture during endovascular treatment of intracranial aneurysm: clinical results and literature review, 114, e605–e615.
Penn D. L., Komotar R. J., Connolly E. S. J. J. o.C. N. (2011). Hemodynamic mechanisms underlying cerebral aneurysm pathogenesis. 18(11) 1435–1438.
Penn D. L., Witte S. R., Komotar R. J., Connolly E. S. J. J. o.c.n. (2014). The role of vascular remodeling and inflammation in the pathogenesis of intracranial aneurysms. J. Clin. Neurosci. 21 (1), 28–32. doi:10.1016/j.jocn.2013.07.004
Pereira V. M., Delattre B., Brina O., Bouillot P., Vargas M. I. J. T. i.M. R. I. (2016). 4D flow MRI in neuroradiology: techniques and applications. Top. Magn. Reson. Imaging 25 (2), 81–87. doi:10.1097/rmr.0000000000000082
Petridis A. K., Kamp M. A., Cornelius J. F., Beez T., Beseoglu K., Turowski B., et al. (2017). Aneurysmal subarachnoid hemorrhage: diagnosis and treatment. Dtsch. Arztebl. Int. 114 (13), 226–236. doi:10.3238/arztebl.2017.0226
Przybycien-Szymanska M. M., Ashley W. W., Jr (2015). Biomarker discovery in cerebral vasospasm after aneurysmal subarachnoid hemorrhage, J Stroke Cerebrovasc Dis. 24 (7), 1453–1464.
Recht M. P., Dewey M., Dreyer K., Langlotz C., Niessen W., Prainsack B., et al. (2020). Integrating artificial intelligence into the clinical practice of radiology: challenges and recommendations. Eur. Radiol. 30, 3576–3584. doi:10.1007/s00330-020-06672-5
Reneman R. S., Arts T., Hoeks A. P. J. J. o.v.r. (2006). Wall shear stress–an important determinant of endothelial cell function and structure–in the arterial system in vivo: discrepancies with theory. J. Vasc. Res. 43 (3), 251–269. doi:10.1159/000091648
Rinkel G. J., Djibuti M., Algra A., Van Gijn J. J. S. (1998). Prevalence and risk of rupture of intracranial aneurysms: a systematic review. Stroke 29 (1), 251–256. doi:10.1161/01.str.29.1.251
Rinkel G. J. R. n. (2008). Natural history, epidemiology and screening of unruptured intracranial aneurysms. Rev. Neurol. 164 (10), 781–786. doi:10.1016/j.neurol.2008.07.012
Rodríguez-Rodríguez A., Egea-Guerrero J. J., de Azúa-López Z. R., Murillo-Cabezas F. J. J. (2014). Biomarkers of vasospasm development and outcome in aneurysmal subarachnoid hemorrhage, 341 (1-2) 119–127.
Sadasivan C., Fiorella D. J., Woo H. H.B.B.J.A.o.b.e. Lieber (2013) Physical factors effecting cerebral aneurysm pathophysiology, Ann. Biomed. Eng., 1347–1365. doi:10.1007/s10439-013-0800-z
Salman S., Gu Q., Sharma R., Wei Y., Dherin B., Reddy S., et al. (2023). Artificial intelligence and machine learning in aneurysmal subarachnoid hemorrhage: future promises, perils, and practicalities. J. Neurol. Sci. 454, 120832. doi:10.1016/j.jns.2023.120832
Samuel N., Radovanovic I. J. N. f. (2019). Genetic basis of intracranial aneurysm formation and rupture: clinical implications in the postgenomic era. Neurosurg. Focus 47 (1), E10. doi:10.3171/2019.4.FOCUS19204
Sanchez S., Hickerson M., Patel R. R., Ghazaleh D., Tarchand R., Paranjape G. S., et al. (2023). Morphological characteristics of ruptured brain aneurysms: a systematic literature review and meta-analysis. 3 (2), e000707. doi:10.1161/svin.122.000707
Saratzis A., Bown M. J. J. H., The genetic basis for aortic aneurysmal disease, 100(12) (2014) 916–922. doi:10.1136/heartjnl-2013-305130
Sawabe M. J. G., international g. (2010). Vascular aging: from molecular mechanism to clinical significance. Geriatr. Gerontol. Int. 10, S213–S220. doi:10.1111/j.1447-0594.2010.00603.x
Shi Z., Hu B., Schoepf U., Savage R., Dargis D., Pan C., et al. (2020b). Artificial intelligence in the management of intracranial aneurysms: current status and future perspectives. AJNR. Am. J. Neuroradiol. 41 (3), 373–379. doi:10.3174/ajnr.A6468
Shi Z., Miao C., Schoepf U. J., Savage R. H., Dargis D. M., Pan C., et al. (2020a). A clinically applicable deep-learning model for detecting intracranial aneurysm in computed tomography angiography images. Nat. Commun. 11 (1), 6090. doi:10.1038/s41467-020-19527-w
Siasos G., Mourouzis K., Oikonomou E., Tsalamandris S., Tsigkou V., Vlasis K., et al. (2015). The role of endothelial dysfunction in aortic aneurysms. Curr. Pharm. Des. 21 (28), 4016–4034. doi:10.2174/1381612821666150826094156
Signorelli F., Gory B., Riva R., Labeyrie P., Pelissou-Guyotat I., Turjman F. J. N. N. (2015). Hemodynamics, inflammation, vascular remodeling, and the development and rupture of intracranial aneurysms: a review. Neuroimmunology and Neuroinflammation 2 (2), 59–67. doi:10.4103/2347-8659.154885
Signorelli F., Sela S., Gesualdo L., Chevrel S., Tollet F., Pailler-Mattei C., et al. (2018). Hemodynamic stress, inflammation, and intracranial aneurysm development and rupture: a systematic review. World Neurosurgery 115, 234–244. doi:10.1016/j.wneu.2018.04.143
Simon M., Grote A. J. I. J. o.M. S. (2021). Interleukin 6 and aneurysmal subarachnoid hemorrhage. A narrative review. Int. J. Mol. Sci. 22 (8), 4133. doi:10.3390/ijms22084133
Staarmann B., Smith M., Prestigiacomo C. J. J. N. f. (2019). Shear stress and aneurysms: a review. Neurosurg. Focus 47 (1), E2. doi:10.3171/2019.4.FOCUS19225
Stehbens W. J. A. o.n. (1963). Histopathology of cerebral aneurysms. Arch. Neurol. 8 (3), 272–285. doi:10.1001/archneur.1963.00460030056005
Steiner T., Juvela S., Unterberg A., Jung C., Forsting M., Rinkel G. J. C. d., et al. (2013). European Stroke Organization guidelines for the management of intracranial aneurysms and subarachnoid haemorrhage. Cerebrovasc. Dis. 35 (2), 93–112. doi:10.1159/000346087
Szajer J., Ho-Shon K. J. M. r.i. (2018). A comparison of 4D flow MRI-derived wall shear stress with computational fluid dynamics methods for intracranial aneurysms and carotid bifurcations—a review. Magn. Reson. Imaging 48, 62–69. doi:10.1016/j.mri.2017.12.005
Tang X., Zhou L., Wen L., Wu Q., Leng X., Xiang J., et al. (2022). Morphological and hemodynamic characteristics associated with the rupture of multiple intracranial aneurysms, 12.811281
Tawk R. G., Hasan T. F., D’Souza C. E., Peel J. B., Freeman W. D. (2021) “Diagnosis and treatment of unruptured intracranial aneurysms and aneurysmal subarachnoid hemorrhage,” in Mayo clinic proceedings. Elsevier, 1970–2000.
Texakalidis P., Sweid A., Mouchtouris N., Peterson E. C., Sioka C., Rangel-Castilla L., et al. (2019). Aneurysm Formation, growth, and rupture: the biology and physics of cerebral aneurysms, World Neurosurg., 277–284. doi:10.1016/j.wneu.2019.07.093
Thompson B. G., Brown R. D., Amin-Hanjani S., Broderick J. P., Cockroft K. M., Connolly E. S., et al. (2015). Guidelines for the management of patients with unruptured intracranial aneurysms: a guideline for healthcare professionals from the American heart association/American stroke association. Heart Association/American Stroke Assoc. 46 (8), 2368–2400. doi:10.1161/STR.0000000000000070
Tsilimigras D. I., Sigala F., Karaolanis G., Ntanasis-Stathopoulos I., Spartalis E., Spartalis M., et al. (2018). Cytokines as biomarkers of inflammatory response after open versus endovascular repair of abdominal aortic aneurysms: a systematic review. Acta Pharmacologica Sinica 39 (7), 1164–1175. doi:10.1038/aps.2017.212
Turan N., Heider R.A.-J., Zaharieva D., Ahmad F. U., Barrow D. L., Pradilla G. J. T. s.r. (2016). Sex differences in the formation of intracranial aneurysms and incidence and outcome of subarachnoid hemorrhage: review of experimental and human studies, Transl. Stroke Res., 12–19. doi:10.1007/s12975-015-0434-6
Turjman A. S., Turjman F., Edelman E. R. J. C., Role of fluid dynamics and inflammation in intracranial aneurysm formation. 129(3) (2014) 373–382. doi:10.1161/CIRCULATIONAHA.113.001444
Turski P., Scarano A., Hartman E., Clark Z., Schubert T., Rivera L., et al. (2016). Neurovascular 4DFlow MRI (Phase Contrast MRA): emerging clinical applications. Neurovasc. Imaging 2, 8–11. doi:10.1186/s40809-016-0019-0
Urbonavicius S., Urbonaviciene G., Honoré B., Henneberg E., Vorum H., Lindholt J. S. J. E. J. o.V., et al. (2008). Potential circulating biomarkers for abdominal aortic aneurysm expansion and rupture-a systematic review. Eur. J. Vasc. Endovasc. Surg. 36 (3), 273–280. doi:10.1016/j.ejvs.2008.05.009
van Amerongen M. J., Boogaarts H. D., de Vries J., Verbeek A. L., Meijer F. J., Prokop M., et al. (2014). MRA versus DSA for follow-up of coiled intracranial aneurysms: a meta-analysis. Am. J. Neuroradiol. 35 (9), 1655–1661. doi:10.3174/ajnr.A3700
Vasconcellos V., Lacchini R., Jacob-Ferreira A. L., Sales M. L., Ferreira-Sae M. C., Schreiber R., et al. (2010). Endothelial nitric oxide synthase haplotypes associated with hypertension do not predispose to cardiac hypertrophy. DNA Cell Biol. 29 (4), 171–176. doi:10.1089/dna.2009.0955
Villablanca J. P., Duckwiler G. R., Jahan R., Tateshima S., Martin N. A., Frazee J., et al. Natural history of asymptomatic unruptured cerebral aneurysms evaluated at CT angiography: growth and rupture incidence and correlation with epidemiologic risk factors, 269(1) (2013) 258–265. doi:10.1148/radiol.13121188
Wang S., Summers R. M. J. M. i.a. (2012). Machine learning and radiology. Mach. Learn. radiology 16 (5), 933–951. doi:10.1016/j.media.2012.02.005
Wang X., Zhu C., Leng Y., Degnan A. J., Lu J. J. A. r. (2019). Intracranial aneurysm wall enhancement associated with aneurysm rupture: a systematic review and meta-analysis. Acad. Radiol. 26 (5), 664–673. doi:10.1016/j.acra.2018.05.005
Wang Z., Ma J., Yue H., Zhang Z., Fang F., Wang G., et al. (2023). Vascular smooth muscle cells in intracranial aneurysms. Microvasc. Res. 149, 104554. doi:10.1016/j.mvr.2023.104554
Waqas M., Chin F., Rajabzadeh-Oghaz H., Gong A. D., Rai H. H., Mokin M., et al. (2020). Size of ruptured intracranial aneurysms: a systematic review and meta-analysis, Acta Neurochir., 1353–1362. doi:10.1007/s00701-020-04291-z
Wardlaw J., White P. J. B. (2000). The detection and management of unruptured intracranial aneurysms. Brain 123 (2), 205–221. doi:10.1093/brain/123.2.205
Watson E., Ding D., Khattar N. K., Everhart D. E., James R. F. J. J. o.t.n.s. (2018). Neurocognitive outcomes after aneurysmal subarachnoid hemorrhage: identifying inflammatory biomarkers. J. Neurol. Sci. 394, 84–93. doi:10.1016/j.jns.2018.06.021
Wermer M. J., van der Schaaf I. C., Algra A., Rinkel G. J. J. S. (2007). Risk of rupture of unruptured intracranial aneurysms in relation to patient and aneurysm characteristics: an updated meta-analysis. Stroke Vasc. Interv. Neurol. 38 (4), 1404–1410. doi:10.1161/01.STR.0000260955.51401.cd
Wiebers D. O., Torner J. C., Meissner I. J. S. (1992). Impact of unruptured intracranial aneurysms on public health in the United States. Stroke Vasc. Interv. Neurol. 23 (10), 1416–1419. doi:10.1161/01.str.23.10.1416
Wu Y., Zhao Z., Kang S., Zhang L., Lv F.J. F. i.N. (2023). Potential application of peripheral blood biomarkers in intracranial aneurysms. Front. Neurol. 14, 1273341. doi:10.3389/fneur.2023.1273341
Xiang J., Tutino V., Snyder K., Meng H. J. A. J. o.N. (2014). CFD: computational fluid dynamics or confounding factor dissemination? the role of hemodynamics in intracranial aneurysm rupture risk assessment. Am. J. Neuroradiol. 35 (10), 1849–1857. doi:10.3174/ajnr.A3710
Xiong W., Chen T., Li J., Xiang L., Zhang C., Xiang L., et al. (2022). Interpretable machine learning model to predict rupture of small intracranial aneurysms and facilitate clinical decision. Neurol. Sci. 43 (11), 6371–6379. doi:10.1007/s10072-022-06351-x
Yamaguchi R., Tanaka G., Liu H. J. I. J. N. N. (2016) Effect of elasticity on flow characteristics inside intracranial aneurysms, 3, 049.
Yang Y., Liu Q., Jiang P., Yang J., Li M., Chen S., et al. (2021). Multidimensional predicting model of intracranial aneurysm stability with backpropagation neural network: a preliminary study, 1–13.
Yi J., Zielinski D., Chen M. J. J. o.S., Diseases C. (2016a). Cerebral aneurysm size before and after rupture: case series and literature review. J. Stroke Cerebrovasc. Dis. 25 (5), 1244–1248. doi:10.1016/j.jstrokecerebrovasdis.2016.01.031
Yi J., Zielinski D., Chen M. J. J. o.S., Diseases C. (2016b). Cerebral aneurysm size before and after rupture: case series and literature review. J. Stroke Cerebrovasc. Dis. 25 (5), 1244–1248. doi:10.1016/j.jstrokecerebrovasdis.2016.01.031
Yin X., Huang X., Feng Y., Tan W., Liu H., Huo Y. J. P. O. (2016). Interplay of proximal flow confluence and distal flow divergence in patient-specific vertebrobasilar system. PLoS One 11 (7), e0159836. doi:10.1371/journal.pone.0159836
Yin X., Huang X., Li Q., Li L., Niu P., Cao M., et al. (2018) Hepatic hemangiomas alter morphometry and impair hemodynamics of the abdominal aorta and primary branches from computer simulations, Front. Physiol., 334, doi:10.3389/fphys.2018.00334
Zhang X., Ares W. J., Taussky P., Ducruet A. F., Grandhi R. J. N. f. (2019). Role of matrix metalloproteinases in the pathogenesis of intracranial aneurysms. Neurosurg. Focus 47 (1), E4. doi:10.3171/2019.4.FOCUS19214
Zhang Y., Jing L., Zhang Y., Liu J., Yang X. J. B. n. (2016). Low wall shear stress is associated with the rupture of intracranial aneurysm with known rupture point: case report and literature review, 16, 1–4.
Zhou G., Zhu Y., Yin Y., Su M., Li M. J. S. r. (2017). Association of wall shear stress with intracranial aneurysm rupture: systematic review and meta-analysis. Sci. Rep. 7 (1), 5331. doi:10.1038/s41598-017-05886-w
Keywords: cerebral aneurysm, morphology, hemodynamics, imaging modalities, blood biomarkers, artificial intelligence, fluid-structure interaction
Citation: Wang X and Huang X (2024) Risk factors and predictive indicators of rupture in cerebral aneurysms. Front. Physiol. 15:1454016. doi: 10.3389/fphys.2024.1454016
Received: 24 June 2024; Accepted: 23 August 2024;
Published: 05 September 2024.
Edited by:
Ran Xiao, Emory University, United StatesReviewed by:
Chao Ma, Beijing Neurosurgical Institute, Beijing Tiantan Hospital, Capital Medical University, ChinaYonghui Qiao, Northwestern Polytechnical University, China
Copyright © 2024 Wang and Huang. This is an open-access article distributed under the terms of the Creative Commons Attribution License (CC BY). The use, distribution or reproduction in other forums is permitted, provided the original author(s) and the copyright owner(s) are credited and that the original publication in this journal is cited, in accordance with accepted academic practice. No use, distribution or reproduction is permitted which does not comply with these terms.
*Correspondence: Xu Huang, aHVhbmcueHVAYW9odWEuY29t